Rapid direct injection LC-MS/MS method for analysis of prioritized indicator compounds in wastewater effluent†
Received
31st March 2015
, Accepted 5th May 2015
First published on 7th May 2015
Abstract
Trace organic compounds (TOrCs) have been detected in drinking water sources for several years, raising concerns due to their potential risks to public health. The main contributor of TOrCs to drinking water is through wastewater discharges. However, there are several hundred TOrCs currently known with numerous new organic chemicals being released daily, making it unfeasible to monitor each one in water. This study used a detailed literature review and scoring system to establish a list of twenty priority indicator TOrCs in US wastewaters. Next, a rapid direct injection LC-MS/MS method for analysis of these compounds was developed without the need for an extraction step and only 80 μL sample volume while providing method reporting limits of 3–39 ng L−1 for all but one TOrC (sucralose: 302 ng L−1). The elimination of an extraction step reduced matrix effects considerably making the method suitable for wastewater analysis. Method validation including matrix spike recoveries, linearity of calibration curve and inter- and intra-day variability was successfully performed. Finally, the twenty indicator TOrCs were evaluated in four different wastewater treatment plant (WWTP) effluents through four sample campaigns spread across a year. The occurrence data indicated that all indicator TOrCs were detected in at least three out of the four WWTP effluents. Sucralose, iohexol, TCPP, acesulfame and gemfibrozil were detected in all samples at the four WWTPs indicating they could be used as indicators of wastewater influence in receiving waters. DEET, caffeine, triclosan, iopromide and others are effective indicators at showing seasonal variations, treatment process efficacy, and consumption patterns. Overall, the impact of this study will help develop more effective monitoring programs for TOrCs in water reuse schemes.
Water impact
Trace organic compounds have been detected in our drinking water sources and pose potential health concerns due to unknown toxicity. There are several hundred TOrCs currently, making monitoring of each one unfeasible. Further, analysis of these compounds is challenging with laborious sample extraction and several human intervention steps that can add contamination and reduce accuracy and reproducibility. This paper provides a prioritized list of ‘indicator’ TOrCs in wastewater through a detailed literature review and scoring system. Further, a rapid analytical method involving minimal sample preparation is developed for all the indicator TOrCs using one injection and <100 μL sample. This paper provides guidance on selection and analysis of indicator TOrCs for effective and rapid monitoring during water reuse schemes.
|
1. Introduction
The presence of trace organic compounds (TOrCs) like pharmaceuticals, personal-care products, pesticides, household chemicals and industrial compounds in wastewater effluents is ubiquitous around the world.1–3 Treated wastewater discharge into drinking water sources in the US is a common phenomenon and has been documented for several decades.4 A major pathway for TOrCs entering our drinking water sources is through wastewater, since many of these compounds are poorly attenuated in conventional water treatment processes.5–7 Indeed, several studies have demonstrated the presence of TOrCs in source waters and finished drinking water in the US.8,9 Dwindling freshwater sources and the adoption of water reuse schemes will only exacerbate the issue of increasing TOrCs being detected in our drinking water. Some of these TOrCs are known to cause adverse effects in wildlife and humans at environmentally relevant concentrations while potential mixture effects of exposure to a large variety of these compounds is still not clear.10–12 Thus monitoring of these compounds in water sources is required while further toxicological studies are performed.
Almost one billion compounds are registered in the chemical abstracts services (CAS) inventory with almost 15
000 new compounds introduced every day.13 Over 3000 pharmaceuticals are registered for use in the US along with several thousand industrial compounds and hence, it is not practically and economically feasible to monitor each one in the environment. Thus, the development of a list of ‘indicator’ compounds that mimic the occurrence and behavior of a wider class of compounds is beneficial. A good indicator compound must possess certain desirable properties such as being easy to analyze, present at concentrations high enough to be easily detected, no or very low background in the natural environment and persistence in the environment for at least as long as the compounds it is used to represent. The use of microbial indicators to detect the presence of fecal coliform and other water-borne pathogens is well established and has been around for some time. However, only recently has work on chemical indicators in wastewater been suggested to counter the difficulty in analyzing the several thousands of new contaminants introduced daily and predicting wastewater influence. Boron isotopes and gadolinium have been used in the past as conservative inorganic tracers for wastewater influence but have their limitations.14 On the other hand, as most TOrCs are fairly recalcitrant they can act as conservative organic markers for influence of wastewater in receiving water. Several researchers have suggested viable TOrC indicator lists for monitoring in wastewater. These lists can be based on available occurrence data, detection frequency, risk assessment, impact of environmental conditions and treatment efficacy.14–18
In the past, analysis of these indicator compounds at trace levels has required an extraction and concentration step that is laborious, and detrimental to reproducibility of results due to several human-manipulation steps involved.19 Further, this is time-consuming and results in longer analysis times which can cause changes in analyte concentrations while delaying potential remedial action to the water source if required. With the advancement in sensitivity of analytical techniques though, it may be possible to eliminate this extraction step and perform rapid analysis of these indicator compounds by large volume injection of the aqueous sample.20 This method has several advantages such as being less time-consuming due to little sample preparation and thus increases throughput and productivity. For example, Backe and colleagues estimated that sample preparation time for direct injection analysis was less than 30 minutes while SPE extracts take several hours to prepare.20 It also eliminates several human intervention steps that are associated with sample extraction which reduces the possibility of contamination while increasing reproducibility of analysis. Further, much lower sample volume collections are required because of the ability to inject larger amount of water on column compared to organic solvent into the instrument. This not only gives savings in sample collection and transport but also significantly reduces the amount of isotopically labeled surrogate standard required per sample.
The aims of this study were to (i) identify a feasible indicator compound list through a literature review of occurrence and detection frequency in wastewater effluents around the US (ii) develop a fast, sensitive and robust analytical method involving no extraction and minimal sample manipulation to quantify the viable indicator compounds in wastewater effluents (iii) perform a monitoring study of US wastewater effluents undergoing conventional and advanced treatments to determine suitability of these TOrCs to pose as indicators.
2. Experimental
2.1. Materials
All analytical standards used in this study were >97% purity and purchased from Sigma Aldrich (St. Louis, MO), Alfa Aesar (Ward Hill, MA), or US Pharmacopeia (Washington, DC). The isotopically labeled surrogate standards were procured from Cambridge Isotope Laboratories (Andover, MA) except meprobamate-d3, triclocarban-13C6 and 13C6-diclofenac from Toronto Research Chemicals (Ontario, Canada); iopromidol-d3 from Santa Cruz Biotechnology (Dallas, TX); gemfibrozil-d6, and diphenhydramine-d5 from C/D/N Isotopes (Quebec, Canada). Acetonitrile (ACN; HPLC grade), methanol (HPLC grade), acetic acid (ACS grade), formic acid (LCMS grade), and ammonium acetate (ACS grade) were purchased from Fisher Scientific (Pittsburgh, PA), while HPLC grade water was purchased from Burdick & Jackson (Muskegon, MI).
2.2. Sample collection and treatment
Individual grab samples were collected from four wastewater treatment plant effluents studied at four different times over the course of one year. Since, diurnal variations of these compounds can be expected at a wastewater treatment plant, care was taken to collect samples between 8 and 10 AM for all sample events. Sodium azide and ascorbic acid were added to each bottle to act as a microbial inhibitor and quenching agent respectively. Samples were placed in an ice-filled cooler and shipped to the laboratory overnight where they were stored at 4 °C prior to analysis.
One mL of all samples was spiked with 2 μg L−1 of an isotopically labeled surrogate standard (ILSS) mixture within 72 hours of sample receipt. The samples were then filtered with a 0.2 μm polyethylene sulfonate (PES) syringe filter from Agilent Technologies (Palo Alto, CA) and placed in an autosampler vial for analysis.
2.3. Wastewater treatment plant selection
Four wastewater treatment plants were selected due to their diverse treatment trains and treatment capacities. All four plants have fairly modern treatment trains with WWTP 1 and 2 undergoing significant modifications in 2013. WWTP 1 and 2 had daily treatment capacities of 25 million gallons a day (MGD) and 32 MGD respectively with a very similar influent source that was primarily domestic in nature. WWTP 1 treatment consisted of primary clarifiers followed by a 5-stage bardenpho clarifier and activated sludge with enhanced biological nutrient removal scheme. Disinfection was done by chlorination and water was dechlorinated with sodium bisulfite before discharge into a receiving body. WWTP 2 had grit filtration, flocculation and sedimentation, modified bardenpho clarification followed by dissolved air floatation and disk filtration. Disinfection was similar to WWTP 1 with chlorination and dechlorination before discharge. WWTP 3 was a 10 MGD plant with primary clarification followed by powdered activated carbon in the activated sludge basin and sand filtration. The resulting effluent was ozonated (dissolved ozone conc.: 1–2 mg L−1) followed by passing through a biologically activated carbon column with a contact time of 15–25 min. Final disinfection at this plant consisted of chlorination. WWTP 4 was the largest plant in terms of treatment capacity servicing 54 MGD. The plant employed chemical coagulation, flocculation and sedimentation followed by a multi-media filter and granular activated carbon with a 10–15 min contact time with final chlorination before discharge into a surface water source. Treatment details and effluent water parameters for all four WWTPs are provided in Table 1.
Table 1 Plant treatment schemes and bulk water quality parametersa
Plant |
Capacity(MGD) |
Treatment processes |
Effluent parameters |
TOC (mg L−1) |
pH |
Turbidity (NTU) |
Alkalinity (mg L−1 as CaCO3) |
NO3− (mg L−1) |
UV254A (cm−1) |
1 °C: primary clarifier; 2 °C: secondary clarifier, AS: activated sludge; B–C: Bardenpho-clarifier; BNRAS: biological nutrient removal activated sludge; CC: chemical coagulation; Cl2: chlorination; DAF: dissolved air floatation; DeCl: dechlorination; DF: disk filtration; F/S: flocculation/sedimentation; GAC: granular activated carbon; GF: grit filter; LA: lime addition; MMF: multi-media filter; O3: ozonation; PAC: powdered activated carbon; RC: recarbonation; SF: sand filter.
|
WWTP 1 |
25 |
1 °C, 5 stage B–C, 5 stage BNRAS, Cl2, DeCl |
6.3 |
7.2 |
0.39 |
154 |
13 |
0.131 |
WWTP 2 |
32 |
GF, F/S, DAF, B-C, 2 °C, DF, Cl2, DeCl |
8.0 |
7.1 |
0.81 |
156 |
9 |
0.147 |
WWTP 3 |
10 |
1 °C, PAC+AS, LA, RC, SF, O3, GAC, Cl2 |
2.7 |
7.1 |
0.09 |
97 |
<2 |
0.038 |
WWTP 4 |
54 |
CC, F/S, MMF, GAC, Cl2 |
4.1 |
7.4 |
0.17 |
100 |
— |
0.065 |
2.4. Liquid chromatography
An Agilent 1260 HPLC pump equipped with a 100 μL syringe loop was used for all analyses. Chromatographic separation was performed using an Agilent Pursuit XRs C-8 column (100 mm × 2.0 mm) with a 3 μm particle size. HPLC water with 0.1% (v/v) formic acid (A) and ACN (B) was used as mobile phases. The initial gradient was held at 2% B for 1.5 min, followed by a linear increases to 60% B at 8 min and 100% B at 10.5 min. This was held till 11 min followed by a step back to the initial gradient of 2% B at 11.5 min. A post-run column equilibration time of 1.5 min was provided which resulted in a total sample run time of 13 min. The mobile phase was operated at 0.4 mL min−1 and column temperature was maintained at 30 °C throughout the analytical run. An injection volume of 80 μL was used after optimization.
2.5. Mass spectrometry
Mass spectrometry was performed using an Agilent 6490 triple quadrupole tandem mass spectrometer. All compound specific parameters were optimized according to our previous work21 while the mass spectrometer parameters were optimized using the Agilent Source Optimizer feature. The compound-specific parameters for the final target analyte list and the mass spectrometer optimized parameters are presented in the ESI† (Tables S1 and S2). Dynamic multiple reaction monitoring mode with a retention time lock was employed for analysis of all target analytes. Simultaneous electrospray ionization (ESI) in positive and negative mode was performed with a fast switching dielectric capillary which allowed for injection of the sample only once. Two transitions were monitored where possible and retention time locking along with ion ratio monitoring allowed for reduction in false positive detections. Isotope dilution method was used for quantification of all analytes. In cases where an identical isotopically-labeled standard was not available, a closely-related compound was used (Table S1†). Data analysis and processing was done with the Agilent MassHunter Quantitative (ver. 6.00) software.
3. Results and discussion
3.1. Selection of indicator compounds
To begin, a list of 48 TOrCs were selected as potential indicators for further evaluation. While selecting this list, TOrCs were divided into seven categories including pharmaceuticals, personal-care products, pesticides, natural & synthetic hormones, perfluorinated chemicals (PFCs), household chemicals and industrial/commercial chemicals. Since pharmaceuticals encompass many categories of drugs and are the most frequently researched group of TOrCs in water, it was decided to select a few representative pharmaceuticals in each sub-category based on previous literature available about usage, sales and detection in water in the US. The pharmaceuticals selected were from seven sub-categories: analgesics (acetaminophen, diclofenac, ibuprofen, naproxen); antibiotics (ciprofloxacin, erythromycin, sulfamethoxazole, trimethoprim); anti-allergens (diphenhydramine); anti-hypertensives (diltiazem, hydrochlorothiazide, meprobamate); beta-blockers (atenolol, propranolol); lipid-regulators (clofibric acid, gemfibrozil); and psychiatrics (carbamazepine, fluoxetine, primidone). Table 2 indicates the compounds selected for the initial screening with their relevant classes.
Table 2 Initial screening of TOrCs to determine indicator list based on PSS
Compound |
Category |
n
|
DFa (%) |
Meana (ng L−1) |
No. of studiesa |
Score |
DF |
Occurrence |
Research |
Total |
n: number of samples; DF: detection frequency; DF scoring: 1 (51–70%), 2 (71–85%), 3 (>85%); occurrence scoring (based on mean): 1 (51–200 ng L−1), 2 (201–500 ng L−1), 3 (>500 ng L−1); research scoring (based on no. of studies): 1 (2–4), 2 (5–9), 3 (>9). HC: household chemical; I/CC: industrial/commercial chemical; PCP: personal-care product; Pest.: pesticide; PFC: perfluorinated compound; Phar.: pharmaceutical; S/H: Steroid/Hormone. Literature cited.1,17,21,25,27–58 |
Acesulfame |
HC |
17 |
94 |
1510 |
2 |
3 |
3 |
1 |
7 |
Acetaminophen |
Phar. |
60 |
25 |
80 |
2 |
0 |
1 |
1 |
2 |
Atenolol |
Phar. |
209 |
94 |
794 |
9 |
3 |
3 |
2 |
8 |
Atrazine |
Pest. |
28 |
32 |
17 |
6 |
0 |
0 |
2 |
2 |
Benzophenone |
PCP |
29 |
93 |
192 |
6 |
3 |
1 |
2 |
6 |
Benzotriazole |
I/CC |
8 |
88 |
24 |
2 |
3 |
0 |
1 |
4 |
Bisphenol A |
HC/I/CC |
52 |
69 |
141 |
8 |
1 |
1 |
2 |
4 |
Caffeine |
HC |
200 |
56 |
6920 |
11 |
1 |
3 |
3 |
7 |
Carbamezapine |
Phar. |
245 |
98 |
162 |
15 |
3 |
1 |
3 |
7 |
Ciprofloxacin |
Phar. |
72 |
57 |
72 |
4 |
1 |
1 |
1 |
3 |
Clofibric acid |
Phar. |
21 |
81 |
32 |
3 |
2 |
0 |
1 |
3 |
DEET |
PCP |
68 |
87 |
522 |
10 |
3 |
3 |
3 |
9 |
Dexamethasone |
S/H |
13 |
0 |
— |
2 |
0 |
0 |
1 |
1 |
Diclofenac |
Phar. |
72 |
76 |
275 |
10 |
2 |
2 |
3 |
7 |
Diphenhydramine |
Phar. |
10 |
90 |
494 |
2 |
3 |
3 |
1 |
7 |
Ditiazem |
Phar. |
59 |
83 |
120 |
3 |
2 |
1 |
1 |
4 |
Estrone |
S/H |
45 |
38 |
3 |
7 |
0 |
0 |
2 |
2 |
17β-Estradiol |
S/H |
150 |
3 |
0.1 |
6 |
0 |
0 |
2 |
2 |
17α-Ethynylestradiol |
S/H |
147 |
1 |
0.1 |
5 |
0 |
0 |
2 |
2 |
Fluoxetine |
Phar. |
67 |
54 |
27 |
6 |
1 |
0 |
2 |
3 |
Gemfibrozil |
Phar. |
118 |
83 |
332 |
13 |
2 |
2 |
3 |
7 |
Hydracortisone |
S/H |
65 |
6 |
1 |
4 |
0 |
0 |
1 |
1 |
Hydrochlorothiazide |
Phar. |
51 |
100 |
1110 |
2 |
3 |
3 |
1 |
7 |
Ibuprofen |
Phar. |
258 |
64 |
471 |
14 |
1 |
2 |
3 |
6 |
Iohexol |
I/CC |
18 |
100 |
5240 |
2 |
3 |
3 |
1 |
7 |
Iopamidol |
I/CC |
0 |
— |
— |
0 |
0 |
0 |
0 |
0 |
Iopromide |
I/CC |
24 |
96 |
282 |
5 |
3 |
2 |
2 |
7 |
Meprobamate |
Phar. |
29 |
97 |
367 |
8 |
3 |
2 |
2 |
7 |
Naproxen |
Phar. |
74 |
84 |
97 |
11 |
2 |
1 |
3 |
6 |
Norgestrel |
S/H |
12 |
50 |
8 |
3 |
1 |
0 |
1 |
2 |
PFBA |
PFC |
9 |
67 |
3 |
2 |
1 |
0 |
1 |
2 |
PFBS |
PFC |
8 |
38 |
1 |
2 |
0 |
0 |
1 |
1 |
PFNA |
PFC |
7 |
100 |
7 |
1 |
3 |
0 |
0 |
3 |
PFOA |
PFC |
27 |
81 |
59 |
4 |
2 |
0 |
1 |
3 |
PFOS |
PFC |
27 |
78 |
23 |
4 |
2 |
0 |
1 |
3 |
Prednisone |
S/H |
54 |
2 |
0 |
2 |
0 |
0 |
1 |
1 |
Primidone |
Phar. |
47 |
96 |
247 |
8 |
3 |
2 |
2 |
7 |
Propranolol |
Phar. |
70 |
83 |
32 |
3 |
2 |
0 |
1 |
3 |
Propylparaben |
I/CC |
17 |
59 |
4 |
3 |
1 |
0 |
1 |
2 |
Simazine |
Pest. |
34 |
56 |
9 |
5 |
1 |
0 |
2 |
3 |
Sucralose |
HC |
52 |
100 |
18 100 |
6 |
3 |
3 |
2 |
8 |
Sulfamethoxazole |
Phar. |
119 |
90 |
1040 |
13 |
3 |
3 |
3 |
9 |
TCEP |
PCP/HC |
48 |
90 |
452 |
9 |
3 |
2 |
2 |
7 |
TCPP |
PCP/HC |
26 |
96 |
2600 |
7 |
3 |
3 |
2 |
8 |
Testosterone |
S/H |
30 |
0 |
— |
5 |
0 |
0 |
2 |
2 |
Triclocarban |
PCP |
90 |
99 |
319 |
6 |
3 |
2 |
2 |
7 |
Triclosan |
PCP |
143 |
88 |
146 |
14 |
3 |
1 |
3 |
7 |
Trimethoprim |
Phar. |
235 |
87 |
185 |
14 |
3 |
1 |
3 |
7 |
To select the final indicator list, a priority scoring system (PSS) was used which incorporated the (i) detection frequency (DF) in wastewater effluents, (ii) mean concentration in effluents and (iii) number of relevant studies reporting the compound in wastewater. To account for the development of more reliable analytical techniques and lower detection limits, a detailed literature search of peer-reviewed research articles for occurrence of these chemicals was performed from 2005–2015 only. Further, with differences in usage trends and consumption patterns of TOrCs, this review was confined to studies in the US only. Nonetheless, this PSS principle could be applied to any region around the world with minimal modifications. The PSS awarded between 0–3 points to every compound for each category (i–iii) listed above. Higher points were awarded for greater detection frequencies, and mean concentrations in the effluent. To minimize bias that could occur due to one or few studies reporting high concentrations at a particular plant, a third category for number of studies reporting the compound (absence or presence) in wastewater effluents was used. Highest points were awarded to compounds reported in more studies. The details of the PSS are presented in the ESI† (Table S3).
While this method is a convenient and cost-effective way to determine an indicator list, certain limitations do exist. This method inherently assumes every article included in the literature review is equally important. Further, some studies reported TOrCs in several wastewater effluents and hence had a greater impact on the detection frequency and mean of TOrCs than other studies. Finally, multiple reporting of the same wastewater effluent may have biased the final concentration and detection frequency of some compounds. While the authors made every effort to not include multiple studies reporting a TOrC at the same wastewater plant, this was hard to discern in many instances. Despite these limitations, the method is still useful in determining an indicator list without costly and labor-intensive experimental studies.
Details of the literature review and the scoring for each TOrC is presented in Table 2. All TOrCs with a score >6 (out of 9) were selected in the final indicator list. This list consisted of twenty compounds including ten pharmaceuticals, five personal-care products, three household chemicals and two industrial/commercial chemicals. No steroid hormones were present in the final indicator list as they had very low and in some cases no detections in wastewater effluents while their mean concentrations were very low too. This is not unexpected as they are generally well attenuated by conventional water treatment processes in wastewater plants.22 Similarly, no PFCs were present in the final list too. In their case, detection frequencies for some of them were relatively high in the studies that reported them but the detected concentrations were low and hence make them hard to measure, thereby hindering their use as good indicator compounds. Further, PFCs are generally released from very specific industries that use them in manufacture and detected at high concentrations in certain areas, while completely absent in others.23,24 Sucralose was detected with the highest mean concentration (18.1 μg L−1) in the literature review while it was one of three compounds ubiquitously detected in all studies that measured them along with hydrochlorothiazide and iohexol. However, the later two TOrCs were only reported in two studies while sucralose concentrations in wastewater effluents was reported in six studies considered in this literature review. Acesulfame, hydrochlorothiazide, iohexol, sucralose and TCPP were all detected at mean concentrations >1 μg L−1 with >95% DF in this study. Caffeine was the second highest compound in terms of mean concentration at 6.9 μg L−1 but was only detected in 56% of the wastewater samples considered. The fact that caffeine is a highly biodegradable compound and would be expected to be very well removed in a secondary activated sludge treatment system could indicate that a large number of wastewater plants considered in this study have antiquated treatment systems that need upgrading especially for attenuation of TOrCs.25,26
3.2. Method validation
3.2.1. LOQ and MRL.
Instrumental limit of quantification (LOQ) was determined to be the lowest concentration in which the signal to noise ratio (SNR) was greater than ten for all transitions. A set of standards ranging from 1 ng L−1 to 200 ng L−1 was analyzed in order to determine LOQs. Limits of quantification ranged from 2 ng L−1 to 100 ng L−1, with most analytes being lower than 20 ng L−1.
Procedures for the determination of MRLs were adapted from previously published literature.59 Briefly, eight injections of a fortified sample with isotopically-labeled surrogate standards and target analytes in ultrapure water at 2–3 times the LOQs were analyzed (fortification levels are shown in Table 3). The standard deviation of the eight replicates was multiplied by the student's t-test value for n − 1 degrees of freedom at 99% confidence levels to determine the MRL of each target compound. MRLs for all compounds ranged from 3 ng L−1 to 39 ng L−1, with the exception of sucralose at 302 ng L−1 (Table 3). The comparatively high MRL for sucralose was still several times lower than levels detected in wastewater effluent traditionally.16 MRLs of DEET and TCPP were adjusted to twice the level in the blank due to small instrumental contamination.
Table 3 LOQs and MRLs for all target analytes in ultrapure water
Compound |
LOQ (ng L−1)a |
Fortification level (ng L−1) |
MRL (ng L−1) |
Lowest standard where signal to noise >10.
MRL adjusted to 2× blank level.
|
Acesulfame |
5 |
10 |
4 |
Atenolol |
20 |
50 |
37 |
Caffeine |
5 |
10 |
8 |
Carbamezapine |
2 |
5 |
3 |
DEET |
10 |
20 |
34b |
Diclofenac |
10 |
20 |
14 |
Diphenylhydramine |
5 |
10 |
7 |
Gemfibrozil |
50 |
100 |
23 |
Hydrochlorothiazide |
10 |
20 |
15 |
Iohexol |
10 |
20 |
13 |
Iopromide |
20 |
50 |
39 |
Meprobamate |
10 |
20 |
8 |
Primidone |
10 |
20 |
9 |
Sucralose |
100 |
200 |
302 |
Sulfamethoxazole |
5 |
10 |
5 |
TCEP |
20 |
50 |
20 |
TCPP |
10 |
20 |
22b |
Triclocarban |
10 |
20 |
14 |
Triclosan |
10 |
20 |
15 |
Trimethoprim |
5 |
10 |
11 |
3.2.2. Spike recovery test.
Recoveries of the target analytes were determined using three replicates in two different WWTP (1 & 2) effluent samples. Two different matrix spike levels were chosen: 1000 ng L−1 and 5000 ng L−1. Spike recoveries were determined by comparing the concentration of the spiked samples with the concentration obtained in the effluent. Recovery levels for WWTP 1 varied from 83% to 138%, with the exception of iohexol (46% for the 1000 ng L−1 level) and sucralose (67% for the 5000 ng L−1 level), for both spiking levels, 18 out of 20 compounds had recoveries between 75% and 125%. The low recoveries of iohexol and sucralose in WWTP 1 might be associated with the high concentrations observed in the effluent sample, more than 20 times higher than the lower spiking level. Seitz and colleagues, using large volume injection for the analysis of TOrCs in wastewaters also reported lower recoveries for iodinated contrast media, such as iohexol, when compared to other analytes classes.60 For the WWTP, 2 recoveries were between 85% and 149%; with the exception of sucralose (74% for the 5000 ng L−1 level), for both spiking levels, 18 out of 20 compounds had recoveries between 75% and 125% for both spiking levels. Overall, 18 out of 20 analytes had RSDs lower than 5% in both WWTPs for both spiking levels. Spike recoveries for all of the target analytes are shown in Table 4.
Table 4 Compound matrix spike recoveries at two levels in WWTP 1 and WWTP 2 (n = 3)
Compounds |
WWTP 1 |
WWTP 2 |
Eff. conc |
Spike level 1000 ng L−1 |
Spike level 5000 ng L−1 |
Eff. conc |
Spike level 1000 ng L−1 |
Spike level 5000 ng L−1 |
ng L−1 |
RSD % |
Spike recovery (%) |
RSD (%) |
Spike recovery (%) |
RSD (%) |
ng L−1 |
RSD % |
Spike recovery (%) |
RSD (%) |
Spike recovery (%) |
RSD (%) |
Acesulfame |
1876 |
1.5 |
107 |
2.6 |
99 |
3.9 |
80 |
5 |
106 |
5 |
98 |
2.1 |
Atenolol |
1241 |
1.3 |
106 |
2.5 |
101 |
4.2 |
271 |
6.4 |
107 |
2.9 |
95 |
4.5 |
Caffeine |
4 |
6.8 |
97 |
3 |
94 |
0.5 |
<MRL |
— |
96 |
2.8 |
92 |
2 |
Carbamezapine |
530 |
1.4 |
101 |
0.9 |
98 |
1.4 |
394 |
0.5 |
102 |
3 |
98 |
2.2 |
DEET |
309 |
4.9 |
101 |
3.9 |
102 |
1.8 |
83 |
16.5 |
99 |
0.7 |
96 |
1.2 |
Diclofenac |
266 |
8.7 |
95 |
2.5 |
83 |
5.2 |
17 |
10.1 |
100 |
2.8 |
102 |
0.6 |
Diphenylhydramine |
3953 |
2.4 |
111 |
2.1 |
104 |
0.4 |
25 |
3.2 |
102 |
2.4 |
95 |
6.4 |
Gemfibrozil |
363 |
8.3 |
100 |
3.1 |
90 |
3.1 |
37 |
17.1 |
97 |
4.3 |
90 |
1.5 |
Hydrochlorothiazide |
2066 |
4.2 |
105 |
4.2 |
114 |
3.1 |
206 |
7.8 |
123 |
2 |
107 |
9.8 |
Iohexol |
23 423 |
6.2 |
46 |
7.9 |
135 |
1.1 |
1262 |
9.5 |
125 |
2.5 |
88 |
0.4 |
Iopromide |
1463 |
1 |
111 |
3 |
128 |
2.5 |
154 |
5.7 |
125 |
13.6 |
119 |
1.6 |
Meprobamate |
507 |
0.3 |
101 |
3.3 |
99 |
1.2 |
583 |
3.1 |
103 |
1.5 |
101 |
1.5 |
Primidone |
166 |
10.2 |
124 |
2.3 |
115 |
2.8 |
290 |
6.7 |
120 |
2.4 |
112 |
1.3 |
Sucralose |
43 873 |
3.7 |
112 |
4.6 |
67 |
6.9 |
49 152 |
2.4 |
85 |
4.3 |
74 |
7 |
Sulfamethoxazole |
1015 |
0.7 |
110 |
1.4 |
99 |
0.2 |
13 |
6.6 |
102 |
1 |
100 |
0.7 |
TCEP |
253 |
4.5 |
109 |
3.4 |
97 |
2.8 |
299 |
11.5 |
104 |
1.4 |
98 |
1.5 |
TCPP |
2730 |
13.4 |
138 |
12.4 |
100 |
1.5 |
2722 |
11.8 |
149 |
0.8 |
116 |
4.3 |
Triclocarban |
307 |
12.4 |
97 |
1.7 |
93 |
2.5 |
165 |
16.5 |
100 |
0.7 |
97 |
1.8 |
Triclosan |
150 |
1.7 |
101 |
1.3 |
94 |
0.8 |
30 |
12.6 |
103 |
0.8 |
96 |
2.3 |
Trimethoprim |
393 |
3 |
99 |
3.7 |
99 |
1.8 |
47 |
6.9 |
104 |
2.8 |
99 |
6.7 |
3.2.3. Matrix effects.
When using LC-MS/MS interfaced with electrospray ionization (ESI), matrix effects resulting from the coelution of matrix components with analytes during HPLC can suppress or enhance the ionization of TOrCs, which will lead to inaccuracy in quantification.61 Isotope dilution technique is most often applied to compensate for the matrix effects in quantification. Since direct injection does not include any other pretreatment procedures (such as extraction or cleanup), the matrix effects can be assessed by comparing the signals of the analytes in spiked water samples and in standards (ultrapure water). ILSSs were used for assessment of matrix effects in this study, instead of the native compounds. The use of ILSSs allows us to evaluate matrix effects in particular samples and also avoid the interference of the extremely high concentration levels of some analytes (such as sucralose, caffeine and iohexol) in wastewater effluents. A total of 10 effluent samples (5 from WWTP 1 and 5 from WWTP 2) spiked with seventeen ILSSs at concentration of 1 μg L−1 were analyzed. Matrix effects (%) were quantified by the following equation:
where ACspiked is the area counts of ILSSs detected in spiked wastewater effluent, ACstandard is the area counts of ILSSs in standards at spiking level. The results are shown in Fig. 1.
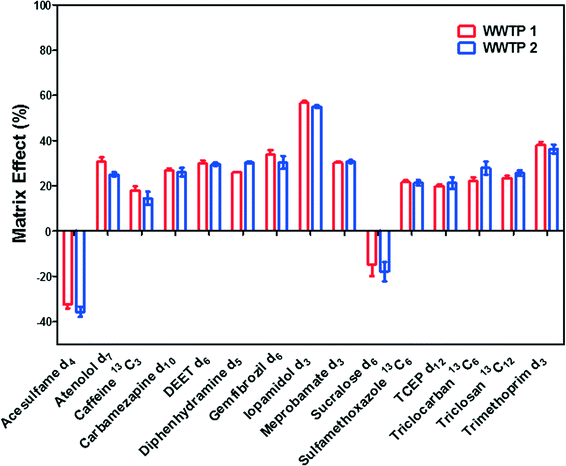 |
| Fig. 1 Matrix effects in wastewater effluent on isotopically labelled surrogate standards (n = 5). | |
Matrix suppression occurred to most of ILSSs, whereas the signals of acesulfame-d4 and sucralose-d6 were enhanced by matrix. Iopamidol-d3 weas severely suppressed by up to approximately 60%, followed by trimethoprim-d3 (about 40%), indicating high ion suppression for iodinated X-ray contrast media (iohexol, iopamidol, and iopromide) in wastewater effluent, which is similar as previously reported.62,63 Acesulfame and sucaralose were reported to have ion enhancement in influents and effluents,64 which was consistent with the fact that acesulfame-d4 and sucralose-d6 exhibited signal enhancement in this study. However, the results indicated limited matrix effects (<30% change in signals) for most of analytes in wastewater effluent. Matrix effects of this direct injection method were significantly lower than those experienced when analyzing similar compounds using SPE-LC-MS/MS in other studies.65,66
3.2.4. Linearity and precision.
Linearity for each calibration curve was expressed with the correlation coefficient (R2), where the calibration curve started above each analyte's MRL and ended at 10 μg L−1. A linear regression fit with 1/X weighting was used for all analytes. All analytes had an R2 > .99 and 15 of those analytes had a R2 > .995. The precision of the method was validated by inter-day and intra-day variation of a 2 μg L−1 standard, expressed as relative standard deviation (RSD). Intra-day variation was determined using a 2 μg L−1 standard run three times on the same day within 3 hours of each other. Inter-day variation compared a 2 μg L−1 standard run once on three different days. The intra-day variation for all analytes were under 5% and the inter-day variation had 14 analytes under 5%. This could be due to storage of the sample in water which could affect the integrity of the sample. However, all analytes except sucralose (12%) and TCEP (14%) had inter-day RSD's <10% which is acceptable. Complete results for the linearity and precision values are presented in Table 5.
Table 5 Linearity and precision for all target analytes (n = 3)
Compound |
Linearity |
Intra-day variability |
Inter-day variability |
Acesulfame |
0.9987 |
0.35 |
1.4 |
Atenolol |
0.9964 |
1.3 |
7.7 |
Caffeine |
0.9967 |
3.8 |
0.69 |
Carbamezapine |
0.9989 |
1.5 |
6.2 |
DEET |
0.9951 |
4.6 |
0.47 |
Diclofenac |
0.9982 |
1.0 |
2.0 |
Diphenhydramine |
0.9904 |
2.1 |
2.7 |
Gemfibrozil |
0.9974 |
3.8 |
3.3 |
Hydrochlorothiazide |
0.9983 |
2.5 |
1.0 |
Iohexol |
0.9988 |
1.9 |
0.48 |
Iopromide |
0.9975 |
1.8 |
2.2 |
Meprobamate |
0.9975 |
1.5 |
1.2 |
Primidone |
0.9963 |
2.7 |
0.12 |
Sucralose |
0.9970 |
0.55 |
12 |
Sulfamethoxazole |
0.9971 |
2.2 |
0.30 |
TCEP |
0.9974 |
0.84 |
14 |
TCPP |
0.9924 |
2.4 |
5.6 |
Triclocarban |
0.9929 |
2.3 |
9.9 |
Triclosan |
0.9927 |
1.0 |
1.1 |
Trimethoprim |
0.9941 |
2.8 |
0.97 |
3.3. Analysis of wastewater effluent samples
The occurrence data for indicator TOrCs in wastewater effluents is presented in Fig. 2. The individual mean concentrations and standard deviation for each indicator TOrC is shown in Fig. 2a and c, while detection frequencies are presented in Fig. 2b and d respectively. Detailed discussion on the occurrence and concentrations of TOrCs in each WWPT effluent is given below.
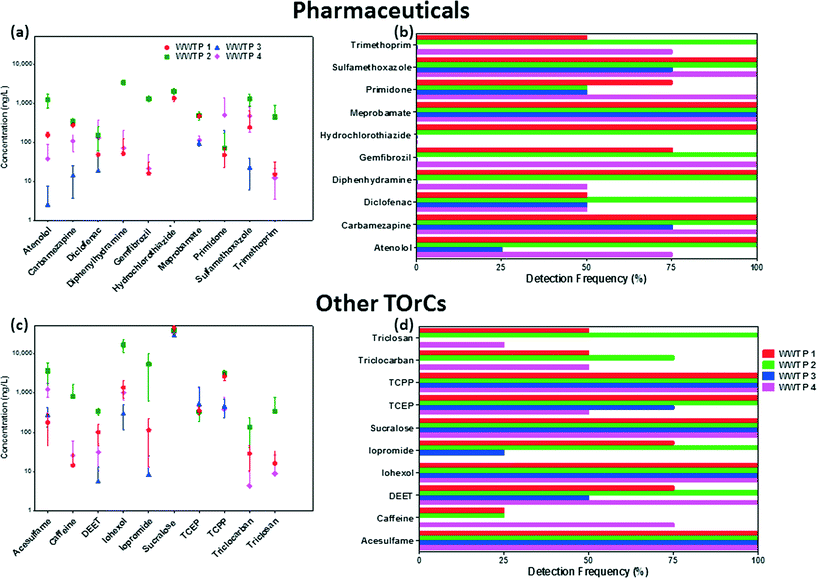 |
| Fig. 2 Mean (with standard deviation) effluent concentrations of indicator pharmaceuticals (a) and other TOrCs (c) with detection frequencies (b and d) for all 4 wastewater treatment plants tested (n = 4). *Hydrochlorothiazide not analyzed in WWTP 3 & 4. | |
3.3.1. Occurrence pattern of indicator TOrCs in wastewater effluent.
Wastewater effluents from four plants around the US with vastly different treatment processes and treatment capacities were analyzed for the twenty indicator TOrCs selected. Each plant was sampled at the effluent four times over a year. All twenty TOrCs were detected at least once in the effluent of WWTP 1 and 2. WWTP 4 also had detections for all indicator TOrCs at least once with the exception of the X-ray contrast media iopromide. However, WWTP 3 had far less detections of TOrCs in the effluent with caffeine, diphenhydramine, gemfibrozil, triclosan, triclocarban and trimethoprim all not detected during any of the four sample campaigns. While, lower concentrations in the influent water may be a reason, all these compounds have high reaction rate constants with ozone and hydroxyl radical and may have been attenuated by the ozonation process in this plant. The effluent from WWTP 2 had the most TOrCs detected with all compounds except caffeine (25%), primidone (50%) and triclocarban (75%) being detected in all four sample campaigns. Acesulfame, iohexol, meprobamate, sucralose and TCPP were detected in every sample at all four WWTP effluents. Hydrochlorothiazide was also detected in every sample collected in WWTP 1 and 2 but was not analyzed in WWTP 3 and 4. Meanwhile, sulfamethoxazole and carbamazepine had 100% detection frequency in WWTPs 1, 2 and 4 with 75% detection in WWTP 3. Statistically, caffeine was the least detected indicator with only five of the sixteen effluent samples testing positive with no detections in WWTP 3, one detection in WWTP 1 and 2, and three in WWTP 4.
The TOrC footprint was highest in the effluent of WWTP 2. The average TOrC concentration in WWTP 2 effluent was 953 ng L−1, while effluent of WWTP 1, 3, and 4 had 708 ng L−1, 409 ng L−1 and 498 ng L−1 respectively. When considering TOrC loading to the environment, WWTP 2 (115 mg d−1) and WWTP 4 (101.8 mg d−1) had similar discharges due to the larger treatment capacity of WWTP 4 (Table 1). WWTP 3 had the lowest impact on the environment in terms of daily TOrC loading at 15.5 mg d−1. WWTP 1 had a daily TOrC discharge of 67.1 mg d−1 which was 40% lower than WWTP 2 even though the treatment capacity is only about 20% smaller. This is interesting as the influent source and type for WWTP 1 and WWTP 2 is the same and may give insights into treatment efficiency of these two plants towards TOrCs. It should be noted that while the influent is similar for both these plants, samples were not collected at the same time and hence diurnal variations may have contributed to this. Nonetheless, the lower TOrC concentrations in the effluent of WWTP 1 compared to WWTP 2 were consistent during all four sample events. The presence of the selected TOrCs in the four WWTP effluents suggests that they have the potential to be good indicator compounds of wastewater. However, to be a suitable indicator, it must be easily detected in water by current analytical techniques such as the one described in this study. The next section will summarize the concentration of the indicator TOrCs in the WWTPs sampled with relation to the MRLs of the analytical method.
3.3.2. Concentration of indicator TOrCs in wastewater effluent.
3.3.2.1. Pharmaceuticals.
The final indicator list consisted of ten pharmaceuticals encompassing seven categories. Two antibiotics (sulfamethoxazole, trimethoprim), anti-hypertensives (hydrochlorothiazide, meprobamate) and psychiatric drugs (carbamazepine, primidone) were selected while one analgesic (diclofenac), anti-allergen (diphenhydramine), β-blocker (atenolol) and lipid-regulator (gemfibrozil) were also on the final list.
Antibiotics.
The mean effluent concentration of sulfamethoxazole in WWTP 1, 2 and 4 were 873 ng L−1, 1290 ng L−1 and 473 ng L−1 respectively. Levels in the effluent of WWTP 3 were significantly lower with an average of 22.8 ng L−1; highest concentration of 41 ng L−1 and one non-detect. The presence of ozone treatment at WWTP 3 could have had an effect on effluent concentrations due to its ability to degrade sulfamethoxazole even in wastewater matrices.67 Several studies have indicated the rapid attenuation of sulfamethoxazole by ozone in wastewater even at low doses.42,68 Trimethoprim was detected in every sample collected at WWTP 2 with a mean concentration of 441 ng L−1. However, it's concentration in the other three effluents were significantly lower and non-detect in all samples collected from WWTP 3. The average concentrations of the two antibiotics in the literature (Table 2) were similar to those detected in the effluents of WWTP 1, 2 and 4. The low concentrations in WWTP 3 may be due to less loading in the influent of this plant, but more likely due to the susceptibility of these compounds to ozonation.69,70
Anti-hypertensives.
Hydrochlorothiazide was detected in every sample collected at both WWTP 1 and 2 with average concentration >1000 ng L−1. This was consistent with a study by Kostich et al. that detected the chemical in every sample from 50 WWTP effluents in the US with an average concentration of 1100 ng L−1.1 Hydrochlorothiazide is estimated to be the most commonly prescribed anti-hypertensive medicine in the US and hence could be expected to be present in water.71 Meprobamate was detected in every sample at all four WWTP effluents. The average concentration at WWTP 1 and 2 were ~500 ng L−1, indicating that it was not affected by different treatment processes at the two plants (which have a very similar influent source). This is not surprising as meprobamate is very recalcitrant to biodegradation in water treatment.72 Concentrations in WWTP 3 and 4, were lower but still present in every effluent sample collected. The prevalence of meprobamate in wastewater effluents undergoing different treatments including ozone and GAC is due to its low reaction rate constant with ozone (kO3: <1 M−1 s−1) and octanol–water partition coefficient (log
Kow: 0.7). The mean concentration of meprobamte in the literature review was 397 ng L−1 which was similar to WWTP 1 and 2 using conventional biological treatment and no advanced treatments (like O3 and GAC).
Anti-psychiatrics.
The anti-psychiatric drug carbamazepine was detected in all samples in WWTP 1, 2 and 4 with average concentrations of 357
339 and 107 ng L−1 respectively. In WWTP 3, only 3 of the 4 effluent samples tested positive for carbamazepine with an average concentration of 14.5 ng L−1. The high reaction rate constants with ozone (kO3: 3 × 105 M−1 s−1) along with previously reported literature on rapid attenuation of carbamazepine even with low doses of ozone corroborate these data.42 Primidone detection frequency and concentrations were notably lower in WWTP 1, 2 and 3, while WWTP 4 effluent had much higher mean concentration of 493 ng L−1 with 100% DF. While the difference in concentrations between WWTP 4 and others cannot be explained clearly, low sorption potential to GAC (log
Kow: −0.8) and different usage patterns of primidone across the country could be potential reasons.
Miscellaneous pharmaceuticals.
Atenolol, diclofenac and diphenhydramine were detected in all effluent samples at WWTP 1 and 2 at average concentrations varying from 103–3, 370 ng L−1. Gemfibrozil was detected in every samples of WWTP 2 with a mean concentration of 1310 ng L−1, while 3 out of 4 samples in WWTP 1 contained it at an average of 201 ng L−1. All four compounds had highest average concentration in WWTP 2 effluent. All these pharmaceuticals had significantly lower average concentrations in WWTP 3 and 4 effluents with means ranging from non-detect to 131 ng L−1. Diphenhydramine and gemfibrozil were not detected in any effluent sample of WWTP 3 while atenolol and diclofenac were detected in only 1 and 2 samples respectively. All these compounds are relatively susceptible to ozonation due to their moderate to large reaction rate constants with ozone and hydroxyl radical which could explain the low concentrations in WWTP 3 effluent.73 Further, these compounds are fairly well removed by GAC adsorbers which could have resulted in lower effluent concentrations in WWTP 4.74,75
3.3.2.2. Other TOrCs.
Ten other TOrCs were also included in the final list based on the results of the literature review. These included two artificial sweeteners (acesulfame, sucralose), anti-microbial agents (triclosan, triclocarban), flame-retardants (TCEP, TCPP), and X-ray contrast media (iohexol, iopromide). Also, a stimulant (caffeine) and insect repellent (DEET) were included in the final selection of indicator TOrCs.
Artificial sweeteners.
Both sucralose and acesulfame were detected in all samples at the four WWTP effluents. Sucralose was the most prevalent indictor in all four wastewater effluents in this study based on its average concentration. Mean sucralose concentrations in the effluent were a lot higher than acesulfame in all plants ranging from 29
300–49
400 ng L−1. Average acesulfame concentrations were atleast 10 fold lower than sucralose in each plant ranging from 139 ng L−1 (WWTP 1) to 3660 ng L−1 (WWTP 2). This is in contrast with a study in Saudi Arabia that had higher effluent acesulfame concentrations compared to sucralose but corroborates a study in the US showing opposite trends.41,76 Thus, consumption patterns can play a large role in selection of appropriate indicators especially in diverse geographical regions and further supports the theory of selection of multiple indicators in a same/similar class of compound.
Anti-microbial agents.
Both triclosan and triclocarban were only detected in WWTP 1, 2 and 4, while only triclosan in WWTP 2 was detected in all four sample campaigns. Triclosan was present at higher mean concentrations than triclocarban in all three WWTPs with an average of 35 ng L−1, 353 ng L−1 and 9 ng L−1 in WWTP 1, 2 and 4 respectively. Triclocarban was present at average effluent concentrations of 19 ng L−1, 136 ng L−1 and 5 ng L−1 in WWTP 1, 2 and 4. Like most of the indicator TOrCs, both anti-microbial agents were detected at highest concentrations in the effluent of WWTP 2.
Flame-retardants.
TCPP was detected in every sample at all four WWTPs, while TCEP was detected in all samples of WWTP 1 and 2 but had 1 and 2 non-detects in WWTP 4 and 3 respectively. Interesting trends were noticed for these two flame retardants. While average concentrations of TCPP were almost 10 fold greater than TCEP in WWTP 1 (3540 ng L−1vs. 378 ng L−1) and WWTP 2 (3130 ng L−1vs. 310 ng L−1), while WWTP 3 (462 ng L−1vs. 543 ng L−1) and WWTP 4 (383 ng L−1vs. 473 ng L−1) had slightly higher TCEP mean concentrations. Previous studies have indicated very poor removal on non-chlorinated flame-retardants in WWTPs using conventional and advanced oxidation treatments,77,78 thus these differences could be due to different consumption and usage patterns. Similar average concentrations in WWTP 1 and 2 effluents that are supplied with the same influent source also indicates that removal efficiency of these compounds is not affected in treatment train and these differences are likely due to usage trends in the area.
X-ray contrast media.
Vastly different trends were noticed for the two X-ray contrast media selected as indicators in this study with regards to occurrence and effluent concentrations. While iohexol was detected in all samples at the four WWTPs, iopromide was only present in all samples at WWTP 2, while it was not detected at all in WWTP 4. Iohexol concentrations had significant variations with means of 1680 ng L−1, 16
700 ng L−1, 302 ng L−1 and 1000 ng L−1 in WWTP 1, 2, 3 and 4 respectively. On the other hand, iopromide concentrations in WWTP 1, 3 and 4 were significantly lower at 76 ng L−1, 8.5 ng L−1 and non-detect. However, WWTP 2 had an average iopromide effluent concentration of 5390 ng L−1. The variations in concentration of the two X-ray contrast media are hard to explain but geographical trends have been reported before. A study in Australia noticed higher iohexol concentrations than iopromide in pre-reverse osmosis wastewater effluent similar to this study.62 However, a study at a German WWTP reported similar concentrations for both compounds in influent and effluent.79
Insect-repellent.
DEET concentrations had significant variations in all plants sampled. It was detected in all samples at WWTP 2 and 4, while 75% and 50% detections were noted in WWTP 1 and 3 respectively. Average effluent concentrations at WWTP 1, 2, 3 and 4 were 98 ng L−1, 345 ng L−1, 16 ng L−1 and 31 ng L−1 respectively. Large seasonal and geographical variations of DEET have been reported previously in the US.80
Stimulant.
Caffeine was statistically the least frequently detected indicator in this study. It was not detected in WWTP 3 and less than 50% in WWTP 1 and 2. WWTP 4 had the highest average concentration of 81 ng L−1 with 75% detection frequency. Low concentrations of caffeine would be expected as it is easily biodegraded by conventional secondary biological treatments in WWTPs and fairly susceptible to ozone (employed in WWTP 3) as well.26,42 Interestingly, the average concentrations reported in this study at the four WWTPs were more than 100 times lower than the average concentration in the literature review. One reason could be that the plants sampled in this study have modern treatment trains which have been upgraded recently or employed advanced treatments specifically for attenuation of some TOrCs.
3.4. Criteria and feasibility of selected TOrCs as indicators
The occurrence data at four different WWTPs presented above suggest that the indicator list selected through the literature review and PSS is robust and reliable. Certain compounds in the final indicator list are present at high concentrations and occur ubiquitously in all four plants like sucralose, hydrochlorothiazide, iohexol, acesulfame and TCPP. These compounds can be considered as ‘universal’ indicators as they can be expected to be present in wastewater effluents regardless of treatment process and efficiency. Since they are also present at significantly higher concentrations than their MRLs, these compounds can be used to predict wastewater impact on a water by back-calculating the observed concentrations and considering dilution. Some compounds like carbamazepine, meprobamate and sulfamethoxazole are present almost ubiquitously in all wastewater samples but either have lower concentrations than the universal indicators or have larger concentration fluctuations due to susceptibility to certain treatment processes. These compounds can be used as indicators when the treatment systems are well classified and not expected to affect concentrations of these compounds regularly. Further, some compounds can be used as indicators of unit process efficacy i.e. if the specific treatment process is working well or not. Examples include caffeine (for biological treatment), sulfamethoxazole, gemfibrozil, triclocarban (for ozone), triclosan (for GAC) etc. If the unit processes are working well, the corresponding indicator compounds would be expected to be completely removed or be present at very low concentrations after treatment. Certain compounds like DEET should be used as part of indicator lists to account for seasonal variations in use, while some redundancy in selecting multiple compounds from similar class of chemicals is required to anticipate consumption and geographical variations (ex. iopromide and iohexol as X-ray contrast media). The selection of a robust indicator list should consider all these factors and include chemicals that satisfy all these criteria while accommodating certain redundancies to account for seasonal variations and consumption patterns.
4. Conclusions
The presence of TOrCs in wastewater effluent is a well-known fact. In this study, a detailed literature review of occurrence of TOrCs in wastewater effluent in the US was conducted and using a priority scoring system, twenty suitable indicator TOrCs in wastewater were selected. Criteria for selection included detection frequency, occurrence concentration and number of peer review articles available detailing occurrence of the compound in wastewaters in the US. The final list included 10 pharmaceuticals and 10 other compounds including personal-care products and industrial chemicals. A rapid analytical method using direct injection LC-MS/MS was developed to analyze all these compounds in one run and without the need for any extraction. Method validation was performed by analyzing matrix spike recoveries, matrix effects, linearity of calibration curves and precision in wastewater. The method reporting limits ranged from 3–39 ng L−1 except for sucralose (302 ng L−1) and were all much lower than background concentrations present in wastewater.
Finally, the 20 indicator TOrCs were analyzed at four disparate WWTPs during four sample events across one year. The results indicate that all the selected TOrCs were detected at least once in at least three of the four plants. Compounds like sucralose, iohexol, TCPP, acesulfame and hydrochlorothiazide were detected in all samples collected and high average concentrations to be considered as universal indicators which you suggest impact of wastewater on receiving waters after taking into account dilution. Other indicators like DEET, caffeine, sulfamethoxazole, triclosan and carbamazepine were also present frequently and at concentrations above their MRLs hence could be used as indicators of seasonal variation, treatment process efficacy and consumption/usage patterns. The use of multiple compounds in the same/similar class of chemicals as indicators adds redundancy and allows accounting of seasonal and geographical differences (e.g. acesulfame and sucralose as artificial sweeteners, iohexol and iopromide as X-ray contrast media) that may occur. The findings of this study provide a list of the most frequently occurring TOrCs in wastewaters in the US through a detailed literature review following by a systematic monitoring approach. The selection of a robust indicator list is essential for monitoring programs that wish to study the fate and attenuation of TOrCs and their larger influence in water reuse.
Acknowledgements
The authors would like to thank the operators at the four WWTPs for their assistance in sample collection. We appreciate assistance in data collation by Justin D. Habit at the University of Arizona. We especially acknowledge Agilent Technologies for help with setup and maintenance of the instrumentation used in this study.
References
- M. S. Kostich, A. L. Batt and J. M. Lazorchak, Environ. Pollut., 2014, 184, 354–359 CrossRef CAS PubMed.
- T. A. Ternes, Water Res., 1998, 32, 3245–3260 CrossRef CAS.
- C. I. Kosma, D. A. Lambropoulou and T. A. Albanis, Sci. Total Environ., 2014, 466–467, 421–438 CrossRef CAS PubMed.
-
M. D. Swayne, G. H. Boone and D. J. S. L. Bauer, Wastewater in Receiving Waters at Water Supply Abstraction Points, U.S. Environmental Protection Agency, Washington, D.C., 1980 Search PubMed.
- T. Heberer, J. Hydrol., 2002, 266, 175 CrossRef CAS.
- P. E. Stackelberg, J. Gibs, E. T. Furlong, M. T. Meyer, S. D. Zaugg and R. L. Lippincott, Sci. Total Environ., 2007, 377, 255–272 CrossRef CAS PubMed.
- P. Westerhoff, Y. Yoon, S. Snyder and E. Wert, Environ. Sci. Technol., 2005, 39, 6649–6663 CrossRef CAS.
- D. W. Kolpin, E. T. Furlong, M. T. Meyer, E. M. Thurman, S. D. Zaugg, L. B. Barber and H. T. Buxton, Environ. Sci. Technol., 2002, 36, 1202–1211 CrossRef CAS.
- M. J. Benotti, R. A. Trenholm, B. J. Vanderford, J. C. Holady, B. D. Stanford and S. A. Snyder, Environ. Sci. Technol., 2009, 43, 597–603 CrossRef CAS.
- F. S. vom Saal and C. Hughes, Environ. Health Perspect., 2005, 113, 926–933 CrossRef CAS.
- A. Kortenkamp, Environ. Health Perspect., 2007, 115, 7–7 CrossRef PubMed.
- C. G. Daughton and T. A. Ternes, Environ. Health Perspect., 1999, 107, 907–938 CrossRef CAS.
- S. A. Snyder, J. - Am. Water Works Assoc., 2014, 106, 38–50 CrossRef CAS.
- E. R. V. Dickenson, S. A. Snyder, D. L. Sedlak and J. E. Drewes, Water Res., 2011, 45, 1199–1212 CrossRef CAS PubMed.
- M. Badruzzaman, J. A. Oppenheimer and J. G. Jacangelo, Water Res., 2013, 47, 6198–6210 CrossRef CAS PubMed.
- J. Oppenheimer, A. Eaton, M. Badruzzaman, A. W. Haghani and J. G. Jacangelo, Water Res., 2011, 45, 4019–4027 CrossRef CAS PubMed.
- S. Merel, T. Anumol, M. Park and S. A. Snyder, J. Hazard. Mater., 2015, 282, 75–85 CrossRef CAS PubMed.
- E. R. V. Dickenson, J. E. Drewes, D. L. Sedlak, E. C. Wert and S. A. Snyder, Environ. Sci. Technol., 2009, 43, 6242–6247 CrossRef CAS.
- B. J. Vanderford, J. E. Drewes, A. Eaton, Y. C. Guo, A. Haghani, C. Hoppe-Jones, M. P. Schluesener, S. A. Snyder, T. Ternes and C. J. Wood, Anal. Chem., 2013, 86, 774–782 CrossRef PubMed.
- W. J. Backe and J. A. Field, Environ. Sci. Technol., 2012, 46, 6750–6758 CrossRef CAS PubMed.
- T. Anumol, S. Merel, B. Clarke and S. Snyder, Chem. Cent. J, 2013, 7, 104 CrossRef PubMed.
- Y. Yu, L. Wu and A. C. Chang, Sci. Total Environ., 2013, 442, 310–316 CrossRef CAS PubMed.
- E. F. Houtz, C. P. Higgins, J. A. Field and D. L. Sedlak, Environ. Sci. Technol., 2013, 47, 8187–8195 CAS.
- F. Xiao, T. R. Halbach, M. F. Simcik and J. S. Gulliver, Water Res., 2012, 46, 3101–3109 CrossRef CAS PubMed.
- P. M. Thomas and G. D. Foster, Environ. Toxicol. Chem., 2005, 24, 25–30 CrossRef CAS.
- M. Alidina, D. Li, M. Ouf and J. E. Drewes, J. Environ. Manage., 2014, 144, 58–66 CrossRef CAS PubMed.
- A. L. Batt, S. Kim and D. S. Aga, Chemosphere, 2007, 68, 428–435 CrossRef CAS PubMed.
- L. J. Fono, E. P. Kolodziej and D. L. Sedlak, Environ. Sci. Technol., 2006, 40, 7257–7262 CrossRef CAS.
- C. J. Lee and T. J. Rasmussen, Sci. Total Environ., 2006, 371, 258–269 CrossRef CAS PubMed.
- G. A. Loraine and M. E. Pettigrove, Environ. Sci. Technol., 2006, 40, 687–695 CrossRef CAS.
- J. T. Yu, E. J. Bouwer and M. Coelhan, Agric. Water Management, 2006, 86, 72–80 CrossRef PubMed.
- A. L. Batt, S. Kim and D. S. Aga, Environ. Sci. Technol., 2006, 40, 7367–7373 CrossRef CAS.
- B. G. Loganathan, K. S. Sajwan, E. Sinclair, K. S. Kumar and K. Kannan, Water Res., 2007, 41, 4611–4620 CrossRef CAS PubMed.
- C. Y. Hao, X. M. Zhao, S. Tabe and P. Yang, Environ. Sci. Technol., 2008, 42, 4068–4075 CrossRef CAS.
- J. Jackson and R. Sutton, Sci. Total Environ., 2008, 405, 153–160 CrossRef CAS PubMed.
- P. M. Palmer, L. R. Wilson, P. O'Keefe, R. Sheridan, T. King and C. Y. Chen, Sci. Total Environ., 2008, 394, 90–102 CrossRef CAS PubMed.
- A. L. Spongberg and J. D. Witter, Sci. Total Environ., 2008, 397, 148–157 CrossRef CAS PubMed.
- R. A. Trenholm, B. J. Vanderford, J. E. Drewes and S. A. Snyder, J. Chromatogr. A, 2008, 1190, 253–262 CrossRef CAS PubMed.
- J. Heidler and R. U. Halden, J. Environ. Monit., 2009, 11, 2207–2215 RSC.
- R. A. Trenholm, B. J. Vanderford and S. A. Snyder, Talanta, 2009, 79, 1425–1432 CrossRef CAS PubMed.
- I. Ferrer and E. M. Thurman, J. Chromatogr. A, 2010, 1217, 4127–4134 CrossRef CAS PubMed.
- E. C. Wert, F. L. Rosario-Ortiz and S. A. Snyder, Water Res., 2009, 43, 1005–1014 CrossRef CAS PubMed.
- T. Rauch-Williams, C. Hoppe-Jones and J. E. Drewes, Water Res., 2010, 44, 449–460 CrossRef CAS PubMed.
- D. B. Mawhinney, R. B. Young, B. J. Vanderford, T. Borch and S. A. Snyder, Environ. Sci. Technol., 2011, 45, 8716–8722 CrossRef CAS PubMed.
- E. D. Nelson, H. Do, R. S. Lewis and S. A. Carr, Environ. Sci. Technol., 2011, 45, 1228–1234 CrossRef CAS PubMed.
- Y. Sapozhnikova, M. Hedgespeth, E. Wirth and M. Fulton, Anal. Methods, 2011, 3, 1079–1086 RSC.
- X. Yang, R. C. Flowers, H. S. Weinberg and P. C. Singer, Water Res., 2011, 45, 5218–5228 CrossRef CAS PubMed.
- P. Gao, Y. Ding, H. Li and I. Xagoraraki, Chemosphere, 2012, 88, 17–24 CrossRef CAS PubMed.
- S. Carbonaro, M. N. Sugihara and T. J. Strathmann, Appl. Catal., B, 2013, 129, 1–12 CrossRef CAS PubMed.
- Y. Yu, L. S. Wu and A. C. Chang, Sci. Total Environ., 2013, 442, 310–316 CrossRef CAS PubMed.
- B. W. Du, A. E. Price, W. C. Scott, L. A. Kristofco, A. J. Ramirez, C. K. Chambliss, J. C. Yelderman and B. W. Brooks, Sci. Total Environ., 2014, 466, 976–984 CrossRef PubMed.
- P. A. Lara-Martin, E. Gonzalez-Mazo, M. Petrovic, D. Barcelo and B. J. Brownawell, Mar. Pollut. Bull., 2014, 85, 710–719 CrossRef CAS PubMed.
- Y. Lester, I. Ferrer, E. M. Thurman and K. G. Linden, J. Hazard. Mater., 2014, 280, 104–110 CrossRef CAS PubMed.
- T. A. Minarik, J. A. Vick, M. M. Schultz, S. E. Bartell, D. Martinovic-Weigelt, D. C. Rearick and H. L. Schoenfuss, J. Am. Water Resour. Assoc., 2014, 50, 358–375 CrossRef CAS PubMed.
- T. Anumol and S. A. Snyder, Talanta, 2015, 132, 77–86 CrossRef CAS PubMed.
- E. D. Schreder and M. J. La Guardia, Environ. Sci. Technol., 2014, 48, 11575–11583 CrossRef CAS PubMed.
- A. Sengupta, J. M. Lyons, D. J. Smith, J. E. Drewes, S. A. Snyder, A. Heil and K. A. Maruya, Environ. Toxicol. Chem., 2014, 33, 350–358 CrossRef CAS PubMed.
- B. Subedi and K. Kannan, Environ. Sci. Technol., 2014, 48, 13668–13674 CrossRef CAS PubMed.
- J. A. Glaser, D. L. Foerst, G. D. McKee, S. A. Quave and W. L. Budde, Environ. Sci. Technol., 1981, 15, 1426–1435 CrossRef CAS.
- W. Seitz, W. Schulz and W. H. Weber, Rapid Commun. Mass Spectrom., 2006, 20, 2281–2285 CrossRef CAS PubMed.
- F. Gosetti, E. Mazzucco, D. Zampieri and M. C. Gennaro, J. Chromatogr. A, 2010, 1217, 3929–3937 CrossRef CAS PubMed.
- F. Busetti, K. L. Linge, J. W. Blythe and A. Heitz, J. Chromatogr. A, 2008, 1213, 200–208 CrossRef CAS PubMed.
- C. Lacey, G. McMahon, J. Bones, L. Barron, A. Morrissey and J. M. Tobin, Talanta, 2008, 75, 1089–1097 CrossRef CAS PubMed.
- M. G. Kokotou and N. S. Thomaidis, Anal. Methods, 2013, 5, 3825–3833 RSC.
- N. Cimetiere, I. Soutrel, M. Lemasle, A. Laplanche and A. Crocq, Environ. Technol., 2013, 34, 3043–3053 CrossRef PubMed.
- S. Wickramasekara, S. Hernandez-Ruiz, L. Abrell, R. Arnold and J. Chorover, Anal. Chim. Acta, 2012, 717, 77–84 CrossRef CAS PubMed.
- A. Rodayan, R. Roy and V. Yargeau, J. Hazard. Mater., 2010, 177, 237–243 CrossRef CAS PubMed.
- I. A. Katsoyiannis, S. Canonica and U. von Gunten, Water Res., 2011, 45, 3811–3822 CrossRef CAS PubMed.
- E. M. Rodríguez, G. Márquez, E. A. León, P. M. Álvarez, A. M. Amat and F. J. Beltrán, J. Environ. Manage., 2013, 127, 114–124 CrossRef PubMed.
- F. J. Beltran, A. Aguinaco, J. F. Garcia-Araya and A. L. Oropesa, Water Res., 2008, 42, 3799–3808 CrossRef CAS PubMed.
- F. H. Messerli and S. Bangalore, Am. J. Med., 2011, 124, 896–899 CrossRef CAS PubMed.
- M. L. Richardson and J. M. Bowron, J. Pharm. Pharmacol., 1985, 37, 1–12 CrossRef CAS PubMed.
- D. Gerrity, S. Gamage, D. Jones, G. V. Korshin, Y. Lee, A. Pisarenko, R. A. Trenholm, U. von Gunten, E. C. Wert and S. A. Snyder, Water Res., 2012, 46, 6257–6272 CrossRef CAS PubMed.
- C. J. Corwin and R. S. Summers, J. - Am. Water Works Assoc., 2012, 104, 43–44 CrossRef CAS.
- T. Anumol, M. Sgroi, M. Park, P. Roccaro and S. A. Snyder, Water Res., 2015, 76, 76–87 CrossRef CAS PubMed.
- M. Alidina, C. Hoppe-Jones, M. Yoon, A. F. Hamadeh, D. Li and J. E. Drewes, Sci. Total Environ., 2014, 478, 152–162 CrossRef CAS PubMed.
- J. Meyer and K. Bester, J. Environ. Monit., 2004, 6, 599–605 RSC.
- J. P. Pocostales, M. M. Sein, W. Knolle, C. von Sonntag and T. C. Schmidt, Environ. Sci. Technol., 2010, 44, 8248–8253 CrossRef CAS PubMed.
- J. L. Kormos, M. Schulz and T. A. Ternes, Environ. Sci. Technol., 2011, 45, 8723–8732 CrossRef CAS PubMed.
- S. Merel, A. I. Nikiforov and S. A. Snyder, Chemosphere, 2015, 127, 238–245 CrossRef CAS PubMed.
Footnote |
† Electronic supplementary information (ESI) available. See DOI: 10.1039/c5ew00080g |
|
This journal is © The Royal Society of Chemistry 2015 |
Click here to see how this site uses Cookies. View our privacy policy here.