DOI:
10.1039/C9LC00014C
(Paper)
Lab Chip, 2019,
19, 1610-1620
Simplified Drop-seq workflow with minimized bead loss using a bead capture and processing microfluidic chip†
Received
4th January 2019
, Accepted 9th February 2019
First published on 28th March 2019
Abstract
Single-cell RNA-sequencing (scRNA-seq) has revolutionized biomedical research by enabling the in-depth analysis of cell-to-cell heterogeneity of tissues with unprecedented resolution. One of the catalyzing technologies is single cell droplet microfluidics, which has massively increased the overall cell throughput, routinely allowing the analysis of thousands of cells per experiment at a relatively low cost. Among several existing droplet-based approaches, the Drop-seq platform has emerged as one of the most widely used systems. Yet, this has surprisingly not incentivized major refinements of the method, thus restricting any lab implementation to the original Drop-seq setup, which is known to suffer from up to 80% bead loss during the process. In this study, we present a systematic re-engineering and optimization of Drop-seq: first, we re-designed the original dropleting device to be compatible with both air-pressure systems and syringe pumps, thus increasing the overall flexibility of the platform. Second, we devised an accompanying chip for post-encapsulation bead processing, which simplifies and massively increases Drop-seq's cell processing efficiency. Taken together, the presented optimization efforts result in a more flexible and efficient Drop-seq version.
Introduction
The generation of high-dimensional gene expression data through single-cell RNA-sequencing (scRNA-seq) is becoming indispensable to explore the diversity of heterogeneous cell populations with the necessary accuracy and resolution.1 For several of the catalyzing methodologies, microfluidics has quickly become a key investigative technique for cell capture, isolation and processing. The first, widely successful, microfluidic implementation of scRNA-seq was introduced and commercialized by Fluidigm, with its C1 system, which employed hydrodynamic traps for single cell capture and microchambers for downstream library preparation. While this approach allowed for convenient sample processing, costs per cell remained high, the throughput limited and doublets – i.e. the unwanted capture of two cells instead of one – was unexpectedly frequent.2
With the emergence and maturation of droplet microfluidics in recent years, researchers have addressed the need for high throughput, low-cost assays by reducing reaction volumes.3 Droplet microfluidics now allows for experiments on massive scales both within and outside of the “-omics” domain.4,5 Focusing on single cell transcriptomics, the possibility to perform rapid compartmentalization has significantly increased the throughput and sensitivity of analyses at the single cell level. For instance, recent publications contained 100
605 “Tabula muris” cells,6 492
949 mouse nervous system cells,7 157
000 ageing fly brain cells,8 and 10× genomics has publicly available datasets with 1.3 M cells in total.9 While commercial systems such as the 10× genomics chromium exist, offering an indisputable ease of use and experimental robustness, customized lab setups are still a magnitude less expensive in terms of per-cell library preparation costs once established and routinely utilized.10 Furthermore, custom-built setups offer the highest degree of flexibility, allowing for freely scalable experimental size and completely open source processing chemistry. Hence, while commercial systems are most arguably dominating the conventional cell profiling market, it is likely that, especially for power-users and research and development, custom-built setups will continue to play an important role.11–14
The two most noteworthy custom-built dropleting systems for scRNA-seq are Drop-seq2 and inDrop.15 Conceptually, both systems are similar: a cell suspension is co-flown with a bead suspension, and encapsulated in nanoliter droplets. The beads harbor barcoded oligonucleotides for capturing polyadenylated RNA (polyA) and for adding a common molecular cell identifier to each mRNA transcript. In the bead suspension, detergent is added to lyse cells upon co-encapsulation and thus to liberate cellular polyA RNAs for subsequent capture. Beads for both systems, hydrogels for inDrops and microspheres for Drop-seq, are commercially available. Alternatively, inDrop beads can be produced in-house by split-and-pool synthesis,16 while the Drop-seq microspheres are more complex to manufacture since the process involves solid-phase oligonucleotide synthesis. Noteworthy, split-and-pool barcode synthesis has recently been demonstrated on solid-state microspheres,17 potentially making this an alternative route for Drop-seq bead generation. Despite having lower detection sensitivity based on artificial RNA molecules,11 and significantly lower cell capture efficiencies, Drop-seq still has found wide adoption in the scRNA-seq community.18–22 This is because the utilization of solid-state (toyopearl) microspheres in Drop-seq, as compared to hydrogels in inDrops, makes Drop-seq relatively straightforward to implement. An additional technical advantage of using microspheres is that they can be directly coated by solid-phase synthesis, resulting in a high cell barcode diversity. Furthermore, the conceptually simple post-encapsulation reverse-transcription (RT) process makes the protocol comparably easy to establish: the RNA-harboring spheres can be retrieved from the emulsion, mRNA reverse-transcribed in a reaction vessel, and unhybridized bead-bound oligos removed by exonuclease I (Exo I) treatment.
However, despite its wide adoption, few improvements, especially for practical use of the system, have so far been described.21,23,24 Thus, if a lab aims to establish Drop-seq today, there is no alternative besides replicating the previously published approach, regardless of the equipment available in the lab or experimental needs. This implicitly forces for example air pressure-based microfluidics lab to purchase syringe pumps and a specialized magnetic stirring system. To this day, only Stephenson and colleagues12 have built a low-cost, 3D printed pressure-based Drop-seq setup with instructions on how to specifically adapt the chip and operating equipment. Moreover, some experimental procedures, such as the post-encapsulation processing of the mRNA-bearing microspheres to form STAMPs (for Single-cell Transcriptomes Attached To MicroParticles), are still rudimentary: microspheres are handled in large vessels requiring a plethora of manual processing steps. While this not only introduces many error prone steps, up to 80% of all processed beads are lost during this process.25 To our knowledge, no improvements have been made to the original bead processing protocol since its release.
To provide the community with a universally implementable system, we re-engineered the Drop-seq system and addressed one of its major limitations: bead loss. First, we show that our newly designed encapsulation chip runs robustly both on air-pressure and syringe pumps, thus providing greater experimental flexibility. Second, we show that the use of an air pressure source compared to syringe pumps allows for the integration of simple sample supply reservoirs, which alleviate the necessity of acquiring expensive micro stirrer setups. Third, we devised a novel capture and processing chip that allows for highly efficient retrieval of the mRNA capture beads. We demonstrate that this approach increases recovery efficiencies of microspheres, either from pre-broken emulsions, or directly from droplets. Using the same device, we show that the complete cDNA generation protocol can be executed on-chip, further streamlining the protocol. Finally, we confirmed that key scRNA-seq performance metrics are comparable to the original implementation of the protocol. Overall, our solution represents in our opinion a more versatile and robust approach compared to the original Drop-seq implementation.
Material and methods
Microfluidic chip design and flow simulations
Chips were designed using Tanner L-Edit CAD software version v 2016.2 (Mentor). Flow simulations were carried out using COMSOL multiphysics version 5.2. Segments were extruded to the same height as the actual device (80 μm), flow conditions were set to “creeping flow”, incompressible flow, water density with no-slip boundary conditions. The inlet and outlet were separated by a pressure gradient of 5 PSI. The chosen mesh type was free triangular with size option set to normal. The velocity profile of the slice running at half channel height was plotted.
Soft-lithography and microfluidic device fabrication
6-Inch chromium masks were exposed in a VPG200 laser writer (Heidelberg instruments) using a 20 mm laser writing head. Masks were developed using an HMR 900 mask processor (Hamatech). 60 μm (e-chip) or 80 μm (cp-chip) thick SU8 photoresist layers were deposited with an LSM-200 spin coater (Sawatec) and then exposed on a MJB4 single side mask aligner (SussMicroTec) and manually developed. The SU8 processing steps were done according to manufacturer's instructions for the 3050 series (Y311075 0500L 1GL, Microchem). Developed wafers were used as a mold for PDMS chips after passivation (10
:
1 ratio PDMS
:
curing agent). PDMS and curing agent were mixed, degassed, and poured on the mold. The PDMS mixture was cured for 1 hour at 80 °C. Next, inlet holes were punched and the chip surface activated with oxygen plasma (45 s at ∼500 mTorr). The chips were bonded on surface activated glass slides and incubated at 80 °C for at least 2 hours. Before use, the chips (e-chip) were surface-treated with 2% Trichloro (1H,1H,2H,2H-perfluorooctyl) silane (448931-10G, Sigma-Aldrich) dissolved in 3M HFE 7500 (297730-93-9) for 5 min.
Procedures for microfluidic device handling
The MFCS-EZ (2 × 345 mbar, 1 × 1045 mbar outlet version) air-pressure driver (Fluigent) was connected to the sample vessels (CG-4909-04, Chemglass) using silicone tubing (1175-8705, Fisher Scientific) and blunt-end needles (23G). Vessels were connected to the microfluidic chip using Tygon tubing (06420-02, Cole-Parmer) as shown in Fig. 2e. The vessels were loaded with cells, beads and EvaGreen droplet generation oil (186-4006, Bio-rad). To maintain constant bead mixing, the plastic adaptor for a vortex shaker (Fig. 2d) was designed in Fusion 360 (Autodesk) and 3D printed in ABS using a M200 3D-printer (Zortrax). Pressure for the bead and cell vessels was set to 345 mbar and for oil phase pressure to 900 mbar. The tubing was directly connected to the microfluidic chip placed on an inverted microscope. The vessels were pressurized in the following order: 1. cell suspension, 2. bead suspension, and 3. oil phase. After droplet formation stabilized and residual polydisperse emulsions exited the collection tubing, droplets were collected in a 50 mL tube. Droplet formation was monitored using a HotShot 1280CC (NAC) high-speed camera.
On-chip droplet breakage and bead recovery strategy
After droplet collection, droplet and oil phase were loaded into a 200 μL pipette tip (2239915, Bio-Rad) and directly injected into the cp-chip. Once beads were recovered on the pillars, washing was performed twice with 6× SSC buffer. To elute the beads from the chip, Tygon tubing was connected to the inlet, the end placed into a microcentrifuge tube, and 6× SSC injected into the outlet.
Off-chip droplet breakage and bead recovery strategy
After droplet collection, the oil phase was removed from the tube and 30 mL 6× SSC buffer was added to the emulsion in the 50 mL tube. Next, 0.5 mL 1H,1H,2H,2H-Perfluoro-1-octanol (370533-5G, Sigma-Aldrich) was added, and the tube was agitated by vigorous shaking. Following this, two washing steps were performed with 6× SSC at 1000×g by lowering the brake by 50% without disturbing the bead interphase. Subsequently, as much 6× SSC as possible was removed without disturbing the beads, leaving only 5 mL of oil–water phase. Both 6× SSC and perfluoro-1-octanol phases were transferred to the bead recovery chip, as described above. After bead capture, the beads were washed two times with 6× SSC to remove any residual oil and perfluoro-1-octanol. Beads were recovered in a microcentrifuge tube by injection of 6× SSC buffer into the chip outlet (as described above).
Original Drop-seq droplet breakage and bead recovery strategy
Bead recovery from droplets was performed as described in the latest published online version of the protocol.25 For experimental results shown in Fig. S1,† an additional washing step was included using 6× SSC prior to pelleting the sample in Falcon tubes followed by the transfer into Eppendorf tubes.
Bead quantification
To evaluate bead recovery strategies, bead numbers were quantified prior to the experiment, and after all processing steps. The bead starting amount was enumerated by scanning the whole sample on a V600 scanner (Epson). Specifically, all beads were pipetted into a 48 well plate lid, placed on the scanner. After a ∼3 min sedimentation period, the lid was scanned at 4800 dpi resolution in transparency mode. Next, the beads were carefully retrieved from the plate lid and placed back into a microcentrifuge tube. An additional scan of the empty lid was used to quantify the remaining beads, which were subtracted from the starting amount. After completion of the experiment, quantification of the remaining beads was executed as above. The ‘sampling loss’ amounted on average to 3% of the processed beads, for each manual transfer of bead solutions between enumeration plate and experimental device.
The obtained images were quantified using Fiji (ImageJ version 1.50g) by thresholding, watershed separation, and particle detection based on a size and circularity threshold. Systematic errors of the quantification (e.g. closely touching beads) were manually corrected in all images.
Cell handling
For species mixing experiments, HEK-293T (ATCC Cat. No. SD-3515) and murine brown preadipocyte (provided by Prof Christian Wolfrum's laboratory, ETH Zürich)26 cell lines were used. Cells were cultured up to 90% confluency in Glutamax DMEM (61965026, Thermo Fisher Scientific) supplemented with 10% FBS (10270-106, Thermo Fisher Scientific) and Pen-Strep (15140-122, Thermo Fisher Scientific). Following this, cells were dissociated using Trypsin-EDTA (59418C, Sigma-Aldrich), washed once with PBS (14040091, Thermo Fisher Scientific) and counted using Trypan-blue live-dead stain (T10282, Thermo Fisher Scientific) using a Countess (Invitrogen) cell counter. Cells were finally re-suspended in PBS supplemented with 0.01% BSA and murine RNAse inhibitor (M0314S, NEB), and mixed in a 1
:
1 ratio to an adjusted concentration of 100 cells per μL.
qPCR assay for performance test of optimized purification strategies
Total RNA from D. melanogaster tissue and HEK-293T (human) cell line was isolated using the Direct-zol RNA miniprep kit (R2056, Zymo Research). Beads were incubated with the extracted human RNA at 110 ng μL−1 for 10 min at room temperature with mixing. Unbound RNA was washed away and beads with bound RNA were encapsulated in droplets. Following this, droplets containing free-floating D. melanogaster RNA at 110 ng μL−1 concentration were collected. Both groups of droplets were mixed in a 1
:
1 ratio and after that, droplet breakage and bead recovery for reverse transcription were performed. Once STAMPS were generated, cDNA was amplified and purified from sub-sampled 800 beads following the original protocol. The purified cDNA was used for qPCR analysis of species cross-contamination. 0.75 ng of cDNA was amplified in PowerUp SYBR Green MM (Thermo Fisher Scientific) containing 200 nM of either human specific GAPDH primer mix (forward: acccactcctccacctttgac, reverse: tgttgctgtagccaaattcgtt) or D. melanogaster specific Rp49 primer mix (forward: gacgcttcaagggacagtatctg, rev: aaacgcggttctgcatgag). Amplification was done in technical triplicates on a StepOnePlus RT-PCR System (Applied Biosystems). As a control, all three droplet breakage strategies were compared to beads bound to HEK-293T RNA only, D. melanogaster RNA only and combined HEK-293T - D. melanogaster (1
:
1 ratio) RNA to ensure primer specificity. Ct values were thresholded at 0.1 RFU and the relative ratio between HEK-293T and contaminating D. melanogaster cDNA was used to assess the cross-contamination/background of each purification protocol.
Library preparation and sequencing
Following droplet breakage and bead (SeqB lot 120817, ChemGenes) recovery, reverse transcription (RT), exonuclease I (ExoI) treatment and PCR were performed as described in the original protocol.25 Libraries were purified using Ampure XP beads (0.6× ratio to remove small fragments), cDNA was quantified using a Qubit HS kit (Thermo Fisher Scientific) and integrity analyzed on a Fragment Analyzer (Agilent). Libraries were prepared using in-house produced Tn5 loaded with adapters, as described.27 Size selected and purified libraries were sequenced paired-end on a NextSeq 500 system (Illumina) in High-Output mode following recommendations from the original protocol (read 1 20 bp and read 2 50 bp).
STAMP generation on chip
Beads were recovered from broken emulsions on-chip without any additional incubation times. Subsequently, RT, ExoI treatment and all washing steps were performed on-chip. To compensate for liquid evaporation from the PDMS device during RT and ExoI treatments, the reaction mix was injected every 15 min into the device allowing complete bead immersion. The microfluidic chip containing the beads was incubated at temperatures and durations as indicated in the original protocol25 using a flat thermoblock. After the last washing steps, beads were eluted in an Eppendorf tube for counting and PCR amplification. Library preparation was performed as described above.
Data analysis
The data analysis was performed using the Drop-seq tools package25 on the Vital-IT HPC platform. After trimming and sequence tagging, reads were aligned to the mixed human
:
mouse reference genome (hg38 and mm10) using STAR (version 2.5.3.a).28 Following the alignment, the gene annotation was added, bead synthesis errors were corrected and cell barcodes extracted. Subsequently, the BAM files containing the processed data were filtered, split into mouse and human annotated BAM files, and digital gene expression matrices were generated for each species. Preliminary data-analysis was done in ASAP.29 Downstream data analysis was done using R (version 3.5.1), plots generated using the R package ggplot2 (version 3.0.0).
Results and discussion
The revised Drop-seq workflow comprises two microfluidic devices, an encapsulation device (e-chip) and a bead capture and processing chip (cp-chip). The e-chip facilitates the cell bead encapsulation process and is compatible with pressure and syringe pumps (Fig. 1a), whereas the cp-chip is employed for efficient bead extraction, either directly from droplets for small samples, or from broken emulsions for larger samples (Fig. 1b). While it is then possible to proceed to cDNA generation in tubes after bead capture on the cp-chip by eluting the microspheres, it is also possible to generate STAMPs on chip (Fig. 1c). The latter has the advantage of both minimizing bead loss and handling errors as well as offering the potential for automating this otherwise error-prone and labor-intensive procedure.
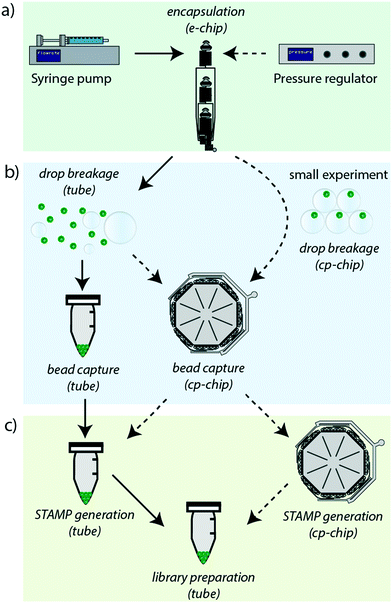 |
| Fig. 1 Overview of the revised Drop-seq workflow: the revised work-flow (dashed lines) is a flexible protocol that simplifies handling and increases the efficiency of the original protocol (solid lines). a) By redesigning the original chip into the e-chip, it is now possible to use either syringe pumps or an air-pressure regulator for the cell-bead encapsulation process. b) Utilizing a new bead capture and processing chip, the cp-chip, beads can now be either captured post droplet breakage, or directly captured from droplets for smaller bead quantities. c) Following the bead capture, it is possible to either retrieve the beads into a tube for the STAMP generation process (reverse transcription and exonuclease treatment), or to perform STAMP generation on-chip to further improve the overall bead recovery efficiency. | |
Design of a versatile cell-bead encapsulation device
To make the workflow compatible with different pressure sources, a new encapsulation chip (e-chip) was designed to facilitate monodisperse emulsion formation with both air-pressure and syringe drivers (Fig. 2a). Syringe pumps are fundamentally different from air-pressure systems as they are controlling volumetric flow rates. In contrast, air pressure systems only control the pressure of a liquid containing vessel. As all of these systems feature minimal operating pressures, the flow-resistance of the chip had to be adjusted to allow for appropriate flow rates. Thus, we first re-designed the original chip by adding microfluidic resistors to each channel, aiming to stabilize the flow rate in the operating range of the air pressure controller. These resistors were designed by considering the following criteria: a) The channel cross-section had to be sufficiently large to allow the easy passage of cells and beads and thus to avoid clogging. b) The resulting flow rate should not exceed the one used in the original Drop-seq (i.e. below 60 μl min−1). These criteria translated into 4.5 cm-long resistors with a squared cross-section with lengths of 60 μm. As the smaller channel width compared to the original device made the chip more sensitive to clogging, the second addition was large input filters to retain abnormally big microspheres and dust from entering the chip. Third, the dropleting T-junction was adapted into an angled conformation. We compared the performance of the newly developed e-chip to the original Drop-seq device by generating emulsions with both syringe pumps and pressure controllers (Fig. 2b). While the original device clearly failed to produce monodisperse emulsions from a pressure controller, the e-chip was able to produce stable emulsions with both drivers. Importantly and independent of the driver system, we also observed that the length of the chaotic advection mixers on the original chip was insufficient to completely mix droplet contents (Fig. 2c). Since passive mixing in droplets by diffusion is slow and uncontrollable, we decided to increase the length of the mixers on the e-chip four-fold. Indeed, a qualitative inspection confirmed that the larger mixing channels enhanced the internal mixing of the microdroplets (Fig. 2c).
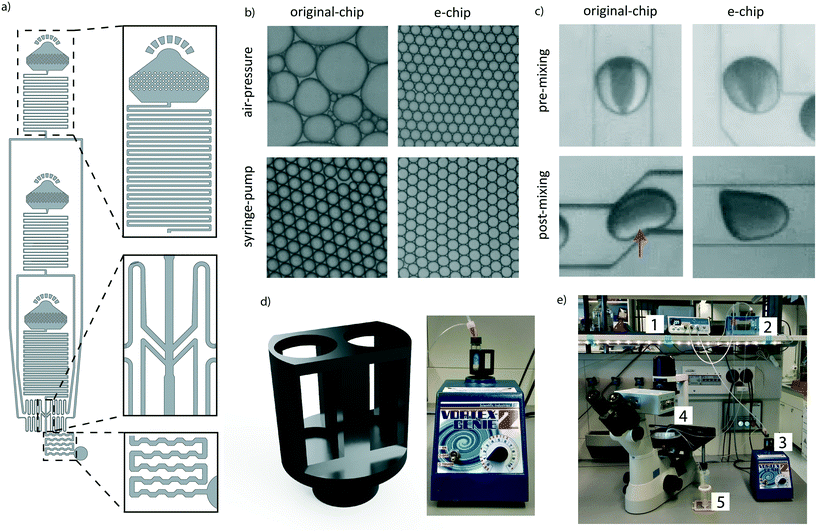 |
| Fig. 2 The e-chip simplifies the experimental setup and renders it more flexible: a) the e-chip includes flow resistors, an optimized flow focusing point, and extended droplet mixing channels (top to bottom). b) Emulsion formation by the original device and e-chip using syringe pumps and an air-pressure source. c) Droplets filled with transparent liquid and food-coloring after droplet formation (pre-mixing), and after exiting the droplet mixing channel (post-mixing). d) Two-slot sample holder for bead suspension, attachable to a standard lab vortex machine. e) Overall setup utilizing air-pressure as driver. An air pressure controller (1) is connected to the oil and cell reservoirs (2), and to the bead vessel (3). All vessels are connected to the dropleting device (4) and the emulsion is captured in a tube (5). | |
A positive side effect of using air pressure systems is the fact that conventional sample vessels can be utilized and interfaced with the chip, as opposed to syringes used in syringe pumps. This is especially significant for solutions containing microbeads, as they have to be agitated for the particles to remain in suspension. In the original Drop-seq implementation, this was achieved by an expensive magnetic stirrer setup (∼$2000), connected in proximity of the syringes. To overcome the need for a dedicated magnetic stirrer, we developed a vessel agitation system that is compatible with conventional laboratory equipment. Using additive manufacturing, we produced a Vortex adapter that can hold and agitate the cell and bead suspension vessels (Fig. 2d). We extensively used the Vortex-based agitation system for cells and beads and did not observe any adverse effect on bead integrity (data not shown). Besides simplifying the overall system (Fig. 2e), this has the additional advantage of reducing the initial set-up costs.
Highly efficient bead retrieval using the cp-chip
Following the cell encapsulation process, the original Drop-seq protocol involves a multistep procedure to recover the microspheres from the emulsion for subsequent STAMP generation. As these processing steps are conducted in vessels and equipment tailored to large volumes, they are naturally prone to bead loss. In the original protocol, this loss was quantified as ranging between 60–80%.25 To identify critical steps during bead processing, we examined the bead recovery efficiency of the workflow by quantifying bead loss for each of the three main protocol steps, i.e.: encapsulation, de-emulsification, and STAMP generation (Fig. 3a, details in the Methods section). The average number of input beads for efficiency measurements were 2249 ± 250 beads. As expected, we found that every manual handling step introduces bead loss (Fig. 3a). Nevertheless, we observed that the steps executed on a microfluidic chip (encapsulation) and in small vessels (STAMP generation) show a much better performance (∼10–20% loss) than the de-emulsification step performed in large vessels (∼58% loss). Overall, we observed cumulative post-encapsulation bead losses of up to ∼64%, which is in accordance with the reported efficiency of the most recent protocol.
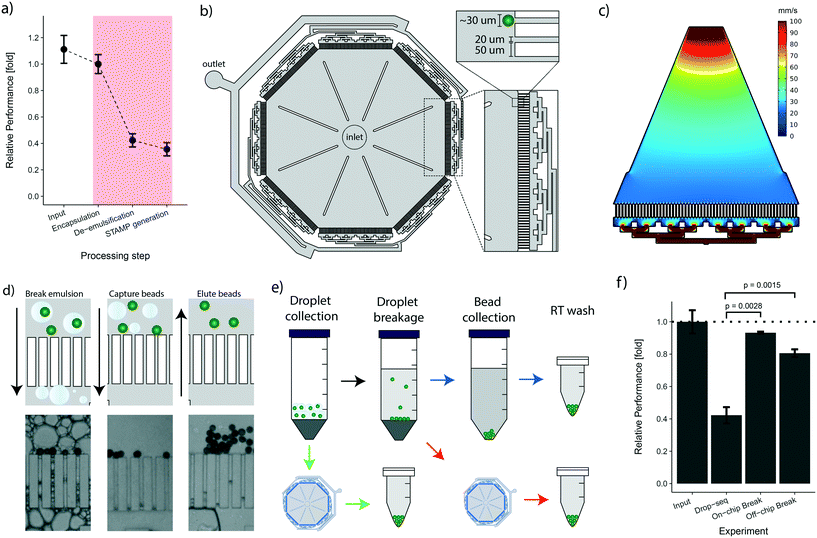 |
| Fig. 3 The novel bead capture and processing cp-chip increases the bead recovery efficiency: a) efficiency of the various bead processing steps for the original drop-seq protocol. Input amount is displayed relative to the encapsulation process. The efficiency of the de-emulsification step is normalized to the encapsulation step. The bead recovery efficiency for the STAMP generation process is shown relative to the de-emulsification process and normalized for ‘sampling loss’. Error bars represent the standard deviation calculated on the normalized values for each processing step. b) Design of the novel bead capture and processing cp-chip. c) Hydrodynamic simulation of one chip section of the chip shown in (b). d) The device from (b) is capable of capturing beads directly from droplets (left) or from a broken emulsion (middle). Captured beads are eluted by reversing flow direction (right). e) Different strategies for bead recovery from droplets: original protocol (blue arrows), collection on-chip from broken emulsion (red arrows), and bead capture from droplets (green arrows). f) Bead recovery efficiencies for the different procedures shown in (e). Values are normalized to encapsulation losses, the error is shown as standard deviation calculated on the normalized values. P-values were determined using t-test. | |
To address the post-enapsulation inefficiencies that cause substantial bead loss, we designed a bead capture and processing chip (cp-chip) that simplifies the de-emulsification process (Fig. 3b). Specifically, the cp-chip is comprised of pillar arrays that allow liquids to pass through while blocking particles above a certain size. Gaps between the pillar were 20 μm, substantially below the average size of the coated oligonucleotide beads (30 μm). The pillars were 50 μm wide, were arranged in eight linear sections of 4.1 mm each, and were combined in an octagonal shape to maximize the capture capacity of the device (Fig. 3b). In principle, the cp-chip can hold more than 1000 beads in a single bead layer, and it can easily purify large Drop-seq samples of more than 10
000 beads in multi-sediment layers. To seed the array evenly across all sections, the channels towards the waste channel were arranged so that flow spreads across all pillars, confirmed with a flow simulation (Fig. 3c). Finally, large support structures were included to prevent collapse of the chip ceiling and to provide a guide for precise hole punching.
Initially, we tested the cp-chip qualitatively to demonstrate that the device was indeed capable of extracting beads from complex solutions. Intuitively, direct on-chip bead capture from emulsions represents the most straightforward approach as it involves no prior processing steps. However, since droplets that contain contaminating unbound mRNA are in direct proximity of beads coated with mRNA-capturing probes, this approach can potentially lead to increased cross-contamination of sequencing libraries. It was therefore important to validate that the cp-chip can capture beads that stem either directly from droplets or from the broken emulsion (Fig. 3d). Next, we further examined both workflows (i.e. beads from droplets vs. broken emulsions) (Fig. 3e) to determine their performance in terms of bead recovery efficiency compared to the original Drop-seq approach. To this end, beads were encapsulated in emulsions and recovered using each of the three different procedures. We found that de-emulsification using the cp-chip increased the recovery efficiency approximately two-fold compared to the original approach, a significant improvement (approximately 81% for bead capture from broken emulsions, and 93% for bead capture from droplets; Fig. 3f).
Bead purification performance testing of the cp-chip
As the two proposed on-chip de-emulsification processes present substantial changes to the original approach, we decided to determine their impact on the molecular integrity of the samples. In particular, since wash buffers that potentially contain environmental RNAs are flown over the bead sediment layer of the cp-chip, we focused on quantifying cross-contamination of the new approach. To this end, species-mixing experiments are conventionally used. However, turnover times for high-throughput sequencing and data-analysis are still not time nor cost-effective, making species-mixing experiments impractical for protocol optimization. Hence, to conveniently measure the impact of bead capture using the cp-chip on the existing experimental setup, we developed an scRNA-seq species purity test based on quantitative PCR (Fig. 4a).
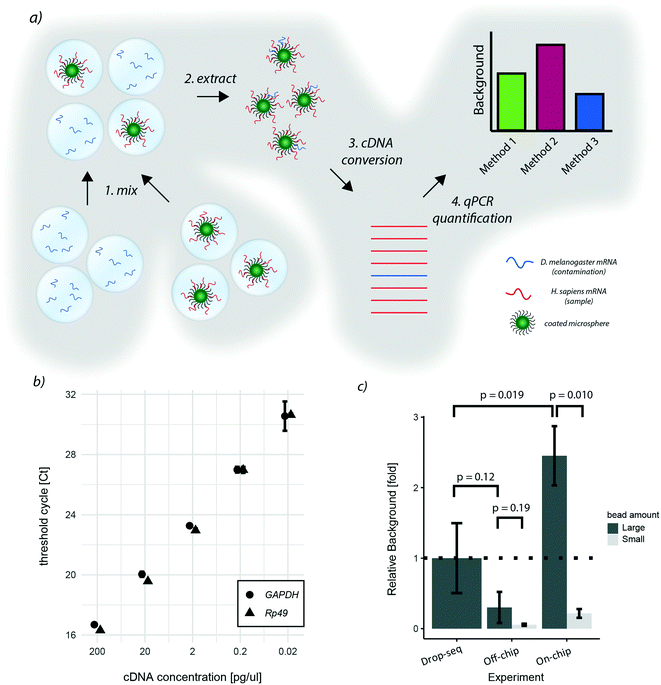 |
| Fig. 4 qPCR species-mixing quantifies cross-contamination for different de-emulsification approaches: a) qPCR approach to quantify species cross-contamination for de-emulsification strategies. Two emulsions were generated: one emulsion with droplets containing free floating Drosophila RNA, and a second emulsion containing microspheres hybridized with human RNA. Both emulsions were mixed, and beads extracted from the emulsion. Next, the captured mRNA was converted into cDNA and qPCR was performed with species-specific primers. Amplification cycles of each reaction allows for the quantification of cross-contamination. b) Threshold cycles for the qPCR primers utilized for human cDNA (GAPDH) and Drosophila cDNA (Rp49). c) Cross-contamination quantification for different de-emulsification strategies. Background values correspond to the ratio between Drosophila and human cDNA. Relative values were calculated by normalizing to the values obtained with the original protocol (Drop-seq), the error is shown as standard deviation. P-values were determined on the normalized values with a t-test. | |
Specifically, we hybridized purified human mRNA derived from HEK 293T cells on oligonucleotide capture beads, and encapsulated them into microdroplets. In parallel, we generated a microemulsion carrying free-floating Drosophila melanogaster RNA in its aqueous phase. Next, both emulsions were mixed, simulating a ‘worst-case scenario’ sample made of few droplets containing beads and a vast number of bead-less droplets containing contaminant mRNA. This way, the performance of different bead capture approaches can be easily quantified through STAMP formation followed by qPCR targeting human and Drosophila genes. The relative ratio between quantified human and Drosophila DNA thereby reflects the signal-to-noise ratio of the purification protocol. As target genes for quantification, we chose Rp49 and GAPDH, two abundant housekeeping genes of Drosophila and humans, respectively. By hybridizing equal amounts of Drosophila and human RNA to beads, we observed sufficient specificity (data not shown) and similar amplification characteristics for both target genes (Fig. 4b).
Applying this benchmarking approach to the previously developed purification procedures, we found that, in comparison to the original approach, bead capture from broken emulsions showed less cross-contamination when a large number of beads (up to 10
000 beads) was used (Fig. 4c). As it is unlikely that the microfluidic chip itself reduced cross-contamination, we assumed that one additional wash step prior to bead capture led to this result. To validate this hypothesis, we added an additional wash step to the original Drop-seq protocol, which yielded a similar decrease in background as observed for the cp-chip-based bead purification (Fig. S1†). In contrast, direct breakage of the emulsion on-chip for bead recovery significantly increased species cross-contamination by approximately 2.5-fold when a large number of beads was used (Fig. 4c). These results suggest that direct emulsion breakage is to be avoided when handling a large number of beads, but could still be useful for small samples that are challenging to process.
We hypothesized that as soon as beads are occupying the whole pillar array, beadless contaminating droplets are forced into contact with beads leading to cross-contamination. To validate this hypothesis, we performed the same experiments with lower bead numbers (up to 1000 beads). Interestingly, we found that the background from contaminating droplets was significantly reduced for small bead numbers compared to large bead numbers for direct emulsion breakage, indicating that this approach is indeed viable for small samples (Fig. 4c).
Integrated STAMP generation on (cp-)chip
Although bead losses are not as pronounced for the STAMP generation process compared to de-emulsification (Fig. 3a), the ten manual handling steps that are required are both error-prone and labor-intensive. To address this issue, we next aimed to explore the possibility of implementing the bead processing steps until the final PCR step on the cp-chip itself, minimizing bead loss and manual work for the whole protocol. Specifically, we aimed to mimic a routine experimental size of approximately 1000 STAMPs, captured from broken emulsions. To test this approach, we first encapsulated a mix of HEK 293T cells and murine brown preadipocytes at 100 cells per μl on the e-chip, broke the emulsion in vessels and purified the beads (∼10
000) on the cp-chip. Subsequently, we performed RT and ExoI treatment directly on-chip. Finally, the beads were eluted from the cp-chip, bead-bound cDNA was PCR-amplified in a reaction tube, and the cDNA profile analyzed (Fig. 5a). As a control, an emulsion containing the same amounts of beads and cells was generated, beads captured, and STAMP generation performed in tubes according to the original Drop-seq protocol. During on-chip STAMP generation, beads were clustering loosely, yet no clumping was noted. We observed that on-chip STAMP generation yielded high-quality cDNA traces comparable to cDNA from STAMPs generated in tubes (Fig. 5b), indicating that it does not negatively impact polyA RNA integrity. The most striking difference between both traces was a strongly pronounced peak in STAMPs produced in tubes at approximately 25 bp, which most likely reflects residual primer. As subsequent bead purification will remove small fragments, this putative primer presence can be neglected.
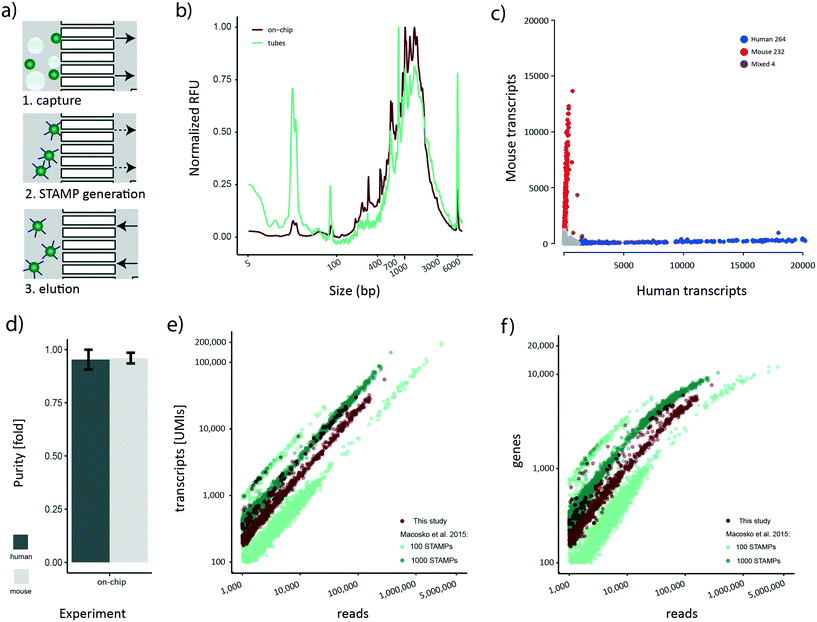 |
| Fig. 5 STAMP generation on-chip: a) for on-chip STAMP generation, beads from a broken emulsion were captured on the cp-chip (arrows indicate flow direction), and reagents for reverse transcription and exonuclease treatment injected onto the chip. After all reactions and washes, beads were eluted by reversing flow. PCR amplification was performed in a tube. b) cDNA profile obtained after STAMP generation in tubes and on-chip. Relative fluorescence units (RFUs) of each sample were normalized to the maximum value of the respective sample (1037 RFU for the on-chip sample and 122 RFU for the tube sample) in the range of 2 bp–21 kb. c) Transcript numbers per cell for mouse and human transcripts. Cells classified as belonging to one species (>90% species purity). Mouse cells are colored red, human cells blue. Purple points represent cells containing mixed transcripts (<90% species purity). d) Average single cell purity for mouse and human cells. e) Number of UMIs detected per read for HEK293T cells in the Macosko et al. 2015 100 STAMP and 1000 STAMP species mixing experiment, and for this study. f) Number of genes detected per read for HEK293T cells in the Macosko et al. 2015 100 STAMP and 1000 STAMP species mixing experiment, and for this study. | |
Sequencing-based assessment of the quality of cp-chip-derived scRNA-seq data
Our novel bead processing approach introduces a variety of changes in the overall workflow: new sample vessels, encapsulation chip, bead recovery strategy, and STAMP generation process. Potentially, this could impair the scRNA-seq data quality at multiple levels, including decreased single-cell accuracy (single-cell purity) and altered sensitivity. To address these concerns, we decided to sequence the previously prepared species-mixed library that was generated utilizing a) the e-chip, b) efficient bead recovery from a broken emulsion using the cp-chip, c) the on-chip STAMP generation protocol, and d) PCR amplification of eluted STAMPs in tubes. We approximated the number of STAMPs in this experiment to be around 500. The resulting Barnyard plot showed a clear organism-specific transcriptome separation (Fig. 5c). To benchmark the single-cell quality, we analyzed mean species purity, which we determined to be 96% for mouse cells and 95% for human cells (Fig. 5d), well within range of the original protocol at 100 cells per μl.2
Finally, we set out to determine whether the new workflow offered a similar data quality as the original published datasets.2 Specifically, we processed the 100 STAMPs and 1000 STAMPs species mixing datasets from the original publication and merged them to account for inter-experimental deviations. For downstream analysis, only HEK 293T cells were maintained since they represent the common cell line for both studies. As each dataset contains a different number of STAMPs and is sequenced to varying read depth, we compared the amount of UMIs detected to the number of sequencing reads obtained per cell (Fig. 5e). Re-assuringly, all datasets showed a comparable linear relationship between the number of UMIs detected per obtained sequencing read. This linearity suggests a similar transcript diversity across all samples, and thus a comparable sensitivity. Importantly, despite various experimental differences such as different bead-batches etc., we observed that the on-chip processed dataset clusters in-between the experimental data derived from the original data, suggesting comparable performance for both protocols. Additionally, we analyzed the gene numbers in relation to the obtained sequencing reads (Fig. 5f). Similar to the transcript counts, we observed that a comparable number of genes per read is detected in both datasets. Furthermore, gene numbers for all datasets were saturating at around 10
000 genes per cell, further confirming the comparable sensitivity among all approaches. Overall, these findings show that our developed microfluidic bead processing workflow achieves a similar experimental quality as the original protocol, but with the advantage of constituting a simpler, more flexible and more efficient approach.
Conclusions
In this study, we present the development of an optimized Drop-seq workflow that simplifies the experimental setup, enhances the overall bead recovery efficiency by more than two-fold and significantly simplifies the bead processing protocol. This workflow involves a newly developed encapsulation chip that is compatible with both syringe-pump or pressure-based driver setups. Utilizing an air pressure-based setup, the expensive magnetic stirrer was replaced by inexpensive laboratory equipment.
Consistent with previous results, we found that the original bead processing strategy, especially during de-emulsification, is prone to large sample losses. Here, we were able to overcome this limitation by developing a dedicated bead capture and processing chip, which enables high-efficiency bead capture and STAMP generation. Using this cp-chip, we explored its potential application to varying experimental situations. In the first operation mode, developed for larger samples, the device was used to capture beads from broken emulsions for efficient bead capture and convenient STAMP generation. We found that bead capture from broken emulsions on the cp-chip decreased cross-contamination compared to the original Drop-seq protocol, which we attributed to the inclusion of an additional washing step in the new protocol rather than to a chip-related property. Beyond the cp-chip-based bead processing approaches, this protocol modification has the potential to increase single-cell purity in high cell-density samples using the original protocol. For the second operation mode, we explored the possibility of directly capturing beads from droplets on-chip, which we found to be the most efficient strategy for de-emulsification. For this approach, we observed a cross-contamination increase of 2.5-fold when introducing a large number of beads. We hypothesized that full bead occupation of the cp-chip pillar array forced beads in contact with contaminating droplets. Indeed, we found that direct emulsion breakage on-chip using smaller bead numbers showed cross-contamination levels below the ones observed for the original Drop-seq protocol, thus representing a viable processing strategy for rare or low-input samples. Finally, we showed that STAMP generation can be efficiently performed on-chip, eliminating all manual handling steps post-bead capture. Complementary to this approach, we established an inexpensive and rapid qPCR method to explore suitable ways to integrate the bead processing approach in an existing experimental workflow. Finally, we showed that our new workflow features a comparable single-cell purity and sensitivity to the original publication.
Globally, our new workflow adds important features for routine experimentation, especially on medium to small samples. Furthermore, although small samples are still rarely used with Drop-seq, future developments of high cell capture efficiency protocols such as the one presented by Chung and colleagues24 will make efficient bead processing strictly necessary. Thus, we expect that our microfluidic-based bead processing will constitute an important experimental cornerstone of these next generation technologies.
Conflicts of interest
There are no conflicts to declare.
Acknowledgements
We thank Wanze Chen, Vincent Gardeux, and Joern Pezoldt for constructive discussions. We thank Michael Frochaux for providing RNA samples from D. melanogaster. We also thank the EPFL CMi and GECF for device fabrication and sequencing support respectively, as well as the VITAL-IT platform (University of Lausanne) for computational support. This research was supported by an Animalfree Research 3R Grant, the Swiss National Science Foundation Grant (IZLIZ3_156815) and a Precision Health & related Technologies (PHRT-502) grant to B. D., as well as by the EPFL SV Interdisciplinary PhD Funding Program to B. D. and E. A.
Notes and references
- A. Regev, S. A. Teichmann, E. S. Lander, I. Amit, C. Benoist, E. Birney, B. Bodenmiller, P. Campbell, P. Carninci, M. Clatworthy, H. Clevers, B. Deplancke, I. Dunham, J. Eberwine, R. Eils, W. Enard, A. Farmer, L. Fugger, B. Goettgens, N. Hacohen, M. Haniffa, M. Hemberg, S. Kim, P. Klenerman, A. Kriegstein, E. Lein, S. Linnarsson, E. Lundberg, J. Lundeberg, P. Majumder, J. C. Marioni, M. Merad, M. Mhlanga, M. Nawijn, M. Netea, G. Nolan, D. Pe'er, A. Phillipakis, C. P. Ponting, S. Quake, W. Reik, O. Rozenblatt-Rosen, J. Sanes, R. Satija, T. N. Schumacher, A. Shalek, E. Shapiro, P. Sharma, J. SHIN, O. Stegle, M. Stratton, M. J. T. Stubbington, F. J. Theis, M. Uhlen, A. van Oudenaarden, A. Wagner, F. Watt, J. Weissman, B. Wold, R. Xavier, N. Yosef and H. C. A. M. Participants, eLife, 2017, 6, 1–30 CrossRef.
- E. Z. Macosko, A. Basu, R. Satija, J. Nemesh, K. Shekhar, M. Goldman, I. Tirosh, A. R. Bialas, N. Kamitaki, E. M. Martersteck, J. J. Trombetta, D. A. Weitz, J. R. Sanes, A. K. Shalek, A. Regev and S. A. McCarroll, Cell, 2015, 161, 1202–1214 CrossRef CAS PubMed.
- A. M. Klein and E. Macosko, Lab Chip, 2017, 17, 2540–2541 RSC.
- E. Brouzes, Droplet Microfluidics for Single-Cell Analysis, Methods Mol. Biol., 2012, 853, 105–139 CrossRef CAS PubMed.
- M. T. Guo, A. Rotem, J. A. Heyman and D. A. Weitz, Lab Chip, 2012, 12, 2146–2155 RSC.
- The Tabula Muris Consortium, Nature, 2018, 562, 367–372 CrossRef CAS PubMed.
- A. Zeisel, H. Hochgerner, P. Loennerberg, A. Johnsson, F. Memic, J. van der Zwan, M. Haering, E. Braun, L. E. Borm, G. La Manno, S. Codeluppi, A. Furlan, K. Lee, N. Skene, K. D. Harris, J. Hjerling-Leffler, E. Arenas, P. Ernfors, U. Marklund and S. Linnarsson, Cell, 2018, 174, 999–1014 CrossRef CAS PubMed.
- K. Davie, J. Janssens, D. Koldere, M. De Waegeneer, U. Pech, Q. Kreft, S. Aibar, S. Makhzami, V. Christiaens, C. B. G. Lez-Blas, S. Poovathingal, G. Hulselmans, K. I. Spanier, T. Moerman, B. Vanspauwen, S. Geurs, T. Voet, J. Lammertyn, B. Thienpont, S. Liu, N. Konstantinides, M. Fiers, P. Verstreken and S. Aerts, Cell, 2018, 174, 982–998 CrossRef CAS PubMed.
- G. X. Y. Zheng, J. M. Terry1, P. Belgrader, P. Ryvkin, Z. W. Bent, R. Wilson, S. B. Ziraldo, T. D. Wheeler, G. P. McDermott, J. Zhu, M. T. Gregory, J. Shuga, L. Montesclaros, J. G. Underwood, D. A. Masquelier, S. Y. Nishimura, M. Schnall-Levin, P. W. Wyatt, C. M. Hindson, R. Bharadwaj, A. Wong, K. D. Ness, L. W. Beppu, H. J. Deeg, C. McFarland, K. R. Loeb, W. J. Valente, N. G. Ericson, E. A. Stevens, J. P. Radich, T. S. Mikkelsen, B. J. Hindson and J. H. Bielas, Nat. Commun., 2017, 8, 1–12 CrossRef PubMed.
- C. Ziegenhain, B. Vieth, S. Parekh, B. Reinius, A. Guillaumet-Adkins, M. Smets, H. Leonhardt, H. Heyn, I. Hellmann and W. Enard, Mol. Cell, 2017, 65, 631–643 CrossRef CAS.
- V. Svensson, K. N. Natarajan, L. Ly, R. J. Miragaia, C. Labalette, I. C. Macaulay, A. Cvejic and S. A. Teichmann, Nat. Methods, 2017, 14, 381–387 CrossRef CAS PubMed.
- W. Stephenson, L. T. Donlin, A. Butler, C. Rozo, B. Bracken, A. Rashidfarrokhi, S. M. Goodman, L. B. Ivashkiv, V. P. Bykerk, D. E. Orange, R. B. Darnell, H. P. Swerdlow and R. Satija, Nat. Commun., 2018, 9, 1–10 CrossRef CAS.
- T. M. Gierahn, M. H. Wadsworth II, T. K. Hughes, B. D. Bryson, A. Butler, R. Satija, S. Fortune, J. C. Love and A. K. Shalek, Nat. Methods, 2017, 14, 395–398 CrossRef CAS PubMed.
- X. Zhang, T. Li, F. Liu, Y. Chen, J. Yao, Z. Li, Y. Huang and J. Wang, Mol. Cell, 2019, 73, 1–13 CrossRef PubMed.
- A. M. Klein, L. Mazutis, I. Akartuna, N. Tallapragada, A. Veres, V. Li, L. Peshkin, D. A. Weitz and M. W. Kirschner, Cell, 2015, 161, 1187–1201 CrossRef CAS PubMed.
- R. Zilionis, J. Nainys, A. Veres, V. Savova, D. Zemmour, A. M. Klein and L. Mazutis, Nat. Protoc., 2017, 12, 44–73 CrossRef CAS PubMed.
- X. Han, R. Wang, Y. Zhou, L. Fei, H. Sun, S. Lai, A. Saadatpour, Z. Zhou, H. Chen, F. Ye, D. Huang, Y. Xu, W. Huang, M. Jiang, X. Jiang, J. Mao, Y. Chen, C. Lu, J. Xie, Q. Fang, Y. Wang, R. Yue, T. Li, H. Huang, S. H. Orkin, G.-C. Yuan, M. Chen and G. Guo, Cell, 2018, 172, 1091–1107 CrossRef CAS PubMed.
- B. A. Biddy, W. Kong, K. Kamimoto, C. Guo, S. E. Waye, T. Sun and S. Morris, Nature, 2018, 564, 219–224 CrossRef CAS PubMed.
- J. A. Farrell, J. A. Farrell, Y. Wang, S. J. Riesenfeld, K. Shekhar, A. Regev and A. F. Schier, Science, 2018, 3131, 1–15 Search PubMed.
- C. Mayer, C. Hafemeister, R. C. Bandler, R. Machold, R. Batista Brito, X. Jaglin, K. Allaway, A. Butler, G. Fishell and R. Satija, Nature, 2018, 555, 457–462 CrossRef CAS PubMed.
- N. Habib, I. Avraham-davidi, A. Basu, T. Burks, K. Shekhar, M. Hofree, S. R. Choudhury, F. Aguet, E. Gelfand, K. Ardlie, D. A. Weitz, O. Rozenblatt-Rosen, F. Zhang and A. Regev, Nat. Methods, 2017, 14, 955–958 CrossRef CAS PubMed.
- M. Plass, J. Solana, F. Alexander Wolf, S. Ayoub, A. Misios, P. Glažar, B. Obermayer, F. J. Theis, C. Kocks and N. Rajewsky, Science, 2018, 360, 1–17 CrossRef PubMed.
- H. Moon, K. Je, J. Min, D. Park, K. Han, S. Shin, W. Park, C. E. Yoo and S. Kim, Lab Chip, 2018, 18, 775–784 RSC.
- M. Chung, D. Núñez, D. Cai and K. Kurabayashi, Lab Chip, 2017, 17, 3664–3671 RSC.
-
E. Macosko, M. Goldman and S. McCarroll, Drop-Seq Laboratory Protocol version 3.1, http://mccarrolllab.org/download/905/ Search PubMed.
- J. Klein, M. Fasshauer, H. H. Klein, M. Benito and C. R. Kahn, BioEssays, 2002, 24, 382–388 CrossRef CAS PubMed.
- S. Picelli, A. Bjoerklund, B. Reinius, S. Sagasser, G. Winberg and R. Sandberg, Genome Res., 2014, 24, 2033–2040 CrossRef CAS PubMed.
- A. Dobin, C. A. Davis, F. Schlesinger, J. Drenkow, C. Zaleski, S. Jha, P. Batut, M. Chaisson and T. R. Gingeras, Bioinformatics, 2013, 29, 15–21 CrossRef CAS PubMed.
- V. Gardeux, F. P. A. David, A. Shajkofci, P. C. Schwalie and B. Deplancke, Bioinformatics, 2017, 33, 3123–3125 CrossRef CAS PubMed.
Footnotes |
† Electronic supplementary information (ESI) available. See DOI: 10.1039/c9lc00014c |
‡ Contributed equally to this work. |
|
This journal is © The Royal Society of Chemistry 2019 |
Click here to see how this site uses Cookies. View our privacy policy here.