Systematic characterization of selenium speciation in coal fly ash†
Received
4th July 2024
, Accepted 20th October 2024
First published on 21st October 2024
Abstract
Millions of tons of coal fly ashes (CFAs) are produced annually during coal combustion in the U.S., which are commonly beneficially used in the concrete industry or disposed of in ash ponds. CFAs contain trace amounts of a range of toxic heavy metals including selenium (Se). Because the toxicity of Se is dependent on its speciation, investigating Se speciation in CFAs as affected by coal source and combustion conditions can help understand the related environmental and human health impacts during disposal or beneficial reuse. In this study, a set of representative CFA samples were characterized for Se speciation using synchrotron X-ray absorption spectroscopy (XAS) and micro-X-ray fluorescence spectromicroscopy (μ-XRF/XAS). Se-containing particles were highly heterogeneous, and individual particles might contain multiple oxidation states including Se(0), Se(IV), and Se(VI). Principal component analysis was performed for sample characteristics including Al2O3, SiO2, CaO, FeO, loss on ignition, average particle size, Se concentration, and Se oxidation state. Selective catalytic reduction (SCR), which is used to limit nitrogen oxide (NOx) emissions during coal combustion, was found to be associated with the presence of reduced Se oxidation states, with up to 90% Se(0) observed in samples with SCR. Alongside SCR, FeO content may also influence Se speciation.
Environmental significance
Coal fly ashes (CFAs) contain a range of toxic heavy metals including selenium (Se). As the toxicity of Se is correlated to its speciation, investigating the effect of coal source and combustion conditions on Se speciation can help understand related environmental and health impacts during disposal or beneficial reuse. This study characterized a set of representative CFA samples for Se speciation using synchrotron X-ray absorption spectroscopy and micro-X-ray fluorescence spectromicroscopy. Se speciation reflects the highly heterogeneous nature of the samples, and individual particles might contain multiple oxidation states. The use of selective catalytic reduction was found to associate with the presence of reduced Se oxidation states.
|
1 Introduction
CFAs are the main byproduct of coal combustion and are typically composed of Al2O3, SiO2, Fe2O3, CaO, and unburned carbon.1 It is estimated that 600–1000 million tons of CFAs are produced globally each year.1,2 In the U.S., CFA management typically involves disposal primarily in ash ponds and beneficial usage in the concrete industry. The typical management of CFAs in the U.S. include >60% being repurposed while the remainder is disposed of.3 For land-limited countries such as Japan, Germany, and the Netherlands, the utilization rates of CFAs and other combustion byproducts are greater than the U.S. Ash ponds are the primary form of CFA disposal in the U.S., but not all sites are lined to prevent leaching into nearby environments and groundwater. A recent study found an estimated 3200 tons of Se discharged into U.S. watersheds throughout the 73 years of exposure to coal combustion residuals (CCRs) such as CFAs.4 Although federal legislation concerning CCRs was passed in 2015 to require liners in ash ponds, there are several exceptions to this rule,5 such as facilities that have closed down or switched resources (e.g., to natural gas), CCRs not received by October 2015, and surface mines.6,7 In May 2023, the EPA proposed changes to the CCR rule to allow for similar federal regulations on inactive ash ponds or legacy contamination sites, although these changes have not been official.8 In addition, there are ongoing great interests in the potential of CFAs for rare earth element extraction.9,10 In all these scenarios (disposal, beneficial usage, resource extraction), the concentration and speciation of toxic heavy metals in CFA should be systematically considered. One such toxic heavy metal is Se, with coal combustion residues (including CFA), mining, and oil refining being important anthropogenic Se inputs.11 Typical concentrations of Se in CFAs are less than 50 ppm, and coal source and combustion conditions might impact its concentration, speciation, and distribution.12
Se is an essential micronutrient and the recommended dose for humans is 70 μg per day.13 Se-containing proteins play essential roles in human function as antioxidants (e.g., glutathione peroxidase) and regulate thyroid metabolism (e.g., iodothyronine deiodinases).14 At high doses (e.g., >400 μg daily or 100 μg dL−1), Se becomes toxic and can potentially lead to selenosis that causes neurological damage, gastrointestinal problems, and hair loss.15,16 For aquatic life, excess Se may lead to teratogenesis, metabolic stress, and death.17 Se typically exists in nature in various oxidation states. For example, in the atmosphere, Se(II) is primarily from oceanic phytoplankton (as dimethyl selenide and dimethyl diselenide) and volcanic emissions. Se(0) is produced from metal refinement and the coal industry, while Se(IV) and Se(VI) are released from the atmosphere during rainfall.18–21 The mobility and toxicity of Se vary significantly with its oxidation state. Se mobility follows the order of Se(VI) > Se(IV) > Se(0).22 Se(IV) is more toxic than Se(VI), while Se(0) is often considered non-toxic.23
Various studies on CFAs have reported the oxidation states and concentrations of Se alongside CFA composition and physical properties.12,24–30 These studies found that CFAs contained Se primarily as Se(IV), while a few studies found ∼15–23% of Se(0).26,30 Se oxidation state has been investigated using X-ray absorption near edge absorption spectroscopy (XANES) and liquid chromatography combined with inductively coupled plasma mass spectrometry (LC-ICP-MS). Despite extensive previous characterizations of CFAs, studies were typically limited by sample numbers and/or incomplete background information. A previous study characterized the coal sources, coal ranks, and particulate matter controls of an extensive set of CFAs,31 but only measured total heavy metal concentration. Most studies characterized a few CFA samples with limited information on coal combustion parameters. As for coal combustion conditions, pulverized coal combustion is most widely used in coal-fired power plants. Along with producing coal combustion residues such as bottom ash and boiler slag, flue gas is generated containing nitrogen oxides (NOx), sulfur oxides (SOx), and fine particles (10–100 μm) known as CFA. Before released into the atmosphere, flue gas undergoes emission controls, including (1) NOx removal with selective catalytic reduction (SCR) or low-NOx burners, (2) particulate matter removal by electrostatic precipitator (ESP) or baghouse filter (BH), and (3) SOx removal with the use of wet flue gas desulfurization (WFGD) or other controls, such as low-sulfur bearing coal. These parameters are of significance due to their potential roles in altering CFA composition and the speciation of heavy metals including Se.
Delving into the chemical speciation of Se in CFA would allow for a more comprehensive understanding of the role of combustion parameters on metal transformation. Since the passing of the coal combustion residuals (CCR) rule in 2015, more stringent guidelines were placed on CFAs and toxic metal release.32 This information may aid the industry in better determining the management options of CFA. To address these needs, the Electric Power Research Institute (EPRI) conducted a survey study across the U.S. to understand the current state for coal technology.33 EPRI selected representative CFAs based on coal source, region, and combustion conditions such as burner type and NOx/SOx/particulate matter control across the U.S. Based on the survey results, a carefully selected set of representative samples were obtained and used in this study to systematically characterize Se speciation. Sample information is summarized in the (ESI) Table S1,† including coal source, coal type, furnace type, SOx control, NOx control, and fly ash capture.34 This representative sample set allows for the analysis of potential correlations between coal type, rank, emission control parameters, and Se speciation. Exploring these correlations may allow for a better understanding of the role these parameters play in Se speciation and downstream beneficially usage or disposal.
2 Materials and methods
2.1. Sample collection
Representative CFA samples were selected and obtained by EPRI based on a recent survey study.33 Coal parameters examined are coal rank (e.g., lignite, subbituminous, bituminous, and anthracite) and coal basin (e.g., Powder River Basin, PRB; Illinois Basin, ILB; and North Appalachia, N. App.). Combustion condition and emission controls examined include furnace type and NOx, SOx, and PM controls. NOx controls include selective catalytic reduction (SCR); and if not specified in Table S1,† low NOx burners were used or electrical generating units (EGUs) units were less than 300 MWg. SOx controls were wet flue gas desulfurization (WFGD) or burning of low-S coal (Table S1†). Particulate matter controls for fly ash capture were baghouses (BH) or electrostatic precipitators (ESP), where ESPc is cold-sided indicating they are located after (downstream of) the air preheater. Furnace type is either tangential or opposed, which refers to the location of the burners. An opposed furnace contains burners on opposite sides and tangential on each wall/corner. Additionally, activated carbon injection (ACI) for Hg emission control was used for sample 1B.
2.2. Composition and particle analysis
Major elements and loss on ignition (LOI) analysis were conducted by the GeoAnalytical Lab at Washington State University using X-ray fluorescence (XRF) with the ThermoARL Advant'XP+ sequential X-ray fluorescence spectrometer. Trace element concentration analysis were conducted at Georgia Institute of Technology and Washington State University using Agilent 7900 inductively coupled mass spectrometer (ICP-MS) and Agilent 7700 ICP-MS respectively after total digestion, using hydrogen as a carrier gas with a total collision cell and indium as an internal standard. Particle morphology characterization was conducted using a Hitachi SU 8230 field emission scanning electron microscope and energy dispersive X-ray spectroscopy (FE-SEM-EDX) at Georgia Institute of Technology. Sample powders were coated in gold using a Hummer 6 Sputterer – Gold Target at Georgia Institute of Technology. SEM analysis was conducted using parameters of 10 μA, 3 keV, high probe current, and the lower secondary electron detector. X-ray diffraction (XRD) analysis was conducted using a Malvern PANalytical Empyrean X-ray diffractometer at Georgia Institute of Technology. Data collection was conducted at 45 keV, 40 mA, a step size of 0.01° 2θ, and a range of 10–70° 2θ. Spectra were normalized by quartz (101) (Fig. S1†). Mineral phase identification for samples 90, 91, and F were from previous studies.35,36 Samples 90 and 91 are Standard Reference Materials Coal Fly Ash 2690 and 2691 from the National Institute of Standards and Technology (NIST).
2.3. Synchrotron X-ray absorption spectroscopy analysis
Se K-edge X-ray absorption near edge structure (XANES) spectroscopy was conducted at beamline 12-BM at the Advanced Photon Source (APS, Lemont, IL, USA) for CFA samples and model compounds. Se foil was provided by APS and used for energy calibration. Multiple scans were taken for each sample, aligned, and averaged for data processing. Se model compounds included (1) Se(0), (2) Se(IV) (selenite) compounds: calcium selenite (CaSeO3), SeO2, selenite doped in the Fe oxide ferrihydrite, selenite adsorbed on ferrihydrite, selenite adsorbed on lime (CaCO3), (3) Se(IV) (selenate) compounds: selenate adsorbed on ferrihydrite, selenate doped in ferrihydrite, and Na2SeVIO4 (origin and synthesis are in Table S2†). The following data processing used the software Athena, version 0.9.26, created by B. Ravel and M. Newville.37 Principal component analysis (PCA) and target transformation were conducted to identify the contributing species. Linear combination fitting (LCF) was then conducted to quantify the relative percentage of identified species in the samples.37 LCF was performed at −30 to +150 eV. Fits for each sample were chosen based on criteria from our previous study,9 where the lowest R-factor was chosen if model compound contributions were ≥10%, due to the typical ∼10% error with LCF of XAS data. Fitting was initially conducted with two compounds, and a third compound was included if it reduced the R-factor by >10% and was more than 10% of the total.9
2.4. Synchrotron X-ray fluorescence microscopy (μ-XRF) and μ-XANES analyses
Combined X-ray fluorescence microscopy (μ-XRF) and μ-XANES analysis were conducted on a subset of the 19 samples, including samples 2, 8, 9, 10, 12, 1B, and 2S (Table S3†). This selection was based on the different combustion conditions and coal basins (Table S1†).34 Petrographic thin sections of sample powers on quartz slides were analyzed at Beamline 2–3 at the Stanford Synchrotron Radiation Lightsource (SSRL, Menlo Park, CA, USA) and beamline 5-ID at the National Synchrotron Light Source (NSLS-II, Upton, NY, USA). Se foil was used for energy calibration. μ-XRF maps were initially collected at a large step size of 10 × 10 μm2 and dwell time of 25 or 100 ms at 13 keV. After identifying Se-containing hot spots/particles, finer scans were collected with a step size of 5 × 5 or 2 × 2 μm2 with the same dwell time. Due to high Fe content, a filter was placed in the path of the beam to attenuate Fe fluorescence. Multi-energy maps were collected at 12
660, 12
664, and 12
672 eV with a step size of 5 × 5 μm2 and 100 ms dwell time. μ-XRF maps were processed using the software SMAK.38 μ-XANES spectra were processed using the software SIXPack and Athena.37–39
2.5. Statistical analysis
Elemental composition (Al2O3, SiO2, FeO, CaO), loss on ignition (LOI), average particle size, Se oxidation state, and Se concentration were analyzed for 16 samples using R-studio.40 Pearson correlation coefficients and a correlation matrix were generated using the corrplot package, and principal component analysis (PCA) was performed.29 Correlation coefficients were classified as strong (R ≤ −0.70 or ≥ 0.70), moderate (R ≤ −0.50 or ≥0.50), or weak (R < −0.30 or ≥0.30).41,42 To analyze compositional data with PCA, the compositions package was used for chemical data: Al2O3, SiO2, CaO, and FeO.43 Data for chemical composition normalized to 100% were transformed with the ‘clr’ function by a centered log ratio.44,45 This transformation was only used for XRF data, since data was normalized to 100%, unlike data for average particle size, loss on ignition (LOI), Se concentration, as well as Se oxidation states. LCF results from normalized bulk XANES spectra were used for Se oxidation state content, and the function ‘prcomp’ was used to perform PCA.46 More details are available in the ESI text S1.†
3 Results and discussion
3.1. Bulk characteristics
Bulk characteristics of CFA samples are summarized in Table S4,† including major element concentration, total Se concentration, average particle size, bulk density, and LOI. Samples were categorized into class C or F based on major element concentration, where class F contains ≥70 wt% of Fe2O3 + SiO2 + Al2O3 and class C contains less than 70 wt%.47 Among these samples, the concentrations of Se range from 5.8 to 260.6 ppm, and the average particle sizes range from 8.3 to 107.5 μm. Our recent SEM analysis of the samples34 showed the dominant presence of spherical morphology of CFA particles, including regular spheres, cenospheres, and plerospheres. Irregularly shaped particles were also identified. XRD analysis revealed the major mineral phases to be quartz (SiO2), anhydrite, magnetite, hematite, portlandite, periclase, gypsum, tricalcium aluminate (C3A), lime, thenardite, and calcite (Fig. S1†). Samples 1–8 that were from the same coal-fired power plant contained thenardite, calcite, and gypsum. Overall, minerals identified were consistent with previous findings by other researchers.48–51
3.2. Average Se speciation
Se speciation was determined using bulk XANES and LCF, which indicate a wide distribution of oxidation states, including Se(0), Se(IV), and Se(VI) (Table S5† and Fig. 1B). Previous XANES speciation studies found Se(IV) as the dominant oxidation state for CFAs, with minor Se(0) content at (11–21%).12,26,28–30,52 Se bulk XANES spectra of samples 90 and 1B were too noisy thus not analyzed. All the CFAs samples in this study (except for samples 13 and 14) contained an appreciable fraction of Se(VI) adsorbed on ferrihydrite (Fig. 2). Other major Se species include selenite doped in ferrihydrite, CaSeO3, and Se(0). Remarkably, samples 13 and 14 contain ≥89% Se(0), while samples 11 and 12 contain ≥45% Se(0) (Table S5†). Some samples were also best fitted by CaSeO3 (Samples 1, 3, 4, 7, 9, 10, and 91) at ≥42%.12,53 All CFAs contain Se associated with Fe (≥13.9%).
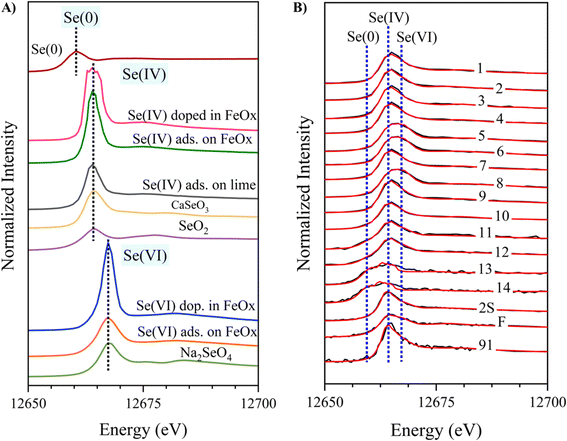 |
| Fig. 1 Se K-edge XANES spectra of (A) model compounds for linear combination fitting (LCF). (Ads: adsorbed and dop: doped), and (B) CFA samples (black solid lines) and corresponding LCF fits (red solid lines). Vertical dashed lines indicate the peak position for Se(0), Se(IV), and Se(VI). | |
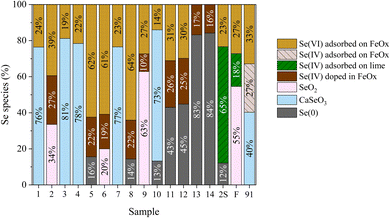 |
| Fig. 2 Relative percentage of different Se species derived by linear combination fitting of Se XANES spectra, summed to 100%. Fitting results are in Table S1.† | |
Interestingly, using this carefully selected representative sample set, this study found a higher percentage of Se(0) in CFA samples compared to previous studies. Huggins et al. suggested that the presence of Se(0) in fly ash was due to activated carbon injection (ACI) for Hg emission control, where activated carbon may have contained organoselenium.29 However, Luo et al. characterized fly ashes with ACI, but Se(0) was detected in a sample with no ACI.52 Luo et al. described an association between Se(0) and unburned carbon (through LOI analysis) for their 5 samples, where LOIs for 4 samples ranged from 0.32–1.57 and 1 sample with an LOI of 17.52.52 In our samples, only sample 1B had ACI but unfortunately its XANES spectra was too noisy to analyze. Based on our results, the correlation coefficients (R) and coefficients of determination (R2) between ACI and Se(0) are 0.5 and 0.27, respectively, suggesting a weak or low association between Se(0) and LOI. More discussion is provided in the statistical analysis section.
3.3. Microscopic speciation
Our bulk Se XANES results indicated the highly heterogeneous nature of CFAs, with a range of Se oxidation states and associations with Fe and Ca-containing phases. Half of the samples contained >10% Se(0). To support such observations on the heterogeneity of CFAs, we conducted μ-XRF/μ-XANES to investigate Se distribution, speciation, and elemental association at micron scale. μ-XRF elemental maps were first obtained to identify Se-containing particles (hot spots), followed by μ-XANES speciation analysis. LCF results of μ-XANES data are summarized in Table S6,†Fig. 3 and 4.
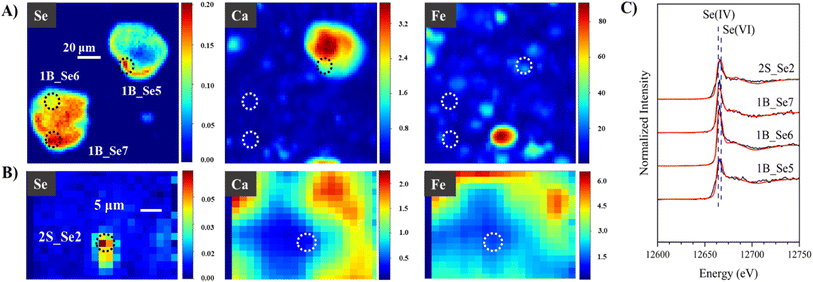 |
| Fig. 3 Representative μ-XRF elemental maps of Se, Ca, and Fe. (A) Hot spots 1B_Se5, 1B_Se6, and 1B_Se7 for sample 1B. (B) Hot spot 2S_Se2 for sample 2S. (C) Raw (black lines) and linear combination fitted μ-XANES spectra (red lines) of the hot spots. Vertical dashed lines indicate the peak position for Se(IV) and Se(VI). | |
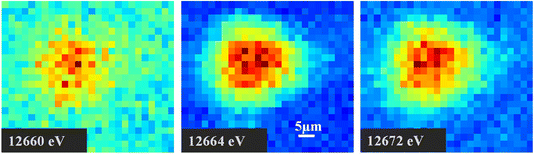 |
| Fig. 4 Representative Se K-edge multi-energy μ-XRF maps at 12 660, 12 664, and 12 672 eV for sample 2. | |
Overall, μ-XANES indicated varied Se speciation within the same micron-sized region and sometimes even within the same particle, as illustrated by sample 1B in Fig. 3A. Se particles with an average size of ∼40 μm are presented in Fig. 3A, and hot spots that are shown as circles indicate where μ-XANES spectra was collected. The bottom particle in Fig. 3A contains two hot spots, with 38% as Se(IV) adsorbed on FeOx and 65% as Se(VI) adsorbed on FeOx for hot spot 1B_Se6, while hot spot 1B_Se7 contains 65% CaSeO3 and 33% as Se(VI) adsorbed onto FeOx. Both hot spots indicate the presence of both Se(IV) and Se(VI), although the phase and elemental association is different within the same particle. As for hotspot 1B_Se5 on the top right particle in Fig. 3A, speciation results were 59% CaSeO3 and 41% Na2SeO4, again indicating multiple Se speciation within a small region. Although μ-XRF elemental maps indicate a high concentration of Ca in hot spot 1B_Se5, this does not necessarily correlate to Se–Ca association unless specified by μ-XANES results. Fig. 3B shows hot spot 2S_Se2 for sample 2S, and LCF results indicate this particle contains 54% CaSeO3 and 44% NaSeO4, showing the presence of two oxidation states and varied elemental associations within a micron-sized spot. Considering that the average speciation results for sample 2S are 12% Se(0), 64% Se(IV) adsorbed on lime, and 23% Se(VI) adsorbed on FeOx, the difference in bulk and micro-scale speciation again highlights the highly heterogeneous nature of CFA particles. Another example is sample 2. Hotspot 2_R5_003 in sample 2 has LCF results of 16% Se(VI) adsorbed on FeOx and 99% NaSeVIO4, compared to the average speciation results of 35% SeIVO2, 28% Se(IV) doped in FeOx, and 41% Se(VI) adsorbed on FeOx (Tables S1 and S2†). Other evidence revealing multiple Se oxidation states within a particle are multi-energy maps, which are μ-XRF maps captured at energies indicative of Se(0), Se(IV), and Se(VI) (Fig. 4) at 12
660, 12
644, and 12
672 eV, respectively. Three oxidation states of Se are visible for sample 2 in Fig. 4 for this ∼35 μm by ∼35 μm particle. Additional μ-XANES spectra and μ-XRF maps are shown in Fig. S2 and S3.†
3.4. Correlation analysis
3.4.1 Correlation coefficients.
To determine potential statistical correlations between quantitative properties of CFAs, for samples 1–14, 1B, and 2S, correlation coefficients (R) were calculated to understand the strength of possible linear relationships. A correlation matrix for SiO2, Al2O3, CaO, FeO, average particle size, Se concentration, Se(0), Se(IV), Se(VI), and LOI is in Fig. 5, where intense warm and cool colors represent strong correlation coefficients, and circle size is dependent on correlation coefficient strength (larger circle implies a higher correlation coefficient value). An R-value of ±0.7 or greater is considered a moderate or strong association. In Fig. 5, strong positive associations are shown by R-values for Se concentration and LOI (R = 0.66), average particle size and FeO (R = 0.75), Se concentration and CaO (R = 0.82), as well as Se(0) and FeO (R = 0.85). Meanwhile, strong negative associations are revealed for Se concentration and Al2O3 (R = −0.78), Se concentration and SiO2 (R = −0.79), Al2O3 and CaO (R = −0.79), as well as SiO2 and CaO (R = −0.86).
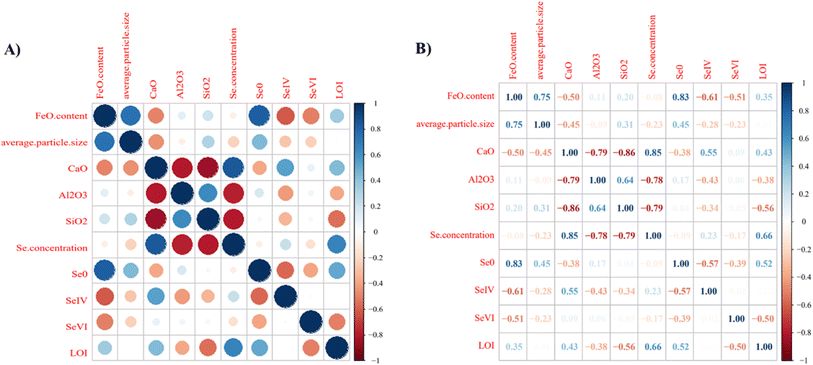 |
| Fig. 5 Pearson correlation coefficient matrices presented as circles (larger circles are closer to 1) (A) and values (B). Warmer colors correspond to negative values and cooler colors correspond to more positive values. | |
A strong positive association between LOI and Se concentration was found, with LOI measuring the amount of unburned carbon. Activated carbon injection has been used to capture heavy metals, so unburnt carbon may act similarly. Reduced Se species as organo-Se is also abundant in coal, suggesting unburned carbon from the coal could be a source of Se(0).28 However, Luo et al. found a low association between LOI and Se concentration with an R2 of 0.25 in their CFA samples.52 A strong positive association for average particle size and Fe content has been explained by raw coal composition where pyrite (FeS2) is commonly present. During the combustion process, pyrite is less easily volatilized compared to carbon so iron oxides exist as larger particles.54 Fu et al. noticed a positive association between Fe content and particle size because Fe oxides are believed to be present as large particles.27 Another strong positive association existed between Se concentration and CaO (for example, sample 10 with 260.6 ppm Se and 51.61 wt% CaO; sample 2S with 13.5 ppm Se and 28.68 wt% CaO). Bulk XANES results revealed CaSeO3 was a dominant compound in these samples (>42%). Seames et al. found a preferential binding of Se with Fe compared to Ca, leading to an increase in Se present as iron selenite (SeVI).54,55 However, CaO has also been studied for SeO2 capture.55 Another positive strong correlation coefficient identified was Se(0) and FeO (0.85). Luo et al. had 1 CFA sample that contained Se(0) which also had the highest FeO content.52
Strong negative associations were identified for both Al2O3 (R = −0.78) and SiO2 (R = −0.79) regarding Se concentration. Previous studies have found Se to react with either Ca or Fe, suggesting little reactivity with the aluminosilicate glass phase during combustion. As for the strong negative association between Al2O3 and CaO, this may stem from the formation of aluminosilicate glass phases as compared to lime and anhydrite due to differences in feed coal composition or combustion parameters. Lastly, there was a strong negative correlation for SiO2 and CaO possibly due to formation of aluminosilicate glass phases.
3.4.2 Principal component analysis.
Principal component analysis (PCA) was conducted to better understand potential relationships between samples and their bulk characteristics. PCA generates components that capture the variability in the data set with the most variability captured by the first component and the lowest variance by the last component. The application purpose of PCA is to better understand the variability in this large data set, since this is a 10 × 16 matrix, with a total of 160 entries. PCA was performed for samples 1–14, 1B, and 2S.
PCA is only for quantitative data, as a result LOI, average particle size, Se concentration, Se oxidation state (Se(0), Se(IV), Se(VI)), and XRF obtained concentration of SiO2, Al2O3, FeO, and CaO were used as variable. Because XRF data is summed to 100%, a centered log-ratio change “clr”, was applied (refer to ESI†).43,56 A clr transformation was applied only to data for SiO2, Al2O3, FeO, and CaO. PCA generated 10 principal components (PCs), where PC1 and PC2 captured the majority of the variability based on eigen values >1 of 3.91 and 3.56 (Tables S7, S8 and Fig. S4†). PCs 1–2 captured 39.1% and 35.6% of the variance, respectively (Table S8†). Loadings produced for PCs 1–2 were considered significant if they are greater than 0.30. Significant loadings for variables in PC1 are FeO (0.46), Se(0) (0.42), Se(VI) (0.32), and LOI (0.31) (Table S9†). Significant loadings from PC2 are CaO (0.43), Al2O3 (−0.37), SiO2 (−0.41), Se concentration (0.47), Se(IV) (0.30), and LOI (0.31) (Table S9†).
To visually interpret results from PCA, a biplot is shown in Fig. S5† with loadings on the left and bottom axes while scores on the top and right axes. In this biplot samples are black numbers, while variables are shown in red with corresponding vectors. Vectors are significant for interpreting the relationship of samples to variables (e.g., Se(0) and SiO2) as well as the relationships for variables. For example, sample 13 is closest in the direction of the Se(0) vector; showing the highest Se(0) content with 90.7% in Table S4.† Similarly, sample 14 is closest to the direction of the FeO vector in Table S10† with 25.5 wt% FeO. Sample 11 has 27.1 wt% FeO, but a closer look reveals that it has the largest average particle size (107.5 μm, Table S4†). As for relationships between variables, this is visible through vector angles. The smaller the angle between vectors the more positive a correlation is for those variables, and a highly negative correlation between variables is visible by a 180° vector angle. Variables with small vectors include Se(IV) and CaO, Al2O3 and SiO2, as well as FeO and Se(0). A potential explanation for a strong association between FeO and Se(0) was discussed above, while Al2O3 and SiO2 may have a strong association due to the presence of aluminosilicates in CFA samples. The strong association between Se(IV) and CaO might be explained by the presence of Se in CFA samples primarily as CaSeIVO3 based on LCF of XANES.
From Fig. S5,† similarities and differences between individual samples are shown by their relative location to one another. There are 5 major clusters, and they are similar to one another. The cluster depicted with a purple circle are samples 11, 14, and 13; these samples have similar values for FeO (27.1, 21.6, and 25.4 wt%, respectively), Se(0) (46, 90.7, and 89.9%, respectively), and some of the largest particle sizes (107.5, 41.3, and 42.6 μm, respectively). Based on Table S1,† samples 11, 14, and 13 are from different coal basins, furnace type, have different SOx, NOx controls, and particulate matter controls. Meanwhile sample 1B is clustered by itself, as well as sample 12, but sample 10 is shown to be the furthest away from all samples in Fig. S5.† Sample 10 had some of the largest (CaO, Se concentration, and LOI) and lowest (average particle size, Al2O3, and SiO2) properties. Lastly, samples 1–9 and 2S are clustered together, while samples 1,4, and 7 are overlaid. Samples 1, 4, and 7 have similar values for FeO (4.7–4.8 wt%), CaO (19.2–19.8 wt%), no Se(0), Se(IV) (22.4–24.4 wt%), and LOI (0.95–1.75). Samples 8 and 5 also overlap, with similar values for Se(VI) (63.8 and 65.6%, respectively), FeO (5.09 and 5.08 wt%), average particle size (14 and 13.7 μm), CaO (17.56 and 17.52 wt%), Al2O3 (20.95 and 20.9 wt%), SiO2 (41.18 and 41.17 wt%), and Se concentration (9.1 and 9.1 ppm). Lastly, samples did not cluster based on parameters shown in Table S1,† except for fly ash class. Class C fly ash samples are clustered to the left of the origin in PC1 (dotted line in Fig. S16†), class F to the right, and sample 10 was distinct.
Another mathematical transformation to better interpret the contribution of each variable to each PC is a varimax rotation (see ESI†). Results for loadings after varimax rotation are shown in Table S11.† Based on these results, FeO has a strong relationship with PC1 (1) and Al2O3 has a strong relationship with PC2 (−1). This suggests FeO and Al2O3 content may capture the majority of the variability within this dataset.
3.5. Qualitative features
Se mobility follows the general order of Se(VI) > Se(IV) > Se(0),22 while toxicity is generally considered as Se(IV) > Se(VI) > Se(0), thus it would be useful to predict Se oxidation state based on qualitative features such as coal source, coal type, combustion condition, SOx control, NOx control, and particulate matter controls. Based on this, trends in Se oxidation for qualitative information were analyzed. The average oxidation states were calculated for each sample based on LCF results (Fig. 6). A majority (60%) of samples have an average oxidation state of ∼5, while ∼13% each have an oxidation state close to 4, 3, and 1. Fig. 6 indicates that samples 1–9 are more oxidized. Samples 2S, 10, 11, 12, 13, and 14 had a more reduced average oxidation state (3.95, 3.72, 3.11, 2.96, 0.73, 0.68, respectively), with samples 13 and 14 originating from the same coal-fired power plant ‘C’, as well as samples 10, 11, and 12 from plant ‘B’ (see ESI†). In agreement with our previous work, samples 13 and 14 contained the greatest amount of reduced As (greater than 40 wt% As(III) while sample 2S contained 19.1 wt% As(III)), the more reduced and toxic form of arsenic.34 Based on Table S1,†34 a common characteristic between samples 13, 14, and 2S is the use of selective catalytic reduction (SCR), which may influence the oxidation state of Se. The average oxidation state of Se is 0.73 and 0.68 for samples 13 and 14, respectively, but 3.95 for sample 2S, indicating that SCR may be related to lower Se oxidation state. However, SCR is not the sole factor that gives rise to Se with oxidation states <5. For instance, the average Se oxidation state in samples 10, 11, and 12 (no SCR use) is 3.72, 3.11, and 2.96, respectively.
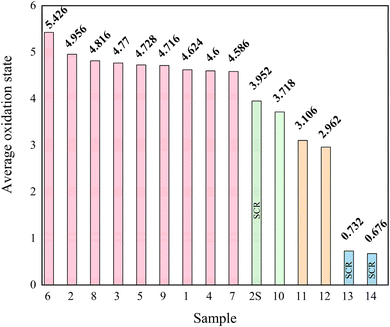 |
| Fig. 6 Average selenium oxidation state for each sample. | |
Based on average selenium oxidation state in Fig. 6, samples 11, 12, 13, and 14 have the lowest Se oxidation state with <+3.1, all originate from bituminous coal. In this study samples that had successful XANES spectra collected and originated from bituminous coal were 11, 12, 13, 14, and 10 (sample 1B had inadequate Se bulk XANES and no spectra was collected on sample F). Coincidentally, these samples also had the lowest Se average oxidation states and are grouped close to one another in Fig. S5† (PCA biplot for principal component 1 and 2), except sample 10, which had vastly different bulk composition (e.g., Al2O3 and SiO2) than the other samples. In Fig. S5,† these samples (11, 12, 13, and 14) also contain the highest FeO content, indicated by the FeO vector. This is also verified by Table S1,† these samples contain the highest Fe2O3 content (15–30 wt%). Bituminous coal is known for having higher iron content compared to subbituminous coal.29Fig. 5 also highlights the correlation coefficient between FeO and Se concentration as 0.83, indicating a strong correlation. This may also suggest that FeO content may influence Se speciation, alongside SCR. For example, sample 2S has a low Fe2O3 content of 6.2 wt%, but it has SCR (Table S4†). Fu also found fly ash with higher FeO content had greater Se(0) enrichment on its surface.27. Taken together, there appears to be a relationship between coal type, iron content, SCR, and Se oxidation state.
4 Environmental implications
More investigations are needed to fully understand the speciation of Se and other toxic trace heavy metals in CFAs as a function of combustion conditions and coal parameters. Findings from this study revealed the high heterogeneity of Se species in CFA particles, where multiple oxidation states (0, +4, and +6) co-exist and sometimes even within the same particle. SCR may be a major contributor to the presence of reduced oxidation state of Se, which is less mobile but more toxic. As a result, CFAs produced by SCR can be considered as a more stable form of coal residue, but better precautions need to be practiced to prevent leaching of Se into the environment. In addition, Se behavior upon in contact with the environment will be subject to other biogeochemical factors such as pH, redox conditions, dissolved organic matter, and microbial interactions. For example, higher concentrations of selenium have been found in oxic environments as compared to anoxic environments.57,58 Findings from this study provide additional guidance and insight for appropriate CFA management and highlight the need for systematic studies on the fate, transport, and impact of heterogeneous Se species in CFAs.
Data availability
Data associated with this study are presented in the ESI† and are available upon request.
Conflicts of interest
There are no conflicts to declare.
Acknowledgements
This study was supported by the U.S. Department of Energy (DOE) National Energy Technology Laboratory (NETL) under grant # DE-FE0031739. This work was performed in part at the Georgia Tech Institute for Electronics and Nanotechnology, a member of the National Nanotechnology Coordinated Infrastructure (NNCI) supported by the National Science Foundation (ECCS-2025462). Portions of this work were conducted at the Stanford Synchrotron Radiation Lightsource (SSRL) at SLAC National Accelerator Laboratory, the Advanced Photon Source (APS) at Argonne National Laboratory, supported by the U.S. Department of Energy, Office of Science, Office of Basic Energy Sciences under Contract No. DE-AC02-76SF00515 and DE-AC02-06CH11357, respectively. This research used beamline 5-ID from the National Synchrotron Light Source II, a U.S. Department of Energy (DOE) Office of Science User Facility operated for the DOE Office of Science by Brookhaven National Laboratory under Contract No. DE-SC0012704.
References
-
American Coal Ash Association, Fly Ash Facts for Highway Engineers, Federal Highway Administration, 2003, https://www.fhwa.dot.gov/pavement/recycling/fafacts.pdf Search PubMed.
- W. Tao, J. Yang, Z. Liu, Y. Zhang, L. Chen, S. Wu, J. Qiao, Z. Xue and Z. Wang, A novel process for recovering aluminum and silicon from fly ash in cryolite molten salt, J. Clean. Prod., 2022, 375, 134170, DOI:10.1016/j.jclepro.2022.134170.
-
Coal Ash Recycling Rate Increases Slightly in 2021; Use of Harvested Ash Grows Significantly, American Coal Ash Association, 2022 Search PubMed.
- A. D. Deonarine, G. E. S. Schwartz and L. S. R. Ruhl, Environmental Impacts of Coal Combustion Residuals: Current Understanding and Future Perspectives, Environ. Sci. Technol., 2023, 57(5), 1855–1869, DOI:10.1021/acs.est.2c06094.
-
U.S. Environmental Protection Agency, Hazardous and Solid Waste Management System: Disposal of Coal Combustion Residuals from Electric Utilities, 2024 Search PubMed.
-
A. Clamp, G&Ts Face Major Cost and Time Challenges in CCR Rule Compliance, National Rural Electric Cooperative Association: Business & Technology Strategies, 2016, pp. 1–24 Search PubMed.
-
T. Schlossberg, For a Texas Ranching Family, Toxic Coal Ash Pollution Hits Home, in Yale Environment 360, Yale School of the Environment, 2019 Search PubMed.
-
ProtectionAgency, U. E., Proposed Changes for Legacy Coal Combustion Residuals Surface Impoundments and CCR Management Units, 2023 Search PubMed.
- P. Liu, R. Huang and Y. Tang, Comprehensive Understandings of Rare Earth Element (REE) Speciation in Coal Fly Ashes and Implication for REE Extractability, Environ. Sci. Technol., 2019, 53(9), 5369–5377, DOI:10.1021/acs.est.9b00005.
- P. Liu, S. Zhao, N. Xie, L. Yang, Q. Wang, Y. Wen, H. Chen and Y. Tang, Green Approach for Rare Earth Element (REE) Recovery from Coal Fly Ash, Environ. Sci. Technol., 2023, 57(13), 5414–5423, DOI:10.1021/acs.est.2c09273.
- X. Gan, J.-C. Huang, M. Zhang, C. Zhou, S. He and W. Zhou, Remediation of selenium-contaminated soil through combined use of earthworm Eisenia fetida and organic materials, J. Hazard. Mater., 2021, 405, 124212, DOI:10.1016/j.jhazmat.2020.124212.
- P. Shah, V. Strezov, K. Prince and P. F. Nelson, Speciation of As, Cr, Se and Hg under coal fired power station conditions, Fuel, 2008, 87(10), 1859–1869, DOI:10.1016/j.fuel.2007.12.001.
- M. Kieliszek and S. Błażejak, Current Knowledge on the Importance of Selenium in Food for Living Organisms: A Review, Molecules, 2016, 21, 609 CrossRef PubMed.
- S. Kurokawa and M. J. Berry, Selenium. Role of the essential metalloid in health, Met. Ions Life Sci., 2013, 13, 499–513, DOI:10.1007/978-94-007-7500-8_16.
- S. B. Goldhaber, Trace element risk assessment: essentiality vs. toxicity, Regul. Toxicol. Pharmacol., 2003, 38(2), 232–242, DOI:10.1016/s0273-2300(02)00020-x.
- L. H. E. Winkel, C. A. Johnson, M. Lenz, T. Grundl, O. X. Leupin, M. Amini and L. Charlet, Environmental Selenium Research: From Microscopic Processes to Global Understanding, Environ. Sci. Technol., 2012, 46(2), 571–579, DOI:10.1021/es203434d.
- A. D. Lemly, Symptoms and implications of selenium toxicity in fish: the Belews Lake case example, Aquat. Toxicol., 2002, 57(1–2), 39–49, DOI:10.1016/S0166-445X(01)00264-8.
- M. Wells and J. F. Stolz, Microbial selenium metabolism: a brief history, biogeochemistry and ecophysiology, FEMS Microbiol. Ecol., 2022, 96(12), fiaa209, DOI:10.1093/femsec/fiaa209.
- H. Wen and J. Carignan, Reviews on atmospheric selenium: Emissions, speciation and fate, Atmos. Environ., 2007, 41(34), 7151–7165, DOI:10.1016/j.atmosenv.2007.07.035.
- G. H. Floor and G. Román-Ross, Selenium in volcanic environments: A review, Appl. Geochem., 2012, 27(3), 517–531, DOI:10.1016/j.apgeochem.2011.11.010.
- W. B. Mosher and A. R. Duce, A global atmospheric
selenium budget, J. Geophys. Res., 1987, 92(D11), 13289–13298, DOI:10.1029/JD092iD11p13289.
-
Agency for Toxic Substances and Disease Registry, Health Effects, Toxicological Profile for Selenium, 2003, pp. 1–135, https://www.ncbi.nlm.nih.gov/books/NBK600364/ Search PubMed.
- C. Garbisu, T. Ishii, T. Leighton and B. B. Buchanan, Bacterial reduction of selenite to elemental selenium, Chem. Geol., 1996, 132(1), 199–204, DOI:10.1016/S0009-2541(96)00056-3.
-
Institute, E. P. R., The Leaching Behavior of Arsenic and Selenium from Coal from Coal Fly Ash, Palo Alto, 2008 Search PubMed.
- T. Narukawa, A. Takatsu, K. Chiba, K. W. Riley and D. H. French, Investigation on chemical species of arsenic, selenium and antimony in fly ash from coal fuel thermal power stations, J. Environ. Monit., 2005, 7(12), 1342–1348, 10.1039/B509817C.
- N. Rivera, D. Hesterberg, N. Kaur and O. W. Duckworth, Chemical Speciation of Potentially Toxic Trace Metals in Coal Fly Ash Associated with the Kingston Fly Ash Spill, Energy Fuels, 2017, 31(9), 9652–9659, DOI:10.1021/acs.energyfuels.7b00020.
- B. Fu, J. C. Hower, S. Dai, S. M. Mardon and G. Liu, Determination of Chemical Speciation of Arsenic and Selenium in High-As Coal Combustion Ash by X-ray Photoelectron Spectroscopy: Examples from a Kentucky Stoker Ash, ACS Omega, 2018, 3(12), 17637–17645, DOI:10.1021/acsomega.8b02929.
- P. Shah, V. Strezov, C. Stevanov and P. F. Nelson, Speciation of Arsenic and Selenium in Coal Combustion Products, Energy Fuels, 2007, 21(2), 506–512, DOI:10.1021/ef0604083.
- F. E. Huggins, C. L. Senior, P. Chu, K. Ladwig and G. P. Huffman, Selenium and Arsenic Speciation in Fly Ash from Full-Scale Coal-Burning Utility Plants, Environ. Sci. Technol., 2007, 41(9), 3284–3289, DOI:10.1021/es062069y.
- Y. Itaya, K. Kuninishi and Y. Hashimoto, Arsenic, selenium, and chromium speciation in fly ash, J. Mater. Cycles Waste Manag., 2022, 24(1), 250–258, DOI:10.1007/s10163-021-01316-2.
- J. C. Hower, J. D. Robertson, G. A. Thomas, A. S. Wong, W. H. Schram, U. M. Graham, R. F. Rathbone and T. L. Robl, Characterization of fly ash from Kentucky power plants, Fuel, 1996, 75(4), 403–411, DOI:10.1016/0016-2361(95)00278-2.
-
Agency, U. E. P., Hazardous and Solid Waste Management System: Disposal of Coal Combustion Residuals from Electric Utilities, 2020 Search PubMed.
-
Institute, E. P. R., Survey of Coal-Fired Power Plants and Analysis of Selected Fly Ashes: A Task toward Elucidating Arsenic and Selenium Speciation, 2022, https://www.epri.com/research/products/000000003002025163 Search PubMed.
- E. Garcia, P. Liu, J. Sanchez, S. Lee, Q. Wang, Y. Wen, S. Shivaprakash, S. Burns and Y. Tang, A survey study on arsenic speciation in coal fly ash and insights into the role of coal combustion conditions, Appl. Geochem., 2024, 170, 106095, DOI:10.1016/j.apgeochem.2024.106095.
- P. Liu, Q. Wang, H. Jung and Y. Tang, Speciation, Distribution, and Mobility of Hazardous Trace Elements in Coal Fly Ash: Insights from Cr, Ni, and Cu, Energy Fuels, 2020, 34(11), 14333–14343, DOI:10.1021/acs.energyfuels.0c02164.
- R. Winburn, D. Grier, G. McCarthy and R. Peterson, Rietveld Quantitative X-Ray Diffraction Analysis of NIST Fly Ash Standard Reference Materials, Powder Diffr., 2000, 15, 163–172, DOI:10.1017/S0885715600011015.
- B. Ravel and M. Newville, ATHENA, ARTEMIS, HEPHAESTUS: data analysis for X-ray absorption spectroscopy using IFEFFIT, J. Synchrotron Radiat., 2005, 12(4), 537–541, DOI:10.1107/S0909049505012719.
- S. Webb, The MicroAnalysis Toolkit: X-ray Fluorescence Image Processing Software, AIP Conf. Proc., 2011, 1365, 196–199, DOI:10.1063/1.3625338.
- S. M. Webb, SIXpack: a graphical user interface for XAS analysis using IFEFFIT, Phys. Scr., 2005, 2005(T115), 1011, DOI:10.1238/Physica.Topical.115a01011.
-
A Language and Environment for Statistical Computing, Vienna, Austria, 2020, https://www.r-project.org/accessed Search PubMed.
- M. M. Mukaka M., Statistics corner: A guide to appropriate use of correlation coefficient in medical research, Malawi. Med. J., 2012, 24(3), 69–71 Search PubMed.
-
D. S. Moore, W. I. Notz and M. A. Flinger, The Basic Practice of Statistics, W. H. Freeman and Company, New York, 2013, pp. 138–138 Search PubMed.
-
Using the R Package "Compositions" Forward to the, 2nd edn, 2005. accessed Search PubMed.
- T. Kim, M. T. Ley, S. Kang, J. M. Davis, S. Kim and P. Amrollahi, Using particle composition of fly ash to predict concrete strength and electrical resistivity, Cement Concr. Compos., 2020, 107, 103493, DOI:10.1016/j.cemconcomp.2019.103493.
-
G. K. Van Den Boogart, Using the R Package “Compositions” Forward to the Second Edition, 2005 Search PubMed.
-
H. Abdi and L. J. Williams, Principal Component Analysis, in Wiley Interdisciplinary Reviews: Computational Statistics, 2010, pp. 433–459 Search PubMed.
-
International, A., Standard Specification for Coal Fly Ash and Raw or Calcined Natural Pozzolan for Use in Concrete, 2023 Search PubMed.
- L. Silva, C. Ward, J. Hower, M. Izquierdo, F. Waanders, M. Oliveira, Z. Li, R. S. Hatch and X. Querol, Mineralogy and Leaching Characteristics of Coal Ash from a Major Brazilian Power Plant, Coal Combustion and Gasification Products, 2010, 2(1), 51–65, DOI:10.4177/CCGP-D-10-00005.1.
- C. M. Weekley, J. B. Aitken, P. K. Witting and H. H. Harris, XAS studies of Se speciation in selenite-fed rats, Metallomics, 2014, 6(12), 2193–2203, 10.1039/c4mt00227j.
-
E. van Hullenbusch, F. Farges, M. Lenz, P. Lens and G. Brown, Selenium Speciation in Biofilms from Granular Sludge Bed Reactors Used for Wastewater Treatment. in International Conference on X-Ray Absorption Fine Structure, Stanford, California, 2006 Search PubMed.
- G. P. Huffman, F. E. Huggins, N. Shah and J. Zhao, Speciation of arsenic and chromium in coal and combustion ash by XAFS spectroscopy, Fuel Process. Technol., 1994, 39(1), 47–62, DOI:10.1016/0378-3820(94)90171-6.
- Y. Luo, D. E. Giammar, B. L. Huhmann and J. G. Catalano, Speciation of Selenium, Arsenic, and Zinc in Class C Fly Ash, Energy Fuels, 2011, 25(7), 2980–2987, DOI:10.1021/ef2005496.
- S. Ghosal and S. A. Self, Particle size-density relation and cenosphere content of coal fly ash, Fuel, 1995, 74(4), 522–529, DOI:10.1016/0016-2361(95)98354-H.
- W. S. Seames and J. O. L. Wendt, Regimes of association of arsenic and selenium during pulverized coal combustion, Proc. Combust. Inst., 2007, 31(2), 2839–2846, DOI:10.1016/j.proci.2006.08.066.
- C. Yuan, C. Zhang, S. Yu, H. Xu, X. Li, Q. Fang and G. Chen, Experimental and Density Functional Theory Study of the Adsorption Characteristics of CaO for SeO2 in Simulated Flue Gas and the Effect of CO2, Energy Fuels, 2020, 34(9), 10872–10881, DOI:10.1021/acs.energyfuels.0c02044.
- P. A. E. Pogge von Strandmann, E. E. Stüeken, T. Elliott, S. W. Poulton, C. M. Dehler, D. E. Canfield and D. C. Catling, Selenium isotope evidence for progressive oxidation of the Neoproterozoic biosphere, Nat. Commun., 2015, 6(1), 10157, DOI:10.1038/ncomms10157.
- G. E. Schwartz, J. C. Hower, A. L. Phillips, N. Rivera, A. Vengosh and H. Hsu-Kim, Ranking Coal Ash Materials for Their Potential to Leach Arsenic and Selenium: Relative Importance of Ash Chemistry and Site Biogeochemistry, Environ. Eng. Sci., 2018, 35(7), 728–738, DOI:10.1089/ees.2017.0347.
- G. E. Schwartz, N. Rivera, S.-W. Lee, J. M. Harrington, J. C. Hower, K. E. Levine, A. Vengosh and H. Hsu-Kim, Leaching potential and redox transformations of arsenic and selenium in sediment microcosms with fly ash, Appl. Geochem., 2016, 67, 177–185, DOI:10.1016/j.apgeochem.2016.02.013.
|
This journal is © The Royal Society of Chemistry 2024 |
Click here to see how this site uses Cookies. View our privacy policy here.