DOI:
10.1039/D4TB01835D
(Review Article)
J. Mater. Chem. B, 2024,
12, 11054-11062
AI-driven precision subcellular navigation with fluorescent probes
Received
14th August 2024
, Accepted 24th September 2024
First published on 11th October 2024
Abstract
Precise navigation within intricate biological systems is pivotal for comprehending cellular functions and diagnosing diseases. Fluorescent molecular probes, designed to target specific biological molecules, are indispensable tools for this endeavor. This paper delves into the revolutionary potential of artificial intelligence (AI) in crafting highly precise and effective fluorescent probes. We will discuss how AI can be employed to: design new subcellular dyes by optimizing physicochemical properties; design prospective subcellular targeting probes based on specific receptors; quantitatively explore the potential chemical laws of fluorescent molecules to optimize the optical properties of fluorescent probes; optimize the comprehensive properties of the probe and guide the construction of multifunctional targeting probes. Additionally, we showcase recent AI-driven advancements in probe development and their successful biomedical applications, while addressing challenges and outlining future directions towards transforming subcellular research, diagnostics, and drug discovery.

Yingli Zhu
| Yingli Zhu is a PhD candidate under the supervision of Professor Wenbin Zeng at Central South University, working in probe development, computer-aided drug design, and cheminformatics. |

Jie Dong
| Jie Dong is an associate professor of Central South University. His research interests include artificial intelligence in drug discovery, the development of software/web servers/databases in chem/bioinformatics and drug discovery. |

Wenbin Zeng
| Wenbin Zeng is a full professor at Central South University since 2008. His research focused on developing novel molecular probes, drugs, and bionanotechnology for the early detection, treatment, and theranostics of diseases. |
1. Introduction
Subcellular organelles are special subunits in eukaryotic cells, including mitochondria, lysosomes, the endoplasmic reticulum, Golgi apparatus, etc. Their structural integrity and functional stability are the basis of cell life activities.1,2 Each subcellular organelle has a unique chemical composition and internal environment, which both closely cooperate with each other and maintain an orderly division of labor, thus forming a precise interaction network to realize the rapid exchange of matter and information.3,4 The disruption of biomolecular homeostasis in subcellular organelles or the abnormal changes of microenvironment can lead to the dysfunction of organelles, and then lead to disorder or diseases. In clinical practice, the dysfunction of organelles or their interaction networks is highly correlated with the occurrence and development of various diseases, such as tumors,5 inflammation,6 Parkinson's disease7 and Pearson's syndrome.8 Therefore, monitoring the structure of subcellular organelles and internal environmental changes can promote the mechanism of disease research, enhance the understanding of diseases, and promote the development of basic medicine.
At the same time, subcellular targeting of diagnostic and therapeutic strategies has received particular attention in a variety of biomedical applications, including diagnosis, sensing, imaging and drug delivery for multiple diseases.9 Subcellular organelle targeting strategies can precisely accumulate therapeutic agents in the organelles, greatly reduce the threshold dose of therapeutic agents, and minimize drug-related side effects,10 gaining insight into the pharmacokinetics of intracellular drugs and study drug distribution at the subcellular level.11
In recent years, the research of targeting, visualization and regulation at the subcellular level has received extensive attention and made a series of remarkable progress, but there are still some problems that are difficult to overcome. (1) Currently, subcellular targeting strategies are mainly divided into chemical fragment-based targeting and nanomaterial-based targeting.12 However, both of these strategies are passive targeting, with limited targeting ability and few targeting groups to choose from. (2) Visual analysis of subcellular organelles is the research frontier of disease diagnosis at present.9 Fluorescent probes have become the preferred tools for visual analysis of subcellular organelles due to their outstanding sensitivity, high resolution, non-invasiveness and synthetic feasibility.13,14 However, many classical subcellular organelle fluorescent probes mostly use an indirect metabolite detection mechanism to image subcellular organelles.15–18 (3) At present, the regulation strategies of the subcellular microenvironment are mostly based on the regulation of related functional proteins.19–22 However, most of these pharmacodynamic molecules have limited subcellular enrichment capacity, require high therapeutic doses, and cannot be visually monitored. (4) The construction of multi-functional diagnosis and treatment integrated molecules is highly dependent on the personal experience of the developer and it is difficult to take into account the optimization of multiple properties. At the same time, it has a series of defects, such as a long research and development cycle, high cost and low success rate.23,24
Artificial intelligence (AI) technology, especially deep generation models,25 as the cutting-edge technology of the times, has gradually penetrated into all aspects of drug development and marketing in recent years,26 and has brought a new data-oriented research and development model to the field of medicine and materials.27,28 By building models with rich potential chemical space through data training and participating in the design, the construction of integrated molecules for probe development and multifunctional diagnosis and treatment is expected to evolve into a repeatable engineering route that does not rely on subjective experience.
Therefore, this paper will discuss the application prospects and revolutionary potential of AI in constructing accurate subcellular targeting fluorescent probes from a unique perspective from the aspects of targeting principle, response mode, and optical property optimization (Fig. 1).
 |
| Fig. 1 AI-driven fluorescent probes for accurate subcellular navigation properties. | |
2. AI-driven subcellular navigation based on physicochemical properties
Different subcellular organelles such as mitochondria, the endoplasmic reticulum and lysosomes have unique physical and chemical properties, including pH,29 charge,30 lipid composition, etc.31,32 These differences in physical and chemical properties provide the basis for the design of specific targeted molecules,33 allowing researchers to precisely deliver different functional molecules to target subcellular organelles.34,35 Some classical subcellular organelle fluorescent dyes are targeted through such pathways. Here, we summarize these localization mechanisms as non-specific subcellular targeting strategies. In the early studies, the targeted subcellular fluorescent probes were mostly designed by the experience of the investigators, or by the introduction of several specific localization groups.14,30 However, in the past two years, artificial intelligence technology has emerged in the field of fluorescent probe design, and through ligand-based models, in-depth exploration of potential chemical signatures with different subcellular localization effects has injected new vitality into the non-subjective data-based design of such probes.
In 2023, Dong et al. explored for the first time the association between chemical structural features of fluorescent probes and subcellular localization using a multilayer machine learning (ML) model.36 According to the interpretation of the model, a series of key molecular fingerprints and laws affecting localization are quantitatively summarized, which provides a pioneering research idea for this field (Fig. 2a).
 |
| Fig. 2 AI driven subcellular navigation based on physicochemical properties. (a) Exploring potential chemical features of subcellular targeting through model interpretation. The important MACCS molecular fingerprints and the SHAP values of the subcellular model. Reproduced from ref. 36 with permission from SPJ, copyright 2024. (b) Flowchart for the development of the machine learning methods for predicting the cell staining ability of fluorescent dyes. Reproduced from ref. 37 with permission from Wiley, copyright 2024. (c) Internal chemical environments in condensates selectively concentrate small molecules in different ways. | |
A few months later, Yang et al. synthesized 1536 dyes using high-throughput experimentation and used these dyes to build more elaborate ML models for predicting the dye's specific ability to stain organelles (Fig. 2b).37
Subsequently, Kilgore et al.38 demonstrated that different types of biomolecular condensates (liquid-like organelles) have different detectable properties.39 In their study, the selective distribution of probes in different condensates is successfully predicted by using deep learning (DL) methods, and the different conditions that may cause such effects are explained microscopically (Fig. 2c).
Then, Park et al. used the concept of quantitative structure–activity relationship (QSAR), and summarized the key factors affecting subcellular localization such as log
D and pKa from the perspective of physicochemical properties.40
These efforts have enabled the exploration of potential associations between subcellular targeting and chemical structure based on physicochemical properties or kinetic processes. This class of models successfully breaks the limitation of traditional intact targeting groups, so that the subcellular targeting ability can be quantitatively predicted, evaluated, and explained. It is expected to be a powerful means of designing novo dyes or probes for subcellular navigation.
3. AI-driven specific targeting and response based on subcellular proteins
Different subcellular structures have their own unique specific receptors or transporters,41–43 which not only play a key role in maintaining the function and homeostasis of the subcellular level, but also provide potential targets for the specific delivery of drug and probe molecules. Different subcellular targeting probes can be designed using proteins that are specifically highly expressed on different subcellular structures. For example, the commercial dye ER-Tracker Green is a derivative of BODIPY coupled with glibenclamide. Glibenclamide acts as a targeted fragment and binds to the sulfonylurea (SUR) receptor of ATP-sensitive potassium channels (K-ATP) in the ER, thereby achieving its specific targeting to the ER.44 In addition, some of the subcellular proteins also have considerable pharmacological properties, which is conducive to the development of integrated probes for diagnosis and treatment.45,46 In recent years, AI has shown great promise in receptor-based drug design, and its methods are gradually being applied to the design of multi-functional probes with specific targets,47 but the research in this part is still relatively scarce.
In 2024, Zhu et al. used a variety of techniques, including machine learning, to screen out targeted inhibitory structures with high affinity for aminopeptidase N (APN), which is highly expressed in tumor cell membranes, and designed a multifunctional probe (ABTP–DPTB) based on the 3D structure of the active pocket of APN.47 At the same time, the response mode of fluorescence signal recovery by the protein limiting the binding conformation of the probe in this work also provides a reference for other specific responses based on subcellular proteins (Fig. 3a).
 |
| Fig. 3 AI driven specific targeting and response based on subcellular proteins. (a) Typical case: AI driven design of the APN target probe. (b) Perspective: developing subcellular targeted structures based on subcellular proteins. (c) Localization of subcellular proteins: non-negative matrix factorization (NMF) based map of the cell generated by t-distributed stochastic neighbour embedding (t-SNE). Reproduced from ref. 42 with permission from Nature, copyright 2024. (d) The mechanism of BindWeb for the ligand binding residue and pocket prediction from protein structures. Reproduced from ref. 48 with permission from Wiley, copyright 2024. | |
The design process of ABTP–DPTB inspires that by designing and screening structures with high affinity for subcellular proteins, the subcellular targeting based on specific proteins can be realized (Fig. 3b and c). The special spatial shape of the subcellular protein pocket combined with the conformational restriction strategy can realize a specific protein-based subcellular fluorescence response. At the same time, the pharmacologically active subcellular proteins can also be used in the development of multifunctional probes for integrated diagnosis and treatment.
Therefore, as long as the specific subcellular proteins are known, it is possible to construct subcellular targeted structures through various AI-based models, and further use these results to build fluorescent probes with specific active targeting functions. However, not all subcellular proteins have definite binding sites, so it becomes particularly important to explore more reasonable binding sites or potential pockets.48–52 In order to solve such problems, many AI-based recognition tools have been proposed in recent years.
In 2017, a webserver named TRAPP was developed by Stank et al. to predict protein binding site flexibility and detect transient binding pockets.50 By using TRAPP, the structural plasticity of a binding pocket could be analyzed. And in 2022, Xia et al. further realized accurate ligand binding residue and pocket prediction via DL (Fig. 3d).48
In 2023, Lei et al. proposed an ensemble learning model called StackCPA for compound-protein binding affinity prediction based on pocket multi-scale features.51 This class of tools holds promise for further application to high-affinity ligand screening based on the detected potential pockets of subcellular proteins. At the same time, a novel method for the automatic detection of pockets on protein molecular surfaces (SiteFerret) was developed by Luca et al. based on the isolation forest. By using SiteFerret a wide range of binding sites could be predicted including the difficult shallow sites.52
With these AI tools, the potential binding pockets of various subcellular proteins will be effectively explored. From the shape of the potential pockets, combined with techniques such as deep learning and virtual screening, different specific subcellular probes can be developed (Fig. 3b).
4. AI-driven improvements of optical properties
In addition to the subcellular targeting ability, optical properties are also key factors to be considered in the development of subcellular fluorescent probes. However, traditional fluorescent dye development processes often rely on subjective experience, have limited chemical parent cores, and may not meet the application scenarios of multifunctional probes. In recent years, AI has made considerable progress in the design of auxiliary fluorescent molecules, which is expected to solve this problem and develop subcellular targeted fluorescent probes with more excellent optical properties.
In 2021, Joung et al. pioneered the use of DL models and experimental databases to develop deep learning optical spectroscopy (Fig. 4a). Their model could predict seven optical and photophysical properties of organic compounds, namely, the absorption peak position and bandwidth, extinction coefficient, emission peak position and bandwidth, photoluminescence quantum yield and emission lifetime (Fig. 4b).53
 |
| Fig. 4 AI-driven design, prediction and generation of fluorescent probes. (a) The steps for fluorophore development via deep learning optical spectroscopy. Reproduced from ref. 53 with permission from ACS, copyright 2024. (b) The comparison of the deep learning optical spectroscopy model predicted emissions with experimental emissions. Reproduced from ref. 53 with permission from ACS, copyright 2024. (c) Workflow of de novo fluorescence generation with embedded molecular PESs. Reproduced from ref. 54 with permission from Science, copyright 2024. | |
Then, in March 2022, Sumita et al. combined quantitative calculation with a machine learn-based de novo molecular generator (based on ChemTS) to achieve de novo generation of novel fluorescent molecules (Fig. 4c).54 At the same time, Shao et al. have realized the prediction of the maximum absorption wavelength of the fluorophore by DL, which has a satisfactory accuracy.55
Furthermore, in August 2022, Bhat et al. used density functional theory (DFT) and time-dependent DFT (TDDFT) to evaluate molecular properties including frontier molecular orbitals, ionization energy, relaxation energy, and low-lying optical excitation energies. This data set is then used for ML modeling, enabling rapid prediction of molecular electronic, oxidation–reduction properties or optical properties based solely on SMILES.28
In addition, more and more predictive models for the prediction and screening of molecules with specific optical effects have been proposed (Fig. 5a and b).55–64 Among these, two particularly notable models are the AIEgens’ prediction model61 proposed by Zhang et al. in 2023 and the excited state intramolecular proton transfer (ESIPT) prediction model64 developed by Huang et al. in 2024. This is the first high-quality ESIPT multi-level prediction system that could accurately identify ESIPT molecules from a large number of compounds under a stepwise process distinguishing them from conventional molecules to fluorescent molecules and then to ESIPT molecules. Furthermore, the potential association between ESIPT and molecular structure is also explored through model interpretation based on the SHapley Additive exPlanations (SHAP) method (Fig. 5a).
 |
| Fig. 5 Models for prediction and screening of molecules with specific optical effects. (a) Hunting for expectant fluorescent molecules with artificial intelligence: the first high-quality ESIPT dataset and a multi-level prediction system for accurate identification of ESIPT molecules from a large number of compounds. Reproduced from ref. 64 with permission from Wiley, copyright 2024. (b) Models for molecular properties associated with fluorescence. | |
These techniques provide valuable insights for the design and optimization of targeting probes, and can enable specialized responses or signal amplification in specific subcellular environments. For example, predicting and modulating the ESIPT properties of molecules can enable the construction of pH-responsive probes for selective imaging of acidic cells or organelles.65,66 By using the model to screen molecules with a potential aggregation-induced emission (AIE) effect, the fluorescence signal can be amplified in the target subcellular organelle.67,68 These AI-based methods enable objective and rapid quantitative screening, saving time in the early stages of design and improving success rates.
5. AI-driven construction of multifunctional subcellular-targeted probes
When constructing multifunctional subcellular targeting probes, many properties need to be taken into account, such as the ADMET (absorption, distribution, metabolism, excretion, and toxicity) properties69–74 and the synthesis accessibility75–77 of the probes. All of these key properties can be predicted and screened by DL or ML. One of the latest examples is Deep-PK,72 a deep learning-based pharmacokinetic and toxicity prediction, analysis and optimization platform, proposed by Myung et al. (Fig. 6a). Through such platforms, the in vivo ADMET properties of probes can be preliminarily predicted and optimized at the design stage, resulting in more expected pharmacokinetics and lower toxicity of the probes. In addition, it is crucial to consider the accessibility of probes beforehand to minimize the waste of research resources. DeepSA, proposed by Wang et al. in 2023, effectively addresses these needs. It can be used to predict the synthesis accessibility of various compounds, including fluorescent probes (Fig. 6b).75
 |
| Fig. 6 Deep learning based molecular property prediction. (a) Deep-PK: deep learning driven prediction of small molecule pharmacokinetics and toxicity. Reproduced from ref. 72 with permission from Oxford University Press, copyright 2024. (b) The architecture of DeepSA, a bidirectional encoder representations from transformers (BERT) model for the synthesis accessibility prediction of molecules. Reproduced from ref. 75 with permission from BMC, copyright 2024. | |
At the same time, the progress made by AI in the field of image analysis can also be applied to the recognition of subcellular structures78–80 in fluorescence images, which is conducive to further strengthening the visualization accuracy of subcellular structures. This technique can be used as a potential aid in integrated work.
A representative case is a recent work by Zhang et al.81 where they used six fluorescent probes to form an organelle-targeted sensor array (OT-SA) to image pancreatic metastatic cancer cells and cell spheroids (Fig. 7). The residual network (ResNet) model is further used to automatically extract and select image features to find the subtle differences among similar samples. With the ResNet model, they successfully identified pancreatic metastatic cells and cell spheroids, providing an attractive new strategy for more refined subcellular fluorescence imaging.
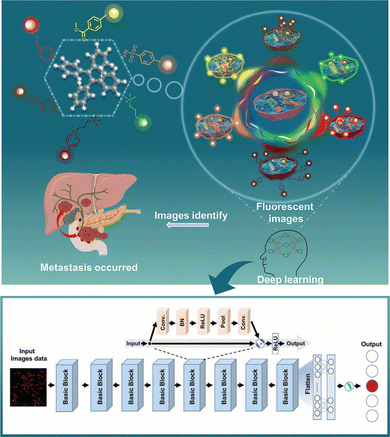 |
| Fig. 7 The combination of an organelle-targeted sensor array with ResNet-based model for pancreatic cancer metastatic analysis. Reproduced from ref. 81 with permission from Wiley, copyright 2024. | |
All these results suggest that AI technology can be applied to various stages of fluorescence probe design, optimization and imaging analysis.
6. Future prospects
The convergence of artificial intelligence, biomedicine, and materials chemistry is rapidly reshaping the landscape of fluorescent probe development. AI-powered machine and deep learning models have emerged as potent tools for designing targeted fluorescent molecules. By analyzing vast chemical spaces and predicting molecular properties, these models accelerate discovery and enhance the precision of probe design. These models excel in predicting properties such as subcellular localization, optical characteristics, and synthetic accessibility, while also identifying key determinants of molecular behavior. This shift from subjective, qualitative assessments to data-driven, quantitative evaluations empowers researchers to design more effective and innovative fluorescent probes.
Despite significant progress, challenges persist in the application of AI to fluorescent probe design. In terms of data for model building, the constraints imposed by limited available data hinder the development of highly accurate models. Therefore, more comprehensive datasets or databases of subcellular localization and fluorescent molecules need to be further developed. For the modelling process, the representation of probe molecules used for subcellular localization differs from that of conventional small molecules, as these probe molecules are often composed of different functional components and require more specific descriptors to reveal these characteristics. Developing such descriptors would contribute to further improvements in model accuracy. Moreover, in cases where selecting representative features is difficult or when addressing the complexities of subcellular fluorescent imaging, deep learning or even large language models could offer unique value. Additionally, when designing subcellular fluorescent probes, critical properties such as excitation and emission wavelengths, stability, signal-to-noise ratio, and cellular dynamics must be closely focused on and accurately predicted. Precise predictions of these properties often require time-consuming quantum mechanics (QM) or molecular dynamics (MD) calculations. For instance, the fluorescent properties of probes can be accurately predicted using QM calculations, while MD can be employed to predict the dynamic trajectories of probes in complex subcellular environments, enabling precise dynamic subcellular imaging. Combining AI with these classical methods holds significant potential to effectively save computational resources while maintaining prediction accuracy.
Overall, integrating AI tools into subcellular navigation and fluorescent probe design presents significant potential. However, overcoming the associated chemical and biological challenges will require ongoing innovation in both AI methodologies and chemical biology strategies. Tackling these issues is essential for advancing the field and achieving more accurate and effective subcellular imaging and targeting.
7. Conclusions
In conclusion, AI has markedly enhanced the efficiency and precision of subcellular fluorescent probe design at multiple levels. Its transformative potential to revolutionize probe design for accurate subcellular navigation is evident, empowering researchers to delve deeper into intricate cellular processes. This interdisciplinary fusion is steering fluorescent probe development towards greater intelligence, efficiency, and accuracy. Nevertheless, AI currently serves as an auxiliary tool, necessitating the development of more sophisticated algorithms and representative descriptors. Despite this, probe designers can leverage AI's potential by integrating existing models to create multifunctional targeting molecules tailored to specific application demands.
Author contributions
Yingli Zhu: conceptualization, writing original draft, review & editing; Yanpeng Fang: preparation of graphics; Wenzhi Huang: literature analysis; Weiheng Zhang: literature analysis; Fei Chen: review & editing; Jie Dong: conceptualization, writing original draft, review & editing, supervision; Wenbin Zeng: funding acquisition, conceptualization, writing original draft and review, supervision.
Data availability
No primary research results, software or code have been included and no new data were generated or analysed as part of the review.
Conflicts of interest
There are no conflicts to declare.
Acknowledgements
This work was supported by the National Natural Science Foundation of China (82272067, 81974386, 22107123, M-0696), the Innovation-Driven Project of Central South University (2023CXQD004), the Science and Technology Foundation of Hunan Province (2022JJ80052, 2022JJ40656), the Science and Technology Innovation Program of Hunan Province (No. 2024RC3004), and the Innovation Fund for Postgraduate Students of Central South University (2021zzts0980, 2023ZZTS0842).
References
- D. Lisse, C. Monzel, C. Vicario, J. Manzi, I. Maurin, M. Coppey, J. Piehler and M. Dahan, Adv. Mater., 2017, 29, 1700189 CrossRef.
- Z. Lv, Z. W. Man, H. T. Cui, Z. Z. Xu, H. H. Cao, S. Li, Q. Liao, Q. H. He, L. M. Zheng and H. B. Fu, Adv. Funct. Mater., 2021, 31, 2009329 CrossRef CAS.
- J. W. Huang, P. Meng, C. Wang, Y. F. Zhang and L. L. Zhou, Theranostics, 2022, 12, 2445–2464 CrossRef CAS.
- W. J. Li, S. H. Zhang and G. Yang, Wiley Interdiscip. Rev.: Mech. Dis., 2021, 13, e1505 CrossRef CAS.
- A. T. Nguyen, J. Chia, M. Ros, K. M. Hui, F. Saltel and F. Bard, Cancer Cell, 2017, 32, 639–653 CrossRef CAS.
- A. Habtezion, A. S. Gukovskaya and S. J. Pandol, Gastroenterology, 2019, 156, 1941–1950 CrossRef CAS.
- M. A. Eldeeb, R. A. Thomas, M. A. Ragheb, A. Fallahi and E. A. Fon, Physiol. Rev., 2022, 102, 1721–1755 CrossRef CAS PubMed.
- Y. Ayami, S. C. Grünert, H. Cario, A. Fisch, U. Gross-Wieltsch, K. Timmermann, U. Kontny, S. Lobitz, H. S. Odenthal, I. Schmid, B. Uetz, T. Höll, A. Rötig, T. Lücke, A. Borkhardt, G. Strauss, A. Hohnecker, M. Metzler, D. Karall and C. M. Niemeyer, Br. J. Haematol., 2021, 193, 1283–1287 CrossRef.
- X. T. Shao, C. C. Meng, W. J. Song, T. Zhang and Q. X. Chen, Adv. Drug Delivery Rev., 2023, 199, 114977 CrossRef CAS PubMed.
- Y. X. Cheng, Z. Qu, Q. Jiang, T. T. Xu, H. Y. Zheng, P. Ye, M. D. He, Y. Q. Tong, Y. Ma and A. Y. Bao, Adv. Mater., 2023 DOI:10.1002/adma.202305095.
- Z. Fang and H. Chen, Adv. Drug Delivery Rev., 2023, 200, 115020 CrossRef CAS PubMed.
- Z. J. Li, S. L. Chu, Y. H. Zhang, W. J. Chen, J. Chen, Y. B. Yuan, S. F. Yang, H. M. Zhou, T. Chen and Z. G. Xiao, Adv. Mater., 2022, 34, 2203529 CrossRef CAS.
- W. Xu, Z. B. Zeng, J. H. Jiang, Y. T. Chang and L. Yuan, Angew. Chem., Int. Ed., 2016, 55, 13658–13699 CrossRef CAS.
- H. Zhu, J. L. Fan, J. J. Du and X. J. Peng, Acc. Chem. Res., 2016, 49, 2115–2126 CrossRef CAS.
- L. W. Huang, Y. T. Zhou, D. Jiao, J. Ren, Y. L. Qi, H. P. Wang, Y. Shi, D. Ding and X. Xue, Aggregate, 2024, 5, e403 CrossRef CAS.
- L. J. Gui, J. Yan, J. Y. Zhao, S. Y. Wang, Y. Y. Ji, J. Liu, J. S. Wu, K. Yuan, H. Liu, D. W. Deng and Z. W. Yuan, Biosens. Bioelectron., 2023, 240, 115660 CrossRef CAS PubMed.
- Z. Q. Wang, C. H. Zhao, Y. W. Li, J. Q. Wang, D. Y. Hou, L. Wang, Y. Z. Wang, X. W. Wang, X. Liu, H. Wang and W. H. Xu, Adv. Mater., 2023, 35, 2210732 CrossRef CAS.
- W. Zhang, J. J. Hu, R. Liu, J. Dai, L. Z. Yuan, Y. H. Liu, B. C. Chen, M. X. Gong, F. Xia and X. D. Lou, Adv. Sci., 2023, 10, 2207228 CrossRef CAS PubMed.
- N. A. Bonekamp, B. Peter, H. S. Hillen, A. Felser, T. Bergbrede, A. Choidas, M. Horn, A. Unger, R. Di Lucrezia, I. Atanassov, X. P. Li, U. Koch, S. Menninger, J. Boros, P. Habenberger, P. Giavalisco, P. Cramer, M. S. Denzel, P. Nussbaumer, B. Klebl, M. Falkenberg, C. M. Gustafsson and N. G. Larsson, Nature, 2020, 588, 712–716 CrossRef CAS PubMed.
- X. Chen and J. R. Cubillos-Ruiz, Nat. Rev. Cancer, 2021, 21, 71–88 CrossRef CAS PubMed.
- A. T. Macias, D. S. Williamson, N. Allen, J. Borgognoni, A. Clay, Z. Daniels, P. Dokurno, M. J. Drysdale, G. L. Francis, C. J. Graham, R. Howes, N. Matassova, J. B. Murray, R. Parsons, T. Shaw, A. E. Surgenor, L. Terry, Y. K. Wang, M. Wood and A. J. Massey, J. Med. Chem., 2011, 54, 4034–4041 CrossRef CAS PubMed.
- H. Y. Li, K. S. Iyer, L. Bao, J. L. Zhai and J. J. Li, Adv. Healthcare Mater., 2024, 13, 2301597 CAS.
- X. C. Tong, X. H. Liu, X. Q. Tan, X. T. Li, J. X. Jiang, Z. P. Xiong, T. Y. Xu, H. L. Jiang, N. Qiao and M. Y. Zheng, J. Med. Chem., 2021, 64, 14011–14027 CrossRef CAS.
- Y. Cheng, Y. S. Gong, Y. S. Liu, B. S. Song and Q. Zou, Briefings Bioinf., 2021, 22, bbab344 CrossRef.
- Y. LeCun, Y. Bengio and G. Hinton, Nature, 2015, 521, 436–444 CrossRef CAS.
- E. J. Topol, Nat. Med., 2019, 25, 44–56 CrossRef CAS PubMed.
- R. Gómez-Bombarelli, J. N. Wei, D. Duvenaud, J. M. Hernández-Lobato, B. Sánchez-Lengeling, D. Sheberla, J. Aguilera-Iparraguirre, T. D. Hirzel, R. P. Adams and A. Aspuru-Guzik, ACS Cent. Sci., 2018, 4, 268–276 CrossRef.
- V. Bhat, P. Sornberger, B. S. S. Pokuri, R. Duke, B. Ganapathysubramanian and C. Risko, Chem. Sci., 2022, 14, 203–213 RSC.
- S. A. Freeman, S. Grinstein and J. Orlowski, Physiol. Rev., 2023, 103, 515–606 CrossRef CAS PubMed.
- Z. W. Xue, R. Zhu, S. Y. Wang, J. Li, J. H. Han, J. Liu and S. F. Han, Anal. Chem., 2018, 90, 2954–2962 CrossRef CAS.
- D. Casares, P. V. Escribá and C. A. Rosselló, Int. J. Mol. Sci., 2019, 20, 2167 CrossRef CAS.
- C. L. Jackson, L. Walch and J. M. Verbavatz, Dev. Cell, 2016, 39, 139–153 CrossRef CAS PubMed.
- A. S. Klymchenko, Acc. Chem. Res., 2023, 56, 1–12 CrossRef CAS PubMed.
- W. X. Ma, L. Q. Zhang, Y. Shi, Y. Ran, Y. Liu and J. S. You, Adv. Funct. Mater., 2020, 30, 2004511 CrossRef CAS.
- D. I. Danylchuk, P. H. Jouard and A. S. Klymchenko, J. Am. Chem. Soc., 2021, 143, 912–924 CrossRef CAS.
- J. Dong, J. Qian, K. Q. Yu, S. Huang, X. Cheng, F. Chen, H. L. Jiang and W. B. Zeng, Research, 2023, 2023, 0075 CrossRef.
- Y. K. Yang, Y. M. Ji, X. Han, Y. X. Long, C. Stewart, Y. Q. Wen, H. Y. Lee, T. Cao, J. S. Han, S. J. Chen and L. X. Li, Adv. Mater. Technol., 2023, 8, 2300427 CrossRef CAS.
- H. R. Kilgore, P. G. Mikhael, K. J. Overholt, A. Boija, N. M. Hannett, C. Van Dongen, T. I. Lee, Y. T. Chang, R. Barzilay and R. A. Young, Nat. Chem. Biol., 2024, 20, 291–301 CrossRef CAS.
- H. R. Kilgore and R. A. Young, Nat. Chem. Biol., 2022, 18, 1298–1306 CrossRef CAS PubMed.
- S. H. Park, H. G. Lee, X. Liu, S. K. Lee and Y. T. Chang, Chemosensors, 2023, 11, 310 CrossRef CAS.
- V. Todorovic, Nat. Methods, 2018, 15, 760 CrossRef CAS PubMed.
- C. D. Go, J. D. R. Knight, A. Rajasekharan, B. Rathod, G. G. Hesketh, K. T. Abe, J. Y. Youn, P. Samavarchi-Tehrani, H. Zhang, L. Y. Zhu, E. Popiel, J. P. Lambert, É. Coyaud, S. W. T. Cheung, D. Rajendran, C. J. Wong, H. Antonicka, L. Pelletier, A. F. Palazzo, E. A. Shoubridge, B. Raught and A. C. Gingras, Nature, 2021, 595, 120–124 CrossRef CAS PubMed.
- Y. X. Jiang, D. L. Wang, Y. F. Yao, H. Eubel, P. Künzler, I. M. Moller and D. Xu, Comput. Struct. Biotechnol. J., 2021, 19, 4825–4839 CrossRef CAS PubMed.
- S. Phaniraj, Z. Gao, D. Rane and B. R. Peterson, Dyes Pigm., 2016, 135, 127–133 CrossRef CAS.
- J. J. Yang, A. Griffin, Z. Qiang and J. Ren, Signal Transduction Targeted Ther., 2022, 7, 379 CrossRef CAS.
- Y. F. Wu, X. H. Chen, L. W. Zhu, D. L. Wang, X. Li, J. Y. Song, D. Wang, X. Y. Yu, Y. Li and B. Z. Tang, ACS Appl. Mater. Interfaces, 2023, 15, 50821–50835 CrossRef CAS PubMed.
- Y. L. Zhu, J. Qian, K. Q. Yu, J. Hou, Y. S. Ma, F. Chen, J. Dong and W. B. Zeng, Chin. Chem. Lett., 2024, 35, 108948 CrossRef CAS.
- Y. Xia, C. Q. Xia, X. Y. Pan and H. B. Shen, Protein Sci., 2022, 31, e4462 CrossRef.
- A. Stank, D. B. Kokh, J. C. Fuller and R. C. Wade, Acc. Chem. Res., 2016, 49, 809–815 CrossRef CAS PubMed.
- A. Stank, D. B. Kokh, M. Horn, E. Sizikova, R. Neil, J. Panecka, S. Richter and R. C. Wade, Nucleic Acids Res., 2017, 45, W325–W330 CrossRef CAS PubMed.
- C. Q. Lei, Z. L. Lu, M. Wang and M. Li, Comput. Biol. Med., 2023, 164, 107131 CrossRef CAS.
- L. Gagliardi and W. Rocchia, J. Chem. Theory Comput., 2023, 19, 5242–5259 CrossRef CAS.
- J. F. Joung, M. Han, J. Hwang, M. Jeong, D. H. Choi and S. Park, JACS Au, 2021, 1, 427–438 CrossRef CAS PubMed.
- M. Sumita, K. Terayama, N. Suzuki, S. Ishihara, R. Tamura, M. K. Chahal, D. T. Payne, K. Yoshizoe and K. Tsuda, Sci. Adv., 2022, 8, eahj3906 CrossRef.
- Z. Tan, Y. Li, X. Wu, Z. Y. Zhang, W. M. Shi, S. Q. Yang and W. L. Zhang, RSC Adv., 2023, 13, 1031–1040 RSC.
- Z. Tan, Y. Li, Z. Y. Zhang, T. Penfold, W. M. Shi, S. Q. Yang and W. L. Zhang, New J. Chem., 2023, 47, 9550–9554 RSC.
- Z. R. Ye, I. S. Huang, Y. T. Chan, Z. J. Li, C. C. Liao, H. R. Tsai, M. C. Hsieh, C. C. Chang and M. K. Tsai, RSC Adv., 2020, 10, 23834–23841 RSC.
- Y. H. Zhao, K. Chen, B. H. Yu, Q. W. Wan, Y. Wang, F. S. Tang and X. M. Li, J. Mol. Struct., 2024, 1317, 139126 CrossRef CAS.
- H. C. Shi, Y. J. Li, S. L. Zhao, B. Qiao, Z. Q. Liang, H. E. Zhao, Z. Xu and D. D. Song, J. Phys. Chem. C, 2023, 127, 23526–23535 CrossRef CAS.
- Y. H. Zhao, K. Chen, L. Zhu and Q. Huang, Dyes Pigm., 2023, 220, 111670 CrossRef CAS.
- Y. B. Zhang, M. Z. Fan, Z. R. Xu, Y. H. Jiang, H. J. Ding, Z. Z. Li, K. X. Shu, M. Y. Zhao, G. Feng, K. T. Yong, B. Q. Dong, W. Zhu and G. X. Xu, J. Nanobiotechnol., 2023, 21, 107 CrossRef CAS.
- M. Jeong, J. F. Joung, J. Hwang, M. Han, C. W. Koh, D. H. Choi and S. Park, npj Comput. Mater., 2022, 8, 147 CrossRef CAS.
- C. W. Ju, H. Z. Bai, B. Li and R. Z. Liu, J. Chem. Inf. Model., 2021, 61, 1053–1065 CrossRef CAS.
- W. Z. Huang, S. Huang, Y. P. Fang, T. Y. Zhu, F. Y. Chu, Q. H. Liu, K. Q. Yu, F. Chen, J. Dong and W. B. Zeng, Adv. Sci., 2024, 11, 2405596 CrossRef CAS.
- F. F. Xiang, H. Zhang, Y. L. Wu, Y. J. Chen, Y. Z. Liu, S. Y. Chen, Y. Z. Guo, X. Q. Yu and K. Li, Adv. Mater., 2024, 36, 2404828 CrossRef CAS PubMed.
- B. Feng, Y. L. Zhu, J. X. Wu, X. Y. Huang, R. Song, L. Huang, X. P. Feng and W. B. Zeng, Chin. Chem. Lett., 2021, 32, 3057–3060 CrossRef CAS.
- P. Alam, W. He, N. L. C. Leung, C. Ma, R. T. K. Kwok, J. W. Y. Lam, H. H. Y. Sung, I. D. Williams, K. S. Wong and B. Z. Tang, Adv. Funct. Mater., 2020, 30, 1909268 CrossRef CAS.
- Y. F. Wang, T. B. Zhang and X. J. Liang, Small, 2016, 12, 6451–6477 CrossRef CAS PubMed.
- G. L. Xiong, Z. X. Wu, J. C. Yi, L. Fu, Z. J. Yang, C. Y. Hsieh, M. Z. Yin, X. X. Zeng, C. K. Wu, A. P. Lu, X. Chen, T. J. Hou and D. S. Cao, Nucleic Acids Res., 2021, 49, W5–W14 CrossRef CAS PubMed.
- L. Fu, S. H. Shi, J. C. Yi, N. N. Wang, Y. H. He, Z. X. Wu, J. F. Peng, Y. C. Deng, W. X. Wang, C. K. Wu, A. Lyu, X. X. Zeng, W. T. Zhao, T. J. Hou and D. S. Cao, Nucleic Acids Res., 2024, 52, W422–W431 CrossRef PubMed.
- J. Dong, N. N. Wang, Z. J. Yao, L. Zhang, Y. Cheng, D. F. Ouyang, A. P. Lu and D. S. Cao, J. Cheminf., 2018, 10, 29 Search PubMed.
- Y. Myung, A. G. C. de Sa and D. B. Ascher, Nucleic Acids Res., 2024, 52, W469–W475 CrossRef PubMed.
- Y. X. Gu, Z. H. Yu, Y. M. Wang, L. Chen, C. F. Lou, C. Yang, W. H. Li, G. X. Liu and Y. Tang, Nucleic Acids Res., 2024, 52, W432–W438 CrossRef PubMed.
- J. C. Yi, S. H. Shi, L. Fu, Z. Y. Yang., P. F. Nie, A. P. Lu, C. K. Wu, Y. F. Deng., C. Y. Hsieh, X. X. Zeng, T. J. Hou and D. S. Cao, Nat. Protoc., 2024, 19, 1105–1121 CrossRef CAS.
- S. H. Wang, L. Wang, F. L. Li and F. Bai, J. Cheminf., 2023, 15, 103 CAS.
- Y. Wang, C. Pang, Y. Z. Wang, J. R. Jin, J. J. Zhang, X. X. Zeng, R. Su, Q. Zou and L. Y. Wei, Nat. Commun., 2023, 14, 6155 CrossRef CAS PubMed.
- W. H. Gao and C. W. Coley, J. Chem. Inf. Model., 2020, 60, 5714–5723 CrossRef CAS.
- J. J. Collier and R. W. Taylor, EMBO Mol. Med., 2021, 13, e14316 CrossRef CAS PubMed.
- A. A. Sekh, I. S. Opstad, G. Godtliebsen, A. B. Birgisdottir, B. S. Ahluwalia, K. Agarwal and D. K. Prasad, Nat. Mach. Intell., 2021, 3, 1071–1080 CrossRef.
- R. Chen, X. Tang, Y. X. Zhao, Z. Y. Shen, M. Zhang, Y. S. Shen, T. T. Li, C. H. Y. Chung, L. J. Zhang, J. Wang, B. B. Cui, P. Fei, Y. S. Guo, S. W. Du and S. H. Yao, Nat. Commun., 2023, 14, 2854 CrossRef CAS.
- G. Y. Zhang, Z. R. Wang, L. J. Ma, J. G. Li, J. H. Han, M. G. Zhu, Z. X. Zhang, S. L. Zhang, X. Zhang and Z. Wang, Adv. Healthcare Mater., 2024, 13, 2400241 CrossRef CAS.
|
This journal is © The Royal Society of Chemistry 2024 |
Click here to see how this site uses Cookies. View our privacy policy here.