DOI:
10.1039/C5RA14672K
(Paper)
RSC Adv., 2015,
5, 85383-85394
Identification of miRNAs and their targets in transgenic Brassica napus and its acceptor (Westar) by high-throughput sequencing and degradome analysis†
Received
24th July 2015
, Accepted 2nd October 2015
First published on 2nd October 2015
Abstract
MicroRNAs (miRNAs) are a class of noncoding small RNAs (sRNAs) that play many roles in plant growth, development, and the stress response. Recently, the Brassica napus (B. napus) genome was reported, which allows the genome-wide identification of miRNAs and their targets in B. napus cv. Westar and its transgenic cultivar. Thus, two sRNAs libraries and two degradome libraries of the transgenic B. napus (TG) and its acceptor (B. napus cv. Westar, WA) were constructed. Following high-throughput sequencing and miRNA identification, 139 unique conserved and 305 unique novel miRNA sequences were identified in the two sRNA libraries, and through degradome analysis, 540 targets corresponding to 167 unique miRNA sequences were identified in the two degradome libraries. 11 differentially expressed unique miRNA sequences were verified by quantitative real-time PCR (qRT-PCR), and the results nearly agreed with the high-throughput sequencing data. The present study increases the number of miRNAs identified in B. napus and suggests that there are possible differences in the miRNA expression between transgenic B. napus and its acceptor.
Introduction
In eukaryotes, gene expression is regulated and controlled at the transcriptional and post-transcriptional levels, and one class of regulators of post-transcriptional gene silencing (PTGS) is small RNAs (sRNAs).1,2 MicroRNAs (miRNAs), which constitute a major class of sRNAs that are approximately 21–24 nucleotides (nt) in length, are endogenous, noncoding, regulatory sRNA molecules that have been widely identified in animals, plants and viruses.2–5 Increasing lines of evidence have shown that miRNAs play an important role in a wide range of plant processes, including development, signal transduction, and various biological and environmental stress responses.5
In higher plants, miRNA genes are transcribed into primary miRNA (pri-miRNA) transcripts by RNA polymerase II, and these transcripts form stable hairpin structures. Dicer-like 1 (DCL 1), hyponastic leaves 1 (HYL 1) and serrate (SE) protein then process the hairpin structures into stem-loop precursor miRNAs (pre-miRNAs) and then cleaved these into miRNA duplexes containing two stands (5p and 3p).1,6,7 The duplexes are then loaded into the RNA-induced silencing complex (RISC) in the cytoplasm. One strand, either the 5p or 3p mature miRNA, binds the Argonaute (AGO) protein in the RISC complex, and the other strand is degraded.1,7 The miRNA–RISC complex then targets mRNAs and guides their cleavage, resulting in the regulation of gene expression.
The recently proposed combination of high-throughput sequencing with bioinformatics analysis has improved the discovery of novel miRNAs in several plants due to the conservation of miRNAs among related plant species.8–11 Furthermore, degradome sequencing combined with gene annotation has also been used for the large-scale confirmation of several miRNA targets in many plants because plant miRNAs completely or almost completely bind their targets.12–15 However, this strategy for identifying miRNAs and their targets strongly depends on the available genomic information and miRNA databases (e.g., miRBase).
Brassica napus (B. napus) is one of the main edible oil crops worldwide.11 Westar (Canola) coming from Canada is a cultivar of B. napus, which was bred to reduce erucic acid that have pathogenic potential and is implicated as a factor in hypercholesterolemia.16 Several previous studies of miRNAs in B. napus have focused on miRNA identification, seed development, the cadmium response and fungus infection.17–25 Xie et al. (2007) detected 21 potential miRNAs only by using computational analysis.17 Buhtz et al. (2008) firstly identified 32 annotated miRNAs in the phloem of B. napus by using high-throughput sequencing with bioinformatics analysis.18 After that, Xu et al. (2012) and Körbes et al. (2012) respectively used high-throughput sequencing to identify miRNAs in the whole B. napus.19,20 In addition, Huang et al. (2013) detected numerous potential miRNA sequences in B. napus seed maturation;21 Zhao et al. (2012) identified 59 miRNAs involved in B. napus oil production;22 Zhou et al. (2012) compared B. napus miRNA expression between cadmium-treated and non-treated.23 Shortly after, Verma et al. (2014) and Shen et al. (2014) respectively researched miRNA response to fungi infection in B. napus.24,25 Although high-throughput sequencing has widely been applied into the B. napus miRNAs and their targets identification in these previous studies, these miRNA identification approaches still lacked the whole genome information of B. napus and were mainly based on expressed sequence tags (ESTs), tentative consensus sequences (TCs) and the genomes of related species (e.g., Brassica rapa and Brassica oleracea). This strongly limits B. napus miRNA researches. However, the B. napus genome was reported recently,26 and miRBase has now been updated to version 21.0 (current edition). Therefore, a genome-wide the identification and comparison of miRNAs and their targets in transgenic B. napus (TG) and its acceptor (Westar, WA) can now be performed.
Materials and methods
Plant materials
The transgenic B. napus constructed by Dr Song Cheng was transformed with a construct (a plasmid pCNFIRnos) containing ihpRNA targeted to the endogenous B. napus fad2 gene.27 Both transgenic B. napus and its acceptor (Westar) were germinated in a glasshouse in the experimental field at the Jiangsu Academy of Agricultural Sciences, Nanjing, China; and were placed under a light/dark (16 h/8 h) photo-period with light intensity of ≥8000 lx at 23 ± 1 °C. Leaves and stalks from three-month-old seedlings were collected. To minimize inter-individual differences, three samples from the same line were mixed together and then frozen in liquid nitrogen and stored at −80 °C.
RNA extraction
Samples from the WA and TG lines were individually subjected to RNA extraction using the TRIzol® reagent (Invitrogen, Carlsbad, CA, USA). The RNA concentrations were measured with a NanoDrop 2000 spectrophotometer (NanoDrop Technologies, Wilmington, DE, USA), and the RNA quality was assessed by agarose gel electrophoresis. The total RNA extracted was used for miRNA identification, degradome analysis and qRT-PCR verification.
High-throughput sequencing
Two sRNA libraries were constructed using the Illumina TruSeq Small RNA Preparation Kit (LC Sciences, Hangzhou, China). The total sRNA was ligated to 3p and 5p adapters (ADTs; 3p adapter: 5′-TGGAATTCTCGGGTGCCAAGG-3′; 5p adapter: 5′-GUUCAGAGUUCUACAGUCCGACGAUC-3′), and the corresponding cDNA was obtained by reverse-transcription PCR. Following purification, the cDNA from the two sRNA libraries was sequenced using an Illumina Genome Analyzer II (LC Sciences, Hangzhou, China). After removing low-quality data and low-copy-number reads (reads < 10), the raw reads were obtained using the Illumina Pipeline v1.5 software (LC Sciences, Hangzhou, China). The ACGT101-miR program (LC Sciences, Hangzhou, China) was then used for further data analysis.28–33
miRNA identification
After filtering out ADTs data, sequences with lengths < 17 and >25 nt, junk data, RFam (http://rfam.janelia.org) and repeats (http://www.girinst.org/repbase), the remaining reads (clean reads) were subjected to miRNA identification using the Brassicaceae pre-miRNAs and miRNAs in miRBase 21.0 (http://www.mirbase.org) and the B. napus genome database (http://www.genoscope.cns.fr/brassicanapus). Three mismatches were allowed between the candidate miRNAs and the known Brassicaceae pre-miRNA and miRNA sequences.31–36 The reads that mapped to known Brassicaceae pre-miRNAs and whose pre-miRNAs or candidate miRNAs mapped to the B. napus genome were identified as conserved miRNAs. In addition, the reads that did not map to known Brassicaceae pre-miRNAs but mapped to the B. napus genome were considered novel miRNAs. Furthermore, the stem-loop structures (secondary structures) of all of the identified and potential pre-miRNAs from the B. napus genomic positions were predicted using the UNAFold software (http://www.mfold.rna.albany.edu/?q=mfold/RNA-Folding-Form),34,37 and only those with high minimal folding energy indexes (MFEIs) were considered (MFEI ≥ 0.7 for conserved miRNAs, MFEI ≥ 0.9 for novel miRNAs).38
Degradome sequencing
Two degradome libraries were constructed based on the method described by Ma et al.39 poly(A)-enriched RNAs were obtained and ligated to a 5p adapter harboring the EcoP15 I recognition site. Through reverse-transcription PCR, the ligated sequences were converted to cDNA. Following EcoP15 I digestion and purification, the cDNA was subjected to cluster analysis with an Illumina Cluster Station (LC Sciences, Hangzhou, China) and sequenced using an Illumina Genome Analyzer II (LC Sciences, Hangzhou, China). Lastly, after removing low-quality data, the raw reads were obtained using the Illumina Pipeline v1.5 software (LC Sciences, Hangzhou, China).
Target identification and annotation
After filtering out ADTs data and reads with lengths <15 nt, the remaining reads were compared with a cDNA library in the B. napus genome database. Afterward, the mapped-cDNA reads were compared with the identified miRNAs to perform an alignment analysis as described previously using CleaveLand 3.0 (LC Sciences, Hangzhou, China).14,40 Low alignment scores (≤4) were considered. Furthermore, based on the number of degradome sequences and their abundance values, the miRNA targets were classified into 5 categories (0, 1, 2, 3 and 4) as previous studies:14,15,20,24,29,31,33 Category 0, the abundance of degradome sequences (>1) was equal to the maximum value, and there was only one maximum value; Category 1, the abundance of degradome sequences (>1) was equal to the maximum value, and there was more than one maximum value; Category 2, the abundance of degradome sequences (>1) was between the maximum and median values; Category 3: the abundance of degradome sequences (>1) was below the median value; and Category 4: only one degradome sequence was detected. To further elucidate the functions of miRNAs and their targets, these were annotated through Gene Ontology (GO, http://www.geneontology.org) and Kyoto Encyclopedia of Genes and Genomes (KEGG, http://www.genome.jp/kegg) pathway analyses.
qRT-PCR
Total RNA was firstly carried out reverse transcription reaction. Sequentially, by using SYBR Premix Ex Taq™ (Takara, Dalian, China) system, quantitative real-time PCR (qRT-PCR) was conducted on a BIOER Line-Gene K RT-PCR machine (BIOER, Hangzhou, China), whose primers were listed in Additional file 10: Table S10.1.† U6 snRNA was used as the internal reference.30,32,33 In addition, all reactions were performed in triplicate, and relative expression levels were quantified by using the 2−ΔΔCt method.
Statistical analysis
log2 ratio, Chi-square 2 × 2 and Fisher's exact tests were performed to identify differences in miRNA expression and in the degradation of their targets between the WA and TG libraries. p values both from Chi-square 2 × 2 test and Fisher's exact test have been automatically adjusted to control False Discovery Rate (FDR) as previous studies,29–33,43 according to Benjamini–Hochberg procedure.41,42
Results
Analysis of sRNA libraries
To identify conserved and novel miRNAs in transgenic B. napus (TG) and its acceptor (B. napus cv. Westar, WA), two sRNA libraries were constructed, firstly. Through high-throughput sequencing, a total of 15
287
111 (from the WA library) and 13
084
245 (from the TG library) raw reads were generated (Additional file 1: Table S1†). After filtering out the 3ADT data, reads with lengths < 17 and >25 nt, junk data, RFam and repeats, 11
285
339 and 9
574
008 clean reads corresponding to 3
073
405 and 2
579
167 unique reads with lengths of 17–25 nt were obtained for the two libraries, respectively (Additional file 1: Table S1, Additional file 2: Table S2.1†). The distribution of the lengths of the clean reads was mainly between 21 and 24 nt in the two libraries (Fig. 1, Additional file 2: Table S2†), which is consistent with previous reports in other species, particularly plants.15,18–25,29–33,43–46 Additionally, we also analyzed Rfam data for confirming the stability of the other non-coding sRNAs. The results roughly shown that rRNA, snRNA, snoRNA should be stable in both libraries as a general understanding (Additional file 2: Table S2.2†).
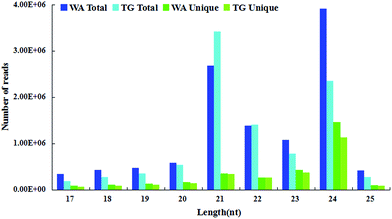 |
| Fig. 1 Length distribution of the clean reads in the TG and WA libraries. (WA total) total sRNA sequences from WA library; (TG total) total sRNA sequences from TG library; (WA unique) unique sRNA sequences from WA library; (TG unique) unique sRNA sequences from TG library. | |
Identification of conserved miRNAs
To identify conserved miRNAs in B. napus, the clean reads were compared with the known B. napus pre-miRNAs in miRBase 21.0, and these pre-miRNAs were mapped to the B. napus genome. A total of 75 conserved unique miRNA sequences (corresponding to 119 miRNAs and 74 pre-miRNAs) were identified as conserved B. napus miRNAs (abbreviated as Con-BN) (Additional file 3: Table S3.1†). Similar to the conserved miRNAs identified in B. napus, 56 unique miRNA sequences (corresponding to 66 miRNAs and 47 pre-miRNAs) were found to be conserved with Brassicaceae miRNAs (abbreviated as Con-BA) (Additional file 3: Table S3.2†). Furthermore, the remaining reads, which mapped to Brassicaceae pre-miRNAs but whose pre-miRNAs did not map to the B. napus genome, were compared with the B. napus genome. 8 conserved unique miRNA sequences (corresponding to 11 miRNAs and 9 predicted pre-miRNAs) were identified as conserved-novel miRNAs (abbreviated as Con-N) (Additional file 3: Table S3.3†). Additionally, all of the identified and predicted pre-miRNAs in the B. napus genome are capable of forming stem-loop structures (secondary structures), and only those with a minimal folding free index (MEFI) of at least 0.7 were considered (Additional file 3: Table S3†). In summary, 139 conserved unique miRNA sequences corresponding to 196 miRNAs and 130 pre-miRNAs and belonging to 52 miRNA families were identified in the WA and TG libraries (Fig. 2, Additional file 3: Table S3, Additional file 4: Table S4†), and among these, 25 unique sequences, such as bra-miR158-3p, bna-miR159, bna-miR166f, bna-miR396a_1ss21TG and bna-miR166a,b,c,d,e, presented high expression (normalized reads ≥ 1000) (Table 1).
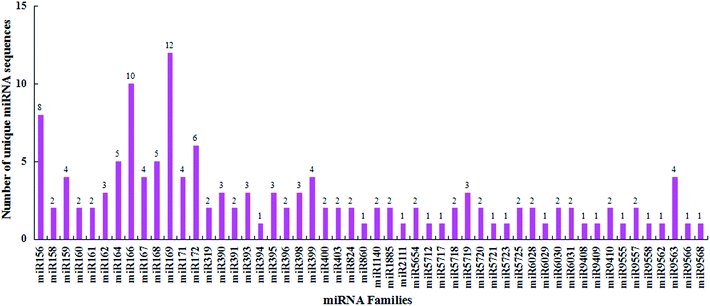 |
| Fig. 2 Distribution of the families of the conserved unique miRNA sequences in the WA and TG libraries. | |
Table 1 Conserved unique miRNA sequences that are highly expressed (normalized reads ≥ 1000) in the WA and TG libraries
Namea |
Sequence |
WA norm |
TG norm |
log2 ratiob |
Name regulation following Additional file 3: Table S3; red marked miRNAs were detected in degradome analysis. log2(WA norm/TG norm); ** indicates highly significant variation (p-value < 0.01 from both Chi-square 2 × 2 & Fisher's exact test and |log2(WA/TG)| ≥ 1) between the WA and TG libraries. |
bna-miR156e_R+1 |
TGACAGAAGAGAGTGAGCACT |
3496 |
687 |
2.35** |
bra-miR158-3p |
TTTCCAAATGTAGACAAAGCA |
18 164 |
26 437 |
−0.54 |
bra-miR158-5p |
CTTTGTCTATCGTTTGGAAAAG |
11 274 |
14 289 |
−0.34 |
bna-miR159 |
TTTGGATTGAAGGGAGCTCTA |
29 676 |
18 564 |
0.68 |
bna-MIR159-p5 |
AGCTGCTAAGCTATGAATCCC |
1078 |
472 |
1.19** |
ath-MIR159a-p5, aly-MIR159a-p5 |
AGCTGCTAAGCTATGGATCCC |
1476 |
867 |
0.77 |
bra-miR162-3p |
TCGATAAACCTCTGCATCCAG |
2464 |
2548 |
−0.05 |
bna-miR166a,b,c,d,e |
TCGGACCAGGCTTCATTCCCC |
131 410 |
213 973 |
−0.70 |
aly-miR166a-5p |
GGAATGTTGTCTGGCTCGAGG |
3809 |
2522 |
0.59 |
bna-miR166f |
TCGGACCAGGCTTCATCCCCC |
36 772 |
59 919 |
−0.70 |
aly-miR166b-3p_L+1 |
TTCGGACCAGGCTTCATTCCCC |
1859 |
2380 |
−0.36 |
bna-MIR167c-p3 |
GATCATGTTCGTAGTTTCACC |
4435 |
3639 |
0.29 |
bna-miR168a |
TCGCTTGGTGCAGGTCGGGAA |
2131 |
1828 |
0.22 |
bra-miR168b-5p,c-5p |
TCGCTTGGTGCAGGTCGGGAC |
3475 |
3766 |
−0.12 |
ath-miR390a-3p_R-1_1ss5AG |
CGCTGTCCATCCTGAGTTTC |
546 |
1043 |
−0.93 |
bna-miR396a_1ss21TG |
TTCCACAGCTTTCTTGAACTG |
22 401 |
24 363 |
−0.12 |
bra-miR398-3p |
TGTGTTCTCAGGTCACCCCTG |
3207 |
39 219 |
−3.61** |
ath-miR399c-3p |
TGCCAAAGGAGAGTTGCCCTG |
3183 |
1257 |
1.34** |
bna-miR403 |
TTAGATTCACGCACAAACTCG |
8430 |
10 001 |
−0.25 |
bna-MIR824-p3 |
CCTTCTCATCGATGGTCTAGA |
2537 |
4809 |
−0.92 |
bna-miR1140 |
ACAGCCTAAACCAATCGGAGC |
6607 |
3771 |
0.81 |
bna-MIR1140-p5 |
TCCGATTGGCTTTAGGCTGTT |
1526 |
671 |
1.19** |
bra-miR1885b |
TACATCTTCTCCGCGGAAGCTC |
8990 |
8240 |
0.13 |
bra-MIR5719-p3_1ss21AT |
TCGGATTATCATCACAACACT |
949 |
1608 |
−0.76 |
bra-MIR9563a-p5_1ss17CT |
AGCGGAATATAAGAACTCGTCTCT |
3901 |
2617 |
0.58 |
For comparison of the WA and TG libraries, the conserved unique miRNA sequences were analyzed through Chi square 2 × 2, Fisher's exact and log2 ratio tests of the normalized data. According to the standard of highly significant variation (p < 0.01 from both Chi-square 2 × 2 and Fisher-exact tests and |log2(WA/TG)| ≥ 1), 30 differentially expressed conserved unique sequences (12 upregulated and 18 downregulated in the TG library) were detected in the WA and TG libraries (Fig. 3, Additional file 4: Table S4†). Among these sequences, 5 unique sequences, e.g., ath-miR399c-3p, presented high expression (normalized reads ≥ 1000). In addition, 7 unique sequences exhibited highly differential expression (p < 0.01 and |log2(WA/TG)| ≥ 2) with above-average expression levels (normalized reads ≥ 100), such as bna-miR156e_R+1 and bra-miR398-3p (Tables 1 and 2).
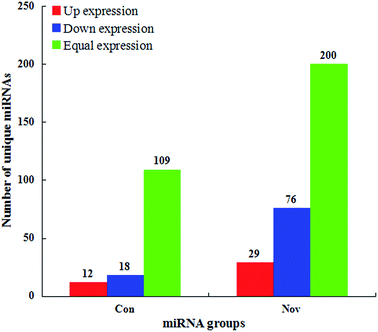 |
| Fig. 3 Comparison of the WA and TG libraries. (Con) conserved unique miRNA sequences; (Nov) novel unique miRNA sequences. (Up expression) up expressed unique miRNA sequences in the TG library; (down expression) down expressed unique miRNA sequences in the TG library; (equal expression) equal expressed unique miRNA sequences in the WA and TG libraries. | |
Table 2 Differentially expressed conserved unique miRNA sequences (p < 0.01 and |log2(WA/TG)| ≥ 1) with above-average expression levels (normalized reads ≥ 100) in the WA and TG libraries
Namea |
Sequence |
WA norm |
TG norm |
log2 ratiob |
Name regulation following Additional file 3: Table S3; red marked miRNAs were detected in degradome analysis. log2(WA norm/TG norm). |
bna-miR156d,e,f,a_R-1 |
TGACAGAAGAGAGTGAGCAC |
291 |
81 |
1.85 |
bna-miR156e_R+1 |
TGACAGAAGAGAGTGAGCACT |
3496 |
687 |
2.35 |
bna-MIR159-p5 |
AGCTGCTAAGCTATGAATCCC |
1078 |
472 |
1.19 |
bna-miR160a,b,c,d |
TGCCTGGCTCCCTGTATGCCA |
104 |
366 |
−1.82 |
bna-MIR162a-p5 |
GGAGGCAGCGGTTGATCGATC |
241 |
91 |
1.41 |
bra-miR168b-3p,c-3p |
CCCGCCTTGCATCAACTGAAT |
87 |
229 |
−1.40 |
bna-MIR169m-p3 |
GCAAGTCGACTTTGGCTCTG |
139 |
30 |
2.21 |
ath-MIR169e-p3_L+1 |
GCAAGTTGACTTTGGCTC |
116 |
21 |
2.47 |
ath-miR171-5p, bna-MIR171g-p5 |
TATTGGCCTGGTTCACTCAGA |
124 |
19 |
2.71 |
bna-miR390a,b,c |
AAGCTCAGGAGGGATAGCGCC |
815 |
408 |
1.00 |
bra-miR391-3p |
ACGGTATCTCTCCTACGTAGC |
77 |
172 |
−1.16 |
bna-miR393_R+1 |
TCCAAAGGGATCGCATTGATCC |
56 |
143 |
−1.35 |
bra-miR398-3p |
TGTGTTCTCAGGTCACCCCTG |
3207 |
39 219 |
−3.61 |
bra-miR398-5p |
GGGTCGACATGAGAACACATG |
21 |
143 |
−2.77 |
bol-miR398a-3p_R-1 |
TGTGTTCTCAGGTCACCCCT |
35 |
366 |
−3.39 |
ath-miR399c-3p |
TGCCAAAGGAGAGTTGCCCTG |
3183 |
1257 |
1.34 |
bna-MIR1140-p5 |
TCCGATTGGCTTTAGGCTGTT |
1526 |
671 |
1.19 |
bra-miR5654a |
ATAAATCCCAAGCATCATCCA |
84 |
192 |
−1.19 |
bra-MIR5719-p5_1ss16AC |
TGTTGTGATGATAATCCGACT |
144 |
330 |
−1.20 |
bna-miR6028_R+2 |
TGGAGAGTAAGGACATTCAGATC |
176 |
72 |
1.29 |
bna-miR6030 |
TCCACCCATACCATACAGACCC |
93 |
370 |
−1.99 |
bna-miR6031 |
AAGAGGTTCGGAGCGGTTTGAAGC |
375 |
118 |
1.67 |
bra-MIR9557-p3_1ss13GA |
AATGAGGGCTGAATTGGAACGC |
170 |
62 |
1.46 |
Identification of novel miRNAs
To identify novel miRNAs, the remaining clean reads (removed conserved miRNAs) mapped to the B. napus genome. A total of 305 novel unique miRNA sequences corresponding to 339 miRNAs and 303 predicted pre-miRNAs were identified. In addition, these predicted pre-miRNAs could form stem-loop structures with a MEFI of at least 0.9 (Additional file 5: Table S5†). Compared with the conserved miRNAs, no highly expressed novel unique miRNA sequences were found in the two libraries; in contrast, most of the unique miRNA sequences presented low expression (normalized reads < 100), and only 22 unique sequences, e.g., PC-3p-9, PC-3p-88, PC-5p-21 and PC-5p-50, exhibited above-average expression (Table 3, Additional file 6: Table S6†). The comparison revealed 105 differentially expressed novel unique miRNA sequences (29 upregulated and 76 downregulated in the TG library) (p < 0.01 and |log2(WA/TG)| ≥ 1), including 8 unique sequences that were identified only in the WA library (Fig. 3, Additional file 6: Table S6†). Among these, 6 differentially expressed unique sequences, for example PC-3p-72, presented above-average expression, and only 1 unique sequence (PC-5p-137) exhibited highly differential expression (p < 0.01 and |log2(WA/TG)| ≥ 2) (Table 3).
Table 3 Novel unique miRNA sequences with above-average expression (normalized reads ≥ 100) in the WA and TG libraries
Namea |
Sequence |
WA norm |
TG norm |
log2 ratiob |
Name regulation following Additional file 5: Table S5; red marked miRNAs were detected in degradome analysis. log2(WA norm/TG norm); ** indicates highly significant variation (p-value < 0.01 from both Chi-square 2 × 2 & Fisher's exact test and |log2(WA/TG)| ≥ 1) between the WA and TG libraries. |
PC-3p-9 |
GCTTTCCCCGGACGACTTTAAATT |
183 |
473 |
−1.37** |
PC-3p-15 |
TATCCCGGACGACCTTAAATT |
209 |
237 |
−0.18 |
PC-3p-20 |
ATTCCGACAAGAACTCCGCCCT |
102 |
212 |
−1.06** |
PC-3p-47 |
AAAGATTGTTGTGGTCTTGTGCCT |
153 |
169 |
−0.14 |
PC-3p-53 |
ATCCATTAGTATCAGACGATC |
156 |
164 |
−0.07 |
PC-3p-72 |
AGAGTCGGGTTCTTATATTCTGCT |
288 |
131 |
1.14** |
PC-3p-88 |
ATATTCCGTTAAGAACTCCACCCT |
215 |
360 |
−0.74 |
PC-3p-101 |
TGCTTTCCCCGGACGACTTTAAAT |
46 |
114 |
−1.31** |
PC-3p-128 |
AACTTCCGCTAAGAACTCCATCCT |
73 |
125 |
−0.78 |
PC-3p-133 |
GGTCATGTCTGACAGCTTCACT |
101 |
66 |
0.61 |
PC-3p-138 |
TAAGAACCCACATTAATCATG |
53 |
105 |
−0.99 |
PC-5p-21 |
TGAACCCAAGATCTCACCCTT |
333 |
377 |
−0.18 |
PC-5p-23 |
AGCGGAATATAAGAACCTGACTCT |
238 |
130 |
0.87 |
PC-5p-50 |
ATCGGAATATAAGAACTCGTCTCT |
353 |
180 |
0.97 |
PC-5p-92 |
ATCGGAATATAAGAAACTGTCTCT |
173 |
97 |
0.83 |
PC-5p-94 |
AACGGAATATAAGAATTTGACTCT |
200 |
122 |
0.71 |
PC-5p-102 |
AGCGGAATATAAGAATTTGTCTCT |
137 |
79 |
0.79 |
PC-5p-110 |
GTTTTGAGAGATTGGGAAGCT |
225 |
69 |
1.71** |
PC-5p-116 |
ACCGGAATATAAGAACTCGTCTCT |
121 |
72 |
0.75 |
PC-5p-127 |
AGCGGAAAATAAGAACTCGTGTCT |
139 |
93 |
0.58 |
PC-5p-137 |
GAGTGAGAAATGGAGTGATGAACA |
339 |
51 |
2.73** |
PC-5p-138 |
AGCGGAATATAAGAACTCGACTCT |
121 |
64 |
0.92 |
Identification and annotation of miRNA targets
To explore the potential miRNA targets and their biological function, a degradome analysis, which is an efficient platform for miRNA-cleaved sequencing combined with bioinformatics-based prediction and annotation, was performed. A total of 31
003
060 and 24
766
218 raw reads were generated from the WA and TG degradome libraries, respectively (Additional file 7: Table S7†). After removing the 3ADT data and reads with lengths <15 nt, the remaining reads were compared with the B. napus cDNA library. A total of 23
597
707 and 18
863
658 mapped cDNA reads corresponding to 6
891
809 and 5
351
033 unique reads were obtained from the two degradome libraries (Additional file 7: Table S7†). The mapped cDNA reads were then compared with the identified miRNAs. Gene annotation [Gene Ontology (GO) and Kyoto Encyclopedia of Genes and Genomes (KEGG) pathway analysis] revealed 741 miRNA targets corresponding to 540 unique target transcripts, which are targeted by 167 unique miRNA sequences, including 81 conserved (Additional file 8: Table S8.1†) and 86 novel sequences (Additional file 8: Table S8.2†), in the two degradome libraries. GO annotation included 3 ontologies: biological process, cellular component, molecular function.47 According to GO annotation, targets corresponding to conserved miRNAs classification revealed 26 categories in biological process were classified, followed by 14 categories for cellular component and 15 categories for molecular function, respectively; while targets corresponding to novel miRNAs classification revealed 12 categories in biological process were classified, followed by 4 categories for cellular component and 11 categories for molecular function, respectively (Fig. 4).
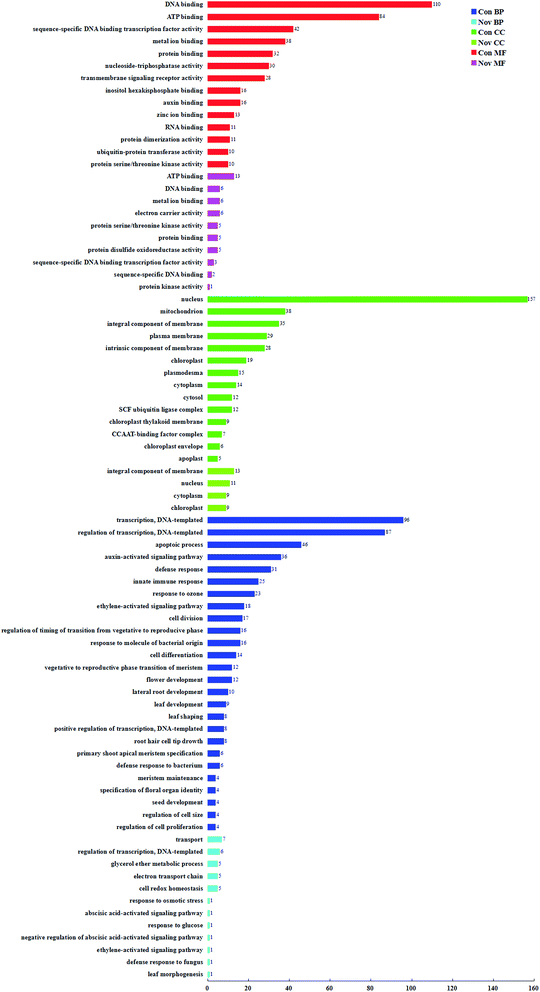 |
| Fig. 4 Classification and count of Gene Ontology (GO) annotation of the miRNA targets in the WA and TG degradome libraries. There are three ontologies in GO: biological process, cellular component, molecular function.47. (Con BP) conserved miRNAs' targets in biological process; (Nov BP) novel miRNAs' targets in biological process; (Con CC) conserved miRNAs' targets in cellular component; (Nov CC) novel miRNAs' targets in cellular component; (Con MF) conserved miRNAs' targets in molecular function; (Nov MF) novel miRNAs' targets in molecular function. | |
To further compare the WA and TG degradome libraries, the high-confidence miRNA targets (categories 0, 1, 2 and 3) were first categorized by gene annotation and then analyzed using the Chi-square 2 × 2 and Fisher's exact tests and log2 ratios based on their abundance values. Of the 441 high-confidence miRNA targets (corresponding to 346 unique target transcripts), 404 (312 target transcripts) were predicted to bind 150 homologous genes, and 37 miRNA targets (34 target transcripts) were absent (Additional file 9: Table S9†). Additionally, 227 differentially expressed high-confidence miRNA targets corresponding to 185 unique target transcripts [97 downregulated miRNA targets (78 downregulated target transcripts) and 130 upregulated miRNA targets (107 upregulated target transcripts) in the TG degradome library] were found in the two degradome libraries (p < 0.01 and |log2(WA TPB/TG TPB)| ≥ 1). Of these miRNA targets, 66 (55 target transcripts) were found only in the WA degradome library, whereas 32 (28 target transcripts) were identified only in the TG degradome library (Additional file 9: Table S9†).
Verification of miRNAs
To verify the miRNA expression profile identified by high-throughput sequencing, 11 differentially expressed unique miRNA sequences at above-average expression (normalized reads ≥ 100) targeting high-confidence transcripts (categories 0, 1, 2 and 3) were selected for verification by quantitative real-time PCR (qRT-PCR). The results nearly agreed with the high-throughput sequencing data: bra-miR168b-3p,c-3p, bna-miR390a,b,c, bna-miR393_R+1, bra-miR398-3p, ath-miR399c-3p and PC-3p-72, were possible differentially expressed in the two libraries (Fig. 5, Additional file 10: Table S10.2†). However, some of them did not exhibit significant differences in two lines in the qRT-PCR verification, such as bna-miR156e_R+1, bna-miR160a,b,c,d and bna-miR6030 (Fig. 5, Additional file 10: Table S10.2†).
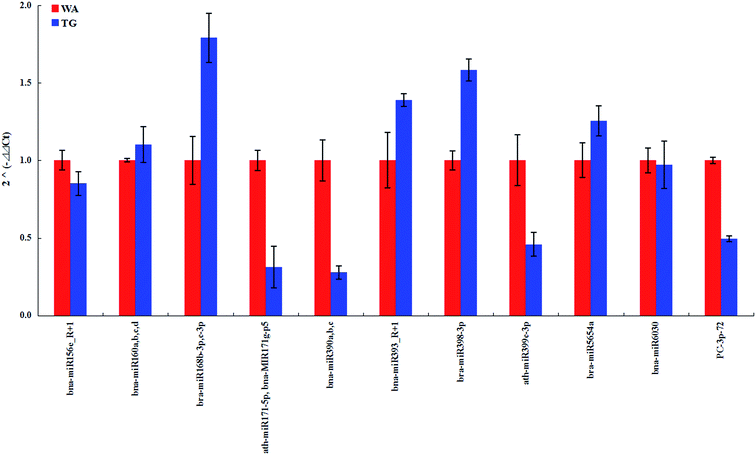 |
| Fig. 5 qRT-PCR validation of 11 potential differentially expressed unique miRNA sequences in the WA and TG lines from high-throughput sequencing. | |
Discussion
Conserved and novel miRNAs
Although high-throughput sequencing is an efficient platform for miRNA identification, it remains incapable of distinguishing highly homologous miRNAs. For example, bna-miR160a, bna-miR160b, bna-miR160c and bna-miR160d, which correspond to the same sequence, had identical raw data (Additional file 3: Table S3.1†). However, through pairwise comparisons, some miRNAs could be recognized. For example, only bna-MIR160c-p3 and bna-MIR160d-p3 were detected, which demonstrates that the sequence only represents bna-miR160c and bna-miR160d because these are from different arms of the same pre-miRNA (Additional file 3: Table S3.1†).
In miRBase 21.0, there are 92 B. napus miRNAs corresponding to 90 pre-miRNAs belonging to 33 miRNA families. As expected, 119 B. napus miRNAs corresponding to 74 pre-miRNAs belonging to 27 miRNA families were identified in this study. Among these miRNAs, 52, including bna-MIR159-p5, bna-MIR166d-p5, bna-MIR167c-p3 and bna-MIR824-p3, are novel strand miRNAs that map to B. napus pre-miRNAs but not B. napus miRNAs because they are generated from the opposite pre-miRNA arm (Additional file 3: Table S3.1†). Furthermore, the analysis also revealed 66 miRNAs (including 24 novel strand miRNAs) corresponding to 47 pre-miRNAs belonging to 33 miRNA families that are included in miRBase 21.0 as detected in other Brassicaceae species, i.e., Brassica rapa, Brassica oleracea, Arabidopsis thaliana and Arabidopsis lyrata, and 24 of the miRNA families are not found in the B. napus data included in miRBase 21.0, particularly the highly expressed miRNAs (normalized reads ≥ 1000) miR158 (e.g., bra-miR158-3p), miR398 (e.g., bra-miR398-3p), miR1885 (e.g., bra-miR1885b), miR5719 (e.g., bra-MIR5719-p3_1ss21AT) and miR9563 (e.g., bra-MIR9563a-p5_1ss17CT) (Additional file 3: Table S3.2†). Based on the analysis of miRNA conservation in related species and the qRT-PCR validation, the results indicated that bna-MIR171g-p5, bra-miR168b-3p,c-3p, bra-miR398-3p, ath-miR399c-3p and bra-miR5654a might exist in B. napus. However, most of the conserved-novel and novel miRNAs presented a low expression level, and most of their targets were in Category 4 (low confidence) (Additional file 3: Table S3.3, Additional file 5: Table S5, Additional file 8: Table S8†) as same as previously reported in other plants.15,18–25,29–33,43–46 Therefore, only 1 novel miRNA (i.e., PC-3p-72) targeting high-confidence transcripts at above-average expression was verified by qRT-PCR. These results suggest that PC-3p-72 might exist in B. napus.
miRNA targets
To evaluate the accuracy of the predicted miRNA–target interactions, the miRNA targets were analyzed by degradome sequencing combined with bioinformatics analysis. In total, 540 targets, including 441 high-confidence targets, were detected. The results demonstrate that many homologous and heterogeneous targets are cleaved by the same unique miRNA sequence, indicating that the same miRNA may be involved in multiple biological activities, as previously reported. For example, SPL2, SPL6, SPL9, SPL10, SPL11, SPL13A and SPL15 (squamosa promoter-binding-like protein family) are targeted by bna-miR156e_R+1, whereas AFB2, AFB3 (auxin signaling F-box family), BH076, BH077 (transcription factor bHLH family), GRH1 (GRR1-like protein family) and TIR1 (transport inhibitor response family) are targeted by bna-miR393_R+1 (Additional file 9: Table S9†). In contrast, many homologous miRNAs usually target homologous and similar targets. For example, TOE2 and TOE3 (AP2-like ethylene-responsive transcription factor TOE family) are cleaved by bna-miR172a, bna-miR172b,c and bna-miR172a_R-1,d_R-1 (bna-miR172 family), and three transcripts, namely BnaA02g06490D, BnaA10g12950D and BnaC09g35430D, which correspond to TOE2, are cleaved by bna-miR172a, bna-miR172b,c and bna-miR172a_R-1,d_R-1 (bna-miR172 family), respectively (Additional file 9: Table S9†). Interestingly, homologous and similar targets are occasionally cleaved by heterogenetic miRNAs; for example, the BnaC09g37630D transcript corresponding to PPR37 (pentatricopeptide repeat-containing protein At1g12620) is targeted by bna-miR161 and bra-miR5654a, and the BnaA03g45020D transcript corresponding to Y4117 (putative disease resistance protein At4g11170) is targeted by bra-miR1885b and bna-MIR6028-p3 (Additional file 9: Table S9†). Similar to miRNA high-throughput sequencing, degradome sequencing is also unable to distinguish highly homologous sequences corresponding to identical cleavage sites, such as the BnaAnng07000D transcript, which is cleaved by bna-miR164a and bna-miR164b,c,d at position 817, in agreement with the raw data (Additional file 9: Table S9†).
Potential differences in miRNA expression
In total, 135 unique miRNA sequences that are differentially expressed (p < 0.01 and |log2(WA/TG)| ≥ 1) between the WA and TG libraries were identified in the present study. Of these sequences, 41 (12 conserved and 29 novel) are significantly upregulated in the TG library, and 94 (18 conserved and 76 novel) are significantly downregulated. Among these unique sequences, 13 (12 conserved and 1 novel) that were predicted to target transcripts and belonged to high-confidence categories (0, 1, 2 and 3) presented above-average expression (normalized reads ≥ 100). Therefore, 11 of these unique miRNA sequences were verified by qRT-PCR, which suggested that most of them, particularly bra-miR168b-3p,c-3p, bna-miR390a,b,c, bna-miR393_R+1, bra-miR398-3p, ath-miR399c-3p and PC-3p-72, were differentially expressed in the two libraries. Vaucheret (2006, 2009) reported that miR168 family response to endogenous or environmental fluctuations through targeting AGO1.48,49 Jiang et al. (2014) suggested that bra-miR168a-3p and bra-miR168b-3p were up-regulated in the pollen development of the male fertile Brassica campestris line ‘Bcajh97-01B’ (vs. line ‘Bcajh97-01A’).31 In our study, the unique sequences of the bra-miR168b-3p,c-3p detected by high-throughput sequencing and qRT-PCR had possible differentially expressed in the TG and WA libraries. In addition, degradome analysis shown that bra-miR168b-3p,c-3p might target NADH-cytochrome b5 reductase 1 (NB5R1) involved in amino sugar and nucleotide sugar metabolism (Additional file 8 and 9: Table S8 and S9†). Previous studies indicated that miR390 and miR393 family take part in the leaf development in Arabidopsis thaliana (A. thaliana) and other plants, particularly miR390 and miR393 family could regulate the auxin level, because miR390 family targets auxin response factors family (ARF) and miR393 family targets auxin signaling F-box family (AFB) and transport inhibitor response 1 (TIR1).50–58 In the present study, we found that bna-miR390a,b,c and bna-miR393_R+1 also have potential differentially expression in the two libraries. In addition, bna-miR390a,b,c might target PHD finger protein ALFIN-LIKE 7 (ALFL7), and bna-miR393_R+1 might not only target AFB, TIR1 but also GRR1-like protein 1 (GRH1) (Additional file 8 and 9: Table S8 and S9†). According to previous researches, miR398 family plays an important role in response and resistance mechanism, especially thermotolerance, in A. thaliana.59–62 Yu et al. (2012) discovered that bra-miR398 might are responsive to heat stress in Brassica rapa.63 The annotation of the bra-miR398-3p target (BnaC09g42920D) in this study was not clear, although bra-miR398-3p highly significant differentially expression between the TG and WA libraries (Additional file 8 and 9: Table S8 and S9†). A potential novel miRNA PC-3p-72 also differentially expressed in two libraries. It might target tonoplast dicarboxylate transporter (TDT) involved in intracellular pH regulation (Additional file 8 and 9: Table S8 and S9†). In short, the results of high-throughput sequencing and qRT-PCR verification showed that the possible potential differences in miRNA expression between transgenic B. napus and its acceptor (Westar).
Conclusions
In summary, through high-throughput sequencing and degradome analysis, the present study identified numerous conserved and novel unique miRNA sequences and their corresponding targets in transgenic B. napus and its acceptor (Westar). These results contribute valuable information for further increases in the number of identified miRNAs and the identification of their target transcripts in B. napus. Furthermore, our results also suggest that some miRNAs might be differentially expressed between transgenic B. napus and its acceptor. Moreover, differential miRNA expression might lead to differential target degradation.
Competing interests
The authors declare that they have no competing interests.
Authors' contributions
CT, MY, and YY contributed to the experiment design and management. CT, HZ and GL performed the experiments. CT, FW, YP and RY participated in the data analyses. CT written the manuscript. All authors checked and approved the manuscript.
Abbreviations
WA | Brassica napus cv. Westar |
TG | Transgenic B. napus |
sRNA | Small RNA |
miRNAs | MicroRNA |
nt | Nucleotides |
PTGS | Post-transcriptional gene silencing |
pri-miRNA | Primary miRNA |
pre-miRNA | Precursor miRNA |
DCL 1 | Dicer-like 1 |
HYL 1 | Hyponastic leaves 1 |
SE | Serrate |
RISC | RNA-induced silencing complex |
AGO | Argonaute |
ESTs | Expressed sequence tags |
TCs | Tentative consensus sequences |
ADTs | Adapters |
MFEIs | Minimal folding energy indexes |
GO | Gene ontology |
KEGG | Kyoto encyclopedia of genes and genomes |
Acknowledgements
This work was financially supported by the Program for Changjiang Scholars and Innovative Research Team in University (IRT_14R27) and the National Natural Science Foundation of China (NSFC). We appreciated Dr Song Chen for providing the seedlings of the transgenic B. napus and its acceptor (B. napus cv. Westar).
References
- D. P. Bartel, MicroRNAs: genomics, biogenesis, mechanism, and function, Cell, 2004, 116(2), 281–297 CrossRef CAS PubMed.
- B. Khraiwesh, M. A. Arif and G. I. Seumel, et al., Transcriptional control of gene expression by microRNAs, Cell, 2010, 140(1), 111–122 CrossRef CAS PubMed.
- V. Ambros, microRNAs: tiny regulators with great potential, Cell, 2001, 107(7), 823–826 CrossRef CAS PubMed.
- V. Ambros, B. Bartel and D. P. Bartel, et al., A uniform system for microRNA annotation, RNA, 2003, 9(3), 277–279 CrossRef CAS PubMed.
- M. W. Jones-Rhoades, D. P. Bartel and B. Bartel, MicroRNAs and their regulatory roles in plants, Annu. Rev. Plant Biol., 2006, 57, 19–53 CrossRef CAS PubMed.
- Y. Kurihara and Y. Watanabe, Arabidopsis micro-RNA biogenesis through Dicer-like 1 protein functions, Proc. Natl. Acad. Sci. U. S. A., 2004, 101(34), 12753–12758 CrossRef CAS PubMed.
- A. C. Mallory, T. Elmayan and H. Vaucheret, MicroRNA maturation and action—the expanding roles of Argonautes, Curr. Opin. Plant Biol., 2008, 11(5), 560–566 CrossRef CAS PubMed.
- S. Griffiths-Jones, R. J. Grocock and S. van Dongen, et al., miRBase: microRNA sequences, targets and gene nomenclature, Nucleic Acids Res., 2006, 34, D140–D144 CrossRef CAS PubMed.
- S. Griffiths-Jones, H. K. Saini and S. van Dongen, et al., miRBase: tools for microRNA genomics, Nucleic Acids Res., 2008, 36, D154–D158 CrossRef CAS PubMed.
- A. Kozomara and S. Griffiths-Jones, miRBase: integrating microRNA annotation and deep-sequencing data, Nucleic Acids Res., 2011, 39(Database issue), D152–D157 CrossRef CAS PubMed.
- M. Hajduch, J. E. Casteel and K. E. Hurrelmeyer, et al., Proteomic analysis of seed filling in Brassica napus. Developmental characterization of metabolic isozymes using high-resolution two-dimensional gel electrophoresis, Plant Physiol., 2006, 141(1), 32–46 CrossRef CAS PubMed.
- M. A. German, M. Pillay and D. H. Jeong, et al., Global identification of microRNA–target RNA pairs by parallel analysis of RNA ends, Nat. Biotechnol., 2008, 26(8), 941–946 CrossRef CAS PubMed.
- B. D. Gregory, R. C. O'Malley and R. Lister, et al., A link between RNA metabolism and silencing affecting Arabidopsis development, Dev. Cell, 2008, 14(6), 854–866 CrossRef CAS PubMed.
- C. Addo-Quaye, T. W. Eshoo and D. P. Bartel, et al., Endogenous siRNA and miRNA targets identified by sequencing of the Arabidopsis degradome, Curr. Biol., 2008, 18(10), 758–762 CrossRef CAS PubMed.
- V. Pantaleo, G. Szittya and S. Moxon, et al., Identification of grapevine microRNAs and their targets using high-throughput sequencing and degradome analysis, Plant J., 2010, 62(6), 960–976 CAS.
- J. Dupont, P. J. White and K. M. Johnston, et al., Food safety and health effects of canola oil, J. Am. Coll. Nutr., 1989, 8(5), 360–375 CrossRef CAS PubMed.
- F. L. Xie, S. Q. Huang and K. Guo, et al., Computational identification of novel microRNAs and targets in Brassica napus, FEBS Lett., 2007, 581(7), 1464–1474 CrossRef CAS PubMed.
- A. Buhtz, F. Springer and L. Chappell, et al., Identification and characterization of small RNAs from the phloem of Brassica napus, Plant J., 2008, 53(5), 739–749 CrossRef CAS PubMed.
- A. P. Körbes, R. D. Machado and F. Guzman, et al., Identifying conserved and novel microRNAs in developing seeds of Brassica napus using deep sequencing, PLoS One, 2012, 7(11), e50663 CrossRef PubMed.
- M. Y. Xu, Y. Dong and Q. X. Zhang, et al., Identification of miRNAs and their targets from Brassica napus by high-throughput sequencing and degradome analysis, BMC Genomics, 2012, 13, 421 CrossRef CAS PubMed.
- D. Huang, C. Koh and J. A. Feurtado, et al., MicroRNAs and their putative targets in Brassica napus seed maturation, BMC Genomics, 2013, 14, 140 CrossRef CAS PubMed.
- Y. T. Zhao, M. Wang and S. X. Fu, et al., Small RNA profiling in two Brassica napus cultivars identifies microRNAs with oil production- and development-correlated expression and new small RNA classes, Plant Physiol., 2012, 158(2), 813–823 CrossRef CAS PubMed.
- Z. S. Zhou, J. B. Song and Z. M. Yang, Genome-wide identification of Brassica napus microRNAs and their targets in response to cadmium, J. Exp. Bot., 2012, 63(12), 4597–4613 CrossRef CAS PubMed.
- D. Shen, I. Suhrkamp and Y. Wang, et al., Identification and characterization of microRNAs in oilseed rape (Brassica napus) responsive to infection with the pathogenic fungus Verticillium longisporum using Brassica AA (Brassica rapa) and CC (Brassica oleracea) as reference genomes, New Phytol., 2014, 204(3), 577–594 CrossRef CAS PubMed.
- S. S. Verma, M. H. Rahman and M. K. Deyholos, et al., Differential expression of miRNAs in Brassica napus root following infection with Plasmodiophora brassicae, PLoS One, 2014, 9(1), e86648 CrossRef PubMed.
- B. Chalhoub, F. Denoeud and S. Liu, et al., Plant genetics. Early allopolyploid evolution in the post-Neolithic Brassica napus oilseed genome, Science, 2014, 345(6199), 950–953 CrossRef CAS PubMed.
- S. Chen, H. M. Pu and J. F. Zhang, et al., Identification of High Oleic Acid Germplasm from the T2 Progeny of the Transgenic Brassica napus L., Jiangsu J. Agric. Sci., 2009, 25(6), 1234–1237 Search PubMed.
- D. Li, L. Wang and X. Liu, et al., Deep sequencing of maize small RNAs reveals a diverse set of microRNA in dry and imbibed seeds, PLoS One, 2013, 8(1), e55107 CrossRef CAS PubMed.
- H. Liu, C. Qin and Z. Chen, et al., Identification of miRNAs and their target genes in developing maize ears by combined small RNA and degradome sequencing, BMC Genomics, 2014, 15, 25 CrossRef PubMed.
- Y. Zhuang, X. H. Zhou and J. Liu, Conserved miRNAs and their response to salt stress in wild eggplant Solanum linnaeanum roots, Int. J. Mol. Sci., 2014, 15(1), 839–849 CrossRef PubMed.
- J. X. Jiang, M. L. Lv and Y. Liang, et al., Identification of novel and conserved miRNAs involved in pollen development in Brassica campestris ssp. chinensis by high-throughput sequencing and degradome analysis, BMC Genomics, 2014, 15, 146 CrossRef PubMed.
- N. Liu, J. Yang and S. Guo, et al., Genome-wide identification and comparative analysis of conserved and novel microRNAs in grafted watermelon by high-throughput sequencing, PLoS One, 2013, 8(2), e57359 CrossRef CAS PubMed.
- J. H. Yang, X. Y. Liu and B. C. Xu, et al., Identification of miRNAs and their targets using high-throughput sequencing and degradome analysis in cytoplasmic male-sterile and its maintainer fertile lines of Brassica juncea, BMC Genomics, 2013, 14, 9 CrossRef PubMed.
- B. C. Meyers, M. J. Axtell and B. Bartel, et al., Criteria for Annotation of Plant MicroRNAs, Plant Cell, 2008, 20(12), 3186–3190 CrossRef CAS PubMed.
- S. Griffiths-Jones, H. K. Saini and S. van Dongen, et al., MiRBase: tools for microRNA genomics, Nucleic Acids Res., 2008, 36, D154–D158 CrossRef CAS PubMed.
- Z. J. Yin, C. H. Li and M. L. Han, et al., Identification of conserved microRNAs and their target genes in tomato (Lycopersicon esculentum), Gene, 2008, 414(1–2), 60–66 CrossRef CAS PubMed.
- M. Zuker, Mfold web server for nucleic acid folding and hybridization prediction, Nucleic Acids Res., 2003, 31(13), 3406–3415 CrossRef CAS PubMed.
- E. Bonnet, J. Wuyts and P. Rouze, et al., Evidence that microRNA precursors, unlike other non-coding RNAs, have lower folding free energies than random sequences, Bioinformatics, 2004, 20(17), 2911–2917 CrossRef CAS PubMed.
- Z. Ma, C. Coruh and M. J. Axtell, Arabidopsis lyrata small RNAs: transient MIRNA and small interfering RNA loci within the Arabidopsis genus, Plant Cell, 2010, 22(4), 1090–1103 CrossRef CAS PubMed.
- C. Addo-Quaye, W. Miller and M. J. Axtell, CleaveLand: a pipeline for using degradome data to find cleaved small RNA targets, Bioinformatics, 2009, 25(1), 130–131 CrossRef CAS PubMed.
- S. Audic and J. M. Claverie, The significance of digital gene expression profiles, Genome Res., 1997, 7(10), 986–995 CrossRef CAS PubMed.
- Y. Benjamini and D. Yekutieli, The control of the false discovery rate in multiple testing under dependency, Annals of Statistics, 2001, 29, 1165–1188 CrossRef.
-
(a) C. Chen, J. M. Unrine and J. D. Judy, et al., Toxicogenomic Responses of the Model Legume Medicago truncatula to Aged Biosolids Containing a Mixture of Nanomaterials (TiO2, Ag, and ZnO) from a Pilot Wastewater Treatment Plant, Environ. Sci. Technol., 2015, 49(14), 8759–8768 CrossRef CAS PubMed;
(b) R. Rajagopalan, H. Vaucheret and J. Trejo, et al., A diverse and evolutionarily fluid set of microRNAs in Arabidopsis thaliana, Genes Dev., 2006, 20(24), 3407–3425 CrossRef CAS PubMed.
- N. Fahlgren, M. D. Howell and K. D. Kasschau, et al., High-throughput sequencing of Arabidopsis microRNAs: evidence for frequent birth and death of MIRNA genes, PLoS One, 2007, 2(2), e219 CrossRef PubMed.
- D. Li, L. Wang and X. Liu, et al., Deep sequencing of maize small RNAs reveals a diverse set of microRNA in dry and imbibed seeds, PLoS One, 2013, 8(1), e55107 CrossRef CAS PubMed.
- Y. Yao, G. Guo and Z. Ni, et al., Cloning and characterization of microRNAs from wheat (Triticum aestivum L.), Genome Biol., 2007, 8(6), R96 CrossRef PubMed.
- M. Ashburner, C. A. Ball and J. A. Blake, et al., Gene ontology: tool for the unification of biology. The Gene Ontology Consortium, Nat. Genet., 2000, 25(1), 25–29 CrossRef CAS PubMed.
- H. Vaucheret, A. C. Mallory and D. P. Bartel, AGO1 homeostasis entails coexpression of MIR168 and AGO1 and preferential stabilization of miR168 by AGO1, Mol. Cell, 2006, 22(1), 129–136 CrossRef CAS PubMed.
- H. Vaucheret, AGO1 homeostasis involves differential production of 21-nt and 22-nt miR168 species by MIR168a and MIR168b, PLoS One, 2009, 4(7), e6442 CrossRef PubMed.
- E. Allen, Z. Xie and A. M. Gustafson, et al., microRNA-directed phasing during trans-acting siRNA biogenesis in plants, Cell, 2005, 121(2), 207–221 CrossRef CAS PubMed.
- L. Williams, C. C. Carles and K. S. Osmont, et al., A database analysis method identifies an endogenous trans-acting short-interfering RNA that targets the Arabidopsis ARF2, ARF3, and ARF4 genes, Proc. Natl. Acad. Sci. U. S. A., 2005, 102(27), 9703–9708 CrossRef CAS PubMed.
- M. S. Krasnikova, D. V. Goryunov and A. V. Troitsky, et al., Peculiar evolutionary history of miR390-guided TAS3-like genes in land plants, Sci. World J., 2013, 2013, 924153 Search PubMed.
- S. H. Cho, C. Coruh and M. J. Axtell, miR156 and miR390 regulate tasiRNA accumulation and developmental timing in Physcomitrella patens, Plant Cell, 2012, 24(12), 4837–4849 CrossRef CAS PubMed.
- M. J. Iglesias, M. C. Terrile and D. Windels, et al., MiR393 regulation of auxin signaling and redox-related components during acclimation to salinity in Arabidopsis, PLoS One, 2014, 9(9), e107678 CrossRef PubMed.
- D. Windels, D. Bielewicz and M. Ebneter, et al., miR393 is required for production of proper auxin signalling outputs, PLoS One, 2014, 9(4), e95972 CrossRef PubMed.
- Z. H. Chen, M. L. Bao and Y. Z. Sun, et al., Regulation of auxin response by miR393-targeted transport inhibitor response protein 1 is involved in normal development in Arabidopsis, Plant Mol. Biol., 2011, 77(6), 619–629 CrossRef CAS PubMed.
- A. Si-Ammour, D. Windels and E. Arn-Bouldoires, et al., miR393 and secondary siRNAs regulate expression of the TIR1/AFB2 auxin receptor clade and auxin-related development of Arabidopsis leaves, Plant Physiol., 2011, 157(2), 683–691 CrossRef CAS PubMed.
- G. Parry, L. I. Calderon-Villalobos and M. Prigge, et al., Complex regulation of the TIR1/AFB family of auxin receptors, Proc. Natl. Acad. Sci. U. S. A., 2009, 106(52), 22540–22545 CrossRef CAS PubMed.
- R. Sunkar, A. Kapoor and J. K. Zhu, Posttranscriptional induction of two Cu/Zn superoxide dismutase genes in Arabidopsis is mediated by downregulation of miR398 and important for oxidative stress tolerance, Plant Cell, 2006, 18(8), 2051–2065 CrossRef CAS PubMed.
- C. Zhu, Y. Ding and H. Liu, MiR398 and plant stress responses, Physiol. Plant., 2011, 143(1), 1–9 CrossRef CAS PubMed.
- X. Lu, Q. Guan and J. Zhu, Downregulation of CSD2 by a heat-inducible miR398 is required for thermotolerance in Arabidopsis, Plant Signaling Behav., 2013, 8(8), e24952 CrossRef PubMed.
- Q. Guan, X. Lu and H. Zeng, et al., Heat stress induction of miR398 triggers a regulatory loop that is critical for thermotolerance in Arabidopsis, Plant J., 2013, 74(5), 840–851 CrossRef CAS PubMed.
- X. Yu, H. Wang and Y. Lu, et al., Identification of conserved and novel microRNAs that are responsive to heat stress in Brassica rapa, J. Exp. Bot., 2012, 63(2), 1025–1038 CrossRef CAS PubMed.
Footnotes |
† Electronic supplementary information (ESI) available. See DOI: 10.1039/c5ra14672k |
‡ These authors contributed equally. |
|
This journal is © The Royal Society of Chemistry 2015 |
Click here to see how this site uses Cookies. View our privacy policy here.