DOI:
10.1039/C6AY01575A
(Minireview)
Anal. Methods, 2016,
8, 6591-6601
Recent approaches for optical smartphone sensing in resource-limited settings: a brief review
Received
3rd June 2016
, Accepted 9th August 2016
First published on 11th August 2016
Abstract
Developments in the emerging fields of smartphone chemical and biosensing have dovetailed with increased interest in environmental and health monitoring for resource-limited environments, culminating in research toward field-ready smartphone sensors. Optical sensors have been a particular focus, in which smartphone imaging and on-board analysis have been integrated into both existing and novel colorimetric, fluorescent, chemiluminescent, spectroscopy-based, and scattering-based assays. Research in recent years has shown promising progress, but substantial limitations still exist due to environmental lighting interference, reliance upon proprietary smartphone attachments, and the undefined sensitivity variations between different smartphones. In this review, recent research in smartphone chemical and biosensing is assessed, and discussion is made regarding the opportunities that new research methods have to improve the scope of resource-limited sensing.
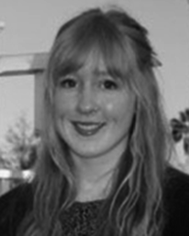 Katherine E. McCracken | Katherine E. McCracken received her B.S. degree in biosystems engineering from the University of Arizona. Currently, she is pursuing her doctorate in agricultural and biosystems engineering at the University of Arizona. Her research focuses on smartphone biosensors for environmental monitoring. Prior to her graduate studies, she served as a United States Peace Corps volunteer in Dodoma, Tanzania. |
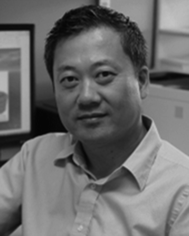 Jeong-Yeol Yoon | Dr Jeong-Yeol Yoon is Professor at the University of Arizona, Tucson, AZ, USA. His research interests include the development of field-deployable or point-of-care biosensor devices for food, environmental, and clinical applications, utilizing smartphone, paper microfluidics, and droplet microfluidics. He is President of Institute of Biological Engineering (IBE) for the calendar year 2015 and is currently Editor-in-Chief of Journal of Biological Engineering (IBE's official journal). |
Introduction
Over the past decade, the revolution and widespread adoption of smartphone technology has spurred research interest in designing smartphone-integrated point-of-care (POC) chemical and biosensors, the history and development of which are extensively surveyed in several topical reviews.1–6 On their own, these portable sensors are designed to identify the presence and concentration of chemical and biological targets, or analytes, based on their interactions with target-specific agents, such as dyes, enzymes, antibodies, and aptamers. These interactions are expressed and thus sensed in a number of ways, including optically, as with colorimetric or fluorescent chemo- and immunosensors, or electrically, as with potentiostat-controlled or interdigitated microelectrode immunosensors.7–11 Smartphone devices have been used in concert with both platform varieties, serving in roles between simple readers and instrument interfaces to fully-integrated sensors, acting as detectors, data processors, and even signal inducers in one pocket-sized package.2,3,6,12–14 With rapid improvements in smartphone optical hardware, though, research has favored the exploration of smartphones as standalone optical sensing platforms for colorimetric, fluorescent, spectroscopic, scattering, and microscopy-based applications.6
Overall improvements in smartphone on-board processing, dual-camera designs, as well as GPS and mobile data connectivity have evolved in parallel with increasingly low-cost devices for emerging markets worldwide.15–17 Thus, recent research has expressed particular interest in adapting POC smartphone sensing techniques for “resource-limited” settings, such as field sites in rural or developing regions.5,18–20 These circumstances are removed from traditional laboratory infrastructure, such as electricity, refrigeration, sanitation, controlled lighting, and stationary precision equipment.21 However, outside of a controlled environment context, variable factors such as ambient lighting, temperature, and humidity can interfere dramatically with chemical and biosensor assays.22–26 Additionally, in-field or in situ sample matrices are frequently complex in nature, containing solid or dissolved materials such as dust, algae, or proteins, that can potentially interfere with or shroud analyte-reagent signals. Much research has led to situational-specific optimized platform layouts, sample preparation techniques, and normalization procedures that help to build on the strengths of smartphones as sensors, but limitations remain in their adaptability. Despite improvements since their early mainstream adoption, smartphones still lack the full sensitivity of designated sensor instrumentation. Compensation must be made for these limitations, and this begins with an assessment of the overall challenges that recent sensing methods have faced.
In this review, we hope to illuminate how recent research in smartphone chemical and biosensing has strived to accommodate robust field detection under disruptive conditions, and discuss the potential technical challenges that must still be overcome to allow sensing in resource-limited environments. Although great strides have been made within individual research groups and among individual sensor targets, a more concerted and broader approach is needed if we are to reach our ultimate goal of truly universal smartphone sensors for pocket-sized and all-in-one data collection, processing, and rapid results-sharing.
Recent optical smartphone sensors
Smartphone-integrated sensor platforms
Smartphones are typically integrated into an optical chemical or biosensing platform through the use of an attached lighting control enclosure that closely fits the device and the assay platform (i.e. strip, cassette, cuvette, etc.) (Fig. 1). In this configuration, the smartphone can function as an illumination source (LED flash), a signal detector (CMOS-based camera), and a signal processor (software application or app). Based on the conditions of the assay, various lenses, filters, diffraction gratings, and alternative light or power sources may be incorporated within the attached enclosure to enhance signal detection from colorimetric, fluorescence-based, chemi/bioluminescence-based, and scattering-based assays. There are, however, several exceptions that utilize image-processing algorithms rather than enclosure attachments. These are further discussed in the Challenges and future needs section.27–29
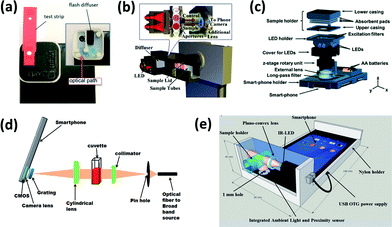 |
| Fig. 1 Examples of smartphone-integrated sensor components and layouts for (a) cholesterol detection by colorimetry (reproduced from ref. 34 with permission from the Royal Society of Chemistry); (b) peanut allergen detecting by colorimetry (reproduced from ref. 53 with permission from the Royal Society of Chemistry); (c) fluorescent cyst detection/microscopy (reproduced from ref. 84 with permission from the Royal Society of Chemistry); (d) protein/enzyme detection by gold nanoparticles using LSPR (reproduced from ref. 119 with permission from the Royal Society of Chemistry); (e) scattering detection of turbidity (reproduced from ref. 96 with permission from the Royal Society of Chemistry). | |
Comparison of these four optical sensing modalities is graphically illustrated in Fig. 2.
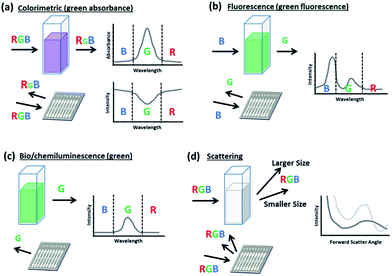 |
| Fig. 2 Typical optical detection for both light transmitted through liquid sample platforms (i.e. cuvette, well plate) and light reflected from solid sample platforms (i.e. test strip, cassette) using (a) colorimetric assays, (b) fluorescence-based assays, (c) bio- and chemiluminescence assays, and (d) scattering-based assays. | |
Colorimetric sensors
In their basic form, colorimetric assays evaluate changes in the absorbance or reflected intensity of analyte-reagent complexes. These changes are commonly due to structural shifts or plasmon resonance phenomena which lead to a shift in the sample's optical properties typically over a wide range of wavelengths.30,31 Since conventional smartphones rely on a CMOS array to assign RGB values to each pixel that it registers, the smartphone is perhaps best equipped to monitor for these broad color shifts since they do not require stringent controls or filters, which can be needed in fluorescence detection to screen for fine changes at a peak wavelength. Thus, simple colorimetric smartphone sensors only require lighting and image processing for unfiltered detection (Fig. 1a). More complex colorimetric smartphone sensors may also incorporate additional lenses and separate lighting sources more specifically attuned to the analyte-reagent absorbance spectrum (Fig. 1b).
Smartphone sensing has been most commonly demonstrated with colorimetric assays for a wide variety of targets using both novel and well-characterized techniques (Table 1). Recent smartphone-integrated colorimetric assays have been demonstrated for organic molecules (furfural,32 toxins,33 hormones,34,35 formaldehyde,36 carbohydrates37–41), ions and inorganic molecules (pH,28,42,43 fluoride,44 chlorine derivatives27), heavy metal targets (Cr species,45 Fe,46 Pb and Cu species,47 Hg48), biological targets (nucleic acids,25,49 enzyme activity,50 antigens,51,52 allergens53), dissolved gases,54,55 as well as radiometric and photometric interactions (ultraviolet radiation,56 soil hue57). These have been detected primarily from liquid media, ranging from buffer solutions to more complex and direct sampling matrices, including blood, sweat, and beverages.
Table 1 Recent highlights in smartphone-integrated assays
Assay type |
Target(s) |
Sample platform |
Sample matrix |
Range of detection |
Assay time |
Smartphone |
Device integrationa |
Reference |
Smartphone integration methods: [A]: light source (flash), [B]: attached enclosure, [C]: imaging, [D]: processing (on-board app), [E]: attached electrical device, [F]: video.
|
Colorimetric |
Chlorine |
Tube |
Water |
0.3–1.0 mg L−1 |
1.9 s |
Samsung Galaxy S GT-19000 |
[C], [D] |
Sumriddetchkajorn et al.27 |
Colorimetric |
Cholesterol |
Commercial strip (CardioChek) |
Blood |
140–400 mg dL−1 |
1 min |
iPhone (unspecified) |
[A], [B], [C], [D] |
Oncescu et al.34 |
Colorimetric |
Cr(III), Cr(VI) |
Tube |
DMSA–Au NP |
10–500 nM |
5 min |
Unspecified |
[C] |
Chen et al.45 |
Colorimetric |
DENV DNA |
— |
SSC buffer–Au NP |
5–50 nM |
10 min |
Unspecified |
[B], [C], [D] |
Choi et al.25 |
Colorimetric |
Fluoride |
Tube |
Groundwater |
0.1–2 mg L−1 |
<1 min |
ASUS Zenphone; Moto G; Samsung DUOS |
[A], [B], [C], [D] |
Levin et al.44 |
Colorimetric |
Formaldehyde |
Tube |
Air sample, dissolved |
1–600 μM |
<4 min |
Samsung Galaxy Note II |
[B], [C], [D] |
Yang et al.36 |
Colorimetric |
Furfural |
Polymeric film |
Beer |
39–500 μg L−1 |
60 min |
Samsung Galaxy S4 |
[B], [C], [D] |
Rico-Yuste et al.32 |
Colorimetric |
Glucose |
PET film chip |
Blood |
3–1000 mg dL−1 |
10 min |
Samsung (unspecified) |
[B], [C], [D] |
Devadhasan et al.38 |
Colorimetric |
Glucose; lactate |
Paper analytical device |
PBS |
0.9–8 mM; 0.06–0.50 mM |
60 s |
Unspecified |
[A], [B], [C] |
Im et al.37 |
Colorimetric |
HIV, (non)treponemal syphilis antigens |
Casette |
Blood |
± Serum classification |
15 min |
iPod Touch |
[E] |
Laksanasopin et al.51 |
Colorimetric |
Peanut allergen (unspecified) |
Commercial kit (Veratox-Neogen kit) |
Water |
1–25 mg L−1 |
20 min |
Samsung Galaxy SII |
[B], [C], [D] |
Coskun et al.53 |
Colorimetric |
Saxitoxin; okadaic acid |
ELISA microplate |
ELISA reagents |
0.02–0.32 μg L−1; 0.2–5 μg L−1 |
<100 min |
iPhone 4S |
[B], [C], [D] |
Su et al.33 |
Colorimetric |
Ultraviolet light |
Photochromic card |
Ormosil |
1–12 UVI |
<30 s |
Samsung Galaxy Grand 2 |
[C], [D] |
Meng et al.56 |
Fluorescent |
Collegenase, trypsin |
Well plate |
TESCA |
3.75–40 μg mL−1; 3.72 ng mL−1 to 1.2 μg mL−1 |
<90 min |
HTC EVO 3D |
[B], [C], [D] |
Wargocki et al.66 |
Fluorescent |
Dissolved O2, pH |
Chitosan film |
Water |
pH 4–8; 5–100% pO2 |
<60 s |
iPhone 5S |
[C] |
Xu et al.29 |
Fluorescent |
HSV-2 virus |
PCR (LAMP) |
DMEM |
21–2100 PFU mL−1 |
<1 h |
Samsung Galaxy S3 |
[A], [B], [C] |
Liao et al.81 |
Fluorescent |
Ochratoxin A |
Commercial columns (Ochraprep) |
Beer |
2 μg L−1 |
5 min |
iPhone 4S |
[B], [C] |
Bueno et al.72 |
Fluorescent |
pH |
Tube |
DI, chemosensor dye |
pH 7–9, Δ0.12 pH unit |
Unspecified |
Agora HD |
[A], [B], [C], [D] |
Hossain et al.89 |
Fluorescent |
Staphylococcus aureus, Ebola, λ-phage |
PCR |
Human serum |
Positive identification (150 bp; 147 bp; 237 bp) |
20 min |
iPhone 4S |
[B], [C], [D] |
Priye et al.80 |
Fluorescent |
Thiram |
Paper test strip |
Apple juice |
0.1 μM to 1 mM |
<1 min |
Xiaomi III smartphone |
[B], [C] |
Mei et al.79 |
Fluorescent/microscopy |
Nanoparticles, viruses |
Cover glass |
DI water, dried |
>100 nm |
0.5 s |
Nokia PureView 808 |
[B], [C], [D] |
Wei et al.69 |
Fluorescent/microscopy |
Red/white blood cells |
Cell counting chamber |
Blood |
3 × 106 to 5.5 × 106 cells μL−1; 3000–12 000 cells μL−1 |
<10 s |
Samsung Galaxy SII |
[B], [C], [D] |
Zhu et al.86 |
Bio/chemi-luminescence |
DMSO toxicity (HEK293T cells) |
Well cartridge |
DMEM |
0.25–30% DMSO (v/v) |
45 min |
Samsung Galaxy Note II |
[B], [C], [D] |
Cevenini et al.67 |
Bio/chemi-luminescence |
H2O2 |
Paper-plastic cartridge |
10 mM TCPO |
250 nM to 100 μM |
5–30 s |
iPhone 5s |
[B], [F] |
Lebiga et al.78 |
Bio/chemi-luminescence |
HIV1-p17 IgG |
Well plate |
Blood plasma |
10 pM to 5 nM |
30 min |
Nokia Lumia 920 |
[C], [D] |
Arts et al.71 |
Spectroscopy |
BSA |
Cuvette |
DI water |
>0.1 mg mL−1 |
10 min |
HTC One (M8) |
[B], [C] |
Zhang et al.88 |
Spectroscopy |
Paraoxon |
Cuvette |
PBS |
5 nM to 25 μM |
30 min |
iPhone 5 |
[B], [C] |
Wang et al.92 |
Spectroscopy |
pH |
Cuvette |
Ground/river water |
pH 6–9 |
Unspecified |
iPhone 4 |
[B], [C] |
Dutta et al.89 |
Spectroscopy |
Protein A IgG |
PC crystal |
PBS, dried |
>4.25 nM |
40 min |
iPhone 4 |
[B], [C], [D] |
Gallegos et al.90 |
Spectroscopy/fluorescent |
miRNA |
Cuvette |
PBS |
10 pM to 1 μM |
1 s |
iPhone 4 |
[B], [C], [D] |
Yu et al.87 |
Scattering |
Eschericia coli K12 |
Paper analytical device |
Field water |
10–105 CFU mL−1 |
90 s |
iPhone 4 |
[C], [D] |
Park and Yoon97 |
Scattering |
Hepatitis B, HIV antigens |
Cuvette |
Blood serum |
10–200 ng mL−1 |
20 min |
HTC Desire HD |
[A], [B], [C], [D] |
Giavazzi et al.102 |
Scattering |
Turbidity |
Tube |
Water |
0.1–400 NTU |
2 h |
Sony Xperia E3, Asus Zenfone2, Moto G xt1033 |
[B], [C], [D] |
Hussain et al.96 |
Liquid matrices potentially benefit lower assay limits of detection due to the increased path length and less restricted diffusion that is afforded for light through a homogenous solution as opposed to reflection from a solid surface sample. According to the Beer–Lambert law, absorbance is directly proportional to path length. Thus, for absorbance changes in colorimetric assays, light transmitted and detected across a liquid sample, with a path length on the order of millimeters or even centimeters, can inherently produce a greater signal response than the same signal detected through a liquid film, which may only be microns across. This is provided that the absorbance or scattering of other components in the sample does not reduce the transmissivity of the whole solution, as is problematic for whole blood matrices.28,51 These analyte-reagent colorimetric signal changes require some time for stabilization, however, with assays using larger sample volumes and/or immobilized reagents requiring 5–15 minutes or up to an hour for development (Table 1). In this manner, mobile-phase strips or thin hydrogel film assays provide shorter assay times, as they reduce diffusion distances while still permitting individual mobility of both the analyte and reagent, overcoming the main source of temporal limitations at the cost of path length. An ideal smartphone colorimetric assay platform might find the optimal union of these two, allowing detection across a longer path length but with a low total volume to reduce diffusional distances when considering low concentrations of analytes.
Fluorescence-based sensors
Smartphones are also popularly integrated with fluorescence-based assays (Table 1). These detect light emitted upon the radiative excitation and decay of a target–fluorophore complex. The photons released within this signal response are distributed across a relatively narrow band of peak emission wavelengths, which can be amplified to allow potentially lower thresholds of target concentration detection.58,59 Additionally, as fluorescence is directly proportional to the intensity of the excitation source, the spectrum and intensity of sensor illumination can be tuned to further reduce the limit of detection as compared with colorimetric assays.26,60 These strong and narrow emission peaks can also potentially benefit multiplexing when targets are fluorescently tagged separately within a single sample.61–63 However, because a smartphone CMOS array is unable to fully distinguish between neighboring wavelength responses, long pass filters are typically inserted between the assay platform and the smartphone lens to allow only emitted light to reach the CMOS (Fig. 1c).
Additional research has designed novel, high-performance filters and materials that can be paired with mobile assays to improve light rejection both spectrally, ensuring that light outside of the target emission wavelength does not interfere with detection, and also spatially, controlling the scattering and diffraction of emitted light. These can be vital for low concentration detection and for sensing applications that are spatially sensitive, as with smartphone fluorescence microscopy. Examples of these advanced filters and surfaces include the “silo-filter” developed by Lee et al., which operates using a grid of light absorbent cells that fits over a CMOS array to improve the spatial resolution for sensing discrete cells.64 Another example is the multilayered photonic crystal surface developed by Ricciardi et al.65 Through controlled diffraction, this offers the advantage of 40 times lower detection limits over detection from comparable surfaces and allows the use of collectors with a lower numerical aperture, which can be potentially less expensive and reduce residual aberrations in the signal. As another option, Wargocki et al. chose to use a polarizer to separate excitation and emission light between a tablet computer screen, which served as an excitation source through rear-illumination of wells containing collagenase- and trypsin-fluorescein, and a smartphone camera detector.66 This allowed low limits of detection (3.75 μg mL−1 collagenase, and 3.72 ng mL−1 trypsin) despite intense proximal excitation light from the tablet computer.
Recently, fluorescence-based detection has been widely used for biological targets, such as bacterial and viral antigens, proteins, nucleic acids, and mycotoxins,62,66–75 but have also been used for some chemical targets.29,76–79 In polymerase chain reaction (PCR) and loop-mediated isothermal amplification (LAMP), the fluorescent emission of an intercalating dye allows identification of specific nucleic acid segments that have been amplified from an organism.80–83 Fluorescent dyes that have been used to tag proteins and other cellular surface antigens have also been sensed through smartphone microscopy.84,85 Wei et al. demonstrated such fluorescent detection paired with smartphone microscopy, which was used to allow counting of individual virus capsids.69
As with colorimetric assays, fluorescent smartphone detection has primarily been used for samples in liquid matrices using larger volume well plates or mobile state film, cartridge, or slide platforms. Recently demonstrated assays have involved incubation and signal development times between one and five minutes.29,72,79 These have allowed for better real-time results, particularly when using an excitation light source for more immediate and on-demand fluorescent detection as opposed to the time-dependent signal development and decay that occurs with some chemiluminescent reactions.
Luminescence-based sensors
Smartphones have also been used for these other luminescence-based assays (i.e. bioluminescence and chemiluminescence assays), which detect narrow-band light emission from biological or chemical reactions arising between a target and a reagent. While it is possible to use an excitation light source to enhance these assays, in principle it is not required due to the spontaneous light emission which fundamentally distinguishes these from fluorescent and colorimetric assays. Such characteristics allow these assays to be highly sensitive to a given target. However, the intensity of a luminescent reaction is time-dependent, decaying exponentially such that the intensity for some reactions is negligible after only a few seconds.78 This can lead to potential challenges in consistent quantification, but can be paired well with applications such as cell counting, cytotoxicity screening, or particle size discrimination, in which exact signal quantification may not be necessary. For example, Zhu et al. have paired luminescent smartphone-based sensing with flow cytometry to facilitate cell counting and particle size discrimination.86 By making accommodations for shorter incubation times and rapid signal detection ahead of the signal decay, though, Lebiga et al. and Arts et al. have demonstrated low detection limits, with 250 nM detection of H2O2 and 10 pM detection of HIV1-p17 antibodies, respectively, through smartphone detection.71,78
Spectroscopy-based sensors
Spectroscopy attachments have often been paired with smartphones to work in coordination with colorimetric, fluorescent, and other luminescent sensing methods because they allow a smartphone to distinguish wavelengths by spatially separating them across CMOS array.87,88 This is typically accomplished through a grating that diffracts light after it has been reflected by or transmitted through the sample, but before it has entered the camera aperture (Fig. 1d). Hossain et al. used such techniques with dual absorbance and fluorescence assays for pH and Zn2+, and it allowed them to separately analyze signal wavelengths at a resolution of 0.42 nm per pixel over a 300 nm bandwidth.89 Recent research has also evaluated assays for proteins and antigens,88,90 enzyme activity,91 nucleic acids,87 neurotoxins,92 and pH93 in similar manners.
Scattering-based sensors
Additional applications of smartphone optical sensors have focused on detecting elastic light scattering – that is, particle-based scattering which results in a change in light direction, but no change in its energy (wavelength).94 This form of scattering can be subdivided into the Rayleigh and Mie scattering regimes, which both formally describe the scattering of spherical particles.95 The Rayleigh regime describes the multidirectional scattering of particles that are significantly smaller than the wavelength of incident light (the size parameter α = πd/λ is ≪1, where d is the diameter of the scattering particle and λ is the wavelength of the incident light). The Mie scattering regime more generally applies to all spherical particles, regardless of size. As such, it encompasses Rayleigh scattering; however, the mathematical complexity of Mie theory prompts the use of simplified Rayleigh scattering theory where applicable. For incident light at visible wavelengths, Mie theory may be used to generally describe the forward angular scattering characteristics of micron and sub-micron scale particles (d ≥ λ). Both forms of scattering have recently been applied in smartphone sensing platforms, with perhaps more emphasis given to Mie scattering. The intensity of Mie scattering drastically changes, or “oscillates,” over scattering angles and particle sizes. This can thus be used to detect particles based on size characteristics and to detect aggregation/agglutination within samples. Mie scattering has been recently applied for quantifying discrete particles in turbidity,96 as well as for quantifying bacteria concentrations in water, meat, and urine samples.97–99
While Rayleigh scattering also varies over the scattering angle, these changes are rather monotonic and may provide weaker signals for distinguishing particle size in aggregation/agglutination-induced biosensing. However, Rayleigh scattering can provide strong sensitivity when used in conjunction with nanotechnology. Recent applications include laser-induced detection of lead by gold nanoparticles,100 as well as a condensation-based nanolens which allows detection of nanospheres and nanorods of diameters below 40 nm and 20 nm, respectively.101
Further work with scattering-based detection has evaluated label-free immunoassays of hepatitis B and HIV antigens.102 Nicolini et al. demonstrated multimodal smartphone detection of bacteria via particle immunoagglutination, combining a scattering-based assay to identify the concentration of E. coli K12 and Salmonella Typhimurium, and a fluorescence-based assay to allow for specificity between the two targets.61
Smartphone microscopy
The final application of smartphone sensing discussed here is smartphone microscopy. Some recent innovations have included work toward computational lensfree microscopy, while others have combined illumination and focusing attachments together with computational methods including convolution, super-resolution algorithms, and machine learning to allow for improved spatial resolution that would not otherwise be possible with the intrinsic optical components of the smartphone camera alone. An example of these combined techniques is CellScope, a smartphone microscope used for applications including ocular imaging and tuberculosis diagnostics, and which has recently been integrated with computational refocusing and illumination techniques using a domed LED array attachment.103–105 This allows the user to conduct brightfield, darkfield, and phase contrast microscopy in real-time with axial resolution of ∼5 μm. Other steps in smartphone light microscopy have demonstrated recognition of targets including micron-scale Bacillus anthracis Sterne spores from growth media and helminth ova directly from stool samples.106,107
Smartphone microscopy has also been woven together with other sensing modalities, especially with fluorescence which can be used as a beacon for discrete targets. Koydemir et al. utilized smartphone-based fluorescence microscopy for detecting Giardia lamblia cysts over a wide field of view (∼0.8 cm2) at concentrations as low as ∼12 cysts per 10 mL directly from a water filter cassette.84 Smartphone fluorescence microscopy has also been used recently for rapidly distinguishing and counting red and white blood cells in flow cytometry applications,86 and has allowed for detection of even smaller particles, such as virions and nanoparticles on the order of 10 nm despite the dimension constraints of the CMOS array itself.69
Further in-depth discussion of smartphone microscopy can be found in other topic-specific reviews.108–111
Challenges and future needs
Overall, perhaps the most basic challenge in smartphone optical sensing is interference from uncontrolled or uneven lighting, which can fundamentally detract from the raw signal being detected. Common control measures include the use of enclosed lighting and imaging attachments for the smartphone, as well as collimating lenses or optical fibers to reduce light dispersion. Similar controls, especially attached lenses, can help to improve sensitivity for smartphone microscopy.69,106,112 Through their pairing with a grating, as in spectroscopy, the smartphone can also achieve sub-nanometer wavelength resolution across a sub-micron bandwidth in the smartphone viewing field, as demonstrated by Yu et al. and Hossain et al.87,89
Tied into these concerns are the physical constraints of the Bayer color filter array (CFA) in a typical smartphone CMOS image sensor. The Bayer CFA functions by providing three filters for incident light (red, green, and blue), which alternate along two orthogonal axes to allow intermingled transmittance of red, green, and blue light to a CMOS sensor to create the spatial perception of color within an image.113 Because the light is separated into discrete units (pixels), the sensitivity of a smartphone optical sensor is ultimately limited by the number and sensitivity of these units. Again, lenses are frequently used to concentrate the signal emitted from a sensor platform before it reaches the smartphone optical hardware, and trade-offs in other parameters such as speed or imaging area and depth can be counterbalanced with improved sensor sensitivity.
Subhash et al. demonstrated a smartphone-based optical coherence tomography system that takes advantage of both a repurposed miniature optical delay from a compact disc optical pick-up head as well as a partial mirror to provide depth-resolved imaging with resolution comparable to an equivalent commercial grade system for under $10, but at the cost of lower speed.114 Balsam et al. paired both physical and computational enhancement for smartphone sensing of fluorescent signals by way of a capillary tube array and image stacking/background normalization processes, reporting a 1000× decrease in their observed fluorescent limit of detection for an adenovirus DNA target.115
While these various physical lighting control mechanisms provide the advantage of controlling uncertain surrounding conditions, though, they can also limit the particular sensing platform to a single device, which in turn limits the broad usability of the sensor platform. For resource-limited sensing, research that focuses on platform-independent or directly platform-translatable techniques, such as computational methods, can be beneficial since reliance upon secondary attachments introduces additional costs, special material requirements, and platform limitations. Separate smartphone models may be available in different countries, and newer models with substantially improved cameras and software technology are frequently introduced.
Work by Sumriddetchkajorn et al., Devadhasan et al., and Xu et al. are notable examples of this alternate focus, as they have each explored the use of image-processing algorithms and simple reference points within the imaging background to provide controls similar to those offered by attachments.27–29 In these works, researchers have successfully demonstrated sensitive colorimetric quantification of dissolved O2/pH, pH, and chlorine, respectively, without the need for an attached enclosure. However, on the whole most current smartphone-integrated sensors still rely on such enclosures for smartphone-integrated colorimetric, fluorescence-based, and scattering-based assays.
It should also be noted that typical smartphone camera apps do adjust both its hardware and the acquired images to allow the images to look natural to human perception. This is done through “auto-focus”, “auto-exposure”, and “white balance” corrections, among other settings. While it is relatively easy to manually turn off the auto-focus and auto-exposure features, though, it is comparatively difficult to turn off or adjust the white balance feature. White balance corrections are specifically problematic in colorimetric and fluorescent sensing, since the RGB pixels in a CMOS array are not entirely perfect in recognizing red, green, and blue colors. For example, red pixels can still exhibit a limited response to blue colors, thus requiring corrective measures. In addition, human eyes are imperfect in their recognition of the three basic colors. Both red and blue cone cells in the human eye respond to ∼420 nm, generating a violet color which looks very similar to purple, a mixture of red and blue colors. When smartphone apps are designed to mimic human perception, they can also mimic these imperfections of the human eye. In addition, it is known that the “tones” of images vary between smartphone manufacturers. Therefore, a complex normalization algorithm must be implemented and/or an app that saves the raw images as they are acquired should be used.
Spatial lighting gradients and variance can also be a serious concern in optical smartphone sensing. Regardless of the light sources (ambient lighting or a separate light source), the level of lighting can vary over a single image. In addition, the four corners of a typical smartphone image tend to be darker than the other areas. In this regard, complex normalization algorithms have been adapted to overcome lighting bias errors and extract select signals or features of interest even in unfiltered and broad-field images.116 A fast Fourier transform (FFT) filter and triple normalization algorithm were successfully utilized to minimize the ambient lighting bias, spatial imaging bias, and inter- and intra-channel variations from multi-channel microfluidic paper analytic device (μPAD) for smartphone-based water quality monitoring (Fig. 3).117 These are limited by the sensitivity of the smartphone optical instrumentation itself, however. A better approach for severe lighting bias would be to provide a normalization algorithm that can function under a more universal lighting control region, such as a known reference background of simple unattached box enclosure – something that might be accessible to anyone anywhere. Recent research has explored these possibilities, but more work is needed to test such methods under rigorous lighting conditions.27–29
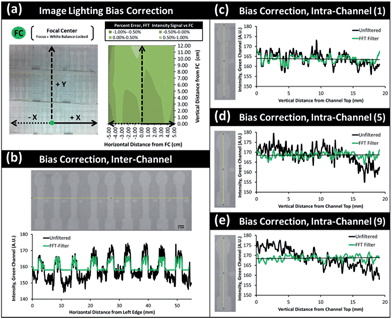 |
| Fig. 3 (a, left) Across a smartphone image, unfiltered lighting contributes to anisotropic illumination and shading that can lead to measurement errors between identical channels, as seen both horizontally and vertically across blank microfluidic paper analytical device channels. (a, right) Image processing techniques even as simple as a multi-reference normalization algorithm or a fast Fourier transform (FFT)-based can help to dramatically reduce such errors. Further work in this area can help to eliminate the need for secondary smartphone attachments to provide consistency both (b) between sensor measurements, and (c–e) along different regions of even a single sensor device. | |
Similarly, more research is needed to evaluate differences between individual smartphone platforms. Although smartphone optical hardware is frequently sourced third-party from similar manufacturers, their configurations within the final smartphone may still differ dramatically, affecting signal measurements. Doeven et al. compared the functional hardware differences between five phones, albeit with a focus on audio outputs designed to mediate chemiluminescence.77 This evaluation identified substantial differences between phone characteristics, even among a single manufacturer. Askim et al. offered a comparison between smartphone (iPhone 5s) sensing and three additional handheld sensing methods – a novel hand-held CMOS-based CIS reader, a consumer-grade flatbed scanner, and a consumer-grade DSLR camera – for colorimetric assay detection.118 They found that the absence of lighting control mechanisms in this standard smartphone platform actually led to higher signal deviation as compared with all other methods, with the handheld and flatbed scanners resulting in the greatest consistency – purportedly on account of uniform controlled lighting capabilities and a constant focal distance. In light of these conclusions, investigation into the consistencies between many separate smartphone devices would be ideal to identify how different smartphone light sources and cameras separately analyze chemical or biosensor assays. This would strongly inform the design of control measures for improved resource-limited sensing.
Conclusions
Across recent research, interests in a diverse range of field applications have brought along unique techniques and devices in the hope of enhancing smartphone sensitivity for instrument-grade quality. The creativity in these advances has brought many promising results, but the broad applicability of this work in many resource-limited settings is still a challenge due to platform limitations, with often uncertain reproducibility across smartphone devices and with potential limitations in the use of advanced smartphone attachments, which may not be easily or directly translatable between devices. Certainly such constraints are necessary for optical sensing techniques that require precise alignment of components, such as smartphone spectroscopy or microscopy, but interesting and practical insights may be offered by further exploration of reductionist designs and platform-translatable computational techniques. If feasible, such measures could lead toward broader future implementation serving a wider base of users in resource-limited settings.
Acknowledgements
KEM acknowledges support from the U.S. National Science Foundation Graduate Research Fellowship under Grant No. DGE-1143953. Any opinion, findings, and conclusions or recommendations expressed in this material are those of the author(s) and do not necessarily reflect the views of the National Science Foundation.
References
- N. D. Lane, E. Miluzzo, H. Lu, D. Peebles, T. Choudhury and A. T. Campbell, IEEE Commun. Mag., 2010, 48, 140–150 CrossRef.
- X. Xu, A. Akay, H. Wei, S. Wang, B. Pingguan-Murphy, B.-E. Erlandsson, X. Li, W. Lee, J. Hu, L. Wang and F. Xu, Proc. IEEE, 2015, 103, 236–247 CrossRef CAS.
- D. Zhang and Q. Liu, Biosens. Bioelectron., 2016, 75, 273–284 CrossRef CAS PubMed.
- X. Liu, T.-Y. Lin and P. B. Lillehoj, Ann. Biomed. Eng., 2014, 42, 2205–2217 CrossRef PubMed.
- S. K. Vashist, P. B. Luppa, L. Y. Yeo, A. Ozcan and J. H. T. Luong, Trends Biotechnol., 2015, 33, 692–705 CrossRef CAS PubMed.
- A. Roda, E. Michelini, M. Zangheri, M. Di Fusco, D. Calabria and P. Simoni, Trends Anal. Chem., 2016, 79, 317–325 CrossRef CAS.
- C. F. Fronczek and J.-Y. Yoon, J. Lab. Autom., 2015, 20, 390–410 CrossRef CAS PubMed.
-
J.-Y. Yoon, Introduction to Biosensors, Springer, New York, NY, 2nd edn, 2016 Search PubMed.
-
A. Sadana and N. Sadana, Handbook of Biosensors and Biosensor Kinetics, Elsevier, Amsterdam, 2011 Search PubMed.
-
B. Wang and E. V. Anslyn, Chemosensors, John Wiley & Sons, Hoboken, NJ, 2011 Search PubMed.
- J. Jiang, X. Wang, R. Chao, Y. Ren, C. Hu, Z. Xu and G. L. Liu, Sens. Actuators, B, 2014, 193, 653–659 CrossRef CAS.
- A. Nemiroski, D. C. Christodouleas, J. W. Hennek, A. A. Kumar, E. J. Maxwell, M. T. Fernández-Abedul and G. M. Whitesides, Proc. Natl. Acad. Sci. U. S. A., 2014, 111, 11984–11989 CrossRef CAS PubMed.
- S. E. Meredith, A. Robinson, P. Erb, C. A. Spieler, N. Klugman, P. Dutta and J. Dallery, Nicotine Tob. Res., 2014, 16, 766–773 CrossRef CAS PubMed.
- J. L. Delaney, E. H. Doeven, A. J. Harsant and C. F. Hogan, Anal. Chim. Acta, 2013, 790, 56–60 CrossRef CAS PubMed.
-
K. Nagamine, IDC Worldwide Quarterly Mobile Phone Tracker, International Data Corporation, 2015 Search PubMed.
- A. Charlesworth, Eng. Tech., 2009, 4, 32–33 CrossRef.
- International Business Times, http://www.ibtimes.com/smartphone-evolution-ibm-simon-samsung-galaxy-s3-697340, accessed March 2016.
- A. Chib, M. H. van Velthoven and J. Car, J. Health Comm., 2014, 20, 4–34 CrossRef PubMed.
- S. Byrnes, G. Thiessen and E. Fu, Bioanalysis, 2013, 5, 2821–2836 CrossRef CAS PubMed.
- R. W. Peeling and R. McNerney, Expert Rev. Mol. Diagn., 2014, 14, 525–534 CrossRef CAS PubMed.
- P. K. Drain, E. P. Hyle, F. Noubary, K. A. Freedberg, D. Wilson, W. R. Bishai, W. Rodriguez and I. V. Bassett, Lancet Infect. Dis., 2014, 14, 239–249 CrossRef PubMed.
- G. Wu, J. Srivastava and M. H. Zaman, Anal. Biochem., 2014, 449, 147–154 CrossRef CAS PubMed.
- D. Y. Zhang, S. X. Chen and P. Yin, Nat. Chem., 2012, 4, 208–214 CrossRef CAS PubMed.
- W. Asghar, M. Yuksekkaya, H. Shafiee, M. Zhang, M. O. Ozen, F. Inci, M. Kocakulak and U. Demirci, Sci. Rep., 2016, 6, 21163 CrossRef CAS PubMed.
- J. R. Choi, J. Hu, S. Feng, W. A. B. Wan Abas, B. Pingguan-Murphy and F. Xu, Biosens. Bioelectron., 2016, 79, 98–107 CrossRef CAS PubMed.
-
F. M. Lanças and E. Carrilho, in Ewing's Analytical Instrumentation Handbook, ed. J. Cazes, CRC Press, Boca Raton, FL, 3rd edn, 2004, ch. 6, pp. 141–161 Search PubMed.
- S. Sumriddetchkajorn, K. Chaitavon and Y. Intaravanne, Sens. Actuators, B, 2013, 182, 592–597 CrossRef CAS.
- J. P. Devadhasan and S. Kim, Anal. Chim. Acta, 2015, 858, 55–59 CrossRef CAS PubMed.
- W. Xu, S. Lu, Y. Chen, T. Zhao, Y. Jiang, Y. Wang and X. Chen, Sens. Actuators, B, 2015, 220, 326–330 CrossRef CAS.
- C. V. Sapan, R. L. Lundblad and N. C. Price, Biotechnol. Appl. Biochem., 1999, 29, 99–108 CAS.
- D. Vilela, M. C. González and A. Escarpa, Anal. Chim. Acta, 2012, 751, 24–43 CrossRef CAS PubMed.
- A. Rico-Yuste, V. González-Vallejo, E. Benito-Peña, T. de las Casas Engel, G. Orellana and M. C. Moreno-Bondi, Anal. Chem., 2016, 88, 3959–3966 CrossRef CAS PubMed.
- K. Su, X. Qiu, J. Fang, Q. Zou and P. Wang, Sens. Actuators, B, 2016 DOI:10.1016/j.snb.2016.02.092.
- V. Oncescu, M. Mancuso and D. Erickson, Lab Chip, 2014, 14, 759–763 RSC.
- M. Zangheri, L. Cevenini, L. Anfossi, C. Baggiani, P. Simoni, F. Di Nardo and A. Roda, Biosens. Bioelectron., 2015, 64, 63–68 CrossRef CAS PubMed.
- X. Yang, Y. Wang, W. Liu, Y. Zhang, F. Zheng, S. Wang, D. Zhang and J. Wang, Biosens. Bioelectron., 2016, 75, 48–54 CrossRef CAS PubMed.
- S. H. Im, K. R. Kim, Y. M. Park, J. H. Yoon, J. W. Hong and H. C. Yoon, Sens. Actuators, B, 2016, 229, 166–173 CrossRef CAS.
- J. P. Devadhasan, H. Oh, C. S. Choi and S. Kim, J. Biomed. Opt., 2015, 20, 117001 CrossRef PubMed.
- M.-Y. Jia, Q.-S. Wu, H. Li, Y. Zhang, Y.-F. Guan and L. Feng, Biosens. Bioelectron., 2015, 74, 1029–1037 CrossRef CAS PubMed.
- Y. Jung, J. Kim, O. Awofeso, H. Kim, F. Regnier and E. Bae, Appl. Opt., 2015, 54, 9183–9189 CrossRef PubMed.
- A. Carrio, C. Sampedro, J. L. Sanchez-Lopez, M. Pimienta and P. Campoy, Sensors, 2015, 15, 29569–29593 CrossRef PubMed.
- V. Oncescu, D. O'Dell and D. Erickson, Lab Chip, 2013, 13, 3232–3238 RSC.
- T. S. Park, C. Baynes, S.-I. Cho and J.-Y. Yoon, RSC Adv., 2014, 4, 24356–24362 RSC.
- S. Levin, S. Krishnan, S. Rajkumar, N. Halery and P. Balkunde, Sci. Total Environ., 2016, 551–552, 101–107 CrossRef CAS PubMed.
- W. Chen, F. Cao, W. Zheng, Y. Tian, Y. Xianyu, P. Xu, W. Zhang, Z. Wang, K. Deng and X. Jiang, Nanoscale, 2015, 7, 2042–2049 RSC.
- D. Igoe and A. V. Parisi, Instrum. Sci. Technol., 2015, 44, 139–147 CrossRef.
- A. K. Yetisen, Y. Montelongo, M. M. Qasim, H. Butt, T. D. Wilkinson, M. J. Monteiro and S. H. Yun, Anal. Chem., 2015, 87, 5101–5108 CrossRef CAS PubMed.
- Q. Wei, R. Nagi, K. Sadeghi, S. Feng, E. Yan, S. J. Ki, R. Caire, D. Tseng and A. Ozcan, ACS Nano, 2014, 8, 1121–1129 CrossRef CAS PubMed.
- H. Nie, W. Wang, W. Li, Z. Nie and S. Yao, Analyst, 2015, 140, 2771–2777 RSC.
- L. Zhang, W. Yang, Y. Yang, H. Liu and Z. Gu, Analyst, 2015, 140, 7399–7406 RSC.
- T. Laksanasopin, T. W. Guo, S. Nayak, A. A. Sridhara, S. Xie, O. O. Olowookere, P. Cadinu, F. Meng, N. H. Chee, J. Kim, C. D. Chin, E. Munyazesa, P. Mugwaneza, A. J. Rai, V. Mugisha, A. R. Castro, D. Steinmiller, V. Linder, J. E. Justman, S. Nsanzimana and S. K. Sia, Sci. Transl. Med., 2015, 7, 273re1 CrossRef PubMed.
- A. Thiha and F. Ibrahim, Sensors, 2015, 15, 11431–11441 CrossRef CAS PubMed.
- A. F. Coskun, J. Wong, D. Khodadadi, R. Nagi, A. Tey and A. Ozcan, Lab Chip, 2013, 13, 636–640 RSC.
- Y. Chen, Y. Zilberman, P. Mostafalu and S. R. Sonkusale, Biosens. Bioelectron., 2015, 67, 477–484 CrossRef CAS PubMed.
- N. Lopez-Ruiz, J. Lopez-Torres, M. Á. C. Rodríguez, I. P. de Vargas-Sansalvador and A. Martinez-Olmos, IEEE Sens. J., 2015, 15, 4039–4045 CrossRef.
- Q. Meng, L. Fang, T. Han, S. Huang and S. Xie, Sens. Actuators, B, 2016, 228, 144–150 CrossRef CAS.
- P. Han, D. Dong, X. Zhao, L. Jiao and Y. Lang, Comput. Electron. Agr., 2016, 123, 232–241 CrossRef.
-
A. Demchenko, Introduction to Fluorescence Sensing, Springer, Dordrecht, 2009 Search PubMed.
- H. S. Jung, X. Chen, J. S. Kim and J. Yoon, Chem. Soc. Rev., 2013, 42, 6019–6031 RSC.
-
J. Lakowicz, Principles of Fluorescence Spectroscopy, Plenum Press, New York, NY, 3rd edn, 2006 Search PubMed.
- A. M. Nicolini, C. F. Fronczek and J.-Y. Yoon, Biosens. Bioelectron., 2015, 67, 560–569 CrossRef CAS PubMed.
- K. Ming, J. Kim, M. J. Biondi, A. Syed, K. Chen, A. Lam, M. Ostrowski, A. Rebbapragada, J. J. Feld and W. C. W. Chan, ACS Nano, 2015, 9, 3060–3074 CrossRef CAS PubMed.
- E. Petryayeva and W. R. Algar, Analyst, 2015, 140, 4037–4045 RSC.
- S. A. Lee, X. Ou, J. E. Lee and C. Yang, Opt. Lett., 2013, 38, 1817–1819 CAS.
- S. Ricciardi, F. Frascella, A. Angelini, A. Lamberti, P. Munzert, L. Boarino, R. Rizzo, A. Tommasi and E. Descrovi, Sens. Actuators, B, 2015, 215, 225–230 CrossRef CAS.
- P. Wargocki, W. Deng, A. G. Anwer and E. M. Goldys, Sensors, 2015, 15, 11653–11664 CrossRef CAS PubMed.
- L. Cevenini, M. M. Calabretta, G. Tarantino, E. Michelini and A. Roda, Sens. Actuators, B, 2016, 225, 249–257 CrossRef CAS.
- V. K. Rajendran, P. Bakthavathsalam and B. M. Jaffar Ali, Microchim. Acta, 2014, 181, 1815–1821 CrossRef CAS.
- Q. Wei, H. Qi, W. Luo, D. Tseng, S. J. Ki, Z. Wan, Z. Göröcs, L. A. Bentolila, T.-T. Wu, R. Sun and A. Ozcan, ACS Nano, 2013, 7, 9147–9155 CrossRef CAS PubMed.
- S. K. J. Ludwig, C. Tokarski, S. N. Lang, L. A. van Ginkel, H. Zhu, A. Ozcan and M. W. F. Nielen, PLoS One, 2015, 10, e0134360 Search PubMed.
- R. Arts, I. den Hartog, S. E. Zijlema, V. Thijssen, S. H. E. van der Beelen and M. Merkx, Anal. Chem., 2016, 88, 4525–4532 CrossRef CAS PubMed.
- D. Bueno, R. Muñoz and J. L. Marty, Sens. Actuators, B, 2016, 232, 462–468 CrossRef CAS.
- A. I. Barbosa, P. Gehlot, K. Sidapra, A. D. Edwards and N. M. Reis, Biosens. Bioelectron., 2015, 70, 5–14 CrossRef CAS PubMed.
- C. F. Fronczek, T. S. Park, D. K. Harshman, A. M. Nicolini and J.-Y. Yoon, RSC Adv., 2014, 4, 11103–11110 RSC.
- T. S. Park, S. Cho, T. G. Nahapetian and J.-Y. Yoon, J. Lab. Autom., 2016 DOI:10.1177/2211068216639566.
- M. A. Hossain, J. Canning, S. Ast, P. J. Rutledge, T. L. Yen and A. Jamalipour, IEEE Sens. J., 2015, 15, 5095–5102 CrossRef.
- E. H. Doeven, G. J. Barbante, A. J. Harsant, P. S. Donnelly, T. U. Connell, C. F. Hogan and P. S. Francis, Sens. Actuators, B, 2015, 216, 608–613 CrossRef CAS.
- E. Lebiga, R. E. Fernandez and A. Beskok, Analyst, 2015, 140, 5006–5011 RSC.
- Q. Mei, H. Jing, Y. Li, W. Yisibashaer, J. Chen, B. N. Li and Y. Zhang, Biosens. Bioelectron., 2016, 75, 427–432 CrossRef CAS PubMed.
- A. Priye, S. Wong, Y. Bi, M. Carpio, J. Chang, M. Coen, D. Cope, J. Harris, J. Johnson, A. Keller, R. Lim, S. Lu, A. Millard, A. Pangelinan, N. Patel, L. Smith, K. Chan and V. M. Ugaz, Anal. Chem., 2016, 88, 4651–4660 CrossRef CAS PubMed.
- S.-C. Liao, J. Peng, M. G. Mauk, S. Awasthi, J. Song, H. Friedman, H. H. Bau and C. Liu, Sens. Actuators, B, 2016, 229, 232–238 CrossRef CAS PubMed.
- S. V. Angus, S. Cho, D. K. Harshman, J.-Y. Song and J.-Y. Yoon, Biosens. Bioelectron., 2015, 74, 360–368 CrossRef CAS PubMed.
- D. K. Harshman, B. M. Rao, J. E. McLain, G. S. Watts and J.-Y. Yoon, Sci. Adv., 2015, 1, e1400061 Search PubMed.
- H. C. Koydemir, Z. Gorocs, D. Tseng, B. Cortazar, S. Feng, R. Y. L. Chan, J. Burbano, E. McLeod and A. Ozcan, Lab Chip, 2015, 15, 1284–1293 RSC.
- J.-H. Kim, H.-G. Joo, T.-H. Kim and Y.-G. Ju, BioChip J., 2015, 9, 285–292 CrossRef CAS.
- H. Zhu, I. Sencan, J. Wong, S. Dimitrov, D. Tseng, K. Nagashima and A. Ozcan, Lab Chip, 2013, 13, 1282–1288 RSC.
- H. Yu, Y. Tan and B. T. Cunningham, Anal. Chem., 2014, 86, 8805–8813 CrossRef CAS PubMed.
- C. Zhang, G. Cheng, P. Edwards, M.-D. Zhou, S. Zheng and Z. Liu, Lab Chip, 2016, 16, 246–250 RSC.
- M. A. Hossain, J. Canning, S. Ast, K. Cook, P. J. Rutledge and A. Jamalipour, Opt. Lett., 2015, 40, 1737–1740 CrossRef PubMed.
- D. Gallegos, K. D. Long, H. Yu, P. P. Clark, Y. Lin, S. George, P. Nath and B. T. Cunningham, Lab Chip, 2013, 13, 2124–2132 RSC.
- E. Petryayeva and W. R. Algar, Anal. Chem., 2014, 86, 3195–3202 CrossRef CAS PubMed.
- L.-J. Wang, Y.-C. Chang, X. Ge, A. T. Osmanson, D. Du, Y. Lin and L. Li, ACS Sens., 2016, 1, 366–373 CrossRef CAS.
- S. Dutta, D. Sarma and P. Nath, AIP Adv., 2015, 5, 057151 CrossRef.
-
M. Quinten, Optical Properties of Nanoparticle Systems: Mie and Beyond, Wiley, Weinhein, 2011 Search PubMed.
- T. Wriedt, Part. Part. Syst. Charact., 1998, 15, 67–74 CrossRef CAS.
- I. Hussain, K. Ahamad and P. Nath, RSC Adv., 2016, 6, 22374–22382 RSC.
- T. S. Park and J.-Y. Yoon, IEEE Sens. J., 2015, 15, 1902–1907 CrossRef CAS.
- P.-S. Liang, T. S. Park and J.-Y. Yoon, Sci. Rep., 2014, 4, 5953 CAS.
- S. Cho, T. S. Park, T. G. Nahapetian and J.-Y. Yoon, Biosens. Bioelectron., 2015, 74, 601–611 CrossRef CAS PubMed.
- J. H. Lin and W. L. Tseng, Talanta, 2015, 132, 44–51 CrossRef CAS PubMed.
- E. McLeod, C. Nguyen, P. Huang, W. Luo, M. Veli and A. Ozcan, ACS Nano, 2014, 8, 7340–7349 CrossRef CAS PubMed.
- F. Giavazzi, M. Salina, E. Ceccarello, A. Ilacqua, F. Damin, L. Sola, M. Chiari, B. Chini, R. Cerbino, T. Bellini and M. Buscaglia, Biosens. Bioelectron., 2014, 58, 395–402 CrossRef CAS PubMed.
- R. N. Maamari, J. D. Keenan, D. A. Fletcher and T. P. Margolis, Br. J. Ophthalmol., 2014, 98, 438–441 CrossRef PubMed.
- A. Tapley, N. Switz, C. Reber, J. L. Davis, C. Miller, J. B. Matovu, W. Worodria, L. Huang, D. A. Fletcher and A. Cattamanchi, J. Clin. Microbiol., 2013, 51, 1774–1778 CrossRef PubMed.
- Z. F. Phillips, M. V. D'Ambrosio, L. Tian, J. J. Rulison, H. S. Patel, A. V. Gande, N. A. Switz, D. A. Fletcher and L. Waller, PLoS One, 2015, 10, e0124938 Search PubMed.
- J. R. Hutchison, R. L. Erikson, A. M. Sheen, R. M. Ozanich and R. T. Kelly, Analyst, 2015, 140, 6269–6276 RSC.
- S. J. Sowerby, J. A. Crump, M. C. Johnstone, K. L. Krause and P. C. Hill, Am. J. Trop. Med. Hyg., 2016, 94, 227–230 CrossRef CAS PubMed.
- A. Greenbaum, W. Luo, T.-W. Su, Z. Göröcs, L. Xue, S. O. Isikman, A. F. Coskun, O. Mudanyali and A. Ozcan, Nat. Methods, 2012, 9, 889–895 CrossRef CAS PubMed.
- S. O. Isikman, A. Greenbaum, M. Lee, W. Bishara, O. Mudanyali, T.-W. Su and A. Ozcan, Anal. Cell. Pathol., 2012, 35, 229–247 CrossRef CAS.
- J. C. Contreras-Naranjo, Q. Wei and A. Ozcan, IEEE J. Sel. Top. Quantum Electron., 2016, 22, 392–405 CrossRef.
- E. McLeod and A. Ozcan, Rep. Prog. Phys., 2016, 79, 076001 CrossRef PubMed.
- Z. J. Smith, K. Chu, A. R. Espenson, M. Rahimzadeh, A. Gryshuk, M. Molinaro, D. M. Dwyre, S. Lane, D. Matthews and S. Wachsmann-Hogiu, PLoS One, 2011, 6, e17150 CAS.
-
B. E. Bayer, US Pat. 3, 971, 065A, 1976.
- M. Leahy, J. Hogan, C. Wilson, H. Subhash and R. Dsouza, Proc. SPIE, 2013, 8580, 85800L CrossRef.
- J. Balsam, R. Rasooly, H. A. Bruck and A. Rasooly, Biosens. Bioelectron., 2014, 51, 1–7 CrossRef CAS PubMed.
-
J.-W. Kim, M.-W. Lee, S.-H. Lee, J.-P. Cho and J.-S. Cha, 2015 International Conference on Information Networking (ICOIN), Cambodia, January 2015 Search PubMed.
- K. E. McCracken, S. V. Angus, K. A. Reynolds and J.-Y. Yoon, Sci. Rep., 2016, 6, 27529 CrossRef CAS PubMed.
- J. R. Askim and K. S. Suslick, Anal. Chem., 2015, 87, 7810–7816 CrossRef CAS PubMed.
- S. Dutta, K. Saikia and P. Nath, RSC Adv., 2016, 6, 21871–21880 RSC.
|
This journal is © The Royal Society of Chemistry 2016 |
Click here to see how this site uses Cookies. View our privacy policy here.