DOI:
10.1039/C6MB00289G
(Paper)
Mol. BioSyst., 2016,
12, 2762-2769
Mastitomics, the integrated omics of bovine milk in an experimental model of Streptococcus uberis mastitis: 3. Untargeted metabolomics†
Received
14th April 2016
, Accepted 16th June 2016
First published on 14th July 2016
Abstract
Intramammary infection leading to bovine mastitis is the leading disease problem affecting dairy cows and has marked effects on the milk produced by infected udder quarters. An experimental model of Streptococcus uberis mastitis has previously been investigated for clinical, immunological and pathophysiological alteration in milk, and has been the subject of peptidomic and quantitative proteomic investigation. The same sample set has now been investigated with a metabolomics approach using liquid chromatography and mass spectrometry. The analysis revealed over 3000 chromatographic peaks, of which 690 were putatively annotated with a metabolite. Hierarchical clustering analysis and principal component analysis demonstrated that metabolite changes due to S. uberis infection were maximal at 81 hours post challenge with metabolites in the milk from the resolution phase at 312 hours post challenge being closest to the pre-challenge samples. Metabolic pathway analysis revealed that the majority of the metabolites mapped to carbohydrate and nucleotide metabolism show a decreasing trend in concentration up to 81 hours post-challenge whereas an increasing trend was found in lipid metabolites and di-, tri- and tetra-peptides up to the same time point. The increase in these peptides coincides with an increase in larger peptides found in the previous peptidomic analysis and is likely to be due to protease degradation of milk proteins. Components of bile acid metabolism, linked to the FXR pathway regulating inflammation, were also increased. Metabolomic analysis of the response in milk during mastitis provides an essential component to the full understanding of the mammary gland's response to infection.
1. Introduction
Bovine mastitis, which generally develops as a sequel to intra-mammary infections, is a major infectious disease problem in dairy cows, costing the global dairy industry €16–26 billion per annum (http://www.dairy.ahdb.org.uk, accessed March 2016). However, recent advances in analytical sciences which have enabled the “omics revolution” have not been fully applied to this most important disease for the dairy industry. This paper reports a metabolomics investigation of an experimental model of Streptococcus uberis mastitis, adding to previous studies which have examined the pathophysiology of the immunological responses,1 the protein changes in the acute phase reaction along with the omic investigation of the peptidome2 and the system-wide quantitative proteomic analysis.3 The overview of mastitis provided by such a systems biology approach to the analysis of proteins, peptides and other metabolites in milk linking to disease progression provides a unique view of the pathological processes involved. In contrast to proteomics and peptidomics that analyse intact proteins and peptides (the latter consisting mostly of degradation-derived short protein fragments (polypeptides)), metabolomics analyses low-molecular weight metabolites of endogenous and exogenous origin. Most metabolites analysed have a mass less than 1.5 kDa.4
Metabolomics applies analytical chemistry techniques such as nuclear magnetic resonance (NMR) spectroscopy or hyphenated mass spectrometry combined with advanced computational and informatics methods to analyse low molecular weight compounds in a biological sample.5–7 It has previously been applied to milk in relation to physiology and composition.8–12 There have also been investigations of mastitis using Gas Chromatography-Mass Spectrometry (GC-MS) and NMR spectroscopy based metabolomics approaches. Hettinga et al., employed two different GC-MS approaches for quantification of volatile metabolites in milk during clinical mastitis caused by one of the five principal causative organisms, and demonstrated the specificity of distinct volatile metabolite profiles in milk for intramammary infections.13–16 Using a NMR spectroscopy approach, Sundekilde et al., identified differentially expressed metabolites in skimmed milk that differed between samples with low or high somatic cell count (SCC).17 They reported increased amounts of lactate, butyrate, isoleucine, acetate and β-hydroxybutyrate, and decreased amounts of hippurate and fumarate in milk samples with high SCC.
Curiously, there has been no previous report of metabolomics profiling of milk during mastitis using a Liquid Chromatography Mass Spectrometry (LC-MS) approach. Compared with NMR spectroscopy or GC-MS, LC-MS has the potential to analyse a larger proportion of the metabolome due to its high sensitivity.7 Hence we used a LC-MS method to quantify temporal changes in metabolite concentrations in milk during mastitis in an experimental model of the disease. The aim of this part of the overall investigation was to assess the variation in the metabolome in bovine milk samples following progression of the experimental intramammary challenge with a host-adapted strain of Streptococcus uberis (FSL Z1-048).1S. uberis represents an important cause of mastitis in dairies in the UK and it has been shown to cause severe disease which is often difficult to control.18 The goal of the metabolomics analysis was to identify the metabolites that demonstrated either an increase or decrease in milk from infected udder quarters over a time course from pre-infection to resolution. This would provide a better understanding of metabolic pathways altered in mastitis, and along with the results of the immunological, acute phase, peptidomic and proteomic investigations provide a novel insight into the bacterial IMI and the host response.
2. Materials and methods
Cows (n = 6) were challenged with Streptococcus uberis strain FSL Z1-048 in a single bacteriologically negative udder quarter per cow as previously described.1 Aliquots of milk samples collected from six selected time points (0, 36, 42, 57, 81 & 312 hours post-challenge) of the challenge study were used to generate untargeted metabolomics data, as was done in the associated peptidomic2 and proteomic studies.3 The time-points were selected on the basis of the changes in the clinical manifestations, bacterial load and somatic cell counts over the course of the experimental challenge study.1 Body temperature of the cows and bacterial concentrations in milk from challenged quarters peaked from 24 hours (bacteria) or 30 hours (temperature) post-challenge up to 57 hours post-challenge and had decreased to a plateau by 81 hours post-challenge, whereby body temperature had returned to normal and bacterial concentrations in culture positive quarters stayed constant until the end of the study at 312 hours post-challenge. All animal experiments were conducted at the Moredun Research Institute (Penicuik,UK) with approval of the Institute's Experiments and Ethical Review Committee in accordance with the Animals (Scientific Procedures) Act 1986.1
2.1 Untargeted metabolomic data generation
The aliquots of milk samples were stored at −20 °C at the Moredun Research Institute, Edinburgh and were transported frozen to Garscube campus of the University of Glasgow for metabolomic data generation and analysis. The samples were thawed at 4 °C and metabolites were extracted using chloroform and methanol (1
:
3 v/v) mixture.19,20 400 μl of 1
:
3 (v/v) chloroform and methanol mixture was added to 100 μl of skimmed milk sample, and vigorously extracted on a vortex mixer for two hours at 4 °C. The mixture was centrifuged at 13
000 × g for 5 minutes at 4 °C, and then the supernatant was separated and stored at −80 °C until used for LC-MS analysis. A Dionex UltiMate 3000 RSLCnano (liquid chromatography) system coupled to a Thermo Scientific Exactive Orbitrap mass spectrometer was used for LC-MS analysis. Glass vials containing 200 μl of the extracted analyte from the samples were loaded on the RSLC Autosampler connected to a 4.6 × 150 mm SeQuant ZIC-pHILIC (Merck KGaA, 6427 Darmstadt, Germany) column. 10 μl of the analyte was injected in every run. Separation of the analyte was achieved by a mobile phase composed of a two solvent system consisting of solvent A: 20 mM ammonium acetate (pH 9) and solvent B: acetonitrile (ACN) with a flow rate of 300 μl min−1. Chromatographic conditions for LC-MS included a gradient of 80% ACN to 5% ACN (solvent B) in 15 minutes, then held at 5% for 3 minutes, returned to 80% in 1 minute, equilibrated for 6 minutes. The total run time was 25 minutes per sample. The MS acquisition was performed in full scan acquisition mode on both negative and positive polarities using ESI ionization mode. The MS was set at 50
000 resolutions with the scan range from 70–1400 amu. The LC-MS analysis was performed at Glasgow Polyomics, College of Medical, Veterinary and Life Sciences, University of Glasgow, UK.
2.2 Untargeted metabolomic data analysis
The raw LC-MS data obtained from each sample were visually examined by generating a number of plots using MZmine (version 2.10) software.21 To examine sample loading and peak resolution, total ion current (TIC) chromatograms and base peak chromatograms were generated from data obtained from each sample. The raw LC-MS data from the quality control passed samples were imported into the IDEOM22 software package (version 18). Raw data was converted from the Thermo Scientific ‘RAW’ file format to an open-source ‘mzXML’ file format, centroided and split into positive and negative polarities using MSConvert tool.23 Chromatographic peak detection was performed using XCMS24 using the centWave algorithm and saved in the peakML format, peak matching and annotation of related peaks were achieved using mzMatch.R.25 Artefacts and noise were filtered out using IDEOM software using the default parameters. Metabolite identification was performed in IDEOM software package by matching retention times and accurate masses of detected peaks with either the authentic standards (MSI confidence level 1) or the predicted retention times and masses from a previously validated model (MSI confidence level 2).26–28 For improved annotation of metabolites, a mixture of 148 authentic standards were run in the LC-MS system to predict retention times using the IDEOM software. Where there are multiple metabolite names associated with a given mass and retention time, the metabolite names were selected automatically in the IDEOM software as the best match to the database entries of the given mass and formula, and then reviewed manually. In the absence of additional information, these metabolite names must be considered as putatively-annotated hits. Using the Partek® Genomics Suite® (version 6.6)29 software, principal components analysis (PCA) and hierarchical clustering analysis (Euclidian distance and average linkage) were performed on the combined peak intensities from positive and negative polarities that were processed using IDEOM. To identify differentially expressed metabolites, a T-test with time as factor, comparing each time-point with time-point 0 hour post-challenge was performed using the IDEOM software. In addition, one-way analysis of variance (ANOVA) test with time as factor was performed on the putatively identified metabolites data, and using a threshold of an absolute fold-change more than 2 and FDR-adjusted p-value less than 0.05, differentially expressed metabolites lists were generated by comparing each time-point with 0 hour time-point. Further, the list of the identified metabolites were exported from IDEOM to Pathos30 and iPath31 web-based metabolomics tools to identify the represented metabolic pathways and to visualize the metabolic pathways in which the metabolites are generally present.
3. Results
3.1 Quantification of metabolites
Out of the 36 samples (milk from six cows at six selected time points), only 32 samples passed the initial quality control and were subsequently included in the downstream analysis. The base peak chromatograms showed overall consistency between the replicates in each time-point (Fig. S1, ESI†). A total of 3828 different peaks were detected over all 32 samples analysed, 1027 peaks were in the positive ionisation mode while 2801 were in the negative ionisation mode. Out of the peaks detected, after resolving adducts and charged states, 1043 features (potential metabolites) were deduced, and from that 740 metabolites were identified by IDEOM (Table S1, ESI†), and then they were reviewed to remove multiple identities, thus reducing the number to 690 putatively identified metabolites (Table S2, ESI†). Overall, the mass of metabolites identified ranged between 69 and 888 Da. Exploratory data analysis such as hierarchical clustering analysis and principal components analysis were performed on the combined chromatographic peak intensities from positive and negative polarities after removing the noisy peaks.
3.2 Hierarchical clustering analysis
To explore the dataset, a hierarchical clustering analysis (HCA) using Euclidean distance and average linkage agglomeration method was performed on the peak intensity data from the 3828 chromatographic peaks combined from both negative and positive polarities. The hierarchical clustering analysis (Fig. 1) shows three top-level clusters in the column dendrogram. Cluster A on the top right hand side includes milk samples from 36 hour (shown in grey) and 42 hour (shown in violet) post-challenge, corresponding to the early stages of the infection and inflammation, which is characterized by bacterial growth and cytokine release.1 It also has milk samples from 57 hour (shown in orange) and 81 hour (shown in red) post-challenge of cow 5, which was previously identified as a late responder based on clinical manifestations and cytokine profiling,1 and 57 hour post-challenge samples from cows 1 and 4. Cluster B, which is in the middle, includes samples exclusively from 57 hour and 81 hour post-challenge, and corresponds to the decreasing bacterial load.1 Cluster C is the farthest from right, and includes all the samples from 0 hour (shown in green) and 312 hour (shown in blue) post-challenge, which reflects the similarity between the pre-infection and the late resolution (mostly cleared of infection) stages. It also includes 36 hour samples from cow 5 and 1, and 42-hour post-challenge sample from cow 5.
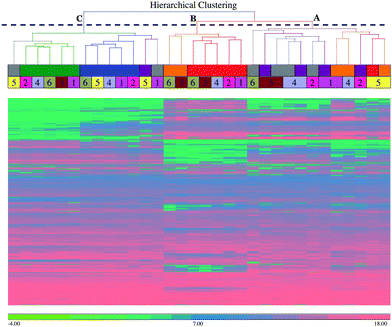 |
| Fig. 1 Hierarchical clustering analysis of the detected peaks showing column dendrograms. Hierarchical clustering analysis was performed on the 3828 detected peak intensities using Euclidean distance and average linkage agglomeration methods. The column dendrograms show the clustering of the milk samples. The column dendrograms also show three top-level clusters, identified by letters (A = early to peak infection based on bacterial numbers; B = post peak infection; C = pre-challenge and resolution stage), time points by colours (see inset), and individual cows by numbers. The scale bar shows the intensities in log2 scale. Please note that there are only 32 samples as data from 4 samples were not included after initial quality analysis at raw data level. | |
3.3 Principal component analysis
To further explore the dataset, a principal component analysis (PCA) was performed on the combined peak intensities (3828 chromatographic peaks) data. The PCA plot (Fig. 2) shows the plotting of samples using principal component 1 (PC1) and principal component 2 (PC2). The clustering pattern of samples in the PCA is similar to the HCA, and reflects the time course. Overall, the clusters are separated on the PC1, which has captured 40.4% of variance in the dataset. The samples at time points 0 hour post-challenge and 312 hour post-challenge formed distinctive clusters, and are shown in Fig. 2 indicated by green and blue respectively, are closer compared to the samples from other time points. The clusters formed by time-points 0 hour and 81 hour post-challenge samples has the greatest distance on PC1, and the clusters formed by samples from other time-points are located between these two extremes. As in the HCA, samples from cow 5 are seen as outliers showing slow response evidenced by the clinical, bacteriological and biochemical parameters.1
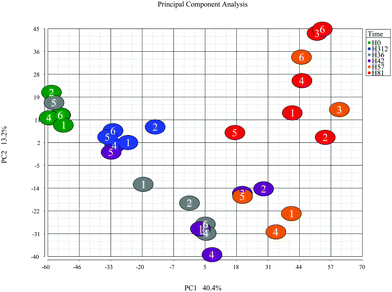 |
| Fig. 2 Principal component analysis of the metabolome after intramammary challenge with Streptococcus uberis. The PCA was based on the intensities from 3828 detected peaks and the plot was generated using the Partek Genomic suite. The data points refer to milk samples obtained from 6 cows at 6 time points post challenge (PC). Cows are identified by number and time points by colour, with hours PC shown in the legend. Please note that there are only 32 samples as data from 4 samples were not included after initial quality analysis at raw data level. | |
3.4 Differential expression analysis
To identify the metabolites that were differentially expressed over the time course, particularly between pre- and post-challenge, a one-way ANOVA test was performed with time as factor. The lists of differentially expressed metabolites (Table S2, ESI†) were created for each comparison using a threshold of an absolute fold-change more than 2 and FDR-adjusted p-value less than 0.05. Compared with the pre-challenge time-point, there were 222 (156 up & 66 down), 310 (193 up & 117 down), 476 (277 up & 199 down), 490 (303 up & 187 down) and 133 (104 up & 29 down) putative metabolites differentially expressed respectively at 36 hours, 42 hours, 57 hours, 81 hours and 312 hours post-challenge.
3.5 Perturbations in the metabolic pathways
Most of the annotated metabolites were mapped to KEGG reference pathways,32 and the results showed alterations to a number of mapped pathways including amino acid metabolism such as alanine, aspartate and glutamate metabolism, nucleotide metabolism such as purine and pyrimidine metabolism, carbohydrate metabolism such as ascorbate and aldarate metabolism, lipid metabolism such as Eicosanoids pathway. There were significant changes in the di-, tri- and tetra-peptides concentrations in milk over the time course of the experimental challenge. Heat map (Fig. 3) plotting the fold-changes of metabolite concentrations mapped to amino acid metabolism, carbohydrate metabolism, lipid metabolism, nucleotide metabolism and di-, tri- and tetra-peptides shows increasing trend in lipid metabolism and di-, tri- and tetra-peptides up to 81 hours post-challenge. Conversely, the majority of the metabolites mapped to carbohydrate metabolism and nucleotide metabolism show a decreasing trend in concentration up to 81 hours post-challenge. The observations were further corroborated by the results from Pathos web-based tool that showed the intensity of changes in KEGG metabolic pathways at each post-challenge time-point compared to the pre-challenge metabolite levels (Tables S3–S7, ESI†). In addition, the mapping of metabolites on the KEGG metabolic, regulatory and biosynthesis pathways were visually examined using iPath web-based tool (Fig. S2–S4, ESI†).
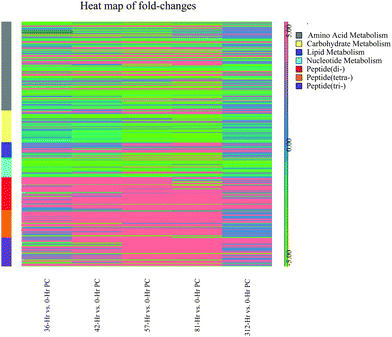 |
| Fig. 3 Heat map showing the fold-changes of putative metabolites mapped to KEGG metabolic pathways. Fold-change of putative metabolites in each contrast (each time-point compared with 0 hour post-challenge) was computed from the one-way ANOVA test. The metabolites were mapped to KEGG metabolic pathways using IDEOM software, and then the heatmap was plotted using the Partek Genomic suite. | |
4. Discussion
This study was an untargeted global metabolomics investigation of skimmed milk, carried out to characterise the metabolite profile of skimmed milk and its changes with time during the course of an intramammary challenge with a host-adapted strain of S. uberis, an important environmental pathogen of mastitis. Of particular importance is the ability to relate the findings of this metabolomic investigation with the pathophysiological, immunological, proteomic and peptidomic changes described in the previous and accompanying reports.1 All data obtained from post infection time-points were statistically compared with values at 0 hours. It is expected that metabolomic investigation of milk would yield a high number of metabolites17 and in this analysis over 3000 chromatographic peaks were detected, of which 690 were putatively annotated with a definitive metabolite. The number of compounds identified in this study is by far the largest in any previous metabolomics study using bovine milk.8,15,33 This may be due to the methodology used, LC-MS, which is known to be of higher sensitivity than other metabolomics techniques such as H-NMR spectroscopy, although having its own disadvantages such as lower reproducibility and difficulty in identifying spectral features.7 While many methods exist for extraction of metabolites, we used chloroform and methanol (1
:
3 v/v) mixture, based on its complementarity with the LC-MS system in our in-house experience.28 This method is based on the original Folch method34 and is known to be effective for the extraction of a broad range of metabolites including lipids.19,20,35
A notable finding of this study is the change in metabolite composition of bovine milk over the course of mastitis caused by the host-adapted strain of Streptococcus uberis. The time-points used in the omics analyses include a pre-infection (0 hours post-challenge), peak bacterial load and peak body temperature of cows (36 hours post-challenge), rapidly declining bacterial load and body temperature of cows (42, 57 and 81 hours post-challenge) and spontaneous clearing of infection with one cow being an exception (312 hours post-challenge). The number of differentially expressed metabolites increased over the course of infection, and peaked at 81 hours post-challenge. The number of modulated metabolites, and the amplitude of change, peaked at 81 hours post-challenge. These patterns were similar to those found by peptidomic analysis and in the proteomic analysis, although in the latter a number of proteins peaked at 57 hours post challenge. However, principal component analysis and hierarchical clustering analysis of both the metabolomic and proteomics datasets showed comparable patterns in that the samples from 57 hours and 81 hours are divergent from 0, 36 and 42 hours post-challenge. However, these patterns are contradictory to the clinical and bacteriological profiles where the largest change occurs at 36 hours post-challenge.
We observed increasing concentrations of bile acids such as taurochenodeoxycholic acid (C26H45NO6S), taurocholic acid (C26H45NO7S), glycocholate (C26H43NO6), glycodeoxycholate (C26H43NO5) and cholate (C24H40O5) over the time course until 81 hours post-challenge (Fig. 4). The bile acids are produced by liver and are thought to have antimicrobial activity through their detergent property in the intestinal tract.36,37 Their immunomodulatory roles are thought to be mediated through the farnesoid X receptor (FXR) pathway,38 which was one of the pathways enriched in the proteomics analysis.3 As there is evidence in both metabolomic and proteomics analysis, the involvement of the FXR pathway in bovine mastitis could be studied in detail. In addition to FXR, 3 other nuclear receptors involved in immunomodulatory activities (pregnane X receptor (PXR), constitutive androstane receptor (CAR) and vitamin D receptor (VDR)) are known to be activated by specific bile acids.39,40 Increased intracellular bile acids concentration results in the transcriptional activation of these nuclear receptors. Activated FXR ligands exert anti-inflammatory activity through their interaction with other transcription factors including activator protein 1 and nuclear factor-κB (NF-κB).41 Similarly, PXR exhibits anti-inflammatory role by inhibiting the expression of NF-κB target genes, and the production of interleukins and chemokines.40,42 Likewise, vitamin D3 plays an inhibitory role in the production of pro-inflammatory cytokines.40,43 Furthermore, immunomodulatory role of bile acids can be linked to TGR5, a bile acid activated G-protein-coupled receptor which increases the production of cAMP in innate immune cells leading to downregulation of inflammatory cytokines such as tumour necrosis factor alpha (TNF-α), interleukin-1 beta (IL-1β), interleukin-6 (IL-6) and interleukin-8 (IL-8).44,45 Interestingly, profiles of pro-inflammatory cytokines in milk (Fig. 8, Tassi et al.1) over the time course in our study were comparable with the concentrations of bile acids in milk quantified in this analysis. Peak concentrations of TNF-α, IL-1β, IL-6 and IL-8 in milk were found between 36 and 48 hours post-challenge,1 and as the concentrations of bile acids increased, the concentration of pro-inflammatory cytokines decreased. Furthermore, peroxisome proliferator-activated receptors (PPAR) signalling, retinoid X receptor (RXR) activation and liver X receptor (LXR) activation signalling pathways, which are known to be associated with bile acids metabolism and signalling39 were found to be enriched in the proteomic analysis.3
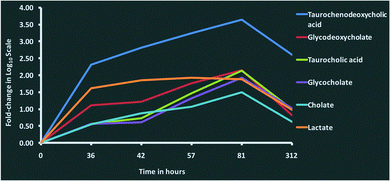 |
| Fig. 4 Changes in the concentration of bile acids and lactate in milk after intramammary challenge with Streptococcus uberis. Fold-changes for each metabolite at 36, 42, 57, 81 and 312 hours post-challenge compared with 0 hours post-challenge were analysed using a one-way ANOVA. The time course profile of fold-changes shows the increasing concentration of bile acids and lactate over the course of the infection, reaching highest levels at 81 hours post-challenge, and then dropping down to pre-infection levels at 312 hours. This figure shows fold-change in log10 scale. | |
We found hippurate (C9H9NO3) concentration decreasing over time, with its lowest level reaching at 57 hours post-challenge. Similarly, lactose (C12H22O11) concentration decreased over time,46,47 and could not be detected at 81 hours post-challenge. The decreasing trend of lactose concentration in milk is supported by the proteomics analysis in which alpha-lactalbumin, a regulatory subunit of lactose synthase involved in the lactose synthesis, was down-regulated over the time course.3 A previous study showed decreased concentrations of hippurate and lactose in milk associated with the elevated SCC,17 and suggested that the decreased concentration of lactose could be to maintain osmotic pressure of milk to compensate the flow of blood constituents into milk. We also observed increased concentrations of lactate (C3H6O3) over the time course with the highest concentration at 42 hours post-challenge. Lactate is an end product of bacterial metabolism15,17 and correlates with the high bacterial load in milk, but could also be due to an increase in anaerobic metabolism in the host. Using a NMR spectroscopy based metabolomics approach, Sundekilde et al., reported increased concentration of isoleucine in milk with the elevated SCC.17 In our study we found up-regulation of leucine (C6H13NO2) over the time course, with its highest concentration at 81 hours post-challenge. Identification of isomers such as leucine and isoleucine is a limitation in the LC-MS based methodology compared with the NMR spectroscopy, and this might well be isoleucine instead of leucine in our case.
Mapping the metabolites to KEGG pathways, we identified perturbations in amino acid metabolism, carbohydrate metabolism, lipid metabolism, nucleotide metabolism and metabolism of di-, tri- and tetra-peptides. This is further supported by our peptidomic study.2 The increasing trend in the metabolism of di-, tri- and tetra-peptides over the time course post-challenge (Fig. 3) could be attributed to the bacterial lysis of proteins. Most of these compounds were not detected at 0 hours, but their concentration increased at 36, 42, 57 and 81 hours post-challenge, and then decreased (or were not detected) at 312 hours post-challenge, by which time the infection was resolved. It is possible that the increase in small molecular weight peptides is due to the activities of plasma proteases such as plasmin, leukocyte associated proteases and cathepsins, as well as bacterial proteases.48,49 There is a decreasing trend in carbohydrate metabolism over the time course, and this could be due to the utilization of carbohydrates by bacteria or their production may be inhibited as part of host response to deprive the bacteria of readily available energy substrates. We also observed down-regulation of lipid metabolism over the time course with the increase of inflammation. The sample extraction method and the chromatographic separation might significantly affect the discovery of the lipid compounds, and a specialised lipidomic method should be used to study the lipid compounds. With this limitation, we found that most lipids were eluted in the first 5 minutes of the LC-MS run. There was a mixed trend in the Eicosanoids pathway, which is an important metabolic pathway for arachidonic acid metabolism. 18-Acetoxy-PGF2alpha-11-acetate (C24H38O8), a prostaglandin in the Eicosanoids pathway was not detected at 0 hours and 312 hours post-challenge, but present in the rest of the time-points, while 2,3-dinor-8-iso-PGF2alpha (C18H30O5) another compound in the Eicosanoids pathway and a product of prostaglandin metabolism showed increasing trend, peaking at 81 hours post-challenge. However, PGF2-alpha methyl ether (C21H38O4) was significantly down-regulated over the course with its lowest level at 81 hours post-challenge (fold-change = −4.3375, FDR-adjusted p-value = 0.0421). Eicosanoids, particularly PGF2-alpha, are important mediators in the acute inflammatory process, and prostaglandins are known to be up-regulated in milk during mastitis.50
The integrated omic analyses that have been undertaken on the milk samples taken over 312 hours following IMI with S. uberis and linking to the clinical and pathophysiological analyses on the same samples provides a unique view on the multifaceted host responses to the bacterial infection. This system-based approach has been most revealing in providing an insight into the integration of the responses mounted by the mammary gland in the face of bacterial invasion. A key finding has been that while the bacterial count reaches a peak within 36–42 hours post challenge, many of the host responses do not reach a peak until a 57 or 81 hours post challenge (Fig. 5). As this pattern is present in all three omics analyses (peptidomics, proteomics and metabolomics), it provides opportunities for further research. The results here would have benefited from further time point analysis in the resolution phase of the infection between 81 and 312 hours post-challenge. However, it is clear that by using multiple omics technologies, the connectivity of bacterial invasion, cytokine mediation leading to protein, peptide and metabolite modifications during mastitis can be characterised. Among omic technologies there are a variety of approaches to be taken. In proteomics, 2 dimension electrophoresis, difference gel electrophoresis as well as the CE-MS and LC-MS/MS approach used in this series of experiments2,3 while alternatives such as nuclear magnetic resonance could also be used for metabolomics. The selected approaches were used as being the most suitable for this sample set in order to demonstrate the value of integrated omics in a systems based investigation of bovine mastitis. This polyomic approach should be applied to assessment of comparative responses to other mastitis causing pathogens to determine how the responses are altered due to bacterial species with the potential of a differential biomarker panel of proteins, peptides and metabolites being developed. The data generated by the LC-MS metabolomics analysis is available in the ESI† (Table S1) and others working in the field may identify further responses to mastitis in pathways not highlighted here. Modification to the multi-analytes covered by these investigations in response to mastitis therapy, whether by pharmacological or biologic agent or by vaccination would be a further potential application of the integrated approach to the study of mastitomics.
 |
| Fig. 5 The relative responses of analytes following experimental induced S. uberis mastitis combining metabolomic results with data from Tassi et al.,1 Thomas et al.,2 and Mudaliar et al.3 The shading represents strength of the response relative to the peak response. Responses were increases compared to day 0 levels except for casein levels (indicated by *), which decreased after challenge. cfu ml−1 – bacteria count in colony forming units per ml;1 SCC – somatic cell count;1 IL – interleukin;1 TNF – tumor necrosis factor;1 LF – lactoferrin;2 Hp (ELISA) – haptoglobin as detected by enzyme linked immunosorbent assay;2 mSAA3 – milk derived serum amyloid A;2 CRP – C-reactive protein;2 IMI77 – peptidomic profile based on 77 peptides;2 Hp (LC-MS/MS) – haptoglobin as detected by liquid chromatography and tandem mass spectrometry;3 PepGly – peptidoglycan recognition protein 1;3 Cath5 – cathelicidin-5;3 Annex1 – Annexin A1;3 PhenylPro – phenylpropanoate. | |
5. Conclusions
The present study used an LC-MS-based untargeted metabolomic approach to profile the changes in metabolite concentration in milk during the course of the experimental S. uberis mastitis infection. We identified changes in several hundred metabolites over the course of the infection. Significantly, we found changes in the concentration of bile acids in milk and compared them with the concentration of cytokines suggesting anti-inflammatory role of the bile acid receptor pathway. Involvement of bile acids in the resolution of mastitis through activation of nuclear receptors could potentially a novel discovery in this study. We compared the observations in the current study with the proteomics and peptidomics studies associated with the same sample set. This system-wide analysis of peptides, proteins and other metabolites in milk and the changes in the clinical manifestations and bacterial load provided a unique view of the pathological processes in bovine mastitis. Particularly, we found links to enrichment of FXR pathway in the proteomics data and the increased concentration of bile acids in the metabolomic data. Similarly, the down-regulation of lactose over the course of mastitis could be associated with the down-regulation of alpha-lactalbumin. We have also demonstrated high correlation in the dynamics of peptides, proteins and metabolites over the course of the experimental infection.
Acknowledgements
University of Agriculture Abeokuta (Nigeria), Tertiary education trust fund and Zoetis are gratefully acknowledged for the funding of the studentship and the research. The work was carried out in Glasgow Polyomics, which is supported by the Wellcome Trust (grant no. 097821/Z/11/Z). It was also financially supported by the Moredun Innovation Fund (RT) and the Scottish Government's Rural and Environment Science and Analytical Services Division (TM and RZ). We thank Yoann Gloaguen, University of Glasgow, for technical support in metabolomics data analysis.
References
- R. Tassi, T. N. McNeilly, J. L. Fitzpatrick, M. C. Fontaine, D. Reddick, C. Ramage, M. Lutton, Y. H. Schukken and R. N. Zadoks, J. Dairy Sci., 2013, 96, 5129–5145 CrossRef CAS PubMed.
- F. C. Thomas, W. Mullen, R. Tassi, A. Ramírez-Torres, M. Mudaliar, T. N. McNeilly, R. N. Zadoks, R. Burchmore and P. D. Eckersall, Mol. BioSyst., 2016 10.1039/c6mb00239k.
- M. Mudaliar, R. Tassi, F. C. Thomas, T. N. McNeilly, S. K. Weidt, M. McLaughlin, D. Wilson, R. Burchmore, P. Herzyk, P. D. Eckersall and R. N. Zadoks, Mol. BioSyst., 2016 10.1039/c6mb00290k.
- R. Fischer, P. Bowness and B. M. Kessler, Proteomics, 2013, 13, 3371–3386 CrossRef CAS PubMed.
- M. Fillet and M. Frédérich, Drug Discovery Today: Technol., 2015, 13, 19–24 CrossRef PubMed.
- U. Roessner and J. Bowne, Biotechniques, 2009, 46, 363–365 CrossRef CAS PubMed.
- D. S. Wishart, Nat. Rev. Drug Discovery, 2016 DOI:10.1038/nrd.2016.32.
- K. J. Boudonck, M. W. Mitchell, J. Wulff and J. A. Ryals, Metabolomics, 2009, 5, 375–386 CrossRef CAS.
- M. S. Klein, M. F. Almstetter, G. Schlamberger, N. Nürnberger, K. Dettmer, P. J. Oefner, H. H. D. Meyer, S. Wiedemann and W. Gronwald, J. Dairy Sci., 2010, 93, 1539–1550 CrossRef CAS PubMed.
- R. Lamanna, A. Braca, E. Di Paolo and G. Imparato, Magn. Reson. Chem., 2011, 49, S22–S26 CrossRef CAS PubMed.
-
U. K. Sundekilde, Milk metabolite variability and heritability and their association with technological properties of bovine milk elucidated by NMR-based metabonomics, PhD thesis, @Århus University, Department of Food Science, @Årslev, 2012 Search PubMed.
- U. K. Sundekilde, L. B. Larsen and H. C. Bertram, Metabolites, 2013, 3, 204–222 CrossRef CAS PubMed.
- K. A. Hettinga, H. J. F. van Valenberg, T. J. G. M. Lam and A. C. M. van Hooijdonk, J. Dairy Sci., 2009, 92, 4901–4905 CrossRef CAS PubMed.
- K. A. Hettinga, H. J. F. van Valenberg, T. J. G. M. Lam and A. C. M. van Hooijdonk, J. Dairy Sci., 2008, 91, 3834–3839 CrossRef CAS PubMed.
- K. A. Hettinga, H. J. F. van Valenberg, T. J. G. M. Lam and A. C. M. van Hooijdonk, Vet. Microbiol., 2009, 137, 384–387 CrossRef CAS PubMed.
- K. A. Hettinga, F. A. M. de Bok and T. J. G. M. Lam, J. Dairy Sci., 2015, 98, 7906–7910 CrossRef CAS PubMed.
- U. K. Sundekilde, N. A. Poulsen, L. B. Larsen and H. C. Bertram, J. Dairy Sci., 2013, 96, 290–299 CrossRef CAS PubMed.
- R. Zadoks, CAB Rev., 2007, 2, 1–15 Search PubMed.
- A. Beltran, M. Suarez, M. A. Rodriguez, M. Vinaixa, S. Samino, L. Arola, X. Correig and O. Yanes, Anal. Chem., 2012, 84, 5838–5844 CrossRef CAS PubMed.
- A. B. Canelas, A. ten Pierick, C. Ras, R. M. Seifar, J. C. van Dam, W. M. van Gulik and J. J. Heijnen, Anal. Chem., 2009, 81, 7379–7389 CrossRef CAS PubMed.
- T. Pluskal, S. Castillo, A. Villar-Briones and M. Oresic, BMC Bioinf., 2010, 11, 395 CrossRef PubMed.
- D. J. Creek, A. Jankevics, K. E. Burgess, R. Breitling and M. P. Barrett, Bioinformatics, 2012, 28, 1048–1049 CrossRef CAS PubMed.
- J. D. Holman, D. L. Tabb and P. Mallick, Curr. Protoc. Bioinformatics, 2014, 46, 13.24.1–13.24.9 Search PubMed.
- R. Tautenhahn, C. Bottcher and S. Neumann, BMC Bioinf., 2008, 9, 504 CrossRef PubMed.
- R. A. Scheltema, A. Jankevics, R. C. Jansen, M. A. Swertz and R. Breitling, Anal. Chem., 2011, 83, 2786–2793 CrossRef CAS PubMed.
- R. M. Salek, C. Steinbeck, M. R. Viant, R. Goodacre and W. B. Dunn, GigaScience, 2013, 2, 13 CrossRef PubMed.
- L. W. Sumner, A. Amberg, D. Barrett, M. H. Beale, R. Beger, C. A. Daykin, T. W. Fan, O. Fiehn, R. Goodacre, J. L. Griffin, T. Hankemeier, N. Hardy, J. Harnly, R. Higashi, J. Kopka, A. N. Lane, J. C. Lindon, P. Marriott, A. W. Nicholls, M. D. Reily, J. J. Thaden and M. R. Viant, Metabolomics, 2007, 3, 211–221 CrossRef CAS PubMed.
- D. J. Creek, A. Jankevics, R. Breitling, D. G. Watson, M. P. Barrett and K. E. Burgess, Anal. Chem., 2011, 83, 8703–8710 CrossRef CAS PubMed.
- C. M. Speakman, T. C. E. Domke, W. Wongpaiboonwattana, K. Sanders, M. Mudaliar, D. F. M. Van Aalten, G. J. Barton and M. P. Stavridis, Stem Cells, 2014, 32, 2605–2615 CrossRef CAS PubMed.
- D. P. Leader, K. Burgess, D. Creek and M. P. Barrett, Rapid Commun. Mass Spectrom., 2011, 25, 3422–3426 CrossRef CAS PubMed.
- T. Yamada, I. Letunic, S. Okuda, M. Kanehisa and P. Bork, Nucleic Acids Res., 2011, 39, W412–W415 CrossRef CAS PubMed.
- M. Kanehisa, Y. Sato, M. Kawashima, M. Furumichi and M. Tanabe, Nucleic Acids Res., 2016, 44, D457–D462 CrossRef PubMed.
- U. K. Sundekilde, N. A. Poulsen, L. B. Larsen and H. C. Bertram, J. Dairy Sci., 2013, 96, 290–299 CrossRef CAS PubMed.
- J. Folch, M. Lees and G. H. Sloane Stanley, J. Biol. Chem., 1957, 226, 497–509 CAS.
- A. Reis, A. Rudnitskaya, G. J. Blackburn, N. Mohd Fauzi, A. R. Pitt and C. M. Spickett, J. Lipid Res., 2013, 54, 1812–1824 CrossRef CAS PubMed.
- A. F. Hofmann and L. Eckmann, Proc. Natl. Acad. Sci. U. S. A., 2006, 103, 4333–4334 CrossRef CAS PubMed.
- J. Y. Sung, E. A. Shaffer and J. W. Costerton, Dig. Dis. Sci., 1993, 38, 2104–2112 CrossRef CAS PubMed.
- Y. Calmus and R. Poupon, Clin. Res. Hepatol. Gastroenterol., 2014, 38, 550–556 CrossRef CAS PubMed.
- J. Y. Chiang, Compr. Physiol., 2013, 3, 1191–1212 Search PubMed.
- S. Sipka and G. Bruckner, Int. Arch. Allergy Immunol., 2014, 165, 1–8 CrossRef CAS PubMed.
- Y. D. Wang, W. D. Chen, M. Wang, D. Yu, B. M. Forman and W. Huang, Hepatology, 2008, 48, 1632–1643 CrossRef CAS PubMed.
- B. Zhang, W. Xie and M. D. Krasowski, Pharmacogenomics, 2008, 9, 1695–1709 CrossRef CAS PubMed.
- Y. Zhang, D. Y. Leung, B. N. Richers, Y. Liu, L. K. Remigio, D. W. Riches and E. Goleva, J. Immunol., 2012, 188, 2127–2135 CrossRef CAS PubMed.
- K. Hogenauer, L. Arista, N. Schmiedeberg, G. Werner, H. Jaksche, R. Bouhelal, D. G. Nguyen, B. G. Bhat, L. Raad, C. Rauld and J. M. Carballido, J. Med. Chem., 2014, 57, 10343–10354 CrossRef CAS PubMed.
- H. Duboc, Y. Tache and A. F. Hofmann, Dig. Liver Dis., 2014, 46, 302–312 CrossRef CAS PubMed.
- H. Ogola, A. Shitandi and J. Nanua, J. Vet. Sci., 2007, 8, 237–242 CrossRef PubMed.
- C. B. Malek dos Reis, J. R. Barreiro, L. Mestieri, M. A. Porcionato and M. V. dos Santos, BMC Vet. Res., 2013, 9, 67 CrossRef PubMed.
- T. Larsen, C. M. Rontved, K. L. Ingvartsen, L. Vels and M. Bjerring, Animal, 2010, 4, 1672–1679 CrossRef CAS PubMed.
- K. Haddadi, F. Moussaoui, I. Hebia, F. Laurent and Y. Le Roux, Reprod., Nutr., Dev., 2005, 45, 485–496 CrossRef CAS PubMed.
- F. Atroshi, J. Parantainen, S. Sankari and T. Osterman, Res. Vet. Sci., 1986, 40, 361–366 CAS.
Footnotes |
† Electronic supplementary information (ESI) available. See DOI: 10.1039/c6mb00289g |
‡ These authors contributed equally to this work, and shared first authorship. |
|
This journal is © The Royal Society of Chemistry 2016 |