DOI:
10.1039/C6AN01797E
(Paper)
Analyst, 2017,
142, 156-168
FTIR imaging of the molecular burden around Aβ deposits in an early-stage 3-Tg-APP-PSP1-TAU mouse model of Alzheimer's disease
Received
9th August 2016
, Accepted 27th November 2016
First published on 28th November 2016
Abstract
Alzheimer's disease is one of the major causes of dementia in the elderly. The disease is caused by the misfolding of water soluble alpha-helical proteins, which leads to the accumulation of β-sheets in the form of amyloid plaques, which can subsequently affect surrounding tissue areas by oxidative stress neurotoxicity. The aim of the present study was to design a novel methodology to analyze the extent to the neuronal burden around protein-rich Aβ plaques suspected to affect molecular components by oxidative stress induced by inflammatory states. To do so, sagittal brain tissue sections from triple transgenic APPxPSP1xTAU mice were used to carry high magnification FTIR-FPA bench-top chemical imaging. The study used the combination of chemometric procedures involving spectral curve fitting and image processing to study the molecular changes occurring around the plaques. The study shows the performance of the approach by demonstrating its usefulness to co-localize molecular changes to different areas around the plaques. The results, although very preliminary, point to the strong interplay between the distance from the plaque and co-accumulation of molecular components indicative of inflammatory states.
Introduction
Of all the human central nervous system diseases, Alzheimer's disease (AD) has deservedly received great attention as this age-associated disease causes the progressive decline in both cognitive and bodily functions.1 AD is the most prevalent dementia in the elderly affecting around 24 million globally which is likely to quadruple before 2050.2 The pathological features of AD appear within the hippocampus and frontal cortex, whilst it is thought that the disease affects the former at the onset.3,4 The main hallmark lesions of the AD pathology are the extracellular plaques of β-amyloid (Aβ) assumed to develop before the emergence of the symptoms.4 They are made of a 40–42 amino-acid long polypeptide called Aβ that derives from β- and γ-secretase-mediated sequential cleavage of the amyloid-precursor-protein (APP). Two major Aβ proteins, Aβ1–42 and Aβ1–40, are thought to differentially contribute to the pathology. A number of studies have reported Aβ1–42 more pathogenic as it is more prone to fibrilization, but much less is known about the reason for this.5 However, the study by Kim and Hecht proposed its ability for aggregation is due to two additional hydrophobic amino-acids at its C-terminal end.6 Evidence suggests that upon its aggregation into Aβ plaque, an innate immune response is triggered on the site involving mostly microglial cells. Importantly, the detection of pathological triggers by microglial cells is stimulated by a variety of cell-surface receptors that recognize different danger-associated molecular patterns (DAMPs) including those present in Aβ fibrils or pathogen-associated molecular patterns (PAMPs).7 Previous studies have reported a causative role for Aβ plaques in the production of reactive oxygen species (ROS) that have a very high potential to damage all cellular components.8 Recently, it was also found that 4-hydroxynonenal (HNE), an aldehyde product of lipid peroxidation, is present in high levels in the brains affected by Aβ pathology, which also supports a putative relationship of oxidative stress neurotoxicity caused by ROS over-production and brain amyloid burden.9
To address the problem of the genesis and spread out of the AD disease, there has been an increasing interest in the analytical methods to extract the subtle biochemical information on Aβ pathology. One of the greatest challenges was to find a technique that is capable of analyzing a myriad of molecules at a (sub)cellular spatial resolution in situ with special emphasis on proteins particularly prone to various effects of oxidative stress.10 Fourier Transform Infrared Spectroscopy (FTIR) has been shown as an excellent method to probe the chemical and structural composition of brain samples at the desired resolution.11 This label-free spectroscopic method does not require any complicated sample preparation protocols, hence thin (∼10 μm) neural tissue sections (predominantly subjected to freeze drying, desiccation) can be analyzed in air with satisfactory signal-to-noise ratio.10 The first serious attempts to analyze AD pathology using FTIR spectroscopy coupled with a synchrotron source (SR) revealed the Congo red-positive human-derived Aβ plaques to exhibit slightly elevated levels of β-sheets and reduced contents of random coils and alpha-helices. However, the FTIR analyses made use of single-point-raster-scanning mode and operating at relatively low spatial resolution of 12 μm (defined by the size of the aperture). In addition, the authors did not conduct any more sophisticated chemometric analyses in an attempt to separate different secondary structure components.12 The study by Miller et al. presented detailed examination of human-derived Aβ plaques using both single-point FTIR to image molecular components and X-Ray fluorescence microprobe imaging (both using 5–10 μm synchrotron beam) to assess for the content of chemical trace elements. In agreement with the previous research, it was demonstrated the Aβ deposits were enriched in β-sheets and accumulated transition metals: Fe, Cu, Zn.13 Importantly, Cu2+, Fe3+ are thought to be implicated in ROS production and further lipid and protein oxidation in AD-affected brains, while Zn2+ was found to even attenuate the toxicity of Aβ in cell cultures.14 The work by Rak et al. involving similar spatial resolution revealed the plaques found in the hippocampal, cortical and caudal tissue of 21-month-old TgCRND8 mice of two distinct types: dense core and diffuse core Aβ plaques. The former, congo-positive, were recognized based on the elevated content of β sheets, whilst the latter were not detectable using FTIR spectroscopy but visible only upon immunohistochemical staining against anti-Aβ antibody 4G8.15 Recent developments in the state-of-art of the instrumentation have led to successful applications of Focal Plane Array (FPA) FTIR detectors to measure thousands of spectra simultaneously.11,16 One of these studies with synchrotron FTIR-FPA imaging by means of the 64 × 64 pixel array detector (at the effective geometric pixel size of 5.5 μm × 5.5 μm) presented an elevated lipid envelope surrounding dense core plaques in the TgCRND8 animal model of AD. The authors proposed the envelope is due to lipid-rich glial cells infiltrating the Aβ deposits. It was also confirmed the spatial resolution of the method is sufficient to distinguish between the plaque areas and glial cells infiltrating/engulfing the surrounding neuropil.17 Liao et al. using SR-FTIR-FPA imaging of dense core plaques confirmed the presence of lipid envelope around the Aβ plaques found both in the animal model and autopsy brain tissue sections from humans affected by advanced AD.18 Another recent study by Benseny-Cases et al. on the human tissue sections from the AD patients set out with the aim of assessing the regional extent of lipid peroxidation around the fibrillar Aβ deposits. By using single-point SR-FTIR spectroscopy (apertures' size of 8 μm × 8 μm), the authors concluded the co-localization of oxidized lipids in the immediate surroundings of the plaques. Importantly, it was also reported that the Aβ plaques found in the AD-affected brains display significantly higher level of lipid oxidation than those identified in the non-AD aged individuals.19 Further upgrades to FTIR-FPA optics enabled high magnification synchrotron chemical imaging at 0.54 μm effective pixel size using multiple synchrotron beams, though the data had to be oversampled.20 In turn, the correct theory-based rigorous figure of 1.1 μm by a bench-top IR source was more recently reported by Reddy et al., and the system is currently used in the latest commercial instruments.21 Using the FTIR instrument equipped with these optics, Findlay et al. confirmed the previous remarks on lipid envelope around the plaques and demonstrated possibility to perform high-magnification FTIR-FPA experiments on Aβ pathology without the use of SR radiation.22 Taken together, one may propose that upon the infiltration of glial cells, Aβ plaques are capable of producing excessive amounts of free radicals which damage proteins and lipids around. An extension of the above-mentioned studies, employed here, is to attempt curve-fitting of amide I to elucidate the protein secondary structure contents for more cogent and quantitative assessment of the results. An important question that remains unanswered therefore is what is the regional extent of oxidative damage to proteins/lipids within and in close proximity of Aβ plaques.
The general objective of this study was design a methodology for the analysis of Aβ plaques and their surrounding tissue areas. We combined chemical mapping, simple chemometrics and image processing to analyze several adjacent areas around Aβ plaques to correlate the changes in the abundance of various molecular components and colocalize them to various tissue areas adjacent to the deposits. We used the triple transgenic (3xTg) APPxPS1xTAU animal model Alzheimer's disease to study the molecular processes present at the onset of the pathology. The (3xTg) model of AD harbors APPSwe, PS1M146V and tauP301L transgenes and therefore develops all neuropathological correlates of the disease.23
Materials and methods
Sample preparation
Triple mutant (3xTg) mice were housed under regular light/dark conditions and had ad libitium access for food and water. The animals were culled at the age of 15 months to have some early stage histopathological features of AD developed. Once sacrificed, three brains (n = 3) were rapidly frozen in −80 °C, carefully cut into 10 μm thin sagittal tissue sections using a cryomicrotome, put on IR transmissive CaF2 substrate and kept frozen at −80 °C around 4 days to enable the water to sublime out of the samples. From each brain specimen, two adjacent tissue sections were taken. All sections were stored in darkness until the FTIR experiments. Just before the experiment, a sample was taken from the freezer and desiccated (to remove the excess of water aggregating at the top of a sample). All procedures were carried out in accordance with the Animals (Scientific Procedures) Act 1986, the specific project license was approved by the UK Home Office.
FTIR experiments
The FTIR-FPA transmission maps were recorded using an Agilent Cary 670-IR spectrometer coupled with a Cary 620-IR imaging microscope using 128 × 128 FPA detector in high-magnification mode to enable the effective pixel size of 1.1 μm × 1.1 μm. Although this pixel size is not the same as the spatial resolution it has been shown that smaller pixel size significantly increases the fidelity of the images obtained20 and it has also been shown that if the spectral output of features are different it is possible to obtain images with a resolution exceeding the expected diffraction limit.24 The spectra were collected in air in 4000–950 cm−1 spectral range at a spectral resolution of 5 cm−1 with a zero filling factor 1 to lead to data spacing of 2.5 cm−1. Just before the background measurements, the 20 minutes purging of the setup was applied to avoid any uncompensated contributions arising from CO2 and water vapor in the FTIR spectra. We used 512 and 256 scans co-added for background and for sample acquisitions, respectively. For the interferograms, the Blackmann-Harris 4-point apodisation function was used.
For orientation purposes Fig. 1a shows a large area optical microscope image of the hippocampus and cortex (the main area where plaques can be found). A plaque in the cortex is identified in the insert Fig. 1b. Fig. 1c and d shows the FTIR image of the same region representing the amide I band intensity and the CH stretching region intensity respectively. Fig. 1e and f show the FTIR images of the plaque in Fig. 1b at 5.5 μm and 1.1 μm pixel resolution respectively.
 |
| Fig. 1 (a) Large tissue area microscopic image; (b) microscopic image of the optically dense dark cortex deposit; (c) the distribution of the absorbance at 1660 cm−1 (amide I); (d) the distribution of the absorbance at 2960 cm−1 (CH str); (e) low resolution (5.5 μm) amide I imaging of the deposit; (f) high magnification (1.1 μm) amide I imaging of the deposit. | |
Data pre-processing
Fig. 2a shows the FTIR spectra acquired within the Aβ plaques. The spectra revealed strong Mie scattering distortions, as their baselines were of oscillatory nature. The absorbance at 2500 cm−1 was used as an indicator of this effect within the Aβ deposits. To alleviate the contribution of this phenomenon, the spectra were subjected to the RMies-EMSC scattering correction involving 7 iterations.25–27 High-throughput computations of thousands of FTIR spectra simultaneously were assisted with the HTCondor pool at the University of Manchester, compromised of thousands of nodes.28 Further data-processing steps were implemented using Python 2.7 with scipy and numpy modules.29 Once corrected, the spectra were quality tested based on the height of the band at 1655 cm−1 with minimal permissible height of this band set to 0.05. The FTIR spectra were subsequently truncated to desired range between 1750–950 cm−1 (fingerprint) as well as 3050–2750 cm−1 (lipid) and baseline corrected (a simple linear baseline).
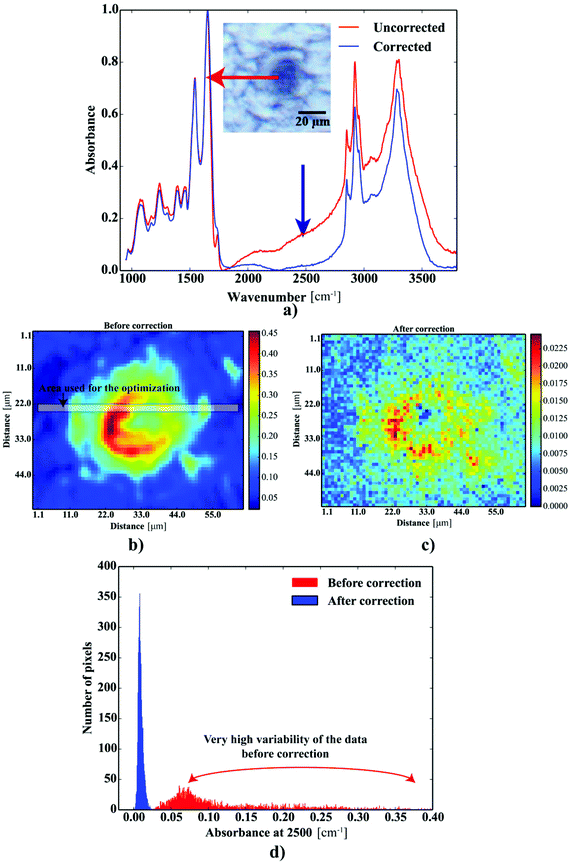 |
| Fig. 2 The results of the scattering correction: (a) FTIR spectra taken from the deposit before and after correction; the maps showing the distribution of the absorbance at 2500 cm−1: (b) before and (c) after correction; (d) the distribution of the heights at 2500 cm−1 before and after correction. | |
Curve-fitting
For this study, to identify the Aβ plaques, and to extract the subtle spectroscopic information on the protein secondary structure contents, we used a simplified curve-fitting approach of amide I with the mixed Gaussian–Lorentzian peak model, involving an iterative Levenburg–Marquardt algorithm to minimize the R2 function (see eqn (1)–(3)).30,31 | 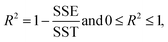 | (1) |
|  | (2) |
|  | (3) |
where: Ŷi – the i-th fitted value of the dependent value Yi.
Only four peaks attributed to major components were taken into account, as proposed in ref. 32. For the purpose of further computations, the spectra were additionally cut to 1600–1700 cm−1 range, baseline corrected (a simple linear baseline), cubically interpolated (to increase the number of data points in the spectra) and finally 0–1 normalized. The data were neither additionally noise reduced or smoothed. The positions of the most intense bands, determined according to the second order derivative spectra of interpolated amide I spectra, revealed they should be present around 1665, 1654, 1645 and 1636 cm−1 (cf.Fig. 3a), which is in good agreement with Miller et al.32 To increase the reliability of the computational procedures, we optimized the fitting model by analyzing the effect of selected parameters, including either the peaks’ half-widths (FWHM) or Lorentzian's function fraction (Lf), on the R2 functions upon analysis of 100 spectra selected from the area indicated in Fig. 2c. The effect of the FWHM parameter was assessed following analysis of the peaks fixed in their nominal positions. The positions were constrained within reasonably small range (±2 cm−1). The second experiment to assess the effect of Lorentzian's fraction was run with the positions and FWHM's to vary within their optimal range. Building upon these experiments, we propose the equally-mixed Lorentzian–Gaussian (50%
:
50%, cf. Fig. 5a–c) fitting model with the peaks’ half-widths to range between 14–16 cm−1 to produce the most accurate fits, since the results were found to minimize the R2 function of the devised model (cf.Fig. 3c and b, respectively). All curve fitting procedures including data preprocessing and curve fitting were implemented in Python 2.7 with scipy, numpy,29 matplotlib33 and lmfit libraries.34
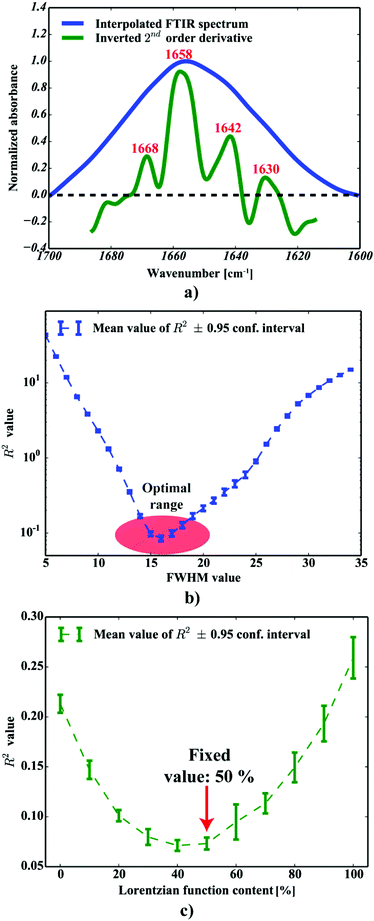 |
| Fig. 3 The optimization of the fitting procedure: (a) the second order derivative FTIR spectrum to determine the peaks’ positions; (b) the optimization of the FWHM for all the peaks included; (c) the optimization of the Lorentzian fraction. | |
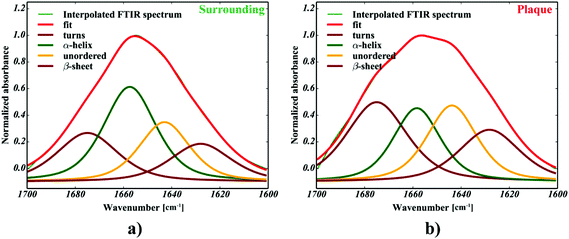 |
| Fig. 4 The curve fitting of a single: (a) neuropil point (b) plaque point. | |
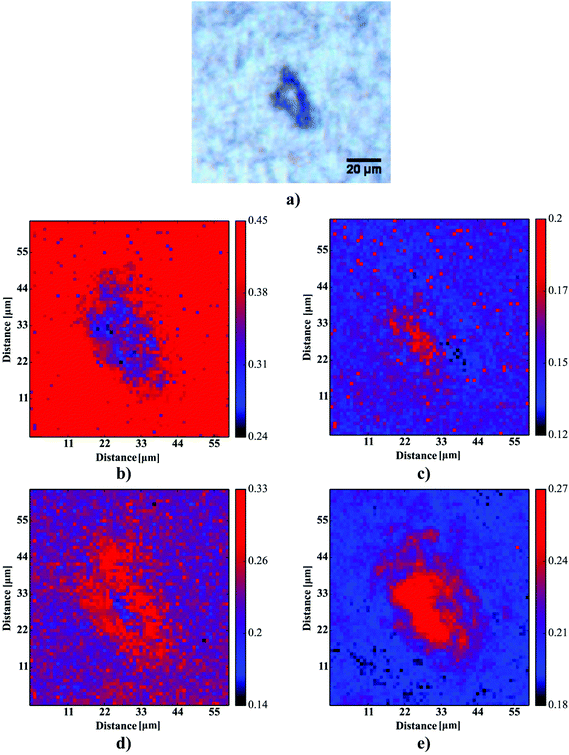 |
| Fig. 5 The results of the curve fitting for one selected plaque: (a) the microscopic picture of the plaque, (b) the fraction of alpha-helices, (c) the fraction of β-sheets, (d) the fraction of random coils, (e) the fraction of β-turns. | |
Image analysis
Based on 12 (∼3–4 plaques taken from each sample) images showing the spatial distribution of β-sheets, our computations aimed at the determination of four ∼4-μm-thick layers around the deposits to define their surrounding areas. For the sake of further computations, to define the coordinates of an Aβ deposit, the binarization with the binarization threshold of 0.16 was found to robustly mark the plaque-like areas. Additional median filtering step (4 pixel × 4 pixel mask) was included to remove any single-pixel binarization artifacts (see Fig. 6a and b). The binarized image was then subjected to the dilation transformation. The mask of 4 × 4 pixels (around 4.4 μm × 4.4 μm) resulted in the plaques’ area enlarged by ∼4 μm in each direction. Finally, to determine the first 4 μm-thick layer around the Aβ core, the dilated image was then subtracted from the binarized one. The whole procedure was repeated additional three times to determine all four areas of the same width around the deposits (cf.Fig. 6c). The scripts for image transformations were written in Python 2.7 with scipy, numpy,29 matplotlib33 and scikit-image35 libraries.
 |
| Fig. 6 The image processing procedure to determine the consecutive layers around Aβ plaques. | |
Principal component analysis
Principal Component Analysis (PCA), involving the fractions of protein secondary structure components computed as highlighted above, was used to compare the protein composition and variability of the spectra taken from the plaque (PLAQUE) and outer (Out) layers. First two PCA variables (PCA1 and PCA2), explaining 45% and 35% of the variance, were taken into account. The scripts for PCA have been written in Python 2.7 using the above-mentioned packages.
Molecular factors
To describe the lipid and protein related processes occurring within and in close proximity of the Aβ deposits, some additional molecular parameters were determined. One of these, the integration area of the νas(–PO42−) band in phospholipids and phosphodiesters was computed within the 1200–1260 cm−1 integration range (baseline 1200–1260 cm−1). In turn, the ν = (CH)/νas(CH3) parameter to describe the level of lipid unsaturation was computed as a ratio of the ν = (CH) oleofinic band area (integration between: 3000 cm−1–3020 cm−1; baseline 3000 cm−1–3020 cm−1) to the νas(CH3) band area (integration between: 2948 cm−1–2990 cm−1; baseline 2800 cm−1–3000 cm−1). So-called lipid to protein ratio was computed as the integration area of the C–H str (integration between: 2800–3000 cm−1; baseline: 2800–3000 cm−1) and the area of amide I (integration between: 1600–1700 cm−1; baseline: 1600–1700 cm−1). The level of lipid oxidation was expressed as a ratio of the C–H stretch area (integration between: 2800–3000 cm−1; baseline: 2800–3000 cm−1) and the C
O ester band centered at 1730 cm−1 (integration between: 1715–1740 cm−1; baseline: 1715–1740 cm−1).
Statistical data analysis
The spectra, protein secondary contents and additional molecular factors computed were divided onto six classes as follows: PLAQUE, 1st layer, 2nd layer, 3rd layer, 4rd layer and outer. The protein secondary structure contents were finally expressed as the mean ± mean standard error. The statistical data analysis was carried out using an in-house code written in Python using the scipy library with the assistance of the above-mentioned Python libraries.
Results and discussion
Previous work has demonstrated that FTIR spectroscopy is a very versatile molecular imaging method for studying different protein- and lipid-related effects underlying Aβ-related neurotoxicity.10 Currently, the significance of the interplay between these two molecular components is gradually emerging as alternative means to explain AD pathology at different clinical stages of the disease.36 Importantly, the increasingly growing spatial resolution of micro-imaging experiments by FTIR allows acquisition of the data at the sub-micron pixel size, which is a pre-requisite for studying pathological processes at the sub-cellular level.20,22,37 By benchtop FTIR imaging at 6.25 μm pixel size, Kong et al. probed large area stromal regions surrounding the melanoma skin tumour. The authors concluded noticeable pathological changes appear 50–100 μm away from the cancerous tissue, which was linked to the commonly known field effect observed when highly malignant tumours progress.38 A more recent study by Kumar et al. set out to gain more insight into breast cancer pathology at the cellular level with particular scrutiny placed upon the analysis of the extracellular matrix by synchrotron FTIR imaging at the pixel size of around 3 μm. With the aid of multivariate methods, collagen-induced modifications in the extracellular matrix were found, and proposed as possible markers of tumour's progression.39,40 Kwak et al., in turn, proposed the paradigm of FTIR chemical imaging at 6.25 μm pixel size for prognosis of prostate cancer recurrence. By analysing a large cohort of large area tissue microarrays (186 patients), the prediction method was found to outperform commonly used methods.41 In our study, as compared in Fig. 1e and f, we took advantage of high-magnification benchtop FTIR-FPA imaging at the pixel size of 1.1 μm justified by radically higher fidelity of sub-cellular histological details that could be resolved with a stand-alone laboratory instrument coupled with state-of-the-art IR optics. As compared with the above-mentioned studies, the goal of the present study was to unravel spectral changes in small tissue areas (up to 100 μm × 100 μm). Since the diameters of the plaques were found to range from 10–40 μm (i.e.Fig. 5a), the higher sub-cellular resolution was a prerequisite to providing with relevant chemical information. It was also to separate out inflammation-related changes which could be attributable to tiny engulfing microglial cells with around 1–2 μm in diameter.42 However, a careful assessment of the results may play a pivotal role as other factors, such as Mie scattering, reflection contributions, electric-field-standing wave artifacts, may obscure the biologically relevant spectroscopic information.43–45 This is due to radically different optical properties of mature Aβ plaques visible as dark spots under the microscope.18 The approximated formula to describe the scattering cross-section is presented below: | 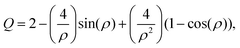 | (4) |
|  | (5) |
where: λ – wavelength [μm], n – the relative refractive index.
One can conclude that the presence of the objects with radically different optical (and physical) properties in tissue sections may result in the presence of multiple undulating baselines. To get rid of the artifacts arising from the Mie-like behavior observed in FTIR spectra, a number of approaches have been developed.46,47 The RMieS-EMSC algorithm, proposed by Bassan et al., has been shown to give acceptable performance and is commonly used in the field.11,26 Our results demonstrate the oscillating baseline is also present in the FTIR spectra taken from the Aβ deposits indicating the inherent problem of Mie-like distortions likely to affect the pure absorbance spectra (cf.Fig. 2a). In Fig. 2b, there is a 2D distribution of the scattering intensities measured at 2500 cm−1 (unlikely to be assigned to any vibration modes but arising from fluctuations in the baseline) for a selected plaque. From this data, we can see that the (dense) core of the plaque is of radically elevated absorbance. Once corrected, as in Fig. 2c, the intensities computed at 2500 cm−1 become 95% lower than the maximum absorbance in the spectra, so that one could assume their influence to the spectroscopic information (here proteomic-related) should be negligible. In addition, the histogram presented in Fig. 2d shows up the corrected data (blue boxes) are of significantly lower heterogeneity, and their baselines’ oscillations are of markedly reduced amplitude as compared with the uncorrected values (red boxes). These results come as no surprise since the previous studies have demonstrated that FTIR spectra taken from the cells were of very high variability in their chemical and physical properties (refractive index, density).26,44 RMieS depends on the scattering particle size and refractive index, therefore any variations either in samples’ morphology or density may distort pure absorbance FTIR spectra which is the case in our raw data taken from the Aβ plaques.43 This issue becomes important when the goal is to bring deeper insight into pure proteomic related information which is encoded within FTIR spectra. In particular, the amide I band of peptides has been shown to contain a myriad of subtle information on innumerable protein backbone C
O vibration modes.48 One should mention that other ranges within the fingerprint region are also commonly used in the proteomic research, but the intensity of underlying modes of vibrations of proteins’ backbones is markedly reduced, compared with those observed within amide I.49–51 To extract the proteomic related information from amide I many solutions have been proposed, be it different machine-learning-based approaches (including artificial neural networks), chemometric approaches (partial least squares) and curve fitting.49,52,53 The former, representing so-called supervised techniques, involve the training using the spectra of known secondary structure components (target values), so that the appropriate optimization may even mirror the results obtained with sophisticated crystallographic measurements.49 The latter represent the unsupervised solutions.49,52 Although they are commonly used, fast, fairly simple and hassle-free for implementation, the proteomic related information they provide is semi-quantitative. This is because the intensities of vibration modes underlying amide I arise from the changes in the dipole moment of the entire peptide during resonant absorption of infrared radiation.48 Usually, asymmetric modes produce the strongest changes in the dipole moment and result in the highest peaks, whilst the symmetric modes are significantly (even several orders of magnitude) weaker making the interaction of IR light with a sample a very complicated quantum-mechanical phenomenon.48,54 Therefore, one should realise that the fraction of a specific amide I band is at least proportional to the number of residues of a particular conformational state.54 Nevertheless, much work involving the curve-fitting approach took advantage of showing the importance of the proteomic related conclusions drawn from the semi-quantitative results.32,50,55–58 In this proof-of-principle paper, the preliminary results demonstrate the feasibility of the amide I curve-fitting for protein secondary structure imaging of the Aβ plaques. The spectroscopic data presented are important to the extent to demonstrate that variation in relative amide I peak areas assigned to alpha-helices, β-turns, random coils and β-sheets is enough to localize and correlate the results with other molecular components found in FTIR spectra of the Aβ deposits. We first optimized our model, fixed the parameters in their optimal range, and subsequently performed the analysis of the FTIR maps for all the plaques. Fig. 4a and b show the results of curve fitting for two spectra taken from the core of the plaque (Fig. 4a) and from its surrounding (3b). In the deposits, the peaks assigned to β-turns, β-sheets and random coils are elevated, while the band attributed to alpha-helices is reduced, compared with the surrounding (cf.3a and b). Interestingly, in the amide I spectrum taken from the deposit, the spectral signatures arising from both elevated β-sheets and random coils were present, which is in line with previous work.15,18 Net peak areas attributed to β-turns, alpha-helices, random coils and β-sheets were finally expressed as the relative fraction of amide I (cf.Fig. 5a–e). One selected deposit was graphically presented in Fig. 5a–e. What is interesting in this data is that alpha-helices are dramatically reduced within the deposit. On the contrary, β-sheets, turns and random coils appear to be somewhat increased, while the random coils seem to surround the region of increased fraction of β-sheets in this case. By using those images, the main challenge behind our work was to extract the information on protein-related chemical effects within and in close proximity of the plaques. We found image-processing-assisted methods (see the algorithm in Fig. 6) to utilize the images showing the spatial variation in β-sheets very convenient. As demonstrated in Fig. 4a and 6, the β-sheets’ fraction of 0.16 discriminates between the areas of increased β-sheets and surrounding regions. The image-processing procedure to determine the immediate areas of the deposits was highlighted in Fig. 6. Given the six adjacent layers (PLAQUE, 1st, 2nd, 3rd, 4th and out), we calculated the average fraction of each protein secondary structure component vs. its position (the layer it falls into). The results (cf.Fig. 7a and b) indicate the plaques are co-localized to increased β-sheets, turns, random coils, and reduced fraction of alpha-helices. In turn, the surrounding regions are enriched in alpha-helices. The transition between alpha-helices and β-sheets is indicative of aggregated proteins and was recently proposed using amide I band fitting of pure Aβ spectra.59,60 This data is also in accord with other work showing FTIR spectra of dense core-plaques exhibiting a characteristic shoulder around 1630 cm−1 suggesting highly aggregated amyloid enriched in β-sheets.13,18,32 Some recent studies using ATR-FTIR have also corroborated that Aβ fragments possess increased amounts of anti-parallel β-sheets.61 Using the amide I curve fitting results, the PCA analysis demonstrated a good separation between the deposits and surrounding ‘Out’ regions along PCA1 (cf.Fig. 8a and b). The points attributed to the Aβ plaques are in the negative PC1 space, whilst the surrounding ones are in the positive space. Fig. 8b shows the corresponding scores plot of the data. One can surmise that the cause for this clustering is mainly due to β-sheets and β-turns. Their loadings are both of the highest amplitude and negative, which supports the idea that these components are co-localized in the Aβ plaques. One possible explanation for this might be that fibrillar Aβ displays additional shoulder at around 1690 cm−1, which could contribute to the β-turns peak.62 The presence of this shoulder can therefore support the idea that fibrillar Aβ deposits to build up senile plaques adapts antiparallel β-sheets motif. However, as demonstrated both in the experimental and theoretical studies, the intensity of this high-wavenumber peak is up to five times weaker than the major one centered
at around 1630 cm−1.63 Another possible scenario is that the phospholipid system, present in high concentrations in the neuropil (but not present in pure in vitro Aβ samples), could modify the Aβ aggregation. Indeed, some recent studies involving the POPC/POPS (1-palmitoyl-2-oleoylphospatidylcholine/palmitoyl-2-oleoylphospatidylserine) system, using Raman spectroscopy, SR-FTIR, and micro XRD confirmed the aggregation of Aβ(25–35) results in detectable amounts of both antiparallel and parallel β-sheets motifs together with some possible β-turns.64 In another study, Gough et al. reported some major obstacles with obtaining pure Aβ spectra in the dense core plaques found in the pristine brain tissue sections drawn from both humans and animal models of AD.54 The authors mentioned that there is some overlap between adjacent FPA pixels blending the spectra taken from the Aβ deposits with the ones from the surrounding neuropil areas.54 Finally, to tackle the problem of protein-related biochemical processes occurring within and in close proximity of the deposits, we verified if there were any statistically significant (p < 0.05) correlations between β/α ratios and additional molecular parameters computed for each consecutive layer. We found decreased lipid/protein ratio coincides with the elevated β/α ratio (cf.Fig. 9b), though the correlation coefficient was not statistically significant (p = 0.13) and the relation between those two parameters seemed to be non-linear. It is probably due to increased amount of protein-like material within the plaques. The single most interesting result to emerge from this data is that we observed a slight increase in the lipid/protein parameter in the first layer (around 4.4 μm apart). This result, though very subtle, should draw our attention to increased accumulation of lipids around Aβ deposits reported elsewhere.18,22 Previous studies appreciated the significance of glial infiltration which may enhance the accumulation of lipid-like material around the deposits. This result is likely to be related to lipid-rich membranes of microglia and astrocytes.7 The second remark that emerges from our data is related to the previous one. It turns out, the average values of β/α are positively correlated with the area of the νas(–PO42−) band (Fig. 9a). It can lead to the conjecture that the gradual accumulation of β-sheets is associated with up-regulated abundance of phosphodiesters and/or lipid esters in the core of a plaque. This result, lends support to the recent study that demonstrated some protein-bound oxidation products of sugar oxidation (advanced glycation end products – AGEs) are secreted by glia surrounding Aβ plaques.65 In addition, Fig. 9c highlights the lipids are significantly less unsaturated in the plaques as indicated by the decrease in the ν(
CH)/νas(CH3) ratio. This observation fits in with the recent results by Benseny-Cases et al., showing the areas of human Aβ deposits exhibit reduced level of lipid unsaturation (the ν(
CH)/νas(CH3) ratio).19 This interesting correlation points to processes associated with lipid peroxidation induced within Aβ-deposits. As mentioned, the redox-active metals, Cu and Fe, are extensively accumulated within the deposits due to their improper chelation.13 Fibrillar Aβ has a very strong positive reduction potential and is capable of simultaneous reduction of Cu2+ and Fe3+ to Cu+ and Fe2+ and subsequent generation of hyper-reactive free oxygen species (ROS) via Fenton or a Haber–Weiss reaction, recognized as major driving forces in the neuropathological processes to tip the scales towards gradual development of the disease.14 Recent evidence suggests that unsaturated lipids are particularly prone to free radicals-induced peroxidation because of their unsaturated chemical bonds in their long fatty acid β-chain.66 As shown in Fig. 9d, the correlation coefficient between β/α and the level of lipid oxidation was found to be negative and highly significant (p < 0.05). This is in good agreement with the latest work by Benseny-Cases et al. using SR-FTIR imaging, which demonstrated reduced level of lipid oxidation within human Aβ plaques raster-scanned at 6 μm pixel size in the brain tissue section taken from the aged human (non-AD tissue section).19 Notably, in our study we utilized an early-stage animal model, which might have not fully mirrored the changes occurring in advanced AD. Nevertheless, it can support the idea that early-stage AD may alter oxidation level of lipids within the deposits. The relationship between normal aging and expression of Aβ plaques has not yet been fully explained and currently remains the matter of debate.67 It is quite challenging to find one robust explanation on our remark, but it seems possible that aggregated Aβ may promote gliosis and subsequent over-production of different oxidation products with reduced abundance of C
O ester bands at the expense of lipid membranes therefore inducing oxidative stress in neurons.68 In this study, the level of lipid oxidation was found to be around five times lower in the plaques as compared with the surrounding (cf.Fig. 9d). This remark draws our attention to the fact that Aβ-driven gliosis at expense oxidation products with reduced abundance of C
O ester bands was recently proposed by Swomley et al. as one of the major mechanisms underlying progression of AD.68 Interestingly, by analysing the levels of 4-hydroxy-nonenal, a major lipid peroxidation product identified in AD brains, McGrath et al. found its plasma displays around 4–5 times lower levels in the control group, suggesting the effect is about one order of magnitude stronger in the affected brains.69
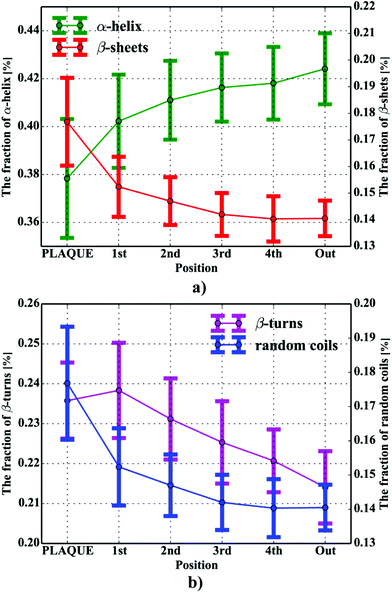 |
| Fig. 7 The percentage contents of protein secondary components within and in close proximity of the plaques for: (a) β-sheets and α-helices; (b) β-turns and random coils. | |
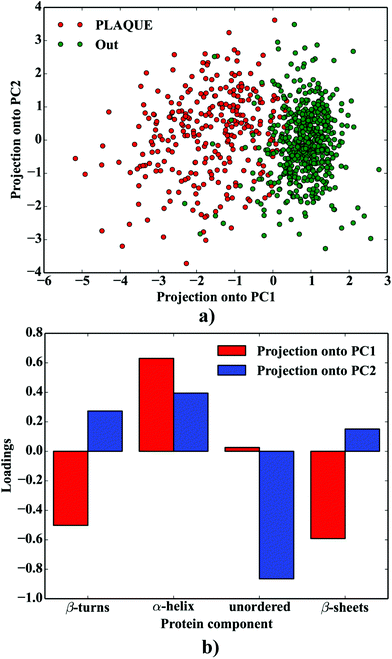 |
| Fig. 8 PCA analysis of the protein composition of AB plaques: (a) the projection of the data onto PCA1 vs. PC2; (b) the corresponding loadings plot. | |
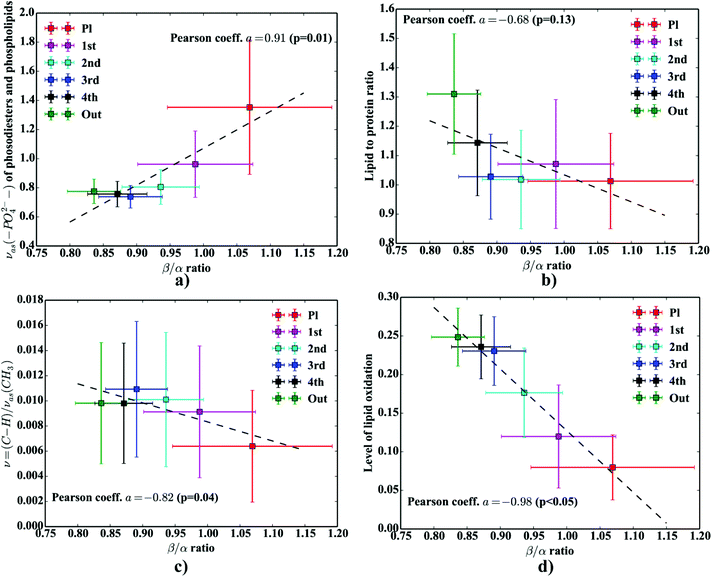 |
| Fig. 9 The relation between β/α ratios and: (a) the area of the νas(–PO42−) band; (b) lipid to protein ratio; (c) level of lipid unsaturation; (d) level of lipid oxidation. | |
Conclusions
Our proof-of-principle study has demonstrated the usefulness of the presented approach by showing that proteomic related information could be drawn from FTIR-FPA imaging of Aβ plaques at an early-stage of the pathology. The combined approach was found very robust in research towards correlative studies on the interplay between the distance from the deposits and the extent to the molecular burden. Our results, although preliminary, demonstrate the areas affected by plaques display different protein and lipid-processes associated with oxidative stress pathology. Also, our data emphasized the areas adjacent to the deposits may play a critical role in the spread of the pathology.
Acknowledgements
The authors would like to kindly acknowledge J. Brooks, Z. Zhang, J. Doherty and A. Keen for technical assistance during FTIR-FPA measurements, and I. Berrueta Razo and E. Correia Faria-Billinton for access to preliminary data. This work was partly supported by the Polish Ministry of Science and Higher Education and its grants for Scientific Research, and partly by the EU ERASMUS+ funding. The FTIR instrumentation used was partly financed by the Williamson Trust.
Notes and references
- J. Götz and L. M. Ittner, Nat. Rev. Neurosci., 2008, 9, 532–544 CrossRef PubMed.
- C. Reitz and R. Mayeux, Biochem. Pharmacol., 2014, 88, 640–651 CrossRef CAS PubMed.
- H. Braak and E. Braak, Acta Neuropathol., 1991, 82, 239–259 CrossRef CAS PubMed.
- J. Brettschneider, K. Del Tredici, V. M.-Y. Y. Lee and J. Q. Trojanowski, Nat. Rev. Neurosci., 2015, 16, 109–120 CrossRef CAS PubMed.
- I. Kuperstein, K. Broersen, I. Benilova, J. Rozenski, W. Jonckheere, M. Debulpaep, A. Vandersteen, I. Segers-Nolten, K. Van Der Werf, V. Subramaniam, D. Braeken, G. Callewaert, C. Bartic, R. D'Hooge, I. C. Martins, F. Rousseau, J. Schymkowitz and B. De Strooper, EMBO J., 2010, 29, 3408–3420 CrossRef CAS PubMed.
- W. Kim and M. H. Hecht, J. Biol. Chem., 2005, 280, 35069–35076 CrossRef CAS PubMed.
- M. T. Heneka, M. J. Carson, J. El Khoury, G. E. Landreth, F. Brosseron, D. L. Feinstein, A. H. Jacobs, T. Wyss-Coray, J. Vitorica, R. M. Ransohoff, K. Herrup, S. A. Frautschy, B. Finsen, G. C. Brown, A. Verkhratsky, K. Yamanaka, J. Koistinaho, E. Latz, A. Halle, G. C. Petzold, T. Town, D. Morgan, M. L. Shinohara, V. H. Perry, C. Holmes, N. G. Bazan, D. J. Brooks, S. Hunot, B. Joseph, N. Deigendesch, O. Garaschuk, E. Boddeke, C. A. Dinarello, J. C. Breitner, G. M. Cole, D. T. Golenbock and M. P. Kummer, Lancet Neurol., 2015, 14, 388–405 CrossRef CAS PubMed.
- D. Allan Butterfield, A. Castegna, C. M. Lauderback and J. Drake, Neurobiol. Aging, 2002, 23, 655–664 CrossRef CAS.
- M. Arimon, S. Takeda, K. L. Post, S. Svirsky, B. T. Hyman and O. Berezovska, Neurobiol. Dis., 2015, 84, 109–119 CrossRef CAS PubMed.
- S. Caine, P. Heraud, M. J. Tobin, D. McNaughton and C. C. A. Bernard, NeuroImage, 2012, 59, 3624–3640 CrossRef PubMed.
- M. J. Baker, J. Trevisan, P. Bassan, R. Bhargava, H. J. Butler, K. M. Dorling, P. R. Fielden, S. W. Fogarty, N. J. Fullwood, K. A. Heys, C. Hughes, P. Lasch, P. L. Martin-Hirsch, B. Obinaju, G. D. Sockalingum, J. Sulé-Suso, R. J. Strong, M. J. Walsh, B. R. Wood, P. Gardner and F. L. Martin, Nat. Protoc., 2014, 9, 1771–1791 CrossRef CAS PubMed.
- L. P. Choo, M. Jackson, W. C. Halliday and H. H. Mantsch, Biochim. Biophys. Acta, 1993, 1182, 333–337 CrossRef CAS.
- L. M. Miller, Q. Wang, T. P. Telivala, R. J. Smith, A. Lanzirotti and J. Miklossy, J. Struct. Biol., 2006, 155, 30–37 CrossRef CAS PubMed.
- D. G. Smith, R. Cappai and K. J. Barnham, Biochim. Biophys. Acta, Biomembr., 2007, 1768, 1976–1990 CrossRef CAS PubMed.
- M. Rak, M. R. Del Bigio, S. Mai, D. Westaway and K. M. Gough, Biopolymers, 2007, 87, 207–217 CrossRef CAS PubMed.
- R. Bhargava, Appl. Spectrosc., 2012, 66, 1091–1120 CrossRef CAS PubMed.
- M. Z. Kastyak-Ibrahim, M. J. Nasse, M. Rak, C. Hirschmugl, M. R. Del Bigio, B. C. Albensi and K. M. Gough, NeuroImage, 2012, 60, 376–383 CrossRef CAS PubMed.
- C. R. Liao, M. Rak, J. Lund, M. Unger, E. Platt, B. C. Albensi, C. J. Hirschmugl and K. M. Gough, Analyst, 2013, 138, 3991–3997 RSC.
- N. Benseny-Cases, O. Klementieva, M. Cotte, I. Ferrer and J. Cladera, Anal. Chem., 2014, 86, 12047–12054 CrossRef CAS PubMed.
- M. J. Nasse, M. J. Walsh, E. C. Mattson, R. Reininger, A. Kajdacsy-Balla, V. Macias, R. Bhargava and C. J. Hirschmugl, Nat. Methods, 2011, 8, 413–416 CrossRef CAS PubMed.
- R. K. Reddy, M. J. Walsh, M. V. Schulmerich, P. S. Carney and R. Bhargava, Appl. Spectrosc., 2013, 67, 93–105 CrossRef PubMed.
- C. R. Findlay, R. Wiens, M. Rak, J. Sedlmair, C. J. Hirschmugl, J. Morrison, C. J. Mundy, M. Kansiz and K. M. Gough, Analyst, 2015, 140, 2493–2503 RSC.
- S. Oddo, A. Caccamo, J. D. Shepherd, M. P. Murphy, T. E. Golde, R. Kayed, R. Metherate, M. P. Mattson, Y. Akbari and F. M. LaFerla, Neuron, 2003, 39, 409–421 CrossRef CAS PubMed.
- T. van Dijk, D. Mayerich, R. Bhargava and P. S. Carney, Opt. Express, 2013, 21, 12822 CrossRef PubMed.
- P. Bassan, A. Kohler, H. Martens, J. Lee, H. J. Byrne, P. Dumas, E. Gazi, M. Brown, N. Clarke and P. Gardner, Analyst, 2010, 135, 268–277 RSC.
- P. Bassan, A. Kohler, H. Martens, J. Lee, E. Jackson, N. Lockyer, P. Dumas, M. Brown, N. Clarke and P. Gardner, J. Biophotonics, 2010, 3, 609–620 CrossRef CAS PubMed.
- P. Bassan, A. Sachdeva, A. Kohler, C. Hughes, A. Henderson, J. Boyle, J. H. Shanks, M. Brown, N. W. Clarke and P. Gardner, Analyst, 2012, 137, 1370–1377 RSC.
- D. Thain, T. Tannenbaum and M. Livny, Concur. Comput. Pract. Exp., 2005, 17, 323–356 CrossRef.
- S. Van Der Walt, S. C. Colbert and G. Varoquaux, Comput. Sci. Eng., 2011, 13, 22–30 CrossRef.
- D. W. Marquardt, J. Soc. Ind. Appl. Math., 1963, 11, 431–441 CrossRef.
- K. Levenberg, Q. Appl. Math., 1944, 2, 196–168 Search PubMed.
- L. M. Miller and P. Dumas, Curr. Opin. Struct. Biol., 2010, 20, 649–656 CrossRef CAS PubMed.
- J. D. Hunter, Comput. Sci. Eng., 2007, 9, 99–104 CrossRef.
-
M. Newville, T. Stensitzki, D. B. Allen and A. Ingargiola, Zendo, 2014 Search PubMed.
- S. van der Walt, J. L. Schönberger, J. Nunez-Iglesias, F. Boulogne, J. D. Warner, N. Yager, E. Gouillart and T. Yu, PeerJ, 2014, 2, e453 Search PubMed.
-
A. Naudi, R. Cabre, M. Jove, V. Ayala, H. Gonzalo, M. Portero-Otin, I. Ferrer and R. Pamplona, in International Review of Neurobiology, 2015, vol. 122, pp. 133–189 Search PubMed.
- C. Hughes, A. Henderson, M. Kansiz, K. M. Dorling, M. Jimenez-Hernandez, M. D. Brown, N. W. Clarke and P. Gardner, Analyst, 2015, 140, 2080–2085 RSC.
- R. Kong, R. K. Reddy and R. Bhargava, Analyst, 2010, 135, 1569 RSC.
- S. Kumar, T. S. Shabi and E. Goormaghtigh, PLoS One, 2014, 9, e111137 Search PubMed.
- S. Kumar, C. Desmedt, D. Larsimont, C. Sotiriou and E. Goormaghtigh, Analyst, 2013, 138, 4058 RSC.
- J. T. Kwak, A. Kajdacsy-Balla, V. Macias, M. Walsh, S. Sinha and R. Bhargava, Sci. Rep., 2015, 5, 8758 CrossRef CAS PubMed.
- S. G. Torres-Platas, S. Comeau, A. Rachalski, G. Bo, C. Cruceanu, G. Turecki, B. Giros and N. Mechawar, J. Neuroinflammation, 2014, 11, 12 CrossRef PubMed.
- H. Brooke, B. V. Bronk, J. N. McCutcheon, S. L. Morgan and M. L. Myrick, Appl. Spectrosc., 2009, 63, 1293–1302 CrossRef CAS PubMed.
- P. Bassan, H. J. Byrne, J. Lee, F. Bonnier, C. Clarke, P. Dumas, E. Gazi, M. D. Brown, N. W. Clarke and P. Gardner, Analyst, 2009, 134, 1171–1175 RSC.
- M. J. Pilling, P. Bassan and P. Gardner, Analyst, 2015, 140, 2383–2392 RSC.
- B. Mohlenhoff, M. Romeo, M. Diem and B. R. Wood, Biophys. J., 2005, 88, 3635–3640 CrossRef CAS PubMed.
- R. Lukacs, R. Blümel, B. Zimmerman, M. Bağcıoğlu and A. Kohler, Analyst, 2015, 140, 3273–3284 RSC.
- A. Barth and C. Zscherp, Q. Rev. Biophys., 2002, 35, 369–430 CrossRef CAS PubMed.
- A. D. Surowka, D. Adamek and M. Szczerbowska-Boruchowska, Analyst, 2015, 140, 2428–2438 RSC.
- E. Goormaghtigh, J.-M. Ruysschaert and V. Raussens, Biophys. J., 2006, 90, 2946–2957 CrossRef CAS PubMed.
- S. Navea, R. Tauler and A. de Juan, Anal. Biochem., 2005, 336, 231–242 CrossRef CAS PubMed.
-
J. A. Hering, P. R. Innocent and P. I. Haris, in Proteomics, 2004, vol. 4, pp. 2310–2319 Search PubMed.
- M. Severcan, P. I. Haris and F. Severcan, Anal. Biochem., 2004, 332, 238–244 CrossRef CAS PubMed.
- K. M. Gough, L. Tzadu, M. Z. Kastyak, A. C. Kuzyk and R. L. Julian, Vib. Spectrosc., 2010, 53, 71–76 CrossRef CAS.
- K. Wehbe, R. Pineau, S. Eimer, A. Vital, H. Loiseau and G. Déléris, Analyst, 2010, 135, 3052–3059 RSC.
- A. Saeed, G. A. Raouf, S. S. Nafee, S. A. Shaheen and Y. Al-Hadeethi, PLoS One, 2015, 10, e0139854 CAS.
- M. Baldassarre, C. Li, N. Eremina, E. Goormaghtigh and A. Barth, Molecules, 2015, 20, 12599–12622 CrossRef CAS PubMed.
- C. Petibois and G. Deleris, Trends Biotechnol., 2006, 24, 455–462 CrossRef CAS PubMed.
- L. M. Miller, M. W. Bourassa and R. J. Smith, Biochim. Biophys. Acta, 2013, 1828, 2339–2346 CrossRef CAS PubMed.
- R. I. Litvinov, D. A. Faizullin, Y. F. Zuev and J. W. Weisel, Biophys. J., 2012, 103, 1020–1027 CrossRef CAS PubMed.
- R. Sarroukh, E. Goormaghtigh, J. M. Ruysschaert and V. Raussens, Biochim. Biophys. Acta, Biomembr., 2013, 1828, 2328–2338 CrossRef CAS PubMed.
- E. Cerf, R. Sarroukh, S. Tamamizu-Kato, L. Breydo, S. Derclaye, Y. F. Dufrêne, V. Narayanaswami, E. Goormaghtigh, J.-M. Ruysschaert and V. Raussens, Biochem. J., 2009, 421, 415–423 CrossRef CAS PubMed.
- Y. N. Chirgadze and N. A. Nevskaya, Biopolymers, 1976, 15, 627–636 CrossRef CAS PubMed.
- A. Accardo, V. Shalabaeva, M. Cotte, M. Burghammer, R. Krahne, C. Riekel and S. Dante, Langmuir, 2014, 30, 3191–3198 CrossRef CAS PubMed.
- A. Kuhla, S. C. Ludwig, B. Kuhla, G. Münch and B. Vollmar, Neurobiol. Aging, 2015, 36, 753–761 CrossRef CAS PubMed.
- H. Esterbauer and P. Ramos, Rev. Physiol., Biochem. Pharmacol., 1996, 127, 31–64 CAS.
- I. Ferrer, Prog. Neurobiol., 2012, 97, 38–51 CrossRef PubMed.
- A. M. Swomley and D. A. Butterfield, Arch. Toxicol., 2015, 89, 1669–1680 CrossRef CAS PubMed.
- L. T. McGrath, Q. J. Med., 2001, 94, 485–490 CrossRef CAS.
|
This journal is © The Royal Society of Chemistry 2017 |
Click here to see how this site uses Cookies. View our privacy policy here.