DOI:
10.1039/C6RA25007F
(Communication)
RSC Adv., 2017,
7, 2587-2593
High-throughput metabolomics enables biomarker discovery in prostate cancer†
Received
10th October 2016
, Accepted 20th November 2016
First published on 12th January 2017
Abstract
Prostate cancer (PCa) is the most frequently diagnosed cancer and the second leading cause of cancer death among men in the world. Therefore, it is important to discover new biomarkers for the diagnosis of PCa, preferably noninvasive ones. Metabolomics can be used to analyse the entire metabolic profile of a biological system and discover potential biomarkers. Advances in liquid chromatography-tandem mass spectrometry technology have led to the application of metabolomics in PCa, toward identifying metabolic alterations in PCa. In this work, the art metabolomics platform by fast ultrahigh performance liquid chromatography-tandem mass spectrometry (FPLC/MS) coupled with multivariate statistical analyses were employed to identify sensitive and economical peripheral urine biomarker(s) associated with the entire measurable metabolome from PCa patients (n = 236) and age-matched healthy controls (n = 233), with the aim of discovering alterations in the metabolic phenotype and thus discovering potential biomarkers for the diagnosis of PCa. Metabolic differences among PCa and control subjects were identified based on orthogonal signal correction-partial least squares discriminant analysis (OPLS-DA). Glycocholic acid, hippurate, 5-hydroxy-L-tryptophan, taurocholic acid, and chenodeoxycholic acid in the PCa subjects were significantly different from the control cases. Bioinformatics analysis found that these differentially-expressed metabolites had a strong correlation with the farnesoid X receptor and retinoic X receptor (FXR/RXR) activation, etc. To demonstrate the utility of urine biomarkers for the early diagnosis of PCa, three metabolites comprising glycocholic acid, hippurate and 5-hydroxy-L-tryptophan were selected as candidate biomarkers (AUC > 0.95) and validation in separate, independent patient cohorts, yielded satisfactory accuracy, sensitivity and specificity. Furthermore, these data suggest that panels of analytes may be valuable to translate metabolomic findings to clinically useful diagnostic tests. Potentially, the present study provides promising diagnosis tool for PCa.
Introduction
Prostate cancer (PCa) is the second most diagnosed cancer in men, principally affecting men over 50 years old, and is the fifth leading cause of cancer-related deaths in men worldwide.1–3 The incidence is increasing in many traditionally low risk countries in Asia. The quantification of the digital rectal examination and prostate serum antigen (PSA) are common techniques used for PCa diagnosis.4–7 However, the value of PSA screening is controversial because of its limited sensitivity and specificity. The lack of a consistent biomarker for PCa diagnosis highlights the need for novel, specific, and cost-effective biomarkers to differentiate patients in the state of the disease. There is an urgent need to develop the new tests that provide risk assessment for PCa, and once diagnosed, could further identify those men with this disease.
Discovery of novel biomarkers is critical for diagnosis of PCa. In an effort to discover viable biomarkers, the researchers have employed metabolomics approaches to aid in the discovery of the biomarkers in biological samples.8 Metabolomics is the systemic study of all metabolites and their concentration as affected by pathological and physiological factors. And therefore, alterations in the “normal” metabolome may be indicative of disease. These metabolites are the small molecule intermediates and end products of the biochemical reactions in a cell, and are represented by compounds with mass typically in the range of 50–1000 Da.9 Current metabolomic approach can be used to screen broadly for metabolic changes in urine.10 Urine as diagnose medium offers an inexpensive, easy and noninvasive approach, has been considered potential tool to monitoring disease status.11 To improve diagnostic specificity, analysis of biomarkers from urine samples can be very useful. Recently, researcher has identified a wide diversity of urine metabolites that can provide information for identifying potential biomarkers.12,13
Recent advancements in analytical tools have enabled mass spectrometry-based metabolomics and chromatographic techniques have facilitated high throughput assays for a broad spectrum of metabolites.14 A faster ultrahigh performance liquid chromatography-mass spectrometry (FPLC-MS) metabonomics analysis technique has been used as to diagnose oral cancer, diabetes, colorectal cancer, hepatocellular carcinoma, and chronic renal failure.15,16 An integrated FPLC-MS method based urine metabonomics analysis of PCa has not been reported hitherto as far as we know. To explore urine biomarkers of PCa, we applied FPLC-MS metabonomics in positive ion mode was examined to investigate urine samples from 236 PCa patients and 233 healthy volunteers. Multivariate data analysis was performed to highlight discriminated variables. The purpose of this study is to develop a urine metabolomics analysis for identifying potential biomarkers to early diagnosis of PCa. This study provides a high-throughput platform for improving the diagnosis and monitoring disease progression, and, hopefully, many other disease scenarios.
Experimental
Chemicals
Methanol (HPLC grade) and acetonitrile (HPLC grade) were purchased from Fisher (USA). Distilled water (18.2 MΩ) was purified using a Milli-Q system (Millipore, Billerica, USA). Leucine enkephalin was purchased from Sigma-Aldrich (St. Louis, MO, USA). Formic acid (HPLC grade) was purchased from J&K Chemical Ltd (Beijing, China).
Ethics statement
The study was approved by the Review Board of Heilongjiang University of Chinese Medicine (HUCM-2014-74931) and complied with the provisions of the Good Clinical Practice Guidelines and the Declaration of Helsinki. Patients had given their written consent before donation of urine samples.
Patient selection
All patients and the control individuals were recruited from the First Affiliated Hospital, Heilongjiang University of Chinese Medicine. Exclusion criteria are that patients (nonsmoker) had cancer, cardiac insufficiency, hepatosis, renal inadequacy, respiratory failure, alimentary tract hemorrhage, or other diseases that will affect the clinical observations and biological indicators. A total of 236 patients together with 233 normal control cases were recruited. Criteria for selection of controls were no history of PCa, denial of clinical manifestations of PCa, and a low level of urine PSA. Clinical characteristics of the subjects used in this study are provided in ESI Table 1.† Before collection of urine samples, patients had given consent and had not undergone therapeutic interventions.
Urine sample collection
Samples were the first morning urine and were processed within 2 h of collection, as follows. The subjects were given insulated ice packs in which they were asked to store the urine samples immediately until they were received by the study investigator. Urine was centrifuged at 10
000 rpm for 10 minutes at 4 °C, and the supernatant was transferred to a 1.5 mL polypropylene tube, and then filtered through a syringe filter (0.22 μm), 5 μL of the supernatant were injected into the FPLC/MS.
FPLC chromatography
Faster ultrahigh performance liquid chromatography-mass spectrometry (FPLC) analysis was performed using an Waters series FPLC system equipped with a degasser, binary pump and column oven (Waters, Milford, USA). Samples were performed on UPLC™ BEH C18 column (1.7 μm, 50 mm × 2.1 mm i.d., Waters, Milford, USA). The column was maintained at 45 °C. The flow rate throughout the separation was maintained at 0.5 mL min−1. The mobile phase used for the separation consisted of acetonitrile/water (50
:
50 v/v) containing 0.1% formic acid (solvent A) and acetonitrile/water (95
:
5 v/v) containing 0.1% formic acid (solvent B). Gradient elution was performed with the following solvent system: (A) 0.1% formic acid–water, (B) acetonitrile (ACN). The gradient conditions were 0–2 min 1–15% B; 2–6 min 15–50% B; 6–9 min 50–95% B; 9–11 min 95% B followed by re-equilibration to the initial starting condition. The samples were kept at 4 °C, and a constant volume of 4 μL was injected for analysis.
Mass spectrometry
Mass spectrometry analysis was performed on an faster time of flight mass spectrometer (Waters, Milford, USA). The mass spectrometer was operated in both electrospray positive ionization modes. The mass scanning range was 100–1500 m/z in the full scan mode, with a capillary voltage of 3000 V, the source temperature of 120 °C, and a nebulizer gas flow rate of 35 L min−1, a collision gas flow rate of 10 L min−1, the capillary temperature of 300 °C and desolvation gas temperature of 400 °C. The flow rates of cone and desolvation gas were set at 60 L h−1 and 400 L h−1, respectively. Nitrogen was used as the collision gas at a collision cell pressure of 2.0 × 10−5 torr.
Data processing and metabolite identification
FPLC-MS data were analyzed to identify potential discriminant biomarkers. The peak finding, filtering, and alignment were performed by MarkerLynx 4.1 application manager (Waters, Manchester, UK). The MS matrix was then introduced to EZinfo 2.0 software for orthogonal signal correction-partial least squares discriminant analysis (OPLS-DA) which produces a score plot showing the separation of the groups based on the content of the loading discriminatory metabolites. VIP-plot from OPLS-DA was used to measure the importance of each metabolite to the classification. MassFragment™ manager (Waters corp., Milford, USA) was used to facilitate the MS/MS fragment ion analysis process by way of chemically intelligent peak-matching algorithms. Compound annotation was performed by the HMDB (http://www.hmdb.ca) Database. Then, we performed using the IPA system (http://www.ingenuity.com/) to exploring the typical metabolic perturbations associated with the related metabolites and molecular interaction networks were generated based on the IPA knowledge.
Strategies used in selection of metabolite biomarkers
The selection strategy provided additional differentiation of PCa and control samples, in order to demonstrate that the selected candidates are not only clinically useful and applicable, but also they are highly sensitive, specific and accurate in differentiation of PCa from controls. After analysis with Programs Used in Statistical methods, any potential candidates will be selected as individual urine metabolite biomarker in diagnosis of PCa, if it met these criteria: (1) sensitivity above 80%; (2) specificity above 50%; (3) all of precision and area under (ROC) curve above 80%.
Statistical analysis
The areas under curve (AUC) of receiver operating characteristic (ROC) curves were performed to determine the diagnostic effectiveness of important metabolites using GraphPad Prism Version 5.00 (GraphPad Software, San Diego, California, USA). The t test analysis of covariance were performed using SPSS software (version 19.0; SPSS, Inc., Chicago, IL), with p < 0.05 deemed to be significant.
Results
Subject characteristics
Demographic and phenotypic characteristics of the subjects are described in ESI Table 1.† The mean ages, sex, body mass index, 2 hour postprandial glucose, total cholesterol, triglycerides, systolic blood pressure, and diastolic blood pressure were not significantly different between patients with PCa cases and age-matched healthy controls. The PSA was 9.8 ± 5.6 for PCa patients, indicating a fairly advanced stage of this disease.
Typical chromatograms
In this study, the urine samples were analyzed by FPLC-MS tool. The separation conditions of urine samples were optimized, and the typical base peak intensity (BPI) chromatograms of urine samples from the controls and the PCa cases in positive ion mode were shown in Fig. 1. The utilization of pattern recognition could enlarge metabolite identification.
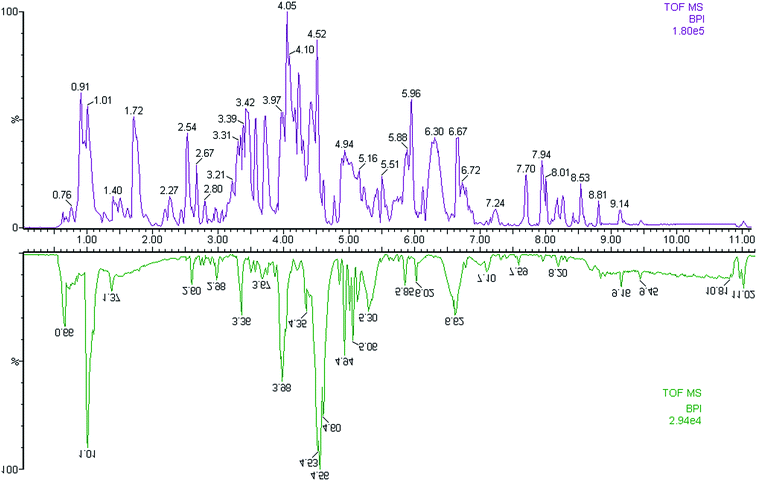 |
| Fig. 1 Typical base peak chromatograms of control subjects (up) and PCa patients (down) by FPLC-MS. | |
Metabolic phenotype of PCa patients
LC/MS-based metabolomics was carried out to analyze the PCa patient and control subjects. Using the LC/MS analysis processes, we found 3581 retention time-exact mass pairs remaining in the sample profile. A supervised, multivariate technique, OPLS-DA, was also used to analyze the data. In the OPLS-DA scores plot (R2X = 0.87, R2Y = 0.91, Q2 = 0.84), the PCa group are clearly separated from the age-matched healthy controls, which clustered together (Fig. 2). There were significant differences between the first component (P[1]) scores of controls and PCa groups, which demonstrated that the OPLS-DA model was valid for positive ion mode. The satisfactory clustering trends are observed in this scores plot, indicating that possibility of urine metabolomics for discriminating PCa cases.
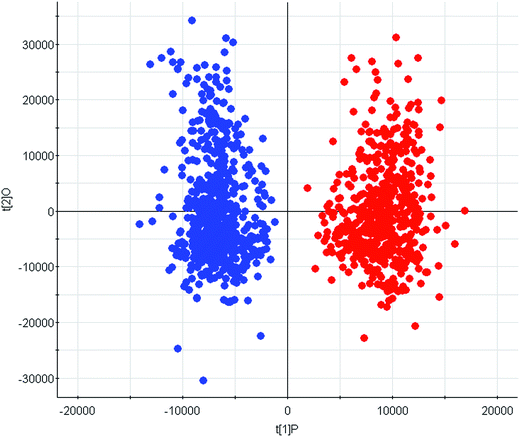 |
| Fig. 2 Score plot of the OPLS-DA model of the FPLC-MS data from patients (blue) and controls (red) group. | |
Abnormal metabolites
We employed an additional multivariate statistical approach termed VIP-plot to select metabolites that contributed to this group behavior observed using OPLS-DA. Higher values of VIP indicate metabolites that are more important to the classification. VIP-plot of PCa patients vs. control individuals was shown in Fig. 3, which is a scatter plot that combines the covariance and correlation for the model variables with respect to model component scores. A total of five discriminate variables as interesting biomarker candidates were found in PCa relative to the control group. These metabolites had robust, statistically significant changes in abundance between PCa and the control group. Five variables were highlighted in VIP-plot (VIP > 15 and P < 0.001) in Fig. 3. Elemental composition was calculated using the Masslynx 4.1 software. Eventually, 5 metabolites were tentatively identified as including glycocholic acid, hippurate, 5-hydroxy-L-tryptophan, taurocholic acid, and chenodeoxycholic acid for early diagnosis of PCa cases and were listed in Table 1. These results suggested that combining FPLC-MS and pattern recognition techniques can be used for a urine metabolomics analysis and screening biomarkers for the early diagnosis of PCa cases. IPA was applied to explore the pathway networks of the differentially-expressed metabolites. It was found that these metabolites had a strong correlation with the farnesoid X receptor and retinoic X receptor (FXR/RXR) activation, hepatic cholestasis, and bile acid biosynthesis, etc. (Fig. 4).
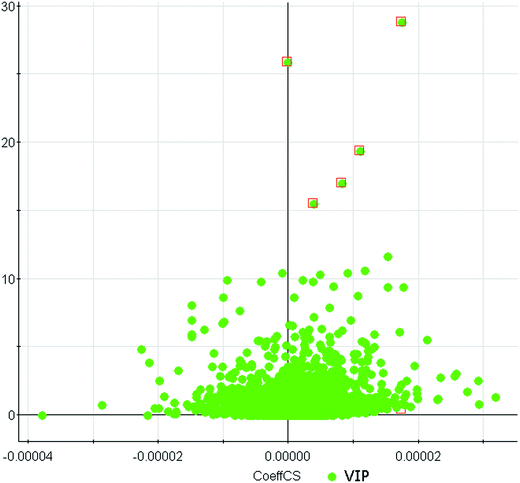 |
| Fig. 3 VIP-plot for selection of interesting variables for patients with PCa and age-matched healthy controls. | |
Table 1 Information of PCa biomarkers detected by high-throughput metabolomics
No. |
VIP |
Rt |
[M + H]+ |
Formula |
Mass error (ppm) |
Metabolites |
HMDB |
Max fold change |
Anova (p) |
Trend |
1 |
28.80 |
8.26 |
466.3082 |
C26H44NO6 |
1.14 |
Glycocholic acid |
HMDB00138 |
7.48 |
0.0000 |
↑ |
2 |
25.89 |
3.93 |
179.0514 |
C9H9NO3 |
1.51 |
Hippurate |
HMDB00714 |
8.54 |
0.0004 |
↑ |
3 |
19.37 |
4.19 |
220.9994 |
C11H14N2O3 |
−1.89 |
5-Hydroxy-L-tryptophan |
HMDB00472 |
4.92 |
0.0007 |
↓ |
4 |
17.02 |
4.35 |
516.2921 |
C26H46NO7S |
−1.88 |
Taurocholic acid |
HMDB00036 |
6.88 |
0.0002 |
↓ |
5 |
15.53 |
2.46 |
393.2948 |
C24H41O4 |
−1.30 |
Chenodeoxycholic acid |
HMDB00518 |
2.46 |
0.0008 |
↑ |
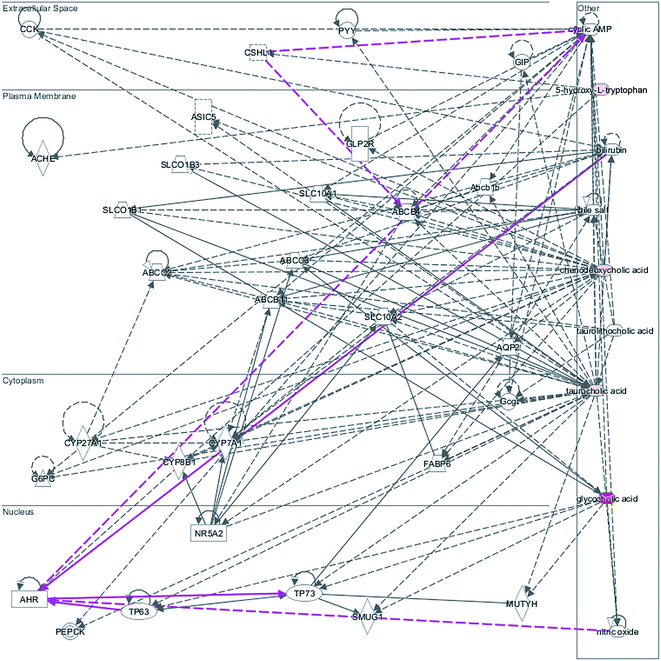 |
| Fig. 4 An overview of the metabolic pathway alterations from PCa patients. | |
Potential diagnostic value
Coupling ROC to its AUC is a widely used method to estimate the diagnostic potential of a classifier in clinical applications. In all biomarkers, 3 potential biomarkers were up-regulated in urine of PCa cases and 2 potential biomarkers were down-regulated. In addition, the AUC values in each selected metabolite were also calculated for evaluating classification test accuracy. Table S2† shows the detailed sensitivity, specificity levels and 95% confidence interval of the 6 identified potential urine biomarkers for PCa early prediction. The values of AUC range from 0.826 to 0.973 indicated the potential capacity of these metabolites for distinguishing PCa patients from normal control subjects. As a single biomarker in urine, glycocholic acid had a sensitivity of 97.2% and a specificity of 96.3% for early predicting PCa. To demonstrate the utility of urine biomarkers for the early diagnosis of PCa, 3 metabolites (AUC > 0.95) comprising glycocholic acid, hippurate, 5-hydroxy-L-tryptophan indicates high predictive ability for PCa patients. Therefore, these urine biomarkers in combination might have important clinical value for the diagnosis of PCa.
Discussion
PCa is a significant cause of morbidity and mortality in men. In clinical practice, the discovery of new biomarkers, the application of omics is emerging, especially metabolomics. Several works have worked to determine how best to applying metabolomics in cancer diagnosis and patient management.17–20 Most metabolomic research on PCa has focused on relatively small metabolites that have long been known to be relevant to the prostate. Despite decades of research, no biomarkers have been less systemically studied for their sensitivity, specificity and accuracy in diagnosis of PCa. Urine assay is inexpensive, and easy to use and rapidly advancing in recent years.21 The collection of urine could reduce the discomfort for patients, particularly if repeated sampling is necessary. Previous studies have demonstrated altered metabolites in urine of PCa patients.22 However, to our knowledge, in-depth study of the relationship between urine metabolite profile and PCa is still needed.
Indeed, some studies have used MS-based metabolomics as a method of discovering biomarkers for diagnosing PCa; however, these studies have used study groups of limited size and invasive sampling methods. The present study examined the urine metabolomics analysis of urine samples from PCa patients and normal controls employing LC/MS-based metabolomics protocols and discriminant analysis was used to construct a panel of urine metabolites that are altered in PCa. FPLC-MS combined with pattern recognition approach could be an advanced tool to help us find metabolites with classifying of sample groups. In this work, we identified the top 5 ranking biochemical markers for PCa in the VIP plot, including glycocholic acid, hippurate, 5-hydroxy-L-tryptophan, taurocholic acid, and chenodeoxycholic acid.
Accurate diagnosis of PCa may facilitate effective prevention and treatment of PCa disease. In this work, an integrated FPLC with MS approach has been developed for performing global metabolomics analysis in urine and identified potential biomarkers for the diagnosis of PCa. To discover potential biomarkers, a total of five potential biomarkers have a close relationship with PCa. Three potential biomarkers were up-regulated in urine of PCa patients and 2 potential biomarkers were down-regulated. Three urine biomarkers (glycocholic acid, hippurate, 5-hydroxy-L-tryptophan) yielded satisfactory accuracy, sensitivity, and specificity in distinguishing PCa patients from the controls. ROC models yielded >95% accuracy of classifications, which suggested that the urine metabolic phenotype of PCa patients was significantly different from that of normal controls. Those 3 metabolites combinations also indicated the possibility of evidence for potential prediction of PCa. Metabolites biomarkers identified in this study had much higher sensitivity, specificity and accuracy simultaneously in diagnosis of PCa. It provided the potential advantages of the application of urine metabolomics in the clinical diagnostics. This study is a pioneering work which explores a new approach to seeking biomarkers for PCa and other diseases. Strengths of our study include its prospective design, the use of large sample size to evaluate the association between urine biomarkers and PCa.
Conclusions
In this study, we attempted to establish the promising the urine metabolites of PCa-specific. It employed FPLC/MS technology to profile the urine metabolites in a cohort of PCa patients and controls. The data were subjected to multivariate analysis in order to discriminate between groups of PCa patients and healthy controls, and then the key-compounds were identified as possible markers of PCa. In the present study, metabolic profiling showed PCa to be associated with a profound abnormality in phenotype. OPLS-DA model yielded class separation for PCa cases and controls. Glycocholic acid, hippurate, 5-hydroxy-L-tryptophan, taurocholic acid, and chenodeoxycholic acid in the urine sample of the PCa cases were significantly different from the control subjects. ROC analysis revealed glycocholic acid, hippurate, 5-hydroxy-L-tryptophan to be potent discriminators between PCa and control groups. This work provides the basis that FPLC/MS-based metabolomics platform could improve the diagnosis of diseases.
Conflict of interests
The authors declare no competing financial interests.
Acknowledgements
This work was supported by grants from the Key Program of Natural Science Foundation of State (Grant No. 81470196, 81302905), Natural Science Foundation of Heilongjiang Province of China (H2015038), Youth Innovative Talent Program of Heilongjiang Province of China (UNPYSCT-2015118).
References
- O. Twu, D. Dessí, A. Vu, F. Mercer, G. C. Stevens, N. de Miguel, P. Rappelli, A. R. Cocco, R. T. Clubb, P. L. Fiori and P. J. Johnson, Trichomonas vaginalis homolog of macrophage migration inhibitory factor induces prostate cell growth, invasiveness, and inflammatory responses, Proc. Natl. Acad. Sci. U. S. A., 2014, 111(22), 8179–8184 CrossRef CAS PubMed.
- L. P. Bokhorst, C. H. Bangma, G. J. van Leenders, J. J. Lous, S. M. Moss, F. H. Schröder and M. J. Roobol, Prostate-specific antigen-based prostate cancer screening: reduction of prostate cancer mortality after correction for nonattendance and contamination in the Rotterdam section of the European Randomized Study of Screening for Prostate Cancer, Eur. Urol., 2014, 65(2), 329–336 CrossRef PubMed.
- J. C. Byrne, M. R. Downes, N. O'Donoghue, C. O'Keane, A. O'Neill, Y. Fan, J. M. Fitzpatrick, M. Dunn and R. W. Watson, 2D-DIGE as a strategy to identify serum markers for the progression of prostate cancer, J. Proteome Res., 2009, 8(2), 942–957 CrossRef CAS PubMed.
- A. Heidenreich, P. A. Abrahamsson, W. Artibani, J. Catto, F. Montorsi, H. Van Poppel, M. Wirth and N. Mottet, European Association of Urology. Early detection of prostate cancer: European Association of Urology recommendation, Eur. Urol., 2013, 64(3), 347–354 CrossRef PubMed.
- S. H. Strand, T. F. Orntoft and K. D. Sorensen, Prognostic DNA methylation markers for prostate cancer, Int. J. Mol. Sci., 2014, 15(9), 16544–16576 CrossRef CAS PubMed.
- A. Dimakakos, A. Armakolas and M. Koutsilieris, Novel tools for prostate cancer prognosis, diagnosis, and follow-up, BioMed Res. Int., 2014, 2014, 890697 Search PubMed.
- V. Medina-Villaamil, S. Martínez-Breijo, P. Portela-Pereira, M. Quindós-Varela, I. Santamarina-Caínzos, L. M. Antón-Aparicio and F. Gómez-Veiga, Circulating MicroRNAs in blood of patients with prostate cancer, Actas Urol. Esp., 2014, 38(10), 633–639 CAS.
- V. M. Barnes, A. D. Kennedy, F. Panagakos and W. Devizio, et al., Global metabolomic analysis of human saliva and plasma from healthy and diabetic subjects, with and without periodontal disease, PLoS One, 2014, 9, e105181 Search PubMed.
- J. Zheng, R. A. Dixon and L. Li, Development of isotope labeling LC-MS for human salivary metabolomics and application to profiling metabolome changes associated with mild cognitive impairment, Anal. Chem., 2012, 84, 10802–10811 CrossRef CAS PubMed.
- M. Yakob, L. Fuentes, M. B. Wang and E. Abemayor, et al., Salivary biomarkers for detection of oral squamous cell carcinoma - current state and recent advances, Curr. Oral Health Rep., 2014, 1, 133–141 CrossRef PubMed.
- T. J. Wang, M. G. Larson, R. S. Vasan and S. Cheng, et al., Metabolite profiles and the risk of developing diabetes, Nat. Med., 2011, 17, 448–453 CrossRef CAS PubMed.
- A. R. Pfenning, et al., Convergent transcriptional specializations in the brains of humans and song-learning birds, Science, 2014, 346, 1256846 CrossRef PubMed.
- K. L. Poulson-Ellestad, E. Hara, O. Whitney and M. V. Rivas, et al., Metabolomics and proteomics reveal impacts of chemically mediated competition on marine plankton, Proc. Natl. Acad. Sci. U. S. A., 2014, 111, 9009–9014 CrossRef CAS PubMed.
- G. Kageyama, J. Saegusa, Y. Irino and S. Tanaka, et al., Metabolomics analysis of saliva from patients with primary Sjögren's syndrome, Clin. Exp. Immunol., 2015, 182(2), 149–153 CrossRef CAS PubMed.
- A. H. Zhang, H. Sun, Y. Han and G. L. Yan, et al., Ultraperformance liquid chromatography-mass spectrometry based comprehensive metabolomics combined with pattern recognition and network analysis methods for characterization of metabolites and metabolic pathways from biological data sets, Anal. Chem., 2013, 85, 7606–7612 CrossRef CAS PubMed.
- X. Zang, C. M. Jones, T. Q. Long, M. E. Monge, M. Zhou, L. D. Walker, R. Mezencev, A. Gray, J. F. McDonald and F. M. Fernández, Feasibility of detecting prostate cancer by ultraperformance liquid chromatography-mass spectrometry serum metabolomics, J. Proteome Res., 2014, 13(7), 3444–3454 CrossRef CAS PubMed.
- W. Struck-Lewicka, M. Kordalewska, R. Bujak, A. Yumba Mpanga, M. Markuszewski, J. Jacyna, M. Matuszewski, R. Kaliszan and M. J. Markuszewski, Urine metabolic fingerprinting using LC-MS and GC-MS reveals metabolite changes in prostate cancer: A pilot study, J. Pharm. Biomed. Anal., 2015, 111, 351–361 CrossRef CAS PubMed.
- D. Kumar, A. Gupta, A. Mandhani and S. N. Sankhwar, Metabolomics-derived prostate cancer biomarkers: fact or fiction?, J. Proteome Res., 2015, 14(3), 1455–1464 CrossRef CAS PubMed.
- A. M. Mondul, S. C. Moore, S. J. Weinstein, S. Männistö, J. N. Sampson and D. Albanes, 1-Stearoylglycerol is associated with risk of prostate cancer: results from serum metabolomic profiling, Metabolomics, 2014, 10(5), 1036–1041 CrossRef CAS PubMed.
- A. Zhang, G. Yan, Y. Han and X. Wang, Metabolomics approaches and applications in prostate cancer research, Appl. Biochem. Biotechnol., 2014, 174(1), 6–12 CrossRef CAS PubMed.
- G. Wang, Y. Zhou, F. J. Huang and H. D. Tang, et al., Plasma metabolite profiles of Alzheimer's disease and mild cognitive impairment, J. Proteome Res., 2014, 13, 2649–2658 CrossRef CAS PubMed.
- R. González-Domínguez, A. García, T. García-Barrera and C. Barbas, et al., Metabolomic profiling of serum in the progression of Alzheimer's disease by capillary electrophoresis-mass spectrometry, Electrophoresis, 2014, 35, 3321–3330 CrossRef PubMed.
Footnote |
† Electronic supplementary information (ESI) available. See DOI: 10.1039/c6ra25007f |
|
This journal is © The Royal Society of Chemistry 2017 |
Click here to see how this site uses Cookies. View our privacy policy here.