DOI:
10.1039/C7RA04057A
(Paper)
RSC Adv., 2017,
7, 34251-34261
1H NMR studies on serum metabonomic changes over time in a kidney-Yang deficiency syndrome model†
Received
10th April 2017
, Accepted 22nd June 2017
First published on 10th July 2017
Abstract
The central aim of this study was to investigate metabolite changes in metabolic pathways via metabonomic approaches in rats suffering from Kidney-Yang Deficiency Syndrome (KYDS) induced by hydrocortisone. Metabonomic analysis using 1H NMR is a well-established approach for the study of metabolic changes in biofluids. In our study, serum samples were obtained at three specific time points during the progression of KYDS, and individual 1H NMR spectra were acquired and statistically assessed using two multivariate analyses (MVA): principal component analysis (PCA) and orthogonal partial least squares-discriminant analysis (OPLS-DA). The profiles on the 15th, 23rd, and 30th day for each sample could be classified, further revealing progression axes from a normal status to KYDS status. Consequently, significant changes in seventeen metabolites, i.e. lactate, valine, alanine, methionine, succinate, glutamine, 3-hydroxybutyrate, creatine, choline, HDL, LDL, VLDL, TMAO, betaine, tyrosine, citrate, and glycerol, were identified. These biochemical changes were found to be directly related to disturbances in energy metabolism, amino acid metabolism, lipid metabolism, choline metabolism, and gut metabolism. We further determined that lactate and the other 16 metabolic markers may be used as specific markers of KYDS over time. Overall, this study demonstrates that this metabonomic method is a valuable tool for studying the pathologic changes of the Chinese medicine syndrome and the underlying mechanisms of KYDS.
1. Introduction
The Kidney-Yang Deficiency Syndrome (KYDS) is a common traditional Chinese medicine (TCM) syndrome.1 Many clinical and experimental studies have shown that the mechanism of KYDS is closely related to the neuroendocrine system (NEIS) of modern medicine. KYDS is usually associated with the hypothalamic–pituitary–target gland (adrenal cortex, thyroid, gonad, and thymus) axes. These axes exhibit various degrees of functional disorder, mainly in the hypothalamus (or higher) parts of this regulatory system.1,2 Different levels of CORT, adrenocorticotropic hormone (ACTH), 3,5,3′-triiodothyronine (T3), thyroxine (T4), and testosterone (T) were analyzed as plasma hormones to evaluate the dysfunction of the hypothalamic–pituitary–target organ axes (HPTOA), as well as the sympathetic/parasympathetic (S/P) nervous system, but these were found to vary significantly.3–5 Moreover, a cortisone species found in blood, ACTH, and 17-OHCS, found in urine, are widely accepted as the biodynamic analytical criteria of KDS-Yang in clinical applications of TCM.6 The analysis of both species can be carried out to assess the success of the hydrocortisone-induced KDS-Yang model.7 The analyses of all four hormone indicators are based on the sensitivity index obtained from our previously reported study.8 In addition, KYDS has also been reported to be critical for the comprehensive performance of multiple systems and organs and has further been associated with energy metabolism, cell signaling system, immune system, and endocrine system.9–12 Our previous studies have shown that in the event of KYDS, the hypothalamic–pituitary–target gland axis hormones change with time, and the changes of KYDS as holistic pathological modifications are also found in this metabolic group. Therefore, herein, we intended to study different metabolic levels of KYDS upon time-dependent dynamic changes.
Metabonomics is one of the important components of phylogenetic biology. Among others, it is used to study dynamic changes of endogenous small molecules in organisms via combination of chemical analysis techniques and chromatographic techniques to characterize the pathophysiological changes in organisms. Furthermore, metabolomics may explain the mechanism of a disease as a whole.13,14 Nuclear magnetic resonance spectroscopy (1H NMR) is a non-destructive, but rarely selective analytical method with high sensitivity for small amounts of samples under physiological conditions.13 Therefore, 1H NMR is one of the most commonly used techniques in current metabonomics.15–17 At present, the hydrocortisone-induced Kidney-Yang Deficiency is the most widely used method of Kidney-Yang Deficiency modeling.18–20 On this basis, male Sprague-Dawley (SD) rats were injected with hydrocortisone to introduce Kidney-Yang Deficiency in stages. Their blood serum was analyzed using 1H NMR combined with multivariate data analysis. Furthermore, specific metabolic markers of the changes of KYDS as well as dynamic changes of the metabolic pathways were studied to gain further insight into the mechanism of the occurrence and development of the Kidney-Yang Deficiency Syndrome.
2. Materials and methods
2.1 Reagents and instruments
A hydrocortisone solution for injection was purchased from Shanxi Jinxin Shuanghe Pharmaceutics Co., Ltd. (State medicine license no.: H14020980, Shanxi, China). A 500 MHz Bruker AVANCE III NMR instrument (produced by Bruker, Switzerland) and D2O were obtained from the Guangdong Pharmaceutical University Center Laboratory. Purified 0.9% saline for injection was purchased from Gaocheng City Sihai Pharmaceutics Co. Ltd (Hebei, China). Sodium dihydrogen phosphate (NaH2PO4) and disodium hydrogen phosphate (Na2HPO4) were of analytical grade and purchased from Qingdao Dragon Technology Co., Ltd.
2.2 Animal studies
Herein, sixty male Sprague-Dawley (SD) rats (SPF grade, age 3 months, weight 200 ± 20 g) were obtained from Guangdong Provincial Experimental Animals Center (License no. SCXK (Yue) 2008-0002). Basic laboratory animal food was supplied by the Guangdong Provincial Experimental Animals Center. The rats were maintained under standard laboratory conditions with a 12 h light/dark cycle at a temperature of 21–23 °C and a relative humidity of 45–65%. All animals were allowed free access to food and water. After acclimatization for 7 day, the rats were randomly divided into a normal control group and a Kidney-Yang Deficiency model group, with 30 rats in each group.
2.3 Animal modeling and handling
Rats in the control group were administered with 0.3 ml of 0.9% saline into the hind limb via intramuscular injection once a day for 29 days in succession. For the Kidney-Yang Deficiency rat model, a hydrocortisone suspension was intramuscularly injected into the hind limb at 2.5 mg/100 g once a day for 29 days in succession.
The control group and the model group were treated via a toxicology experiment method (batch slaughter). Moreover, to obtain dynamic and reliable experimental data, the rats were sacrificed in batches with parallel processing on the 15th day (10 rats), 23rd day (10 rats), and 30th day (10 rats). Serum and tissue samples from the above mentioned animals at each time point were obtained. The samples were pre-processed and stored in a freezer at −80 °C until analysis. Serum samples were used for 1H NMR and related biochemical tests, and tissue samples were used for pathological analysis. All experimental procedures and animal care protocols were performed in conformity with the international guidelines outlined in ‘Principles of Laboratory Animal Care’ (NIH publication no. 85-23 revised 1985) and approved by the institutional ethical committee (IEC) of Guangdong Pharmaceutical University (Guangzhou, China).
2.4 Hormone assays and histopathology
The levels of T3 (triiodothyronine), T4 (tetraiodothyronine), T (testosterone), and CORT (corticosterone) in the serum were measured via radioimmunoassay, and the test kit was operated in accordance with the provided instructions. The freshly extracted pituitary, adrenal, thyroid, and testis tissues were quickly fixed with 10% formalin solution. After dehydration, paraffin-embedding, and slicing, the tissues were stained with hematoxylin and eosin (HE). The stained tissue sections were placed under an optical microscope to observe and obtain the desired pathology images.
2.5 Preparation of the NMR samples
The serum samples were thawed to room temperature prior to NMR analysis and centrifuged at 4 °C for 10 min (14
500 rpm). Then, 300 μl of the supernatant was mixed with 180 μl of phosphate buffer solution (0.2 mol l−1 Na2HPO4/NaH2PO4, pH = 7.4) to minimize the chemical shift variation and 120 μl D2O in a clean EP tube. After complete mixing, the mixture was centrifuged (14
500 rpm, 5 min, 4 °C) to remove any precipitates. Then, 600 μl of the supernatant was pipetted into a 5 mm NMR tube.
2.6 NMR data acquisition
The 1H NMR spectra of all serum samples were obtained using a Bruker spectrometer (Avance III 500 MHz) at 298 K. A one-dimensional Carr–Purcell–Meiboom–Gill (CPMG) [recycle delay-90°(τ-180°-τ)n-acquisition] pulse sequence with water suppression was used to selectively obtain signals with low molecular weight. A total of 128 transients were obtained with 32k data points using a spectral width of 10 kHz with a relaxation delay of 3 s and a total echo time (2nτ) of 100 ms. An exponential function corresponding to a line broadening factor of 0.3 Hz was applied to all acquired free induction decays (FIDs) before Fourier transformation.
2.7 Data processing and multivariate analysis
All serum spectra were introduced into TopSpin (Version 2.1, Bruker Biospin, Germany) to manually perform phase- and baseline-corrections, and a methylation peak of lactate (δ = 1.33 ppm) was used as the reference peak for all chemical shifts. The range of δ 0.5–9.0 ppm was segmented into integral regions with equal widths of 0.004 ppm using AMIX (Bruker, Germany), and the integral value range of δ 4.7–5.2 ppm was set to 0 to eliminate any effects of water suppression. The rest of the integral value of the total area was set to 100% for normalization. The resulting normalized integral data obtained from all serum samples were saved in Excel (Microsoft, USA) for pattern recognition analysis.
Principal component analysis (PCA) and orthogonal partial least squares discriminant analysis (OPLS-DA) were performed on the obtained results using SIMCA 13.0 (Umetrics, Sweden). PCA is an unsupervised analysis, and score plots were used to represent the spatial distribution of the samples. OPLS-DA is a supervised analysis using loading plot changes of metabolites in both groups. The coefficient diagram in this study was obtained by introducing the chemical shift value, the loading value of the scores plot, and the coefficient value (|r|) of the loading plots into MATLAB (The Mathworks Inc, USA). The colored bars on the right side of the figure indicate the degree of difference between both groups. When the difference between the two groups is red, the difference is significant, whereas blue indicates that the difference is not significant. The spectra of the samples and HMDB, KEGG, MetaboAnalyst, and other databases were combined to identify significant differences in the metabolic markers and related metabolic pathways.
2.8 Statistical analysis
Using SPSS16.0 (Chicago, IL, USA) on the relevant data for an independent sample t test, P < 0.05 as a numerical difference was determined to be statistically significant. On the basis of this notion, |r| ≥ 0.707 was used as a candidate metabolic biomarker according to the correlation coefficient threshold.
3. Results
3.1 Changes in the body weight and hormone parameters
Table 1 shows the changes in the body weight and the concentrations for four hormone types of the pituitary-target gland axis in the control group and model group at three time points (15th, 23rd, and 30th day). The overall rat weight in the model group was found to be significantly lower than that in the control group, and the difference was determined to be statistically significant (P < 0.01). The indices of T3, T4, T, and CORT were found to decrease over time, and the concentrations in the model group were significantly lower than those in the control group. The abovementioned two findings indicated that the Kidney-Yang Deficiency model was successfully established in this study.
Table 1 Summary of the body weight and serum hormone parameters at the end of the 15th, 23rd, and 30th daya
Index |
15th day |
23rd day |
30th day |
Control |
Model |
Control |
Model |
Control |
Model |
Values are presented as mean ± SD. N = 8 per group. △: indicates the same index in the same period when compared with that of the control, △P < 0.01; ☆ and ★: represent the same index in the same group when compared with the 15th day ☆P < 0.01, and compared with the 30th day ★P < 0.01. |
Weight (g) |
275.20 ± 29.26 |
216.60 ± 14.39△ |
283.70 ± 17.56 |
204.70 ± 23.31△ |
299.40 ± 28.63△ |
201.86 ± 21.74△ |
T3 (nmol L−1) |
6.18 ± 0.45 |
4.44 ± 0.54△ |
6.11 ± 0.47 |
4.33 ± 0.49△ |
6.41 ± 0.45 |
1.71 ± 0.44△★ |
T4 (nmol L−1) |
73.36 ± 2.50 |
43.40 ± 5.84△ |
84.05 ± 2.81 |
44.58 ± 3.40△ |
82.68 ± 2.68 |
24.62 ± 2.43△★ |
T (ng dL−1) |
177.75 ± 4.81 |
63.29 ± 2.30△ |
178.71 ± 1.93 |
21.85 ± 1.44△☆ |
180.07 ± 3.73 |
14.54 ± 0.39△★ |
CORT (ng mL−1) |
151.53 ± 2.74 |
97.23 ± 2.95△ |
150.67 ± 2.95 |
65.53 ± 4.63△☆ |
149.66 ± 3.19 |
32.98 ± 3.73△★ |
3.2 Influence of hydrocortisone on histopathology
Fig. 1 shows the H&E-stained histological evaluation of the pathology of the pituitary, adrenal gland, thyroid, and testicular tissue sections at the end of the 30th day. As can be seen in the figure, as compared to the control, in the model group, pituitary tissue eosinophils increased, basophilic cells decreased, and vacuoles degenerated (A). In the model group, the boundaries and levels of the adrenal cells were not clearly defined. Furthermore, the intracellular lipid concentration was found to significantly increase and vacuoles were formed; moreover, the cell volume decreased and arranged sparsely, and the cell was translucent (B). In the model group, the number of thyroid tissues was found to be reduced, and the follicles became unclear and filled with glial, and their overall number was reduced (C). The levels of testicular stromal cells and spermatogenic cells in the model rats were found to be reduced. The spermatogenic cells in the seminiferous tubules were also found to be reduced. In addition, the overall sperm number was also reduced to a varying degree (D). The above mentioned changes indicate that the rats demonstrate the typical pathological features of the Kidney-Yang Deficiency Syndrome, indicating that the establishment of this model was successful.
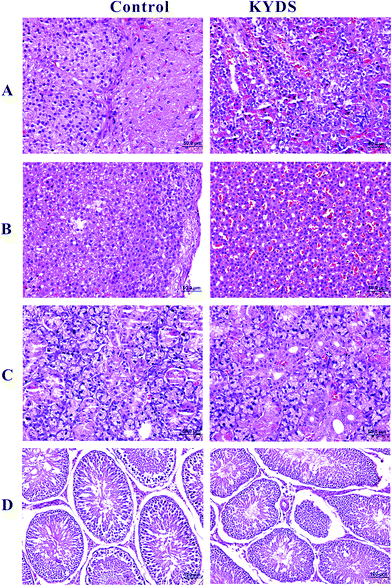 |
| Fig. 1 H&E staining for histological evaluation. Typical images of pituitary (A), adrenal (B), thyroid (C), testis (D) sections stained with H&E at the end of the 30th day ((A)–(C) magnification 40× and (D) magnification 20×). | |
3.3 1H NMR spectroscopy
Serum 1H NMR spectra for the control and KYDS groups obtained on the 30th day are shown in Fig. 3. The magnification rate at low field (δ 5.2–8.5) and high field (δ 0.5–4.7) is 16× and 2×, respectively. Assignments of endogenous metabolites were based on reported literature.21–23 The main metabolites that can be seen in the spectra include amino acids (leucine, isoleucine, valine, alanine, and lysine), TCA cycle intermediate metabolites, lipids, lactate, and glucose. To detect further potential biomarkers in the KYDS model rats, we performed multivariate data analysis.
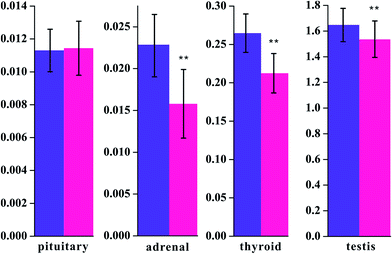 |
| Fig. 2 Visceral index of pituitary, adrenal, thyroid, and testis at the end of the 30th day (unit: g). **P < 0.01 vs. control group ( : control group, : model group). | |
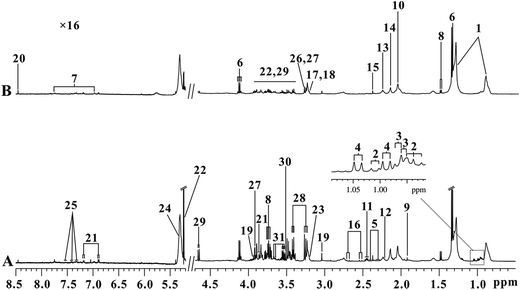 |
| Fig. 3 Representative serum ((A) control, (B) model) 1H NMR spectra for controls and KYDS model rats at the end of the 30th day. Keys: (1) lipid; (2) isoleucine; (3) leucine; (4) valine; (5) 3-hydroxybutyrate; (6) lactate; (7) 1-methylhistine; (8) alanine; (9) acetate; (10) N-acetylglycoprotein; (11) glutamine; (12) acetone; (13) acetoacetate; (14) O-acetylglycoprotein; (15) pyruvate; (16) citrate; (17) PC; (18) GPC; (19) creatine; (20) formate; (21) tyrosine; (22) α-glucose; (23) choline; (24) unsaturated lipid; (25) phenylalanine; (26) TMAO; (27) betaine; (28) taurine; (29) β-glucose; (30) glycine; and (31) glycerol. | |
3.4 Analysis of metabolic patterns and identification of potential biomarkers
Fig. 4A1 shows the score plots of PCA, representing the distribution of all serum samples at the end of the 15th, 23rd, and 30th day in the KYDS group. A progression in classification could be observed at the three time points, whereas the control group displayed no obvious differences (cf. Fig. 4A2).
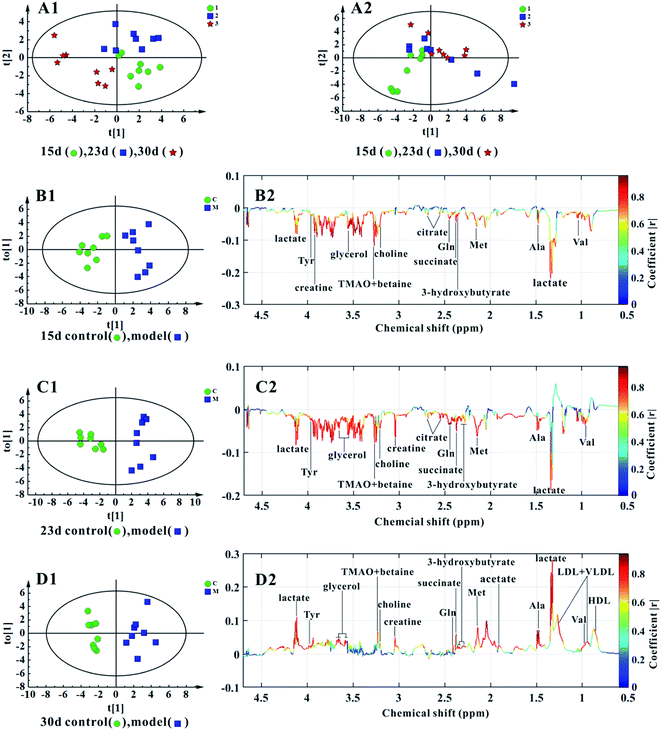 |
| Fig. 4 Multivariate data analyses of the 1H NMR spectra of serum at the end of the 15th, 23rd, and 30th days. (A2, A1) PCA score plots of the control (R2X = 76.4%, Q2 = 64.5%) and KYDS model (R2X = 95.7%, Q2 = 79.2%) rats at three time points. (B1, B2) Score plots and coefficient-coded loading plots of OPLS-DA between the control and KYDS model groups on the 15th day (R2X = 76.3%, R2Y(cum) = 88.3%, Q2(cum) = 77.5%). (C1, C2) Score plots and coefficient-coded loading plots of OPLS-DA between the control and KYDS model groups on the 23rd day (R2X = 70.7%, R2Y(cum) = 86.8%, Q2(cum) = 70.3%). (D1, D2) Score plots and coefficient-coded loading plots of OPLS-DA between the control and the KYDS model groups on the 30th day (R2X = 84.5%, R2Y(cum) = 92.1%, and Q2(cum) = 76.5%). | |
OPLS-DA models were established for the classification of the controls and the KYDS rats to identify the associated potential biomarkers. The score plots of OPLS-DA (Fig. 4B1–D1) show a clear separation between the controls and the KYDS model rats. The coefficient-coded loading plots (cf. Fig. 4B2) showed that the levels of lactate, valine, alanine, methionine, 3-hydroxybutyrate, succinate, glutamine, creatine, choline, TMAO, betaine, tyrosine, citrate, and glycerol decreased, whereas HDL, LDL, VLDL, and acetate did not display any significant concentration changes at the end of the 15th day. Note that at the end of the 23rd day, the metabolite concentrations were essentially the same as at the end of the 15th day (cf. Fig. 4C2). On the contrary, elevated levels of lactate, HDL, LDL, VLDL, valine, alanine, acetate, methionine, succinate, glutamine, 3-hydroxybutyrate, creatine, choline, TMAO, betaine, tyrosine, and glycerol could be observed in the serum samples of the KYDS models at the end of the 30th day (cf. Fig. 4D2). Table 2 summarizes the statistical analysis results of the normalized integrals of screened urine metabolites (cf. Fig. 4), further differentiating between two groups at the three time points.
Table 2 Statistical analysis results for metabolite changes in the serum of the control and the model group at the 15th, 23rd, and 30th daya
Metabolites |
Chemical shift |
Variations |
15th day |
23rd day |
30th day |
*: indicates significant changes as compared to that of the control *P < 0.05; **P < 0.01. |
Lactate |
1.33(d), 4.11(q) |
↓** |
↓* |
↑** |
HDL |
0.84(m) |
— |
— |
↑** |
LDL + VLDL |
0.89(m), 1.29(m) |
— |
— |
↑** |
Valine |
0.97(d), 3.57(d), 1.02(d) |
↓** |
↓* |
↑** |
Alanine |
1.46(d) |
↓* |
↓* |
↑** |
Acetate |
1.91(s) |
— |
— |
↑** |
Methionine |
2.13(s) |
↓* |
↓** |
↑** |
3-Hydroxybutyrate |
2.31(m), 2.38(m), 4.13(m) |
↓* |
↓** |
↑** |
Succinate |
2.41(s) |
↓** |
↓** |
↑** |
Glutamine |
2.08(m), 2.09(m), 2.41(m) |
↓* |
— |
↑** |
Creatine |
3.93(s), 3.04(s) |
↓* |
↓** |
↑** |
Choline |
3.21(s) |
↓** |
↓** |
↑* |
TMAO + betaine |
3.27(s) |
↓** |
↓** |
↑** |
Tyrosine |
3.94(dd), 3.06(dd) |
↓** |
↓* |
↑** |
Citrate |
2.52(d), 2.69(d) |
↓** |
↓** |
— |
Glycerol |
3.56(dd) |
↓* |
↓** |
↑** |
3.5 Construction and analysis of the metabolic pathway
The construction, interaction, and pathway analyses of potential biomarkers were performed using the MetPA software based on database sources including KEGG, SMPD, and the Human Metabolome Database to identify the metabolic pathways. The possible biological roles were evaluated via enrichment analysis of MetaboAnalyst. By relating the metabolic pathways, the metabolic network of the potential biomarkers could be established, as shown in Fig. 5.
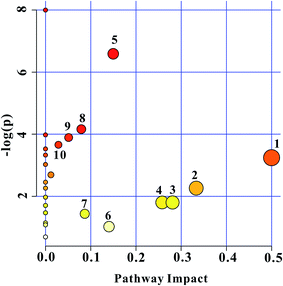 |
| Fig. 5 Summary of the pathway analysis using MetPA. (1) Phenylalanine, tyrosine, and tryptophan biosynthesis; (2) valine, leucine, and isoleucine biosynthesis; (3) glyoxylate and dicarboxylate metabolism; (4) glycerolipid metabolism; (5) alanine, aspartate, and glutamate metabolism; (6) tyrosine metabolism; (7) citrate cycle (TCA cycle); (8) pyruvate metabolism; (9) glycolysis or gluconeogenesis; and (10) cysteine and methionine metabolism. The individual spot size is proportional to the impact of each pathway, and the spot color denotes the significance from highest (red) to lowest (white). | |
Metscape represents a tool for the interactive exploration and visualization of experimental metabonomics and gene expression data in the context of human metabolic networks. It allows users to build and analyze networks of genes or compounds, identify enriched pathways from expression profiling data, and visualize changes in metabolite data. To gain further insight into the relationship between metabolites, 18 statistically significant differential biomarkers were mapped to KEGG IDs, and a compound network was generated (cf. Fig. 6) consisting of nodes (metabolites) and edges that represented biochemical reactions. The network reflects the complex pathology and provides further evidence for the involvement of amino acid metabolism ((1) phenylalanine, tyrosine, and tryptophan biosynthesis; (2) valine, leucine, and isoleucine biosynthesis; (3) alanine, aspartate, and glutamate metabolism; (4) cysteine and methionine metabolism; and (5) tyrosine metabolism), glyoxylate and dicarboxylate metabolism, glycerolipid metabolism, the citrate cycle (TCA cycle), pyruvate metabolism, and glycolysis or gluconeogenesis.
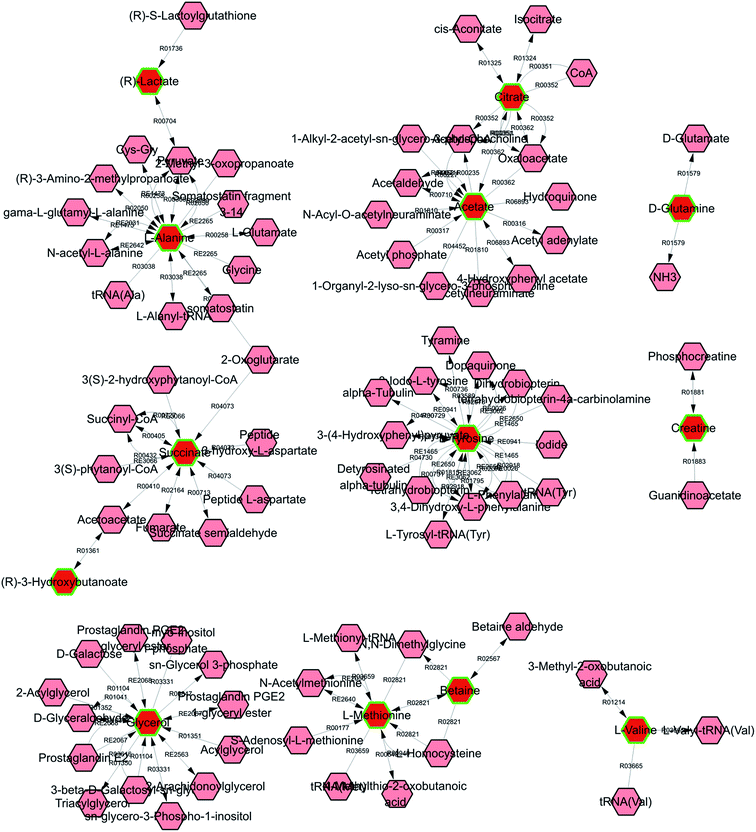 |
| Fig. 6 Network of potential biomarkers associated with KYDS via metscape analysis. | |
4. Discussion
To validate the successful implementation of this animal model, the concentrations of T3, T4, T, and CORT were widely used as biodynamic criteria of KYDS, as determined in the serum of the rats.24,25 The results of weight and radioimmunoassy are provided in Table 1 and are in accordance with the results obtained from previously reported research.26 The T3, T4, T, and CORT concentrations significantly decreased in the model rats when compared with those in the control group. The biochemical results obtained indicated that the expression of the hypothalamic–pituitary–thyroid, adrenal, and gonad axes was restrained. Moreover, the disease severity was further verified by H&E staining of hypothalamic, pituitary, adrenal, and thyroid sections. Fig. 1 shows the histopathological results of the tissues for both the control and the model groups. The visceral index of the model groups shows significant decreases in adrenal, thyroid, and testis tissues at the end of the 30th day (P < 0.01) and an increase in the pituitary index as compared to that of the controls. The visceral index is a reflection of the immune suppression state in the model rats (cf. Fig. 2). In our study, we showed a series of metabolite changes in the serum of KYDS model rats using a combination of pattern recognition techniques and 1H NMR-based metabonomics, revealing the systematic metabolic progression of KYDS to further identify the potential biomarkers for KYDS.
4.1 Disturbed energy metabolism
Energy generation in vivo requires ATP, mainly formed from glucose, glycolysis, and lipid oxidation. Generally, many substrates, including glucose, amino acids, fatty acids, and ketone bodies, can be used for this biochemical process. The mitochondrial TCA cycle is the final metabolic pathway, which oxidizes and decomposes carbohydrates, amino acids, and fatty acids, and it also represents a critical link in the energy supply of an organism. The levels of citrate and succinate, important intermediates in the TCA cycle, were found to continuously decrease in the KYDS rats. This latter fact was most likely due to the inhibition of the TCA cycle, suggesting that energy metabolism was disturbed and mitochondrial function was impaired by hydrocortisone.
Creatine is composed of three amino acids: arginine, glycine, and methionine. Its main function is to provide energy for muscles and nerve cells. When the body provides ATP in an insufficient amount, creatine can quickly synthesize ATP for energy restoration. Over the past few decades, numerous studies have shown that creatine supplementation exhibits a variety of favorable effects, including enhanced muscle mass (growth/hypertrophy), on skeletal muscle physiology and metabolism.27 Therefore, creatine may rapidly increase muscle strength and accelerate fatigue recovery. In this experiment, the decrease of creatine in serum leads to the symptoms of excessive fatigue and reduced activity in rats.28 This is consistent with the behavioral characteristics of patients with KYDS.
Additionally, due to the interference of hydrocortisone, the content of lactate and acetate increased. Under normal physiological conditions, lactate obtained from the lactic acid cycle in the body eventually decomposes to produce CO2 and water. This indicates that in energy metabolism disorders, anaerobic conditions of glycolysis and ketone body metabolism are generally increased.
4.2 Alteration in the amino acid pathway
Tyrosine is an essential amino acid that plays an important role in protein biosynthesis and energy production. It is the precursor of catecholamines (including dopamine, norepinephrine, epinephrine) and studies have shown that injection of hydrocortisone may lead to transient induction of tyrosine aminotransferase and accelerate the synthesis and secretion of catecholamines.29,30 In the present study, the concentration of tyrosine significantly increased at the end of the 30th day. This finding is consistent with the results obtained in previously reported research.31 Studies have shown that unilateral ureteral obstruction in rats significantly decreases the valine levels in the body by enhancing the protein synthesis activities to repair damaged membrane protein structures.32 We determined a significant reduction in the serum valine at the end of the 15th and 23rd days possibly due to the destruction of cell membrane proteins by hydrocortisone.
Under normal circumstances, through the alanine–glucose cycle of toxic ammonia in the muscle, a non-toxic form of alanine may be formed and transported to the liver, where urea is synthesized from two ammonia molecules and one carbon dioxide molecule before excretion. At the end of the 15th day and at the end of the 23rd day, the alanine content was found to decrease. However, the alanine content at the end of the 30th day indicated that the alanine–glucose cycle was disturbed and the ammonia transport function was inhibited. This finding is in accordance with the perspective that the accumulation of alanine may be a result of the effect of hydrocortisone on gluconeogenesis via stimulation of the synthesis of certain key enzymes in the glucose and alanine cycle.33
Methionine is an initial amino acid in the protein synthesis in vivo, which can be converted into S-adenosylmethionine (SAM). The latter is a donor of an active methyl group in the methylation reactions in vivo. Methionine can also be converted to cysteine and homocysteine via demethylation, whereas the increased presence of homocysteine in renal tissue is an indicator of impaired renal function and potential kidney failure.34 In this study, methionine was also found to be up-regulated, providing evidence for kidney damage. In addition, Y. Tang et al.28 have reported that the increase in betaine content in KYDS rats suggests that KYDS may be related to the acceleration of energy metabolism, immunosuppression, and oxidative stress, that are associated with methionine metabolism.
Glutamine is the most abundant type of free amino acid in animal tissue and acts as the primary intestinal energy source.35 Furthermore, glutamine acts as an intestinal function regulator and antioxidant. Clinical and experimental studies have shown that maintaining a defined level of glutamine is essential for the normal function of the intestinal tract, particularly in the case of intestinal inflammation and diarrhea.36,37 It has been reported that glutathione synthesis using glutamine has a positive effect on improving the antioxidant capacity of the intestine, potentially protecting the intestinal tract from bacterial growth and toxins38 while also regulating the barrier function of intestinal cells and enhancing the transmembrane transport of nutrients.39 At the end of 15th day and 23rd day, the level of glutamine was found to significantly decrease. This could be due to the fact that the intestinal flora of the KYDS rats was disturbed, resulting in intestinal damage, diarrhea, and loss of appetite.
4.3 Disturbed lipid metabolism
Lipoproteins play a key role in regulating plasma and tissue levels of cholesterol. Apolipoprotein B (apoB)-containing lipoproteins include chylomicrons, very-low density lipoprotein (VLDL), and low-density lipoprotein (LDL).40 HDL is the smallest lipoprotein that transports cholesterol from extra-hepatic tissues to the liver for excretion, whereas LDL and VLDL transport about 75% of the body's cholesterol to the cells. Numerous preclinical studies have demonstrated various atheroprotective effects of HDL such as promoting reverse cholesterol transport, anti-inflammatory and anti-thrombotic effects, and beneficial effects on endothelial cell function.41,42 Furthermore, it has been reported that elevated HDL levels result in a decreased risk of coronary heart disease (CHD),43 and abnormal concentrations of LDL and VLDL are generally associated with atherosclerosis.44 Herein, the level of LDL and VLDL was found to be up-regulated at the end of the 30th day in conjunction with lower levels of HDL on the 15th and 23rd days, indicating that the risk of cardiovascular disease in KYDS rats was increased. Moreover, George et al.45 have reported that chronic kidney disease (CKD) is characterized by specific changes, including LDL and HDL reduction as well as an increase in VLDL, in the levels of lipoprotein. Therefore, KYDS rats may also suffer from symptoms that are characteristic of the CKD.
3-Hydroxybutyrate, a ketone body belonging to the fatty acids family, can be utilized as an energy source when blood glucose levels are decreased.46 The compound has been shown to mediate energy metabolism in the body as an intermediate of the tricarboxylic acid (TCA) cycle. Levels of ketone bodies have been shown to increase if acetyl-CoA derived from β-oxidation of free fatty acids exceeds the capacity of the TCA cycle.47 Furthermore, it has been reported that an increased plasma level of 3-hydroxybutyrate can be observed in chronic atrophic gastritis (CAG) rats; this indicates that a promotion of fatty acid oxidation is involved in the pathogenesis of CAG.48 Therefore, lower levels of 3-hydroxybutyrate at the end of the 15th and 23rd day may be an indication of impaired fatty acid metabolism.
4.4 Disturbed choline metabolism
Choline is an important precursor of phosphocholine and represents one of the major cell membrane components that can be activated by choline kinase and phosphorylcholine cytidylyltransferase. Choline-like compounds, along with myo-inositol, are integral components of membrane phospholipids.49 In addition, choline acts as a precursor of the neurotransmitter acetylcholine via oxidation to betaine. Choline functions as a methyl group donor in pathways that produce S-adenosylmethionine.50 As a methyl donor group, choline and betaine may influence DNA and histone methylation.51 Moreover, choline deficiency has been linked to neurological impairment,52 and targeting enzymes related to choline metabolism have been postulated to provide promising therapeutic opportunities for tumour growth arrest.53 Serum obtained after 15 and 23 days demonstrated that choline levels were significantly reduced; however, the levels were found to be significantly increased on the 30th day. This finding indicates that the cell membrane phosphocholine metabolism was interrupted by hydrocortisone. Betaine, an oxidation product of choline, is generally regarded as safe for dietary ingestion.54 It has two main physiological functions: it may act as an osmolyte and as a methyl donor (transmethylation). Betaine can protect cells, proteins, and enzymes from environmental stress (such as low water, high salinity, or extreme temperature) and participates in the methionine cycle together with phosphate as a methyl donor.55 In addition, betaine homocysteine methyl transferase (BHMT) plays a crucial role in the cycle pathway and is regarded as an auto-antigen associated with immune reactivity.56 Betaine and creatine are products of choline involvement in the methylation reaction, and their decreased levels indicate that methyl transfer reactions may be inhibited. The latter reaction type is involved in the synthesis of many important physiological molecules such as adrenal cortical hormones.57 Melanie et al.58 have shown that the lack of choline in animals can lead to the formation of large areas of renal necrosis and renal failure in renal tissue. Therefore, a significant reduction in choline in the first two time periods (15th and 23rd day) may lead to renal failure in the KYDS rats. In addition, choline is an important source of methyl groups involved in the S-adenosylmethionine synthesis pathway of betaine. Thus, the reduction of choline in the serum also leads to the accumulation of betaine.
4.5 Disturbed gut metabolism
TMAO, produced from betaine by the gut flora, has been shown to be able to predict an increased cardiovascular disease (CVD) risk as well as an increased incidental risk of major adverse cardiovascular events (myocardial infarcts, stroke, and death) in large-scale human clinical studies.59–61 There are many strains in the intestine that can decompose choline to produce trimethylamine (TMA). TMA, in turn, is oxidized to trimethylamine oxide (TMAO) in the liver in the presence of trimethylamine oxidase.62 Studies have shown that the levels of TMAO in urine and plasma of hypertensive rats are increased over time that are generally associated with disturbances in intestinal flora activity.63 In this experiment, TMAO in the serum was significantly decreased on days 15 and 23, but was found to increase at the end of the 30th day. The latter result indicates that the intestinal flora was indeed disturbed. In a healthy body, kidneys use small molecule organo-osmotic agents (TMAO, betaine, taurine, etc.) to maintain osmotic balance.64 Ogbron et al.65 have reported that reductions in TMAO may lead to unilateral ureteral obstruction in the kidney cells of rats and may result in osmotic disorders. Therefore, decreased TMA concentrations in the serum obtained on days 15 and 23 also indicate severe kidney damage in the KYDS rats together with osmotic disorders.
Upon injection of an exogenous glucocorticoid (hydrocortisone), the hypothalamus produces a negative feedback regulation. As a result, hypothalamus–pituitary–target gland (thyroid, adrenal, and gonadal) atrophy and histopathological changes could be observed and the secretion of hormones (T, T3, T4, and CORT) was significantly reduced. This further lead to disorders of energy metabolism (citrate, succinate, lactate, glycerol, and acetate), amino acid metabolism, (tyrosine, valine, alanine, methionine, and glutamine), lipid metabolism (HDL, LDL, VLDL, and 3-hydroxybutyrate), choline metabolism (choline, betaine, and creatine), and flora metabolism (TMAO) and ultimately results in a significant increase or decrease in the concentrations of the corresponding metabolites in the serum.
After statistical analysis, we found that the levels of nearly all important metabolites (colored in red) were decreased or increased together. The specific situation was that the levels of the metabolic markers decreased together in the first two time points of the Kidney-Yang Deficiency syndrome, whereas in the late time point, the levels increased. This may indicate whether the rats are in the state of Kidney-Yang Deficiency. In the first two periods, as a result of hydrocortisone injection, the hypothalamus–pituitary–target gland axis was in a state of inhibition, resulting in a decrease in hormone secretion and a decrease in metabolite levels. The later results may be because the rats leap out of the state of KYDS, a sharp decline in the hormone levels occurs, and the effect of hormones on the regulation of metabolites becomes very weak, leading to the recovery of the metabolite levels. This may be due to the long-term toxicity of hydrocortisone. The results of this experiment have also aroused our great concern. Thus, our latest subject will be an in-depth study and verification of this interesting phenomenon to find a more reasonable and credible explanation. Moreover, the Kidney-Yang Deficiency modeling time should also be considered. Therefore, we suggest that while studying the Kidney-Yang Deficiency model, the mid-term selection (23 days or so) will be better. If we want to conduct a different period or longer study, we should pay attention to appropriately shorten the modeling time or increase the amount of injection to ensure that the duration of the Kidney-Yang Deficiency model is long enough.
5. Conclusions
In this study, 1H NMR was used to study the metabolic markers of time-dependent changes in the serum induced by hydrocortisone. 1H NMR combined with multivariate analysis was used to study the serum of KYDS rats, and the changes of endogenous metabolites from the normal state to Kidney-Yang Deficiency were evaluated. We found that at the end of the 15th day and 23rd day, the concentration of serum lactate, acetate, citrate, succinate, HDL, LDL, VLDL, glycerol, betaine, creatine, TMAO, valine, alanine, methionine, glutamine, tyrosine, and 3-hydroxybutyrate significant decreased. However, a significant increase was observed at the end of the 30th day. The metabolic pathways associated with this observation include energy metabolism, the amino acid pathway, lipid metabolism, choline metabolism, and gut metabolism. These metabolic markers and metabolic networks provide a new method to study Kidney-Yang Deficiency from the system's and overall perspective. In future studies, we will identify the metabolic markers of KYDS with genomics to gain further insight into the relationship between genes and enzymes. Simultaneously, we will obtain clinical samples and data to further verify the relationship between these metabolic markers and KYDS.
Conflict of interest
The authors declare no conflicts of interest.
Acknowledgements
We would like to acknowledge the financial support received from the National Natural Science Foundation of China (No. 81403153), Science and Technology Planning Project of Guangdong Province, China (No. 2014A020212603), and Opening Project of Guangdong Province Key Laboratory of Computational Science at the Sun Yat-sen University (No. 2016003).
References
- X. Lu, Z. Xiong, J. Li, S. Zheng, T. Huo and F. Li, Talanta, 2011, 83, 700–708 CrossRef CAS PubMed.
- J. Xiao, Y. X. Wang, J. D. Gao, D. Huang, M. H. Shao and L. Q. He, Acta Univ. Tradit. Med. Sin. Pharmacol. Shanghai, 2008, 22, 73–76 Search PubMed.
- X. M. Lu, Z. L. Xiong, J. J. Li, S. N. Zheng, T. G. Huo and F. M. Li, Talanta, 2011, 83, 700–708 CrossRef CAS PubMed.
- D. X. Huang, J. Yang, X. M. Lu, Y. Deng, Z. L. Xiong and F. M. Li, J. Pharm. Biomed. Anal., 2013, 76, 200–206 CrossRef CAS PubMed.
- B. Li, Q. Luo, M. Nurahmat, H. L. Jin, Y. J. Du, Y. B. Wu, M. Sun, W. Y. Gong and J. C. Dong, Evid. base. Compl. Alternative Med., 2013, 658364 Search PubMed.
- Z. Y. Shen, Zhongguo Zhongxiyi Jiehe Zazhi, 1997, 17, 50–52 CAS.
- L. Zhao, H. Wu, M. Qiu, W. Sun and R. Wei, Evid. base. Compl. Alternative Med., 2013, 540957 Search PubMed.
- X. F. Wang, J. Li, L. Zhang, N. Ma, L. C. Luo and Q. H. Wu, Chin. J. Integr. Tradit. West. Med., 2013, 33, 825–829 Search PubMed.
- A. H. Zhang, X. H. Zhou, H. W. Zhao, S. Y. zou, C. W. Ma, Q. Liu, H. Sun, L. Liu and X. J. Wang, Mol. BioSyst., 2017, 13, 320–329 RSC.
- J. S. Wang, T. Reijmers, L. J. Chen, R. V. D. Heijden, M. Wang, S. Q. Peng, T. Hankemeier, G. W. Xu and J. V. D. Greef, Metabolomics, 2009, 5, 407–418 CrossRef CAS PubMed.
- T. G. Huo, S. Cai, X. M. Lu, Y. Sha, M. Y. Yu and F. M. Li, J. Pharm. Biomed. Anal., 2009, 49, 976–982 CrossRef CAS PubMed.
- S. N. Zheng, M. Y. Lu, X. M. Lu, T. G. Huo, L. Ge, J. Y. Yang, C. F. Wu and F. M. Li, Clin. Chim. Acta, 2010, 411, 204–209 CrossRef CAS PubMed.
- G. Gao, G. J. Yang and Z. Y. Lou, Acad. J. Second Mil. Med. Univ., 2009, 30, 565–568 CrossRef CAS.
- M. J. Chen, L. P. Zhao and W. Jia, J. Proteome Res., 2013, 4, 2391–2396 CrossRef PubMed.
- J. L. Zhang, J. C. Li, B. L. Li, L. J. Wang and Y. X. Yang, J. Third Mil. Med. Univ., 2016, 38, 522–526 CAS.
- J. T. Brindle, H. Antti, E. Holmes, T. Tranter, J. K. Nicholson, H. W. L. Bethell, S. Clarke, P. M. Schofield, E. Mckilligin, D. E. Mosedale and D. J. Grainger, Nat. Med., 2002, 8, 1439–1445 CrossRef CAS PubMed.
- G. A. Nagana Gowda, Y. N. Gowda and D. Raftery, Anal. Chem., 2015, 87, 706–715 CrossRef CAS PubMed.
- Z. J. Zou, M. J. Gong, Y. Y. Xie, S. M. Wang and S. W. Liang, Chin. J. Exp. Tradit. Med. Formulae, 2012, 18, 133–136 CAS.
- H. Jiang, Y. Z. Wang and J. R. Hu, Chin. J. Exp. Tradit. Med. Formulae, 2011, 17, 211–214 Search PubMed.
- J. Du, N. Li and H. M. Wang, J. Clin. Rehabil. Tissue Eng. Res., 2010, 14, 9433–9436 Search PubMed.
- C. Y. Jiang, K. M. Yang, L. Yang, Z. X. Miao, Y. H. Wang and H. B. Zhu, PLoS One, 2013, 8, e66786 CAS.
- J. K. Nicholson, P. J. D. Foxall, M. Spraul, R. D. Farrant and J. C. Lindon, Anal. Chem., 1995, 67, 793–811 CrossRef CAS PubMed.
- A. Pechlivanis, S. Kostidis, P. Saraslanidis, A. Petridou, G. Tsalis, V. Mougios, H. G. Gika, E. Mikros and G. A. Theodoridis, J. Proteome Res., 2010, 9, 6405–6416 CrossRef CAS PubMed.
- C. H. Liu, Y. M. Sun, Y. Li, W. X. Yang, M. M. Zhang, C. L. Xiong and Y. C. Yang, Nutr. Diet., 2012, 69, 64–68 CrossRef.
- S. N. Zheng, M. Y. Yu, X. M. Lu, T. G. Huo, L. Ge, J. Y. Yang, C. F. Wu and F. M. Li, Clin. Chim. Acta, 2010, 411, 204–209 CrossRef CAS PubMed.
- Y. Nan, X. H. Zhou, Q. Liu, A. H. Zhang, Y. Guan, S. H. Lin, L. Kong, Y. Han and X. J. Wang, J. Chromatogr. B: Anal. Technol. Biomed. Life Sci., 2016, 1026, 217–226 CrossRef CAS PubMed.
- F. Farshidfar, M. A. Pinder and S. B. Myrie, Curr. Protein Pept. Sci., 2017, 18, 1 CrossRef PubMed.
- Y. Tang, X. R. Liu, C. Lu, X. J. He, J. Li, C. Xiao, M. Jiang, J. Yang, K. Zhou, Z. X. Zhong, W. D. Zhang and A. P. Lu, J. Ethnopharmacol., 2014, 152, 585–593 CrossRef PubMed.
- V. N. Chesnokov, V. G. Gadzhiev, G. I. Leontyeva, S. M. Zelenin and N. P. Mertvetsov, Biomed. Biochim. Acta, 1990, 49, 1177–1186 CAS.
- A. S. Tischler, R. L. Perlman, G. M. Morse and B. E. Sheard, J. Neurochem., 1983, 40, 364–370 CrossRef CAS PubMed.
- X. M. Tao, ph.d. Dissertation, Shanghai Jiao Tong university, 2009.
- Z. Y. Li, A. P. Li, J. N. Gao, H. Li and X. M. Qin, Front. Pharmacol., 2016, 7, 307–319 Search PubMed.
- T. Mckee and J. R. Mckee, Biochemistry: an introduction, McGraw-Hill companies, inc, 1999 Search PubMed.
- Y. E. C. Taes, J. R. Delanghe, A. S. De vriese, R. Rombaut, J. Van camp and N. H. Lameire, Kidney Int., 2003, 64, 1331–1337 CrossRef CAS PubMed.
- H. G. Windmueller and A. E. Spaeth, J. Biol. Chem., 1978, 253, 69–76 CAS.
- J. M. Rhoads and G. Y. Wu, Amino Acids, 2009, 37, 111–122 CrossRef CAS PubMed.
- W. W. Wang, S. Y. Qiao and D. F. Li, Amino Acids, 2009, 37, 105–110 CrossRef CAS PubMed.
- J. Wang, G. Wu, H. Zhou and F. Wang, Amino Acids, 2009, 37, 177–186 CrossRef CAS PubMed.
- R. A. Kozar, S. G. Schultz, R. J. Bick, B. J. Poindexter, R. Desoignie and F. A. Moore, Shock, 2004, 21, 433–437 CrossRef CAS PubMed.
- C. R. White, G. Datta and S. Giordano, Adv. Exp. Med. Biol., 2017, 982, 407–429 CrossRef PubMed.
- E. A. Fisher, J. E. Feig, B. Hewing, S. L. Hazen and J. D. Smith, Arterioscler., Thromb., Vasc. Biol., 2012, 32(12), 2813–2820 CrossRef CAS PubMed.
- M. Riwanto, L. Rohrer, B. Roschitzki, C. besler, P. Mocharla, M. Mueller, D. Perisa, K. Heinrich, L. Altwegg, A. Von eckardstein, T. F. Lüscher and U. Landmesser, Circulation, 2013, 127, 891–904 CrossRef CAS PubMed.
- J. J. Genest, J. R. Mcnamara, D. N. Salem and E. J. Schaefer, Am. J. Cardiol., 1991, 67, 1185 CrossRef CAS PubMed.
- W. P. Castelli, R. J. Garrison, P. W. Wilson, R. D. Abbott, S. Kalousdian and W. B. kannel, JAMA, J. Am. Med. Assoc., 1986, 256, 2835–2838 CrossRef CAS.
- G. A. Kaysen, J. Renal Nutr., 2011, 21, 120–123 CrossRef CAS PubMed.
- J. Cui, Y. Liu, Y. Hu, J. Tong, A. Li, T. Qu, X. Qin and G. Du, J. Pharm. Biomed. Anal., 2017, 132, 77–86 CrossRef CAS PubMed.
- P. Liao, L. Wei, X. Zhang, X. Li, H. Wu, Y. Wu, J. Ni and F. Pei, Anal. Biochem., 2007, 364(2), 112–121 CrossRef CAS PubMed.
- T. Laeger, C. C. Metges and B. Kuhla, Appetite, 2010, 54, 450–455 CrossRef CAS PubMed.
- A. Chauhan, U. Sharma, N. R. Jagannathan and Y. K. Gupta, Eur. J. Pharmacol., 2015, 757, 28–33 CrossRef CAS PubMed.
- Z. Li and D. E. Vance, J. Lipid Res., 2008, 49, 1187–1194 CrossRef CAS PubMed.
- S. H. Zeisel, Mutat. Res., 2012, 733, 34–38 CrossRef CAS PubMed.
- L. Paoletti, C. Elena, P. Domizi and C. Banchio, IUBMB Life, 2011, 63, 714–720 CAS.
- K. Glunde, M. A. Jacobs and Z. M. Bhujwalla, Expert Rev. Mol. Diagn., 2006, 6, 821–829 CrossRef CAS PubMed.
- Z. N. Wang, W. H. Wilson tang, J. A. Buffa, X. M. Fu, E. B. Britt, R. A. Koeth, A. K. Robert, J. S. Bruce, Y. Y. Fan, Y. P. Wu and L. H. Stanley, Eur. Heart J., 2014, 35(14), 904–910 CrossRef CAS PubMed.
- S. A. Craig, Am. J. Clin. Nutr., 2004, 80, 539–549 CAS.
- K. Van den bergh, M. Vercammen, S. Regenass, R. Derua, P. Vermeersch, P. Pokreisz, A. Ocmant, K. O. De beéck, S. Janssens, E. Waelkens and X. Bossuyt, Clin. Chim. Acta, 2012, 18, 105–108 CrossRef PubMed.
- L. Wei, P. Liao, H. Wu, X. Li, F. Pei, W. Li and Y. Wu, Toxicol. Appl. Pharmacol., 2009, 234, 314–325 CrossRef CAS PubMed.
- M. D. Spencer, T. J. Hamp, R. W. Reid, L. M. Fischer, S. H. Zeisel and A. A. Fodor, Gastroenterology, 2011, 140, 976–986 CrossRef CAS PubMed.
- Z. Wang, E. Klipfell, B. J. Bennett, R. Koeth, B. S. Levison, B. Dugar, A. E. Feldstein, E. B. Britt, X. Fu, Y. M. Chung, Y. Wu, P. Schauer, J. D. Smith, H. Allayee, W. H. Tang, J. A. Di donato, A. J. Lusis and S. L. Hazen, Nature, 2011, 472, 57–63 CrossRef CAS PubMed.
- R. A. Koeth, Z. Wang, B. S. Levison, J. A. Buffa, E. Org, B. T. Sheehy, E. B. Britt, X. Fu, Y. Wu, L. Li, J. D. Smith, J. A. Didonato, J. Chen, H. Li, G. D. Wu, J. D. Lewis, M. Warrier, J. M. Brown, R. M. Krauss, W. H. Tang, F. D. Bushman, A. J. Lusis and S. L. Hazen, Nat. Med., 2013, 19, 576–585 CrossRef CAS PubMed.
- W. H. Tang, Z. Wang, B. S. Levison, R. A. Koeth, E. B. Britt, X. Fu, y. wu and S. L. hazen, N. Engl. J. Med., 2013, 368, 1575–1584 CrossRef CAS PubMed.
- J. R. Baker and S. Chaykin, J. Biol. Chem., 1962, 237, 1309–1313 CAS.
- L. L. Wang, L. Y. Zheng, R. Luo, X. S. Zhao, Z. H. Han, Y. L. Wang and Y. X. Yang, RSC Adv., 2014, 5, 281–290 RSC.
- P. Q. Liao, L. Wei, H. F. Wu, W. S. Li, Y. J. Wu, J. Z. Ni and F. K. Pei, J. Rare Earths, 2009, 27, 280–287 CrossRef.
- M. R. Ogbron, S. Sareen, J. Prychitko, R. Buist and J. Peeling, Am. J. Physiol., 1997, 272, 63–69 Search PubMed.
Footnote |
† Electronic supplementary information (ESI) available. See DOI: 10.1039/c7ra04057a |
|
This journal is © The Royal Society of Chemistry 2017 |
Click here to see how this site uses Cookies. View our privacy policy here.