DOI:
10.1039/C7RA04314G
(Paper)
RSC Adv., 2017,
7, 38479-38489
Identification of potential tubulin polymerization inhibitors by 3D-QSAR, molecular docking and molecular dynamics†
Received
17th April 2017
, Accepted 5th July 2017
First published on 7th August 2017
Abstract
Combretastatin A-4 (CA-4) is one of the most potent tubulin polymerization inhibitors. In this paper, the identification of some new CA-4 analogues as potential tubulin polymerization inhibitors is performed by combination of molecular modeling techniques including 3D-QSAR, molecular docking and molecular dynamics (MD) simulation. The built 3D-QSAR models show significant statistical quality and excellent predictive ability. The square correlation coefficient (r2) and cross-validated squared correlation coefficient (q2) of CoMFA are 0.974 and 0.724; the r2 and q2 of CoMSIA are 0.976 and 0.710, respectively. To further verify the reliability of the models, the inhibitory activity of our synthetic CA-4 analogues to tubulin polymerization was evaluated and predicted. It is interesting to find that the predicted values of the 3D-QSAR models are in good agreement with the experimental values. Several new inhibitors were designed and predicted. Molecular docking elucidates the conformations of compounds and key amino acid residues at the active site of tubulin protein. 30 ns MD simulations were successfully performed to confirm the detailed binding mode and calculate the binding free energies. Some new CA-4 analogues were identified as good potential tubulin polymerization inhibitors.
Introduction
Microtubules, formed by polymerization of αβ-tubulin heterodimers, are essential components of cell structure and are involved in many important cellular processes including mitosis, morphogenesis, intracellular transport and secretion.1 One of the most important roles for antivascular agents is disrupting tubulin–microtubule dynamics, inducing mitosis arrest and leading to cell death.2 So tubulin protein has also been a major target for anticancer drug discovery in the past decades.3,4 Antimitotic agents play an important role in the current anticancer drugs.
There are three distinct small-molecule binding sites in tubulin, i.e. the taxane-binding site, the vinca-binding site, and the colchicine binding site.5 The taxanes and vinca alkaloids had been used successfully in clinical therapies for cancer.6 While colchicine, a potent compound, had been restrained by its toxicity to normal tissues at effective drug concentrations. But the combretastatin family of colchicine binding site agents is progressing through clinical trials for cancer treatment.2,7
Among the combretastatins, CA-4 (Fig. 1), isolated from a South African tree Combretum caffrum,8 strongly inhibits the polymerization of tubulin by binding to the colchicine site. As seen from Fig. 1, the pharmacological features of CA-4 are composed of 3,4,5-trimethoxy substitutions in ring A, 4-methoxy group in ring B and a cis-configuration between rings A and B. CA-4 shows strong cytotoxicity against a variety of cancer cells, including multi-drug resistant cancer cell lines. It has also been demonstrated to exert highly selective effects in proliferating endothelial cells.9 However, CA-4 suffers from poor aqueous solubility, low bioavailability, and short biological half-life.10 To overcome these limitations, a water soluble phosphate prodrug of combretastatin A4 (CA-4P, Fig. 1) had been explored and is currently in clinical trials for the treatment of solid tumors.11
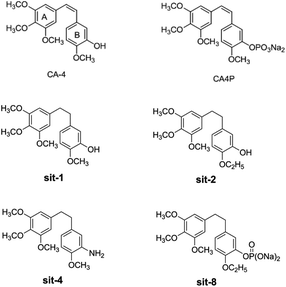 |
| Fig. 1 The structures of CA-4 and CA-4P, and some synthesized CA-4 analogues. | |
Due to its strong activity as a potential anticancer candidate, a number of CA-4 analogues had been designed and synthesized. Hall et al.12 had designed and synthesized eight trifluorinated nitrogen-containing combretastatin analogues and carried out initial biochemical and biological evaluations. The results show that (Z)-2-(4′-methoxy-3′-aminophenyl)-1-(3,4,5-trifluorophenyl)ethane was found to be a potent inhibitor of tubulin assembly (IC50 = 2.9 μM). Pinney et al.13 have prepared two new aryl azide CA-4 analogues, both of which interact with tubulin. These CA-4 aryl azide analogues also inhibit binding of colchicine to tubulin, as does the parent CA-4. Alloatti et al.14 had performed the synthesis and biological evaluation of a series of fluorinated combretastatin analogues by introducing F atoms into the double bond of the cis-stilbene moiety. The results indicate that the position of fluorine atom on the double bond may affect the inhibition of tubulin polymerization and cytotoxic activity. In the course of search for new synthetic antitumor drugs, our great importance had been focused on modifications to B ring of CA-4. For example, we had recently designed and synthesized a series of new CA-4 analogues.15 Results indicate that some compounds, such as sit-1, sit-2, sit-4, and sit-8 (Fig. 1), have potent anti-proliferative activity against multiple cancer cell lines. The success enlightens us to design and synthesize more potent anticancer CA-4 derivatives. On the other hand, as the above mentioned, the introduction of F atom into combretastatin analogues is an effective method to design and synthesize new tubulin inhibitors. In the present work, we want to further modify the structure of CA-4 by introduction of F atoms or fluorine-containing groups and hope to provide a basis for the development of new potent tubulin polymerization inhibitors.
As the above described, to obtain new tubulin inhibitors, great effort had been made in experimental research. However, theoretical studies on the mechanism of these compounds toward tubulin as well as the structural factors responsible for the cytotoxicity remain limited. A molecular modeling study using Comparative Molecular Field Analysis (CoMFA) was undertaken to develop a predictive model for combretastatin analogues.16 The built model can accurately predict the IC50 for tubulin polymerization with an r2 of 0.88 (n = 6) and those of [3H]colchicine displacement with an r2 of 0.80 (n = 7). This study represents the first predictive model for the colchicine binding site over a series of 23 combretastatin analogues. Docking and CoMFA methods were synthetically applied to study a series of 32 CA-4 analogs as inhibitors toward tubulin at the colchicine-binding site.17 In this paper, the appropriate binding orientations and conformations of compounds interacting with tubulin were revealed by the docking study; and a 3D-QSAR model with significant statistical quality and satisfactory predictive ability was established. αβ-Tubulin colchicine site inhibitors (CSIs) from four scaffolds were modeled to better understand their effect on microtubules.18 This study successfully solved the problem how to deal with low-resolution target structures. Zhang et al.19 had performed a 3D-QSAR study of combretastatin A-4 analogs using CoMFA method based on molecular docking. The built QSAR model is statistically significant and has high predictability. The results may facilitate the design of novel tubulin inhibitors with good chemical diversity. Compared with the outstanding previous studies, our work is more comprehensive and convincing. In the present work, the identification of some new CA-4 analogues as potential tubulin polymerization inhibitors is performed by combined 3D-QSAR, molecular docking, and MD simulation techniques. 3D-QSAR involves the techniques such as CoMFA and CoMSIA were used to identify the key structural factors influence on inhibitory activity. Compared with CoMFA, CoMSIA is known as one of the newer 3D-QSAR method. This technique is most commonly used in drug discovery to find the common features that are important in binding to the relevant biological receptor. The reliability of the built models had been further verified by experimental results. Molecular docking had been carried out to study the binding modes of inhibitors using tubulin crystal structures at the colchicine binding site. MD simulation had been performed to calculate the binding free energy via MM/GBSA and MM/PBSA. MD simulation and binding free energy calculation had been proved to be very useful and successful in designing new potent drug molecules. The theoretical results will help our and other research teams to design and synthesize more potent CA-4 analogues.
Materials and methods
Molecular optimization and database alignment
The molecular modeling and 3D-QSAR studies were performed using the molecular modeling package SYBYL-X 2.1 (Tripos, Inc., USA). All structures were minimized by the BFGS20 with the MMFF94 force field and MMFF94 charge.21 The maximum iterations for the minimization were set to 1
000
000. The minimization was terminated when the energy gradient convergence criterion of 0.005 kcal mol−1 A−1 was reached. To obtain a consistent alignment, the database alignment method was performed.
CoMFA and CoMSIA modeling
3D-QSAR models involve the techniques such as CoMFA and CoMSIA to correlate biological activity with 3D structure of compounds. CoMFA values were carried out using steric and electrostatic descriptors. The CoMSIA values were performed using steric, electrostatic, hydrophobic, hydrogen-bond donor, and hydrogen-bond acceptor descriptors. Partial least squares (PLS)22 analysis was used to obtain the 3D-QSAR models. In the PLS analysis, two latent variables based on the CoMFA descriptors were used in the PLS model, and six latent variables based on the CoMSIA descriptors were used in the PLS model. The regression analysis was performed using the leave-one-out (LOO) cross-validation method. All the 3D-QSAR contour map results were graphically represented by using the field type “Stdev*Coeff”.
Models validation
Some statistical parameters were used to analysis the stand or fall of the built models, including the classical square correlation coefficient (R2), F-statistic values (F), and standard error of estimate (SEE). However, these statistical parameters are not sufficient to check the predictive ability of the models. Thus, to further check the predictive ability of the models, internal, external, and overall validations were performed. For the internal validation of model, parameters such as predicted residual sum of squares (PRESS), root mean square error of the estimation (RMSEE), and cross-validated squared correlation coefficient (q2) were used. The PRESS, RMSEE, and q2 values were calculated by using eqn (1)–(3),23 respectively. |
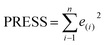 | (1) |
|
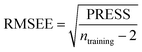 | (2) |
|
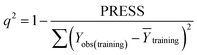 | (3) |
In eqn (1), e(i) indicates the differences between observed and predicted Y-values of training set. In eqn (2), ntraining is the number of training molecules. Yobs(training) and Ȳtraining represent observed and average activity values of training molecules in eqn (3). A high q2 value (q2 > 0.5) is generally considered as a proof of high predictive ability of the model.24,25
To truly estimate model's predictive power, a test set consisting of 10 molecules for external validation was used. The root mean square error of prediction (RMSEP), predicted correlation coefficient (rpred2) and mean absolute error (MAE) were calculated by using eqn (4)–(6),23,26 respectively.
|
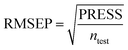 | (4) |
|
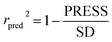 | (5) |
|
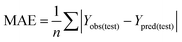 | (6) |
In eqn (4), ntest is the number of test molecules. In eqn (5), SD means the sum of squared deviations between the experimental activities of the compounds in the test set and the mean activity of the training molecules. For a predictive QSAR model rpred2 value should be higher than 0.5,24,25 and the MAE value should satisfy MAE ≤ 0.1 × training set range.26 Golbraikh A and Tropsha A27 had formulated the following criteria to measure the external validation capability of a model: (1) high value of cross-validated R2(q2). (2) Correlation coefficient R between the predicted and observed activities of compounds from an external test set close to 1. At least one (but better both) of the correlation coefficients for regressions through the origin (predicted versus observed activities, or observed versus predicted activities), i.e. R02 or
should be close to R2. (3) At least one slope (k or k′) of regression lines through the origin should be close to 1 (it will correspond to R02 or
that is closer to R2). The correlation coefficient (R), slopes (k, k′), R02 and
were calculated by using eqn 7–11, respectively.
|
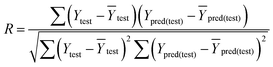 | (7) |
|
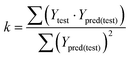 | (8) |
|
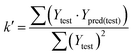 | (9) |
|
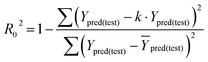 | (10) |
|
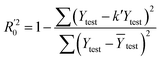 | (11) |
In eqn 7–11, Ytest and Ȳtest represent experimental and average activity values of test molecules, Ypred(test) represents predictive activity values of test molecules.
To further check the predictive ability of the models, we had calculated some novel metrics like rm(LOO)2,
, and Δrm(LOO)2 for internal validation, rm(test)2,
, and Δrm(test)2 for external validation. rm(overall)2,
, and Δrm(overall)2 for overall validation.28 The rm2 and
were calculated by using eqn (12) and (13), respectively.
|
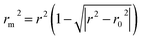 | (12) |
|
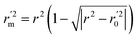 | (13) |
In the above equations, r2, r02, and
are determination coefficients of linear relations between the observed and predicted values of the training or test set compounds. The calculation for r2, r02, and
values is according to the methods provided by Roy's group.28 The values of rm2 and
(calculated by interchanging the axes) should be close.
(average of rm2 and
) value should be greater than 0.5 for an acceptable model. The difference between rm2 and
(Δrm2) should be lower than 0.2.
Molecular docking
Molecular docking was performed using Surflex-Dock module in SYBYL-X 2.0. The crystal structure of tubulin complex was taken from the RCSB Protein Data Bank (PDB ID: 3UT5 (ref. 29)). The complex with colchicine forming by chains C and D of the tubulin heterodimer were considered for docking. Ligands were docked into the colchicine site by an empirical scoring function and a patented search engine. Protein was prepared by using biopolymer module implemented in Sybyl. AMBER7 FF99 charges30 were assigned to protein atoms. Automated docking manner was applied.
Molecular dynamics (MD) simulations
MD simulations were performed by Amber 12.0 package.31 The ff99SB force field was used for the protein, and the general AMBER force field (gaff) was used for ligands. All the MD simulations follow the procedure of minimization, heating, density, equilibration, and production. In the beginning, the entire system suffered from energy minimization in the following two steps. Firstly, the atom position of all solute species was restrained by the force of 100 kcal mol−1 Å−2. Secondly, the whole system was minimized without restraint condition. The entire systems were minimized by 1000 steps of steepest descent method followed by 4000 steps of conjugated gradient method. Then the systems were heated in the NVT ensemble from 0 to 300 K over 20 ps, followed by equilibration in the NPT ensemble at 300 K over 100 ps. In this process, the SHAKE algorithm32 was applied to all bonds involving hydrogen atoms, and the Langevin dynamics was used for temperature control. Finally, a 30 ns production run was carried out by NPT ensemble at 300 K with 1.0 atm. Coordinate trajectories were recorded every 2 ps for the whole MD runs. Visualization and analysis were performed using VMD.33 RMSD and cluster analysis were done for selected compounds using the Xmgrace program.
The conformations obtained from the MD simulations were used for the following binding free energy calculations. The Molecular Mechanics/Poisson–Boltzmann Surface Area (MM/PBSA)34 and the Molecular Mechanics/Generalized Born Surface Area (MM/GBSA)35 methods were used. The corresponding calculation equations are listed as follows:
ΔGbind = ΔH − TΔS ≈ ΔGgas + ΔGsol − TΔS; |
ΔGgas = ΔEele + ΔEvdw; ΔGsol = ΔGPB/GB + ΔGSA |
where Δ
Ggas is the gas-phase interaction energy between protein and ligand, it includes Δ
Eele (electrostatic), and Δ
Evdw (van der Waals) energies. Δ
Gsol is the sum of electrostatic solvation energy (polar contribution), Δ
GPB/GB, and the nonelectrostatic solvation component (nonpolar contribution), Δ
GSA. The change of conformational entropy (−
TΔ
S) upon ligand binding was ignored because of expensive computational cost and similar entropy owing to the ligands binding to the same protein.
36 All energy components were calculated using 50 snapshots extracted from 28 to 30 ns.
Principal descriptors and ADMET properties
The absorption, distribution, metabolism, elimination, and toxicity (ADMET) properties were predicted using ADMET descriptors. The module uses six mathematical models to quantify the prediction characteristics by a set of rules of the threshold ADMET characteristics based on the available drug information. The parameters included in the analysis are human intestinal absorption (HIA), aqueous solubility, blood brain barrier (BBB) penetration, cytochrome P450_2D6 (CYP2D6) enzyme inhibition, hepatotoxicity, plasma-protein binding (PPB), ADMET_Alog
P98 plane, and polar surface area (PSA). Based on the calculation of Alog
P (ADMET_Alog
P98) and 2D polar surface area (PSA_2D), the model was developed using five compounds. ADMET aqueous solubility predicts the solubility of each compound in water at 25 °C. The aqueous solubility has the level 1 (no, very low but possible), 2 (yes, low), 3 (yes, good). ADMET blood brain barrier model predicts blood brain penetration (blood brain barrier, BBB) of a molecule after oral administration. The level 0, 1, 2, 3, and 4 denotes very high, high, medium, low and undefined respectively. The ADMET plasma protein binding model predicts whether the compound may be highly bound to the carrier protein in the blood. ADMET_CYP2D6 binding predicts cytochrome P450_2D6 enzyme inhibition using 2D chemical structure as input. ADMET hepatotoxicity predicts a wide range of potential human hepatotoxicity of structurally diverse compounds.
In vitro tubulin polymerization assay
Pig brain microtubule protein was isolated by three cycles of temperature-dependent assembly/disassembly according to Shelanski et al.37 in 100 mM PIPES (pH 6.5), 1 mM MgSO4, 2 mM EGTA, 1 mM GTP and 1 mM 2-mercaptoethanol. In the first cycle of polymerization, glycerol and phenylmethylsulfonyl fluoride were added to 4 M and 0.2 mM, respectively. Homogeneous tubulin was prepared from microtubule protein by phosphocellulose (P11) chromatography. The purified proteins were stored in aliquots at −70 °C. Tubulin protein was mixed with different concentrations of YHHU0895 in PEM buffer (100 mM PIPES, 1 mM MgCl2, and 1 mM EGTA) containing 1 mM GTP and 5% glycerol. Microtubule polymerization was monitored at 37 °C by light scattering at 340 nm using a SPECTRA MAX 190 (Molecular Device) spectrophotometer. The plateau absorbance values were used for calculations. The extent of polymerization after 20 min incubation was determined. IC50 values were determined graphically by GraphPad Prism v5.0 software.
Results and discussion
Data sets
To construct a global predictive 3D-QSAR model for the inhibition of tubulin polymerization, various CA-4 analogues, with two-atom bridge heads, were collected from a series of different studies.12–14,38–45 The structures and inhibition activities to tubulin polymerization are shown in ESI (Table 1S†). However, a problem was encountered that the data from different sources are hard to compare and analyze. Furthermore, the activity values of CA-4 are not the same in different studies. To eliminate data errors from different sources, a normalization method of processing data was used in the present study. Details are as follows: all data were normalized to an experimental value for combretastatin A-4 from the paper in which the data was taken. The active value of CA-4 is set to 1, corrected IC50 (IC50c) = IC50 (compound)/IC50 (CA-4). If one compound has activity higher than CA-4, its IC50c are less than 1, and vice versa. Then the IC50c values were converted to the negative logarithm of this values (pIC50c value), which were used as dependent variables in the 3D-QSAR models. The CoMFA and CoMSIA descriptors were used as independent variables. Samples were randomly divided into a training set of 50 compounds (83.3%) and a test set of 10 compounds (16.7%), respectively. The training and test set compounds were selected based on the distribution of biological data and structural diversity (Fig. 2 and Table 1S†). The model helps us comprehend the structure–activity relationships of this class of compounds and facilitate the design of novel inhibitors with good activity. In the 3D-QSAR study, alignment rule and biological conformation selection are two important factors to construct reliable model. In this study, compound CA-4 was selected as the template molecule. Fig. 1S (see ESI†) describes the common substructure for the alignment and the molecular alignment of the training set.
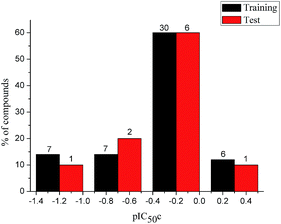 |
| Fig. 2 Distribution of the inhibitory activities of the training set and test set compounds in the 3D-QSAR analysis. | |
CoMFA and CoMSIA statistical results
The statistical results for the final CoMFA and CoMSIA models are summarized in Table 1. The CoMFA model gives a cross-validated q2 of 0.724 with an optimal number of components (ONC) of 6 and square correlation coefficient r2 of 0.974. The standard error of estimate (SEE) value is 0.073 and F-statistic value is 270.623. The corresponding steric and electrostatic field descriptors explain 38.7% and 61.3% of the total variance, respectively. The main contribution in the model is electrostatic field. For the CoMSIA model, five descriptor fields (steric, electrostatic, hydrophobic, hydrogen bond-donor, and hydrogen bond-acceptor) are considered. It gives a cross-validated q2 of 0.710 with an ONC of 7 and square correlation coefficient r2 of 0.976. The standard error of estimate (SEE) value is 0.071 and F-statistic value is 244.906. The steric, electrostatic, hydrophobic, H-bond donor, and acceptor field contributions are 11.5%, 25.3%, 19.7%, 19.3%, and 24.2%, respectively. The main contributions in the model are electrostatic and H-bond acceptor fields. As listed in Table 1, The CoMFA rpred2 value is 0.748 and the CoMSIA rpred2 value is 0.687. MAEtraining (0.050 for CoMFA, 0.047 for CoMSIA) and MAEtest (0.149 for CoMFA, 0.152 for CoMSIA) values are both less than the 0.1 × training set range (0.174). The CoMFA R2 value is 0.873 and the CoMSIA R2 value is 0.837. R02 (0.990 for CoMFA, 0.999 for CoMSIA) and
(0.946 for CoMFA, 0.962 for CoMSIA) values are both close to R2 values. The slopes k (1.056 for CoMFA, 0.997 for CoMSIA) and k′ (0.851 for CoMFA, 0.874 for CoMSIA) values are both close to 1. Therefore, the developed models have good predictive ability and can be used for the design of new compounds with improved activities. Regarding the values of rm2 metrics, rm(test)2,
, rm(LOO)2,
, rm(overall)2 and
are all more than 0.5. Most differences between rm2 and
metrics, Δrm(test)2, Δrm(LOO)2, and Δrm(overall)2, are less than 0.2. This further indicates the good predictive quality of the models. It is very interesting that the values of r2, rm(LOO)2,
,
are equal, and the Δrm(LOO)2 is 0.000 for both CoMFA and CoMISA models. As shown in Table 1, compared with the statistical quality of CoMFA and CoMSIA models, the higher r2, rm(LOO)2,
,
values indicate the internal predictive ability of CoMSIA is a little stronger; but the lower rm2,
,
values for the external prediction and overall prediction indicate its poorer statistical quality. Moreover, the
value (0.390) of CoMSIA is less than 0.5, and the Δrm(test)2 value (0.230) is more than 0.2. Therefore, we think the overall statistical quality of CoMFA is superior to CoMSIA. The experimental pIC50c, predicted pIC50c, and residues between predicted and experimental values are shown in ESI (Table 2S†). The plots of experimental versus predicted pIC50c values for training and test set are shown in Fig. 3a and b for CoMFA and CoMSIA models, respectively. The predicted pIC50c values are close to the experimental values and most of the points were located on the diagonal line, indicating that the predicted pIC50c values are in good agreement with the experimental pIC50c.
Table 1 Statistical results for the CoMFA and CoMSIA models
Statistical parameters |
CoMFA |
CoMSIA |
q2 |
0.724 |
0.710 |
ONC |
6 |
7 |
r2 |
0.974 |
0.976 |
rpred2 |
0.748 |
0.687 |
MAEtraining |
0.050 |
0.047 |
MAEtest |
0.149 |
0.152 |
0.1 × training set range |
0.174 |
SEE |
0.073 |
0.071 |
F |
270.623 |
244.906 |
R |
0.934 |
0.915 |
R2 |
0.873 |
0.837 |
R02 |
0.990 |
0.999 |
 |
0.946 |
0.962 |
k |
1.056 |
0.997 |
k′ |
0.851 |
0.874 |
rm(LOO)2 |
0.974 |
0.976 |
 |
0.974 |
0.976 |
 |
0.974 |
0.976 |
Δrm(LOO)2 |
0.000 |
0.000 |
PRESS(LOO) |
0.226 |
0.210 |
RMSEE |
0.069 |
0.066 |
rm(test)2 |
0.698 |
0.620 |
 |
0.514 |
0.390 |
 |
0.606 |
0.505 |
Δrm(test)2 |
0.184 |
0.230 |
PRESS(test) |
0.289 |
0.359 |
RMSEP |
0.170 |
0.189 |
rm(overall)2 |
0.948 |
0.943 |
 |
0.906 |
0.891 |
Δrm(overall)2 |
0.042 |
0.052 |
PRESS(overall) |
0.515 |
0.568 |
![[thin space (1/6-em)]](https://www.rsc.org/images/entities/char_2009.gif) |
Field contribution (%) |
Steric |
0.387 |
0.115 |
Electrostatic |
0.613 |
0.253 |
H-Acceptor |
— |
0.242 |
H-Donor |
— |
0.193 |
Hydrophobic |
— |
0.197 |
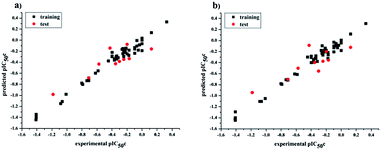 |
| Fig. 3 Plots of experimental versus predicted pIC50c values for all the molecules based on CoMFA (a) and CoMSIA (b) models. | |
Models experimental validation
To further verify the reliability and predictive ability of the models, the inhibitory activity of our previously synthesized four CA-4 analogues, sit-1, sit-2, sit-4 and sit-8, to tubulin polymerization was attempted to evaluate and predict in the present study. The plots of experimental tubulin enzyme inhibition IC50 results are shown in Fig. 4. For comparison, CA-4 was evaluated as the reference compound. The experimental IC50 values for sit-1, sit-2, sit-4, and sit-8 are 9.5 μM, 14.2 μM, 20.3 μM, and 54.5 μM, respectively. The corresponding IC50 value for CA-4 is 2.9 μM. The experimental pIC50c values for sit-1, sit-2, sit-4, and sit-8 are −0.515, −0.690, −0.845, and −1.274, respectively. Among these four CA-4 analogues, sit-1 has the highest inhibitory activity. For comparison, their inhibitory activities had been predicted by the built 3D-QSAR models. The predicted CoMFA pIC50c values for sit-1, sit-2, sit-4, and sit-8 are −0.333, −0.336, −0.421, and −0.097, respectively. The corresponding CoMSIA values are −0.399, −0.399, −0.568, and −0.304, respectively. From the predicted results although there is somewhat discrepancy between observed and predicted values, the activity order for these compounds is basically consistent except for sit-8, and the errors are acceptable on the whole. The discrepancy for sit-8 is comparatively larger. The reason might result from this compound is a CA-4 phosphate, while the models were built based on neutral derivatives.
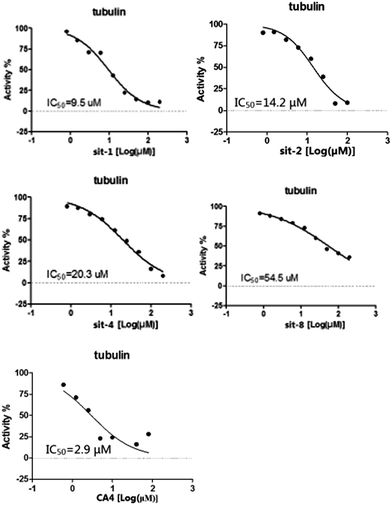 |
| Fig. 4 Plots of experimental tubulin enzyme inhibition IC50 results. | |
CoMFA and CoMSIA contour maps
Fig. 5 and 6 show the steric and electrostatic contour maps of CoMFA and CoMSIA. The default values of field favored and disfavored proportion are 80% and 20% level contribution. It can be observed that the field impact on inhibition activity of tubulin polymerization from the contour maps. As shown in Fig. 5, steric factors influencing on activity are expressed with green and yellow contour maps. The green (bulky substitute favorable) and yellow (bulky substitute unfavorable) contours represent 80 and 20% level contributions, respectively. A green region near the B-ring reveals that bulky groups introduced in these positions are helpful for increasing activity. For example, at the C3 position of B-ring, the inhibitory activity order of some compounds is: 1 (–OH) > 2 (–H), 22 (–NO2) > 20 (–OH), 31 (–NO2) > 30 (–H). At the C4 position of B-ring, the inhibitory activity order is: 48 (–NMe2) > 49 (–OCH3). The appearance of a large yellow contour at C3 position of A-ring indicates that substitution by bulky groups at this location will result in a lower inhibitory activity. For example, at the C3 position of A-ring, the inhibitory activity order is: 33 (–H) > 18 (–F) > 51 (–OCH3).
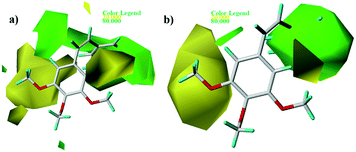 |
| Fig. 5 Contour maps of CoMFA (a) and CoMSIA (b) based on 22. Steric fields: favored (green) and disfavored (yellow). | |
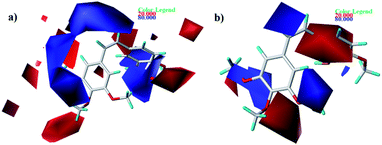 |
| Fig. 6 Contour maps of CoMFA (a) and CoMSIA (b) based on 22. Electrostatic fields: electropositive (blue) and electronegative (red). | |
As shown in Fig. 6, electrostatic factors influencing on activity were expressed with blue (electropositive groups are favorable to the activity) and red (electronegative groups are favorable to the activity) contour maps. Fig. 6a and b show that the B-ring is partly covered by the red colored contour map. For instance, at the C4 position of B-ring, the order of inhibitory activity is: 47 (–Br) > 48 (–NMe2), 35 (–Cl) > 43 (–OCH3).
As shown in Fig. 7a, yellow and white contours highlight areas where hydrophobic properties are favored and disfavored, respectively. The appearance of white contour at C3, C4, and C5 position of B-ring indicates that substitution by hydrophilic groups at these locations will result in a higher inhibitory activity. For example, at the C3 position of B-ring, the order of inhibitory activity is: 31 (–NO2) > 30 (–H), 32 (–OH) > 30 (–H). At the C4 position of B-ring, the order of inhibitory activity is: 41 (–Cl) > 39 (–OCH3). A small white contour at the bridged position indicates that the position is suitable for substitution with hydrophilic group. For example, at the bridged position near A-ring, the order of inhibitory activity is: 38 (–CONH2) > 43 (–CN), 38 (–CONH2) > 39 (–H). In addition, a yellow contour at the C3 position of A-ring indicates that this position is suitable to be substituted with hydrophobic group.
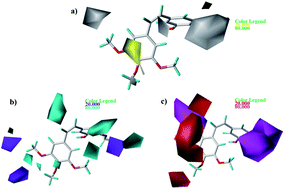 |
| Fig. 7 Contour maps based on 22. (a) Hydrophobic field. (b) Hydrogen bond donor field. (c) Hydrogen bond acceptor field. | |
The CoMSIA contour maps of H-donor field are shown in Fig. 7b, where the cyan and purple areas denote hydrogen bond donor are favorable and unfavorable, respectively. Three pieces of cyan contours near the B-ring indicate that introduction of hydrogen bond donor moieties should improve the biological activity. A small purple at the C4 position of B-ring indicates that substitution by hydrogen bond donor at the location maybe decrease the inhibitory activity. The contour maps of the A-ring are far from the compound, so we don't care them.
As shown in Fig. 7c, the magenta contours represent the position where hydrogen bond acceptor would be beneficial to the inhibitory activity and the red contours show that the presence of acceptor groups in this region should decrease inhibitory activity. A big magenta polyhedron near the B-ring suggests this region is favored for hydrogen bond acceptor interactions. Another magenta contour map is at the bridged position near A-ring, suggesting that acceptor groups may increase activity. For example, the good activity of 22 appears to be partly dependent upon the presence of acceptor group - F substituent at this site. A big red polyhedron covering the C4–C6 positions of A-ring indicates the presence of acceptor groups in this region will decrease inhibitory activity.
Molecular design of new inhibitors
Based on the contour maps obtained from 3D-QSAR models and the known synthesized CA-4 analogues, some new compounds 22a–c were designed by introduction of various substituent groups into B ring of CA-4. The most active 22 was taken as a template to design these new compounds. The structures and the predicted pIC50c values of 22a–c based on CoMFA and CoMSIA models are listed in Table 2. Table 2 shows that the pIC50c values of all designed new molecules are higher than those of CA-4, suggesting their good inhibitory activity.
Table 2 The predicted pIC50c values of representative compounds
No. |
Structure |
Predicted pIC50c |
CoMFA |
CoMSIA |
1(CA-4) |
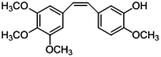 |
−0.189 |
−0.181 |
22a |
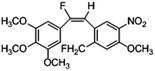 |
0.002 |
0.413 |
22b |
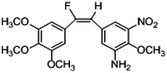 |
0.115 |
0.192 |
22c |
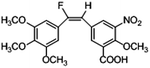 |
0.066 |
0.194 |
MD simulations analysis
To clarify the dynamic interaction patterns between the inhibitors and tubulin, MD simulations were conducted for five representative inhibitors, 1(CA-4), 22, 22a–22c. The binding free energy calculations were performed for each tubulin–inhibitor complex. The system stability and overall convergence of simulations were monitored in terms of root-mean-square deviation (RMSD) of tubulin–inhibitor backbone atoms (C, Cα, N, and O). The average RMSD value of each step versus time is shown in Fig. 2S (see ESI†). It can be seen that all complexes are stable after 10 ns of simulation time, and the fluctuations in the average RMSD values during the whole simulation run are between 1.5 Å and 2.7 Å, indicating the complexes keep the proximate native state. The initial structure of 22c–3UT5 complex and the equilibrium structure after 30 ns MD simulation are superimposed in the Fig. 8. It can be recognized that the equilibrium structure and the initial docked structure are docked into the same binding site. Except for a slight drift and rotation of bonds, there seems to be no significant difference. This confirms the reliability of docking results.
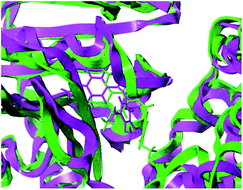 |
| Fig. 8 The superposition of the initial structure (green) and the equilibrium structure (magenta) of 22c–3UT5 complexes. | |
Root mean square fluctuation (RMSF) may reflect the mobility of proteins trajectory MD simulation composite. Fig. 3S (see ESI†) shows a detailed analysis of the relationship between the RMSF and the number of protein residues of the five complexes. It was observed that the five inhibitor–protein complexes had similar fluctuation profiles and RMSF distributions, indicating that these inhibitors have similar binding mode with 3UT5. All the residues labeled in Fig. 3S† are involved in hydrogen bonds, Gln11, Ala12, Asn101, Gly144, Thr145, Val177, Tyr224, Gys241 and Lys254, show rigid behavior with lower RMSF values, indicating that the compounds have stable nature with the complex. Furthermore, the residues Ala12, Lys254 form two hydrogen bonds with 22a, the residues Asn101, Cys241 form two hydrogen bonds with 22b, the residues Gln11, Val177, Tyr224, Cys254 form six hydrogen bonds with 22c, the residues Asn101, Cys241 form two hydrogen bonds with 22, and the residues Asn101, Gly144, Thr145 form four hydrogen bonds with 1(CA-4), respectively. It can be seen from Fig. 3S† that compounds 1(CA-4) and 22c are more stable at the active site because the number of hydrogen bonds formed by CA-4 and 22c is more than that formed by 22, 22a, and 22b, and the binding affinity seems stronger.
Based on the MD simulation, the binding free energy calculations for the five inhibitors of the last 2 ns trajectory were performed and are listed in Table 3. MM/PBSA and MM/GBSA were both used in free energy calculations. Although the two methods, MM/PBSA and MM/GBSA had been used to calculate the ΔGbind in the present study, it is generally think that MM/PBSA performs better than MM/GBSA in calculating binding free energy.36 As listed in Table 3, for all the compounds, the gas phase energy ΔGgas and the solvation free energy ΔGsol is negative and positive, respectively, indicating that the former is favorable and the latter is unfavorable energetic contribution to the total binding free energy. It can also be seen that the most important contribution to the overall binding energy is the van der Waals energy ΔEvdw of ΔGgas. The binding free energy of the inhibitors 1(CA-4), 22, and 22a–22c via MM-GBSA are predicted to be −22.65, −44.57, −43.34, −37.78 and −47.46 kcal mol−1, respectively, and via MM-PBSA was predicted to be −16.83, −30.47, −30.29, −29.71 and −31.09 kcal mol−1, respectively. It can be seen that all binding free energies of 22, 22a–22c are far more negative than that of CA-4, indicating their higher inhibitory activity. From the structure of viewpoint, the reason might partly result from the introduction of F atom into the double bond of the cis-stilbene moiety in 22 and 22a–22c. On the other hand, the substituent at the 3′ position on the B-ring for 22, 22a–22c is nitro, while the corresponding substituent for CA-4 is hydroxy. To further confirm the relative importance of nitro and F for activity, the structure–activity relationship between CA-4 (1) and 5 was analyzed. As shown in Table 2S,† the sole difference in structure between CA-4 (1) and 5 is the substituent at the 3′ position on the B-ring. The substituent for CA-4 and 5 is hydroxyl and nitro, respectively. However, CA-4 (IC50 = 1.2 μm) is distinctly more potent than compound 5 (IC50 = 2.6 μm). Therefore, the presence of nitro at the 3′ position on the B-ring seems not the key factor for the high activity of the new designed molecules. The introduction of F atom into the double bond of the cis-stilbene moiety plays key role, which is consistent with the experimental results of Alloatti et al.14
Table 3 Binding free energy (ΔGbind, kcal mol−1) (last 2 ns) of ligand–tubulin complexes along with the different energy (kcal mol−1) contributions
No. |
ΔEvdw |
ΔEele |
ΔGgas |
ΔGGB |
ΔGSA |
ΔGsol (GB) |
ΔGbind (GB) |
ΔGPB |
ΔGSA |
ΔGsol (PB) |
ΔGbind (PB) |
1(CA-4) |
−35.99 |
−8.90 |
−44.89 |
27.12 |
−4.88 |
22.24 |
−22.65 |
32.19 |
−4.12 |
28.07 |
−16.83 |
22 |
−52.80 |
−12.40 |
−65.19 |
27.52 |
−6.90 |
20.62 |
−44.57 |
39.15 |
−4.43 |
34.72 |
−30.47 |
22a |
−54.24 |
−10.34 |
−64.57 |
28.18 |
−6.94 |
21.23 |
−43.34 |
38.82 |
−4.53 |
34.29 |
−30.29 |
22b |
−48.69 |
−12.55 |
−61.24 |
29.78 |
−6.31 |
23.46 |
−37.78 |
35.94 |
−4.41 |
31.53 |
−29.71 |
22c |
−60.08 |
−11.83 |
−71.90 |
32.04 |
−7.60 |
24.44 |
−47.46 |
45.62 |
−4.81 |
40.81 |
−31.09 |
Compared with the total binding free energy of the template 22, the values of 22b are bigger, the value of 22a is similar, and only the value of 22c is more negative. That is, from the binding free energy of viewpoint, 22c is more active than 22, which has the highest activity among the known reported CA-4 and CA-4 analogues. So the carboxyl at the 5′ position on the B-ring in 22c is also very important for bioactivity. Although the ΔGbind values for 22a and 22b are bigger than that for 22, their ΔGbind values are far smaller than that for CA-4. So the three designed compounds 22a–22c could be used as good potential tubulin polymerization inhibitors.
Docking analysis
To understand the discrepancy of activity between CA-4, 22, and 22a–22c for tubulin polymerization, a series of molecular docking simulations were carried out. Fig. 9 shows the 3D binding modes of the compounds CA-4, 22, 22a, 22b, and 22c at the colchicine binding site of tubulin (PDB ID: 3UT5). As shown in Fig. 9, compound 22, 22c could be perfectly docked into the active site of tubulin. The binding pattern with the active site is very similar to that of CA-4. The trimethoxyphenyl group occupies a same position as the corresponding moiety of CA-4. For compounds 22a and 22b, by contrast, Fig. 9 shows that their conformations seem to be out of the colchicine binding site, which is consistent with the conclusion that 22c has the most negative binding free energy (the strongest binding affinity) in molecular dynamics simulations. Fig. 10 shows the docking results of 22a, 22b, 22c, CA–4, 22 and colchicine crystal structure. As shown in Fig. 10, it can be easily seen that some key amino acids such as Gly10, Gln11, Ala12, Asp69, Asp98, Ala99, Ala100, Asn101, Ser140, Gly143, Gly144, Thr145, Val177, Ser178, Glu183, Tyr224 and Lys254 play key roles in the interaction with compound 22c at the binding site of 3UT5. The initial docking results show that the Gly10, Gln11, Ala12, Asp69, Ala99, Asn101, Ser140, Gly144, Thr145, Val177, Ser178, Glu183, Tyr224 and Lys254 form electrostatic interaction, and the Asp98, Ala100 and Gly143 form van der Waals interaction with compound 22c. Six hydrogen bonds are formed between 22c and the colchicine site. The observed hydrogen bond distances are 2.65 Å (Gln11–OH⋯–NO2), 3.16 Å (Val177–OH⋯O–), 2.74 Å (Tyr224–OH⋯O–), 2.95 Å (Tyr224–OH⋯–OCH3), 2.94 Å (Lys254–HN–H⋯–OCH3) and 2.97 Å (Lys254–HN–H⋯–OCH3). Similarly, 22, 22a–22b and CA-4 were docked into the same protein as shown in Fig. 10, which result in four hydrogen bond interactions for CA-4, two hydrogen bond interactions for 22a, 22b, and 22. The presence of more hydrogen bonds in 22c seems the key factor for its high activity. Docking results could correlate well with the CoMFA and CoMSIA results. For example, docking results show that the nitryl and carboxyl of B-ring in 22c are in favor of the formation of stable hydrogen bond interaction and enhancement activity. As shown in Fig. 7a and b, the appearance of white contour at C3, C4, and C5 positions in B-ring indicates that substitution by hydrophilic groups at these locations will result in a higher inhibitory activity. Three pieces of cyan contours near the B-ring indicate the hydrogen bond donor moieties should improve the biological activity. Herein the nitryl and carboxyl are just hydrophilic and donor groups. Docking results show that the trimethoxy substitutions in ring A interact with many hydrophobic amino acid residues, such as Gly10, Asp98, Asn101, Asp69. A yellow contour at the A-ring shown in Fig. 7a also indicates that this position is suitable to be substituted with hydrophobic group.
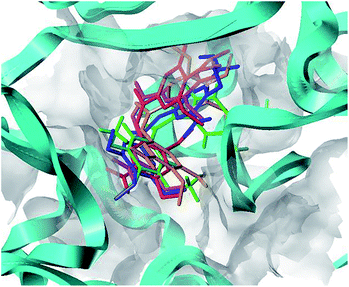 |
| Fig. 9 Proposed binding modes of CA-4 (in green), 22 (in magenta), 22a (in orange), 22b (in red), 22c (in blue) in the colchicine site of tubulin. | |
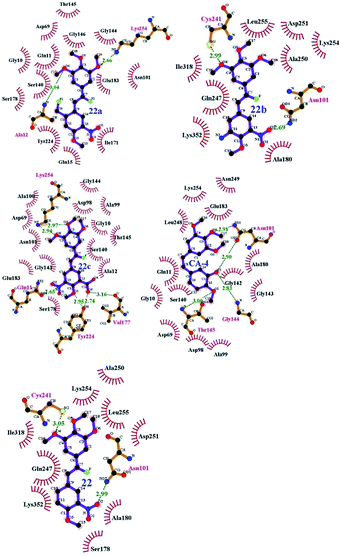 |
| Fig. 10 Compounds 22a–22c, CA-4 and 22 docked into the binding site of 3UT5. The inhibitor is shown as purple stick model. | |
ADMET prediction
Pharmacokinetics is mainly a quantitative study of the process of drugs in the organism (absorption, distribution, metabolism and excretion). The prediction results for some representative compounds (CA-4, 22, 22a–22c) are reported in Table 4. Fig. 4S (see ESI†) shows that all the compounds have an optimum cell permeability (PSA < 140 Å2 and Alog
P98 < 5).46 As shown in Table 4, the absorption levels of CA-4, 22, 22a, 22b are 0, indicating that they can be well absorbed by the cell membrane. The absorption level of 22c is 1, which has moderate absorption. The aqueous solubility plays a dominant role in the bioavailability of the candidate drugs. With the exception of CA-4 having good aqueous solubility (level 3), all other compounds have low aqueous solubility levels (level 2). Compared with CA-4, the designed compounds 22b and 22c have undefined BBB penetration level (level 4) values, 22a has a medium level (level 2). As to CYP2D6 probability, with the exception of CA-4 inhibiting cytochrome P450, all the designed molecules exhibit satisfactory effect, the value is less than 50% (level 0). This indicates that the designed molecules can readily undergo oxidation and hydroxylation in the phase-I metabolism. Further, the prediction of ADMET plasma protein binding property denotes that compound 22c has a binding 90%–95% (level 1) and the other compounds CA-4, 22, 22a, 22b have binding ≥95% (level 2). This suggests that the most potent 22c has good bioavailability and is not likely to be highly bound to carrier proteins in the blood. Finally, the designed molecules have a predicted hepatotoxicity probability of 70%–80%, which is lower than that of CA-4 (91.3%).
Table 4 ADMET prediction of designed compounds
No. |
ADMET absorption level |
ADMET solubility |
ADMET solubility level |
ADMET hepatotoxicity probability |
ADMET CYP2D6 |
ADMET CYP2D6 probability |
ADMET PPB level |
ADMET Alog P98 |
ADMET BBB level |
ADMET PSA_2D |
1(CA-4) |
0 |
−3.93 |
3 |
0.913 |
1 |
0.534 |
2 |
3.508 |
1 |
56.535 |
22 |
0 |
−4.456 |
2 |
0.807 |
0 |
0.455 |
2 |
3.554 |
2 |
78.543 |
22a |
0 |
−4.722 |
2 |
0.807 |
0 |
0.415 |
2 |
3.89 |
2 |
78.543 |
22b |
0 |
−4.601 |
2 |
0.854 |
0 |
0.396 |
2 |
2.808 |
4 |
105.083 |
22c |
1 |
−4.16 |
2 |
0.708 |
0 |
0.386 |
1 |
3.184 |
4 |
116.659 |
Conclusion
The identification of some CA-4 analogues as potential tubulin polymerization inhibitors is performed by a combination of computer-aided drug design techniques, i.e. 3D-QSAR study, molecular docking, MD simulations, and binding free energy calculation. The established 3D-QSAR models show significant statistical quality and excellent predictive ability. To verify the reliability of the models, the inhibitory activity of some synthetic CA-4 analogues was evaluated and predicted. Molecular docking for some typical CA-4 analogues was carried out. Some vital residues (Gln11, Ala12, Asn101, Gly144, Thr145, Val177, Tyr224, Gys241 and Lys254) as well as hydrogen bonds between the representative compounds and residues were located. Based on the 3D-QSAR and docking results, some new potent inhibitors (22a–22c) were designed and predicted. 30 ns of MD simulations had been successfully run for five representative compounds and their binding free energies were calculated. The total binding free energies of all designed compounds are much more negative than that of CA-4 and they are meaningful for experimental synthesis. Both 3D-QSAR study and MD simulation results theoretically indicate that the F atom of the double bond in the cis-stilbene moiety could distinctly improve the inhibitory activity of the CA-4 analogues.
Conflict of interest
The authors declare that there is no financial/commercial conflict of interest.
Acknowledgements
Thanks for financial support given by Shanghai Municipal Natural Science Foundation (No. 15ZR1440400), Collaborative Innovation fund (XTCX2016-14) Shanghai Municipal Education Commission (Plateau Discipline Construction Program). Authors are also thankful to Shandong Huawei Pharmaceutical Com. Ltd. for their help in Tubulin Polymerization Assay.
References
- Y. Han, H. Malak, A. G. Chaudhary, M. D. Chordia, D. G. Kingston and S. Bane, Biochemistry, 1998, 37, 636–644 Search PubMed.
- M. A. Jordan and L. Wilson, Nat. Rev. Cancer, 2004, 4, 253–265 CrossRef CAS PubMed.
- F. Pellegrini and D. R. Budman, Cancer Invest., 2005, 23, 3264–3273 CrossRef.
- G. M. Cragg and D. J. Newman, J. Nat. Prod., 2004, 67, 232–244 CrossRef CAS PubMed.
- R. B. Ravelli, B. Gigant, P. A. Curmi, I. Jourdain, S. Lachkar, A. Sobel and M. Knossow, Nature, 2004, 428, 198–202 CrossRef CAS PubMed.
- G. A. Orr, P. Verdier-Pinard, H. Mcdaid and S. B. Horwitz, Oncogene, 2003, 22, 7280–7295 CrossRef CAS PubMed.
- R. A. Stanton, K. M. Gernert, J. H. Nettles and R. Aneja, Med. Res. Rev., 2011, 31, 443–481 CrossRef CAS PubMed.
- G. Pettit, S. B. Singh, E. Hamel, C. M. Lin, D. S. Alberts and D. Garcia-Kendal, Experientia, 1989, 45, 209–211 CrossRef CAS PubMed.
- N. H. Nam, Curr. Med. Chem., 2003, 10, 1697–1722 CrossRef CAS PubMed.
- K. Ohsumi, R. Nakagawa, Y. Fukuda, T. Hatanaka, Y. Morinaga, Y. Nihei, K. Ohishi, Y. Suga, Y. Akiyama and T. Tsuji, J. Med. Chem., 1998, 41, 3022–3032 CrossRef CAS PubMed.
- G. R. Pettit and M. R. Rhodes, Anti-Cancer Drug Des., 1998, 13, 183–191 CAS.
- J. J. Hall, M. Sriram, T. E. Strecker, J. K. Tidmore, C. J. Jelineka, G. D. Kumar and G. R. Pettit, Bioorg. Med. Chem. Lett., 2008, 18, 5146–5149 CrossRef CAS PubMed.
- K. G. Pinney, M. P. Mejia, V. M. Villalobos, B. E. Rosenquist, G. R. Pettit and P. Verdier-Pinard, et al., Bioorg. Med. Chem., 2000, 8, 2417–2425 CrossRef CAS PubMed.
- D. Alloatti, G. Giannini, W. Cabri, I. Lustrati, M. Marzi and A. Ciacci, et al., J. Med. Chem., 2008, 51, 2708–2721 CrossRef CAS PubMed.
- L. Zhao, J. J. Zhou, X. Y. Huang, L. P. Cheng, W. Pang and Z. P. Kai, et al., Chin. Chem. Lett., 2015, 26, 993–999 CrossRef CAS.
- M. L. Brown, J. M. Rieger and T. L. Macdonald, Bioorg. Med. Chem., 2000, 8, 1433–1441 CrossRef CAS PubMed.
- S. Y. Liao, J. C. Chen, T. F. Miao, Y. Shen and K. G. Zheng, J. Enzyme Inhib. Med. Chem., 2010, 25, 421–429 CrossRef CAS PubMed.
- C. Da, S. L. Mooberry, J. T. Gupton and G. E. Kellogg, J. Med. Chem., 2013, 56, 7382–7395 CrossRef CAS PubMed.
- Y. H. Jin, P. Qi, Z. W. Wang, Q. R. Shen, J. Wang, W. G. Zhang and H. R. Song, Molecules, 2011, 16, 6684–6700 CrossRef CAS PubMed.
- T. A. Halgren, J. Comput. Chem., 1996, 17, 490–519 CrossRef CAS.
- F. M. Ranaivoson, B. Gigant, S. Berritt, M. Joullie and M. Knossow, Acta Crystallogr., Sect. D: Biol. Crystallogr., 2012, 68, 927–934 CAS.
- L. Ståhle and S. Wold, J. Chemom., 1987, 1, 185–196 CrossRef.
- Z. Gagic, K. Nikolic, B. Ivkovic, S. Filipic and D. Agbaba, J. Taiwan Inst. Chem. Eng., 2016, 59, 33–44 CrossRef CAS.
- P. K. Ojha and K. Roy, Chemom. Intell. Lab. Syst., 2011, 109, 146–161 CrossRef CAS.
- A. Tropsha, Mol. Inf., 2010, 29, 476–488 CrossRef CAS PubMed.
- R. Kunal, N. D. Rudra, A. Pravin and B. A. Rahul, Chemom. Intell. Lab. Syst., 2016, 152, 18–33 CrossRef.
- A. Golbraikh and A. Tropsha, J. Mol. Graphics Modell., 2002, 20, 269–276 CrossRef CAS PubMed.
- P. K. Ojha, I. Mitra, R. N. Das and K. Roy, Chemom. Intell. Lab. Syst., 2011, 107, 194–205 CrossRef CAS.
- F. M. Ranaivoson, B. Gigant, S. Berritt, M. Joullie and M. Knossow, Acta Crystallogr., Sect. D: Biol. Crystallogr., 2012, 68, 927–934 CAS.
- U. Essmann, L. Perera, M. L. Berkowitz, T. Darden, H. Lee and L. G. Pedersen, J. Chem. Phys., 1995, 103, 8577–8593 CrossRef CAS.
- D. A. Case, T. Darden, T. E. Cheatham III, C. Simmerling, J. M. Wang and R. E. Duke, et al., AMBER 12, University of California, San Francisco, 2013, 1, p. 3 Search PubMed.
- S. Miyamoto and P. A. Kollman, J. Comput. Chem., 1992, 13, 952–962 CrossRef CAS.
- W. Humphrey, A. Dalke and K. Schulten, J. Mol. Graphics Modell., 1996, 14, 33–38 CrossRef CAS.
- P. A. Kollman, I. Massova, C. Reyes, B. Kuhn, S. Huo and L. Chong, et al., Acc. Chem. Res., 2000, 33, 889–897 CrossRef CAS PubMed.
- N. Homeyer and H. Gohlke, Mol. Inf., 2012, 31, 114–122 CrossRef CAS PubMed.
- T. Hou, J. Wang, Y. Li and W. Wang, J. Chem. Inf. Model., 2011, 51, 69–82 CrossRef CAS PubMed.
- M. L. Shelanski, F. Gaskin and C. R. Cantor, Proc. Natl. Acad. Sci. U. S. A., 1973, 70, 765–768 CrossRef CAS.
- G. R. Pettit, B. Toki, D. L. Herald, P. Verdier-Pinard, M. R. Boyd and E. Hamel, et al., J. Med. Chem., 1998, 41, 1688–1695 CrossRef CAS PubMed.
- G. Pettit, M. R. Rhodes, D. L. Herald, E. Hamel, J. M. Schmidt and R. K. Pettit, J. Med. Chem., 2005, 48, 4087–4099 CrossRef CAS PubMed.
- G. R. Pettit, C. R. Anderson, D. L. Herald, M. K. Jung, D. J. Lee and E. Hamel, et al., J. Med. Chem., 2003, 46, 525–531 CrossRef CAS PubMed.
- R. Siles, J. F. Ackley, M. B. Hadimani, J. J. Hall, B. E. Mugabe and R. Guddneppanavar, et al., J. Nat. Prod., 2008, 71, 313–320 CrossRef CAS PubMed.
- K. A. Monk, R. Siles, M. B. Hadimani, B. E. Mugabe, J. F. Ackley and S. W. Studerus, et al., Bioorg. Med. Chem., 2006, 14, 3231–3244 CrossRef CAS PubMed.
- K. Ohsumi, R. Nakagawa, Y. Fukuda, T. Hatanaka, Y. Morinaga and Y. Nihei, et al., J. Med. Chem., 1998, 41, 3022–3032 CrossRef CAS PubMed.
- M. Cushman, D. Nagarathnam, D. Gopal, A. K. Chakraborti, C. M. Lin and E. Hamel, J. Med. Chem., 1991, 34, 2579–2588 CrossRef CAS PubMed.
- R. Shirai, K. Tokuda, Y. Koiso and S. Iwasaki, Bioorg. Med. Chem. Lett., 1994, 4, 699–704 CrossRef CAS.
- W. J. Egan, K. M. Merz and J. J. Baldwin, J. Med. Chem., 2000, 43, 3867–3877 CrossRef CAS PubMed.
Footnotes |
† Electronic supplementary information (ESI) available. See DOI: 10.1039/c7ra04314g |
‡ These two authors (Tian Chi Wang and Li Ping Cheng) contributed equally to this work and should be considered as co-first authors. |
|
This journal is © The Royal Society of Chemistry 2017 |
Click here to see how this site uses Cookies. View our privacy policy here.