DOI:
10.1039/C7RA05800D
(Paper)
RSC Adv., 2017,
7, 35426-35437
Regulation of fatty acid and flavonoid biosynthesis by miRNAs in Lonicera japonica†
Received
23rd May 2017
, Accepted 10th July 2017
First published on 14th July 2017
Abstract
Lonicera japonica (honeysuckle) is an important herb with various pharmaceutically active secondary metabolites. MicroRNAs (miRNAs) are a class of small endogenous noncoding RNAs (sRNA), which play vital regulatory roles in plant secondary metabolism. Although sufficient data is available on the identification and functions of plant miRNAs, research on miRNA regulation of secondary metabolism in different varieties and producing areas of medical herbs has been scarce. In this study, we identified 28 conserved and 2517 novel miRNAs in honeysuckle using deep-sequencing analysis. The variety-specific regulation of miRNA expression was comparatively analysed by Cluster 3.0 algorithm. A total of 37 miRNAs showed differential expression among the three samples including two varieties of honeysuckle at two locations. The Kyoto Encyclopedia of Genes and Genomes (KEGG) pathway analyses indicated that 19 miRNAs with significantly different expression were putatively involved in the response against various factors and plant development. Additionally, the identification of target transcripts revealed that some of the miRNAs, namely U436803, U977315, U805963, U3938865, and U4351355, could be involved in fatty acid and secondary metabolite biosynthesis. To our knowledge, this is the first report on differentially expressed miRNAs in different varieties of honeysuckle. The results from this study can further facilitate the discovery of functional regulatory miRNAs involved in the secondary metabolism in L. japonica.
1. Introduction
Flos Lonicerae Japonicae (FLJ, Lonicera japonica Thunb., commonly known as honeysuckle) is an important medicinal plant used for its anti-inflammatory and hypolipidemic properties.1 This important herb is used to treat various diseases, such as H1N1 influenza, severe acute respiratory syndromes, pancreatic cancer, and hand-foot-and-mouth disease.2 A new type of anthocyanin-rich L. japonica tea, with its red colour, flavour, and taste has become popular in the market. It is prepared from the buds of L. japonica Thunb. ‘Chinensis’ (rFLJ), a Chinese endemic variety, which is widely planted in different regions of China.3 Both FLJ and rFLJ, synthesise large amounts of secondary metabolites, such as bioflavonoids, quercetin, phenolic acids, and fatty acids, which are believed to be responsible for their various pharmacological activities.4 However, the contents of active compounds differ significantly between the two varieties and in L. japonica plants obtained from different regions, resulting in variations in their medicinal effects.5,6 The contents of chlorogenic acid, luteoloside, quercetin, and isopropyl laurate were found to be higher in the rFLJ buds compared to that in the FLJ buds.3,7 Difference in the contents of the chemicals is an important consideration in using this medicinal plant. Immense efforts have been put into understanding of the regulatory mechanisms responsible for the chemical variations between FLJ and rFLJ. Previous investigations revealed that the key enzymes involved in fatty acid metabolism were regulated differently among the different varieties of honeysuckle, in agreement with the metabolic data.3 Moreover, the expression levels of genes involved in fatty acid metabolism was significantly correlated with the accumulation of the active compounds (chlorogenic acid and caffeic acid) in honeysuckle (R > 0.8).3 Therefore, there is an urgent need to elucidate the regulatory mechanisms of fatty acid metabolism at the molecular level and the biosynthesis of secondary metabolites in both the varieties of L. japonica obtained from different production areas.
MicroRNAs (miRNAs) are one of the most important players in the regulation of gene expression and can inhibit the gene expression via RNA interference.8 MiRNAs are endogenous small RNAs (sRNAs), approximately 19–24 nucleotides (nt) in length.9 They are noncoding, and regulate gene expression in eukaryotes. MiRNAs are transcribed as longer precursors in the nucleus, and are then further processed into their mature forms. A variety of miRNAs has been studied in several plant species.10 A total of 8496 mature plant miRNAs have been reported from viridiplantae, and are publicly available in the miRBase database (http://www.mirbase.org). There is increasing evidence that indicates miRNAs play crucial regulatory roles in plant development, secondary metabolism, and environmental stress resistance.11,12 MiR156, miR172, miR159/319, miR390, and miR399 families are involved in the regulatory network of the flowering time in Arabidopsis thaliana.13 MiRID9 promotes indole-3-acetic acid (IAA) biosynthesis via indole-3-acetaldoxime (IAOx) pathway in A. thaliana, by blocking the indole glucosinolates and camalexin biosynthetic pathways.14 Similarly, miR163 could alter the production of secondary metabolites in Arabidopsis species.15 MiR393-mediated attenuation of auxin signalling modulates the adaptation of roots to drought stress.16 A detailed understanding of these regulatory mechanisms may help in rationally tinkering with the biosynthetic pathways for secondary metabolites. This class of regulators could be a critical tool in determining the secondary metabolism regulation in both the varieties of honeysuckle when responding to multiple environmental factors.
In the present study, deep-sequencing and direct cloning strategies were used to identify and characterise miRNAs from honeysuckle, using three small RNA libraries prepared with the samples of rFLJ from Beijing, rFLJ from Shandong, and FLJ from Shandong. We also determined the variety-specific expression levels of miRNAs and evaluated the link between miRNA regulation, fatty acid metabolism, and secondary metabolism to get a better understanding of their functions in the biosynthesis of various secondary metabolites in L. japonica.
2. Experimental
2.1. Plant materials and RNA extraction
Honeysuckle flowers were randomly collected from 3 year old FLJ and rFLJ plants from Doudian (Beijing, China) and Yate plantations (Linyi, Shandong Province, China) (Table 1). Each sample was divided into five biological replicates, and was immediately frozen in liquid nitrogen. The samples were then stored at −80 °C for RNA extraction. Total RNA was extracted from each sample using a TRIzol kit (Invitrogen, Carlsbad, CA, USA), according to the manufacturer's protocol. The small RNA (sRNA) samples extracted from the five biological replicates were pooled together and named as SO1 (rFLJ from Beijing), SO2 (rFLJ from Linyi), and SO3 (FLJ from Linyi).
Table 1 Collection of honeysuckle samples from China
Varieties |
Sample symbol |
Location |
GPSc information |
rFLJ, Lonicera japonica Thunb. ‘Chinensis’ (Watts). FLJ, L. japonica Thunb. Global positioning system. |
rFLJa |
SO1 |
Doudian, Beijing Province |
39°37′46.3′′ N, 116°01′37.5′′ E, 20.9 M |
SO2 |
Linyi, Shandong Province |
35°19′07.8′′ N, 118°57′36.9′′ E, 273 M |
FLJb |
SO3 |
Linyi, Shandong Province |
35°19′07.8′′ N, 118°57′36.9′′ E, 273 M |
2.2. Library preparation and sequencing
To detect and characterise the expressed miRNAs, equal amounts of sRNAs from the three samples were used for transcriptome library construction. Three small RNA (sRNA) libraries (SO1, SO2, SO3) were constructed following previously reported procedures.17 Briefly, the sRNAs of 18–30 nt were separated and the purified sRNAs were ligated with 5′- and 3′-adaptors by using T4 RNA ligase (TaKaRa). The RNAs were subsequently transcribed to single-stranded cDNAs using Superscript II reverse transcriptase (Promega, Madison, WI, USA). These three small RNA libraries were then sequenced using Illumina HiSeq 2000 (San Diego, CA, USA) and submitted to the Beijing Institute of Genomics (Chinese Academy of Sciences, Beijing, China).
2.3. Deep sequencing analysis
The clean reads from the libraries of SO1, SO2, and SO3 were screened by trimming the 5′- and 3′-adaptors and filtering the low-quality reads. The raw sequences were then categorised to unique reads, and the read counts were recorded. Only 18–30 nt were retained for further analysis. The clean reads were aligned against the annotated non-coding RNA sequences (GenBank, ftp://ftp.ncbi.nlm.nih.gov/genbank/; snoRNABase-human Version 3, https://www.snorna.biotoul.fr/index.php; Rfam 11.0, http://rfam.janelia.org/; Plant-snoRNA-Database, http://bioinf.scri.sari.ac.uk/cgi-bin/plant_snorna/get-sequences)18,19 to detect the other RNA types, including repeat RNAs, rRNAs, scRNAs, snRNAs, snoRNAs, and tRNAs, by BLASTN, with an E-value of 100. Only the reads that completely matched the sequences of the other RNA types were discarded. The remaining sequences were then mapped to the L. japonica reference genome (Yuan et al. unpublished), and the mapped sRNAs were further aligned against miRNA version 21 (http://www.mirbase.org/ftp.shtml) to distinguish the conserved and non-conserved miRNAs. A maximum of two mismatches were allowed for the alignment. For the identification of novel miRNAs, the predicted secondary structures, along with the genome mapping information, were processed using the parameterised plant-specific miRDeep2 for miRNA prediction. The identification of miRNA precursor candidates was performed by an all-to-all alignment, to avoid the inclusion of repeats.
2.4. Target identification with modified 5′-RLM-RACE
To experimentally determine the potential target transcripts of the identified honeysuckle miRNAs, a modified version of the 5′-RNA ligase-mediated rapid amplification of cDNA ends (RLM-RACE) assay was performed.20 The target genes of 18 differentially expressed miRNAs were predicted using microHARVESTER (http://ab.inf.uni-tuebingen.de/software/microHARVESTER/) (Table 2). The RACE PCRs were performed using computationally predicted miRNA target genes (Table 2).
Table 2 Sequences of miRNAs with differential expression in different varieties of Lonicera japonica and primers used for RLM-RACE for the selected target genes
miRNA name |
Mature miRNA sequence |
Targeted gene |
Gene annotation |
Forward primer sequence (5′-3′) |
Reverse primer sequence (5′-3′) |
U436803 |
CAAUACAUGGCUUUGGCC |
FLJ622887 |
Aketoacyl-ACP synthase |
AGATAACACCAGGAAGA |
GGGATGCATGGTCTTACAGT |
FLJ623552 |
NAC domain-containing protein |
GCCCTTCCGTTTCCAA |
GCATCATCATCCCATTCCT |
U2899953 |
CCAUACAUAGCUUUGGCC |
FLJ614363 |
Zinc phosphodiesterase ELAC protein |
CAGGGAAACTTATCAGAGCC |
TTCACGCCGATCACCAT |
FLJ621731 |
Lysine-specific demethylase |
AACGATGTTACGGACGCA |
GACAGCACGGAAAGAAAG |
U1874742 |
CCACCAAAUUUUAUCAAAAAAUU |
FLJ602301 |
Glucose-6-phosphate 1-dehydrogenase |
AATCGTATGGCTCTGGTGC |
GGGATGGCGTTCCTTTCT |
U3938865 |
UGCUAAAUGCAUUUAGCACC |
FLJ575285 |
Calcium-dependent protein kinase |
TTGTTGGAACGGAATGTCA |
GATTTGGATAAGGATGGTAG |
FLJ613583 |
Transcription factor MYB34 |
TATCACGGTCCCACCTAAAG |
TCACAAGCCCAAAAGCAA |
U3767208 |
UAAAUGCAUUUAGCACCAUCUGG |
FLJ613583 |
Transcription factor MYB34 |
TATCACGGTCCCACCTAAAG |
TCACAAGCCCAAAAGCAA |
U4351355 |
UAAAUGCAUUUAGCACUUUCUGA |
FLJ613583 |
Transcription factor MYB34 |
TATCACGGTCCCACCTAAAG |
TCACAAGCCCAAAAGCAA |
U3692923 |
CAGAGGCUCCUCUGAUCGAUC |
FLJ626117 |
Disease resistance protein |
GGCTCTGCCGCTATGC |
ACCCACTAATGACTTTCCCTC |
FLJ617315 |
TMV resistance protein |
CGTTTGTCGTGGTTATCTTGT |
ACGGGTGCTTCTTGTTCTT |
U3528275 |
UUUAUAAUUAUUAGACAUUGCCCC |
FLJ626356 |
F-box/LRR-repeat protein |
AGGATTGAAGTAGCTCAGGG |
TTGACAGTCTTGGGTCGC |
FLJ622616 |
O-Fucosyltransferase family protein isoform |
AAAGGAACCAAAAGACGAG |
CACGGAGCCGAATAGCC |
U3400834 |
UUUCAUAAUCAUUAGACCUUGCCC |
FLJ622616 |
O-Fucosyltransferase family protein isoform |
AAAGGAACCAAAAGACGAG |
CACGGAGCCGAATAGCC |
FLJ626356 |
F-box/LRR-repeat protein |
AGGATTGAAGTAGCTCAGGG |
TTGACAGTCTTGGGTCGC |
U805963 |
UUAGCACCAUAUGUGAAC |
FLJ621761 |
Long chain acyl-CoA synthetase |
GCACAGTTGCCCTAAATCTT |
GTTGCCTGGAGGTCGC |
FLJ419428 |
Zinc finger CCCH domain-containing protein |
ACTGGCAGGGACATTGATAC |
GGCTTGAGGGACTACTTCG |
U4196784 |
CUCUCAGAGGACGAAAGCUGG |
FLJ613223 |
Disease resistance protein |
AAATCACTGTTCTTGGGAGGA |
GTCTGAAACTGAATGGCTGTC |
FLJ625614 |
Disease resistance protein |
TGCTTTAGTGGCGATGGT |
AGGCTTTGTTGGGAATAGG |
U490396 |
UUUUAGUGACGGUUUUUCAUCGC |
FLJ615228 |
3-Ketoacyl-CoA synthase |
TTCATTCGCAGACCAAACA |
CGCTTCGGCAACACCT |
U2743257 |
CAUGAUCGAUCAUGGGUC |
FLJ626161 |
Isoflavone 2′-hydroxylase |
TCTTGTCGTCGTAGTGTCCTC |
CTCTTGGTCTTCAACTTCTTCC |
U1645883 |
UAUAUUUCAAUUUUGUAGUUAACU |
FLJ602301 |
Glucose-6-phosphate 1-dehydrogenase |
AATCGTATGGCTCTGGTGC |
GGGATGGCGTTCCTTTCT |
U892530 |
GGGUUUAACUCAGCAAAC |
FLJ616544 |
Xyloglucan glycosyltransferase |
CAAGAACGCCAAGCAACT |
CAAAACAATCGACACCCAT |
U977315 |
UUAAGAGUCCAGUUUCCUUUUG |
FLJ527490 |
Fatty acid hydroxylase |
CGAGGAATGGGTACACCG |
CAAATGAAGATGCCAACAAC |
U4992168 |
AUGUGCAUGUUACCUUGGUCC |
FLJ617272 |
Dihydroflavonol-4-reductase |
ACAGAGCAGTAAACGGAAC |
GGCATTGAGGGCAAATAAAA |
U5207555 |
UUUUUAUCGGGUGUAUUA |
FLJ257558 |
Glucuronosyltransferase |
TAATAACAAGTCCAAGCCAC |
CCTCGCCTTTCCACCCTA |
FLJ621815 |
Carotenoid cleavage dioxygenase |
CTTTTCTTTCTACATTGTCTCACC |
GTGAGACAATGTAGAAAGAA |
The corresponding putative miRNA target transcripts were firstly translated in silico before RLM-RACE analyses. These were then BLASTed against the protein databases of viridiplantae using BLASTp (http://blast.ncbi.nlm.nih.gov/Blast.cgi/) for standard protein searches. The genes with the highest number of matches were selected for the designing of specific primers for the assay. Subsequently, the gene-specific primers were used for amplification of cDNA ends by using GeneRacer™ kit (Invitrogen/Thermo Fisher Scientific). The PCR products from a positive 5′-RACE reaction were then gel purified, sequenced, and searched against GenBank and miRBase v.21.
2.5. Annotation of miRNA target transcripts
The putative mature miRNA sequences were used to query the honeysuckle transcriptome library for potential target sequences using Patscan with default parameters. The alignment between each miRNA and its putative miRNA target(s) should meet the criteria described in the literature.21 Target genes were then compared with the National Center for Biotechnology Information (NCBI) database and annotated with SwissProt and KEGG database.21,22
2.6. Validation of miRNAs and their expression analysis using qRT-PCR
To confirm the presence of the identified L. japonica miRNA and to assess their expression levels, 18 differentially expressed miRNAs were selected for quantitative real time-PCR (qRT-PCR) analysis (ESI Tables 1 and 2†). The total RNA was isolated using TRIzol reagent, as described previously. The total RNAs were treated with DNase I (TaKaRa Biotechnology Co., Dalian, China) to remove the genomic DNA. Subsequently, 1 μg of DNase-treated RNA from each sample was used to generate single-stranded miRNA cDNA by miRcute miRNA first-strand cDNA Synthesis Kit (TIANGEN, Beijing, China). A combination of the genes, U534122 and U3868172, was used as the internal control in the detection of miRNA expression by qRT-PCR.2 The specific forward primers for the selected miRNAs (ESI Table 3†) were obtained from Sangon Biotech (Shanghai, China).
The differential expression levels of the 18 L. japonica miRNAs were quantified in SO1, SO2, and SO3 by SYBR Green I qRT-PCR assay, using the miRcute miRNA qPCR Detection kit (TIANGEN, Beijing, China), and then by using an ABI 7500 real-time quantitative PCR instrument (Applied Biosystems, Carlsbad, CA, USA). The reaction conditions were as follows: 94 °C for 2 min, followed by 40 cycles at 94 °C for 10 s and 60 °C for 34 s. The melting curve analysis was performed immediately after the completion of qRT-PCR by measuring the fluorescence at temperatures ranging from 55 to 95 °C.
2.7. Validation of miRNA targets and their expression analyses
To validate the predicted targets of L. japonica miRNAs and to assess their expression levels, qRT-PCR assays were performed with 18 miRNA-target transcript pairs. The target transcripts were identified using microHARVESTER, as described previously. The specific PCR primers were designed using the Primer Premier 5.0 software (ESI Table 4†). LJ18S was used as an internal control for the target genes in these experiments.
The expression levels of 20 target genes identified for the 18 L. japonica miRNAs were quantified in SO1, SO2, and SO3 using a SYBR Green I qRT-PCR assay, with miRcute miRNA qPCR Detection kit (TIANGEN, Beijing, China), performed on an ABI 7500 real-time quantitative PCR instrument (Applied Biosystems, Carlsbad, CA, USA). The reaction was performed as described above.
3. Results
3.1. An overview of high-throughput sRNA sequencing
A total of 28 million distinct sequences (sizes ranging from 18 to 30 nt) were generated from three L. japonica sRNA libraries prepared from samples of rFLJ from Beijing (SO1, 11
057
240), rFLJ from Shandong (SO2, 8
673
800), and FLJ from Shandong (SO3, 8
743
421) (Table 3). The sRNAs were further classified into different categories by performing BLAST searches against Rfam. The noncoding RNAs included miRNAs, rRNAs, tRNAs, snoRNAs, snRNAs, and other unannotated RNAs. More categories of sRNAs were present in SO1 than in SO2 and SO3 (Table 4). The sequences were deposited in the NCBI sequence read archive (Accession number SRR3567675). After removal of the structural non-coding RNAs, repeat sequences, and genomic sequences, the remaining 6
845
497 reads were used for miRNA prediction. The number of unique sRNAs ranged from 1.97 to 2.77 million for the individual samples.
Table 3 Data profile of sequenced reads in the three libraries
Sample symbol |
Total reads |
‘N’ reads |
Length < 18 |
Length > 30 |
Clean reads |
SO1 |
12 789 869 |
0 |
469 707 |
1 262 922 |
11 057 240 |
SO2 |
9 144 718 |
0 |
169 631 |
301 287 |
8 673 800 |
SO3 |
9 221 561 |
0 |
132 246 |
345 894 |
8 743 421 |
Table 4 Distribution of small RNAs among different categories in the three libraries of Lonicera japonica buds
Type |
SO1 |
SO2 |
SO3 |
Num. |
Per. |
Num. |
Per. |
Num. |
Per. |
Genome |
6 440 503 |
58.25% |
5 328 411 |
61.43% |
5 660 017 |
64.73% |
rRNA |
1 625 216 |
14.70% |
1 180 662 |
13.61% |
1 056 022 |
12.08% |
scRNA |
0 |
0.00% |
0 |
0.00% |
0 |
0.00% |
snRNA |
2185 |
0.02% |
580 |
0.01% |
1774 |
0.02% |
snoRNA |
784 |
0.01% |
41 |
0.00% |
47 |
0.00% |
tRNA |
208 568 |
1.89% |
53 445 |
0.62% |
41 357 |
0.47% |
Repbase |
13 588 |
0.12% |
6138 |
0.07% |
9626 |
0.11% |
Other |
2 766 396 |
25.02% |
2 104 523 |
24.26% |
1 974 578 |
22.58% |
Clean reads |
11 057 240 |
100.00% |
8 673 800 |
100.00% |
8 743 421 |
100.00% |
The size distribution of the filtered sequence reads indicated the high quality of the data. The largest fraction (35.34%) of sRNAs was 24-nt long in all the samples that were analysed, indicating abundant representation of the endogenous sRNAs (Table 5). The 21-, 22-, and 23-nt sRNAs accounted for 21.22, 16.01, and 9.95% of the total numbers of small RNAs, respectively (Table 5). As expected, more than 82% of the sRNAs was within the range of 21–24 nt. These observations were consistent with those reported in previous studies (Table 5).23
Table 5 Length distribution and frequency percentage of small RNA sequences in the three libraries of Lonicera japonica buds (Mrd: miRNA reads identified by miRDeep2 software with mrd value > −10; miRNA: miRNA reads identified by miRDeep2 software with mrd value > 0)
Length |
Clean reads |
Genome mapped reads |
Mrd |
miRNA |
Num. |
Per. |
Num. |
Per. |
Num. |
Per. |
Num. |
Per. |
18 |
654 849 |
2.30% |
213 910 |
32.67% |
72 378 |
11.05% |
7193 |
1.10% |
19 |
818 688 |
2.88% |
328 938 |
40.18% |
82 426 |
10.07% |
33 751 |
4.12% |
20 |
1 447 178 |
5.08% |
754 327 |
52.12% |
146 206 |
10.10% |
36 803 |
2.54% |
21 |
6 043 099 |
21.22% |
4 340 560 |
71.83% |
1 904 849 |
31.52% |
1 034 012 |
17.11% |
22 |
4 558 588 |
16.01% |
2 720 737 |
59.68% |
212 703 |
4.67% |
76 396 |
1.68% |
23 |
2 831 917 |
9.95% |
1 743 009 |
61.55% |
122 055 |
4.31% |
31 694 |
1.12% |
24 |
10 063 421 |
35.34% |
6 790 646 |
67.48% |
558 327 |
5.55% |
207 417 |
2.06% |
25 |
677 097 |
2.38% |
355 477 |
52.50% |
10 816 |
1.60% |
3341 |
0.49% |
26 |
279 908 |
0.98% |
45 576 |
16.28% |
635 |
0.23% |
292 |
0.10% |
27 |
298 232 |
1.05% |
41 661 |
13.97% |
199 |
0.07% |
70 |
0.02% |
28 |
256 926 |
0.90% |
38 500 |
14.98% |
137 |
0.05% |
86 |
0.03% |
29 |
240 574 |
0.84% |
26 723 |
11.11% |
88 |
0.04% |
56 |
0.02% |
30 |
303 984 |
1.07% |
28 867 |
9.50% |
146 |
0.05% |
95 |
0.03% |
Total |
28 474 461 |
100% |
17 428 931 |
61.21% |
3 110 965 |
10.93% |
1 431 206 |
5.03% |
3.2. Conserved miRNAs
To identify the known miRNAs in the two varieties of L. japonica buds and in the buds from the two different regions, the unique sequences were aligned to the mature sequences of known miRNAs deposited in miRBase. A total of 28 miRNAs were identified in the three libraries, belonging to 13 conserved miRNA families (Table 6). Among these, the miR156 family had nine members, whereas five families, miR162, miR172, miR390, miR398, and miR408, had only one member each. In addition, the secondary structures of these known miRNA precursors were predicted and are shown in Fig. 1. The average minimal folding free energy (MFE) value of these miRNA precursors was 2.08 kcal mol−1, and the length of these precursors ranged from 18 to 21 nt. The expression levels of the 13 identified known miRNA families showed a broad range. The expression levels of several miRNA families, such as miR166, miR160, miR162, and miR168, were extremely high. miR166 (1
511
444 reads) was most abundant in the three libraries, accounting for 95.72% of all the known miRNA reads. On the contrary, miR398, miR408, and miR395 families had less than 500 reads in the three libraries. Moreover, different members of each miRNA family displayed drastically different expression levels (ESI Table 5†).
Table 6 Conserved miRNA families identified in Lonicera japonica buds and their abundance
Family |
Members |
Count |
Total reads |
Ratio |
SO1 |
SO2 |
SO3 |
SO1/SO2 |
SO2/SO3 |
miR156 |
9 |
3007 |
2696 |
1353 |
7479 |
1.115 |
1.993 |
miR157 |
2 |
1944 |
1016 |
484 |
4223 |
1.913 |
2.099 |
miR160 |
6 |
637 |
801 |
1542 |
30 030 |
0.795 |
0.519 |
miR162 |
1 |
3047 |
4054 |
3790 |
10 898 |
0.752 |
1.07 |
miR164 |
2 |
883 |
479 |
482 |
1907 |
1.843 |
0.994 |
miR166 |
2 |
337 584 |
688 401 |
485 444 |
1 511 444 |
0.49 |
1.418 |
miR167 |
3 |
1192 |
1360 |
1309 |
4974 |
0.876 |
1.039 |
miR171 |
7 |
1193 |
875 |
686 |
2804 |
1.363 |
1.257 |
miR172 |
1 |
145 |
175 |
414 |
735 |
0.829 |
0.423 |
miR390 |
1 |
2294 |
1140 |
758 |
4193 |
2.012 |
1.504 |
miR395 |
2 |
212 |
10 |
6 |
236 |
21.2 |
1.667 |
miR398 |
1 |
10 |
5 |
6 |
29 |
2 |
0.833 |
miR408 |
1 |
22 |
51 |
50 |
149 |
0.431 |
1.02 |
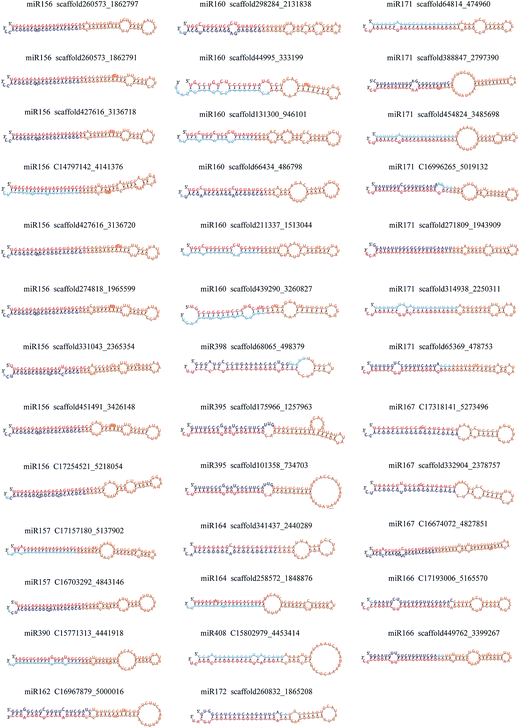 |
| Fig. 1 Secondary structures of the predicted miRNA precursors in Lonicera japonica. | |
3.3. Novel miRNAs
A total of 2517 potential novel miRNAs were detected in L. japonica; these miRNAs met our criteria and had no matches to any previously known plant miRNAs. The list of the novel miRNAs of L. japonica is shown in ESI Table 6.† The secondary structures of these novel miRNA precursors were predicted and identified, and had an average MFE value of −46.09 kcal mol−1. The average length of these miRNA precursors was 134 nt, and ranged from 72 to 340 nt. As in the case of the conserved miRNAs, the majority of the nucleotides in the mature sequences of the novel miRNAs contained uracil (Fig. 2A and ESI Table 6†).
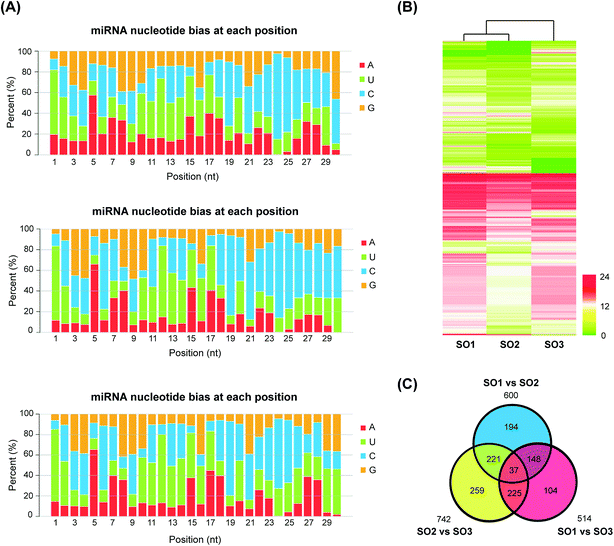 |
| Fig. 2 Transcriptome analysis of different honeysuckle varieties. (A) Nucleotide bias in miRNAs at each position in the three honeysuckle databases. (B) Heatmap analysis of miRNA expression in FLJ and rFLJ from Beijing and Shandong. (C) Venn diagram representing the number of common and different miRNAs in three samples. SO1, rFLJ from Beijing; SO2, rFLJ from Shandong; SO3, FLJ from Shandong. | |
3.4. Variety-specific miRNA expression
To investigate the differences in the expression of miRNAs in the two varieties, the expression levels of all the identified homologous/conserved miRNAs in the three samples were compared using the Cluster 3.0 algorithm (Fig. 2B). The expression clustering with different samples showed the expression characteristics of rFLJ from the different regions and rFLJ/FLJ from the same region. The libraries of rFLJ form the different regions (SO1 and SO2) were closer than those of rFLJ and FLJ from same regions (SO1 and SO3).
The distribution of all the differentially expressed miRNA candidates identified from the three libraries is displayed in the Venn diagram (Fig. 2C). A total of 600, 514, and 742 differentially expressed miRNAs were detected in SO1 vs. SO2, SO1 vs. SO3, and SO2 vs. SO3 comparisons, respectively (ESI Table 7†). Additionally, 185 differentially expressed miRNAs were common in the SO1 vs. SO2 and SO1 vs. SO3 comparisons. Similarly, 258 miRNAs were common between the SO2 vs. SO3 and SO1 vs. SO2 comparisons and 262 miRNAs were common between the SO2 vs. SO3 and SO1 vs. SO3 comparisons. Among these, 37 differentially expressed miRNA candidates were common in all the libraries, which might effectively distinguish the three varieties of L. japonica.
3.5. Prediction and annotation of target transcripts
A total of 731 mRNA transcripts putatively targeted by 1021 L. japonica miRNAs (including 22 conserved novel miRNAs and 999 non-conserved novel miRNAs) were predicted. To evaluate the potential functions of these miRNA target genes, the gene ontology (GO) analysis was performed. All the target sequences were successfully classified into three GOs using blast2go program; these included cellular components, molecular functions, and biological process (Fig. 3). The main terms were “cell part” (GO: 0044464), “cell” (GO: 0005623), and “organelle” (GO: 0043226) in the cellular components category. For their molecular functions, the “catalytic activity” (GO: 0003824) and “binding” (GO: 0005488) were the most abundant subcategories. The predominant terms implicated in the biological processes were “metabolic process” (GO: 0008152) and “cellular process” (GO: 0009987).
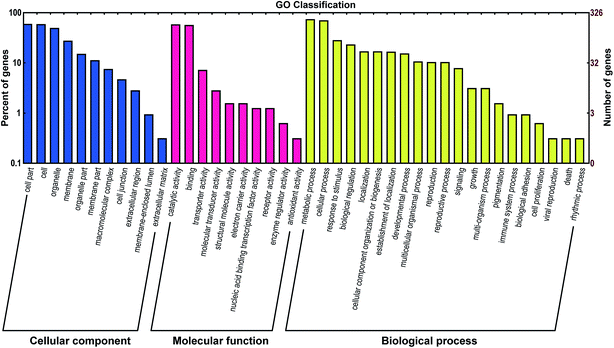 |
| Fig. 3 Gene ontology (GO) classification of potential target genes for differentially expressed miRNAs. Blue, red, and yellow represent three GOs, cellular component, molecular function, and biological progress, respectively. | |
For further evaluation of the transcriptome completeness and the annotation effectiveness, we searched the annotated sequences assigned to the clusters of orthologous group (COG) classifications. Overall, 326 sequences were assigned to the COG classifications, and COG-annotated putative proteins were functionally classified into at least 22 molecular families (Fig. 4). The cluster for general function prediction was largest, and was followed in the descending order by translation, replication/recombination/repair, and signal transduction mechanisms.
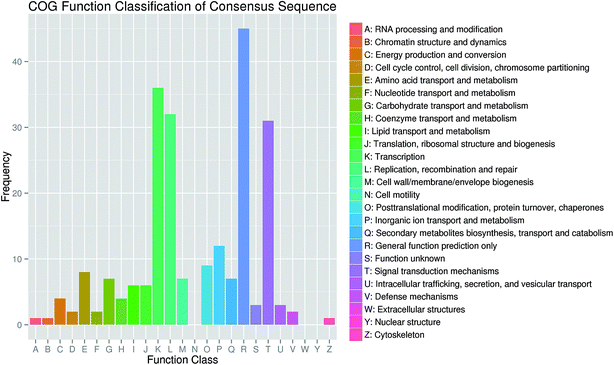 |
| Fig. 4 Clusters of orthologous groups (COG) functional classification of consensus sequences in honeysuckle. | |
3.6. Experimental identification of miRNA targets
To identify the target transcripts for the selected miRNAs, the experiments of 5′-RLM-RACE were performed. Ten of the experimentally confirmed transcripts included the miRNA cleavage sites (ESI Table 8†). MiRNA U436803 cleaved two target genes (FLJ623552 and FLJ622887). Similarly, two target transcripts (FLJ626117 and FLJ617315) were cleaved by miRNA U3692923. Each of the remaining validated miRNAs cleaved one target gene. In the 5′-end sequencing most of the cleavage sites that were identified were located at the 10th and 11th positions upstream of the miRNA-binding site (Fig. 5).
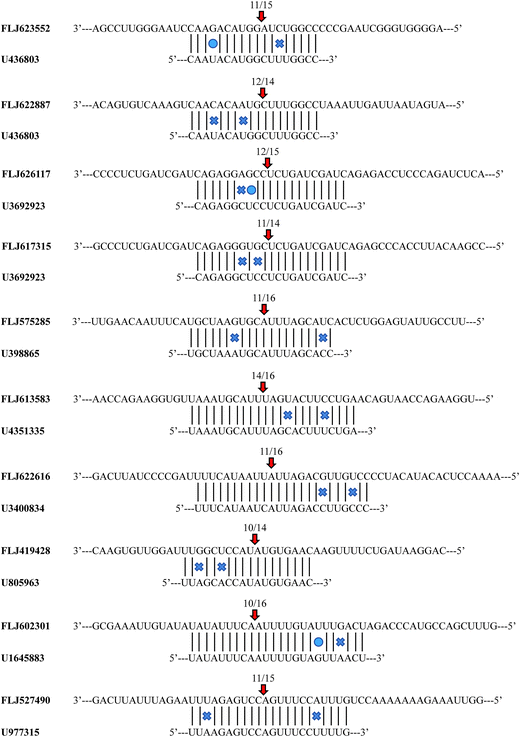 |
| Fig. 5 Cleavage sites of the miRNAs identified by a modified 5′-RNA-ligase mediate rapid amplification of cDNA ends procedure. | |
3.7. Expression of miRNA and target mRNA
Eighteen expressed miRNAs and their target transcripts were selected for verification by the real-time quantitative PCR. As expected, the miRNA expression in the different varieties was negatively correlated with the expression levels of the target transcripts. Additionally, the data obtained from the Venn diagram analysis were also validated by qRT-PCR. In both analyses, the expression levels of U436803, U1874742, U3692923, U3400834, U1645883, U892530, and U977315 (including those of the confirmed target transcripts of U436803, U3692923, U3400834, U1645883, U977315) were higher in rFLJ from Shandong compared to those in the other samples; U3767208, U805963, U4992168, and U5207555 (including those of the confirmed target transcripts of U805963 and U4992168) were highly expressed in rFLJ from Beijing; the target transcripts identified for U3938865 and U4351355 were highly expressed in FLJ from Shandong. As expected, the miRNA expression levels in the different varieties were negatively correlated with those of target transcripts, especially for the confirmed targets of U436803, U3400834, U977315, U3938865, U4351355, and U805963 (Fig. 6 and Table 2). Thus, U436803, U977315, U805963, U3938865, and U4351355 were differentially expressed in those samples, whereas an opposite pattern was detected for their target transcripts, which were all related to the fatty acid and secondary metabolism.
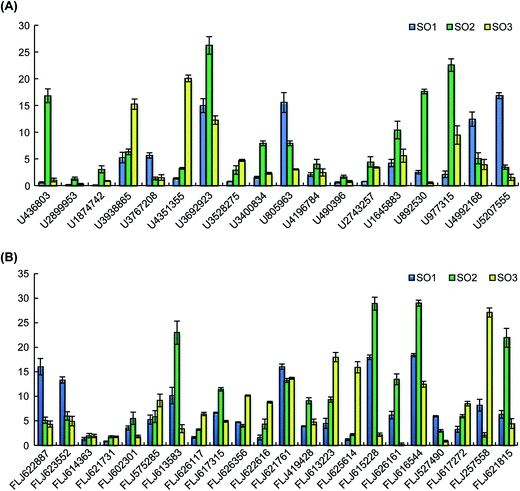 |
| Fig. 6 Expression levels of selected miRNAs and target transcripts in FLJ and rFLJ from Beijing and Shandong as assessed by quantitative real time polymerase chain reaction (qRT-PCR). (A) qRT-PCR results of the selected miRNAs; (B) qRT-PCR results of the target transcripts. SO1, rFLJ from Beijing; SO2, rFLJ from Shandong; SO3, FLJ from Shandong. | |
3.8. miRNA-regulated genes involved in fatty acid and secondary metabolism
The expression levels of U3938865 and U4351355 were measured, and their presence in the samples was further validated by qRT-PCR. The expression levels of these miRNAs were higher in FLJ than in rFLJ. As expected, the expression of MYB34 (FLJ613583, the common target gene of the above two miRNAs) was repressed more in FLJ compared to that in rFLJ (Fig. 6). MYB34 can positively regulate the biosynthesis of glucosinolates (GSLs) and camalexin and can be triggered by methyl jasmonate (MeJA) and salicylic acid (SA),24,25 which might also play a key role in fatty acid and secondary metabolite biosynthesis in honeysuckle. This might be the reason for the differences in the contents of active compounds in the rFLJ and FLJ buds.
Moreover, the expression levels of the target genes of U4992168 and U2743257 (FLJ617272 and FLJ626161), involved in flavonoid biosynthesis, were higher in honeysuckle from Beijing than in the samples from Shandong (Fig. 6A). Conversely, as expected, the target gene was expressed more in Shandong samples, which might be the reason for the differences in the contents of the active compounds (e.g., luteoloside and quercetin) in the samples from the different regions (Fig. 6B). Usually, Shandong Linyi is considered to be an important region for the production of honeysuckle. Thus, U4992168 and U2743257 can be used as potential biomarkers for predicting the good quality of honeysuckle samples.
4. Discussion
MicroRNAs play important roles in the regulation of many plant biological processes, and their regulatory roles have been revealed. The functions of miRNAs, such as miR163, miR393, pso-miR13, pso-miR2161, and pso-miR408, in plant secondary metabolism have been described in Arabidopsis and opium poppy.15,16,20
Previous studies have revealed that the biosynthesis of fatty acids and flavonoids might depend on the interaction between the two pathways in FLJ and rFLJ.3 However, the possible relationship between these secondary biosynthesis pathways and miRNA regulation is still unclear in honeysuckle. Although numerous miRNAs have been extensively investigated in different plants recently, only limited information is available on the miRNAs and their target genes in L. japonica.26 Moreover, there are no reports on the miRNAs present in the different varieties of honeysuckle. Thus, to better characterise the differentially expressed miRNAs among the different varieties of honeysuckle, a comprehensive experimental approach was taken in this work. In addition, qRT-PCR was used to determine the variety-specific miRNA expression profiles. Furthermore, the target transcripts were analysed using both bioinformatics and experimental methods.
By sequencing of sRNAs, 28 conserved and 2517 novel miRNAs were identified in honeysuckle. A broad variation in miRNA expression could be observed. Some of the conserved miRNAs (e.g. miR166, miR160, miR162, miR168) were shown to be the most abundant, consistent with results of the study by Xia et al.26 In our study, miR395 was identified as one of the most highly expressed miRNAs in Beijing rFLJ. Our results confirmed that the plant miRNA expression levels vary across the varieties. Moreover, the miRNA expression was also found to be different in the samples obtained from the different regions. For comparative analysis of the miRNA profiles, we used two varieties from two regions. Overall, 37 differentially expressed miRNAs were identified from the three libraries. The qRT-PCR results revealed that the expression levels of U436803, U977315, and U805963 were higher in rFLJ compared to those in FLJ. The modified 5′-RLM-RACE experiments revealed that the targets of the above-mentioned miRNAs (U436803, U977315, and U805963) were long-chain acyl-CoA synthetase (LACS), acyl carrier protein (ACP), and fatty acid hydroxylase (FAH) genes, all of which are directly related with fatty acid metabolism (Fig. 7). LACS could be activated during both the synthesis of cellular lipids and their degradation through β-oxidation and also participates in the cutin pathway.27–29 ACP is involved in the pathway for fatty acid synthesis and export.30,31 FAH could catalyse the synthesis of hydroxylated fatty acid moieties, absence of which could increase the salicylate levels and resistance towards obligate biotrophic fungal pathogens.32 In contrast, the expression levels of U3938865 and U4351355 were higher in FLJ compared to those in rFLJ. MYB34, the common target gene of these two miRNAs, can positively regulate the biosynthesis of glucosinolates and camalexin, which could be triggered by MeJA and SA (Fig. 7).24,25 Therefore, all the differentially expressed miRNAs were directly or indirectly related to the fatty acid metabolism, suggesting that the fatty acid biosynthesis was quite different between FLJ and rFLJ.
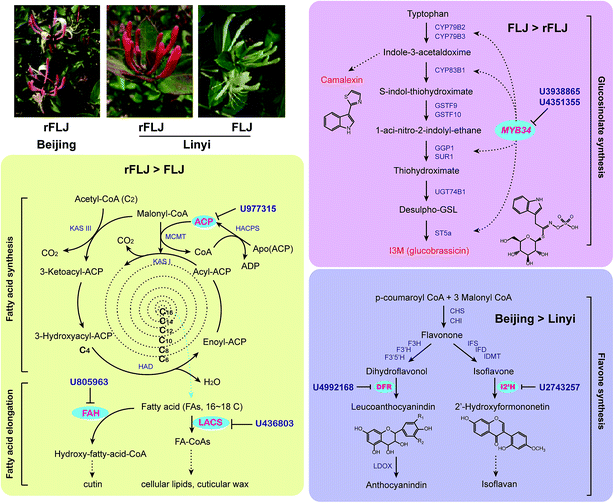 |
| Fig. 7 Differentially expressed miRNAs predicted for the target transcripts encoding enzymes involved in fatty acid, glucosinolate, and flavone metabolism. ACP, acyl carrier protein; CHI, chalcone isomerase; CHS, chalcone synthase; DFR, dihydroflavonol 4-reductase; FAH, fatty acid hydroxylase; F3H, flavanone 3-hydroxylase; F3′H, flavonoid 3′-hydroxylase; F3′5′H, flavonoid 3′5′-hydroxylase; GSL, glucosinolates; HACPS, holo-ACP synthase; HAD, hydroxyacyl-ACP dehydratase; IFD, 2-hydroxyisoflavanone dehydratase; IFS, 2-hydroxyisoflavanone synthase; I2′H, isoflavone 2′-hydroxylase; IOMT, isoflavonoid-O-methyltransferase; KAS, ketoacyl-ACP synthase; LACS, long-chain acyl-CoA synthetase; LDOX, leucoanthocyanidin dioxygenase; MCMT, malonyl-CoA. | |
In contrast, the expression of U4992168 and U2743257 target genes involved in flavonoid biosynthesis was higher in Beijing honeysuckle than in the Shandong samples (Fig. 7). Thus, miRNAs play a key role in secondary metabolite biosynthesis in honeysuckle, which might be the reason for the difference in the contents of active compounds in the samples obtained from the different regions.
In general, the difference in the miRNAs involved in the regulation of fatty acid and flavonoid biosynthesis was found to be higher between the two honeysuckle varieties compared to the difference among the honeysuckle varieties. Moreover, some honeysuckle-based miRNAs might also have certain therapeutic effects in animals.33,34 In this study, the selected miRNAs were mostly related to fatty acid and secondary metabolism in the plants. Whether these miRNAs have similar effects in animals, such as the hypolipidemic effect, is an interesting question that warrants further investigation.
Author contributions
YY and HL designed the study. LJ and YY wrote the main manuscript text and analysed the data of high-throughput sRNA sequencing. LJ prepared the figures, tables and supplemental materials. ZY and ZJ collected samples. ZF and CT contributed to miRNA library construction and sequencing. WY and JC conducted the experiments associated with 5′-RLM-RACE and qRT-PCR. All authors read and approved the final manuscript.
Conflict of interest
The authors declare no conflicts of interest.
Acknowledgements
This work was supported by the National Natural Science Foundation of China (81373959, 81325023) and the Fundamental Research Funds for the Central public welfare research institutes (ZZ10-008).
References
- N. Vukovic, M. Kacaniova, L. Hleba and S. Sukdolak, Nat. Prod. Commun., 2012, 7, 641–644 CAS.
- Y. Wang, J. Liu, X. Wang, S. Liu, G. Wang, J. Zhou, Y. Yuan, T. Chen, C. Jiang, L. Zha and L. Huang, Front. Plant Sci., 2016, 7, 1101 Search PubMed.
- Y. Yuan, L. Song, M. Li, G. Liu, Y. Chu, L. Ma, Y. Zhou, X. Wang, W. Gao, S. Qin, J. Yu, X. Wang and L. Huang, BMC Genomics, 2012, 13, 195 CrossRef CAS PubMed.
- Z. Shi, Z. Liu, C. Liu, M. Wu, H. Su, X. Ma, Y. Zang, J. Wang, Y. Zhao and X. Xiao, Front. Pharmacol., 2016, 7, 12 CrossRef PubMed.
- W. Li, Z. Cheng, Y. Wang and H. Qu, J. Pharm. Biomed. Sci., 2013, 72, 33–39 CrossRef CAS PubMed.
- T. Tan, C. J. S. Lai, H. O. Yang, M. Z. He and Y. Feng, J. Pharm. Biomed. Anal., 2016, 120, 134–141 CrossRef CAS PubMed.
- S. Y. Y. Qin, G. Hu, X. Chen and X. Li, Chin. J. Exp. Tradit. Med. Formulae, 2010, 16, 2 Search PubMed.
- D. P. Bartel, Cell, 2004, 116, 281–297 CrossRef CAS PubMed.
- A. Barakat, K. Wall, J. Leebens-Mack, Y. J. Wang, J. E. Carlson and C. W. dePamphilis, Plant J., 2007, 51, 991–1003 CrossRef CAS PubMed.
- V. Eldem, S. Okay and T. Unver, Turk. J. Agric. For., 2013, 37, 1–21 CAS.
- S. Lu, Y. H. Sun and V. L. Chiang, Plant J., 2008, 55, 131–151 CrossRef CAS PubMed.
- D. P. Bartel, Cell, 2009, 136, 215–233 CrossRef CAS PubMed.
- E. Spanudakis and S. Jackson, J. Exp. Bot., 2014, 65, 365–380 CrossRef CAS PubMed.
- W. Kong, Y. Li, M. Zhang, F. Jin and J. Li, Plant Cell Physiol., 2015, 56, 715–726 CrossRef CAS PubMed.
- D. W. Ng, C. Zhang, M. Miller, G. Palmer, M. Whitelev, D. Tholl and Z. J. Chen, Plant Cell, 2011, 23, 1729–1740 CrossRef CAS PubMed.
- H. Chen, Z. Li and L. Xiong, FEBS Lett., 2012, 586, 1742–1747 CrossRef CAS PubMed.
- L. Xu, Y. Wang, L. Zhai, Y. Xu, L. Wang, X. Zhu, Y. Gong, R. Yu, C. Limera and L. Liu, J. Exp. Bot., 2013, 64, 4271–4287 CrossRef CAS PubMed.
- P. P. Gardner, J. Daub, J. Tate, B. L. Moore, I. H. Osuch, S. Griffiths-Jones, R. D. Finn, E. P. Nawrocki, D. L. Kolbe, S. R. Eddy and A. Bateman, Nucleic Acids Res., 2011, 39, D141–D145 CrossRef CAS PubMed.
- A. Kozomara and S. Griffiths-Jones, Nucleic Acids Res., 2011, 39, D152–D157 CrossRef CAS PubMed.
- H. Boke, E. Ozhuner, M. Turktas, I. Parmaksiz, S. Ozcan and T. Unver, Plant Biotechnol. J., 2015, 13, 409–420 CrossRef CAS PubMed.
- Z. H. Gao, Y. Yang, Z. Zhang, W. T. Zhao, H. Meng, Y. Jin, J. Q. Huang, Y. H. Xu, L. Z. Zhao, J. Liu and J. H. Wei, Int. J. Biol. Sci., 2014, 10, 500–510 CrossRef PubMed.
- Y. Moriya, M. Itoh, S. Okuda, A. C. Yoshizawa and M. Kanehisa, Nucleic Acids Res., 2007, 35, W182–W185 CrossRef PubMed.
- M. Jain, W. Chevala and R. Garg, J. Exp. Bot., 2014, 65, 5945–5958 CrossRef CAS PubMed.
- H. Frerigmann, E. Glawischnig and T. Gigolashvili, Front. Plant Sci., 2015, 6, 654 Search PubMed.
- G. E. Yi, A. H. Robin, K. Yang, J. I. Park, B. H. Hwang and I. S. Nou, Molecules, 2016, 21, E1417 CrossRef PubMed.
- H. Xia, L. Zhang, G. Wu, C. Fu, Y. Long, J. Xiang, J. Gan, Y. Zhou, L. Yu and M. Li, PLoS One, 2016, 11, e0164140 Search PubMed.
- S. Lü, T. Song, D. K. Kosma, E. P. Parsons, O. Rowland and M. A. Jenks, Plant J., 2009, 59, 553–564 CrossRef PubMed.
- D. Jessen, C. Roth, M. Wiermer and M. Fulda, Plant Physiol., 2015, 167, 351–366 CrossRef CAS PubMed.
- B. Jia, Y. Song, M. Wu, B. Lin, K. Xiao, Z. Hu and Y. Huang, Biotechnol. Biofuels, 2016, 9, 184 CrossRef PubMed.
- X. Guan, Y. Okazaki, A. Lithio, L. Li, X. Zhao, H. Jin, D. Nettleton, K. Saito and B. J. Nikolau, Plant Physiol., 2017, 173, 2010–2028 CrossRef CAS PubMed.
- Z. W. Ye, J. Xu, J. Shi, D. Zhang and M. L. Chye, Plant Mol. Biol., 2017, 93, 209–225 CrossRef CAS PubMed.
- S. König, K. Feussner, M. Schwarz, A. Kaever, T. Iven, M. Landesfeind, P. Ternes, P. Karlovsky, V. Lipka and I. Feussner, New Phytol., 2012, 196, 1086–1097 CrossRef PubMed.
- J. Yang, L. M. Farmer, A. A. Agyekum and K. D. Hirschi, Cell Res., 2015, 25, 517–520 CrossRef CAS PubMed.
- Z. Zhou, X. Li, J. Liu, L. Dong, Q. Chen, J. Liu, H. Kong, Q. Zhang, X. Qi, D. Hou, L. Zhang, G. Zhang, Y. Liu, Y. Zhang, J. Li, J. Wang, X. Chen, H. Wang, J. Zhang, H. Chen, K. Zen and C. Y. Zhang, Cell Res., 2015, 25, 39–49 CrossRef CAS PubMed.
Footnote |
† Electronic supplementary information (ESI) available. See DOI: 10.1039/c7ra05800d |
|
This journal is © The Royal Society of Chemistry 2017 |
Click here to see how this site uses Cookies. View our privacy policy here.