DOI:
10.1039/C7RA08030A
(Paper)
RSC Adv., 2017,
7, 48102-48112
Structural predictions for curli amyloid fibril subunits CsgA and CsgB†
Received
20th July 2017
, Accepted 5th October 2017
First published on 16th October 2017
Abstract
Curli are amyloid fibrils that grow from many enteric bacteria and play a structural role in the biofilm extracellular matrix (ECM). Although curli biogenesis is one of the best understood amyloidogenic pathways, the exact atomistic structure of the major subunit CsgA is still unknown. We assess structural models of CsgA and the minor subunit CsgB obtained using the Robetta, Quark, FALCON@home and RaptorX protein structure prediction servers, as well as previously published models. Our objective is to identify or produce models of CsgA and CsgB that exhibit (1) beta-helical structure, (2) sizing in agreement with experiment, (3) alignment among conserved residues, and (4) stability in MD simulations. To this end, an additional CsgA model is created by threading the sequence to the only CsgB model that meets these criteria. Static models are first assessed in terms of structure, sizing, and residue alignment. Additionally, short MD simulations are used to rule out models exhibiting instability. Of the servers used, only Robetta and Raptor produced beta-helical structures. We propose candidate models of CsgA and CsgB that meet all four selection criteria, and remain stable in 150 ns simulations. The development of these subunit structural models will enable molecular-level investigation of curli properties.
Introduction
Amyloid fibrils are highly ordered, beta-sheet rich structures that are resistant to degradation and mechanical and environmental stresses.1 Although initially believed to be the product of protein misfolding and aggregation, some functional amyloids are the result of a highly specific folding pathway.2 For example, while dysfunctional amyloids are closely associated with Alzheimers,3 Parkinson's disease,4 type II diabetes5 and more, naturally occurring functional amyloids have been found to contribute to normal physiology of cells and tissues through functions including adhesins in biofilms,6 scaffolding,7 and cell protection.8 So far, amyloids have been harnessed for bioengineering applications such as enhanced adhesives,9 templates for conducting nanowires,10 functionalized biosensors,11 scaffolds for cell adhesion,12 and more.
Curli fibrils are one such amyloid that grow on the surface of bacteria such as E. coli and are a structural component of the biofilm scaffold.13 These fibrils play a key role in adhesion to surfaces14,15 and host cell invasion,16 stimulate autoimmunity,17–19 and curli biogenesis is one of the best-understood pathways of amyloidogenesis. Curli are made up of beta-helical protein monomers arranged with beta-strands stacked parallel to the fibril axis, consisting mainly of a major subunit, CsgA.20,21 In its mature form, CsgA is 13.1 kDa (after the N-terminal signalling peptide is cleaved).22 CsgA is secreted as an unstructured, soluble protein and is nucleated by the membrane-associated minor subunit, CsgB.23–26 CsgA and CsgB possess similar sequences (∼30%) and are believed to have similar structures. However, CsgB has been shown to achieve an amyloid conformation more quickly than CsgA and have an inherent propensity for aggregation, directing CsgA polymerization.23,24,27 It has been proposed that CsgB templates soluble CsgA to induce a conformational transition to a folded structure, although the exact molecular changes during growth are still unknown.23,28 In vitro, CsgA alone can form amyloid, but without CsgB in vivo, CsgA is secreted away from the cell.23,26 As the presence of CsgB shortens the lag phase in CsgA polymerization,29 Hammer et al. noted that the use of CsgB to quickly convert soluble CsgA to stable fibrils may be a strategy employed to reduce cytotoxicity.24 The robust nature of CsgA formation30 permits disassembly and reassembly into films,31 as well as incorporation of mutations and conjugations. Curli have already been engineered to develop strong underwater adhesives,9 multifunctional biofilms,10 template nanoparticles10,32 and quantum dots,32 and more.33,34 However, the complete atomistic structure of neither CsgA nor CsgB has been experimentally determined. As the structure of a protein dictates its' function, knowledge of specific protein structure is of great importance in fully understanding curli formation and function, and utilizing these structures in bioengineering applications.
Amyloid fibrils do not easily permit the use of traditional methods such as X-ray crystallography or solution state NMR due to their insolubility, and repeating beta-strands.20,35 In these cases, solid-state NMR (ssNMR) has been applied to amyloids and has provided structural insight for Alzheimer's beta-amyloid,36 β2-microglobulin fibrils,37 prion proteins,38 tau paired helical filaments,39 and more. X-ray fiber diffraction has also been used to probe structural details of amyloids40,41 including prions.42 For CsgA, obtaining high-quality structural information using ssNMR is difficult, as they may form polymorphisms in vitro and the sequence repeats produce spectral overlaps.35 Although there is no full experimental structural model for curli, a coarse-grained model has been developed to study curli adhesion and desorption.43 For the CsgA monomer, a novel approach using multiple sequence alignment contacts as structural restraints has created two CsgA models with left and right-hand chirality.44 This approach successfully produced beta-helical structures from extended structures using enhanced Monte Carlo simulations, but did not further study the produced structures in regard to dynamics or stability. Use of ssNMR has also begun to uncover details of the structure.20,35 The CsgA structure contains multiple amyloidogenic domains, as well as an N-terminal domain that is necessary for secretion and is protease susceptible, but is not part of the amyloid core.21,45,46 Within the core, repeats R1, R3 and R5 are found to be amyloidogenic, although specific gatekeeper residues have been noted to reduce amyloidogenicity.45,47 CsgA is unstructured during secretion, but a folding intermediate has been detected with a conformation specific antibody. Finally, studies indicate the mature curli fiber contains a beta-helical structure ∼3 nm in diameter,20 with Ser, Gln, Asn and Gln residues conserved along repeating strands.13 The salmonella analog, AgfA is also predicted to favor a parallel beta-helix structure, with Ser, Gln, and Asn residues conserved and aligned across repeating beta-strands.46 In the AgfA model, these residues may contribute to stability by flanking the turns of the helix and hydrogen bonding with the chain backbone.46 Such “polar zippers” have been reported in other amyloids rich in Asn and Gln residues, and stabilize the structure through formation of hydrogen bond networks.48–51 The minor subunit CsgB, is believed to have a comparable beta-helical structure, similar to the salmonella analog, AgfB.52 Within CsgB, the fifth repeat unit (R5) has been shown to be necessary for surface association, indicating that R5 associates with the membrane, and the remaining repeats form an amyloid core that can template CsgA.13,24 Additionally, experiments have found CsgB incorporated in small amounts along the fiber structure, and also located in areas where fibers appeared to branch. Overexpression of CsgB can also lead to short CsgB polymers on the cell surface, indicating CsgB is capable of polymerization in addition to nucleation.28 However, further molecular details about the interactions between CsgB and CsgA subunits are unknown. Interestingly, a recent study was able to monitor curli growth in great detail and found that fibers in situ show anisotropic growth (one end elongates more rapidly than the other). Additionally, temporary defects were observed that may or may not result in a “scar”, indicating imperfections within the fibers are possible.53
So far, no study has critically assessed CsgA or CsgB structural model stability using molecular dynamics (MD) simulations. However, MD has been used to study proteins with similar structures to assess or compare potential models,54–56 gain insight on mechanics57 and folding pathways,58 and guide nanoengineering design.59 Uncovering details of CsgA and CsgB structure will not only give additional insight into their formation and structural properties, but can provide crucial knowledge for opportunities in engineering the fibrils to create functionalized biomaterials. For proteins lacking experimental data, computational methods may be applied to protein structure determination. Determining a protein's topology from sequence alone is currently a grand challenge in the field of protein structure determination and is tested regularly in worldwide CASP (Critical Assessment of protein Structure Prediction) competitions.60–62 The structure of a protein sequence can be template-based, using a known protein with similar sequence or structure to guide predictions, or ab initio, using physical principles rather than previously solved structures. Despite remarkable progress since the advent of structural determination, for ab initio modelling, the vast conformational search space and force field accuracy are two issues still impeding fast and accurate structure determination.63
To study possible CsgA structural models, we choose to take an ab initio approach when possible, as the vast majority of known protein structures are of globular proteins. Threading or template-based approaches may be implicitly biased toward these motifs. Here, we utilize four freely available web servers, Robetta,64,65 FALCON@home,66,67 Quark68 and RaptorX.69,70 The Robetta ab initio method was used, which uses Rosetta's fragment insertion method,71 which has been used successfully72–74 and unsuccessfully75 on a variety of proteins. While the Robetta server is well-established, it requires a significant calculation time, which can be weeks to months for challenging models. FALCON@home has both template-based and ab initio modules; this focuses first on remote homologue identification, and uses the ab initio model when no homologues are identified for the target protein.67 The ab initio module uses a position-specific hidden Markov model to generate structural predictions, using fragments to obtain information on local biases, rather than as building blocks in an assembly process method.66 The template-free protein structure prediction using the Quark server builds fragment structures of variable sizes from unrelated experimental structures, along with a knowledge-based force field method.68 This method has been successfully applied to model ECM-associated proteins,76 beta-barrel components77 and more,78 although benchmark results have indicated that incorrect secondary structure predictions can misguide overall topology predictions, and that decoy segments may be biased toward the fragment library used.68 The RaptorX server uses template-based structure prediction with a threading protocol, incorporating a nonlinear scoring function method70 and can be used for remote homolog detection and structural prediction.79–81 While RaptorX can produce high-quality models for proteins with remote templates, structural predictions are inherently limited by the sequence and structure databases used.70 We reiterate that many servers may be optimized for globular protein structures, and use of a sequence known to form amyloid fibrils may not generate optimal results.
In this work, we present and assess various CsgA and CsgB models obtained through protein structure prediction servers and other methods. Our objective is to identify or produce CsgA and CsgB models that meet all four of the following criteria: (1) beta-helical tertiary structure,20,35,46 (2) sizing in agreement with experiment,20 (3) conserved residues aligned,46 and (4) stability in MD simulations. We first compare initial structural models of CsgA and CsgB, and conduct all-atomistic molecular dynamics simulations in explicit water solvent to assess stability. Finally, we present candidate models that demonstrate stability and agreement with our current understanding of CsgA and CsgB features.
Results and discussion
Model assessment
CsgA models studied were obtained using the Robetta,64,65 RaptorX,69 Quark68 and FALCON@home66,67 servers, as well as two previously published models for CsgA, Tian-LH and Tian-RH (more details in Materials and methods). We created an additional model (CsgA-map) to supplement the models created using prediction servers (more details in Materials and methods). All models produced were beta-rich and representative structural models for each server can be found in Fig. 1. Models produced by the Robetta server, RaptorX server, and through previously published methods44 were predominately beta-helical, while models created using Quark and FALCON@home servers tended to have beta-meander (antiparallel beta-strands linked by hairpin loops) and beta-sandwich structures (two opposing antiparallel beta-sheets), respectively. For all initial models, the beta-sheet content was measured and compared among models (Fig. 2). Across all servers, good agreement was seen in which residues are classified as beta-strand. Models that were not beta-helical in structure often had beta-content near the N-terminus, while beta-helical structures had a disordered region in the 22 N-terminal residues. This analysis reveals that although the tertiary structure varies among predicted models, there is good consistency within secondary structure prediction.
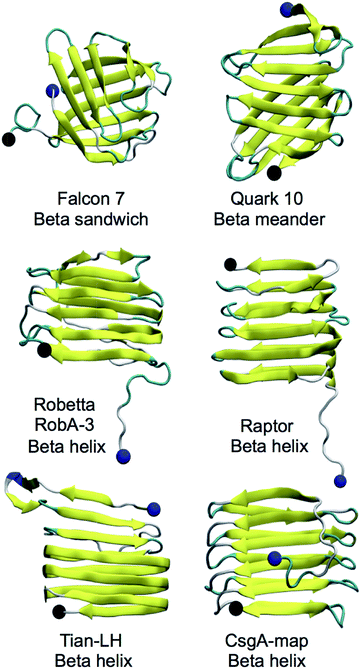 |
| Fig. 1 Representative models from each server. C- and N-termini are labeled with the alpha carbon colored black and blue, respectively. The Robetta, RaptorX and Tian models tended to have a beta-helix structure although some Robetta models contained disordered regions or strand-loop-strand motifs connected by a hairpin turn. Models created using the Quark server tended to have a beta-meander structure (although not all), and models created using the FALCON@home server tended to have a beta-sandwich structure (although not all). | |
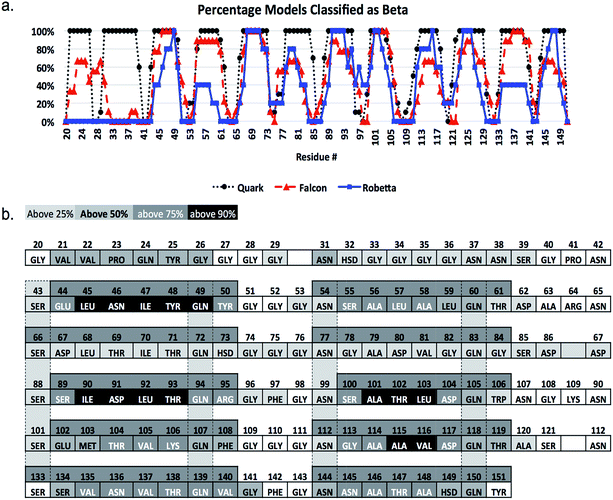 |
| Fig. 2 Secondary structure alignment of CsgA models. In (a), the percentage of models classifying a particular residue as “beta” structured is plotted for servers that produced multiple models (RaptorX excluded). Here, while beta-structured regions after the N-terminal 22 residues generally agree well regarding beta-strand and loop placement, the Quark server has much more consistent agreement between individual models compared to FALCON@home and Robetta, which have less uniform beta-structure assignment. The sequence is shown in (b) with residues labeled by name and number, and shaded columns indicating conserved residues. Each cell is colored by whether above 25%, 50%, 75% or 90% of all models classify that residue as beta-structured. Sequence position 30 indicates a glycine that is present in experimental work using ssNMR,35 but not the TIAN-LH and TIAN-RH models.44 This amino acid was not included in sequences submitted to prediction servers for consistency. | |
The size and structural detail of possible models was examined, and can be found in Fig. 3 and Table 1. Beta-helical models generally had a beta-sheet face roughly 18–21 Å tall, were 28–30 Å wide (along the beta-strand length), and opposite beta-sheets were roughly 8–12 Å apart. The spacing between beta strands in model CsgA-map aligns well with a previous study using X-ray fiber diffraction,20 which found primary spacing between beta-strands within a beta-sheet to be ∼4.7 Å, and a spacing of ∼9 Å between beta-sheet layers (compare to ∼4.9 Å and ∼9.6 Å in Table 1). To assess how well each beta-sheet face fits together, the pocket volume inside each beta helix was estimated as shown in Table 1 using MOLE 2.0.82 A notable feature of amyloid structures that promotes stability is the dry “steric zipper” of closely meshing internal side chains.83 Models CsgA-map and RobA-3 had the smallest pocket volume values, showing that these models had more side chains facing inward and had better meshing inside the helix core.
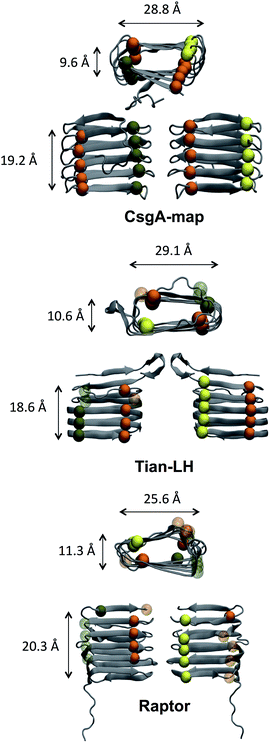 |
| Fig. 3 Helix sizing and conserved residue alignment. Three beta-helical structures are shown. Models CsgA-map and Tian-LH had less than 1 Å difference in helix height, width, and length, while the RaptorX model had a shorter strand length (∼26 Å) and wider core. Although local regions may differ in organization, beta-helical models showed general agreement in beta-helix size. For highlighted residues, Ser is shown in yellow, Gln in orange, and Asn in green. The alpha carbon of each residue is shown as a sphere, represented as solid if within the core, and transparent if surface-exposed. CsgA-map is the only model with all four rows of Ser, Gln, Asn, Gln aligned and on the inside of the structure. | |
Table 1 Beta helix sizing and protein pocket volume
|
Adjacent strand distance (Å) |
Helix height (Å) |
Helix thickness (Å) |
Helix width (Å) |
Pocket volume (Å3) |
Tian-LH |
5.1 ± 0.8 |
18.6 ± 0.9 |
10.6 ± 2.4 |
29.1 ± 2.1 |
1514 |
Tian-RH |
5.1 ± 0.8 |
19.3 ± 1.5 |
10.1 ± 2.1 |
28.5 ± 2.4 |
1520 |
Raptor |
5.6 ± 1.5 |
20.3 ± 1.3 |
11.3 ± 2.2 |
25.6 ± 2.8 |
947 |
RobA-3 |
5.5 ± 1.4 |
20.5 ± 2.5 |
8.7 ± 2.0 |
30.0 ± 2.5 |
467 |
CsgA-map |
4.9 ± 0.4 |
19.2 ± 0.9 |
9.6 ± 1.7 |
28.8 ± 2.0 |
348 |
Alternatively, Tian-LH, Tian-RH and RaptorX have fewer inward facing side chains, leaving large pockets inside the core. The orderly beta-helical models predicted using web servers RobA-3 and RaptorX had right-handed helices. Additionally, the helical portions of CsgA models produced using Robetta were right-handed. The handedness of the CsgA subunit is so far unknown, and previous studies44,84 were unable to discern whether one chirality was favorable over the other. In models created using Robetta and Raptor, the N-terminal 22 residues were generally unstructured, although in both Quark and FALCON@home models, this area contained beta-strand content. Both Tian-LH and RH had an overall beta-helix structure, with a meander motif near the first two beta strands near the N-terminus. RobA-3 is the only of the Robetta models that forms some kind of helix (although disordered), and the other four models contain beta helix-like loops connected with meander motifs.
The distance between adjacent strands, helix height and width were calculated for initial models, and the average value over backbone atoms of each strand is shown with the standard deviation included. Here, we can see that CsgA-map has the smallest distance between adjacent strands and second smallest helix height and thickness. Model CsgA-map also has the smallest standard deviation values, indicating a more organized structure. In the last column, models CsgA-map and RobA-3 are also among the smallest pocket volume values. Additionally, intermolecular distances were calculated for Val, Leu and Phe in the same or neighboring beta-strands and beta-sheets. The average intermolecular distances calculated were 7.7 Å for Val and 7.8 Å for Leu, in accord with experiment.20 Further details can be found in the ESI.†
Next, the alignment of conserved residues was assessed for the initial models. Within the CsgA structure, each repeat contains internally conserved residues Ser, Gln, Asn, and Gln, and we expect these residues to be aligned along repeating beta-strands, based on placement and alignment of these same conserved residues in the analogous protein AgfA.46 In other amyloids, polar residues conserved across beta-strands have been reported facing both inward and outward.85–89 However, in the CsgA sequence, these sets of residues are covalently bonded to (i + 1) mainly charged and polar residues. The next covalently bonded (i + 2) residues are predominately hydrophobic, suggesting the conserved Ser, Gln, and Asn residues likely face inward. Selected models with these residues highlighted can be found in Fig. 3. Most of these residues were inward facing for beta-helical models. No server produced a model of CsgA with all four rows of Ser, Gln, Asn, Gln aligned and on the inside of the structure. Based on fulfilling the criteria of these four sets of amino acids aligned and within the helix core, the structure CsgA-map was created (details in Materials and methods). From these results, if the conserved residues are all indeed aligned and within the helix core, CsgA-map is the only feasible CsgA model of the set assessed here.
The CsgB sequence was also submitted to Robetta, FALCON@home, Quark and RaptorX servers. No predicted models of CsgB atomic structure have been made available, although the salmonella analog AfgB is predicted to also adopt a beta-helical conformation.52 The RaptorX model contained all 151 amino acids and had a right-handed beta-helical structure, with a nine residue alpha helical portion at the N-terminus. Like CsgA, models from the FALCON@home server contained mostly beta-sandwich motifs and Quark server models contained mostly beta-meander motifs. Five models were produced with Robetta (RobB) for the 130 residue C-terminal domain (models in Fig. S1†). Only model RobB-5 had a fully beta-helical structure, and had an unstructured N-terminus. The structure of RobB-5 was right-handed, and the helical portions of models RobB-1, RobB-4 were also right-handed. Models RobB-2 and RobB-3 contained left-handed helical portions.
The CsgB model is also expected to contain residues conserved through helical repeats, although with Asn and two rows of Gln, but not Ser.29 The alignment of conserved residues was assessed for models RobB-3 and RobB-5 (Fig. S2†). In model RobB-3, in one row of Gln (residues 73, 95, 117 and 139), all residues are inward facing and aligned except Q73. For Asn (residues 56, 78, 100, 122), all residues are inward facing, but N56 is separated by a beta-strand and not aligned. Likewise, Gln residues 84, 106, 128 and 150 are inward facing and aligned, but Q62 is also separated by a beta-strand and not aligned. Model RobB-5 takes a full, organized helix shape with all three rows of residues (Gln, Asn, and Gln) inward facing and aligned. From the CsgB models, if all conserved residues are aligned and inward, RobB-5 is the only feasible model.
MD simulations
In addition to the assessment of static models, these structures were run in all-atom MD simulations to rule out unstable models. The root mean square deviation (RMSD) was calculated for each model relative to the initial starting structure over the course of a 10 ns equilibration simulation, known as “iRMSD”. Because of the lack of complete experimental data regarding CsgA structure, iRMSD is taken here as our main indicator of protein stability. This is a measure of how much the structure changes over time, and a low iRMSD reflects a protein model that does not unfold. Because we simulate single monomers, it is reasonable to expect higher mobility in terminal regions of the protein than would occur in a mature fibril. The iRMSD does not consider the N-terminal 22 residues, as they are unstructured in most beta-helical models and are not part of the amyloid core. The average iRMSD with standard deviation over the last 1 ns of equilibration can be found in Table 2. All models produced using the Robetta server are below 4 Å, and on average, Robetta models have an iRMSD of 2.72 Å across all models. By lowest iRMSD, the best five CsgA models are (1) CsgA-map, (2) RobA-3, (3) RobA-5, (4) RobA-4 and (5) RobA-1.
Table 2 iRMSD of CsgA models
iRMSD (Å) |
Tian-LH |
4.31 ± 0.15 |
Falcon-6 |
4.10 ± 0.26 |
Quark-4 |
4.42 ± 0.19 |
Raptor |
4.42 ± 0.19 |
Tian-RH |
4.87 ± 0.15 |
Falcon-7 |
4.47 ± 0.16 |
Quark-5 |
7.20 ± 0.56 |
RobA-1 |
2.93 ± 0.19 |
Falcon-1 |
5.92 ± 0.23 |
Falcon-8 |
3.47 ± 0.33 |
Quark-6 |
5.11 ± 0.15 |
RobA-2 |
3.76 ± 0.33 |
Falcon-2 |
7.13 ± 0.33 |
Falcon-9 |
4.97 ± 0.18 |
Quark-7 |
3.35 ± 0.12 |
RobA-3 |
2.06 ± 0.10 |
Falcon-3 |
4.05 ± 0.17 |
Quark-1 |
3.66 ± 0.14 |
Quark-8 |
3.74 ± 0.24 |
RobA-4 |
2.55 ± 0.08 |
Falcon-4 |
5.36 ± 0.20 |
Quark-2 |
3.74 ± 0.17 |
Quark-9 |
9.38 ± 0.37 |
RobA-5 |
2.30 ± 0.09 |
Falcon-5 |
5.49 ± 0.29 |
Quark-3 |
6.42 ± 0.25 |
Quark-10 |
3.07 ± 0.15 |
CsgA-map |
1.76 ± 0.12 |
The iRMSD was calculated for each frame per trajectory, and the mean and standard deviation over the last nanosecond of simulation are shown for each model. Here, we can see that model CsgA-map has the smallest iRMSD during the last ns. Of the server-created models, RobA-3 from the Robetta server had the lowest iRMSD at 2.06 Å. Comparing across servers, Robetta appeared to have the most stable models, and was the only server to have an average iRMSD across all models to be below 3 Å. FALCON@home and Quark both had averages at 5 Å. The beta-sheet content, number of hydrogen bonds, and solvent-accessible surface area were also calculated over the course of each equilibration run. These metrics are taken as indirect indicators of structure quality, as each alone does not guarantee a near-native structure, but taken together may indicate the stability of protein models relative to one another. This information is averaged over the last ns of each simulation and summarized in Table S1.† The CsgA-map model was found to have the highest percentage of beta-sheet secondary structure. All beta-helical models had little change in overall topology as indicated by iRMSD, but some other models tended to unfold. CsgA-map also had the highest number of hydrogen bonds, and lowest measured solvent-accessible surface area (SASA). Overall, CsgA-map was among the best models for not only low iRMSD, but for high beta structure content, high number of hydrogen bonds, and low increase in SASA. This is also the only CsgA model that has all sets of conserved residues aligned and facing inwards.
The structures for CsgB were subjected to the same 10 ns equilibration in MD. Here, we can see that Models RobB-3 and RobB-5 have the overall lowest iRMSD values (<2 Å). Model RobB-5 has an overall increase in iRMSD of 0.23 Å from the first ns average to the last ns average and the lowest standard deviation in measurement, indicating increased stability relative to the other Robetta models. In addition to having the lowest final iRMSD, model RobB-5 had the highest overall percentage of beta content and of hydrogen bonds, and the second lowest SASA value (Table S2†). Model RobB-3 had the lowest SASA, and second lowest iRMSD, secondary structure content and number of hydrogen bonds.
Replicate simulations
To confirm the assessment of potential models, we included additional trials by repeating simulations, and conducting longer simulations. For two representative models from FALCON@home, Quark and Robetta, as well as the Tian models and CsgA-map, three additional simulations of 10 ns were conducted to acquire more data. Models were chosen by lowest iRMSD per group (Falcon-8, Quark-10 and RobA-3), or highest percentage of beta structure and number of hydrogen bonds (Falcon-7, Quark-7, and RobA-5). The results of these simulations were conducted in the same manner as previously, and iRMSD results for replicates can be seen averaged in Fig. 4. Here, the model CsgA-map has the lowest iRMSD on average during the last nanosecond of equilibration (1.63 ± 0.05 Å). The next lowest scoring model was Tian-RH, followed by RobA-3. The Tian models both had markedly lower iRMSD values in replicate simulations than the original simulations. This could be partly since the iRMSD calculation is sensitive to outliers in the structure, and increased movement occurred near the termini in the original simulations. Still, in all cases, the CsgA-map performs best in terms of iRMSD. The CsgA-map model also had the highest total percentage of beta-structure and number of hydrogen bonds. These replicate simulations serve to confirm that CsgA-map remains stable when additional simulations are conducted.
 |
| Fig. 4 iRMSD replicate averages for representative models. Each model represented shows the average of three 10 ns equilibration simulations, and standard deviation amongst these is shown in error bars. Only the last ns of each simulation are used to calculate these averages. Among replicate trials, the model CsgA-map has both the lowest iRMSD and standard deviation between trials. | |
In addition to replicate simulations, single trials of longer timescale simulations were conducted (150+ ns) to confirm that selected models remain stable over longer periods of time. The models that meet our outlined criteria were CsgA-map for CsgA, and RobB-5 for CsgB. The iRMSD for each model can be found plotted in Fig. 5, and each model iRMSD values below 2.5 Å across the entire simulation. Model CsgA-map had an average iRMSD within the last nanosecond of 1.92 ± 0.06 Å and RobB-5 had and average iRMSD of 1.65 ± 0.09 Å. These longer trials underscore that the CsgA model CsgA-map and CsgB model RobB-5 maintain stability over additional simulation time.
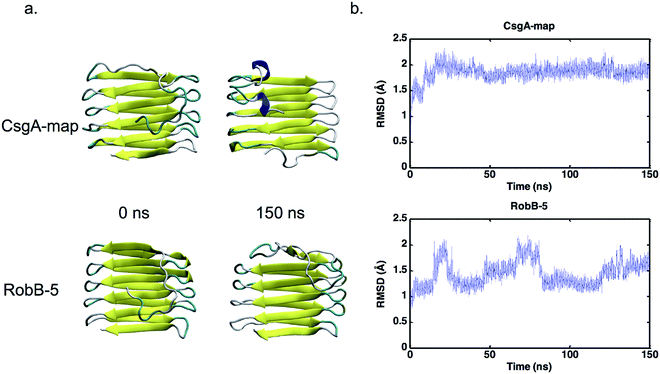 |
| Fig. 5 CsgA structure and iRMSD plots over long trajectories. In (a), the models CsgA-map and RobB-5 are shown at the 0 ns and 150 ns frames of MD simulation. Both models are shown to maintain their overall structure; conformational changes occur mainly in the N-terminus and turn regions. In (b), iRMSD is plotted over time for long simulations. Both CsgA-map and RobB-5 maintain an iRMSD value below 2.5 Å for the entirety of the simulation. During the last ns of simulation, the average iRMSD for CsgA-map was 1.92 Å and was 1.65 Å for RobB-5. Variations in iRMSD for RobB-5 are reflected in conformational changes at either terminus throughout the simulation. | |
Refinement
The CsgA and CsgB models that meet our selection criteria were next refined by taking the most organized structure of respective trajectories (in each case, the last frame), and applying the same minimization protocol as used on the initial set of models. All three models demonstrate improved stability over initial models. Each model has an average iRMSD over the course of 10 ns below 1.55 Å for the course of 10 ns simulations (Fig. S3†). As identical protein sequences with experimentally determined structures exhibited differences of up to 1.2 Å RMSD between pairs in the PDB,90 we find these values acceptable, considering inherent protein flexibility.
Overall, these results show the Robetta models to have the lowest iRMSD, and demonstrate the fewest indicators of unfolding of all CsgA models. While all servers generally had good agreement in which residues are beta-structured, the FALCON@home and Quark servers predicted areas near the N-terminus to contain beta-strands that are disordered in other models. For CsgA, the only model that has all four sets of conserved residues aligned and inwards facing is CsgA-map. This model also has the lowest iRMSD value, and is among the best models for other metrics including high beta-sheet content, high number of protein hydrogen bonds, and low solvent accessible surface area. These results are proven reproducible over replicate simulations, and stable over longer simulations. For CsgB, only RobB-5 had a fully organized beta-helix, and also performed best in terms of stability (iRMSD), secondary structure, and number of hydrogen bonds. Based on secondary and tertiary structure, sizing, residue alignment, and equilibration results, we suggest the CsgA-map model and RobB-5 model as structures that meet known criteria for CsgA and CsgB, respectively.
Conclusions
The curli biogenesis pathway is one of the best studied for amyloid biogenesis. Yet, the structures of CsgA and CsgB, the major and minor curli fibril subunits are still not definitively resolved, impeding detailed study of curli mechanics. Here, we compare the output of multiple freely available protein structure prediction servers in the context of amyloid structure determination. Models are assessed relative to selection criteria outlined in the introduction regarding tertiary structure, sizing, residue alignment, and stability in MD simulations. We find that although secondary structure predictions are consistent among servers, only the Robetta and RaptorX servers provide beta-helical models, which are the assumed conformation of these curli subunits.20,35,46 For both CsgA and CsgB, the models with beta-helical structure had the lowest iRMSD values. Although many servers may be optimized for determining the structure of globular proteins, the Robetta server produced the only CsgB model meeting our selection criteria, while other models produced may represent transient folding conformations. No server-created model of CsgA met these requirements, and thus a new model, CsgA-map, was created to meet this need. Although this highlights the difficulty in predicting amyloid structures using current protein structure prediction servers, the performance of these methods in general is well-documented in several reviews and the CASP competitions.62,91–94 Finally, we present candidate models of CsgA and CsgB subunits that meet the necessary structural criteria. The structural prediction of the main curli fibril subunits paves the way for further studies probing native curli dynamics or using mutations to engineer curli fibrils possessing advanced functionalities.
Materials and methods
Models used
The FALCON@home server identified ten homologues and produced nine models.67 The Quark server from the Zhang lab produced ten models.68 One model was produced from the RaptorX server.69,70 Five models were produced using the Robetta server (prefix RobA).64,65 The Robetta ab initio method uses Rosetta's fragment insertion method.71 This begins with an extended conformation and replaces fragments of 3 or 9 residues with backbone torsional angles from a known protein. These fragments are assembled using a Monte Carlo search and a Metropolis selection criterion for energy minimization. Refinement is conducted using an all-atom energy function and decoy structures are generated using simulations with different random seeds, and subsequent clustering. Models are then selected based on knowledge-based energy scoring functions. This process may take weeks to months for challenging models. The default parameters for each server were used to produce predicted models. The left-handed and right-handed models from a study by P. Tian et al. were utilized.44
Based on the models resulting for CsgA, the CsgB sequence was submitted to all web servers used for CsgA. However, only simulations were run on CsgB models produced using Robetta (RobB). Because the N-terminal 22 residues were often unstructured in Robetta models, the CsgA sequence was also submitted to Robetta with the N-terminal 22 residues omitted. Nevertheless, the N-terminal 20 residues in this model were still unstructured and therefore these models were not analyzed.
CsgA-map model
Of all CsgB models, only model RobB-5 met all required structural criteria. No server-produced model of CsgA met all desired criteria. Because CsgA and CsgB have sequence similarity, associate in the building of curli fibrils, and are expected to have similar structure, we used the RobB-5 model as a template for CsgA. The model CsgA-map was created by threading the CsgA sequence onto model RobB-5 such that conserved residues are inward facing and aligned. This was completed using MODELLER,95 and the alignment used can be found in ESI.†
System setup
Each model was solvated in an explicit water box of CHARMM modified TIP3P water molecules using the VMD “solvate” command.96 For simulations with CsgA, 20
072 water molecules were used and neutralized with 6 sodium ions. For simulations with CsgB, 20
072 water molecules were used and the system was neutralized with 3 Cl ions.
Simulation protocol
All-atomistic simulations were performed using NAMD97 with a 1 fs time step. Periodic boundary conditions were applied in three directions and an NPT ensemble with constant pressure of 1 atm and constant temperature of 300 K was used. All bonded and non-bonded interactions were modeled using the latest CHARMM force field (Aug 2016 C36).98 Although this force field has been shown to bias toward left-handed alpha helices,99 alpha helical motifs are not expected within the CsgA structure. The standard LJ potential was used for long-range non-bonded interactions and the particle mesh Ewald technique was employed for electrostatic interactions. Trajectory information was recorded at 2 ps intervals, and results were visualized using VMD and analyzed using tcl scripts in VMD.100 For replicate simulations, trajectory information was recorded at 10 ps intervals.
For each model simulated, first a simulation with all alpha carbons fixed was conducted to allow side-chain relaxation. An energy minimization of 10
000 steps was conducted, followed by a 100
000 fs equilibration. Next, each system underwent unfixed energy minimization for 10
000 steps followed by a 100
000 fs equilibration. Simulations were then run for 10 ns for all models.
Analysis
Unless otherwise noted, calculations from equilibration data refer to the average value measured over the last 1 ns of the simulation. For the pocket volume estimation, total volume of the cavities within the core of each model was calculated with a probe radius of 3.00 Å and interior threshold 1.25 Å. Sizing measurements were taken as the average distances between backbone atoms of the appropriate sections. All secondary structure assessment was calculated using the STRIDE algorithm.101 Because of the unstructured N-terminal 22 residues, these are not used in the radius of gyration or iRMSD calculation. The number of hydrogen bonds within the protein was measured using a 20 degree and 3 Å cutoff. Solvent Accessible Surface Area (SASA) was calculated using a 1.4 Å probe size.
Conflicts of interest
The authors state there are no conflicts to declare.
Acknowledgements
The authors acknowledge a supercomputing grant from the Northwestern University High Performance Computing Center and the Department of Defense Supercomputing Resource Center. This research was sponsored by an award from the Office of Naval Research Young Investigator Program (grant #N00014-15-1-2701). E. P. D. was supported by the Department of Defense (DoD) through the National Defense Science and Engineering Graduate Fellowship (NDSEG) Program. E. P. D. gratefully acknowledges support from the Ryan Fellowship and the Northwestern University International Institute for Nanotechnology. The authors thank Dr David Zanuy Gómara and Dr Yongbo Zhang for helpful discussions.
References
- M. Hammar, A. Arnqvist, Z. Bian, A. Olsen and S. Normark, Mol. Microbiol., 1995, 18, 661–670 CrossRef CAS PubMed.
- M. M. Barnhart and M. R. Chapman, Annu. Rev. Microbiol., 2006, 60, 131–147 CrossRef CAS PubMed.
- J. Hardy and D. J. Selkoe, science, 2002, 297, 353–356 CrossRef CAS PubMed.
- D. J. Irwin, V. M.-Y. Lee and J. Q. Trojanowski, Nat. Rev. Neurosci., 2013, 14, 626–636 CrossRef CAS PubMed.
- G. Cooper, A. Willis, A. Clark, R. Turner, R. Sim and K. Reid, Proc. Natl. Acad. Sci. U. S. A., 1987, 84, 8628–8632 CrossRef CAS.
- P. Larsen, J. L. Nielsen, M. S. Dueholm, R. Wetzel, D. Otzen and P. H. Nielsen, Environ. Microbiol., 2007, 9, 3077–3090 CrossRef CAS PubMed.
- D. M. Fowler, A. V. Koulov, C. Alory-Jost, M. S. Marks, W. E. Balch and J. W. Kelly, PLoS Biol., 2005, 4, e6 Search PubMed.
- V. A. Iconomidou, G. Vriend and S. J. Hamodrakas, FEBS Lett., 2000, 479, 141–145 CrossRef CAS PubMed.
- C. Zhong, T. Gurry, A. A. Cheng, J. Downey, Z. Deng, C. M. Stultz and T. K. Lu, Nat. Nanotechnol., 2014, 9, 858–866 CrossRef CAS PubMed.
- P. Q. Nguyen, Z. Botyanszki, P. K. R. Tay and N. S. Joshi, Nat. commun., 2014, 5, 4945 CrossRef CAS PubMed.
- C. A. Hauser, S. Maurer-Stroh and I. C. Martins, Chem. Soc. Rev., 2014, 43, 5326–5345 RSC.
- S. L. Gras, A. K. Tickler, A. M. Squires, G. L. Devlin, M. A. Horton, C. M. Dobson and C. E. MacPhee, Biomaterials, 2008, 29, 1553–1562 CrossRef CAS PubMed.
- M. L. Evans and M. R. Chapman, Biochim. Biophys. Acta, Mol. Cell Res., 2014, 1843, 1551–1558 CrossRef CAS PubMed.
- C. Prigent-Combaret, G. Prensier, T. T. Le Thi, O. Vidal, P. Lejeune and C. Dorel, Environ. Microbiol., 2000, 2, 450–464 CrossRef CAS PubMed.
- L. Cegelski, J. S. Pinkner, N. D. Hammer, C. K. Cusumano, C. S. Hung, E. Chorell, V. Åberg, J. N. Walker, P. C. Seed and F. Almqvist, Nat. Chem. Biol., 2009, 5, 913–919 CrossRef CAS PubMed.
- U. Gophna, M. Barlev, R. Seijffers, T. Oelschlager, J. Hacker and E. Ron, Infect. Immun., 2001, 69, 2659–2665 CrossRef CAS PubMed.
- P. M. Gallo, G. J. Rapsinski, R. P. Wilson, G. O. Oppong, U. Sriram, M. Goulian, B. Buttaro, R. Caricchio, S. Gallucci and Ç. Tükel, Immunity, 2015, 42, 1171–1184 CrossRef CAS PubMed.
- S. A. Tursi, E. Y. Lee, N. J. Medeiros, M. H. Lee, L. K. Nicastro, B. Buttaro, S. Gallucci, R. P. Wilson, G. C. Wong and Ç. Tükel, PLoS Pathog., 2017, 13, e1006315 Search PubMed.
- Ç. Tükel, M. Raffatellu, A. D. Humphries, R. P. Wilson, H. L. Andrews-Polymenis, T. Gull, J. F. Figueiredo, M. H. Wong, K. S. Michelsen and M. Akçelik, Mol. Microbiol., 2005, 58, 289–304 CrossRef PubMed.
- F. Shewmaker, R. P. McGlinchey, K. R. Thurber, P. McPhie, F. Dyda, R. Tycko and R. B. Wickner, J. Biol. Chem., 2009, 284, 25065–25076 CrossRef CAS PubMed.
- N. Van Gerven, R. D. Klein, S. J. Hultgren and H. Remaut, Trends Microbiol., 2015, 23, 693–706 CrossRef CAS PubMed.
- L. S. Robinson, E. M. Ashman, S. J. Hultgren and M. R. Chapman, Mol. Microbiol., 2006, 59, 870–881 CrossRef CAS PubMed.
- N. D. Hammer, J. C. Schmidt and M. R. Chapman, Proc. Natl. Acad. Sci. U. S. A., 2007, 104, 12494–12499 CrossRef CAS PubMed.
- N. D. Hammer, B. A. McGuffie, Y. Zhou, M. P. Badtke, A. A. Reinke, K. Brännström, J. E. Gestwicki, A. Olofsson, F. Almqvist and M. R. Chapman, J. Mol. Biol., 2012, 422, 376–389 CrossRef CAS PubMed.
- M. R. Chapman, L. S. Robinson, J. S. Pinkner, R. Roth, J. Heuser, M. Hammar, S. Normark and S. J. Hultgren, Science, 2002, 295, 851–855 CrossRef CAS PubMed.
- M. Hammar, Z. Bian and S. Normark, Proc. Natl. Acad. Sci. U. S. A., 1996, 93, 6562–6566 CrossRef CAS.
- N. N. Louros, G. M. Bolas, P. L. Tsiolaki, S. J. Hamodrakas and V. A. Iconomidou, J. Struct. Biol., 2016, 195, 179–189 CrossRef CAS PubMed.
- Z. Bian and S. Normark, EMBO J., 1997, 16, 5827–5836 CrossRef CAS PubMed.
- X. Wang and M. R. Chapman, J. Mol. Biol., 2008, 380, 570–580 CrossRef CAS PubMed.
- M. S. Dueholm, S. B. Nielsen, K. L. Hein, P. Nissen, M. Chapman, G. Christiansen, P. H. Nielsen and D. E. Otzen, Biochemistry, 2011, 50, 8281–8290 CrossRef CAS PubMed.
- N. m.-M. Dorval Courchesne, A. Duraj-Thatte, P. K. R. Tay, P. Q. Nguyen and N. S. Joshi, ACS Biomater. Sci. Eng., 2016, 3(5), 733–741 CrossRef.
- A. Y. Chen, Z. Deng, A. N. Billings, U. O. Seker, M. Y. Lu, R. J. Citorik, B. Zakeri and T. K. Lu, Nat. Mater., 2014, 13, 515–523 CrossRef CAS PubMed.
- M. T. Abdelwahab, E. Kalyoncu, T. Onur, M. Z. Baykara and U. O. S. Seker, Langmuir, 2017, 33, 4337–4345 CrossRef CAS PubMed.
- E. Kalyoncu, R. E. Ahan, T. T. Olmez and U. O. S. Seker, RSC Adv., 2017, 7, 32543–32551 RSC.
- T. Schubeis, P. Yuan, M. Ahmed, M. Nagaraj, B. J. van Rossum and C. Ritter, Angew. Chem., Int. Ed., 2015, 54, 14669–14672 CrossRef CAS PubMed.
- A. T. Petkova, Y. Ishii, J. J. Balbach, O. N. Antzutkin, R. D. Leapman, F. Delaglio and R. Tycko, Proc. Natl. Acad. Sci. U. S. A., 2002, 99, 16742–16747 CrossRef CAS PubMed.
- K. Iwata, T. Fujiwara, Y. Matsuki, H. Akutsu, S. Takahashi, H. Naiki and Y. Goto, Proc. Natl. Acad. Sci. U. S. A., 2006, 103, 18119–18124 CrossRef CAS PubMed.
- B. R. Groveman, M. A. Dolan, L. M. Taubner, A. Kraus, R. B. Wickner and B. Caughey, J. Biol. Chem., 2014, 289, 24129–24142 CrossRef CAS PubMed.
- V. Daebel, S. Chinnathambi, J. Biernat, M. Schwalbe, B. Habenstein, A. Loquet, E. Akoury, K. Tepper, H. Müller and M. Baldus, J. Am. Chem. Soc., 2012, 134, 13982–13989 CrossRef CAS PubMed.
- M. R. Sawaya, S. Sambashivan, R. Nelson, M. I. Ivanova, S. A. Sievers, M. I. Apostol, M. J. Thompson, M. Balbirnie, J. J. Wiltzius and H. T. McFarlane, Nature, 2007, 447, 453–457 CrossRef CAS PubMed.
- L. C. Serpell, P. E. Fraser and M. Sunde, Methods Enzymol., 1999, 309, 526–536 CAS.
- H. Wille, W. Bian, M. McDonald, A. Kendall, D. W. Colby, L. Bloch, J. Ollesch, A. L. Borovinskiy, F. E. Cohen and S. B. Prusiner, Proc. Natl. Acad. Sci. U. S. A., 2009, 106, 16990–16995 CrossRef CAS PubMed.
- Y. Zhang, A. Wang, E. P. DeBenedictis and S. Keten, Nanotechnology, 2017 DOI:10.1088/1361-6528/aa8f72.
- P. Tian, W. Boomsma, Y. Wang, D. E. Otzen, M. H. Jensen and K. Lindorff-Larsen, J. Am. Chem. Soc., 2014, 137, 22–25 CrossRef PubMed.
- X. Wang, D. R. Smith, J. W. Jones and M. R. Chapman, J. Biol. Chem., 2007, 282, 3713–3719 CrossRef CAS PubMed.
- S. Collinson, J. Parker, R. Hodges and W. Kay, J. Mol. Biol., 1999, 290, 741–756 CrossRef CAS PubMed.
- X. Wang, Y. Zhou, J.-J. Ren, N. D. Hammer and M. R. Chapman, Proc. Natl. Acad. Sci. U. S. A., 2010, 107, 163–168 CrossRef CAS PubMed.
- Y. Zhang, V. H. Man, C. Roland and C. Sagui, ACS Chem. Neurosci., 2016, 7, 576–587 CrossRef CAS PubMed.
- A. Schmidt, K. Annamalai, M. Schmidt, N. Grigorieff and M. Fändrich, Proc. Natl. Acad. Sci. U. S. A., 2016, 113, 6200–6205 CrossRef CAS PubMed.
- M. F. Perutz, T. Johnson, M. Suzuki and J. T. Finch, Proc. Natl. Acad. Sci. U. S. A., 1994, 91, 5355–5358 CrossRef CAS.
- J. Zheng, B. Ma, C.-J. Tsai and R. Nussinov, Biophys. J., 2006, 91, 824–833 CrossRef CAS PubMed.
- A. P. White, S. K. Collinson, P. A. Banser, D. L. Gibson, M. Paetzel, N. C. Strynadka and W. W. Kay, J. Mol. Biol., 2001, 311, 735–749 CrossRef CAS PubMed.
- M. Sleutel, I. Van den Broeck, N. Van Gerven, C. Feuillie, W. Jonckheere, C. Valotteau, Y. F. Dufrêne and H. Remaut, Nat. Chem. Biol., 2017, 13, 902 CrossRef CAS PubMed.
- F.-H. Lin, P. L. Davies and L. A. Graham, Biochemistry, 2011, 50, 4467–4478 CrossRef CAS PubMed.
- B. Zhao, M. A. C. Stuart and C. K. Hall, Soft Matter, 2016, 12, 3721–3729 RSC.
- K. C. Kunes, S. C. Clark, D. L. Cox and R. R. Singh, Prion, 2008, 2, 81–90 CrossRef PubMed.
- L. P. Heinz, K. M. Ravikumar and D. L. Cox, Nano Lett., 2015, 15, 3035–3040 CrossRef CAS PubMed.
- B. Zhao, M. A. C. Stuart and C. K. Hall, PLoS Comput. Biol., 2017, 13, e1005446 Search PubMed.
- M. D. Peralta, A. Karsai, A. Ngo, C. Sierra, K. T. Fong, N. R. Hayre, N. Mirzaee, K. M. Ravikumar, A. J. Kluber and X. Chen, ACS Nano, 2015, 9, 449–463 CrossRef CAS PubMed.
- J. Moult, J. T. Pedersen, R. Judson and K. Fidelis, Proteins: Struct., Funct., Bioinf., 1995, 15(3), 285–289 Search PubMed.
- A. Zemla, Č. Venclovas, J. Moult and K. Fidelis, Proteins: Struct., Funct., Bioinf., 2001, 45, 13–21 CrossRef PubMed.
- J. Moult, Curr. Opin. Struct. Biol., 2005, 15, 285–289 CrossRef CAS PubMed.
- J. Chen and C. L. Brooks, Proteins: Struct., Funct., Bioinf., 2007, 67, 922–930 CrossRef CAS PubMed.
- P. Bradley, K. M. Misura and D. Baker, Science, 2005, 309, 1868–1871 CrossRef CAS PubMed.
- D. Chivian, D. E. Kim, L. Malmström, P. Bradley, T. Robertson, P. Murphy, C. E. Strauss, R. Bonneau, C. A. Rohl and D. Baker, Proteins: Struct., Funct., Bioinf., 2003, 53, 524–533 CrossRef CAS PubMed.
- S. C. Li, D. Bu, J. Xu and M. Li, Protein Sci., 2008, 17, 1925–1934 CrossRef CAS PubMed.
- C. Wang, H. Zhang, W.-M. Zheng, D. Xu, J. Zhu, B. Wang, K. Ning, S. Sun, S. C. Li and D. Bu, Bioinformatics, 2016, 32, 462–464 CrossRef CAS PubMed.
- D. Xu and Y. Zhang, Proteins: Struct., Funct., Bioinf., 2012, 80, 1715–1735 CrossRef CAS PubMed.
- J. Xu, M. Li, D. Kim and Y. Xu, J. Bioinf. Comput. Biol., 2003, 1, 95–117 CrossRef CAS.
- M. Källberg, H. Wang, S. Wang, J. Peng, Z. Wang, H. Lu and J. Xu, Nat. Protoc., 2012, 7, 1511–1522 CrossRef PubMed.
- C. A. Rohl, C. E. Strauss, K. M. Misura and D. Baker, Methods Enzymol., 2004, 383, 66–93 CAS.
- V. R. R. Malapaka and B. C. Tripp, J. Mol. Model., 2006, 12, 481–493 CrossRef CAS PubMed.
- T. D. Do, A. Chamas, X. Zheng, A. Barnes, D. Chang, T. Veldstra, H. Takhar, N. Dressler, B. Trapp and K. Miller, Biochemistry, 2015, 54, 4050–4062 CrossRef CAS PubMed.
- M. Da Silva, L. Shen, V. Tcherepanov, C. Watson and C. Upton, Bioinformatics, 2006, 22, 2846–2850 CrossRef CAS PubMed.
- G. Kochan, D. Escors, J. M. González, J. M. Casasnovas and M. Esteban, Cell. Microbiol., 2008, 10, 149–164 CAS.
- W. Zhang, J. Sun, W. Ding, J. Lin, R. Tian, L. Lu, X. Liu, X. Shen and P.-Y. Qian, Front. Cell. Infect. Microbiol., 2015, 5(40) DOI:10.3389/fcimb.2015.00040.
- D. Nagarajan, G. Deka and M. Rao, BMC Biochem., 2015, 16, 18 CrossRef PubMed.
- K. K. Biggar, E. Kotani, T. Furusawa and K. B. Storey, FASEB J., 2013, 27, 3376–3383 CrossRef CAS PubMed.
- A. M. Goswami, Meta Gene, 2015, 5, 162–172 CrossRef PubMed.
- A. Banerjee and S. Ray, Gene, 2016, 576, 72–78 CrossRef CAS PubMed.
- K. Rosti, A. Goldman and T. Kajander, BMC Biochem., 2015, 16, 8 CrossRef PubMed.
- D. Sehnal, R. S. Vařeková, K. Berka, L. Pravda, V. Navrátilová, P. Banáš, C.-M. Ionescu, M. Otyepka and J. Koča, J. Cheminf., 2013, 5, 39 CAS.
- M. R. Sawaya, S. Sambashivan, R. Nelson, M. I. Ivanova, S. A. Sievers, M. I. Apostol, M. J. Thompson, M. Balbirnie, J. J. Wiltzius and H. T. McFarlane, Nature, 2007, 447, 453–457 CrossRef CAS PubMed.
- E. P. DeBenedictis, J. Liu and S. Keten, Sci. Adv., 2016, 2, e1600998 Search PubMed.
- D. S. Eisenberg and M. R. Sawaya, Annu. Rev. Biochem., 2017, 86(1), 69–95 CrossRef CAS PubMed.
- H.-H. G. Tsai, K. Gunasekaran and R. Nussinov, Structure, 2006, 14, 1059–1072 CrossRef CAS PubMed.
- R. Nelson, M. R. Sawaya, M. Balbirnie, A. Ø. Madsen, C. Riekel, R. Grothe and D. Eisenberg, Nature, 2005, 435, 773 CrossRef CAS PubMed.
- A. V. Kajava, U. Baxa, R. B. Wickner and A. C. Steven, Proc. Natl. Acad. Sci. U. S. A., 2004, 101, 7885–7890 CrossRef CAS PubMed.
- J. J. Wiltzius, S. A. Sievers, M. R. Sawaya and D. Eisenberg, Protein Sci., 2009, 18, 1521–1530 CrossRef CAS PubMed.
- I. Kufareva and R. Abagyan, Homology Modeling: Methods and Protocols, 2012, pp. 231–257 Search PubMed.
- D. Fischer, Curr. Opin. Struct. Biol., 2006, 16, 178–182 CrossRef CAS PubMed.
- Y. Zhang, Curr. Opin. Struct. Biol., 2008, 18, 342–348 CrossRef CAS PubMed.
- K.-C. Chou and H.-B. Shen, Nat. Sci., 2009, 1, 63 CAS.
- K. Ginalski, Curr. Opin. Struct. Biol., 2006, 16, 172–177 CrossRef CAS PubMed.
- B. Webb and A. Sali, Protein Struct. Predict., 2014, 1–15 Search PubMed.
- A. D. MacKerell Jr, D. Bashford, M. Bellott, R. L. Dunbrack Jr, J. D. Evanseck, M. J. Field, S. Fischer, J. Gao, H. Guo and S. Ha, J. Phys. Chem. B, 1998, 102, 3586–3616 CrossRef PubMed.
- J. C. Phillips, R. Braun, W. Wang, J. Gumbart, E. Tajkhorshid, E. Villa, C. Chipot, R. D. Skeel, L. Kale and K. Schulten, J. Comput. Chem., 2005, 26, 1781–1802 CrossRef CAS PubMed.
- R. B. Best, X. Zhu, J. Shim, P. E. Lopes, J. Mittal, M. Feig and A. D. MacKerell Jr, J. Chem. Theory Comput., 2012, 8, 3257–3273 CrossRef CAS PubMed.
- S. Rauscher, V. Gapsys, M. J. Gajda, M. Zweckstetter, B. L. de Groot and H. Grubmüller, J. Chem. Theory Comput., 2015, 11, 5513–5524 CrossRef CAS PubMed.
- W. Humphrey, A. Dalke and K. Schulten, J. Mol. Graphics, 1996, 14, 33–38 CrossRef CAS PubMed.
- D. Frishman and P. Argos, Proteins: Struct., Funct., Bioinf., 1995, 23, 566–579 CrossRef CAS PubMed.
Footnote |
† Electronic supplementary information (ESI) available. See DOI: 10.1039/c7ra08030a |
|
This journal is © The Royal Society of Chemistry 2017 |
Click here to see how this site uses Cookies. View our privacy policy here.