DOI:
10.1039/C7AN01471F
(Paper)
Analyst, 2018,
143, 241-249
Detection of sepsis in patient blood samples using CD64 expression in a microfluidic cell separation device†
Received
5th September 2017
, Accepted 8th November 2017
First published on 9th November 2017
Abstract
A microfluidic affinity separation device was developed for the detection of sepsis in critical care patients. An affinity capture method was developed to capture cells based on changes in CD64 expression in a single, simple microfluidic chip for sepsis detection. Both sepsis patient samples and a laboratory CD64+ expression model were used to validate the microfluidic assay. Flow cytometry analysis showed that the chip cell capture had a linear relationship with CD64 expression in laboratory models. The Sepsis Chip detected an increase in upregulated neutrophil-like cells when the upregulated cell population is as low as 10% of total cells spiked into commercially available aseptic blood samples. In a proof of concept study, blood samples obtained from sepsis patients within 24 hours of diagnosis were tested on the chip to further validate its performance. On-chip CD64+ cell capture from 10 patient samples (619 ± 340 cells per chip) was significantly different from control samples (32 ± 11 cells per chip) and healthy volunteer samples (228 ± 95 cells per chip). In addition, the on-chip cell capture has a linear relationship with CD64 expression indicating our approach can be used to measure CD64 expression based on total cell capture on Sepsis Chip. Our method has proven to be sensitive, accurate, rapid, and cost-effective. Therefore, this device is a promising detection platform for neutrophil activation and sepsis diagnosis.
Introduction
Sepsis is life-threatening organ dysfunction caused by a dysregulated host response to invasive infection.1–6 Complications from sepsis were the 11th cause of death nationwide in 2014, and cases continue to increase annually.7 Since sepsis is not a specific disease but a syndrome with a still uncertain pathology, at this time there is no “gold standard” for sepsis diagnosis. Currently, the routine operation in clinical is the culture of sputum, urine, and/or blood for suspicious pathogens. Bacterial culture results which typically take 2–14 days, and not all septic patients have positive blood cultures.8 After a positive blood culture is confirmed, a series of steps are taken to identify the pathogen and to test the antimicrobial susceptibility. The total testing time ranges from 2–16 days, although 2–4 days is typical.9 Due to the lengthy time required for bacterial culture, clinical diagnosis (quick Sepsis-Related Organ Failure Assessment (qSOFA))10 is instead used, and antibiotic treatment is initiated immediately. This prophylactic antibiotic use results in higher health care costs and may increase antibiotic resistance of pathogenic bacteria.11 In addition, the diagnostic scoring of symptoms is not quantitative.
Since sepsis is initiated by an infectious agent stimulating the immune system, physiological response can have an important place in sepsis diagnosis.5,11 More than 100 biomarkers, including cell surface antigens and soluble receptors, have been reported as useful indicators of sepsis.12–14 Soluble biomarker, such as C-reactive protein (CRP), procalcitonin (PCT), cytokines (IL-6, IL-8, IL-10), etc., are the most widely reported.15–17 However, CRP has not been considered as a clinical parameter due to its absence of specificity and short half-time.12 The diagnostic performance of PCT is still debated.17,18 Kemmler et al.,19 and Buchegger et al.20,21 have reported different microarrays for diagnosis of sepsis by testing CRP, IL-6, PCT and neopterin (NPT) with on-chip immunofluorescence assays.
Neutrophils play an important role in the pathological response to sepsis. Numerous studies have shown that there are changes in neutrophil morphology, mechanics, and motility during sepsis.22–25 Increased size, volume, and granularity were observed during sepsis via traditional microscopy, flow cytometry, and automated hematology analysis.26–30 Neutrophil mechanics and motility both decrease in septic conditions.31–33 Butler et al. developed a microfluidic device to precisely measure chemotaxis speed at single-cell resolution which could potentially serve as an early indicator for sepsis.34 Spontaneous neutrophil migration can be quantified in microfluidic channels.23 Neutrophil dielectrophoresis and pathogen extraction have also been evaluated as sepsis detection methods.35–38 While these approaches have made progress toward sepsis detection, there is room to improve with respect to analysis time, reagent consumption, ease of use, etc.
The high-affinity receptor for IgG (FcγRI, CD64) has shown to be a highly sensitive and specific biomarker for diagnosis of bacterial infection in many studies.18,39–47 CD64 expression on resting macrophages, monocytes, and neutrophils is low.48 For lymphocytes, the expression of CD64 is low and the increase of expression during sepsis is negligible.49 For monocytes, the expression of CD64 is higher than neutrophils during both healthy and septic conditions. However, the population of CD64+ monocytes is one-tenth of the neutrophil population. Neutrophil CD64 expression was found to be upregulated during sepsis.18,42,45 The specificity and sensitivity of CD64 detection in the blood of septic patients were 95.9% and 97.6%, respectively, and AUC (area under receiver operating characteristic curve) value of the CD64 index was 0.985.47 Rogina et al. compared several biomarkers including CD64 expression, CRP concentration, PCT concentration, leukocytes counts, and neutrophil percentage and results showed CD64 expression was the best biomarker for sepsis diagnosis among these candidates.47
In this work, an affinity capture method was developed to capture leukocytes based on changes in CD64 expression in a single microfluidic chip to detect sepsis (Fig. 1). The approach uses the innovative strategy of anti-CD64 isolation, modulating the cell capture so that highly expressing CD64+ cells are captured and low expressing CD64+ cells are not. The key innovations in this work are the use of a tuned affinity surface for selective capture of highly expressing CD64+ cells, optimization with a CD64-expression cell line model, and validation using blood samples from septic patients in the surgical intensive care unit. CD64+ cells were captured with 96% purity, with a 5-fold enrichment. We also observed that cell capture was correlated to CD64 expression. Finally, we observed that patient CD64+ cell counts were significantly elevated relative to healthy controls. This approach allows us to provide an alternative and complementary assay for sepsis in a label-free format, with a quantitative test for sepsis diagnosis.
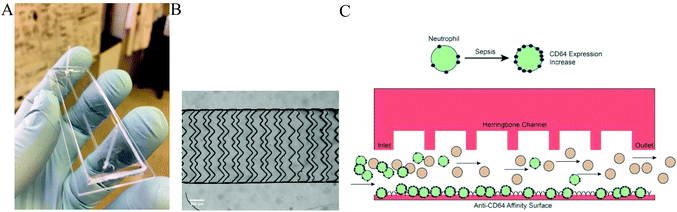 |
| Fig. 1 A: Prototype of the Sepsis Chip (A). The chip contains a herringbone cell capture channel for affinity separation using anti-CD64 antibodies. The chip is bonded to a glass slide (25 × 75 mm). A microscope image (B) of one repeat unit of the herringbone structure is shown (scale bar = 100 μm); the structure results in enhanced cell-surface interactions for isolation of CD64+ cells from blood samples (channel width is 1000 μm). During sepsis, CD64 expression increases on neutrophils (C), enhancing their cell capture in the chip. As a mixture of leukocytes pass through the separation channel, the increased expression of CD64 results in cell capture of neutrophils. The chip is then imaged by a CCD camera for label-free cell counting. | |
Experimental
Device fabrication
Microfluidic devices with herringbone features on the top surface were fabricated using soft lithography procedures.50 A computer based microfluidic design using Canvas software was printed out on a high resolution mask (20
000 dpi, CAD Art Services). The mold for creating channels on the chip was made by spin coating negative photoresist SU-8 2015 (Micro Chem.) at 500 rpm for 6 s, followed by 1000 rpm for 30 s, which formed a layer of 40 μm thick photoresist on a 4-inch silicon wafer (University Wafer). The coated wafer was first pre-baked at 95 °C for 6 min then exposed to UV light for 15 s with the chip design on top. After being post-baked for 6 min, the wafer was developed in SU-8 developer (Micro Chem.) for 6 min to etch unexposed photoresist. Finally, the wafer was coated in 1H,1H,2H,2H-perfluorooctyltrichlorosilane (Alfa Aesar) atmosphere overnight under vacuum to form a hydrophobic surface.
Microfluidic chips were made using a mixture of poly-dimethylsiloxane (PDMS) pre-polymer and curing agent (Ellesworth Adhesives) at a weight ratio of 5
:
1 and baked at 95 °C for 1 h. After curing, the PDMS slab was peeled off of the wafer, and holes were punched to form fluid inlets and outlets. Finally, the PDMS microfluidic slab was sealed onto a glass slide using an oxygen plasma (Harrick). 30-gauge poly-(tetrafluoroethylene) (PTFE) tubing (Small Parts) was attached to the inlet and outlet to connect the syringe while in use. The effective affinity region had a dimension of 1 cm × 1 mm × 40 μm (L × W × H).
Affinity surface modification
The affinity surface on the chip was created using a sandwich deposition approach.51–56 To generate the anti-CD64 capture surface, 8 μL of biotinylated bovine serum albumin (Biotin-BSA, Sigma-Aldrich) (1 mg mL−1, in 10 mM Tris-HCl, pH 8.0, 50 mM NaCl) solution was loaded into channel and incubated for 45 min at room temperature, then rinsed with 8 μL T50 buffer (10 mM Tris-HCl buffer) and air dried. 8 μL of neutravidin (Pierce) solution (0.2 mg mL−1 in 10 mM Tris-HCl buffer) was then introduced into the channel and incubated for 20 min. This neutravidin-modified chip was washed with 8 μL of T50 buffer and 8 μL of deionized water once and stored at 4 °C after air drying. Before cell separation, 3.5 μL of 6.25 μg mL−1 biotin mouse anti-human CD64 antibody (BD Biosciences) was injected into affinity region at the desired concentration and incubated for 20 min. Excess antibodies were flushed out with air bubbles followed by phosphate-buffered saline (PBS) buffer. Before on chip analysis, the chip was filled with 3% BSA in PBS solution to minimize nonspecific binding.
CD64+ cell culture cell differentiation
In order to optimize device development, a controllable sepsis model was used. HL-60 human promyelocytic leukemia cells (American Type Culture Collection) were cultured in 30 mL of complete culture medium (composed of 1 L RPMI 1640 medium, 110 mL fetal bovine serum, and 20 mL penicillin–streptomycin stabilized solution) at 37 °C in an incubator with 5% CO2. Cells were subcultured one to two times each week. HL-60 cells have the potential to differentiate into various cell lines and differentiation can be facilitated by multiple chemical inducers.57 Dimethyl sulfoxide (DMSO, Alfa Aesar) is a common inducer used to differentiate HL-60 cells into neutrophils.58 1.5% (v/v) sterile DMSO was pre-mixed with complete medium before culturing cells. HL-60 cells were first removed from the original flask and centrifuged at 500 rpm for 10 min. The supernatant was discarded and the cell pellet was resuspended in DMSO-medium at a concentration of 3 × 105 cells per mL. Differentiated HL-60 cells (dHL-60 cells) used in this work underwent 4 days of differentiation to guarantee their viability. To simulate sepsis conditions, dHL-60 neutrophils were stimulated to upregulate CD64 expression.59,60 To upregulate CD64 expression on dHL-60 cells, 200 IU mL−1 of interferon-γ (IFN-γ) was added to the dHL-60 cell culture and incubated for 24 h.61
Blood sample processing
The patient blood study was reviewed and approved by the Texas Tech University Health Sciences Center Institutional Review Board. Blood samples used in this project include commercially-obtained aseptic blood, health volunteer blood, and septic patient collected. Blood from healthy volunteers and septic patients were collected from the University Medical Center in Lubbock, TX. Patients were undergoing treatment in the surgical intensive care unit and informed consent was obtained from all patients. Clinical blood samples were collected from surgery patients who were admitted to the UMC between the ages of 21–89 years. Patients were identified as septic based on qSOFA scores.10 In brief, patients were identified as septic if at least two of the following criteria were met in a 2 hours window: (i) altered mental status, (ii) systolic blood pressure equal to or less than 100 mmHg, (iii) respiratory rate equal to or greater than 22 min−1. Patient blood samples were drawn within 24 hours of diagnosis (limited by logistical demands of the hospital). For healthy volunteers, the criteria was to not have any acute or chronic medical conditions. No medications except birth control was allowed. Blood samples were collected in 4 mL BD Vacutainer™ Plastic Blood Collection Tubes with K2EDTA (Fisher Scientific) and were stored at 4 °C until analysis. Prior to use, commercial and clinical blood samples were homogenized by shaking and were warmed to room temperature, respectively. Erythrocytes were lysed by diluting 100 μL blood samples to 1 mL with deionized water for 30 s. Osmolarity was restored by adding 110 μL of concentrated saline buffer (10×) to the diluted blood. The mixture was centrifuged at 4500 rpm for 5 min. The supernatant was then discarded and leukocytes in the precipitate were resuspended with 100 μL PBS to their original concentration.
Flow cytometry analysis
Flow cytometry analysis was conducted prior to cell separation to verify the antigen expression on dHL-60 cells, IFN-γ upregulated dHL-60 cells, and blood samples. One portion of each sample was diluted to 500 μL in PBS as a control. Another portion of each sample was stained with fluorescent conjugated antibodies PE-anti-CD64 (BD Bioscience) or FITC-anti-CD71 (BD Bioscience) for 20 min then resuspended in 500 μL of PBS. Anti-CD71 staining was used to verify HL-60 cell differentiation, and anti-CD64 staining was used to measure relative expression of CD64.
Microfluidic separation and detection
To optimize cell capture, the biotin-anti-CD64 antibody was coated onto the chip at concentrations ranged from 0.625 μg mL−1 to 6.25 μg mL−1. In this work, we optimized the coating concentration to minimize cell capture of low expression CD64 cells. Prior to separation, dHL-60 cells were stained with MitoTracker Green (Invitrogen); IFN-γ upregulated dHL-60 cells were stained with MitoTracker Red (Invitrogen); and lysed blood was stained with Hoechst 33342 (Thermo Fisher Scientific) to distinguish each cell line. Cell mixtures contained different percentages of IFN-γ upregulated dHL-60 cells, with the remaining cells either resting dHL-60 cells or blood leukocytes. Cells were introduced into the chip using a syringe pump (KD Scientific). Once the cell sample had filled the entire fluidic channel, the syringe pump was stopped and cells settled on the channel surface for 30 min.52 Afterwards, 3% BSA in PBS solution was injected into the channel via syringe pump at a flow rate of 0.03 mL h−1 for 20 min to remove unbound cells. Captured cells were observed with an inverted epifluorescence microscope (Olympus IX71) with appropriate excitation and emission filters, and images were taken with a charged-coupled device camera. The capture purity of each cell line were calculated at different experimental conditions to optimize the concentration of the coated antibody. Images were analyzed using Image J software (National Institutes of Health). Each experiment was repeated three times and the standard deviations were reported. The capture purity was calculated as (eqn (1)): |  | (1) |
where NIFN-γ upregulated dHL-60 is the number of captured IFN-γ upregulated dHL-60 cells and NTotal is the number of total captured cells.
The capture efficiency was calculated as (eqn (2)):
| 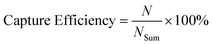 | (2) |
where
N is the number of captured cells on chip and
NSum is the theoretical number of total blood cells loaded into channel (based on on-chip cell concentration per quare micrometers after loading and total area of affinity surface). Error bars represent the standard deviation of each measurement.
Results and discussion
Cell differentiation and CD64 upregulation of a CD64+ cell model for cell capture studies
In order to optimize the method, we used a cell model for CD64+ expression, including basal regulation and upregulated CD64 phenotypes. HL-60 cells can be induced by various chemical stimuli to differentiate into several cell types including granulocytes, monocytes, macrophage-like cells, and eosinophils.57 HL-60 cells have been used in many biological/biochemical studies including (1) providing information on the control mechanism of cell differentiation; (2) guiding new therapeutic approaches for leukemia treatment; (3) explaining possible mechanism of rapid gene transcription; and (4) serving as a model to study specific cellular responses.62–64 In this study, DMSO was used as an inducer to differentiate HL-60 cells into neutrophils (dHL-60 cells). dHL-60 cells and HL-60 cells were stained with Hoechst 33342 to observe nucleus morphology. Wild-type HL-60 cells have classical promyelocytic morphology with a round nucleus. After 4 days treatment with 1.5% DMSO, dHL-60 cells exhibited a morphological change in the nucleus shape and decreased cell size (Fig. 2), which were indicative of neutrophil differentiation.65 However, morphological change alone is not a definitive measurement of differentiation.63 HL-60 cells continuously proliferate in culture medium, while DMSO introduces terminal differentiation of dHL-60 cells, rendering them incapable of proliferation. CD71 expression, a proliferation marker, was analyzed during the differentiation process by flow cytometry. The expression of CD71 on dHL-60 cells decreased with each day, indicating successful differentiation of HL-60 cells (Fig. 2).
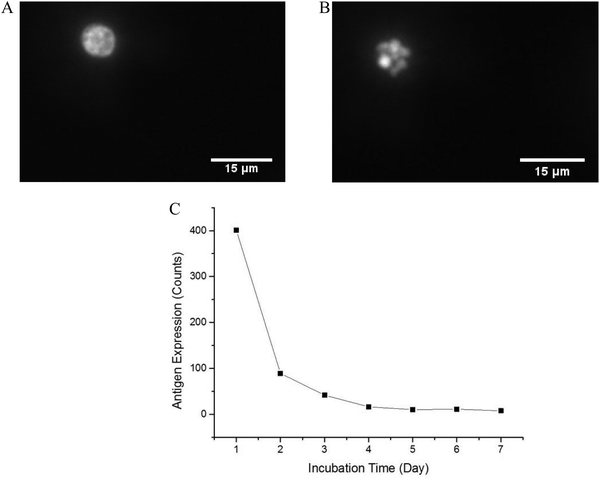 |
| Fig. 2 Representative fluorescence image of HL-60 (A) cells and dHL-60 cells (B) after 4 days of differentiation with 1.5% DMSO. The differentiated cells were used as a basis for a CD64+ cell model for method optimization. HL-60 cells (A) had round nucleus while neutrophil-differentiated (dHL-60) cells (B) exhibited polylmorphonuclear morphology. Scale bar = 15 μm. (C) CD71 expression on dHL-60 cells was tested each day after differentiation with 1.5% DMSO. The decreased CD71 expression on dHL-60 cells indicated these cells went through a terminal differentiation and were no longer capable of proliferation. Each day 10 000 cells were measured by flow cytometry. | |
Interferon-γ (IFN-γ) has been shown to activate polymorphonuclear neutrophils and increase presentation of CD64.66 Flow cytometry analysis showed that CD64 expression on HL-60 cells remained low when treated with IFN-γ, but dHL-60 CD64 expression increased after IFN-γ treatment (Fig. 3). Expression of CD64 on dHL-cells was increased significantly 20 times higher after overnight treatment with IFN-γ from a fluorescence signal average of 63 counts to average 1491 counts. Livaditi et al.45 reported a 10-fold increase in CD64 expression on neutrophils between 16 h to 24 h from onset of sepsis, which is line with the increase in dHL-60 neutrophils when exposed to IFN-γ. Cytograms of PE-Anti-CD64 and side scatter are available in ESI (Fig. S1†).
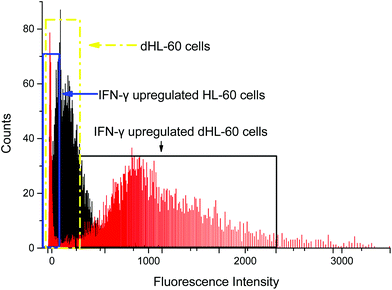 |
| Fig. 3 Flow cytometry analysis of CD64 expression on neutrophil-differentiated HL-60 cells (dHL-60, black shadow in dashed yellow box) and neutrophil-differentiated dHL-60 cells when exposed to interferon gamma (IFN-γ) (red shadow in solid black box). The mean fluorescence for dHL-60 cells and normal HL-60 after CD64 upregulation was 63 and 74 counts, respectively. The mean fluorescence for upregulated dHL-60 cells was 1491 counts. The result showed that IFN-γ had no effect on HL-60 cells, while CD64 expression on dHL-60 cells was upregulated. This approach serves as a controlled model CD64+ expression. | |
Baseline cell capture and affinity surface tuning
The chip readout is an image of the captured cells. This approach is label-free and can be automated in the future. To assess a baseline, aseptic blood samples were run through chips to determine the mean cell capture and the standard deviation between chips. The blood samples did not contain activated neutrophils and represent the baseline normal blood cell capture. The average cell capture for the same blood sample was 32 ± 11 cells (ESI Fig. S2†). A threshold of three standard deviations larger than the mean cell capture was used for detection of CD64+ cells. Therefore, in this work, we set cell capture detection threshold of 65 cells as the baseline (3 standard deviations larger than the mean). The concentration of antibody on the surface was varied between 0.625 μg mL−1 to 6.25 μg mL−1 to minimize capture under these baseline conditions. In stop flow, when cells attach to the surface, with increasing antibody number, equilibrium bond number increased. Meanwhile, when the antibody concentration was high, the affinity interactions between cells and the capture surface increased.67 In addition to the force advantage of specific binding over non-specif binding, antigen–antibody interaction overweight non-specific binding in probability as well. This tuning of the affinity surface allowed for a low background cell count, which would increase with increasing CD64 expression.
CD64+ cell concentration effects
In this work, IFN-γ upregulated dHL-60 cells were mixed with blood leukocytes in different ratios, from 10% to 90%, to determine the activated neutrophil population that could be detected with a background of resting neutrophil-like cells. The captured cell number of CD64+ blood cells remained at a low level (<65 cells per chip), irrespective of initial percentage (Fig. 4). The number of captured IFN-γ upregulated dHL-60 cells increased by nearly 9 times, from 170 cells to 1472 cells, and capture purity reached 95.1% (Fig. 4). It should be noted that when the upregulated cell population was 10% of the total cells, the device was still able to positively measure this increase in the sample.
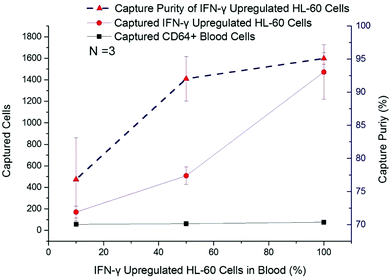 |
| Fig. 4 IFN-γ up-regulated HL-60 cells with portion of 10%, 50%, and 90% were spiked into lysed blood to test concentration effects in cell samples. Absolute captured cell number of IFN-γ up-regulated HL-60 cells and CD64+ blood cells are shown in red dots and black squares, respectively. Capture purity of IFN-γ up-regulated HL-60 cells is shown in red triangles. The capture purity reached 95%. Even with 10% IFN-γ up-regulated HL-60 cells in mixture, the captured cell number was above detection baseline for neutrophil activation. Error bar represents the standard deviation (error bars of the CD64+ cells in aseptic blood samples cannot be seen at this scale). | |
CD64 expression effects on cell capture
The up-regulation of CD64 expression on neutrophils has a specificity of 96% and sensitivity of 98% as an indicator of sepsis.18,42,45,47 Upregulated cells were tested by flow cytometry along with the Sepsis Chip to determine CD64 expression effects on cell capture. Within 24 h after addition of IFN-γ, the expression of CD64 on dHL-60 cells increased along with incubation time, which was in agreement with the aforementioned studies (Fig. S3a†). The number of captured cells also increased with IFN-γ incubation time (Fig. 5a and Fig. S3b†). This result indicated that our device was sensitive to increasing CD64 expression (Fig. S4,† linear regression y = 0.1987x + 129.38, R2 = 0.96). This result was consistent with our previous work on antigen expression and cell capture in cancer cells.56 In this case the y-intercept is not 0 because the average CD64 expression on dHL-60 (IFN-γ upregulated dHL-60 cells at 0 h up-regulation) is a nonzero value. The key finding from this study is that the captured cell number exceeds the detection threshold in 4 hours, which is only one twelfth of the shortest time to run a blood culture in clinical test. While a model for CD64+ expression was used, our method has proven to be sensitive, rapid, and requires a minimal amount of antibody (22 ng of biotin-anti-CD64 per chip).
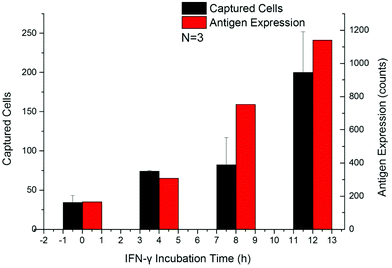 |
| Fig. 5 Temporal changes in CD64 expression of dHL-60 neutrophil-like cells after introducing IFN-γ. A: Flow cytometry from 0 h to 12 h (red) and cell capture (black) were measured every 4 hours. Cell capture was consistent with CD64 expression as a function of time. After 4 hours, the captured cell number exceeded the detection threshold for neutrophil activation, registering as a positive result in the Sepsis Chip. Error bars represent the standard deviation. This chip is therefore capable of detecting neutrophil activation via antigen expression changes. | |
Analysis of septic patient samples
To further evaluate chip performance in detecting sepsis, blood from septic patients and healthy volunteers was tested on the Sepsis Chip. Blood from ten septic patients was obtained within 24 hours. All ten patients were identified as septic using qSOFA methods and began antibiotic treatment. Each patient had a qSOFA score of 2. For the control group using commercial blood, the total captured cell number ranged from 21 to 46. For the healthy volunteer group, the total on-chip captured cell number ranged from 132 to 441. For septic patient samples, the captured cell number in Sepsis Chips ranged from 332 to 1294, which was very statistically significant different from control group and healthy volunteer group (Fig. 6A). We also conducted receiver-operating characteristic (ROC) analysis of current data (Fig. 6B).68 ROC analysis demenstrate the diagnostic ability of a binary classifier system, which in this paper is whether the patient is septic or not. The ROC curve is generated by plotting the true positive rate (Y-axis, also known as Sensitivity) against the false positive rate (X-axis, also known as 1-Specificity) at different threshold settings. Area under ROC curve (AUC) indicates the accuracy of a method. AUC of 1 represents a perfect prediction; an AUC of 0.5 represents a worthless method, just a random selection. In this study, the area under the curve was 0.90, indicating excellent accuracy of our method. Meanwhile, on-chip detection was compared with clinical blood culture for suspected pathogens. Among the 10 of confirmed septic cases, 7 of them had positive blood culture for pathogen identification. However, within 72 h of the study window, only 1 culture sample showed positive results and the rest of 6 were all negative regardless of qSOFA score. The false negative culture results could be due to antibiotics treatment prior to study start or slow growth of pathogens. On the other hand, in all 10 septic patient samples, the on-chip cell capture of CD64+ cells exceeded both commercial samples and healthy volunteer samples, indicating the presence of infection. Each patient was determined clinically to be septic, indicating that bacterial culture is less reliable than clinical parameters. One critical characteristic of sepsis, which distinguishes sepsis from systemic inflammatory response syndrome (SIRS), is that sepsis must results from infections.69 However, qSOFA criteria is a quick bedside prompt that may identify patients with suspected sepsis but it is unable to differentiate sepsis from sterile inflammations.10 Results from on-chip detection of CD64 in our study showed significant upregulation of CD64, which suggesting the occurrence of infection since CD64 is a highly sensitive and specific indicator of infections.70 Moreover, the turnaround time of on-chip detection (about 2 h) is much faster than blood culture (72 h, most cases in this study without positive result). This decrease detection time can allow clinicians to stop antibiotic treatment in time if the case is sterile inflammation rather than sepsis. Although current sample size was small (n = 10 of each sample type), it demonstrates feasibility and a promising potential for sepsis diagnosis. To further validate the performance of Sepsis Chip, we investigated the relationship between CD64 expression and on chip cell capture. We normalized CD64 expression to the ratio of sample/control (sample represents fluorophore stained sample and control represents the same sample without stain) and normalized on-chip captured cell number to capture efficiency to reduce variances caused by chip fabrication, initial cell concentration, instrument setting, etc. The on-chip cell capture efficiency had a linear relationship with the CD64 expression (y = 0.182x + 9.44, R2 = 0.952) (Fig. S6, ESI†). This result was consistent with our previous work on antigen expression and cell capture in cancer cells56 and also in agreement with CD64 expression model in this study.71 Therefore, CD64 expression of an unknown sample can be predicted based on total cell capture on Sepsis Chip. The chip was capable of detecting statistically significant elevation in CD64+ cells, allowing us to identify septic patients in a label free manner.
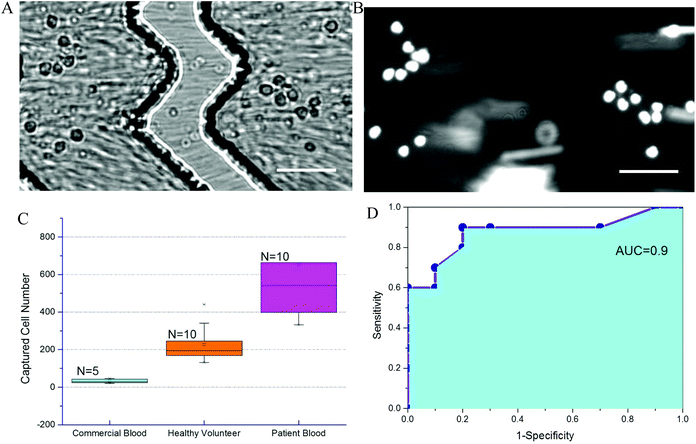 |
| Fig. 6 CD64+ cell capture from septic patient blood samples. (A) White light image of CD64+ leukocytes captured on the anti-CD64 affinity surface. Herringbone structures are seen above the capture surface. Images were recorded during sample introduction; moving cells are visible as streaks in the image. (B) Fluorescence image of captured cells. Leukocytes were stained with for visualization. Scale bar = 50 μm. (C) CD64+ cell capture comparison between sepsis patient, healthy volunteer, and control blood samples. Septic patient blood showed higher CD64+ cell counts than aseptic control blood samples and healthy volunteer samples (n = 10 blood samples for each group). Differences in on-chip cell capture of septic patient sample was very statistically significant control group (p = 0.0019, 95% confidence interval) and very statistically significant from healthy volunteer group (p = 0.0068, 95% confidence interval). Error bars represent the standard deviation in cell counts. (D) Receiver-operating characteristic analysis of blood draw from septic patients. The area under curve is 0.90, which indicated high accuracy of current method. | |
It is important to note that while neutrophils are the primary cell type that increase CD64 expression during sepsis, it is possible that other CD64+ cells are captured from whole blood. It is possible to add reagents such as fluorophore conjugated antibodies to identify captured cells. However, captured cell counts have the advantage of being label free and reduce the instrument demands for readout. In this case, the total cell counts reflects all cells with high expression of CD64. Unlike other clinical methods, our approach can quantify cell counts as a sepsis measurement. A simple microscope or smart-phone based imaging system could be envisioned for point-of-care diagnostics with the Sepsis Chip. Our method demonstrates the first affinity separation method for sepsis early diagnosis validated with patient samples.
Conclusion
We have demonstrated a sepsis detection method using CD64+ cell capture in a microfluidic cell affinity chip. To our knowledge, this is the first reported affinity separation method for sepsis diagnosis validated with patient samples. This method reports total on-chip cell counts as a label free and quantitative detection for sepsis. Compared to flow cytometry, the cost per analysis is less, with reduced operator burden. While this is a single-parameter method, it has shown a strong analytical performance, and it can be coupled with other markers in the future if needed. On-chip tests of blood samples from septic patients showed significant difference from control samples and further proved the applicability and accuracy of the device. Since our chips measure immune system response to sepsis, rather than the pathogens that trigger immune response, they are particularly well suited to situations when the blood culture is negative but sepsis symptoms are present. In the meantime, our method can reduce the rate of false positive sepsis diagnosis due to contamination during pathogen culture, and therefore reduce unnecessary use of broad-spectrum antibiotics. The current validation set from patient samples is small, and future work will further validate the approach. The positive detection of CD64+ cell can be as early as 4 h in a CD64+ expression model and within 24 h for real patient samples. The timing for patient samples was limited by sampling and sample transport, rather than the temporal resolution of the method. In future work, we must consider the phenotype of captured cells and CD64 distribution on the cell surface in order to further improve the assay. In addition, the sample size used for this device validation must be expanded in order to make additional conclusions about diagnostic and prognostic utility. In conclusion, this approach is rapid, can detect neutrophil activation early, and can be operated with any simple microscope at minimum cost. It is promising to apply this approach as an alternative and complementary method to diagnose sepsis especially in time-limited and instrument-limited condition. Our method could reduce the unnecessary use of antibiotics, as the accuracy of the method could reduce false positives. Future work for this method include testing the approach with whole blood and expansion of clinical studies, including studies that focus on sampling timing to determine the earliest detection points for sepsis.
Conflicts of interest
There are no conflicts to declare.
References
- R. P. Dellinger, M. M. Levy, A. Rhodes, D. Annane, H. Gerlach, S. M. Opal, J. E. Sevransky, C. L. Sprung, I. S. Douglas, R. Jaeschke, T. M. Osborn, M. E. Nunnally, S. R. Townsend, K. Reinhart, R. M. Kleinpell, D. C. Angus, C. S. Deutschman, F. R. Machado, G. D. Rubenfeld, S. Webb, R. J. Beale, J. L. Vincent and R. Moreno, Intensive Care Med., 2013, 39, 165–228 CrossRef CAS PubMed.
- A. Gullo, N. Bianco and G. Berlot, Crit. Care Clin., 2006, 22, 489–501 CrossRef PubMed.
- M. M. Levy, R. P. Dellinger, S. R. Townsend, W. T. Linde-Zwirble, J. C. Marshall, J. Bion, C. Schorr, A. Artigas, G. Ramsay, R. Beale, M. M. Parker, H. Gerlach, K. Reinhart, E. Silva, M. Harvey, S. Regan and D. C. Angus, Crit. Care Med., 2010, 38, 367–374 CrossRef PubMed.
- C. Pierrakos and J. L. Vincent, Crit. Care, 2010, 14, R15 CrossRef PubMed.
- E. P. Rivers, A. K. Jaehne, H. B. Nguyen, D. G. Papamatheakis, D. Singer, J. J. Yang, S. Brown and H. Klausner, Shock, 2013, 39, 127–137 CAS.
- M. Singer, C. S. Deutschman and C. Seymour,
et al.
, J. Am. Med. Assoc., 2016, 315, 801–810 CrossRef CAS PubMed.
- K. D. Kochanek, S. L. Murphy, J. Xu and B. Tejada-Vera, Natl. Vital Stat. Rep., 2016, 65, 1–122 Search PubMed.
- N. de Prost, K. Razazi and C. Brun-Buisson, Crit. Care, 2013, 17, 1001–1001 CrossRef PubMed.
- A. E. Jones, N. I. Shapiro, S. Trzeciak, R. C. Arnold, H. A. Claremont and J. A. Kline, J. Am. Med. Assoc., 2010, 303, 739–746 CrossRef CAS PubMed.
- P. Bhattacharjee, D. P. Edelson and M. M. Churpek, Chest, 2017, 151, 898–907 CrossRef PubMed.
- M. Mahalanabis, H. Al-Muayad, M. D. Kulinski, D. Altman and C. M. Klapperich, Lab Chip, 2009, 9, 2811–2817 RSC.
- J. Cohen, J. L. Vincent, N. K. Adhikari, F. R. Machado, D. C. Angus, T. Calandra, K. Jaton, S. Giulieri, J. Delaloye, S. Opal, K. Tracey, T. van der Poll and E. Pelfrene, Lancet Infect. Dis., 2015, 15, 581–614 CrossRef PubMed.
- J. C. Marshall and K. Reinhart, Crit. Care Med., 2009, 37, 2290–2298 CrossRef CAS PubMed.
- J. ten Oever, M. G. Netea and B. J. Kullberg, J. Infect., 2016, 72, 1–18 CrossRef PubMed.
- M. Assicot, D. Gendrel, H. Carsin, J. Raymond, J. Guilbaud and C. Bohuon, Lancet, 1993, 341, 515–518 CrossRef CAS.
- B. M. Tang, G. D. Eslick, J. C. Craig and A. S. McLean, Lancet Infect. Dis., 2007, 7, 210–217 CrossRef CAS PubMed.
- C. Wacker, A. Prkno, F. M. Brunkhorst and P. Schlattmann, Lancet Infect. Dis., 2013, 13, 426–435 CrossRef CAS PubMed.
- J. Cid, R. Aguinaco, R. Sanchez, G. Garcia-Pardo and A. Llorente, J. Infect., 2010, 60, 313–319 CrossRef PubMed.
- M. Kemmler, U. Sauer, E. Schleicher, C. Preininger and A. Brandenburg, Sens. Actuators, B, 2014, 192, 205–215 CrossRef CAS.
- P. Buchegger and C. Preininger, Biomed. Tech. (Berl.), 2013, 58, 4146–4147 Search PubMed.
- P. Buchegger, U. Sauer, H. Toth-Szekely and C. Preininger, Sensors, 2012, 12, 1494–1508 CrossRef CAS PubMed.
- R. Zonneveld, G. Molema and F. B. Plotz, Crit. Care Med., 2016, 44, 218–228 CrossRef CAS PubMed.
- M. M. Caroline, N. Jones, L. Dimisko, A. Alexander, A. Ibrahim, B. A. H. Ibrahim, H. Shaw Warren, R. G. Tompkins, D. I. Shawn and P. Fagan, PLoS One, 2014, 9, e11450–e11466 Search PubMed.
- I. H. Celik, G. Demirel, H. T. Aksoy, O. Erdeve, E. Tuncer, Z. Biyikli and U. Dilmen, Pediatr. Res., 2012, 71, 121–125 CrossRef CAS PubMed.
- A. J. Lee and S.-G. Kim, Blood Res., 2013, 48, 193–197 CrossRef CAS PubMed.
- D. Mardi, B. Fwity, R. Lobmann and A. Ambrosch, Int. J. Lab. Hematol., 2010, 32, 410–418 CAS.
- F. Chaves, B. Tierno and D. Xu, Am. J. Clin. Pathol., 2005, 124, 440–444 CrossRef PubMed.
- B. Senthilnayagam, T. Kumar, J. Sukumaran, M. Jeya and K. R. Rao, Patholog. Res. Int., 2012, 2012, 6 Search PubMed.
- P. J. Cornbleet, Clin. Lab. Med., 2002, 22, 101–136 CrossRef PubMed.
- M. Roussel, A. Gros, A. Gacouin, N. Le Meur, Y. Le Tulzo and T. Fest, Cytometry, Part B, 2012, 82, 345–352 CrossRef PubMed.
- H. Saito, J. Lai, R. Rogers and C. M. Doerschuk, Blood, 2002, 99, 2207–2213 CrossRef CAS PubMed.
- J. P. Duignan, P. B. Collinst, A. H. Johnson and D. Bouchier-Hayes, Br. J. Surg., 1986, 73, 238–240 CrossRef CAS PubMed.
- B. M. Tavares-Murta, M. Zaparoli, R. B. Ferreira, M. L. Silva-Vergara, C. H. Oliveira, E. F. C. Murta, S. H. Ferreira and F. Q. Cunha, Crit. Care Med., 2002, 30, 1056–1061 CrossRef PubMed.
- K. L. Butler, V. Ambravaneswaran, N. Agrawal, M. Bilodeau, M. Toner, R. G. Tompkins, S. Fagan and D. Irimia, PLoS One, 2010, 5, e11921 Search PubMed.
- X. Lu, C. Lv, Y. Qu, Y. Luo, B. Lin, L. Zhan, Y. Song and Z. Fan, Immunol. Lett., 2016, 173, 55–60 CrossRef CAS PubMed.
- P. Ohlsson, M. Evander, K. Petersson, L. Mellhammar, A. Lehmusvuori, U. Karhunen, M. Soikkeli, T. Seppa, E. Tuunainen, A. Spangar, P. von Lode, K. Rantakokko-Jalava, G. Otto, S. Scheding, T. Soukka, S. Wittfooth and T. Laurell, Anal. Chem., 2016, 88, 9403–9411 CrossRef CAS PubMed.
- J. L. Prieto, H. W. Su, H. W. Hou, M. P. Vera, B. D. Levy, R. M. Baron, J. Han and J. Voldman, Lab Chip, 2016, 16, 4333–4340 RSC.
- S. Bose, R. Singh, M. Hanewich-Hollatz, C. Shen, C. H. Lee, D. M. Dorfman, J. M. Karp and R. Karnik, Sci. Rep., 2013, 3, 2329 CrossRef PubMed.
- E. Allen, A. C. Bakke, M. Z. Purtzer and A. Deodhar, Ann. Rheum. Dis., 2002, 61, 522–525 CrossRef CAS PubMed.
- E. de Jong, D. W. de Lange, A. Beishuizen, P. M. van de Ven, A. R. Girbes and A. Huisman, Int. J. Lab. Hematol., 2016, 38, 576–584 CrossRef CAS PubMed.
- G. Fischer, E. M. Schneider, L. L. Moldawer, C. Karcher, E. Barth, H. Suger-Wiedeck, M. Georgieff and M. Weiss, Intensive Care Med., 2001, 27, 1848–1852 CrossRef CAS PubMed.
- A. Gros, M. Roussel, E. Sauvadet, A. Gacouin, S. Marque, L. Chimot, S. Lavoue, C. Camus, T. Fest and Y. Le Tulzo, Intensive Care Med., 2012, 38, 445–452 CrossRef CAS PubMed.
- H. S. Lam, H. M. Cheung, T. C. Poon, R. P. Wong, K. T. Leung, K. Li and P. C. Ng, Clin. Chem., 2013, 59, 1753–1760 CAS.
- S. Li, X. Huang, Z. Chen, H. Zhong, Q. Peng, Y. Deng, X. Qin and J. Zhao, Int. J. Infect. Dis., 2013, 17, e12–e23 CrossRef CAS PubMed.
- O. Livaditi, A. Kotanidou, A. Psarra, I. Dimopoulou, C. Sotiropoulou, K. Augustatou, C. Papasteriades, A. Armaganidis, C. Roussos, S. E. Orfanos and E. E. Douzinas, Cytokine, 2006, 36, 283–290 CrossRef CAS PubMed.
- J. Nuutila, U. Hohenthal, I. Laitinen, P. Kotilainen, A. Rajamaki, J. Nikoskelainen and E. M. Lilius, J. Immunol. Methods, 2007, 328, 189–200 CrossRef CAS PubMed.
- P. Rogina, D. Stubljar, T. Lejko-Zupanc, J. Osredkar and M. Skvarc, Clin. Chem. Lab. Med., 2015, 53, e89–e91 CrossRef CAS PubMed.
- P. C. Ng and H. S. Lam, Curr. Opin. Pediatr., 2006, 18, 125–131 CrossRef PubMed.
- M. Groselj-Grenc, A. Ihan and M. Derganc, Mediators Inflammation, 2008, 2008, 10 Search PubMed.
- M. A. Unger, H. P. Chou, T. Thorsen, A. Scherer and S. R. Quake, Science, 2000, 288, 113–116 CrossRef CAS PubMed.
- P. Li, Y. Gao and D. Pappas, Anal. Chem., 2011, 83, 7863–7869 CrossRef CAS PubMed.
- P. Li, Y. Gao and D. Pappas, Anal. Chem., 2012, 84, 8140–8148 CrossRef CAS PubMed.
- P. Li, Y. T'Ian and D. Pappas, Anal. Chem., 2011, 83, 774–781 CrossRef CAS PubMed.
- W. Li, Y. Gao and D. Pappas, Biomed. Microdevices, 2015, 17, 113 CrossRef PubMed.
-
D. Pappas, in Practical Cell Analysis, John Wiley & Sons, Ltd, 2010, pp. 125–163, DOI:10.1002/9780470688441.ch5.
- Y. Zhang and D. Pappas, Analyst, 2016, 141, 1440–1447 RSC.
- R. A. Fleck, S. Romero-Steiner and M. H. Nahm, Clin. Diagn. Lab. Immunol., 2005, 12, 19–27 CAS.
- S. J. Collins, Blood, 1987, 70, 1233–1244 CAS.
- J. J. Hoffmann, Clin. Chem. Lab. Med., 2009, 47, 903–916 CrossRef CAS PubMed.
- J. J. Hoffmann, Biochem. Med., 2011, 21, 282–290 CrossRef CAS.
- C. Jacob, M. Leport, C. Szilagyi, J. M. Allen, C. Bertrand and V. Lagente, Int. Immunopharmacol., 2002, 2, 1647–1656 CrossRef CAS PubMed.
- A. B. Hauert, S. Martinelli, C. Marone and V. Niggli, Int. J. Biochem. Cell Biol., 2002, 34, 838–854 CrossRef CAS PubMed.
- S. J. Martin, J. G. Bradley and T. G. Cotter, Clin. Exp. Immunol., 1990, 79, 448–453 CrossRef CAS PubMed.
- I. D. Trayner, T. Bustorff, A. E. Etches, G. J. Mufti, Y. Foss and F. Farzaneh, Leuk. Res., 1998, 22, 537–547 CrossRef CAS PubMed.
- S. J. Collins, F. W. Ruscetti, R. E. Gallagher and R. C. Gallo, Proc. Natl. Acad. Sci. U. S. A., 1978, 75, 2458–2462 CrossRef CAS.
- T. N. Ellis and B. L. Beaman, Immunology, 2004, 112, 2–12 CrossRef CAS PubMed.
- D. A. L. Daniel and A. Hammer, Cell Biophys., 1987, 14, 139–173 Search PubMed.
- J. A. Hanley and B. J. McNeil, Radiology, 1982, 143, 29–36 CrossRef CAS PubMed.
- K. Reinhart, M. Bauer, N. C. Riedemann and C. S. Hartog, Clin. Microbiol. Rev., 2012, 25, 609–634 CrossRef CAS PubMed.
- P. R. Bauer, R. Kashyap, S. C. League, J. G. Park, D. R. Block, N. A. Baumann, A. Algeciras-Schimnich, S. M. Jenkins, C. Y. Smith, O. Gajic and R. S. Abraham, Diagn. Microbiol. Infect. Dis., 2016, 84, 175–180 CrossRef CAS PubMed.
- U. Hassan, T. Ghonge, B. Reddy Jr., M. Patel, M. Rappleye, I. Taneja, A. Tanna, R. Healey, N. Manusry, Z. Price, T. Jensen, J. Berger, A. Hasnain, E. Flaugher, S. Liu, B. Davis, J. Kumar, K. White and R. Bashir, A point-of-care microfluidic biochip for quantification of CD64 expression from whole blood for sepsis stratification, 2017, 8, 15949 CAS.
Footnote |
† Electronic supplementary information (ESI) available. See DOI: 10.1039/c7an01471f |
|
This journal is © The Royal Society of Chemistry 2018 |
Click here to see how this site uses Cookies. View our privacy policy here.