DOI:
10.1039/C8RA02311E
(Paper)
RSC Adv., 2018,
8, 19470-19478
1H-NMR metabolomics analysis of nutritional components from two kinds of freshwater fish brain extracts†
Received
16th March 2018
, Accepted 17th May 2018
First published on 29th May 2018
Abstract
There are a number of bioactive compounds in freshwater fish brains, and their functional roles have not been clearly elucidated. NMR-based metabolic profiling could enable rapid characterization of the nutritional composition a fish's brain. Here, two kinds of freshwater fish brains were investigated, crucian carp and yellow catfish. A 1H-NMR based metabolomic approach was used to illustrate the nutritional components of these two kinds of brain. At first, the microwave method was utilized to cease the activity of the enzymes in the brain, and the chemicals were extracted for NMR analysis. These two kinds of brain had significant differences in metabolic patterns, and the chemical compositions of the yellow catfish brain were similar to those of rodent and human brains. Furthermore, most of the different metabolites were significantly higher in the yellow catfish, except for acetamide. This study could provide comprehensive information regarding the utilization of fish heads during processing of fish and dietary nutrition guidance.
1 Introduction
Fish is a food source that contains high-quality proteins, essential fatty acids, vitamins, minerals and trace elements.1 It can provide people with large amounts of animal proteins, especially in developing countries.2 China is the largest country for aquaculture production accounting for 62% of the total production in the world.3 The production of freshwater fish accounts for about 41% of the total aquaculture yields and reached more than 27 million tons in 2016. During the processing of freshwater fish, lots of byproducts are leftover, such as the fish head, bones, skin and scales. In order to improve the value of the freshwater fish byproducts and reduce wasted resources, many research studies have been done, such as making ultrafine fish bone powder from silver carp fishbone;4 fish gelatin from fish scales;5 and collagen extracts from freshwater fish skin,6 etc. However, to the best of our knowledge, there are few reports about the nutritional components of fish brains. The whole fish (including brain) is usually cooked for a meal rather than being processed for industrial use. Different cooking approaches could bleach and firm the brain tissue and change the soft texture for eating. As the most important nervous system, metabolites in the fish brain are very useful for human health,7 even for cosmetics.7,8 Thus it is important to investigate the metabolic profiles of the fish brain, especially using the metabolomics analysis with NMR method.
Metabolomics analysis is the study concerning the metabolic analysis of small molecules in cells, bio-fluids, tissues and organisms.9 Mass spectrometry (MS) and nuclear magnetic resonance (NMR) spectroscopy are two leading analytical approaches for measuring the metabolites in biological samples. Both methods could explore the information of potential metabolites and perform well on metabolic profiling analysis, using global profiling or targeted profiling.10 NMR-based metabolomics has a number of advantages, such as being non-biased, having minimal sample preparation, non-destructive, high throughput and easy quantification. Therefore it is a robust and reliable technique in metabolomics.11,12 Recently, due to being a powerful and versatile tool, NMR based metabolomics have been highlighted in nutrition research.9,10,13
The NMR based global metabolomics methods involved in food nutrition research usually produce high-dimensional and complex data sets. Multivariate pattern recognition techniques including principal component analysis (PCA), partial least squares discriminant analysis (PLS-DA), or orthogonal partial least squares discriminant analysis (OPLS-DA) are often used to reduce the dimensionality of the data.14 These technologies are very useful to screen the key metabolites, which are responsible for discriminating between various groups, to explain the major characteristics among different kinds of samples that are very useful for food nutrition assessment and food function interpretation.9,15–17 Thus, the method of OPLS-DA was used to screen the major metabolites in the freshwater fish brains in the current study.
Among various fish species, two normal kinds of freshwater fish were selected in the current study – crucian carp (Carassius carassius) and yellow catfish (Pelteobagrus fulvidraco). The crucian carp is one of the most important freshwater fish species,18 and the yellow catfish is also one of the most favorite inland aquaculture species in Asian countries.19 These two kinds of freshwater fish are usually prepared to make fish soup using the whole body in many Asia-Pacific countries.19,20 This study investigated the nutritional components of fish brains in two kinds of freshwater fish based on the 1H-NMR metabolic profiling approach. Multivariate pattern recognition methods were used to analyze the 1H-NMR spectra data to identify the overall similarity and different significant metabolites among various groups. 1H-NMR-based metabolic profiling could provide a rapid characterization of the fish brain, thus allowing a better understanding of its nutritional function. Furthermore, it could provide comprehensive information for the use of the fish head in processing and dietary nutrition guidance.
2 Experiments and materials
2.1 Materials
Fresh live crucian carp (Carassius carassius, ∼350 g) and yellow catfish (Pelteobagrus fulvidraco, ∼150 g) were purchased from a local supermarket (Wuhan, Hubei, China) and transferred to the laboratory within 30 minutes. It is well known that the nutritional components of the fish are altered during the post-mortem period, thus the fresh fish is usually prepared on the same day for cooking. In order to avoid changes, the microwave method was employed in the current study. Different kinds of microwaving equipment have been utilized in previous studies, such as the Muromachi Microwave Fixation system (5 kW),21 the microwave fixation system (1 kW),22 and the microwave machine used in daily life (800 W–1 kW).9 Here, the last method is suitable for inactivating enzymes and ceasing the metabolism in the fish head.
Thus, these two kinds of fish were immediately decapitated and the fish heads were washed with tap water and microwaved (90 s in winter, Wuhan, China).21–23 Then, the fish brains were artificially removed and weighed. The tissues were placed into EP (epoxy epoxide) tubes and frozen at −80 °C for further analysis.
2.2 Tissue extraction
The extraction of brain tissue was achieved with the following steps, which were published in former studies.9,21,22 Thus, only brief steps were provided in the current study. The weighted tissue was successively grounded with 0.1 M HCl/methanol (300 μl) and 60% ethanol (v/v, 900 μl) using a tissue-lyser (Qiagen TissueLyser, Retsch GmBH, Germany) with a shaking frequency of 20 Hz for 1.5 min. Then the mixture was centrifuged (10 min, 16
099 g, 4 °C) and the supernatant was collected. The remaining sediment was further extracted twice under the same procedure using 60% ethanol and the supernatants were combined. The combined mixture was lyophilized after evaporating methanol and ethanol in normal vacuum machine under a normal temperature. Then the lyophilized products were re-dissolved and transferred to 5 mm NMR tubes using a D2O buffer (60 μl D2O with K2HPO4 and KH2PO4, 120 mg L−1 3-(trimethylsilyl) propionic-2,2,3,3,d4 acid sodium salt (TSP, 269913-1G, Sigma-Aldrich) as the inner standards, pH = 7.4) and DD water buffer for further 1H NMR analysis.
2.3 1H-NMR analysis
All of the NMR detection was completed on a Bruker Avance III 600 MHz NMR spectrometer equipped with an inverse cryogenic probe (Bruker Biospin, Germany). The proton NMR spectra were acquired with the standard WATERGATE pulse sequence under room temperature (298 K).21 The 90° pulse length was adjusted to 10.1 μs for each sample and 128 transients were collected into 32 k data points over a spectral width of 20 ppm.
Furthermore, a series of two-dimensional (2D) NMR spectra were collected for a random sample in every fish brain, for assignment of the metabolites in the 1H NMR spectra, such as 1H–1H correlation spectroscopy (COSY), 1H–1H total correlation spectroscopy (TOCSY), 1H–13C heteronuclear single quantum correlation (HSQC) and 1H–13C heteronuclear multiple bond correlation (HMBC). All the NMR studies were achieved using the commercial software TOPSPIN (V3.0, BrukerBiospin, Germany).
2.4 NMR data analysis
2.4.1 NMR spectra analysis. All 1H-NMR spectra were pre-processed with a parameter of 1 Hz line broadening. Then the phase and baseline correction were manually corrected in TOPSPIN (Version 2.1, Bruker Biospin). The corrected spectra were automatically imported into the homemade software NMRSpec.24 This software has been utilized for 1H-NMR spectra analysis in several metabolomics studies.23,25 To be more specific, the spectra were initially aligned with the inner standard TSP (0.0 ppm). Then the whole spectra alignment was achieved, then the peak integration with even gaps (0.002 ppm) was calculated. Furthermore, the peak intensity was related to the concentration of the specified chemical and the number of protons. Thus it is also important to integrate the specific regions for measuring the absolute concentration of the metabolites. All these steps were automatically achieved in NMRSpec.
2.4.2 NMR data processing. The weights of the tissues were similar in each group, thus the 1H NMR spectra could be normalized by tissue weight: every spectrum was normalized with tissue weight to generate a normalized spectrum. Then all the normalized spectra in the same group were averaged point by point with the total number of animals in every group after the 1H NMR spectra were aligned with NMRSpec. The average spectrum was utilized to illustrate the comparison of chemicals among different samples. It was calculated from all the samples in the same group, and the detailed calculation procedure was described in former study.23
2.5 Absolute concentration of the metabolites
The weights of the same kinds of fish brain were very similar, thus the absolute concentration of nutritional components could be calculated with the 1H NMR spectra.
The absolute concentrations of nutritional components (μmol g−1 wet weight) were related with the integrated values of the related peak area, which also depended on the number of similar protons and the weight of specimens. Normally, NMR signals from most of the chemicals were overlapped in the NMR spectra. However, there were many peaks with different chemical shifts for the same metabolites, and it is better to select a pure signal, which was not overlapping with other signals. However, if there is no pure region to calculate, then a constant ratio (Rmet) would be used, which would be a constant and calculated from the pure chemicals. If the signals totally overlapped, then it would be impossible to use them to calculate the absolute concentration. The inner standard (TSP) is used for the calculation. The related calculation of absolute concentration (Cmet) is shown in the following:
|
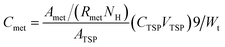 | (1) |
where
ATSP and
Amet are the related peak areas for TSP and the specific chemical;
NH is the number of protons in the related region of (
Amet) for the aim chemicals;
CTSP and
VTSP are the concentration and volume of the inner standard solution added in the NMR tubes for measurement and the constant 9 is the number of proton in TSP;
Wt is the weight of the specimen.
2.6 Data analysis
Multivariate data analysis was performed with the software SPSS (Version 22) and homemade code in MATLAB.
2.6.1 PCA analysis. Principal Component Analysis (PCA) was used as a traditional projection and dimensionality reduction approach. It used the algorithm for transforming the original multi-dimension data into low-dimensional space, and obtained the new, uncorrelated variables called principal components, which retained as much as possible of the information that existed in the original data.26 Each principal component (PC) was a linear combination of the original measurement variables. New subsets of orthogonal variables, which represented the direction of greatest variance in the original data, were found. The reduced features were submitted to the multivariate analysis.27,28 The results of integration of even gaps were used for PCA analysis.
2.6.2 Statistical analysis. All results from the study are presented as Ave. (mean value) ± STD (standard deviation). In order to pursue the metabolic patterns of these two different kinds of brains, the traditional two-sample t-test method was utilized to compare all the intensities of the peak gaps (Δδ = 0.002 ppm). ANOVA (analysis of variance) analyses followed by least significant difference (LSD) post hoc tests were employed to determine the significance of differences for the metabolite levels in these two kinds of brains. Furthermore, orthogonal partial least squares discriminant analysis (OPLS-DA) was used to visually discriminate between the groups. The quality and predictability of the OPLS-DA model was evaluated using R2Y (cum) and Q2 (cum) values respectively. The criterion for statistical significance was a probability value of 0.05.
3 Results and discussion
3.1 Physical properties of microwaved fish brain
In the current study in order to deactivate the brain metabolism, the fish head was initially microwaved. The figures of the two kinds of fish brains under these two different conditions (fresh and microwaved) were illustrated in Fig. 1. Comparing these different fish brains, the fresh brains are redder and shinier, and the microwaved brains are pale and dull. Furthermore, the elasticity of the fresh sample is much stronger and it is pasty during the tissue extraction; However, the microwaved brain is fragile and easy to be triturated. The weights of these two kinds of microwaved fish brains (crucian carp and yellow catfish) were 278.7 ± 37.6 mg and 90.1 ± 20.7 mg, respectively. The difference between the weights of these two kinds of brain was mainly caused by the body weights of the fish species.
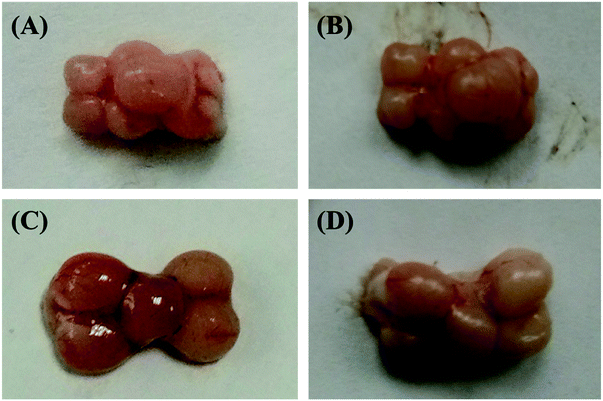 |
| Fig. 1 Comparison of the two kinds of fish brains under fresh and microwaved conditions. (A) Fresh yellow catfish brain; (B) microwaved yellow catfish brain; (C) fresh crucian carp brain; (D) microwaved crucian carp brain. | |
3.2 Nutrition components of freshwater fish brain extracts
Representative one-dimensional 1H-NMR spectra of aqueous fish brain extract samples from two kinds of freshwater fish head are shown in Fig. 2. Most features in these two representative spectra were quite similar, which means the nutritional components measured in these two fish brains were almost similar. However, there have few spectral regions with significant differences. Furthermore, the intensities of the peaks were also different, which represented the different concentrations of these metabolites. As simple visual inspection of NMR spectra did not allow for discrimination of difference involved in the nutrition profile, it was attempted to identify the metabolites in the samples by analyzing the NMR spectra with publications,29 chemical shifts and 2D-NMR spectra at first. For identification, the metabolites from 2D-NMR spectra, COSY and TOCSY spectra were used to measure the proton–proton spin–spin correlation in chemical 1H NMR spectra; HSQC and HMBC spectra illustrated the correlation of heteronuclear (1H and 13C) with one-bond and multiple bond connections in the metabolites. Here, a series of 2D NMR spectra for identification of GABA was collected in Fig. 3. At the end, twenty signals were identified through 1H-NMR spectra of two fish brain extracts, including isoleucine, leucine, valine, lactate, alanine, lysine, GABA (gamma amino butyric acid), acetamide, NAA (N-acetylaspartate), Glx-glutamate + glutamine, glutamate, oxoglutarate, glutamine, methylamine, creatine, choline, phosphocholine, taurine, myo-inositol and glycine. The assignments of 1H-NMR spectra were collected in Table S1.† The variations of the concentrations of metabolites could be linked with the difference in genotypes of fish.
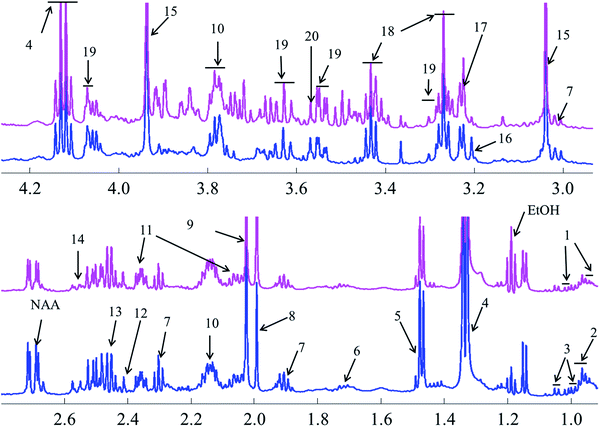 |
| Fig. 2 Comparison of nutrition profiles of the freshwater fish brain using 1H-NMR methods (pink: crucian carp; blue: yellow catfish). Note: (1) isoleucine; (2) leucine; (3) valine; (4) lactate; (5) alanine; (6) lysine; (7) GABA; (8) acetamide; (9) NAA; (10) Glx-glutamate + glutamine; (11) glutamate; (12) oxoglutarate; (13) glutamine; (14) methylamine; (15) creatine; (16) choline; (17) phosphocholine; (18) taurine; (19) myo-inositol; (20) glycine. | |
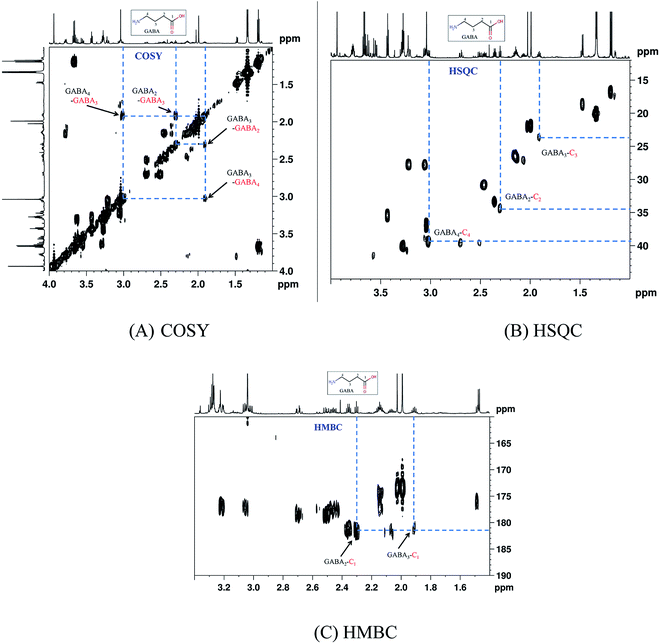 |
| Fig. 3 A series of 2D-NMR spectra for identification of metabolite GABA. (A) COSY; (B) HSQC; (C) HMBC. | |
3.3 PCA analysis of freshwater fish brain extracts
PCA is a classic classification approach requiring no a prior knowledge of the data set and acts to reduce the dimensionality of complicated original data whilst generating information within it.30 To provide comparative interpretations and visualization of these nutritional metabolic differences that exist in the two kinds of fish brain extracts, all the intensities of peaks in the 1H-NMR spectra were submitted for PCA analysis. The PCA score plots showed clear differentiation between two kinds of freshwater brain samples presented in Fig. 4. The result of the PCA gave two major PCs that explained 68.1% of the variation in the original data (49.0%, 19.1%). From this figure, it can be seen that the extractions from different fish brain samples were separated very well in the 2D-space. The nutritional metabolites detected by 1H-NMR spectra of both freshwater fish are representative and are reliable for the assessment of the differences in nutritional metabolic profiling of different foodstuff by PCA model.
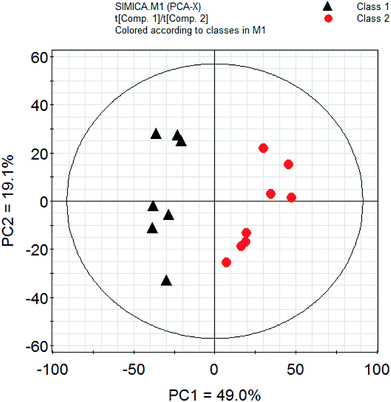 |
| Fig. 4 PCA analysis of nutrition profiles of the freshwater fish brain. Note: class 1: crucian carp; class 2: yellow catfish. | |
3.4 Statistical analysis of the whole nutrition components in NMR spectra
To reveal the differences in the concentrations of metabolites in the two types of freshwater fish brains, the traditional two-sample t-test method was initially used to compare 1H-NMR fingerprint spectra between these two kinds of fish brains (p < 0.05). The results were illustrated in Fig. 5 and S1.† As a supervised pattern recognition approach, the OPLS-DA method has a higher ability for the detection of biomarkers compared to other approaches due to its partial least square algorithm.31 Thus it was also used to screen the metabolic patterns for these two kinds of fish brains, and OPLS-DA scores and coefficient-coded loading plots were collected and used to denote the differential metabolites (Fig. 6). There was a distinguished classification between these two kinds of different fish brains, and the classification model showed good stability and predictability (R2Y and Q2 were 0.952 and 0.915, respectively).
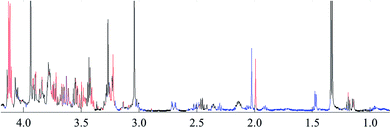 |
| Fig. 5 t-test comparison map of the 1H-NMR for both of freshwater fish brain (p < 0.05). Note: blue: yellow catfish > crucian carp; red: yellow catfish < crucian carp; black: no difference. | |
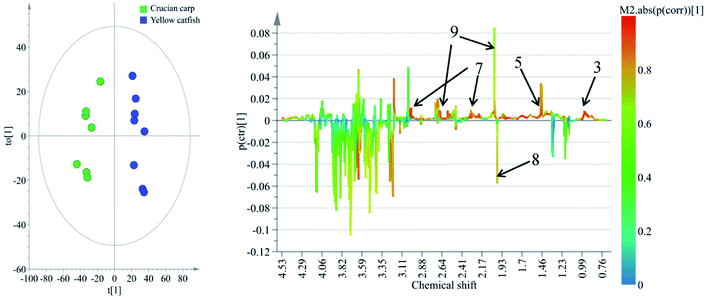 |
| Fig. 6 OPLS-DA scores (left) and coefficient-coded loadings plots (right) for the model, discriminating these two kinds of fish brains. Note: chemical identifications (number) were refer to Fig. 1. | |
From the results of both of the two-sample t-test and OPLS-DA approaches, the whole spectra were significantly divided into two separate spectral parts. The first spectral part was from 0.7 ppm to 3.0 ppm, and the second one is from 3.0 ppm to 4.2 ppm. The concentrations of most of the metabolites in the first spectral region are higher in the yellow catfish brain, except acetamide (Fig. S1†). The signal in 1.2 ppm (triple peaks) is the signal of the group CH3 in ethanol – an impurity during the tissue extraction. The other double peaks beside the ethanol signal were not assigned.
The metabolites in the second region were much higher in the brain of crucian carpand. However, the signals of the chemical in this region seriously overlapped, and it was very difficult to identify the metabolites. Then the absolute concentrations of the assigned metabolites (Fig. 2) were measured and compared (Fig. 7) in the current study.
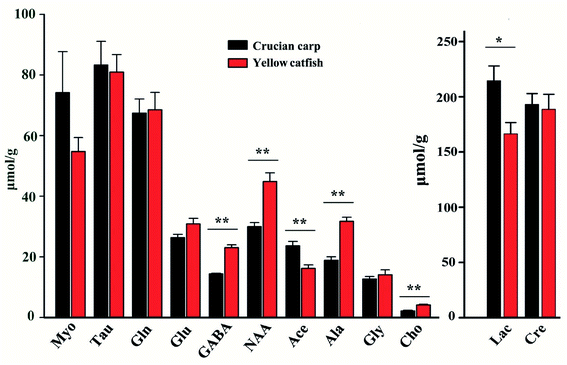 |
| Fig. 7 Comparison of the concentrations (μmol g−1) of metabolites in the fresh water fish brain. | |
3.5 Metabolites patterns of the freshwater fish brain extracts
The concentrations of the assigned metabolites were measured according to the weights of the tissues, peak intensities, number of protons, and the information of the standard chemical TSP. The results were collected in Fig. 7 and Table S2.† The concentrations of the metabolites in these two groups were compared with the classical statistical method ANOVA analyses followed by the least significant difference (LSD) post hoc tests. There was no significant difference among the metabolites of creatine, glycine, taurine, glutamine, glutamate, and myo-inositol in these two kinds of fish brains. Furthermore, the rest of the metabolites were significantly higher (p < 0.05) in the yellow catfish, except for acetamide.
Between these two kinds of fish brains, the yellow catfish has better nutritional effects, as it contains higher concentrations of most metabolites, such as lactate, choline, GABA, NAA, and alanine. There is no meaning regarding the difference in lactate as it is highly related with time since death and it is also generated from the anaerobic respiration of the tissue.
Choline plays a key role in the biosynthesis of the phospholipids in cell membranes, trans-membrane signaling, cholinergic neurotransmission, lipid–cholesterol transport and metabolism, methyl metabolism, are essential nutrients for humans.32 Choline can modulate the basic singling processes within cells as a structural element in membranes, it is crucial in brain development for most living organisms.33
GABA is a non-protein amino acid with four free carbons and it is also considered as the major inhibitory neurotransmitter in CNS, which was involved in the regulation of cardiovascular function and provided positive effects of many neurological disorders such as Huntington's disease, Parkinson's disease, senile dementia, seizures, and Alzheimer's disease34–36 etc. Therefore eating more yellow catfish brain may be beneficial for stressed people or elder people to reduce the risk of neuro-metabolic disorders.
NAA is the second most abundant amino acid in the human brain that is synthesized and predominantly stored in neurons, it undergoes a rapid rise in level during babyhood.37,38 NAA is responsible for the removal of large amounts of water from human brain as a molecular water pump, to assure brain functions.39 In China, there is a dietary appetite for infants to drink yellow catfish soup to contribute to brain development; the high concentration of NAA existing in the fish head may be the best theoretical basis.
Alanine is one of the amino acids used in the biosynthesis of proteins, although it is not essential in humans, it still has many physiological functions for human beings, alanine plays a key role in glucose–alanine cycle between tissues and liver, links to diabetes to assist the glucose metabolism and prevent the occurrence of kidney stone disease.40–42
3.6 Metabolic components comparison with the rodent/human brain
As a normal fish source, it was valuable to compare the nutrition patterns of these two kinds of fish brains with rodent and human brains.
In the rodent and human brains, there are two different significant metabolites, which are aspartate and acetamide. The concentration of aspartate is ∼1.4 μM g−1 in the human brain43 and ∼2.0 μmol g−1 in the rodent brain,44 however, it was undetected in the current study for both of the fish brains. Aspartate is one of the major excitatory amino acids, which mainly exists in high concentrations in the mammalian central nervous system (CNS) and plays a key metabolic role. It undertakes physiological effects by binding different ion-dependent transporters.45–47 Fish belong to the non-mammalian vertebrates,48 they have different nutritional profiles for brain tissue compared to mammals, maybe that is the reason for the phenomena where aspartate has not been detected in the fish brain. The other reason may be the concentration of aspartate existing in the fish brain is too low to reach the limit of detection of NMR.
The chemical acetamide could not be detected using the NMR method in the rodent/human brain, but some acetamide content was measured in the fish brain. Acetamide is a solvent, usually, it is used in the fiber industry as a dye solvent.49 Moreover, acetamide compounds are also used to make pharmaceuticals50 and fungicides,51 it is one of the common chemical materials. It is found in the freshwater fish brain, the reason may be environmental pollution issues.
Besides these two kinds of metabolites, the metabolic pattern of the yellow catfish is much more similar to rodent and human brains.52 The brain of crucian carpand has many unknown metabolites in the regions of 3.1 ppm to 4.0 ppm, and most of the signals overlapped. This information provides valuable information for the neuro-scientific research area, as they should have their own function for the crucian carpand.
4 Conclusion
In the present study, 1H-NMR based metabolomics combined with multivariate analysis was applied to explore the nutritional metabolites involved in two kinds of freshwater fish brains. The microwave method was utilized to cease the activity of the enzymes in the brain, and the metabolic components in the fish brains were identified with the 1H-NMR method. With the help of the PCA data analysis approach, the nutrition components of these two kinds of brains were completely different. Comparing of the metabolite concentrations of these two kinds of fish brains, the metabolic pattern of the yellow catfish is much more similar with the rodent rat brain, and most of the different metabolites are significantly higher in yellow catfish, except for acetamide. The results of the study could provide comprehensive information for the utilization of fish heads in fish processing and dietary nutrition guidance.
Conflicts of interest
All authors declare that they have no conflict of interest.
Ethical approval
All animal (fish) procedures were performed in accordance with the Guidelines for Care and Use of Laboratory Animals of Huazhong Agricultural University and Experiment were approved by the Animal Care and Use Committee of Huazhong Agricultural University.
Informed consent
Not applicable.
Acknowledgements
The authors would like to express their gratitude to Dr Xiaohua Wang (Hubei Provincial Institute for Food Supervision and Test) during the discussion of the data analysis, Mr Bruno Hamish Unger (University of Otago, New Zealand) for the proofreading. This research was supported by National Nature Science Foundation of China (31501495), Fundamental research funds for the Central Universities (2014QC015), Start Fund for New Recruitment of Huazhong Agricultural University (52902), the open project from State Key Laboratory of Magnetic Resonance and Atomic and Molecular Physics in China (T151403).
References
- G. Schaafsma, Nutr. Health, 2008, 19, 229 CrossRef.
- J. L. Li, Z. C. Tu, L. Zhang, D. R. Lin, X. M. Sha, K. Zeng, H. Wang, J. J. Pang and P. P. Tang, J. Food Process. Preserv., 2017, 41, e12995 CrossRef.
- D. Chen, J. Song, H. Yang, S. Xiong, Y. Liu and R. Liu, J. Food Qual., 2016, 39, 707–713 CrossRef.
- T. Yin, H. Du, J. Zhang and S. Xiong, J. Aquat. Food Prod. Technol., 2016, 25, 1045–1055 CrossRef.
- F. Zhang, S. Xu and Z. Wang, Food Bioprod. Process., 2011, 89, 185–193 CrossRef.
- U. H. Mohamad Razali, J. Bakar, D. Mat Hashim and A. Q. Sazili, 3rd IMT-GT International Symposium on Halal Science and Management 2009, 2009 Search PubMed.
- K. Jayathilakan, K. Sultana, K. Radhakrishna and A. S. Bawa, J. Food Sci. Technol., 2012, 49, 278–293 CrossRef PubMed.
- C. E. C. C. Ejike and T. N. Emmanuel, Afr. J. Biochem. Res., 2009, 3, 95–97 Search PubMed.
- F. Savorani, M. A. Rasmussen, M. S. Mikkelsen and S. B. Engelsen, Food Res. Int., 2013, 54, 1131–1145 CrossRef.
- L. Brennan, Prog. Nucl. Magn. Reson. Spectrosc., 2014, 83, 42–49 CrossRef PubMed.
- D. S. Wishart, Trends Anal. Chem., 2008, 27, 228–237 CrossRef.
- D. Yoon, M. Lee, S. Kim and S. Kim, Journal of the Korean Magnetic Resonance Society, 2013, 17, 1–10 CrossRef.
- J. L. Sébédio, J. F. Martin and E. Pujos, Ol., Corps Gras, Lipides, 2008, 15, 341–345 CrossRef.
- S. Hongseok, H. Geumsook, A. Hyukjin, P. Wonmok, L. Cherlho and Y. S. Hong, Food Res. Int., 2009, 42, 1483–1491 CrossRef.
- U. K. Sundekilde, L. B. Larsen and H. C. Bertram, Metabolites, 2013, 3, 204–222 CrossRef PubMed.
- T. M. D. Ebbels and R. Cavill, Prog. Nucl. Magn. Reson. Spectrosc., 2009, 55, 361–374 CrossRef.
- Y. Ye, R. Yang, Y. Lou, J. Chen, X. Yan and H. Tang, J. Food Nutr. Res., 2014, 2, 749–756 CrossRef.
- X. Y. Tang, J. Chen, L. I. Geng geng, L. J. Zhu and Y. F. Dai, Sci. Technol. Food Ind., 2008, 10, 248–255 Search PubMed.
- Y. K. Hong, H. J. Jin, D. Heejin, M. R. Kim, J. S. Choi, D. Harwanto and G. H. Lee, Curr. Nutr. Food Sci., 2017, 13, 16–20 CrossRef.
- Q. Q. Xia and X. U. Hui-Qing, Cuisine Journal of Yangzhou University, 2005, 3, 24–26 Search PubMed.
- J. Wang, H. Y. Du, L. H. Jiang, X. X. Ma, R. A. de Graaf, K. L. Behar and G. F. Mason, Proc. Natl. Acad. Sci. U. S. A., 2013, 110, 14444–14449 CrossRef PubMed.
- J. Wang, H.-L. Zeng, H. Du, Z. Liu, J. Cheng, T. Liu, T. Hu, G. M. Kamal, X. Li, H. Liu and F. Xu, Talanta, 2018, 179, 369–376 CrossRef PubMed.
- H. L. Zeng, Q. Yang, H. Du, H. Li, Y. Shen, T. Liu, X. Chen, G. M. Kamal, Q. Guan, L. Cheng, J. Wang and F. Xu, Oncotarget, 2017, 8, 102020–102032 Search PubMed.
- Y. Liu, J. Cheng, H. L. Liu, Y. H. Deng, J. Wang and F. Q. Xu, Chemom. Intell. Lab. Syst., 2017, 162, 142–148 CrossRef.
- Y. Huo, G. M. Kamal, J. Wang, H. Liu, G. Zhang, Z. Hu, F. Anwar and H. Du, J. Cereal Sci., 2017, 76, 243–252 CrossRef.
- Q. Y. Li and H. Y. Quan, Appl. Mech. Mater., 2014, 687–691, 4037–4041 CrossRef.
- A. C. Dona, M. Kyriakides, F. Scott, E. A. Shephard, D. Varshavi, K. Veselkov and J. R. Everett, Comput. Struct. Biotechnol. J., 2016, 14, 135 CrossRef PubMed.
- D. A. Markowitz, C. E. Curtis and B. Pesaran, Proc. Natl. Acad. Sci. U. S. A., 2015, 112, 11084–11089 CrossRef PubMed.
- V. Govindaraju, K. Young and A. A. Maudsley, NMR Biomed., 2000, 13, 129–153 CrossRef PubMed.
- Y. Jung, J. Lee, J. Kwon, K. Lee, D. H. Ryu and G. Hwang, J. Agric. Food Chem., 2010, 58, 10458–10466 CrossRef PubMed.
- F. Qu, S. J. Zheng, C. S. Wu, Z. X. Jia, J. L. Zhang and Z. P. Duan, Anal. Bioanal. Chem., 2014, 406, 555 CrossRef PubMed.
- S. H. Zeisel, FASEB J., 2000, 16, 669–671 Search PubMed.
- S. H. Z. And and J. K. Blusztajn, Annu. Rev. Nutr., 1994, 14, 269 CrossRef PubMed.
- J. G. Xu, Q. P. Hu, J. L. Duan and C. R. Tian, J. Agric. Food Chem., 2010, 58, 9759–9763 CrossRef PubMed.
- S. H. Oh, Y. J. Moon and C. H. Oh, Prev. Nutr. Food Sci., 2003, 8, 75–78 CrossRef.
- M. Diana, J. Quílez and M. Rafecas, J. Funct. Foods, 2014, 10, 407–420 CrossRef.
- K. B. Jacobson, J. Gen. Physiol., 1959, 43, 323–333 CrossRef PubMed.
- M. H. Baslow, Neurochem. Res., 2003, 28, 941 CrossRef PubMed.
- E. Kolwijck, R. A. Wevers, U. F. Engelke, J. Woudenberg, J. Bulten, H. J. Blom and L. F. Massuger, PLoS One, 2010, 5, e10293 Search PubMed.
- J. Sánchez, Biochem. Biophys. Res. Commun., 2008, 373, 589–592 CrossRef PubMed.
- C. J. Danpure, M. J. Lumb, G. M. Birdsey and X. Zhang, Biochim. Biophys. Acta, 2003, 1647, 70 CrossRef.
- S. C. Kalhan, R. H. Hertz, K. Q. Rossi and S. M. Savin, Metabolism, 1991, 40, 629–633 CrossRef PubMed.
- P. J. Pouwels and J. Frahm, Magn. Reson. Med., 1998, 39, 53 CrossRef PubMed.
- J. Wang, L. Jiang, Y. Jiang, X. Ma and F. M. Graeme, J. Neurochem., 2010, 113, 1447–1458 Search PubMed.
- R. Bodnar and M. M. Heinricher, Neurosurgery, 2016, 1, 3439–3464 Search PubMed.
- M. B. Robinson and L. A. Dowd, Adv. Pharmacol., 1997, 37, 69 Search PubMed.
- J. Lehmann, J. A. Schneider and M. Williams, in Annu. Rep. Med. Chem., ed. D. M.Bailey, Academic Press, 1987, vol. 22, pp. 31–40 Search PubMed.
- R. P. Ruggiero, Y. Bourgeois and S. Boissinot, Front. Genet., 2017, 8, 44 Search PubMed.
- P. N. Everett, United States: N. p., 1989, Web..
- S. Kurhade, S. Kandalkar, R. Kaduskar, B. Kobal, K. Naik, B. Dave, A. Deshpande and D. Bhuniya, WO2011IB53473, 2012.
- R. Salmon, D. Bacon Philip, E. Chrystal James Turner, D. Langton William, A. Knee Jonathan, G. Munns Richard, L. Quaranta, H. G. Brunner, R. Beaudegnies and F. Cederbaum, CA20052583022, 2006.
- R. A. de Graaf, G. M. I. Chowdhury and K. L. Behar, Anal. Chem., 2011, 83, 216–224 CrossRef PubMed.
Footnote |
† Electronic supplementary information (ESI) available. See DOI: 10.1039/c8ra02311e |
|
This journal is © The Royal Society of Chemistry 2018 |