DOI:
10.1039/C8RA03124J
(Paper)
RSC Adv., 2018,
8, 18851-18858
Uncovering the action mechanism of polydatin via network pharmacological target prediction†
Received
12th April 2018
, Accepted 14th May 2018
First published on 23rd May 2018
Abstract
Polydatin (PD), a small natural compound originally extracted from Polygonum cuspidatum, exerts distinct biological functions in a variety of diseases. However, the action mechanism of PD has yet to be systematically explored. In this study, we firstly corroborated the druggability of PD by evaluating the medicinal properties of PD using a TCMSP server. We then conducted in silico target-prediction for PD using PharmMapper and ChemMapper, which led to the identification of 15 potential targets overlapping in both approaches. These 15 targets were subsequently evaluated by GeneMANIA, GO biological process and KEGG pathway analysis, which finally contribute to the construction of a drug-target-pathway network for PD. The network analysis revealed that these targets were mainly associated with cancer, cell growth and apoptosis, hormones and other physiological processes, outlining the pharmacological influences of PD on multiple integrated pathways involved in a particular network.
Introduction
Nowadays, Chinese herb medicine (CHM) has been widely recognized as a valuable resource for innovative drug discovery, since more and more compounds identified from CHM have shown distinct therapeutic activities in various diseases.1,2 Polydatin (PD; 3,4′,5-trihydroxystibene-3-β-mono-D-glucoside; Fig. 1A) is one of the major active compounds originally extracted from the root and rhizome of Hu zhang (Polygonum cuspidatum), a typical CHM. PD could be found in red wine, peanuts, hop cones, cocoa-containing products and many daily diets,3 exhibiting a series of pharmacological activities, such as anti-oxidative, anti-inflammatory, and protective effects in multiple organs (nephron-, hepatic- and cardiovascular system).4–10 Furthermore, recent studies have shown that PD may act as an anti-tumor agent by inhibiting cell proliferation and inducing apoptosis in a variety of tumors.11,12 However, current knowledge about the medicinal characteristics as well as the action mechanism of PD is still fragmented, and its system-level mechanism warrants further investigation.
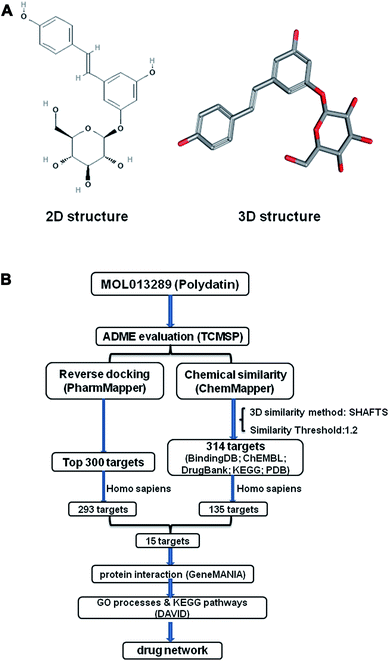 |
| Fig. 1 Chemical structure of polydatin (PD) and roadmap of PD target-identification. (A) Chemical structure of PD downloaded from the ZINC database (ZINC:04098633); (B) technology roadmap for the identification of potential PD targets using ADME evaluation, reverse docking, chemical similarity, GO processes & KEGG pathways analysis, which finally led to a PD-target-pathway network. | |
With the advance in computational methods, bioinformatics has been applied to the target prediction for herb medicines,13 which has significantly promoted drug discovery and corresponding drug design processes, while saving money, time and manpower. Thus, as a natural active compound from CHM, PD is equally suitable for deciphering its system-level mechanism by using in silico target-prediction assisted with network pharmacological analysis.
Here, we started the study with evaluating the medicinal property of PD using TCMSP server. Then, having validated the druggability of PD, we performed compound-target prediction by reverse docking and chemical similarity analysis, respectively. The overlapped targets identified by both approaches were chosen for gene ontology (GO) biological process and KEGG pathway analysis. Finally, we constructed a drug-target-pathway network to provide an overview on mechanisms of PD action, thus achieving a comprehensive understanding of PD at system-level. The technical route for identifying the potential targets of PD is shown in Fig. 1B.
Results
ADME-related parameters of polydatin (PD)
ADME-related parameters of PD were explored by TCMSP, which provides the details on important ADME-related parameters such as human oral bioavailability (OB), drug-likeness (DL), Caco-2 permeability (Caco-2), blood–brain barrier permeability (BBB) and Lipinski's rule of five (MW, Alog
P, TPSA, Hdon, Hacc).14 Generally, the concept of DL has been widely used to filter out drug compounds with unsatisfactory characteristics, especially those with poor ADMET-related profiles.15 The average value of DL for Drugbank compounds ≥0.18 has been reported as a standard for screening bioactive drug compounds in systematic analysis of TCM.14,16,17 As shown in Table 1, the DL value of PD was calculated to be 0.5 by TCMSP, indicating PD possessed a good drug-likeness.
Table 1 Pharmacological and molecular parameters of PD
MOL_ID |
Name |
MW |
Alog P |
Hdon |
Hacc |
OB (%) |
Caco-2 |
BBB |
DL |
TPSA |
RBN |
MOL013289 |
Polydatin |
390.42 |
1.11 |
6 |
8 |
21.44 |
−0.9 |
−1.81 |
0.5 |
139.84 |
5 |
Lipinski's rule of five identifies the important characteristics requested to oral delivery for drug compounds, which include the molecular weight (MW) < 500 daltons (Da), the calculated log
P (Clog
P) < 5 (or Mlog
P > 4.15), number of hydrogen-bond donors < 5 and number of hydrogen-bond acceptors < 10, respectively.18,19 Currently, the “rule of five” is commonly referred to as a “druglike” guideline in drug lead optimisation.20 As listed in Table 1, our result showed that the PD's characteristics met most of the requirements, suggesting PD is a good candidate for drug development.
Identification of potentially drug targets
We used two different approaches as described in the method to predict the potentially drug targets. Fig. 1B showed the 293 potentially protein targets of PD from over 7000 receptor-based pharmacophore models obtained using PharmMapper. Likewise, the potential 314 targets were predicted using ChemMapper. In a ChemMapper job, upon selection of the Homo sapiens, 135 protein targets met this requirement were identified. Finally, a total of 15 potentially protein targets overlapped in both sets of results were selected for further analysis (Table 2).
Table 2 Potentially drug targets of PD identified by PharmMapper and ChemMapper
Rank |
PDB ID |
Name |
Target gene |
1 |
1IVO |
Epidermal growth factor receptor |
EGFR |
2 |
1P49 |
Steryl-sulfatase |
STS |
3 |
1FDT |
Estradiol 17-beta-dehydrogenase 1 |
HSD17B1 |
4 |
3OS8 |
Estrogen receptor |
ESR1 |
5 |
1NDE |
Estrogen receptor beta |
ESR2 |
6 |
1H10 |
RAC-alpha serine/threonine-protein kinase |
AKT1 |
7 |
2XYG |
Caspase-3 |
CASP3 |
8 |
4GUB |
Casein kinase II subunit alpha |
CSNK2A1 |
9 |
3IXK |
Beta-secretase 1 |
BACE1 |
10 |
1H92 |
Tyrosine-protein kinase Lck |
LCK |
11 |
1CW3 |
Aldehyde dehydrogenase, mitochondrial |
ALDH2 |
12 |
2QM9 |
Fatty acid-binding protein, adipocyte |
FABP4 |
13 |
5EA2 |
NAD(P)H dehydrogenase [quinone] 1 |
NQO1 |
14 |
1WSZ |
Histo-blood group ABO system transferase |
ABO |
15 |
2QMY |
Ribosyldihydronicotinamide dehydrogenase [quinone] |
NQO2 |
Analysis by GeneMANIA
To find additional genes that may have missed, the 15 candidate targets identified in above screening were further analyzed using GeneMANIA, which offers a simple, intuitive web interface to show the composite gene–gene functional interactions from a input gene list, and extends the gene list by finding more genes and/or gene products that are most related to them, and shows how they are networked.21 Our result revealed that among the 15 drug targets and 20 interacting proteins, 43.32% shared similar protein domains and 20.09% shared the same reaction within a pathway. Other results characteristics were co-expression, predicted, physical interactions and co-localization, respectively (Fig. 2). All above results suggested that GeneMANIA represents a high accuracy of prediction algorithm.
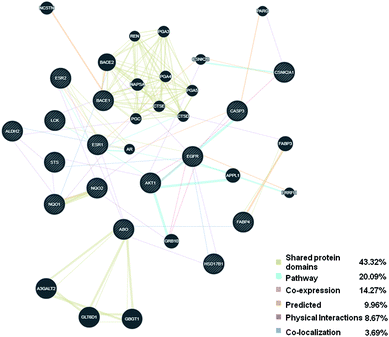 |
| Fig. 2 Network of potential PD targets. Black protein nodes refer to protein targets, and different connecting colors represent different correlations. Functional association of protein targets were analyzed by GeneMANIA. | |
GO and KEGG pathway analysis, and network construction
To further explore the action mechanism of PD, analysis on interaction network for its 15 potential protein targets was performed by DAVID Bioinformatics Resources 6.8. According to the significance of P value, the top five processes involving in the PD action include positive regulation of nitric oxide biosynthetic process (GO:0045429), positive regulation of neuron apoptotic process (GO:0043525), regulation of phosphatidylinositol 3-kinase (PI3-K) signaling (GO:0014066), positive regulation of cell growth (GO:0030307) and response to UV-A (GO:0070141) (Table 3 and Fig. 3A). Some of the mechanisms mentioned above have been reported in other studies, such as the PI3-K signaling dependent mechanisms, through which a prominent nephroprotective effects against renal I/R injury has been documented previously.22 Meanwhile, these 15 targets participate in five KEGG pathways including estrogen signaling pathway (hsa04915), proteoglycans in cancer (hsa05205), prolactin signaling pathway (hsa04917), MAPK signaling pathway (hsa04010) and endometrial cancer (hsa05213) (Table 4 and Fig. 3B). Notably, all of these pathways were associated with cancer-related diseases, in line with the notion that PD showed the potential as anti-tumor agent.11,12
Table 3 GO analysis of PD potentially targets
Term |
P value |
GO:0045429 – positive regulation of nitric oxide biosynthetic process |
5.72 × 10−4 |
GO:0043525 – positive regulation of neuron apoptotic process |
5.72 × 10−4 |
GO:0014066 – regulation of phosphatidylinositol 3-kinase signaling |
0.0018695 |
GO:0030307 – positive regulation of cell growth |
0.002164 |
GO:0070141 – response to UV-A |
0.00249926 |
GO:0032355 – response to estradiol |
0.0025336 |
GO:0051091 – positive regulation of sequence-specific DNA binding transcription factor activity |
0.0033557 |
GO:0048015 – phosphatidylinositol-mediated signaling |
0.0034186 |
GO:0043066 – negative regulation of apoptotic process |
0.00575773 |
GO:0031659 – positive regulation of cyclin-dependent protein serine/threonine kinase activity involved in G1/S transition of mitotic cell cycle |
0.00665179 |
GO:0006809 – nitric oxide biosynthetic process |
0.01078828 |
GO:0007165 – signal transduction |
0.01299481 |
GO:0097194 – execution phase of apoptosis |
0.01326248 |
GO:0030520 – intracellular estrogen receptor signaling pathway |
0.0173734 |
GO:0051000 – positive regulation of nitric-oxide synthase activity |
0.01819367 |
GO:0050999 – regulation of nitric-oxide synthase activity |
0.0214684 |
GO:0071392 – cellular response to estradiol stimulus |
0.02554755 |
GO:0045907 – positive regulation of vasoconstriction |
0.02636148 |
GO:0071364 – cellular response to epidermal growth factor stimulus |
0.02717479 |
GO:0007611 – learning or memory |
0.02961091 |
GO:0038128 – ERBB2 signaling pathway |
0.03123184 |
GO:0030163 – protein catabolic process |
0.03365853 |
GO:0048146 – positive regulation of fibroblast proliferation |
0.04410908 |
GO:0071407 – cellular response to organic cyclic compound |
0.04810051 |
GO:0048661 – positive regulation of smooth muscle cell proliferation |
0.04889694 |
GO:0050727 – regulation of inflammatory response |
0.0512825 |
GO:0006468 – protein phosphorylation |
0.05398018 |
GO:0043154 – negative regulation of cysteine-type endopeptidase activity involved in apoptotic process |
0.05603698 |
GO:0071260 – cellular response to mechanical stimulus |
0.05761688 |
GO:0050729 – positive regulation of inflammatory response |
0.05919432 |
GO:0031295 – T cell costimulation |
0.06312722 |
GO:0042060 – wound healing |
0.0646961 |
GO:0006919 – activation of cysteine-type endopeptidase activity involved in apoptotic process |
0.06704484 |
GO:0046854 – phosphatidylinositol phosphorylation |
0.07561013 |
GO:0007169 – transmembrane receptor protein tyrosine kinase signaling pathway |
0.07715958 |
GO:0006469 – negative regulation of protein kinase activity |
0.07947925 |
GO:0055114 – oxidation-reduction process |
0.08536772 |
GO:0006979 – response to oxidative stress |
0.08793844 |
GO:0030168 – platelet activation |
0.09175962 |
GO:1901796 – regulation of signal transduction by p53 class mediator |
0.0986003 |
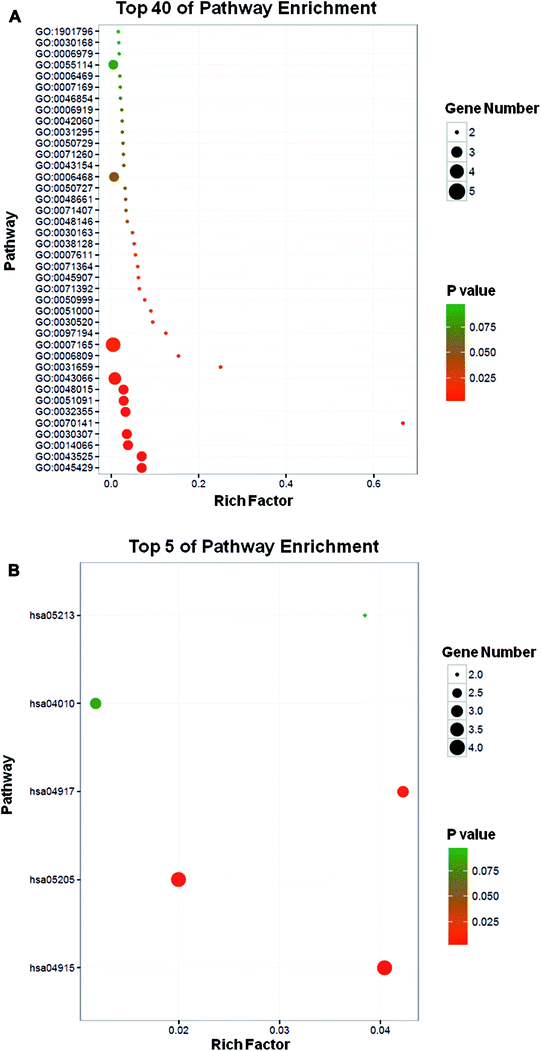 |
| Fig. 3 Enrichment analysis. (A) GO enrichment analysis map of potential PD targets by DAVID Bioinformatics Resources 6.8; (B) KEGG enrichment analysis map of potential PD targets by DAVID Bioinformatics Resources 6.8. | |
Table 4 KEGG analysis of PD potentially targets
Term |
P value |
hsa04915: estrogen signaling pathway |
7.35 × 10−4 |
hsa05205: proteoglycans in cancer |
0.00551241 |
hsa04917: prolactin signaling pathway |
0.007546708 |
hsa04010: MAPK signaling pathway |
0.08096943 |
hsa05213: endometrial cancer |
0.093609348 |
Based on target predicting and pathway enrichment analysis, a systematic network was constructed using Cytoscape 3.2.1. As shown in Fig. 4, the interaction network contains 61 nodes and 125 edges. Interestingly, we found that EGFR, CASP3, AKT1, and ESR1 were identified as potential hub proteins in this network. Notably, the functions and pathways associated with these hub nodes play important roles in different diseases, such as cardiovascular disease and cancer.
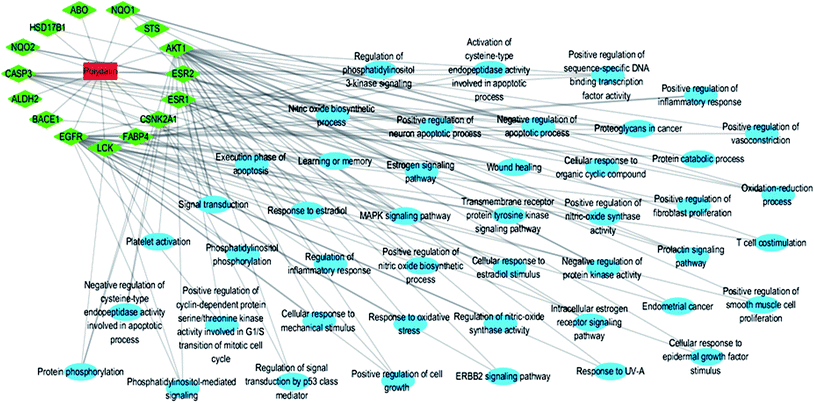 |
| Fig. 4 Polydatin-target-pathway network. Red rectangle = polydatin; green diamond = protein targets; blue oval = pathways. | |
Discussion
Nowadays, by using the methods of network pharmacology and bioinformatics, the strengths to speculate and model pharmacokinetic, toxicity and metabolic endpoints have been greatly improved, leading to an accelerated process of drug discovery. As drug toxicity and poor pharmacokinetics are the major causes of failures during drug development, it has been widely accepted that these technical aspects should be prioritized in the drug discovery process.18 Here, we started the study with evaluating the medicinal property of PD using TCMSP server, suggesting PD is a good candidate for drug development.
So far, a myriad of in silico target predicting methods have been developed and widely used in the field of drug research.23 In the present study, we employed PharmMapper and ChemMapper bioinformatic tools for target prediction, through which we identified 15 potential targets of PD overlapped by using both algorithms. Among these 15 putative targets, some have been well documented previously. For instance, the epidermal growth factor receptor (EGFR) is a transmembrane receptor that belongs to the family of receptor tyrosine kinases (RTK).24 It could be activated by a series of ligands, leading to the activation of many signaling pathways related to control proliferation, differentiation, and survival.25 In particularly, EGFR plays an important role in carcinogenesis.26,27 In vitro experimental assays revealed that PD could reduce the phosphorylation of EGFR in ovarian cancer cell lines.28 Moreover, Caspase-3 (CASP3), as a member of Caspases, is an executioner that implements apoptosis.29,30 It mediates apoptosis by destroying a series of cellular substrates, and activates the degradation of DNA.30,31 E2 binds to its classical receptors-estrogen receptor 1 (ESR1) and estrogen receptor 2 (ESR2) and triggers genomic and non-genomic actions.32,33 The detailed mechanisms of E2/ERs interactions contain ligand binding, receptor dimerization and activation of estrogen response elements (ERES) in target genes, leading to activation or repression of related genes.34,35 Resveratrol, as an analogue of PD, can be used to treat infertility at low concentration, while exhibiting potential benefit to reduce proliferation of human endometrium at high doses.36 Furthermore, the GeneMANIA results (Fig. 2) provided additional information on shared protein domains, pathway, co-expression, indicating these targets and their interacting proteins may have the same or similar functions.
In recent years, studies on PD have revealed that it plays important roles in a wide range of cellular activities, such as anti-thrombotic formation and anti-neoplastic, strengthening the systolic and diastolic function of cardiac myocytes, improving microcirculation of important organs postshock.37–45 Indeed, our drug-target-pathway network based on the target-prediction and GO/KEGG pathway analysis showed that PD possesses multiple protein targets that involve in an array of interactive pathways. Thus, we have reasons to believe that PD is associated with a variety of pharmacological activities against many diseases.
Materials and methods
Evaluation of drug-likeness (DL) by TCMSP server
The TCMSP server (http://ibts.hkbu.edu.hk/LSPtcmsp.php) is a unique systems-level pharmacology platform for TCM that can calculate ADME-related characteristics for naturally occurring compounds.14 It contains chemicals, targets, drug-target networks and associated drug-target-disease networks. Meanwhile, it also provides a systematic evaluation about pharmacokinetic properties for natural compounds, which included drug-likeness (DL), oral bioavailability (OB), Caco-2 permeability (Caco-2), blood–brain barrier permeability (BBB) and Lipinski's rule of five (MW, Alog
P, TPSA, Hdon, Hacc).14,16,17
DL is a qualitative notion used in drug design, and it also helps to optimize pharmacokinetic and pharmaceutical properties, such as chemical stability and solubility. A database-dependent model is constructed on account of the molecular descriptors and Tanimoto coefficient.16,17
Oral bioavailability (OB) is also one of the most important pharmacokinetic properties of medicine by orally because it plays an key role for the efficiency of the drug delivery to the systemic circulation in human body. Its value was evaluated by OBioavail 1.1 model in the TCMSP database.14,16 Furthermore, the Caco-2 permeability prediction model preCaco-2 could solve a problem for oral medicine, that was movement across the intestinal epithelial barrier, which determines the extent and rate of human drug absorption and eventually affects its bioavailability. This model was used in the TCMSP database.14,16 In this research, the drug name “Polydatin (PD)” was entered as the search term, and its druggability was analyzed at the molecular level.
Identification of potentially drug targets by PharmMapper and ChemMapper
PharmMapper, as a reverse docking server, can identify the potentially protein target candidates for the given probe small molecules using pharmacophore mapping approach.46 For a given compound, it can provide the top 300 targets, sorted by fit score in descending order. Meanwhile, the ChemMapper server can speculate potentially protein targets for small molecules by chemical similarity analysis.47 Both are powerful tools for potential drug targets prediction.
Analysis by GeneMANIA
GeneMANIA uses a database of organism-specific weighted networks to construct the resulting composite network, and it can analyze gene lists, generating hypotheses about gene function and prioritising genes for functional assays.21,48 After selecting Homo sapiens from the nine optional organisms, the candidate gene was entered into the search bar and the results were emerged.
GO and KEGG pathway analysis, and network construction
The functional annotation tool DAVID Bioinformatics Resources 6.8, as a web-based software, can be utilized to help comprehend relationships within gene expression data and provide visual systematization information on the candidate gene. Potentially protein targets were uploaded to the DAVID Bioinformatics Resources 6.8 server (https://david.ncifcrf.gov/summary.jsp) following the online instructions, and GO and KEGG pathway detailed information for PD was generated and analyzed. For a deeper understanding of the complex relationships among drug, protein targets and pathways, we constructed a PD-target-pathway network, and analyzed them using Cytoscape 3.2.1.
Conclusions
In the present study, the DL of PD was calculated by TCMSP server, and our result showed that PD was a good drug candidate with a DL value of 0.5. Next, we performed targets prediction by using PharmMapper and ChemMapper, and the resultant 15 overlapped targets identified by using both algorithms were chosen for further analysis. Further GO and KEGG pathway analysis were performed and a drug-target-pathway network of PD action was finally constructed. Our results showed that PD exhibited many functions in anticancer, metabolic, endocrine and other physiological activities. Together, this work provides a visual systematization overview of PD involved signaling pathways and molecular mechanisms of action.
Conflicts of interest
The authors declare no conflicts of interest in association with this manuscript.
List of abbreviations
PD | Polydatin |
CHM | Chinese herb medicine |
OB | Oral bioavailability |
DL | Drug-likeness |
Caco-2 | Caco-2 permeability |
BBB | Blood–brain barrier permeability |
MW | Molecular weight |
Clog P | Calculated log P |
PI3-K | Phosphatidylinositol 3-kinase |
EGFR | Epidermal growth factor receptor |
RTK | Receptor tyrosine kinases |
Acknowledgements
This study was funded by the National Natural Science Foundation of China (No. 81572416), the Tianjin Natural Science Funds (No. 15JCYBJC54500), the National Key Technologies R&D Program of China (No. 2016YFC1303200), and the Tianjin Medical University Cancer Institute & Hospital Cancer Translational Medicine Seed Funds (No. 1701-1).
Notes and references
- M. Schenone, V. Dancik, B. K. Wagner and P. A. Clemons, Nat. Chem. Biol., 2013, 9, 232–240 CrossRef PubMed.
- B. Shen, Cell, 2015, 163, 1297–1300 CrossRef PubMed.
- Q. H. Du, C. Peng and H. Zhang, Pharm. Biol., 2013, 51, 1347–1354 CrossRef PubMed.
- P. Li, X. Wang, M. Zhao, R. Song and K. S. Zhao, Expert Opin. Ther. Targets, 2015, 19, 997–1010 CrossRef PubMed.
- X. Wang, R. Song, Y. Chen, M. Zhao and K. S. Zhao, Expert Opin. Invest. Drugs, 2013, 22, 169–179 CrossRef PubMed.
- Q. H. Meng, H. B. Liu and J. B. Wang, Food Chem. Toxicol., 2016, 96, 215–225 CrossRef PubMed.
- Q. Miao, S. Wang, S. Miao, J. Wang, Y. Xie and Q. Yang, Phytomedicine, 2011, 19, 8–12 CrossRef PubMed.
- L. T. Liu, G. Guo, M. Wu and W. G. Zhang, Chin. J. Integr. Med., 2012, 18, 714–719 CrossRef PubMed.
- J. Du, L. N. Sun, W. W. Xing, B. K. Huang, M. Jia, J. Z. Wu, H. Zhang and L. P. Qin, Phytomedicine, 2009, 16, 652–658 CrossRef PubMed.
- Y. Ma, X. Gong, Y. Mo and S. Wu, Int. J. Mol. Med., 2016, 37, 1652–1660 CrossRef PubMed.
- S. Chen, J. Tao, F. Zhong, Y. Jiao, J. Xu, Q. Shen, H. Wang, S. Fan and Y. Zhang, PLoS One, 2017, 12, e0176501 Search PubMed.
- H. Li, B. Shi, Y. Li and F. Yin, J. Biochem. Mol. Toxicol., 2017, 31, e21900 CrossRef PubMed.
- H. Liu, S. Zhan, Y. Zhang, Y. Ma, L. Chen, L. Chen, H. Dong, M. Ma and Z. Zhang, BMC Complement Altern. Med., 2017, 17, 491 CrossRef PubMed.
- J. Ru, P. Li, J. Wang, W. Zhou, B. Li, C. Huang, P. Li, Z. Guo, W. Tao, Y. Yang, X. Xu, Y. Li, Y. Wang and L. Yang, J. Cheminf., 2014, 6, 13 Search PubMed.
- S. Tian, J. Wang, Y. Li, D. Li, L. Xu and T. Hou, Adv. Drug Delivery Rev., 2015, 86, 2–10 CrossRef PubMed.
- T. Pei, C. Zheng, C. Huang, X. Chen, Z. Guo, Y. Fu, J. Liu and Y. Wang, J. Ethnopharmacol., 2016, 190, 272–287 CrossRef PubMed.
- W. Zhang, Q. Tao, Z. Guo, Y. Fu, X. Chen, P. A. Shar, M. Shahen, J. Zhu, J. Xue, Y. Bai, Z. Wu, Z. Wang, W. Xiao and Y. Wang, Sci. Rep., 2016, 6, 32400 CrossRef PubMed.
- H. Van de Waterbeemd and E. Gifford, Nat. Rev. Drug Discovery, 2003, 2, 192–204 Search PubMed.
- C. A. Lipinski, F. Lombardo, B. W. Dominy and P. J. Feeney, Adv. Drug Delivery Rev., 2001, 46, 3–26 CrossRef PubMed.
- P. D. Leeson, Adv. Drug Delivery Rev., 2016, 101, 22–33 CrossRef PubMed.
- D. Warde-Farley, S. L. Donaldson, O. Comes, K. Zuberi, R. Badrawi, P. Chao, M. Franz, C. Grouios, F. Kazi, C. T. Lopes, A. Maitland, S. Mostafavi, J. Montojo, Q. Shao, G. Wright, G. D. Bader and Q. Morris, Nucleic Acids Res., 2010, 38(suppl.), W214–W220 CrossRef PubMed.
- H. B. Liu, Q. H. Meng, C. Huang, J. B. Wang and X. W. Liu, Oxid Med Cell Longev., 2015, 2015, 362158 Search PubMed.
- A. Cereto-Massague, M. J. Ojeda, C. Valls, M. Mulero, G. Pujadas and S. Garcia-Vallve, Methods, 2015, 71, 98–103 CrossRef PubMed.
- J. Schlessinger, Cell, 2002, 110, 669–672 CrossRef PubMed.
- K. Karin and S. Maria, Int. J. Mol. Sci., 2016, 17, 30 Search PubMed.
- S. Napolitano and F. Ciardiello, Clin. Cancer Res., 2018, 24, 727–729 CrossRef PubMed.
- N. T. Solberg, J. Waaler, K. Lund, L. Mygland, P. A. Olsen and S. Krauss, Mol. Cancer Res., 2018, 16, 543–553 CrossRef PubMed.
- S. J. Hogg, K. Chitcholtan, W. Hassan, P. H. Sykes and A. Garrill, Obstet. Gynecol. Int., 2015, 2015, 279591 Search PubMed.
- M. O. Hengartner, Nature, 2000, 407, 770–776 CrossRef PubMed.
- R. M. Friedlander, N. Engl. J. Med., 2003, 348, 1365–1375 CrossRef PubMed.
- Y. Shi, Mol. Cell, 2002, 9, 459–470 CrossRef PubMed.
- K. Grandien, Mol. Cell. Endocrinol., 1996, 116, 207–212 CrossRef PubMed.
- P. T. Saunders, S. M. Maguire, J. Gaughan and M. R. Millar, J. Endocrinol., 1997, 154, R13–R16 Search PubMed.
- F. Mauvais-Jarvis, D. J. Clegg and A. L. Hevener, Endocr. Rev., 2013, 34, 309–338 CrossRef PubMed.
- L. A. Helguero, M. H. Faulds, J. A. Gustafsson and L. A. Haldosén, Oncogene, 2005, 24, 6605–6616 CrossRef PubMed.
- S. C. Amaya, R. F. Savaris, C. J. Filipovic, J. D. Wise, E. Hestermann, S. L. Young and B. A. Lessey, Reprod. Sci., 2014, 21, 1362–1369 CrossRef PubMed.
- J. P. Gao, C. X. Chen, W. L. Gu, Q. Wu, Y. Wang and J. Lü, Fitoterapia, 2010, 81, 953–960 CrossRef PubMed.
- L. P. Zhang, H. J. Ma, H. M. Bu, M. L. Wang, Q. Li, Z. Qi and Y. Zhang, Shengli Xuebao, 2009, 61, 367–372 Search PubMed.
- P. W. Zhang, C. L. Yu, Y. Z. Wang, S. F. Luo, L. S. Sun and R. S. Li, Acta Pharmacol. Sin., 1995, 16, 265–268 Search PubMed.
- C. Wang, Y. Luo, J. Lu, Y. Wang and G. Sheng, J. Biochem. Mol. Toxicol., 2016, 30, 200–205 CrossRef PubMed.
- Y. Zhang, Z. Zhuang, Q. Meng, Y. Jiao, J. Xu and S. Fan, Oncol. Lett., 2014, 7, 295–301 CrossRef PubMed.
- X. Wang, R. Song, Y. Chen, M. Zhao and K. S. Zhao, Expert Opin. Invest. Drugs, 2013, 22, 169–179 CrossRef PubMed.
- X. Wang, R. Song, H. N. Bian, U. T. Brunk, M. Zhao and K. S. Zhao, Am. J. Physiol.: Regul., Integr. Comp. Physiol., 2012, 302, R805–R814 CrossRef PubMed.
- Y. Cheng, H. T. Zhang, L. Sun, S. Guo, S. Ouyang, Y. Zhang and J. Xu, Brain Res., 2006, 1110, 193–200 CrossRef PubMed.
- Q. Miao, S. Wang, S. Miao, J. Wang, Y. Xie and Q. Yang, Phytomedicine, 2011, 19, 8–12 CrossRef PubMed.
- X. F. Liu, S. S. Ouyang, B. Yu, Y. Liu, K. Huang, J. Gong, S. Zheng, Z. Li, H. Li and H. Jiang, Nucleic Acids Res., 2010, 38, W609–W614 CrossRef PubMed.
- J. Gong, C. Cai, X. Liu, X. Ku, H. Jiang, D. Gao and H. Li, Bioinformatics, 2013, 29, 1827–1829 CrossRef PubMed.
- J. Montojo, K. Zuberi, H. Rodriguez, G. D. Bader and Q. Morris, F1000Research, 2014, 3, 153 Search PubMed.
Footnote |
† Electronic supplementary information (ESI) available: Table S1: the potentially protein targets of PD predicted by PharmMapper and ChemMapper. See DOI: 10.1039/c8ra03124j |
|
This journal is © The Royal Society of Chemistry 2018 |
Click here to see how this site uses Cookies. View our privacy policy here.