DOI:
10.1039/C8RA03629B
(Paper)
RSC Adv., 2018,
8, 24932-24941
LC-MS-based plasma metabolomics reveals metabolic variations in ovariectomy-induced osteoporosis in female Wistar rats†
Received
27th April 2018
, Accepted 24th June 2018
First published on 11th July 2018
Abstract
Osteoporosis with a reduction in bone mineral density has become one of the most common metabolic bone diseases. Postmenopausal women are a high-risk group that suffers from osteoporosis when the production of estrogen in their body rapidly declines. Early prediction and diagnosis of osteoporosis will be conducive to having a greater chance to control the deterioration in condition of osteoporosis patients; however, there are still no effective measures in practice. In this study, we aimed at exploring metabolic variations in postmenopausal osteoporosis using ovariectomized female rats as an animal model. The research was performed using liquid chromatography/mass spectrometry (LC/MS) combined with multivariate statistical analysis for plasma metabolome analysis. The results reveal that metabolic variations of ovariectomized-induced osteoporosis involve 18 differentially expressed metabolites and 13 related metabolism pathways such as valine, leucine and isoleucine biosynthesis as well as arachidonic acid and glycerophospholipid metabolism. Notably, the ingenuity pathway analysis platform for further understanding the relationship between metabolic alteration and osteoporosis shows that the change in the levels of metabolites mainly lead to the abnormal state of cellular compromise, cell signaling, inflammation, molecular transport and lipid metabolism. Metabolomics, as a novel way to characterize resolute endogenous small metabolites in organisms, describes the variation in the early stages of metabolic alteration for offering valuable information on pathogenic mechanisms.
1. Introduction
As a progressive bone disease, osteoporosis has become a global medical issue, which poses a serious economic and health burden on families and societies.1,2 It is characterized by the reduction and deterioration of microarchitecture of bone tissue, leading to reduced bone mass and density and consequently, increased susceptibility to fractures.3,4 There are three types of osteoporosis, namely, postmenopausal osteoporosis (PMO), age-related osteoporosis, and secondary osteoporosis.5 Though the disease is more common in the elderly, women of all ages are more prone to the onset of osteoporosis than men, resulting particularly from their smaller and thinner bones; hence, they lose bone density more rapidly than men after menopause.6,7 It was reported that most women of 45 to 55 years of age suffer from estrogen and progesterone deficiency, which accelerates the unbalance between the rates of bone formation and bone resorption, related to bone loss and reduced bone flexibility.8,9 Hence, PMO is the most familiar type of osteoporosis in clinical practice. The precise mechanism of PMO is not fully understood. Patients still depend on biochemical index, imaging examination and bone density analysis for screening suspicious lesions. Currently, hormone replacement therapy (HRT) is recognized as a typical therapeutic method to promote bone formation, which effectively inhibits fractures in postmenopausal women.10,11 However, it also brings out inevitable risks such as higher incidence of thromboembolic complications,12 breast and gynecological tumors,13,14 mandible osteonecrosis15–17 and hot flushes,18 which significantly restrict clinical applications; thus, employing HRT is a major concern in clinical settings. Most individuals at risk for osteoporosis have not been effectively screened in the early stages. Hence, there is an urgent need for a fast and sensitive screening method to detect early stage lesions and disease development for ameliorating the morbidity rate of female patients with osteoporosis.
Metabolomics provides powerful, complex and robust data sets from metabolites in cells, tissues, organs or biological fluids evaluation, which bring new insights into disease pathophysiology for early diagnosis, biomarkers discovery, and seeking disturbed pathways related with disease or treatment of humans.19,20 Complete process framework of metabolomics processes covers sample collection, pretreatment, compound separation, data analysis and metabolic variation interpretation. Multiple separation and combination methods of metabolomics research have been applied including chromatography, mass spectrometry, magnetic resonance, infrared spectroscopy, Coulomb analysis, UV absorption, fluorescence scattering, radioactivity detection and light scattering. Liquid chromatography-mass spectrometry (LC-MS) as a metabolic technique is widely used analytical method.21 Due to the higher sensitivity, a large number of small molecules can be generally detected.22,23 This study attempts to reveal metabolic variations in ovariectomy-induced osteoporosis in female Wistar rats by LC-MS-based plasma metabolomics, which appraise the value of metabolomics for early detection and prediction of osteoporosis in female Wistar rats and offers a new platform for drug discovery and medical development.
2. Experimental section
2.1 Reagents
HPLC grade acetonitrile and formic acid were purchased from Merck (Darmstadt, Germany). Methanol (HPLC grade) was purchased from Honeywell Company (Morristown, New Jersey, USA). Distilled water was obtained from Watson's Food & Beverage Co., Ltd. (Guangzhou, China). Leucine enkephalin was purchased from Sigma-Aldrich (St. Louis, MO, USA) and pentobarbital sodium was purchased from the Beijing Reagent Company (Beijing, China). Other chemicals, unless otherwise stated, were of analytical grade and obtained from the Shanghai Chemical Agent Company of China Medicine Clique (Shanghai, China). The assay kits for estrogen 2 (E2), and calcitonin (CT) were purchased from the Biosino Bio-technology and Science Inc (Beijing, China).
2.2 Animal model
Six-week-old female Wistar rats weighing 140–160 g were provided by GLP center of Shan Dong University of Chinese Medicine (Shandong, China). A total of 16 rats were raised in polycarbonate cages and acclimatized in temperature-controlled rooms at 21 ± 4 °C with 60 ± 5% humidity. A 12 h light–dark cycle was alternatively set and there was free access to standard research diet and water for an adaptation period of seven days. After adaptation, the rats were divided into two groups in a random way with 8 animals for each group, namely, control group and model group. Female Wistar rats in the model group were anesthetized with 3% isoflurane (1.5 mL/100 g), and then fixed in a supine position. The skin was peeled from the intercostal margin, the midaxillary line and about 1 cm on both sides of the spine. After disinfecting the skin, muscles and peritoneum with 70% alcohol and iodine tincture, the ovaries were first separated and then removed bilaterally after the oviduct was ligated with silk. The experimental scheme was approved by the Animal Care and Use Review Committee of Wujiang Hospital Affiliated to Nantong University. All animal care and experimental procedures were performed in accordance with the Ethical Committee of Wujiang Hospital Affiliated to Nantong University.
2.3 Plasma sample collection and preparation
Blood of all the animals in the control and model groups was collected after a 12 h overnight fast at the 14th day after modeling. Rats were anesthetized by injecting 10% chloral hydrate (5 mL kg−1) into the abdominal cavity. Blood samples (approximately 6 mL) from the ventral vena cava were collected in plasma-separating tubes immediately, followed by natural precipitation for 2 h. After centrifugation at 3500 rpm for 10 min at 4 °C, the plasma layer was stored at 75 °C until detection. Plasma levels of E2 and CT were measured by standard methods as per the respective kits. Then, 40 μL plasma sample was extracted for metabolomics analysis in a 2 mL microcentrifuge tube, to which 280 μL of ice-cold methanol was added at an optimal ratio of 1
:
7. After vortexing for 3 min, the mixture was homogenized by a mixer mill (Retsch GmbH & Co, Haan, Germany) and was maintained at 20 °C for 30 min. Then, the sample was centrifuged at 12
000 rpm for 10 min at 4 °C. The supernatants were filtered using a 0.2 μm filter and finally poured into Eppendorf tubes. The supernatant was dried by nitrogen with a speed vacuum machine (Biotron, Seoul, Korea). Before LC/MS analyses, the dried samples were redissolved in methanol and filtered with a 0.22 μm filter membrane.
2.4 Bone density and biomechanical analysis
After blood collection, all the rats were separated at the fifth lumbar spine and left femur for bone density analysis, and the fourth lumbar spine and right femur vertebrae were used for biomechanical testing. Using Norland-XR36 DEXA, the fifth lumbar spine and the left femoral bone density were detected in the small object scan mode with accuracy of 0.01%, scan speed of 60 mm s−1, resolution of 1.0 mm × 1.0 mm and scan width of 5.0 cm. At the end of the scan, the bone mineral density value of each specimen was analyzed using the instrument auto-selection tool. Biomechanical analysis of rats was performed using the AG-IS biomechanical analysis system. Before the analysis, all attachments of the fourth lumbar spine were removed, such as the transverse processes, articular processes, pedicles and lamina. The vertebral body was polished to make a standard 5 mm segment, which was then fixed on the test platform using glue. The axial compression of the vertebral body was performed at a speed of 5 mm min−1 until it broke. The right femur vertebrae of all the rats were subjected to a three-point bending test until the femur fractured. The maximum compressive load and elastic modulus were recorded and analyzed.
2.5 LC -MS analysis
The LC/MS analyses for the plasma samples were first performed on a Waters ACQUITY UPLC system controlled with Masslynx (V4.1, Waters Corporation, Milford, USA) set with a binary solvent manager and an auto sample manager. Initially, 2 mL plasma sample solution was injected into a ACQUITY™ UPLC BEH C18 column (100 × 2.1 mm, 1.7 μm, Waters), which was regulated at 40 °C at a flow rate of 0.4 mL min−1. Then, the chromatographic operations, i.e., absorbance measurements, were performed on an ACQUITY™ UPLC Tunable UV (TUV) Detector (Waters). The mobile phase consisted of a linear gradient system of A (0.1% formic acid in water) and B (0.1% formic acid in acetonitrile) at a flow rate of 0.4 mL min−1 and injection volume of 5 μL. The solvent gradient condition was set as follows with respect to B: 0–3 min: 1.0–15.0%, 3–4 min: 15.0–25.0%, 4–6 min: 25–50%, 6.0–8.0 min: 50–70%, 8.0–10.0 min: 70–99%, 10.0–11.0 min: 99%, 11.0–12.0 min: 1%.
Mass spectrometry analyses were performed on a Waters Micromass Q-TOF microt Synapt High Definition Mass Spectrometer (Manchester, UK) equipped with electrospray ionization. The full-scan mass spectral range was 50–1000 m/z with a 0.4 s scan time and a 0.1 s interscan delay over a 12 min run time in both positive and negative ion modes. In the positive ionization mode, the capillary voltage was controlled at 4.0 kV, the cone voltage was set at 45 V and the extraction cone voltage was set at 4 V. The cone and desolvation gas flow rates were respectively regulated at 55 L h−1 and 480 L h−1. The temperature of the source was set at 120 °C and the desolvation temperature was set at 400 °C. In the negative ionization mode, the capillary voltage was maintained at 3.5 kV, the sample cone voltage was maintained at 30 V and the extraction cone voltage was set at 3.5 V. The source and desolvation temperatures were 150 °C and 370 °C, respectively. The cone gas flow rate was set at 45 L h−1 and the desolvation gas flow rates was set at 400 L h−1. All the acquired data were digitally stored by the Masslynx TM NT4.1 software (Waters Corp., Milford, MA/USA). A lock-mass of leucine enkephalin at a level of 0.2 ng mL−1 was applied for ensuring accurate mass acquisition using a lock spray interface at flow rate of 100 mL min−1, which was monitored in the positive ion mode ([M + H]+ = 556.2771) and the negative ion mode ([M − H]− = 554.2614). As they contained most of the information of all the plasma samples, the QC samples were used to ensure the stability and consistent performance of the analytical system each time after instrument calibration.
2.6 Data processing and statistical analysis
The latest UPLC/MS technology offers a large amount of signals for unknown compounds due to the high resolution and high sensitivity performance. Chemometrics computer technology was applied to solve this problem in metabolomics analysis. The raw data from UPLC/MS were imported into the Progenesis QI software (Nonlinear Dynamics, 2014, version 1.0) for data preprocessing including noise reduction, normalization and peak picking before the multivariate data analysis. Then, the intensity of each ion was normalized to the total ion count, and a data matrix contained the Rt and m/z values, and the normalized peak areas were produced and exported. The multivariate data matrix was imported into the EZinfo2.0 software (Waters Corporation, Manchester, UK) for analysis such as orthogonal partial least squares discriminant analysis (OPLS-DA) and variable importance projection (VIP) analysis to discern the similarities and differences of metabolomes between the control and model groups. PLS-DA, a model that separates classes of observations of the Y axis (or vertical line) according to their X variables (or horizontal line) by calculating the cumulative goodness of fit (R2) and the cumulative goodness of prediction (Q2), was performed to highlight the discrepancies between the different groups. Metabolites with the furthest distance from the origin ions that had VIP values greater than 1 and a P value less than 0.05 were regarded as potential biomarkers. A comparison of these values between the control and model groups of the disease was conducted. The structural characterization information of the potential ions was performed by MS/MS analysis and MassFragment TM application manager (Waters Corp., Milford, USA). Potential biomarkers were selected and identified by using modern biochemical databases such as HMDB (http://www.hmdb.ca/), KEGG (http://www.genome.jp/kegg/), Chemspider (http://www.chemspider.com), and LIPIDMAPS (http://www.lipidmaps.org/) as well as Scripps Center for Mass spectrometry (http://masspec.scripps.edu/index.php). MetaboAnalyst 4.0 and the Ingenuity pathway analysis (IPA) software were used in an attempt to illuminate the concentration change, interaction, and core pathways of potential biomarkers. All statistical analyses were calculated by the two-tailed, two-sample Student's t-test and expressed as mean ± SD. A P value less than 0.05 suggested that the differences were statistically significant. In addition, P values less than 0.01 indicated extremely significant difference between the control and model groups.
3. Results
3.1 Biochemical index, bone density and biomechanical change in osteoporosis rats
The animal model of PMO was established by ovariectomy in female Wistar rats, which was verified by plasma biochemical indicators, bone density and biomechanical analysis. Compared with the control group, the level of E2 in the plasma of the model group were more significantly decreased (P < 0.01), and the level of CT in the plasma observably decreased (P < 0.05). The bone densities of vertebra and femur were observably decreased (P < 0.05) (Fig. 1). The distinct dynamics variation between the control and the ovariectomy-induced osteoporosis rats shows that biological alteration was present in the model group. The maximum load of vertebrae in osteoporosis female rats were significantly decreased (P < 0.01) compared to those of the control group, and elastic modulus of vertebrae also observably decreased (P < 0.05) in the model group. There was a decrease in the maximum load and elastic modulus in the femur, but this was not statistically significant. Subsequently, the study of potential biomarkers by plasma metabolite analysis was conducted for the mechanism of osteoporosis (Fig. 2).
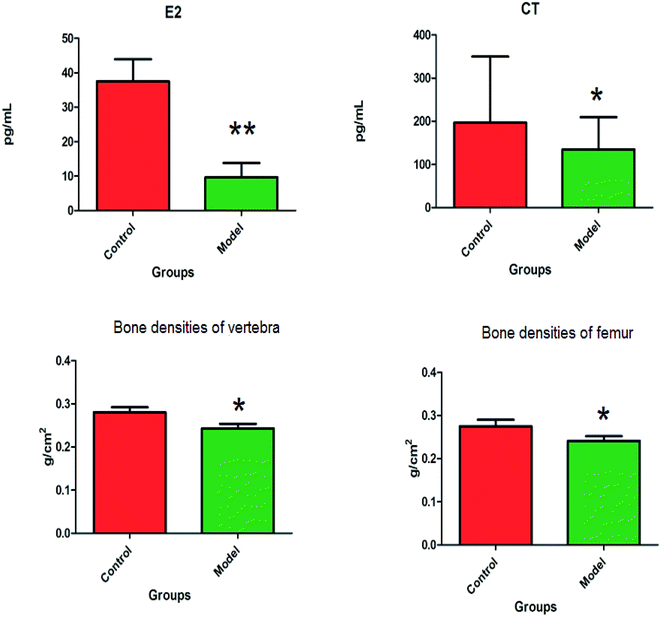 |
| Fig. 1 The biochemical index and bone density changes of female Wistar rats in the control group and the ovariectomy-induced osteoporosis group.*P < 0.05; **P < 0.01. | |
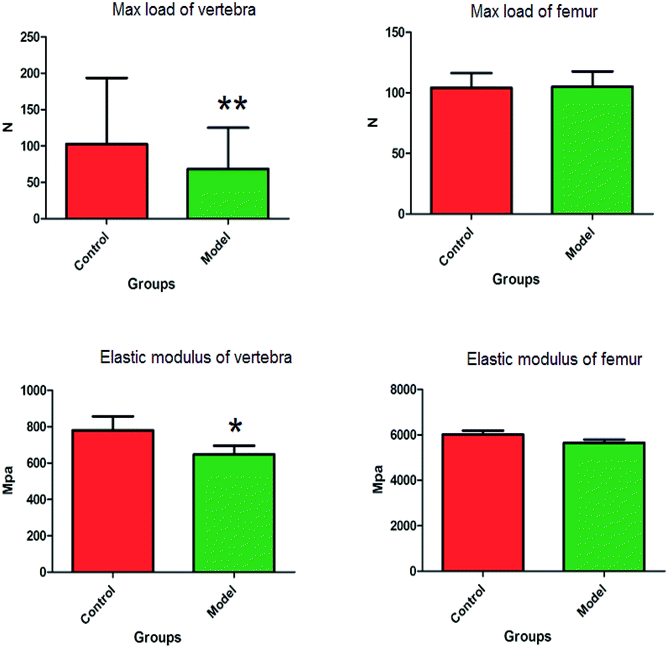 |
| Fig. 2 The biomechanical change of female Wistar rats vertebrae and femur in the control group and the ovariectomy-induced osteoporosis group.*P < 0.05; **P < 0.01. | |
3.2 Metabolic variations of ovariectomy-induced osteoporosis
A comprehensive multidimensional matrix containing RT, m/z, and peak height intensity was obtained by Progenesis QI pretreatment, which was applied for further detection and more sophisticated analysis in EZinfo plugins. Combined with the t-test, the OPLS-DA score plots discovered a series of interesting ions by accurate calculation of discrepancy between the scores of control and model rats. In the positive ion mode (Fig. 3A and B) and negative ion mode (Fig. 4A and B) of the 2D and 3D score plots, the control and model groups were clearly well separated, which indicated that significant metabolic disturbance in plasma had already occurred in the female Wistar rats after the ovariectomy operation. The VIP score obtained from the OPLS/DA data present the contribution of the variables to the cluster and optimization of discrimination against potential markers (Fig. 3C and 4C), in which the ions furthest from the origin were considered as potential biomarkers through the differences between the osteoporosis and healthy rats in different groups. The differentially expressed metabolite ions were selected according to the following threshold: the VIP value is more than 1 and the P value is less than 0.05 by Student's t-test analysis. After MS and MS/MS signals data matching, 18 novel biomarkers were finally tentatively identified with the help of network databases such as Mass-Bank, ChemSpider, KEGG, LIPIDMAPS and HMDB. The identified biomarkers were taurocholic acid, glycocholic acid, docosahexaenoic acid, LysoPC(15
:
0), LysoPC(20
:
0), leucinic acid, palmitic acid, L-lysine, arachidonic acid, corticosterone, LysoPE(18
:
0/0
:
0), LysoPE(20
:
0/0
:
0), PS(15
:
0/24
:
0), L-isoleucine, palmitoleic acid, 4-oxoretinol, glyceraldehyde, and ursocholic acid (ESI Table 1†). From the hierarchical clustering heat map, 9 of the 18 differential biomarkers were up-regulated, namely, taurocholic acid, glycocholic acid, LysoPC(15
:
0), LysoPC(20
:
0), arachidonic acid, corticosterone, L-isoleucine, palmitoleic acid and ursocholic acid, while the others were down-regulated in model group by Pearson's linear correlation analysis (Fig. 5A). As shown in Fig. 5B and C, the PCA score plot presented clear separation of the two groups and the cluster diagram revealed that the two groups could be separated completely by 7 metabolites, including glyceraldehyde, 4-oxoretinol and L-lysine with VIP score > 1.0. In total, 13 pathways involved in metabolic alteration in ovariectomy-induced osteoporosis in female Wistar rats were identified by biomarkers imported into the MetaboAnalyst 4.0 (Fig. 6). Among them, valine, leucine and isoleucine biosynthesis, arachidonic acid metabolism and primary bile acid biosynthesis with impact indices of 0.33, 0.32 and 0.06 closely stimulate metabolic alteration. Then, IPA analysis for further interpretation of detailed functional changes in ovariectomy-induced osteoporosis of rats showed that many types of endogenous substances such as, cytokines, enzymes, G-protein coupled receptors, transporters, and kinases are mainly related to the metabolism of cellular compromise, cell signaling, inflammation, molecular transport and lipid metabolism, and have potential to become predictive targets of PMO (Fig. 7).
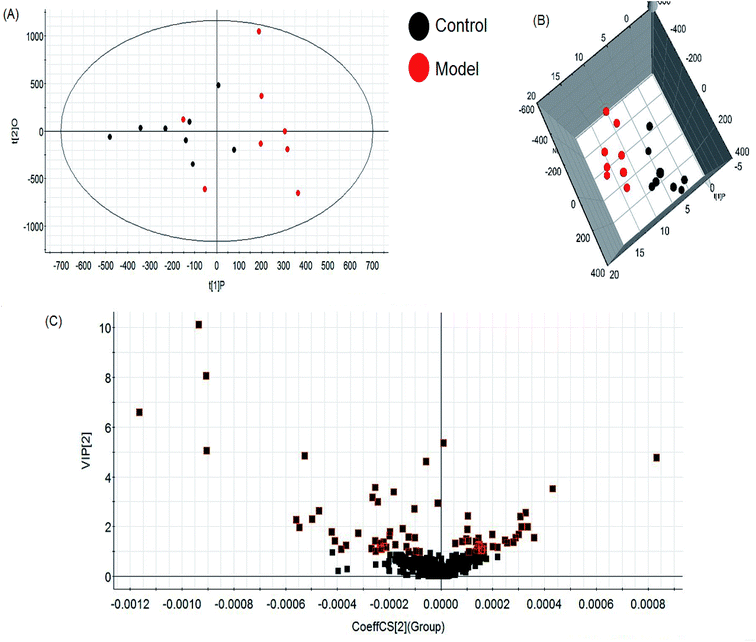 |
| Fig. 3 Multivariate statistical analysis of LC/MS data in positive ion mode. OPLS-DA score plot (A) and 3D PLS-DA (B) of the plasma metabolic profile between the control and ovariectomy-induced osteoporosis in female Wistar rats. VIP-plot (C) of OPLS-DA model of LC-MS spectra data in positive ion mode. | |
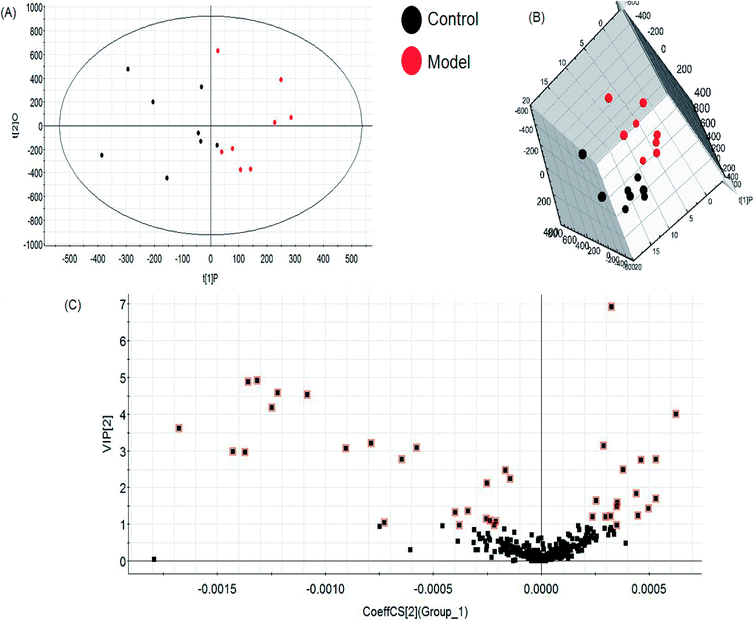 |
| Fig. 4 Multivariate statistical analysis of LC/MS data in negative ion mode. OPLS-DA score plot (A) and 3D PLS-DA (B) of the plasma metabolic profile between the control and ovariectomy-induced osteoporosis in female Wistar rats. VIP-plot (C) of OPLS-DA model of LC-MS spectra data in negative ion mode. | |
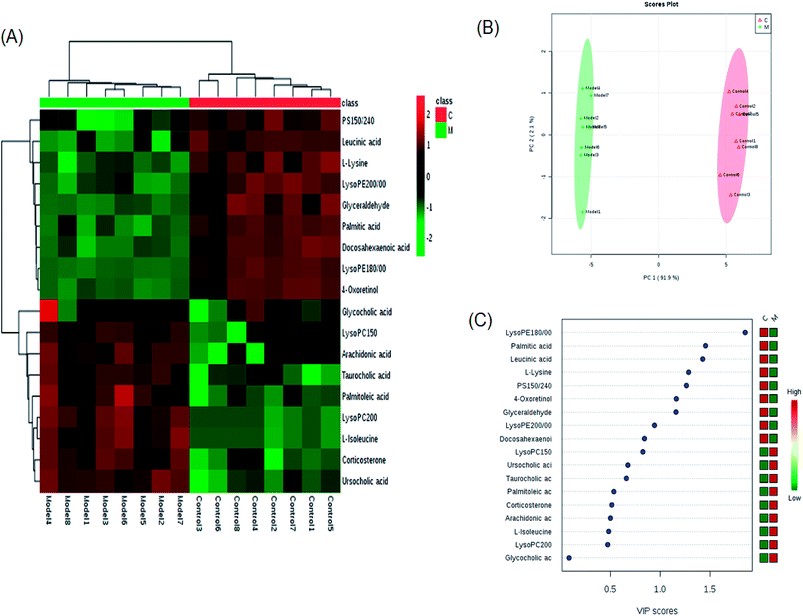 |
| Fig. 5 Significant changes in metabolite biomarker candidates in control and model groups. (A) Heatmap visualization for plasma samples from the control and model groups. (B) Unsupervised PCA analysis of different groups. (C) VIP scores of the metabolite marker candidates. | |
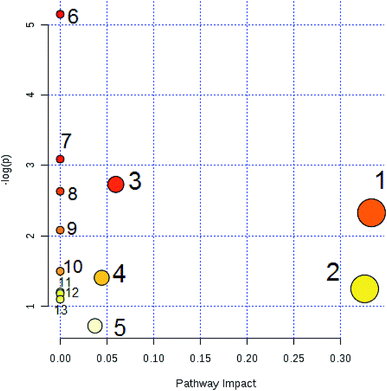 |
| Fig. 6 Pathway analysis of metabolic variations in ovariectomy-induced osteoporosis in female Wistar rats. Note: (1) valine, leucine and isoleucine biosynthesis; (2) arachidonic acid metabolism; (3) primary bile acid biosynthesis; (4) glycerophospholipid metabolism; (5) steroid hormone biosynthesis; (6) biosynthesis of unsaturated fatty acids; (7) biotin metabolism; (8) taurine and hypotaurine metabolism; (9) aminoacyl-tRNA biosynthesis; (10) fatty acid elongation in mitochondria; (11) valine, leucine and isoleucine degradation; (12) fatty acid metabolism; (13) fatty acid biosynthesis. | |
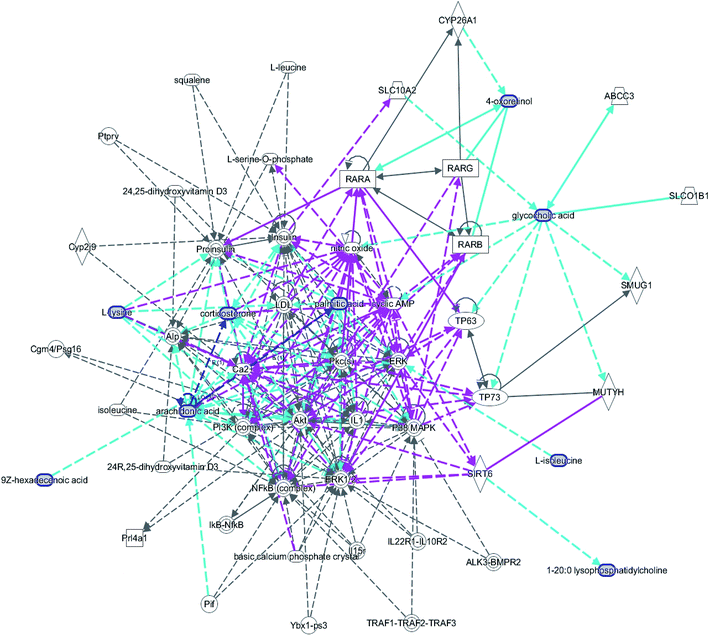 |
| Fig. 7 IPA prediction networks associated with ovariectomy-induced osteoporosis rats in this study. | |
4. Discussion
The choice of animal model is crucial for this experimental study. Excision of bilateral ovaries in mice can mimic osteoporosis caused by decreased estrogen after menopause. Currently, this method is widely used in most experimental studies; hence, it was chosen to prepare a rat model of osteoporosis in our experiment. However, it still has some considerable limitations:24,25 the ovary endocrine function of ovariectomized rats completely disappears, but some cells in the ovary still possess endocrine function in case of human beings after the menopause; surgical operation lead to body stress response that bring deviations to laboratory measurements; model establishment studies cannot meet the requirements for repeated sampling and long-term determination of continuous samples when the lifespan of the rats and the amount of blood in their tissues are not adequate; low-calcium food is commonly applied to promote rapid establishment of the model. In addition, by inhibiting endocrine drugs in order to reduce estrogen production or release in the model, the following inhibitors are mainly used for modeling: casodex, a non-steroidal androgen antagonist that can deplete estradiol in vivo; buserelin, a progesterone-secreting hormone analogue that acts to lower serum estradiol levels;26 and gonadotropin-releasing hormone, which can cause bone loss in animals after use. Glucocorticoids such as prednisone, prednisolone or dexamethasone induced osteoporosis is different from primary osteoporosis in the pathogenesis and disease course, which is not suitable for drugs evaluation of the therapeutic effect on osteoporosis.27,28 With the rapid development of genetic engineering, the emergence of transgenic animals has provided possible pathways and ideal models for various experiments. The osteoporosis transgenic model is mainly produced by knocking out the alpha, beta-estrogen receptor or aromatase genes to prepare homozygotes.
CT can inhibit the activity of osteoclasts and enhance the activity of osteoblasts for reducing bone loss, thus promoting bone regeneration and increasing the deposition of calcium, phosphorus as well as other substances in the bone tissue. In addition, it is also characterized by having strong analgesic activity on waist pain in senile osteoporosis, thoracolumbar osteoporotic fractures and bone pain caused by bone disease. E2 is an important hormone marker for evaluating ovarian function, which lowers blood cholesterol and increases calcium deposition in the bone. In this study, the level of CT, E2 and bone mineral density in the model group decreased compared with those of the control group, indicating that the rats lacked estrogen secretion after ovariectomy, leading to bone loss. The biomechanical analysis of the maximum load and elastic modulus in the model group showed significant degeneration, which suggests that the model was successfully established. Compared with the femur, the extent of vertebral damage was more significant. Protein is an important raw material that constitutes a bone matrix whose deficiency leads to insufficient synthesis of bone matrix and inhibition of new bone formation in the long-term. At the same time, excessive intake of some amino acids can accelerate the loss of calcium in the bones, causing negative calcium balance and osteoporosis. In this study, it was found that leucine and proline metabolism, which promote calcium absorption, were abnormal in the model rats, resulting in decreased calcium absorption. Arachidonic acid is one of the essential free fatty acids in the body that play an important role in regulating the body's inflammatory response, blood vessel elasticity, leukocyte function, bone remodeling and platelet activation. Recent studies have found that changes in the level of arachidonic acid in the blood of postmenopausal women were associated with postmenopausal hypertension, obesity, and osteoporosis.29 This study found that compared with the blank group, the arachidonic acid content in the plasma of the model group of rats increased, indicating that it is related to the occurrence of osteoporosis after ovariectomy.
Metabolomics faces many challenges such as the identification of unknown metabolites, developing a standard data set for inquiries and the integration of metabolomics and other system data.30–35 Mass spectrometry is so sensitive that it yields highly complex data, with numerous unknown metabolites.36,37 The similarity of these molecules is a key factor in identifying these metabolites. The development and optimization of high-quality databases allows us to predict similar or modified variants of spectra from known spectral data.38,39 In order to face the challenges brought about by data integration and search ability, the metabolome community has taken some initiatives, such as the development of data standards for metabolomics and the establishment of data storage libraries.40–43 Even after having a database of stored data in the metabolomics community, the primary challenge for systems biology is still biological interference, which needs to be integrated with other system data in order to help metabolomics continue to evolve.
5. Conclusion
Osteoporosis is a severe clinical bone disease that is hard to treat and remains incurable; thus, early detection and prevention are critically important. An untargeted plasma metabolomics strategy has been attempted to discover differentially expressed metabolites and interpret metabolic alteration in ovariectomy-induced osteoporosis in female Wistar rats by a high throughput technology platform. There is major discrepancy in the plasma standards of many metabolites between control and model groups. In total, 18 potential biomarkers and 13 metabolic pathways were identified, including valine, leucine and isoleucine biosynthesis, arachidonic acid metabolism and primary bile acid biosynthesis, which were closely related to ovariectomy-induced osteoporosis development and were mainly involved in some functional pathways such as cellular compromise, cell signaling, inflammation, molecular transport and lipid metabolism. This experiment is in the primary research stage of metabolic biomarkers of menopausal osteoporosis, and provides a reference for subsequent research endeavors that aim at finding and validating suitable biomarkers for clinical applications. It was shown that metabolites of osteoporosis may be used as markers, and relevant metabolic pathways revealed marked metabolic variations, which are able to give insight into molecular mechanisms and offer novel ideas for the prevention, prediction, and therapy of PMO. Metabolomics have become a promising method that can be applied as a medical tool for much more precise predictive and prognostic assessments.
Conflicts of interest
There are no conflicts to declare.
Acknowledgements
This study was supported by grants from the Project of the First Peoples' Hospital of Wujiang (201802).
References
- B. Ma, Q. Zhang, D. Wu, Y. L. Wang and Y. Y. Hu, et al., Strontium fructose 1,6-diphosphate prevents bone loss in a rat model of postmenopausal osteoporosis via the OPG/RANKL/RANK pathway, Acta Pharmacol. Sin., 2012, 33, 479–489 CrossRef PubMed.
- C. Cooper, G. Campion and L. J. Melton, Hip fractures in the elderly: a world-wide projection, Osteoporosis Int., 1992, 2, 423–424 CrossRef.
- H. K. Genant, P. D. Delmas and P. Chen, et al., Severity of vertebral fracture reflects deterioration of bone microarchitecture, Osteoporosis Int., 2007, 18, 69–76 CrossRef PubMed.
- T. D. Galsworthy, Osteoporosis: statistics, intervention, and prevention, Ann. N. Y. Acad. Sci., 1994, 736, 158–164 CrossRef PubMed.
- S. Y. Choi, D. Park and G. Yang, et al., Effects of Sigma Anti-bonding Molecule Calcium Carbonate on bone turnover and calcium balance in ovariectomized rats, Laboratory Animal Research, 2011, 301–307 CrossRef PubMed.
- R. A. Adler, Osteoporosis in men: a review, Bone Res., 2014, 2, 14001 CrossRef PubMed.
- G. I. Meniru and B. R. Hecht, Prevention of Infertility and Complications in Women, New York, NY, USA, 2003 Search PubMed.
- P. A. Balch, Prescription for Nutritional Healing, Penguin, New York, NY, USA, 2006 Search PubMed.
- X. Li, M. S. Ominsky and K. S. Warmington, et al., Sclerostin antibody treatment increases bone formation, bone mass, and bone strength in a rat model of postmenopausal osteoporosis, J. Bone Miner. Res., 2009, 24, 578–588 CrossRef PubMed.
- J. A. Cauley, D. G. Seeley and K. Ensrud, et al., Estrogen replacement therapy and fractures in older women. Study of Osteoporotic Fractures Research Group, Ann. Intern. Med., 1995, 122, 9–16 CrossRef PubMed.
- M. B. Cetinkaya, A. Kökçü and F. F. Yanik, et al., Comparison of the effects of transdermal estrogen, oral estrogen, and oral estrogen–progestogen therapy on bone mineral density in postmenopausal women, J. Bone Miner. Metab., 2002, 20, 44–48 CrossRef PubMed.
- D. B. Petitti, Hormone replacement therapy for prevention: more evidence, more pessimism, JAMA, 2002, 288, 99–101 CrossRef PubMed.
- D. Z. Tang, W. Hou and Q. Zhou, et al., Osthole stimulates osteoblast differentiation and bone formation by activation of beta-catenin-BMP signaling, J. Bone Miner. Res., 2010, 25, 1234–1245 CrossRef PubMed.
- G. A. Colditz, Hormone replacement therapy increases the risk of breast cancer, Ann. N. Y. Acad. Sci., 1997, 833, 129–136 CrossRef PubMed.
- Z. H. Baqain, F. A. Sawair and Z. Tamimi, et al., Osteonecrosis of jaws related to intravenous bisphosphonates: the experience of a Jordanian teaching hospital, Ann. R. Coll. Surg. Engl., 2010, 92, 489–494 CrossRef PubMed.
- S. Khosla, D. Burr and J. Cauley, et al., Bisphosphonate associated osteonecrosis of the jaw: report of a task force of the American Society for Bone and Mineral Research, J. Bone Miner. Res., 2007, 22, 1479–1491 CrossRef PubMed.
- M. Muratore, E. Quarta and A. Grimaldi, et al., Clinical utility of clodronate in the prevention and management of osteoporosis in patients intolerant of oral bisphosphonates, Drug Des., Dev. Ther., 2011, 5, 445–454 CrossRef PubMed.
- P. Hadji, The evolution of selective estrogen receptor modulators in osteoporosis therapy, Climacteric, 2012, 15, 513–523 CrossRef PubMed.
- A. Zhang, H. Sun and G. Yan, et al., Mass spectrometry-based metabolomics: applications to biomarker and metabolic pathway research, Biomed. Chromatogr., 2016, 30(1), 7–12 CrossRef PubMed.
- H. Chu, A. Zhang and Y. Han, et al., Metabolomics approach to explore the effects of Kai-Xin-San on Alzheimer's disease using UPLC/ESI-Q-TOF mass spectrometry, Journal of Chromatography B, 2016, 1015, 50–61 CrossRef PubMed.
- A. Zhang, H. Fang and Y. Wang, et al., Discovery and verification of the potential targets from bioactive molecules by network pharmacology-based target prediction combined with high-throughput metabolomics, RSC Adv., 2017, 7, 51069–51078 RSC.
- A. Zhang, Q. Liu and H. Zhao, et al., Phenotypic characterization of nanshi oral liquid alters metabolic signatures during disease prevention, Sci. Rep., 2016, 6, 19333 CrossRef PubMed.
- A. H. Zhang, H. Sun and S. Qiu, et al., NMR-based metabolomics coupled with pattern recognition methods in biomarker discovery and disease diagnosis, Magn. Reson. Chem., 2013, 51(9), 549–556 CrossRef PubMed.
- X. Zhang, X. Xu and X. Liu, et al., Bis enoxacin blocks alveolar bone resorption in rats with ovariectomy induced osteoporosis, Mol. Med. Rep., 2018, 17(2), 3232–3238 Search PubMed.
- S. H. Song, D. Wang and Y. Y. Mo, et al., Antiosteoporotic effects of naringenin on ovariectomy-induced osteoporosis in rat, Yaoxue Xuebao, 2015, 50(2), 154–161 Search PubMed.
- A. Goulding and E. Gold, Buserelin-mediated osteoporosis: effects of restoring estrogen on bone resorption and whole body calcium content in the rat, Calcif. Tissue Int., 1990, 46(1), 14–19 CrossRef PubMed.
- H. Matsuno, Assessment of Distal Radius Bone Mineral Density in Osteoporosis Patients Receiving Denosumab, Including Those with Rheumatoid Arthritis and Those Receiving Oral Glucocorticoids, Drugs R&D, 2016, 16(4), 347–353 CrossRef PubMed.
- S. Abell, H. Zeimer and A. Chong, et al., Prevalence of osteoporosis and the use of bone protective therapies in dermatology clinic patients on long-term glucocorticoids, Australas. J. Dermatol., 2015, 56(2), 147–148 CrossRef PubMed.
- B. Ma, Q. Zhang and G. J. Wang, et al., GC -TOF/MS -based metabolomic profiling of estrogen deficiency induced obesity in ovariectomized rats, Acta Pharmacol. Sin., 2011, 32(2), 270–278 CrossRef PubMed.
- A. Zhang, H. Sun and H. Xu, et al., Cell metabolomics, OMICS: J. Integr. Biol., 2013, 17(10), 495–501 CrossRef PubMed.
- Q. Liang, H. Liu, H. Xing and Y. Jiang, et al., UPLC-QTOF/MS based metabolomics reveals metabolic alterations associated with severe sepsis, RSC Adv., 2016, 6, 43293–43298 RSC.
- Q. Liang, H. Liu and T. Zhang, et al., High-throughput metabolic profiling for discovering metabolic biomarkers of sepsis-induced acute lung injury, RSC Adv., 2016, 6, 11008–11013 RSC.
- Q. Liang, H. Liu and T. Zhang, et al., Potential urine biomarkers from a high throughput metabolomics study of severe sepsis in a large Asian cohort, RSC Adv., 2015, 5, 102204–102209 RSC.
- Q. Liang, H. Liu and L. Xie, et al., High-throughput metabolomics enables biomarker discovery in prostate cancer, RSC Adv., 2017, 7, 2587–2593 RSC.
- Q. Liang, H. Liu and T. Zhang, et al., Discovery of serum metabolites for diagnosis of mild cognitive impairment to Alzheimer's disease progression using an optimized metabolomics method, RSC Adv., 2016, 6, 3586–3591 RSC.
- Q. Liang, T. Zhang and Y. Jiang, et al., Metabolomics-Based Screening of Salivary Biomarkers for Early Diagnosis of Alzheimer's Disease, RSC Adv., 2015, 5, 96074–96079 RSC.
- Q. Liang, H. Liu and X. Li, et al., High-throughput metabolomics analysis discovers salivary biomarkers for predicting mild cognitive impairment and Alzheimer's disease, RSC Adv., 2016, 6, 75499–75504 RSC.
- Q. Liang, H. Liu and H. Xing, et al., Urinary UPLC-MS metabolomics dissecting the underlying mechanisms of huaxian capsule protects against sepsis, RSC Adv., 2016, 6, 40436–40441 RSC.
- A. Zhang, H. Sun and X. Wang, Mass spectrometry-driven drug discovery for development of herbal medicine, Mass Spectrom. Rev., 2018, 37(3), 307–320 CrossRef PubMed.
- K. E. V. Burgess, Y. Borutzki and N. Rankin, et al., MetaNetter 2: A Cytoscape plugin for ab initio network analysis and metabolite feature classification, J. Chromatogr. B: Anal. Technol. Biomed. Life Sci., 2017, 1071, 68–74 CrossRef PubMed.
- A. H. Zhang, J. B. Yu and H. Sun, et al., Identifying quality-markers from Shengmai San protects against transgenic mouse model of Alzheimer's disease using chinmedomics approach, Phytomedicine, 2018, 45, 84–92 CrossRef PubMed.
- H. Sun, A. Zhang and L. Yang, et al., High-throughput chinmedomics strategy for discovering the quality-markers and potential targets for Yinchenhao decoction, Phytomedicine, 2018 DOI:10.1016/j.phymed.2018.04.015.
- X. Wang, A. Zhang and X. Zhou, et al. An integrated chinmedomics strategy for discovery of effective constituents from traditional herbal medicine, Sci. Rep., 2016, 6, 18997 CrossRef PubMed.
Footnote |
† Electronic supplementary information (ESI) available. See DOI: 10.1039/c8ra03629b |
|
This journal is © The Royal Society of Chemistry 2018 |
Click here to see how this site uses Cookies. View our privacy policy here.