DOI:
10.1039/C8RA08590K
(Paper)
RSC Adv., 2018,
8, 41150-41162
Performance, process kinetics and functional microbial community of biocatalyzed electrolysis-assisted anaerobic baffled reactor treating carbohydrate-containing wastewater
Received
17th October 2018
, Accepted 21st November 2018
First published on 10th December 2018
Abstract
In this study, an anaerobic baffled reactor (ABR) coupled with a microbial electrolysis cell (MEC) was set up to treat carbohydrate-containing wastewater at 55 ± 1 °C. The MEC was employed to accelerate the degradation of volatile fatty acids (VFAs). The removal of chemical oxygen demand (COD) and production of methane and the corresponding kinetics were determined for different organic load rates (OLRs). The highest COD removal rate was 95.8% at an OLR of 7.0 kg COD m−3 d−1, but it declined to 90.4% when the OLR was 19.4 kg COD m−3 d−1 and finally stabilized at 65.3% when the OLR was increased to 34.3 kg COD m−3 d−1. The volumetric production of methane was 1.5 L (L−1 d−1) when the OLR was 7.0 kg COD m−3 d−1 and increased to 4.1 L (L−1 d−1) at an OLR of 34.3 kg COD m−3 d−1, when the methane yield stabilized at 0.20–0.25 L g−1 CODremoved. The kinetics and predictions according to the Stover–Kincannon and Van der Meer–Heertjes models closely agreed with the experimental data for the removal of COD and volumetric production of methane, respectively. An analysis of the microbial community suggested that hydrolytic bacteria, syntrophic fatty acid-oxidizing bacteria (SFOB), exoelectrogens and hydrogenotrophic methanogens achieved a significant synergistic effect and enhanced the degradation of VFAs, which made the thermophilic anaerobic system stable and efficient at high OLRs.
1. Introduction
The accumulation of VFAs is a key issue that affects the stability of an anaerobic system when used to treat high-concentration organic wastewater.1 Specifically, the accumulation of acids is more likely to occur in a thermophilic system because hydrolysis is more rapid.2,3 In the past, researchers have tried to enhance the capacity and stability of anaerobic digestion (AD) by regulating the microbial community and engineering.4 As for regulating the microbial community, bioaugmentation technology has been widely employed to increase the abundance of functional microbes. For instance, in order to alleviate the accumulation of VFAs that results from an imbalance in metabolic rates between hydrolytic bacteria, acidogens and methanogens, SFOB and acid-resistant methanogens were acclimated to improve the degradation of VFAs.5 Nevertheless, the basic biological characteristics (i.e., long generations, slow metabolic rate and narrow niche) of methanogens are difficult to change via acclimation.6 Besides, how to ensure the sustainability and enrichment of target microorganisms in an AD system is a major challenge for conventional bioaugmentation. A microbial electrochemical system could provide a new way to solve these problems.7 In a typical microbial electrolysis cell (MEC), exoelectrogens transfer electrons to the anode by oxidizing organic matter, and then the electrons pass through the circuit to the cathode to produce H2. MECs have also been employed in AD systems. Whereas previous studies of AD-MECs mainly focused on improvements in the production of hydrogen,8 the microbial community in the cathode for the generation of methane,9 the removal efficiency for specific pollutants,10 and the electron transfer mechanism of the exoelectrogens,11 little work has been done to investigate the synergistic degradation of VFAs by functional bacteria. However, acetic acid is a favorable substrate for exoelectrogens.12 It is a fact that exoelectrogens efficiently enhance the degradation of acetic acid and relieve suppression by acetic acid for the decomposition of propionic and butyric acid by SFOB, which provides the theoretical possibility of the rapid dissociation of VFAs. Furthermore, early studies declared that exoelectrogens have many advantages over methanogens, such as longer generations, a faster metabolic rate and a wider niche.13 Hence, it would be a novel idea to intensify the degradation of VFAs via an AD system coupled with MECs (AD-MECs). This not only overcomes the shortcomings of methanogens but also ensures the robustness of target microorganisms in the system by providing continuous electrical stimulation. Although a single-phase reactor such as an up-flow anaerobic sludge bed (UASB) has been adapted to perform an AD-MEC process by the insertion of electrodes, the different niches of acidogenic bacteria, methanogens and exoelectrogens make it difficult to optimize the different microbes in an integral reactor and further limit its efficiency.14 It is feasible to set up a coupled AD-MEC process by connecting a continuous stirred-tank reactor (CSTR) with an MEC in series.15 Nevertheless, the construction of the MEC reactor will increase the cost. Owing to its baffled structure, an ABR is an ideal reactor for achieving the phase separation and distribution of dominant microorganisms in different regions.16 It provides a structural support for better coupling with an MEC in an integral reactor. Therefore, an ABR was used to construct an ABR-MECs system.
On the other hand, although AD technology is widely applied, its optimum operation is seldom achieved because of the empiricism that is prevalent in the design and daily operation of an anaerobic system. With the increasing demand for efficient operation and model-based design, kinetic modeling of the AD process has received extensive attention and has been addressed via substrate utilization models, microbial growth models and product formation models.17,18 The determination of the conversion of methane is the most common on-line measurement and is easily performed as it is directly proportional to the degradation of the substrate.19 In many studies, the production of methane and removal of COD were used to estimate model parameters.20 Many types of mathematical models have been developed. Among these, the anaerobic digestion model 1 (ADM1) is the most advanced owing to its precise predictability and strong generalizability.21 The ADM1 model reflects the major processes in the conversion of complex organic substrates into methane.22 However, the model requires a large number of constants and coefficients, which should be calibrated according to the characteristics of the substrate. Such calibration requires the use of special assays and computing skills, which is difficult for scientists and engineers in engineering applications.23 Therefore, simplified models that consist of only a few variables have been widely studied. The first-order rate equation, Stover–Kincannon model, and Van der Meer–Heertjes model have been used to satisfactorily predict the production of methane and removal of the substrate in an AD process.24,25
In this study, an ABR-MECs system was set up to treat synthetic carbohydrate-containing wastewater at 55 ± 1 °C. The efficiencies of the removal of COD and production of methane at different OLRs were analyzed qualitatively and quantitatively. The first purpose was to reveal the intrinsic relationship between the microbial composition and degradation of VFAs so as to establish a theoretical foundation for improving the stability of an anaerobic system when used to treat an easily acidified substrate. The second aim was to optimize the operation of the system and improve the efficiency of regulation via the establishment of dynamic models.
2. Materials and methods
2.1. Characteristics of the substrate and inoculum
The fresh substrate was prepared in the laboratory, and its parameters are listed in Table 1. Glucose was used as the carbon source as it is easily acidified at high OLRs. NH4Cl and KH2PO4 were provided as sources of nitrogen and phosphorus, respectively. In addition, NaHCO3 was added to regulate the pH. A solution of elements and minerals with a concentration of 1.5 mL L−1 was also added to provide nutrients. It was prepared by dissolving 50 mg L−1 H3BO3, 30 mg L−1 CuCl2, 50 mg L−1 MnSO4, 50 mg L−1 (NH4)6MoO24·4H2O, 50 mg L−1 AlCl3, 50 mg L−1 CoCl2·6H2O and 50 mg L−1 NiCl2 in distilled water.26 A thermophilic inoculum was obtained from a stable thermophilic digester for the treatment of carbohydrates. The substrate in the stable thermophilic digester was synthetic wastewater prepared using glucose. Its seed sludge was collected from the secondary settling tank at Tongan sewage plant in Xiamen, China. The contents of suspended solids (SS) and volatile suspended solids (VSS) of the inoculum were 31.2 g L−1 and 15.3 g L−1, respectively. With respect to the microbial community in the inoculum, Firmicutes were the most abundant bacteria and accounted for about 44.2% of the bacterial community, whereas Methanosaeta, Methanobacterium and Methanosarcina constituted the most significant archaeal components with contents of about 33.6%, 25.8% and 21.2%, respectively.
Table 1 Operational and process performance of the ABR-MECs system in each stagea
Parameter |
Units |
Phase I |
Phase II |
Phase III |
Phase IV |
Stage 1 |
Stage 2 |
Stage 3 |
Stage 4 |
Stage 5 |
Stage 6 |
Stage 7 |
Stage 8 |
Stage 9 |
Stage 10 |
Stage 11 |
Stage 12 |
All values obtained by calculation are based on the average measured results. ‘T’ denotes ‘total’. ‘Red.’ denotes ‘reduction’. |
Temperature |
°C |
35 |
35 |
35 |
35 |
35 |
35 |
35 |
35 |
35 |
35 |
35 |
35 |
HRT |
Hours |
24 |
21 |
18 |
48 |
24 |
21 |
48 |
24 |
21 |
21 |
21 |
21 |
Influent Tb-COD |
g L−1 |
6 |
6 |
6 |
14 |
14 |
14 |
17 |
17 |
17 |
20 |
25 |
30 |
NH4+–N |
mg L−1 |
300 |
300 |
300 |
700 |
700 |
700 |
850 |
850 |
850 |
1000 |
1250 |
1500 |
PO43– |
mg L−1 |
20 |
20 |
20 |
47 |
47 |
47 |
57 |
57 |
57 |
67 |
83 |
100 |
Influent ALK |
g L−1 |
1.8 |
1.8 |
1.8 |
4.2 |
4.2 |
4.2 |
5.1 |
5.1 |
5.1 |
6.0 |
7.5 |
9.0 |
OLR |
kg m−3 d−1 |
6.0 |
6.9 |
8.0 |
7.0 |
14.0 |
16.0 |
8.5 |
17.0 |
19.4 |
22.9 |
28.6 |
34.3 |
Effluent pH |
|
7.55 ± 0.02 |
7.42 ± 0.02 |
7.52 ± 0.02 |
7.61 ± 0.04 |
7.58 ± 0.03 |
7.56 ± 0.03 |
7.61 ± 0.02 |
7.63 ± 0.02 |
7.62 ± 0.03 |
7.34 ± 0.08 |
7.15 ± 0.1 |
6.85 ± 0.1 |
T-COD Red.c |
% |
95.4 |
94.6 |
80.5 |
95.8 |
95.5 |
94.7 |
92.4 |
91.6 |
90.4 |
84.9 |
77.2 |
65.3 |
Effluent ALK |
g L−1 |
3.03 ± 0.11 |
3.00 ± 0.13 |
3.01 ± 0.13 |
3.03 ± 0.10 |
3.12 ± 0.15 |
3.04 ± 0.15 |
3.66 ± 0.10 |
3.54 ± 0.15 |
3.64 ± 0.15 |
3.98 ± 0.25 |
3.86 ± 0.23 |
4.05 ± 0.24 |
ORP |
mV |
−284 ± 15 |
−305 ± 14 |
−310 ± 9 |
−325 ± 5 |
−325 ± 5 |
−325 ± 5 |
−325 ± 5 |
−325 ± 5 |
−325 ± 5 |
−307 ± 6 |
−302 ± 8 |
−298 ± 10 |
CH4 composition |
% |
81.3 ± 0.2 |
82.4 ± 0.2 |
67.6 ± 2.4 |
82.6 ± 0.3 |
81.5 ± 0.4 |
81.3 ± 0.2 |
81.4 ± 0.2 |
83.9 ± 0.5 |
85.3 ± 0.3 |
82.4 ± 0.2 |
83.1 ± 0.2 |
80.8 ± 0.2 |
T-CH4 production rate |
L (L−1 d−1) |
1.2 ± 0.1 |
1.4 ± 0.1 |
0.9 ± 0.1 |
1.5 ± 0.1 |
2.6 ± 0.1 |
2.9 ± 0.1 |
1.8 ± 0.1 |
3.1 ± 0.2 |
3.2 ± 0.2 |
3.4 ± 0.2 |
3.8 ± 0.2 |
4.1 ± 0.2 |
Predicted COD |
g L−1 |
Se = Si − 107.527Si/(104.344 + Si/HRT), R2 = 0.979 |
Predicted CH4 |
mL d−1 |
Vm = 0.4680525(Si − Se)/HRT + 0.27811, R2 = 0.968 |
2.2. Experimental setup and operation
The ABR was made of plexiglass and had a working volume of 2.55 L. It was divided into five equal compartments by vertical baffles (Fig. 1). Each compartment was divided into lower corner and upper corner regions by baffles at 45°. Electrodes were fixed in the up-flow regions of the three central compartments to construct the ABR-MECs system and were connected to an external power source (0.9 V). The anode film was made of carbon cloth (WOS1009, CeTech, Taiwan) with an area of 54.00 cm2, whereas the cathode film was made of stainless-steel mesh catalyzed by Ni nanoparticles, according to our previous studies.26,27 The system consisted of gas measuring units (LML-1, Changchun Automobile Filter, China), a temperature control device, inlets and outlets. A water seal was used to ensure the airtightness of the reactor and to collect the biogas (Fig. 1). There was an inverted measuring cylinder in the water seal bottle. When the volume of biogas reached the limit of the measuring range of the cylinder, it overflowed and was discharged into the gas-measuring units. The measurement accuracy of the gas-measuring units could be improved by the centralized release of biogas. The temperature of the substrate tank was controlled by a water jacket connected to a cooler (4 °C), whereas the ABR was equipped with a water jacket that connected a heater (55 °C) with submerged thermometers. Feeding was carried out with peristaltic pumps (BT100-2i, Longer Pump, China). The electrodes were introduced into the ABR at the beginning of the start-up process. After a successful start-up process, the operation of the system lasted for a further 120 days, and the average values of the parameters in each stage are listed in Table 1. The process was divided into 4 phases based on different OLRs, and stability was quickly achieved in each stage owing to the successful start-up and reasonable regulation.
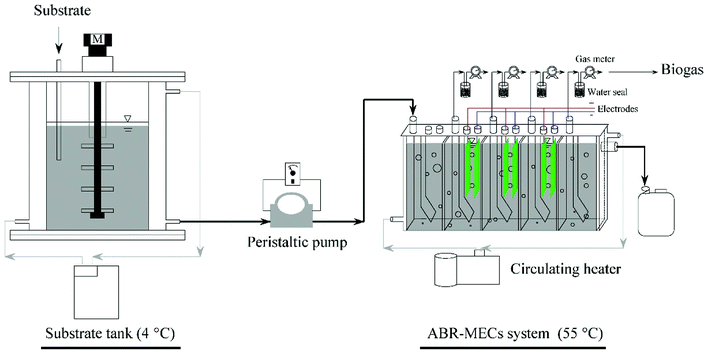 |
| Fig. 1 Schematic diagram of the ABR-MECs system. | |
2.3. Sampling and analytical methods
Effluent samples from the reactor and substrate tank were taken via sampling ports. All chemical analyses such as those of pH, alkalinity, COD, NH4+–N and PO43− followed standard methods.28 Data for the steady state were collected at least three times. The volume of biogas was recorded by a gas meter on a daily basis. A gas chromatograph (GC9790II, Fuli, China) was used to determine the composition of biogas. Gas samples were taken from each gas pipeline using a 1 mL syringe equipped with metal hub needles to measure the gas composition. Gas samples with volumes of 0.5 mL were injected into the GC with nitrogen as the carrier gas and hydrogen for combustion in the flame to determine the relative proportions of different biogas components. The biogas was monitored with a thermal conductivity detector equipped with a stainless-steel column (TDX-01, 2 m × 3 mm), and the temperatures of the column, injector, and detector were 120 °C, 120 °C and 150 °C, respectively. The contents of VFAs were measured with a flame ionization detector using a capillary column (ATFFAP, 30 m × 0.32 mm × 0.50 μm), and the temperatures of the column, injector, and detector were 150 °C, 210 °C, and 220 °C, respectively. A detailed protocol for the analysis of anaerobic metabolites and the gas composition was discussed in our previous study.26
Sludge samples from all five compartments (C1–C5) in the steady state in Stage 9 were collected to analyze the microbial community. Sludge samples from C1 were collected three times at different heights and were then mixed to extract DNA. The samples from C5 were handled in the same way as those from C1. Only the biofilms on the anodes and cathodes in C2–C4 were collected. The samples collected from the anode and cathode in each compartment were mixed to extract DNA using a FastDNA™ SPIN Kit for Soil (MP Biomedicals, LLC) three times, and then the DNA samples were mixed to conduct high-throughput 16S rRNA pyrosequencing. The V3–V4 regions of the 16S rRNA gene were amplified by PCR using the primers 341F (CCTACGGGNGGCWGCAG) and 785R (GACTACHVGGGTATCTAATCC) for bacteria and 787 (ATTAGATACCCSBGTAGTCC) and 1059R (GCCATGCACCWCCTCT) for archaea. Additional information about the DNA extraction, PCR and sequencing can be found elsewhere.29 The raw sequence data were deposited in the NCBI Short Reads Archive database under the accession number SRP150392.
2.4. Kinetic models
The first-order substrate removal model and Stover–Kincannon model were used to optimize the removal of COD.25,30
The removal of organic matter from anaerobic systems is expressed by the first-order model according to eqn (1):
|
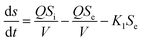 | (1) |
In pseudo-steady-state conditions, there is only a slight change in the substrate concentration (−ds/dt), and hence it can be eliminated from eqn (1) and eqn (2) can be introduced:
|
 | (2) |
where
Si and
Se represent the COD concentrations (g L
−1) of the influent and effluent, respectively, HRT is the hydraulic retention time (
d),
K1 is the speed constant for the removal of organic matter (1/
d),
Q is the influent flow (L d
−1), and
V is the working volume of the reactor (2.55 L).
Eqn (3)–(5), which describe the Stover–Kincannon model, are as follows:
|
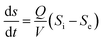 | (3) |
|
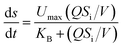 | (4) |
|
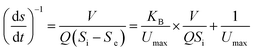 | (5) |
where d
s/d
t is the COD removal rate (g L
−1 d
−1),
Umax is the maximum consumption (utilization) rate (g L
−1 d
−1),
KB is the saturation constant (g L
−1 d
−1),
V is the working volume of the reactor (2.55 L),
Si and
Se are the COD concentrations of the influent and effluent, respectively (g L
−1), and
Q is the influent flow (L d
−1).
Similarly, the Van der Meer–Heertjes model and the modified Stover–Kincannon model were employed to determine the production rates of total gas and methane.
The model developed by Van der Meer and Heertjes has been widely used to determine methane production rates in anaerobic systems.31 In this model, the production of methane is associated with the Van der Meer–Heertjes kinetic constant (Ksg), the influent flow and the COD removal efficiency, as shown in eqn (6):
where
Vm is the methane production rate (L d
−1),
Q is the wastewater flow rate (L d
−1),
Si and
Se are the COD concentrations of the influent and effluent (g L
−1), respectively, and
Ksg is the Van der Meer–Heertjes kinetic constant (L g
−1 COD).
According to the modified Stover–Kincannon model, the quantity of biogas and the methane production rate depend on the removal of COD and the OLR, as expressed in eqn (7) and (8):
|
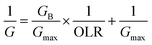 | (7) |
|
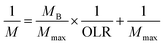 | (8) |
where
G is the specific biogas production rate (L (L
−1 d
−1)),
Gmax is the maximum specific biogas production rate (L (L
−1 d
−1)),
GB is the constant of proportionality (L (L
−1 d
−1)) for biogas production,
M is the specific methane production rate (L (L
−1 d
−1)),
Mmax is the maximum specific methane production rate (L (L
−1 d
−1)), and
MB is the constant of proportionality (L (L
−1 d
−1)) for methane production.
3. Results and discussion
3.1. Effect of OLR on substrate removal
In Phase I (Fig. 2a and b), the system was operated for different HRTs of 24 h, 21 h and 18 h with an influent COD concentration of 6 g L−1. Correspondingly, the COD removal rate was 95.4%, 94.6% and 80.5%, respectively. The COD removal efficiency declined sharply in Stage 3 when the HRT was changed from 21 h to 18 h. The COD removal efficiency in the five compartments at an HRT of 18 h was about 50.6%, 13.9%, 7.6%, 7.4% and 1.0%, respectively. The COD reduction rate in the last four compartments was low because the VFAs produced in the first compartment could not be effectively used in the subsequent compartments. This was consistent with the findings of Wu et al., who declared that exoelectrogens had longer generations and the efficiency of the degradation of VFAs decreased when the HRT was reduced.27 After Phase I, the operational scheme that was adopted is shown in Table 1. In Phase II, a longer HRT of 48 h was introduced for the acclimation of microbes, and the COD removal rate stabilized at about 95.8% at an OLR of 7.0 kg COD m−3 d−1. Thereafter, the HRT was reduced to 24 h and 21 h with OLRs of 14.0 and 16.0 kg COD m−3 d−1, respectively. Correspondingly, the COD removal efficiency declined instantly to about 91.8% and 90.3%, respectively, and then efficiencies of 95.5% and 94.7% were observed after the system attained a stable condition. Notably, although the OLR increased from 7.0 to 16.0 kg COD m−3 d−1, the COD removal rate declined slightly. Therefore, the influent COD concentration was further increased to 17 g L−1 in Phase III. Similar performance in terms of removal efficiency was observed with new OLRs in the range of 8.5–19.4 kg COD m−3 d−1, and the COD removal rate stabilized at 92.4%, 91.6% and 90.0% at HRTs of 48 h, 24 h and 21 h, respectively. Clearly, the COD removal efficiency decreased from 95.8% to 90.0% when the OLR increased from 7.0 to 19.4 kg COD m−3 d−1. In order to investigate the performance of the system at high OLRs, a rapid increase in the OLR to 22.9, 28.6 and 34.3 kg COD m−3 d−1 was carried out in Phase IV, and the COD removal rate finally reached 84.9%, 77.2% and 65.3%, respectively. Consequently, the highest removal efficiency was 95.8% when the OLR was 7.0 kg COD m−3 d−1, and the lowest COD reduction rate was 65.3% at an OLR of 34.3 kg COD m−3 d−1. When the OLR was less than 19.4 kg COD m−3 d−1, the COD removal rate exceeded 90.0%.
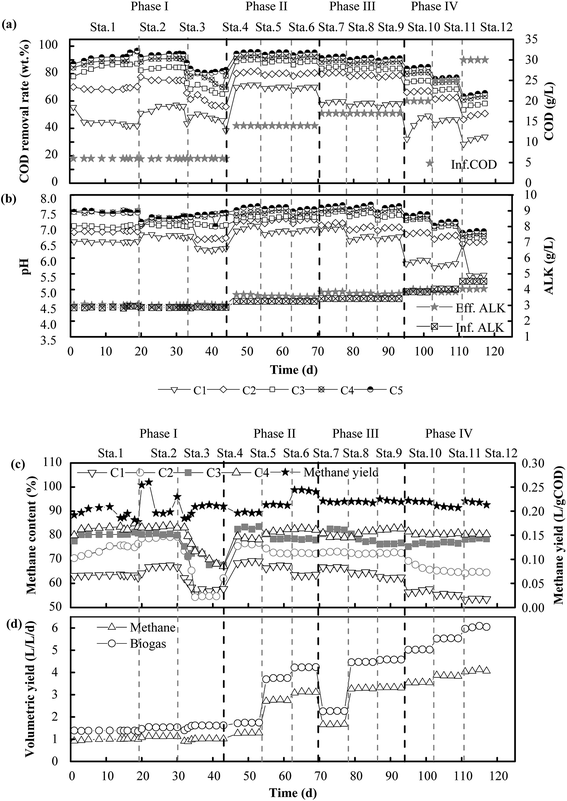 |
| Fig. 2 Performance of the ABR-MECs system at different COD concentrations and HRTs: COD removal rate and COD concentration (a), pH and ALK (b), methane content and methane yield (c), and volumetric yield of biogas and methane (d). C1, C2, C3, C4 and C5 denote the first to the fifth compartments of the reactor, respectively, in the direction of flow. | |
In terms of favorable OLRs, Fang et al. (2011)32 and Jing et al. (2013)33 reported that the best OLR ranges for anaerobic digestion were 2.5–3.2 kg COD m−3 d−1 and 1.4–16.0 kg COD m−3 d−1, respectively. Obviously, the OLRs of 7.0–19.4 kg COD m−3 d−1 that were found to be favorable in this experiment were higher than the values in these reports. Higher OLRs resulted from the effective degradation of VFAs (Fig. 3a–c). Acetic acid and butyric acid were dominant when the OLR was less than 19.4 kg COD m−3 d−1, and VFAs were rapidly degraded so that less than 500 mg L−1 remained in the effluent. In contrast, the concentration of propionic acid increased rapidly with a further increase in the OLR, and it eventually became dominant in all the compartments when the OLR increased to 34.3 kg COD m−3 d−1 (Fig. 3d–f). The degradation of VFAs at high OLRs could result from the synergistic action of microorganisms. Hydrolytic bacteria in the first compartment degraded organics to VFAs, which were further consumed by exoelectrogens and SFOB in the subsequent compartments. This was the reason why the concentration of propionic acid and acetic acid always remained low in the effluent of the system. This was consistent with the findings of a previous study, namely, that propionic acid could be rapidly oxidized to acetic acid by propionate oxidation bacteria in an AD process.34 One direct evidence was the fact that Syntrophobacter (5.0%), which is a propionic acid-degrading bacterium,35 was well enriched in the MEC compartments. Moreover, acetic acid can be substantially degraded in MECs.36 During the utilization of acetate for biogas production, a significant increase in the removal of COD, which was 1.7 times higher than that in the control group, was observed in an AD-MECs system.37 Another reason could be that their shorter generations and faster metabolic rate made exoelectrogens adapt to variations in the HRT more effectively than methanogens. Thus, the growth of exoelectrogens was boosted in the AD-MECs, and the bacterial population increased to a greater extent in the AD-MECs than in an AD system.38 This confirmed that SFOB and exoelectrogens were well enriched and performed well in the ABR-MECs system. Hence, an effective way to improve the stability of a thermophilic AD system comprises efficiently degrading VFAs by the introduction of the MECs. The results also suggested that 19.4 kg COD m−3 d−1 was the most favorable OLR and 34.3 kg COD m−3 d−1 was the maximum value (critical point for further acidification) for the ABR-MECs system used to treat carbohydrate-containing wastewater in thermophilic conditions.
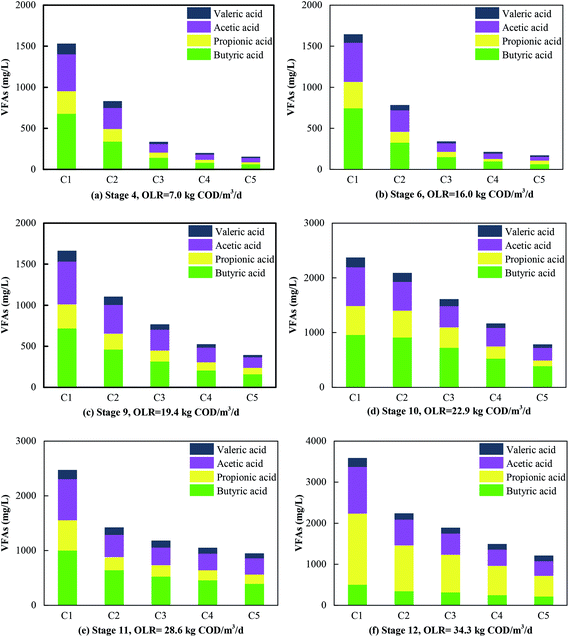 |
| Fig. 3 Contents of VFAs in the five compartments (C1–C5) at different OLRs. | |
3.2. Effect of OLR on methane production
The content and production rate of methane are illustrated in Fig. 2c and d. As the HRT was reduced from 48 h to 21 h with the variation in the COD concentration in the feed, the OLR increased from 7.0 to 34.3 kg COD m−3 d−1. Correspondingly, the methane production rate rose from 1.2 to 4.1 L (L−1 d−1) and the biogas production rate rose from 1.6 to 6.1 L (L−1 d−1). The results indicated that the increase in the volumetric production of methane was related to the increase in the OLR, whereas the methane yield was stable at 0.20–0.25 L g−1 CODremoved. Besides, the methane content in each compartment ranged from 60.4% to 85.3% in Phase II and Phase III and from 53.2% to 80.8% in Phase IV. The maximum methane content decreased from 85.3% to 80.8% when the OLR increased from 19.4 to 34.3 kg COD m−3 d−1. The results suggested that the performance of the ABR-MECs system was impaired when the OLR exceeded 19.4 kg COD m−3 d−1. The decrease was due to the reduction in pH with the increase in the OLR. The pH was stable at 6.50–7.70 when the OLR was lower than 19.4 kg COD m−3 d−1, which was favorable for acidogenic bacteria, methanogens and exoelectrogens,39 and the phenomenon of an imbalance between acid producers and consumers did not exist.
The methane content was higher than the value of 73.0–75.4% recorded in a pilot-scale UASB.40 Owing to the efficient utilization of VFAs via extracellular electron transfer by exoelectrogens, more carbon was converted into methane in situ.41 Therefore, purer methane was detected in the MEC compartments. This could have been due to the significant enrichment of hydrogenotrophic methanogens (Methanobacterium, 58.7%) in the ABR-MECs system. The proportion of Methanobacterium in the system was twice that in the inoculum (25.8%). The percentage of hydrogenotrophic methanogens was also much higher than that in a traditional AD system in which aceticlastic methanogens were always dominant among archaea. The increase in hydrogenotrophic methanogens was due to the enhanced production of H2 by exoelectrogens. Nevertheless, if this was the only way in which hydrogen was consumed by hydrogenotrophic methanogens, hydrogen should have been detected in the system, as hydrogenotrophic methanogens cannot completely remove hydrogen if the production of methane is only caused by the conversion of hydrogen.42 In fact, hydrogen was not detected in the ABR-MECs system. There could be other ways in which methane was generated. Electrochemical systems provide possibilities for new methanogenic pathways. Exoelectrogens are defined as kinds of microorganism that perform extracellular respiration. In particular, Methanobacterium could be a kind of methanogen with electrochemical activity.41 Methane could be produced by Methanobacterium via direct electron transfer via the reaction CO2 + 8H+ + 8e− → CH4 + 2H2O. This provides the theoretical possibility of the purification of carbon in situ, and more carbon can be converted stably into methane. Thus, the methane yield stabilized in the range of 0.20–0.25 L g−1 CODremoved when the OLR was in the wide range of 7.0–34.3 kg COD m−3 d−1. Moreover, the stable yield of methane provided a guarantee for simulating the dynamic process and predicting the volumetric production of methane.
3.3. Microbial community analysis
Sequence clustering analysis was conducted in accordance with similarity and the representation of OTUs.43 To confirm the diversity and richness of the microbial community, the Shannon, ACE and Chao indices were estimated. The Chao and ACE indices are related to the richness of microbes, whereas the Shannon index is negatively related to the diversity of species.44 The average values of the Chao, ACE and Shannon indices in C2–C4 were 298, 301 and 3.55, respectively, which were lower than those in C1 (352, 358, 3.59) and C5 (382, 395, 4.01). This also suggested that although the richness of microbes was lower in C2–C4 than in C1 and C5, the diversity increased in C2–C4. A visual expression is presented in Fig. 4. PCA analysis visually indicates that the bacteria in C2–C4 are far away from those in C1 and C5. The microbes in C2–C4 were greatly different from those in C1 and C5 owing to the introduction of the MECs. This was further elaborated as follows.
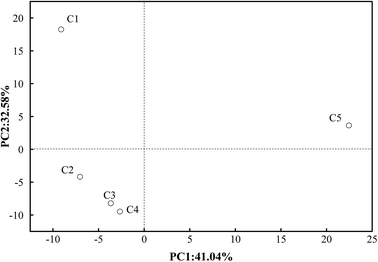 |
| Fig. 4 PCA analysis of eubacteria in the five compartments (C1–C5). | |
At the phylum level, the bacterial components in C1 were Firmicutes (31.8%), unclassified bacteria (28.2%), Chloroflexi (12.5%), Spirochaetes (10.1%), Verrucomicrobia (4.3%) and Proteobacteria (3.2%). However, in C2 (which represents the MEC compartments), they were unclassified bacteria (52.6%), Firmicutes (14.7%), Proteobacteria (9.7%), Acetothermia (4.6%), Chloroflexi (3.9%), Planctomycetes (3.5%), Verrucomicrobia (2.6%), Spirochaetes (1.9%), Thermotogae (1.8%) and Actinobacteria (1.3%). From these results, it can be observed that Firmicutes were the dominant bacteria in both the inoculum and the MEC compartments of the ABR-MECs system, but the abundance of Firmicutes in the MECs was less than that in the inoculum, and the proportion of unclassified bacteria in the MECs increased significantly. Moreover, the dominant archaea changed from Methanosaeta in the inoculum to Methanobacterium in the MECs. These phenomena confirmed the influence of the MECs on the microbial community in the AD system. Besides, more unclassified bacteria and Proteobacteria and fewer Chloroflexi were detected in C2 in comparison with C1. Recently, similar phyla, including Proteobacteria, Firmicutes, Chloroflexi and Bacteroidetes, were reported to be the dominant phyla in anaerobic sludge.44 Firmicutes and Proteobacteria were reported to be the dominant phyla in high-temperature electrochemical systems and anaerobic systems.45 Therefore, the presence of Chloroflexi in C1 was attributed to hydrolytic fermentative functionalities.46 The synergistic coexistence of Chloroflexi and Firmicutes in C1 was suggested to have contributed to the hydrolysis and acidogenesis processes, and Proteobacteria and unclassified bacteria exercised heterotrophic and electrochemical functionalities in the MEC compartments. Thus, the high abundance of dominant bacteria in different regions supplied the biological basis for the enhancement in the performance of the ABR-MECs system.
At the genus level, the relative abundances are shown in Fig. 5. The dominant bacteria in C1 were hydrolytic acidification bacteria such as Thermohydrogenium (17.1%), unclassified Chloroflexi (8.7%), and Treponema (6.7%), whereas the main bacteria in C2 were Clostridium (6.0%), Syntrophobacter (5.0%) and Acetothermia (4.6%). The content of unclassified bacteria increased from 28.2% in C1 to 52.9% in C2 and then decreased to 48.3%, 40.7% and 35.7% in C3, C4 and C5, respectively. The obvious downward trend could be due to the concentration of the substrate in the different compartments. Notably, the microbial communities were significantly different in the MEC compartments in comparison with those in C1 and C5, and unclassified bacteria grew in large quantities. The thermophilic members of the exoelectrogen group need further investigation in future work. Fig. 5b shows the content of archaea. Eight species of archaea were identified in the thermophilic ABR-MECs system. The dominant archaea, taking C4 as an example, were Methanobacterium (58.7%), Methanosaeta (18.2%), Methanomassiliicoccus (14.9%) and Methanolinea (3.2%), which belong to the phylum Euryarchaeota. Specifically, Methanobacterium in the ABR-MECs was suggested to exercise hydrogenotrophic and extracellular electron transfer functionalities.47 Methane could be produced by Methanobacterium by direct electron transfer in the biocathode via the reaction CO2 + 8H+ + 8e− → CH4 + 2H2O.48 In all, high contents of hydrolytic bacteria (e.g., Thermohydrogenium and Chloroflexi) were found in C1, whereas more SFOB (e.g., Syntrophobacter), exoelectrogens (e.g., Clostridium and Methanobacterium) and hydrogenotrophic methanogens (e.g., Methanobacterium) were detected in C2–C4.
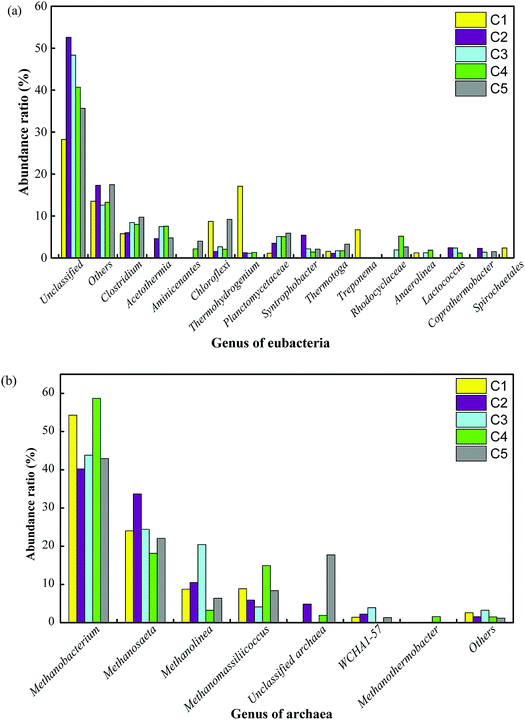 |
| Fig. 5 Distribution of functional bacterial and archaeal populations (sequence reads ≥ 1%) in the anaerobic sludge obtained from the five compartments (C1–C5): genus of eubacteria (a) and genus of archaea (b). | |
The reciprocity of hydrolytic bacteria, SFOB, exoelectrogens and hydrogenotrophic methanogens in the ABR-MECs system smoothly degraded organic matter via the following metabolic pathways: (1) hydrolytic bacteria in the first compartment degraded the substrate into micromolecular organic matter. (2) Organic substances that are difficult to utilize (e.g., propionic acid and butyric acid) were rapidly degraded to acetic acid by SFOB and in turn exploited by exoelectrogens in the anode. (3) Electrons were transferred to the cathode, directly obtained by Methanobacterium or transferred to H2 and then used by hydrogenotrophic methanogens to generate methane. Thus, the consumption of acetic acid by exoelectrogens relieved the inhibition of SFOB by acetic acid and further promoted the enrichment of hydrogenotrophic methanogens. The MECs in the AD system provided continuous electrical stimulation for the enrichment of target microorganisms. The microbial communities revealed that hydrolytic acidification bacteria, SFOB, exoelectrogens and hydrogenotrophic methanogens could form dominant communities in different regions of the system and synergistically degrade VFAs at high OLRs. The distribution of microorganisms theoretically explained the phenomena that the efficient removal of the substrate and production of methane could be achieved in the ABR-MECs system, but the dynamic relations need to be further revealed to optimize the system.
3.4. Modeling of substrate removal and methane production
For a quantitative analysis of the relationship between the OLR, the removal of the substrate and the generation of methane, the kinetic coefficients of the first-order and Stover–Kincannon models were used for determining the removal of COD. Then, the coefficients and models that were obtained were evaluated and the removals of COD were predicted.
3.4.1. First-order and Stover–Kincannon models for substrate removal. As shown in Fig. 6a, data for the steady state of each stage were used to determine the kinetic coefficients of the first-order model. A linear equation was achieved with a kinetic constant K1 of 3.08789, and the correlation coefficient (R2) was equal to 0.597. It is clear that the linear fitting was poor and the degradation of the substrate was not consistent with the first-order model.
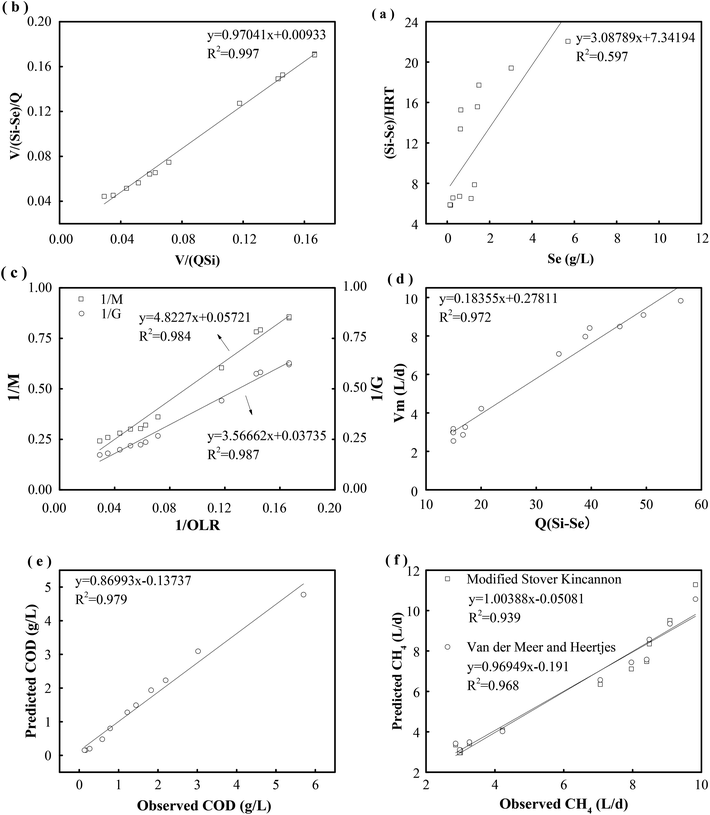 |
| Fig. 6 Determination of kinetic constant in first-order model for removal of the substrate (a). Determination of kinetic constant in Stover–Kincannon model for removal of the substrate (b). Kinetic constants for the production of biogas and methane determined by the modified Stover–Kincannon model (c). Kinetic constant for the production of methane determined by the Van der Meer–Heertjes model (d). Observed and predicted COD concentrations in effluent determined by the Stover–Kincannon kinetic model (e). Observed and predicted production of methane determined by the modified Stover–Kincannon and Van der Meer–Heertjes models (f). | |
The results of a kinetics study using the Stover–Kincannon model30 are presented in Fig. 6b. A positive linear correlation is observed between the reduction of COD and the OLR. From the intercept and slope of the plotted line, the maximum utilization rate (Umax) and saturation constant (KB) for the removal of COD were 107.5 and 104.3 g COD L−1 d−1, respectively, and the correlation was strong (R2 = 0.997). The maximum utilization rate (Umax) in each compartment was also estimated to determine the rate of degradation of the substrate. The Umax values were 95.7 and 45.4 g COD L−1 d−1 in C1 and C2, respectively, which indicated that the maximum rate of consumption of COD in C1 was twice that in C2. Therefore, a large percentage of the COD in the reactor was consumed in the first compartment. Besides, the Umax values in C3, C4 and C5 were 26.3, 11.4 and 9.8 g COD L−1 d−1, respectively. This reveals that the maximum rate of consumption of COD gradually decreased from C1 to C5.
According to the Stover–Kincannon model, the relationship between Se, Si and the HRT is described by eqn (9). To further confirm the accuracy of the Stover–Kincannon model, eqn (9) was used to predict the concentration of COD (Fig. 6e). Notably, the predicted values agree with the experimental values with a strong correlation (R2 = 0.979). This suggests that eqn (9) based on the Stover–Kincannon model is able to make reliable predictions of the removal of COD:
|
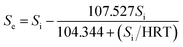 | (9) |
where
Si and
Se are the COD concentrations of the influent and effluent (g L
−1) and HRT is the hydraulic retention time (
d).
3.4.2. Modified Stover–Kincannon model and Van der Meer–Heertjes model for the production of biogas. The results of a kinetics study using the modified Stover–Kincannon model are presented in Fig. 6c. The coefficients (R2) of the modified Stover–Kincannon model for the production of biogas and methane were 0.987 and 0.984, respectively. The maximum specific gas production rate (Gmax) and the constant of proportionality (GB) were 26.8 L (L−1 d−1) and 95.492 (dimensionless). The highest methane gas production rate (Mmax) and the constant of proportionality (MB) were 17.5 L (L−1 d−1) and 84.298 (dimensionless), respectively. On the other hand, the results of kinetics studies using the Van der Meer–Heertjes model are presented in Fig. 6d. The R2 coefficient was 0.972. It is difficult to judge these two models because their R2 coefficients were similar.Predicted values of methane yields obtained from the two models are plotted against experimental values for the production of methane (Fig. 6f). The R2 value of the modified Stover–Kincannon model was 0.939, whereas it was 0.968 for the Van der Meer–Heertjes model. The output of the Van der Meer–Heertjes model agrees better with the experimental results. Hence, the Van der Meer–Heertjes model was employed to estimate the production of methane. According to the Van der Meer–Heertjes model, the relationship between Vm, Si, Se and HRT can be described by eqn (10) to make reliable predictions of the production of methane:
|
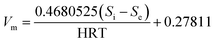 | (10) |
where
Vm is the methane production rate (mL d
−1),
Si and
Se are the COD concentrations of the influent and effluent (g L
−1), respectively, and HRT is the hydraulic retention time (
d).
By the establishment of these two models, the relationship between the removal of COD, the production of methane and the OLR was clearly determined for the treatment of such carbohydrate-containing wastewater. It further revealed the quantitative relationships between the concentrations of organics (in and outside the substrate, measured in terms of COD) and the HRT. Plotting the variables is helpful for determining the optimal control and providing precise predictions for further improving the performance of a thermophilic ABR-MECs system. In addition, it is beneficial for predicting trends in the removal of organics and the production of methane at a high OLR and avoiding decreases in performance with an increase in the OLR when dealing with easily acidified substrates.
4. Conclusion
An investigation of the performance of an ABR-MECs system in the thermophilic digestion of carbohydrate-containing wastewater was conducted in this study in qualitative and quantitative terms. The highest COD removal efficiency was 95.8% at an OLR of 7.0 kg COD m−3 d−1. It decreased to 65.3% when the OLR was 34.3 kg COD m−3 d−1, and the methane yield stabilized at 0.20–0.25 L g−1 CODremoved. The kinetics and predictions according to the Stover–Kincannon and Van der Meer–Heertjes models closely agreed with the removal of COD and volumetric production of methane, respectively, with high correlation coefficients (R2) of 0.979 and 0.968. The proposed models enabled precise control of the system. A microbial analysis revealed that the dominant microbes found in C1 were hydrolytic bacteria (e.g., Thermohydrogenium and Chloroflexi), whereas SFOB (e.g., Syntrophobacter), exoelectrogens (e.g., Clostridium and Methanobacterium) and hydrogenotrophic methanogens (e.g., Methanobacterium) were found to be dominant in the MEC compartments. The microbial community suggested that the rapid degradation of VFAs and the production of considerable quantities of methane were achieved by the synergistic effect of different functional microbes in the thermophilic ABR-MECs system.
Conflicts of interest
The authors declare that they have no conflict of interest.
Acknowledgements
The authors would like to thank the National Key Research and Development Program of China (Contract No. 2017YFD0800804-03), the National Natural Science Foundation of China (Contract No. 21477122, 51678553, 21276248, 51808525, 21876167), the Project of the Natural Science Foundation of Fujian Province (Contract No. 2016J01273, 2017J05092), IUE CAS Young Talents Frontier Project (Contract No. IUEQN201501), the Xiamen Science and Technology Project (Contract No. 3502Z20182003) and FY2015 Japanese-China Research Cooperative Program (Contract No. 2016YFE0118000) for their support for this study.
References
- G. Capsontojo, R. Moscoviz, D. Ruiz, G. Santacatalina, E. Trably and M. Rouez, et al., Bioresour. Technol., 2018, 260, 157–168, DOI:10.1016/j.biortech.2018.03.097.
- X. Liu, B. Dong and X. Dai, Bioresour. Technol., 2013, 148, 461–466, DOI:10.1016/j.biortech.2013.08.118.
- D. Zhang, H. Yuan, B. Yu, X. Dai, X. Huang, Z. Lou and N. Zhu, RSC Adv., 2016, 6, 99524–99531, 10.1039/c6ra21111a.
- Y. Li, Y. Zhang, X. Kong, L. Li, Z. Yuan and R. Dong, J. Chem. Technol. Biotechnol., 2017, 92, 2538–2545, DOI:10.1002/jctb.5260.
- J. R. Town and T. J. Dumonceaux, Appl. Microbiol. Biotechnol., 2016, 100, 1009–1017, DOI:10.1007/s00253-015-7058-3.
- I. A. Fotidis, L. Treu and I. Angelidaki, J. Cleaner Prod., 2017, 166, 1305–1313, DOI:10.1016/j.jclepro.2017.08.151.
- E. J. Martinez-Conesa, V. M. Ortiz-Martinez, M. J. Salar-Garcia, A. P. De Los Rios, F. J. Hernandez–Fernandez and L. J. Lozano, Chem. Eng. Commun., 2017, 204, 97–104, DOI:10.1080/00986445.2016.1236336.
- S. Gajaraj, Y. Huang, P. Zheng and Z. Hu, Biochem. Eng. J., 2017, 117, 105–112, DOI:10.1016/j.bej.2016.11.003.
- Z. Dou, C. M. Dykstra and S. G. Pavlostathis, Sci. Total Environ., 2018, 663, 1012–1021, DOI:10.1016/j.scitotenv.2018.03.255.
- H. Liu, F. Leng, Y. Guan, Y. Yao, Y. Li and S. Xu, Water, Air, Soil Pollut., 2017, 228, 179, DOI:10.1007/s11270-017-3342-4.
- J. Zhang, Y. Zhang, X. Quan and S. Chen, Water Res., 2013, 47, 5719–5728, DOI:10.1016/j.watres.2013.06.056.
- K. S. Choi, S. Kondaveeti and B. Min, Bioresour. Technol., 2017, 245, 826–832, DOI:10.1016/j.biortech.2017.09.057.
- G. Mohanakrishna, M. S. Venkata and P. N. Sarma, J. Hazard. Mater., 2010, 177, 487–494, DOI:10.1016/j.jhazmat.2009.12.059.
- Z. Yu, X. Leng, S. Zhao, J. Ji, T. Zhou and A. Khan, et al., Bioresour. Technol., 2018, 255, 340–348, DOI:10.1016/j.biortech.2018.02.003.
- A. Zhou, J. Zhang, W. Cai, R. Sun, G. Wang and W. Liu, et al., Int. J. Hydrogen Energy, 2016, 42, 9044–9050, DOI:10.1016/j.ijhydene.2016.02.075.
- M. I. Baloch, Water Environ. J., 2011, 25, 171–180, DOI:10.1111/j.1747-6593.2009.00206.x.
- D. I. Masse and R. L. Droste, Water Res., 2000, 34, 3087–3106, DOI:10.1016/S0043-1354(00)00064-6.
- J. Fernandez-Rodríguez, M. Perez and L. I. Romero, Chem. Eng. J., 2013, 232, 59–64, DOI:10.1016/j.cej.2013.07.066.
- A. Donoso-Bravo, J. Mailier, C. Martin, J. Rodríguez, C. A. Aceves-Lara and A. V. Wouwer, Water Res., 2011, 45, 5347–5364, DOI:10.1016/j.watres.2011.08.059.
- K. B. Prajapati and R. Singh, Bioresour. Technol., 2018, 263, 491–498, DOI:10.1016/j.biortech.2018.05.036.
- H. Kil, Y. Xi and D. Li, Chem. Eng. Commun., 2017, 204, 1428–1444, DOI:10.1080/00986445.2017.1367670.
- D. J. Batstone, D. Puyol, X. Flores-Alsina and J. Rodríguez, Rev. Environ. Sci. Bio/Technol., 2015, 14, 595–613, DOI:10.1007/s11157-015-9376-4.
- C. Liu, X. Yuan, G. Zeng, W. Li and J. Li, Bioresour. Technol., 2008, 99, 882–888, DOI:10.1016/j.biortech.2007.01.013.
- D. Yang, L. Deng, D. Zheng, G. Liu, H. Yang and L. Wang, Fuel, 2015, 144, 237–243, DOI:10.1016/j.fuel.2014.12.044.
- G. Abbas, L. Wang, W. Li, M. Zhang and P. Zheng, Ecol. Eng., 2015, 74, 356–363, DOI:10.1016/j.ecoleng.2014.09.035.
- R. Zou, G. Zhu, J. A. Kumar, C. Liu, X. Huang and L. Liu, Int. J. Hydrogen Energy, 2014, 39, 13498–13504, DOI:10.1016/j.ijhydene.2014.02.086.
- T. Wu, G. Zhu, A. K. Jha, R. Zou, L. Liu and X. Huang, Int. J. Hydrogen Energy, 2013, 38, 11117–11123, DOI:10.1016/j.ijhydene.2013.03.029.
- APHA, Standard Methods for the Examination of Water and Wastewater, American Public Health Association, Washington DC, 22nd edn, 2012, http://www.standardmethods.org/ Search PubMed.
- M. Qiao, Y. Qu, W. Shen, Z. Zhang, J. Wang, Z. Liu, D. Li, H. Li and J. Zhou, Bioresour. Technol., 2015, 179, 436–443, DOI:10.1016/j.biortech.2014.12.041.
- Q. S. Kusçu and D. T. Sponza, J. Hazard. Mater., 2009, 161, 787–799, DOI:10.1016/j.jhazmat.2008.04.039.
- A. Ebrahimi, H. Hashemi, H. Eslami, R. A. Fallahzadeh, R. Khosravi and R. Askari, J. Environ. Manage., 2017, 206, 707–714, DOI:10.1016/j.jenvman.2017.10.038.
- C. Fang, K. Boe and I. Angelidaki, Bioresour. Technol., 2011, 102, 5734–5741, DOI:10.1016/j.biortech.2011.03.013.
- Z. Jing, Y. Hu, Q. Niu, Y. Liu, Y. Li and X. Wang, Bioresour. Technol., 2013, 137, 349–357, DOI:10.1016/j.biortech.2013.03.137.
- Y. Li, Y. Zhang, Y. Sun, S. Wu, X. Kong, Z. Yuan and R. Dong, Bioresour. Technol., 2017, 231, 94–100, DOI:10.1016/j.biortech.2017.01.068.
- V. T. Sedanonunez, S. Boeren, A. Stams and C. M. Plugge, Environ. Microbiol., 2018, 20, 1842–1856, DOI:10.1111/1462-2920.14119.
- P. D. Kiely, D. F. Call, M. D. Yates, J. M. Regan and B. E. Logan, Appl. Microbiol. Biotechnol., 2010, 88, 371–380, DOI:10.1007/s00253-010-2757-2.
- T. Bo, X. Zhu, L. Zhang, Y. Tao, X. He and D. Li, Electrochem. Commun., 2014, 45, 67–70, DOI:10.1016/j.elecom.2014.05.026.
- B. Lee, J. G. Park, W. B. Shin, D. J. Tian and H. B. Jun, Bioresour. Technol., 2017, 234, 273–280, DOI:10.1016/j.biortech.2017.02.022.
- G. C. Gil, I. S. Chang, B. H. Kim, M. Kim, J. K. Jang and H. S. Park, Biosens. Bioelectron., 2003, 18, 327–334, DOI:10.1016/S0956-5663(02)00110-0.
- Y. Li, Y. Yang, W. Jiang, H. Wei and C. Sun, Environ. Sci. Pollut. Res., 2015, 22, 2599–2610, DOI:10.1007/s11356-014-3514-3.
- T. Sangeetha, Z. Guo, W. Liu, M. Cui, C. Yang and W. Ling, Int. J. Hydrogen Energy, 2016, 41, 2189–2196, DOI:10.1016/j.ijhydene.2015.11.111.
- H. V. M. Hamelers, A. T. Heijne and T. H. J. A. Sleutels, et al., Appl. Microbiol. Biotechnol., 2010, 85, 1673–1685, DOI:10.1007/s00253-009-2357-1.
- Z. Cheng, X. Hu and Z. Sun, Chem. Eng. J., 2016, 303, 137–144, DOI:10.1016/j.cej.2016.05.131.
- J. Guo, Y. Peng, B. Ni, X. Han, L. Fan and Z. Yuan, Microb. Cell Fact., 2015, 14, 33, DOI:10.1186/s12934-015-0218-4.
- B. C. Jong, B. H. Kim, I. S. Chang, P. W. Y. Liew, Y. F. Choo and G. S. Kang, Environ. Sci. Technol., 2006, 40, 6449–6454, DOI:10.1021/es0613512.
- L. R. Lynd, P. J. Weimer, W. H. Van Zyl and I. S. Pretorius, Microbiol. Mol. Biol. Rev., 2002, 66, 506–577, DOI:10.1128/MMBR.66.4.739.2002.
- H. D. Ariesyady, T. Ito and S. Okabe, Water Res., 2007, 41, 1554–1568, DOI:10.1016/j.watres.2006.12.036.
- S. Cheng, D. Xing, D. F. Call and B. E. Logan, Environ. Sci. Technol., 2009, 43, 3953–3958, DOI:10.1021/es803531g.
|
This journal is © The Royal Society of Chemistry 2018 |
Click here to see how this site uses Cookies. View our privacy policy here.