DOI:
10.1039/C8LC00792F
(Paper)
Lab Chip, 2019,
19, 59-67
An inexpensive smartphone-based device for point-of-care ovulation testing†
Received
1st August 2018
, Accepted 1st November 2018
First published on 11th December 2018
Abstract
The ability to accurately predict ovulation at-home using low-cost point-of-care diagnostics can be of significant help for couples who prefer natural family planning. Detecting ovulation-specific hormones in urine samples and monitoring basal body temperature are the current commonly home-based methods used for ovulation detection; however, these methods, relatively, are expensive for prolonged use and the results are difficult to comprehend. Here, we report a smartphone-based point-of-care device for automated ovulation testing using artificial intelligence (AI) by detecting fern patterns in a small volume (<100 μL) of saliva that is air-dried on a microfluidic device. We evaluated the performance of the device using artificial saliva and human saliva samples and observed that the device showed >99% accuracy in effectively predicting ovulation.
Introduction
Family planning reinforces people's rights to determine the number and spacing of their children. The timing of sexual intercourse in relation to ovulation strongly influences the chance of successful conception and can be an effective method for natural family planning.1 Nearly 44% of all pregnancies worldwide are unplanned or unintended.2,3 Unintended pregnancies can have adverse consequences to the health of both mother and child and are subject to significant economic and social burden. In addition, expenditure on births, abortion, and miscarriages resulting from unintended pregnancies have cost over $21 billion of public funds in the US in 2010 alone.4,5 A study has also shown that family planning services alone have helped in reducing unintended pregnancies and the costs related to it by nearly 70%.5,6 A simple, low-cost tool, which can aid in family planning, will empower women in avoiding unintended pregnancies and will be of great value to those patients who are trying to conceive.
Natural family planning uses physical signs, symptoms, and physiologic changes to predict a woman's fertility.7,8 Some of the methods for monitoring woman's fertility include ovulation detection through luteinizing hormone (LH) level determination, salivary beta-glucuronidase activity evaluation, rectal or oral basal body temperature analysis, cervical mucus characterization, and salivary ferning analysis.9 Salivary ferning analysis is relatively inexpensive and simple, making it an attractive alternative to most available approaches.10 During the follicular phase of the menstrual cycle, with the increase of estradiol levels in the blood there is an increase in the salivary electrolytes which results in consistent ferning appearance, a crystallized structure resembling fern leaves, in air-dried saliva and can be used to determine the ovulation period.10,11 Ferning structures have been observed in ovulating women within a 4-day window around the day of ovulation.12 However, current ovulation tests based on salivary ferning are manual and highly subjective, which causes misinterpretation when performed by a lay consumer.13
Artificial Intelligence (AI) is gaining acceptance in medicine and one of its major applications in healthcare is its ability to accurately interpret complex medical data with super-human accuracy and consistency.14 AI has already been shown to perform exceptionally well in applications such as predicting cardiovascular risk,15 skin cancer screening,16 and diabetic retinopathy detection.17 Advances in microelectronics and machine learning have enabled current AI-based methods to work equally well on portable systems, especially smartphones. More than 4.4 billion people across the globe use smartphones making it an excellent candidate for the development of point-of-care technologies.18 In fact, smartphone cameras have been used in point-of-care tests for both qualitative and quantitative detection of clinically relevant biomarkers for disease detection or treatment monitoring such as HIV/AIDS and syphilis detection,19–21 herpes,22 sickle cell,23 male infertility,24–29 and Zika.30,31
Here, we report a simple, low-cost, and automated smartphone-based device for point-of-care ovulation testing that uses AI for the accurate detection of ferning patterns in a small volume (∼100 μL) of air-dried saliva samples placed on a microfluidic device (Fig. 1). We used a neural network to rapidly (<31 seconds) analyze and detect ferning patterns in air-dried saliva samples. The neural network utilizes the MobileNet architecture pre-trained with 1.4 million ImageNet images and retrained with salivary ferning images. The smartphone-based device was able to detect ovulation with an accuracy of 99.5% when tested with 200 images of human saliva collected during the ovulating and non-ovulating phases of the menstrual cycle among 6 women.
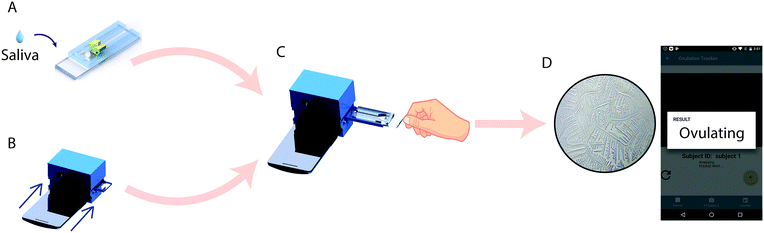 |
| Fig. 1 Steps of operation for smartphone-based salivary ferning test. (A) Saliva is collected on the microfluidic device and is smeared with a smearing block and is left to be air-dried. (B) The optical system is attached to a compatible smartphone for testing. (C) The microfluidic device with the air-dried sample is inserted into the optical attachment and the test is initialized using the developed custom android application. (D) The software analyzes the fern pattern exhibited by the air-dried saliva samples and identifies whether the subject is in her ovulating or non-ovulating phase of the menstrual cycle. | |
Materials and methods
Study design
The goal of this study was to develop an automated device for detecting ovulation in women by salivary ferning test. 6 healthy women, aged from 20 to 22 participated in the study. The subjects were selected based on the following criteria: 1) they had a history of normal menstrual cycles; 2) they did not consume tobacco and alcohol and 3) they did not use hormonal contraception during the study period. The Human Studies Institutional Review Boards of the Brigham and Women's Hospital approved the subject recruitment and use of human saliva specimens (IRB2017P002139). Recruited subjects provided informed consent prior to the collection of saliva samples. The subjects were each given 1.2 ml micro-centrifuge tubes to collect the sample early morning prior to brushing their teeth. The saliva samples were collected during their ovulating and non-ovulating phases. The two different phases were identified using the Clear Blue® urine test (LH + estrogen) as the reference. The samples were loaded on the microfluidic device and analyzed using a smartphone-based optical system. Manual analysis was also performed for the presence of salivary ferning using a microscope during the training phase of the network.
Optical attachment
To carry out this study, we developed a smartphone-based optical attachment (83 × 97 × 74 mm) that was 3D printed with an Ultimaker 2 Extended (Ultimaker) and polylactic acid (PLA) (Ultimaker) as the printing material (Fig. 2A). The optical setup of the device contained a plano-convex lens of 9 mm diameter with a focal length of 13.5 mm for magnification and a standard acrylic lens with 12 mm diameter and 30 mm focal length as a condenser. The lenses were aligned with the optical axis of the smartphone's front camera. The attachment was designed in SolidWorks (Dassault Systèmes) for the Moto X smartphone (Motorola, XT1575). The X-axis (parallel to the lens setup) movement of the microfluidic device was achieved by using a 6 V, 100 rpm DC gear motor (B07556CZL1, Walfront) with a M3 lead screw attached to the shaft. The device was powered by a 9 V alkaline battery (B000099SKB, Energizer). A 5 V diffused white light emitting diode (LED) (IL153, Microtivity) was used to illuminate the microfluidic chip. A single-board microcontroller (NodeMCU) was used to control the movement of the microfluidic device. The circuit diagram of the setup is provided in the supplementary information (Fig. S1†).
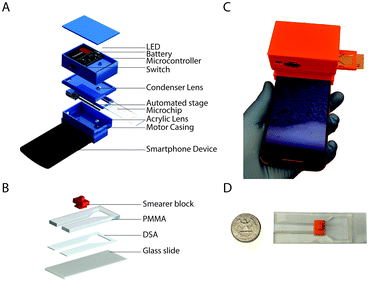 |
| Fig. 2 Schematic illustration of the smartphone-based optical system and the disposable microfluidic device. (A) The exploded image of the stand-alone optical system and its various components. The system is wirelessly controlled using a smartphone to image fern structures on the microfluidic device. (B) The actual image of the fabricated stand-alone optical system with a smartphone for imaging and analysis of the fern structures in air-dried saliva samples. (C) The exploded view of the microfluidic device with the smearer block. (D) An actual microfluidic device placed next to a US quarter coin. | |
Microfluidic device fabrication
The microfluidic device was composed of poly(methyl methacrylate) (PMMA) (8560K239, McMaster Carr), double-sided adhesive (DSA), polylactic acid (PLA) and glass slide (VWR, 48300-025 corning 75 × 25 mm). Transparent PMMA and DSA sheets were cut using a laser cutter (VLS 2.3, Universal Laser Systems Inc.). The laser cutter was also used to etch the PMMA in a controlled manner to create grooves. The grooves were etched on the PMMA such that the inner side of the fully built microfluidic chip would act as guide-ways for the smear block. A small area (25 × 12 mm2) was left open on one end of the glass slide for handling the microfluidic device (Fig. 2B–D). One side of DSA was stuck to the etched groove side of the PMMA and the other side was stuck to the glass slide, which was used as the lower substrate for the microchip. The smearer block (12 × 8 × 9.6 mm) was printed using an Ultimaker 2 Extended (Ultimaker) 3D printer and PLA as the printing material. The smearer block was used to smear the sample within the channel (40 × 5 mm2) of the microfluidic device to get a controlled thin film of saliva. The microfluidic device also had a small reservoir for sample loading on-chip.
Android application
An android application was developed for image data acquisition using the smartphone optical attachment and analyzing the fern patterns present in air-dried saliva to predict the ovulation status. When a microfluidic device with a loaded sample was inserted into the optical attachment, the microfluidic device was moved in parallel to the lens setup to automatically image the entire channel for salivary ferning. The android application controlled the motors through the NodeMCU and therefore the movement of the microfluidic device. Wireless communication between the smartphone and the optical system was achieved by configuring the NodeMCU as a HTTP web server. The application captured videoframes of the microchannel at a rate of ∼5 frames per second (fps).
The videoframes were sampled at 4× magnification and each frame was passed into a trained network to detect the presence of ferning. Inference time to get the result for each frame was ∼200 ms for the Moto X smartphone and may vary for different smartphones. The user interface of the smartphone application was designed to be simple and convenient to use. Furthermore, the results were stored in a calendar event to track the ovulation cycle. The Android application automatically captured video frames, analyzed the images locally, and reported the result in <31 s. The system does not require access to the internet for analysis.
Data collection
The voluntary participants were asked to collect the saliva samples early morning before brushing to exclude any discrepancies in the salivary electrolyte concentration due to individual's daily activities. The Human Studies Institutional Review Boards of the Brigham and Women's Hospital approved the subject recruitment and use of human saliva specimens (IRB2017P002139). We collected samples every day from each participant for one complete menstrual cycle and tested them using our microfluidic devices through both the ovulating and non-ovulating phases. We also used artificial saliva to simulate ferning patterns for the training and testing of the AI algorithm. We simulated 30 non-ovulating samples ranging from 0.1% to 1.4% and 34 ovulating samples ranging from 5.6% to 100% of the artificial saliva stock. The samples were prepared to cover a large range of ferning and non-ferning structures. The prepared samples were loaded on to the microfluidic devices and were smeared across the narrow channel with the help of a smearing block. The air-dried saliva samples were then imaged using the smartphone-based optical system. Overall, 1640 images were obtained from both human and artificial saliva samples and were classified into 476 ovulating images and 1164 non-ovulating images based on the ferning patterns. The annotations for ferning and non-ferning samples were achieved through manual inspection under a desktop microscope for simulated samples. The ovulation status for human saliva samples was established through the urine test (LH + estrogen) in addition to manual verification.
MobileNet training
To classify the fern structures on a smartphone, MobileNet pre-trained with 1.4 million images of ImageNet with a width multiplier parameter α = 0.50 and an image size of 224 × 224 pixels was used as our neural network architecture.32 ImageNet, an open source dataset that contains non-saliva images from various categories, helped in pre-training the network. For a complex neural network architecture such as MobileNet, a dataset of ∼1500 images is not sufficient to optimize its weights during training. Therefore, we used a varied large-scale dataset to ‘pre-train’ the network and then refine it to work for our application of analyzing salivary ferning. The utilized model of MobileNet performed with a top-1 accuracy of 64.0% and a top-5 accuracy of 85.4% across 1000 classes of ImageNet database.
MobileNet was specifically designed for efficient use of limited resources available on embedded devices such as smartphones. The network makes use of depth-wise separable convolutions to build light-weight deep neural networks unlike traditional convolution neural networks that use standard 2D convolution. A standard 2D convolution applies convolution kernel to all channels of the input image. 2D convolution slides the kernels across all input image channels and computes a weighted sum of input pixels applied by kernel for all input channels. Depth-wise convolution is a spatial separable convolution performed on separate channels whose output is computed into a new channel by a pointwise convolution (standard convolution with the 1 × 1 kernel).
MobileNet structures have been built with depth-wise separable convolutions except for the first layer, which has been built with a standard 3 × 3 convolution. Each convolutional layer is followed by a rectified linear unit (ReLU) and batch normalization. The last layer of MobileNet contains an average pooling layer to reduce the spatial resolution to 1 followed by a fully connected layer that was fed to our classification layer for detection. Down sampling was handled with strided convolution in the depth-wise convolutions as well as in the first layer. Transfer learning technique was used instead of training the model from scratch. We used the pre-trained weights of MobileNet, which was trained on ImageNet to reuse its feature extraction capabilities. The classification layer was added at the end and was trained to classify the saliva samples into ovulating and non-ovulating based on the ferning patterns. Only the classification layer was trained while the other layers were intact. The output from the fully connected layer remained the same for every image and the output was used multiple times. Since calculating the outputs took a significant amount of time, we created bottleneck outputs to reduce the training time. The output from fully connected layer was used as an input in the classification layer. The true labels were fed to the network as ground truth and the accuracy, the cross-entropy, and the gradients were calculated (Fig. 3A).
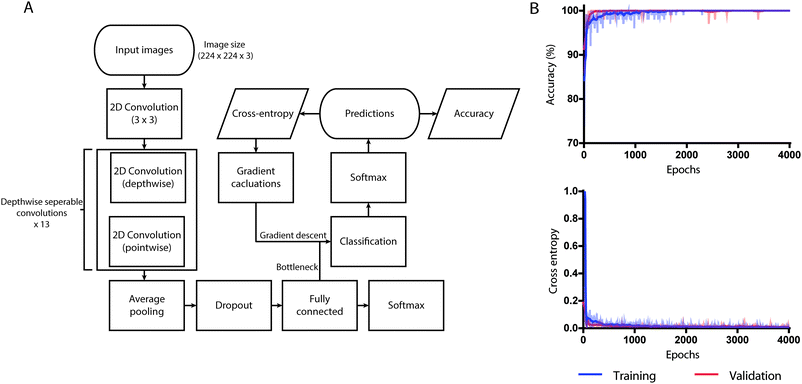 |
| Fig. 3 Graphical depiction of data flow with training and validation curves for accuracy and cross-entropy. (A) The computational flow of data. (B) The dataset of ferning and non-ferning images were trained and validated over 4000 training steps with a learning rate of 0.01. By the 4000th training step, the validation accuracy and cross entropy loss were saturated. | |
Training performance was measured by cross-entropy, which was used as a loss function to know how well the network is learning. Training accuracy is the percentage of correctly labeled images on training batch and validation accuracy is the percentage of correctly labeled images other than the images on training batch. The difference between the two accuracies was used in determining if the model is overfitting.
For transfer learning, we retrained MobileNet pre-trained on ImageNet for 4000 training steps (epochs) with learning rate set to 0.01 using our salivary datasets. The weights of the model that achieved the lowest cross-entropy loss was saved and used. At the 1000th training step, the validation cross-entropy loss was 0.1177 and the validation accuracy was 97.45% (Fig. 3B). As a part of the training process, the dimensions of all images used during training were resized to 224 × 224 pixels using computer vision libraries (OpenCV) and was trained within the TensorFlow environment. The trained neural network performed all processing and analysis locally, without the requirement for access to internet.
Data visualization techniques
Saliency maps were generated using TensorFlow. SmoothGrad applied to the output obtained from fully connected convolution layer was used to sharpen gradient-based sensitivity maps.33,34 The activation maps for the fully connected convolution layer was utilized for saliency visualizations. Both the artificial saliva and human saliva test set images were used in the generation of saliency maps. The t-distribution stochastic neighbor embedding (t-SNE) was performed to observe the distribution of the test dataset in a 2D space and to verify if our model was able to differentiate ovulating and non-ovulating samples accurately.
Artificial saliva
Artificial saliva was used to simulate ferning patterns. The composition of artificial saliva is given in the following: 0.92 g L−1 xanthan gum (Q8185, MP Biomedicals), 1.2 g L−1 potassium chloride (L1117, Chemcruz), 0.85 g L−1 sodium chloride (031M0215V, Sigma), 0.05 g L−1 magnesium chloride (030M0060V, Sigma), 0.13 g L−1 calcium chloride (057 K0005, Sigma), 0.13 g L−1 di-potassium hydrogen orthophosphate (SLBS6839, Sigma), and 0.35 g L−1 methyl p-hydroxybenzoate (Q7915, MP Biomedicals). The pH of the artificially prepared saliva was 7.0. The pH of normal saliva samples ranges from 6.2 to 7.6. We prepared a stock solution and diluted it with de-ionized water to mimic saliva samples obtained from ovulating and non-ovulating human populations. The following was the breakdown of concentrations of artificial saliva showing the three phases of ferning: non-ferning – 0.1% to 1.4%, transitional – 1.5% to 5.6% and ferning – 5.6% to 100% of the artificial saliva stock prepared.
Results
Smartphone-based ovulation assay hardware characterization
The smartphone-based system for ovulation testing was designed to be simple with minimal user interference (Fig. 1 and 2). The hardware components of the system consisted of an optical smartphone attachment for sample image magnification and a microfluidic device for saliva sample handling (Fig. 1 and 2). The 3D-printed optical smartphone attachment was both lightweight (208 g) and compact (83 × 70 × 74 mm). It used the smartphone's front camera for imaging and was attached to the phone through a simple slide-on mechanism. The image magnification achieved by the smartphone system was 4×, which was comparable to the digital images taken using a standard lab-based microscope (Carl Zeiss AG Axio Observer D1) at 10× magnification. The effective field-of-view of the system was 2.19 × 2.95 mm. Through imaging a micrometer scale using the optical system, we observed that 1 μm on the recorded image is represented by 0.226 pixels (Fig. S2A†), however, the resolution of the cellphone optical system was 7 μm, which was established by imaging a 1951 US Air Force (USAF) resolution target (Fig. S2B†). The system was motorized along a single axis and focal plane to automate the imaging of the microfluidic channel. The microfluidic device containing the saliva sample was optimally focused by placing the device at the working distance of the lens setup, which helped eliminating manual focusing by the user. The total material cost to fabricate the smartphone accessory and the microfluidic device was $13.91, which includes $13.58 for the optical attachment and $0.33 for the microfluidic device (Table S1†). The smartphone application was designed to guide the user through each step of testing (Fig. S3†). The developed system recorded videos at a rate of ∼5 fps, covering an area of 2.1 × 22.6 mm2 of the microfluidic device in <31 seconds per sample, during which the smartphone application imaged, locally analyzed, and reported the result.
The microfluidic device for sample handling
The microfluidic device was designed with a reservoir for saliva sample loading and a narrow microchannel for imaging the air-dried sample. The saliva sample is smeared on the imaging zone of the device using a simple slide-on 3D-printed smearer block (Fig. 2C).
The average saliva sample drying time on the microfluidic device was 6.7 ± 1.5 minutes as compared to a minimum of 25 minutes on a regular glass slide (n = 8, 3 replicates each) (Fig. S4†). Least squares regression analysis was performed and the slopes of lines for the microfluidic device and glass slide groups were 0.008 (95% confidence interval: −0.003 to 0.02) and 0.52 (95% confidence interval: 0.29 to 0.74) with R2 values of 0.30 and 0.97, respectively (Fig. S4†). By smearing the sample, a thin film is created over the glass substrate of the microfluidic device, which minimizes the drying time of the sample regardless of its volume.
System performance in artificial saliva
Artificial saliva samples were used to train the neural network. Using 492 annotated images, comprising of 168 images with ferning patterns and 324 images without ferning patterns, we retrained and validated our model to classify images into two categories of ovulating and non-ovulating samples. The ability of retrained MobileNet model to predict the correct result was evaluated using 100 images with ferning patterns and 100 images without ferning patterns of simulated artificial saliva. The network's performance in evaluating air-dried saliva samples was 90% with 95% confidence intervals (CI) that ranged from 84.98% to 93.78% (Fig. 4A and S5A†). The network performed with a sensitivity of 97.62% (CI: 91.66% to 99.71%) and a specificity of 84.48% (CI: 76.59% to 90.54%) (Fig. 4A and S5A†) when evaluating ferning patterns of artificial saliva samples. For the given test set, the positive predictive value (PPV) and negative predictive value (NPV) of the system were 82% (CI: 74.85% to 87.46%) and 98% (CI: 92.56% to 99.48%), respectively (Fig. 4A and S5A†). A t-SNE graph was generated for visualizing the degree of data separability in a 2D space (Fig. 4C). A good degree of separation was observed between the two patterns.
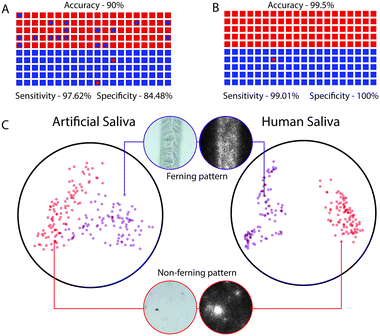 |
| Fig. 4 System performance with artificial and human saliva samples along with TSNE visualization. (A) The dot plot illustrates the system's performance in evaluating air-dried artificial saliva samples (n = 200). (B) The plot illustrates the system's performance in evaluating air-dried human saliva samples (n = 200). The squares represent true labels and the circles within them represent the system's classification. Blue squares and circles represent non-ovulating saliva samples while red squares and circles represent ovulating saliva samples. (C) The scatter plot helps visualize the separation of ovulating and non-ovulating samples based on the fern structures exhibited by the air-dried artificial and human saliva samples. The saliency map was extracted from the network to highlight the highest weighted features of the image. Black indicates the lowest weighted features while white indicates the highest weighted features of the image. | |
System performance with human saliva
We also evaluated the system's ability in differentiating ovulating and non-ovulating human saliva samples. Women subjects (n = 6) collected and tested their saliva samples using the cellphone system during both the ovulating and non-ovulating phases of their menstrual cycle and the results were confirmed using Clear Blue® (LH + estrogen) urine test. The subjects were asked to spit on the reservoir of the microfluidic device and smear it on the microchannel for air drying. Using an additional 748 annotated images of human saliva samples comprising of 108 ovulating images and 640 non-ovulating images collected from subjects (n = 6), we retrained and validated the developed neural network. The whole network was trained for 4000 training steps with a learning rate of 0.01, using which the lowest validation weights were achieved. At 1000th training step, validation cross entropy was 0.1177 and validation accuracy was 97.45%. At 4000th training step, we observed that the accuracy remained unchanged with no decrease in cross entropy loss. There was a marginal difference between the training and validation curves of accuracy indicating that the model was not overfitting (Fig. 3B). The ability of retrained MobileNets model in predicting the correct result was evaluated using a set of 100 images with fern patterns and 100 images without fern patterns. The accuracy of the neural network in predicting a saliva sample as ovulating and non-ovulating was 99.5% with CI ranging from 97.25% to 99.99% (Fig. 4B and S5B†). The sensitivity and specificity of the AI smartphone system in evaluating air-dried human saliva samples were 99.01% (CI: 94.61% to 99.97%) and 100% (CI: 96.34% to 100%), respectively (Fig. 4B and S5B†). We performed t-SNE to visualize the separation of the dataset by the network in a 2D space (Fig. 4C). With the observation of an excellent separation between the two classes, we further probed the network by mapping the final activation layers to visualize the saliency. Through the saliency maps,34 we could identify the pixels that are being used by the network and confirmed that it focused on features pertaining to the ferning pattern in its decision-making process (Fig. 4C). The PPV and NPV of the system for the given test set was 100% and 99.0% (CI: 93.37% to 99.86%), respectively.
System adaptability to different smartphones
To evaluate the generalizability of the developed AI algorithm across devices and imaging systems, we tested air-dried artificial saliva samples of varying concentrations of analytes representing ovulating and non-ovulating phases using 5 different smartphones. In this study we used Samsung Galaxy 5, Xiaomi Redmi Note 4, OnePlus 5 T, LG G6, and Moto X. The base algorithm trained with both images of air-dried artificial and human saliva samples imaged by the Moto X device was used and no additional training was performed to adapt the network to the tested devices. The network performed with excellent consistency across all tested devices achieving an excellent score of 1.0 (lower 95% CI: 1.0) in a Cronbach alpha's test for consistency (n = 15 with 5 phones) (Table S2†). These results suggest that the developed network generalizes well across different smartphones tested in this study.
Discussion
Accurate detection of ovulation is useful in family planning. The current home-based urinary test identifies sudden surge in LH that helps in finding out the ovulation phase. Though urinary LH is easy-to-test, people from less developed countries do not have access to these relatively expensive test kits. Additionally, the ferning test may be more reliable in patients that have false positive responses to the urine LH kit, such as those women with polycystic ovarian syndrome and elevated LH levels. Currently, salivary ferning tests are carried out using a drop of sublingual saliva sample placed on the glass slide, which is then dried and manually tested for ferning structures.35 Manual testing is highly subjective and can lead to misinterpretation of the results by lay users.
We developed an automated smartphone-based optical system that can accurately detect ovulation in women using a small volume (<100 μL) of air-dried saliva samples loaded onto a microfluidic device through detecting saliva ferning using an AI-based analysis on-phone. Our microfluidic device enables rapid (<7 min) saliva sample drying by creating thin films and provides a controlled sample volume for the analysis. Traditional spot placement methods have no control on sample volume and thus often tend to induce false signal.
Although saliva sample volume control is achieved in the microfluidic device, we observed that the fern patterns are not equally prevalent throughout the microchannel but are found as packets. This is likely due to the re-distribution of solute across the microfluidic device during the formation of the thin films combined with the uneven evaporation. In such a case, for the accurate prediction of fern patterns, we imaged the entire microchannel to ensure all the fern patterns on the microfluidic device were captured and analyzed. The system's accuracy in classifying saliva samples and detecting fern structures in undiluted, unprocessed saliva samples was 99.5% (Fig. 4). The optical focus and uniform light intensity were fixed in the optical system to avoid errors from the manual adjustment.
The developed imaging system can be used for other applications that involve imaging of microstructures in an illuminated environment, such as detecting fern structures in amniotic fluid for diagnosing premature rupture of membranes (PROM) in pregnant women36 and tear film for detecting dry eye disease.37 Rupture of membranes (ROM) is the breaking of amniotic sac that occurs before the onset of labor.36 On the other hand, dry eye disease is diagnosed by testing the ocular tear film for fern patterns. Healthy tear samples produce dense fern patterns, which is absent in dry eye samples.37
A rapid point-of-care ovulation testing also has an important application in animal breeding. Animal breeding is mainly dependent on the breeding cycle of the species and it is time-constrained. One of the important criteria for animal breeding is to determine the optimum time to breed an animal in-order-to achieve higher conception rate. For an egg fertilized later in its maturity, the chances for abortion are greater. Therefore, there is a need for advanced prediction of ovulation. The developed smartphone-based automated optical system can be potentially used for analyzing the saliva ferning patterns in animals such as buffalos,38 dogs,39 and other mammals to predict and confirm its ovulation and detect the optimum insemination time. To determine insemination time, many animal breeders rely on manual techniques such as heat indications, but it is not always accurate; ovulation can take place before, during, or after visible heat.40 Other devices such as electronic pedometers or activity tags are used to detect ovulation by monitoring changes in behavior such as restlessness, standing heat, mounting behavior, and elevated physical activity of animals.41 Some animals are sensitive to these types of measurements and it becomes difficult for the animal breeders to test them through these methods. To eliminate such complications, a regular, point-of-care, easily available and convenient system for ovulation detection is necessary for effective management of animal breeding.
One of the major advantages of the reported approach over all other available methods is its cost effectiveness in the long term.10 The total material cost for our approach is ∼$14 USD and is completely reusable. Urine-based tests can range from $1–2 per test, however, they are not reusable and thus per cycle the overall test can cost approximately $35–40 for a user in the US. According to prior reports most women get pregnant within 6 cycles, therefore, we estimate that these non-reusable tests can cumulatively cost upwards of $200 for most women.42 It should be noted that a direct comparison of costs associated with urine tests and the AI-based saliva testing is limited, because our reported material costs do not include costs associated with product commercialization and distribution channels. Furthermore, since it performs all the required analysis on-phone without the need for internet connectivity it is especially attractive for use in resource-limited settings.
One of the limitations of the developed smartphone-based optical system is to detect ovulation in women with estrogen imbalance, cysts in the ovaries, and those who take fertility medications. Also, after smoking or alcohol consumption, an adequate amount of time gap should be provided before testing. If these conditions are not looked upon properly, they may cause fern structures in the absence of ovulation. Our system can detect the entire fertile window including the exact day of ovulation when the chances of conception are higher. Further, the inclusion of basal body temperature may help in overcoming the system's current limitations and thus warrants further research. The work demonstrated here is an example of how AI integrated with smartphones along with inexpensive hardware can be developed into an effective point-of-care diagnostic assay that can address a need in home-based fertility management and family planning.
Author contributions
H. S. and M. K. K. designed the study. P. V. and P. S. K. conducted the experiments and optimized test readout. M. K. K. advised and assisted through the experimental process. J. C. P., A. S., and K. B. assisted in collecting the samples from the subjects and in performing experiments. D. Y., P. T., H. K. and S. K. S. P. designed the device and performed device fabrication and assisted in device optimization studies. P. V. and P. S. K. designed the microfluidic device for sample handling. H. K. and R. G. developed the smartphone code for fern structure detection and developed the graphical user interface of the smartphone application. N. G. helped in developing the smartphone code. P. V. and P. S. K. analyzed the data and M. K. K advised on data analysis. J. C. P. provided clinical support and logistics. P. V., P. S. K., M. K. K., and H. S. wrote the manuscript. All authors edited the manuscript.
Conflicts of interest
The authors have no conflicts to declare.
Acknowledgements
We would like to thank Sneha Sundar and Fenil Doshi for productive discussions. This work is partially supported by the Brigham Research Institute Pilot Grant, the Innovation Evergreen Fund (Brigham Research Institute, Brigham and Women's Hospital, Harvard Medical School), 1R01AI118502 and P30ES000002, R21HD092828 (National Institute of Health) a Harvard National Institute of Environmental Health Sciences Grant (Harvard T. H. Chan School of Public Health, Harvard Center for Environmental Health), and an American Society of Reproductive Medicine Award (American Board of Obstetrics and Gynecology, American College of Obstetricians and Gynecologists, American Society for Reproductive Medicine, and Society for Reproductive Endocrinology and Infertility).
References
- A. J. Wilcox, C. R. Weinberg and D. D. Baird, N. Engl. J. Med., 1995, 1517–1521 CrossRef CAS PubMed.
- J. Bearak, A. Popinchalk, L. Alkema and G. Sedgh, Lancet Glob. Health, 2018, 6, e380–e389 CrossRef PubMed.
- K. Keenan, Lancet Glob. Health, 2018, 6, e352–e353 CrossRef PubMed.
-
A. Sonfield, K. Hasstedt and R. B. Gold, Moving Forward: Family Planning in the Era of Health Reform, Guttmacher Institute, New York, 2014 Search PubMed.
-
A. Sonfield and K. Kost, Public Costs from Unintended Pregnancies and the Role of Public Insurance Programs in Paying for Pregnancy-Related Care: National and State Estimates for 2010, Guttmacher Institute, New York, 2015 Search PubMed.
-
J. J. Frost, L. F. Frohwirth and M. R. Zolna, Contraceptive Needs and Services, 2014 Update, Guttmacher Institute, New York, 2016 Search PubMed.
- J. B. Stanford, J. C. Lemaire and P. B. Thurman, J. Fam. Pract., 1998, 46, 65–71 CAS.
- S. R. Pallone and G. R. Bergus, J. Am. Board. Fam. Med., 2009, 22, 147–157 CrossRef PubMed.
- M. Guida, G. A. Tommaselli, M. Pellicano, S. Palomba and C. Nappi, Gynecol. Endocrinol., 1997, 11, 203–219 CrossRef CAS PubMed.
- H. W. Su, Y. C. Yi, T. Y. Wei, T. C. Chang and C. M. Cheng, Bioeng. Transl. Med., 2017, 2, 238–246 CAS.
- R. M. Ersyari, R. Wihardja and M. Dardjan, Padjadjaran Journal of Dentistry, 2014, 26, 194–202 CrossRef.
- A. Salmassi, A. G. Schmutzler, F. Püngel, M. Schubert, I. Alkatout and L. Mettler, Gynecol. Obstet. Invest., 2013, 76, 171–176 CrossRef CAS PubMed.
- M. Guida, G. A. Tommaselli, S. Palomba, M. Pellicano, G. Moccia, C. Di Carlo and C. Nappi, Fertil. Steril., 1999, 72, 900–904 CrossRef CAS PubMed.
- Artificial intelligence in health care: within touching distance, Lancet, 2017, vol. 390, p. 2739 Search PubMed.
- R. Poplin, A. V. Varadarajan, K. Blumer, Y. Liu, M. V. McConnell, G. S. Corrado, L. Peng and D. R. Webster, Nat. Biomed. Eng., 2018, 2, 158–164 CrossRef.
- A. Esteva, B. Kuprel, R. A. Novoa, J. Ko, S. M. Swetter, H. M. Blau and S. Thrun, Nature, 2017, 542, 115 CrossRef CAS PubMed.
- V. Gulshan, L. Peng, M. Coram, M. C. Stumpe, D. Wu, A. Narayanaswamy, S. Venugopalan, K. Widner, T. Madams, J. Cuadros, R. Kim, R. Raman, P. C. Nelson, J. L. Mega and D. R. Webster, JAMA, J. Am. Med. Assoc., 2016, 316, 2402–2410 CrossRef PubMed.
-
P. Jonsson, S. Carson, J. S. Sethi, M. Arvedson, R. Svenningsson, P. Lindberg, K. Öhman and P. Hedlund, Ericsson Mobility Report, Ericsson, Stockholm, Sweden, 2017 Search PubMed.
- M. K. Kanakasabapathy, H. J. Pandya, M. S. Draz, M. K. Chug, M. Sadasivam, S. Kumar, B. Etemad, V. Yogesh, M. Safavieh, W. Asghar, J. Z. Li, A. M. Tsibris, D. R. Kuritzkes and H. Shafiee, Lab Chip, 2017, 17, 2910–2919 RSC.
- T. Laksanasopin, T. W. Guo, S. Nayak, A. A. Sridhara, S. Xie, O. O. Olowookere, P. Cadinu, F. Meng, N. H. Chee, J. Kim, C. D. Chin, E. Munyazesa, P. Mugwaneza, A. J. Rai, V. Mugisha, A. R. Castro, D. Steinmiller, V. Linder, J. E. Justman, S. Nsanzimana and S. K. Sia, Sci. Transl. Med., 2015, 7, 273re271 Search PubMed.
- M. S. Draz, K. M. Kochehbyoki, A. Vasan, D. Battalapalli, A. Sreeram, M. K. Kanakasabapathy, S. Kallakuri, A. Tsibris, D. R. Kuritzkes and H. Shafiee, Nat. Commun., 2018, 9, 4282 CrossRef PubMed.
- B. Berg, B. Cortazar, D. Tseng, H. Ozkan, S. Feng, Q. Wei, R. Y.-L. Chan, J. Burbano, Q. Farooqui, M. Lewinski, D. Di Carlo, O. B. Garner and A. Ozcan, ACS Nano, 2015, 9, 7857–7866 CrossRef CAS PubMed.
- S. M. Knowlton, I. Sencan, Y. Aytar, J. Khoory, M. M. Heeney, I. C. Ghiran and S. Tasoglu, Sci. Rep., 2015, 5, 15022 CrossRef CAS PubMed.
- P. Thirumalaraju, C. L. Bormann, M. Kanakasabapathy, F. Doshi, I. Souter, I. Dimitriadis and H. Shafiee, Fertil. Steril., 2018, 110, e432 CrossRef.
- I. Dimitriadis, R. Xu, M. K. Kanaksabapathy, P. Thirumalaraju, V. Yogesh, C. Tanrikut, J. Hsu, C. L. Bormann and H. Shafiee, Fertil. Steril., 2018, 109, e24 Search PubMed.
- M. Kanakasabapathy, P. Thirumalaraju, V. Yogesh, V. Natarajan, C. L. Bormann, J. C. Petrozza and H. Shafiee, Fertil. Steril., 2017, 108, e74–e75 Search PubMed.
- M. K. Kanakasabapathy, M. Sadasivam, A. Singh, C. Preston, P. Thirumalaraju, M. Venkataraman, C. L. Bormann, M. S. Draz, J. C. Petrozza and H. Shafiee, Sci. Transl. Med., 2017, 9, eaai7863 CrossRef PubMed.
- M. Kanakasabapathy, P. Thirumalaraju, V. Yogesh, V. Natarajan, C. L. Bormann, P. Bhowmick, C. Veiga, J. C. Petrozza and H. Shafiee, Fertil. Steril., 2017, 108, e74 Search PubMed.
- C. L. Bormann, M. Kanakasabapathy, P. Thirumalaraju, V. Yogesh, V. Natarajan, J. Demick, A. Blanchard, J. C. Petrozza and H. Shafiee, Fertil. Steril., 2017, 108, e74 CrossRef.
- M. S. Draz, N. K. Lakshminaraasimulu, S. Krishnakumar, D. Battalapalli, A. Vasan, M. K. Kanakasabapathy, A. Sreeram, S. Kallakuri, P. Thirumalaraju, Y. Li, S. Hua, X. G. Yu, D. R. Kuritzkes and H. Shafiee, ACS Nano, 2018, 12, 5709–5718 CrossRef CAS PubMed.
- A. Priye, S. W. Bird, Y. K. Light, C. S. Ball, O. A. Negrete and R. J. Meagher, Sci. Rep., 2017, 7, 44778 CrossRef CAS PubMed.
-
A. G. Howard, M. Zhu, B. Chen, D. Kalenichenko, W. Wang, T. Weyand, M. Andreetto and H. Adam, ArXiv e-prints, 2017 Search PubMed.
-
D. Smilkov, N. Thorat, B. Kim, F. Viégas and M. Wattenberg, ArXiv e-prints, 2017 Search PubMed.
-
K. Simonyan, A. Vedaldi and A. Zisserman, ArXiv e-prints, 2013 Search PubMed.
- V. Gunther, I. Bauer, J. Hedderich, L. Mettler, M. Schubert, M. T. Mackelenbergh, N. Maass and I. Alkatout, Eur. J. Obstet. Gynecol. Reprod. Biol., 2015, 194, 38–42 CrossRef CAS PubMed.
- A. B. Caughey, J. N. Robinson and E. R. Norwitz, Rev. Obstet. Gynecol., 2008, 1, 11–22 Search PubMed.
- A. M. Masmali, C. Purslow and P. J. Murphy, Clin. Exp. Optom., 2014, 97, 399–406 CrossRef PubMed.
- R. Ravinder, O. Kaipa, V. S. Baddela, E. Singhal Sinha, P. Singh, V. Nayan, C. S. Velagala, R. K. Baithalu, S. K. Onteru and D. Singh, Theriogenology, 2016, 86, 1147–1155 CrossRef CAS PubMed.
- B. Pardo-Carmona, M. R. Moyano, R. Fernandez-Palacios and C. C. Perez-Marin, J. Small Anim. Pract., 2010, 51, 437–442 CrossRef CAS PubMed.
- S. Dash, A. K. Chakravarty, A. Singh, A. Upadhyay, M. Singh and S. Yousuf, Vet. World, 2016, 9, 235–244 CrossRef CAS PubMed.
- P. Løvendahl and M. G. G. Chagunda, J. Dairy Sci., 2010, 93, 249–259 CrossRef PubMed.
- C. Gnoth, D. Godehardt, E. Godehardt, P. Frank-Herrmann and G. Freundl, Hum. Reprod., 2003, 18, 1959–1966 CrossRef CAS PubMed.
Footnotes |
† Electronic supplementary information (ESI) available. See DOI: 10.1039/c8lc00792f |
‡ These authors contributed equally to this work. |
|
This journal is © The Royal Society of Chemistry 2019 |
Click here to see how this site uses Cookies. View our privacy policy here.