DOI:
10.1039/C8RA10163A
(Paper)
RSC Adv., 2019,
9, 6328-6334
In silico post-SELEX screening and experimental characterizations for acquisition of high affinity DNA aptamers against carcinoembryonic antigen†
Received
11th December 2018
, Accepted 11th February 2019
First published on 21st February 2019
Abstract
DNA aptamers against carcinoembryonic antigen (CEA) have been identified through the systematic evolution of ligands by exponential enrichment (SELEX) technique, but their affinity needs to be improved. In this study, an in silico approach was firstly used to screen the mutation sequences of a reported DNA aptamer (the parent aptamer, denoted as P) against CEA. The affinities of several high-score DNA mutants were determined by the biolayer interferometry technique. Finally, the newly obtained aptamers were verified in an aptasensor application. For the in silico approach, Mfold and RNA Composer were combined to generate the 3D RNA structures of the DNA mutants. The RNA structures were then modified to 3D DNA structures with the Write program. The docking model and binding ability of the 3D DNA structures with CEA were simulated and predicted with the ZDOCK program. Two mutation sequences (P-ATG and GAC-P) exhibited significantly higher ZDOCK scores than P. The dissociation constant of P-ATG and GAC-P to CEA was determined to be 4.62 and 3.93 nM respectively, obviously superior to that of P (6.95 nM). The detection limit of the P-ATG and GAC-P based aptasensors was 1.5 and 1.2 ng mL−1, respectively, markedly better than that based on P (3.4 ng mL−1). The consistency between the in silico and the experimental results indicates that the developed in silico post-SELEX screening approach is feasible for improving DNA aptamers. The P-ATG and GAC-P aptamers found in this study could be used for future CEA aptasensor design and fabrication, promisingly applicable for highly sensitive CEA detection and early cancer diagnosis.
Introduction
Carcinoembryonic antigen (CEA) is a tumor marker over-expressed on a wide range of tumors, such as pancreatic, lung, colon, breast, ovarian, and cervical tumors.1–5 It is a highly glycosylated glycoprotein of 180 kDa with 50–60% sugar content.6 Sensitive and accurate detection of CEA in serum and tissue is of great importance for early cancer diagnosis. Immunoassays are well-developed CEA detection methods.7,8 With the specific and affinitive recognition between antigen and antibody, immunoassays have the advantages of sensitivity and specificity. However, the long-term stability and the synthesis reproducibility of antibodies are not very satisfactory. Aptamers are single-strand oligonucleotide ligands selected in vitro by the systematic evolution of the ligand by the exponential enrichment (SELEX) process from random-sequence nucleic acid libraries.9,10 They can recognize various targets, ranging from ions, small molecules, macromolecules to whole cells.11 Compared with antibodies, aptamers have many advantages, such as low cost, repeatable synthesis, easy modification, and long-term stability.12,13 They can possess similar or even stronger affinity and specificity compared to antibodies.14,15 Since their discovery, aptamers have been increasingly used in bioanalysis and biotechnology.16,17 However, although SELEX is an efficient screening method, it sometimes fails to obtain aptamers with high affinity.18,19 For the SELEX technique, firstly, the diversity of oligonucleotide sequences in the initial library is limited because of the difficulty of maintaining the diversity of the sequence pool in laboratory manipulation during in vitro selection.18 Secondly, the polymer chain reaction (PCR) process in SELEX may amplify multiple oligonucleotides unequally, causing bias in the sequence diversity of an oligonucleotide population as the selection cycles continue.19
A CEA aptamer with the sequence of 5′-ATACCAGCTTATTCAATT-3′ has been selected and identified by Smith.20 CEA aptasensors based on this aptamer with various designs have been reported.21–23 We recently reported a label-free, antibody-free, and lectin-based electrochemical sandwich CEA aptasensor.21 A single enzyme-based amplification strategy was used in this aptasensor design and fabrication. The limit of detection (LOD) value of this CEA aptasensor is 3.4 ng mL−1. Although the LOD value could satisfy cancer diagnosis of malignant tumors, whose threshold level in human serum is 10 ng mL−1,24 early cancer diagnosis needs higher CEA detection sensitivity. Using high affinity CEA aptamers in aptasensor fabrication could be a simple and effective strategy to enhance the CEA detection sensitivity.
In silico post-SELEX screening has been used as a rational approach to overcome or make up the shortcomings of SELEX technique.25,26 A thrombin binding aptamer possessing higher affinity than its parent aptamer has been identified and characterized.26 For in silico screening, firstly, various mutation strategies, such as genetic algorithm,27 truncation,28 maturation and multimerization,19 have been applied on SELEX-selected parent aptamers to produce DNA libraries with high sequence diversities. Secondly, DNA structures and aptamer–target interaction models were simulated.29 Several bioinformatic tools have been invented and used to predict the secondary/3 dimensional (3D) structures, as well as the conserved motifs of nucleic acid sequences.30,31 Compared to DNA, RNA molecule can form diverse 3D structures owing to their non-Watson–Crick base pairing and 2-OH groups.11 Therefore, most of these tools, such as RNA Composer, are optimized and applied to predict the structures of RNA aptamers.32 For simulating aptamer–target interaction models, computational docking has emerged as a common tool to predict and identify protein bound small-molecule ligands, such as oligopeptides,33,34 small organic compounds,35,36 and RNA aptamers.37 For the computational docking, the 3D structures of the target proteins also need to be understood, which can be obtained from the Protein Data Bank. Among various docking tools, ZDOCK program has demonstrated its feasibility to dock DNA molecules to target proteins.28,38,39 The ZDOCK program possesses a scoring function in evaluating the ligand–target interactions, by taking shape complementary, electrostatics, and pairwise atomic potential into consideration.40
In this study, a DNA library was firstly produced via base substitution and base addition on the parent CEA aptamer (5′-ATACCAGCTTATTCAATT-3′), denoted as P. Mfold web server was then applied to predict the secondary structures of the DNA mutants. Based on the predicted secondary structures, their corresponding 3D RNA structures were predicted via RNA Composer web server. The 3D RNA structures were then modified to 3D DNA structures in Write program, and optimized by steepest descent energy minimization method. Afterwards, the docking model and binding ability of the 3D DNA structures with CEA were simulated and predicted by using ZDOCK program. High ZDOCK score DNA mutants were then tested by using biolayer interferometry (BLI) technique, to determine their affinity to CEA. Finally, the obtained high affinity DNA aptamers were characterized and verified in our electrochemical aptasensor application.
Materials and methods
Reagents and apparatus
Gold electrodes and platinum electrodes are disk electrodes with a diameter of 2 mm (CHI, Shanghai Chenhua Instrument, China). Nucleic acid sequences (listed in Table 1) were synthesized and purified by Shanghai Sangon Biotechnology Co. Ltd. (China). The DNA mutants generated by the base addition strategy were named by putting the added nucleotide bases at the left side or the right side of the letter P (denoted for the parent aptamer), depending on the 5′ end or the 3′ end, respectively, that was modified. Biotinylated (denoted as Biotin) triethylene glycol (TEG, C6H12O4) moiety modified DNA sequences at their one end were used in the BLI assay. Alkylthiol moiety [HS-(CH2)6-] modified DNA sequences at their one end were used in aptasensor application experiment. Horse radish peroxidase (HRP, ≥300 U mg−1) and hydroquinone (H2Q, purity: ≥99%) were purchased from Aladdin Industrial (Shanghai, China). Concanavalin A (ConA, Type IV), human serum albumin (HSA, purity: ≥96%) and γ-globulin (purity: ≥99%) were obtained from Sigma-Aldrich (USA). Carcinoembryonic antigen (CEA, 150–210 kDa), human alpha fetoprotein antigen (AFP, purity: 90%), and c-reactive protein antigen (CRP, purity: ≥90%) were purchased from Shanghai Linc-Bio Science Co. Ltd. (Shanghai, China). All other chemicals were of analytical grade and purchased from Sinopharm Chemical Reagent (Shanghai, China). All nucleic acid sequences were received as lyophilized form and then dissolved in TE buffer (10 mM Tris–HCl containing 1 mM EDTA, pH = 8.0) and stored at −20 °C. Deionized water (Millipore, ≥18 MΩ cm) obtained from a Millipore water system was used throughout the experiment.
Table 1 Nucleic acid sequences used in this studya
Name |
Sequence |
Underlined part refers to mutations in the sequence. |
Biotin-P |
5′-Biotin-TEG-ATA CCA GCT TAT TCA ATT-3′ |
Biotin-P-ATG |
 |
Biotin-GAC-P |
 |
Biotin-P-GTG |
 |
HS-P-ATG |
 |
HS-GAC-P |
 |
BLI assay was performed on Octet Red 96 system equipped with data acquisition and analysis software (FortéBio, USA). Super streptavidin-coated (SSA) sensors and related consumables were purchased from FortéBio (China). Both association and dissociation buffers were 20 mM Tris–HCl (pH: 7.4) containing 150 mM NaCl, 5 mM KCl and 0.05% Tween-20. Loading buffer consists of 20 mM Tris–HCl (pH: 7.4), 150 mM NaCl, and 5 mM KCl. The association buffer was also used for CEA dilution in BLI assay.
In the aptasensor application experiment, differential pulse voltammetry (DPV) was carried out on an electrochemical work station (CHI-660E, Shanghai Chenhua Instrument, China) consisting of a three-electrode system: a gold working electrode, a platinum counter electrode, and a 3 M KCl–Ag/AgCl reference electrode, at room temperature (∼25 °C). All potentials were quoted versus this reference electrode. Immobilization buffer was 10 mM phosphate buffer solution (PBS, pH: 7.0) containing 1.0 mM ethylene diamine tetraacetic acid (EDTA) and 0.6 M NaCl. Protein-dissolving buffer was 10 mM Tris–HCl buffer (pH: 7.4) containing 150 mM NaCl. Washing buffer was 10 mM Tris–HCl buffer (pH: 7.4) added with 150 mM NaCl and 0.05% Tween-20. ConA activating buffer was 50 mM Tris–HCl buffer (pH: 7.0) added with 10 mM CaCl2 and 10 mM MnCl2.
Establishment of DNA mutant library and prediction of DNA structures
A DNA mutant library was established through nucleotide base substitution or addition on the P sequence (5′-ATACCAGCTTATTCAATT-3′), which was selected and identified by Smith.20 For nucleotide base substitution, we wrote an in silico mutation (denoted as ISM) program using C++ in Microsoft Visual Studio (version 2010), through which random single base mutation was executed. In the first cycle of single base substitution, the DNA mutant number was set as 30, and 26 DNA mutants were generated after repeated DNA mutant sequences were removed. After their 3D DNA structures were docked with CEA through the ZDOCK program, the second cycle of single base mutation was executed on the top 5 docking score DNA mutants in the ISM program. For the second cycle of single base substitution, the DNA mutant number was set as 4 or 5 on each of the top docking score DNA mutants, generating 24 new DNA mutants. The steps of random single base mutation, 3D DNA structure prediction, and DNA–CEA docking were repeated 5 cycles. The method for single base mutation was kept the same from the second cycle. Finally, through the nucleotide base substitution strategy, totally 122 DNA mutants were generated.
For nucleotide base addition, all possible combinations of 3 nucleotide bases were added on the 5′ or 3′ terminal of P, generating 128 DNA mutants. The DNA mutant sequences with higher CEA docking scores than P are listed in Table S1.† The DNA mutants generated with the nucleotide base substitution strategy were named by pointing out the position of each modified nucleotide base with Arabic number, and putting the nucleotide base that was substituted and the new nucleotide base at the left side and the right side of the Arabic number, respectively.
Mfold web server (http://unafold.rna.albany.edu) was utilized to predict the secondary structures of the DNA sequences.41 The folding temperature and the ionic condition were set at 30 °C and 150 mM NaCl, respectively. The secondary structures with the minimum free energy (i.e. the lowest ΔG value) were selected, and the Vienna output formats (i.e. dot-bracket notation) (listed in Table S1†) were used for prediction of 3D RNA structures through the RNA Composer server (http://rnacomposer.ibch.poznan.pl/Home).42 The RNA Composer server works according to the machine translation principle, and operates on the RNA FRABASE database, a search engine associated with the database of RNA 3D structures. The outcome (downloaded as PDB files) is 3D structures of RNA form in which an additional hydroxyl group is present in 2′-carbon atom of ribose and thymine is replaced by uracil. The PDB files obtained from the RNA Composer server were opened by Write program, and then modified to 3D structures of DNA form by replacing all O2's (oxygen attached to the 2′ carbon atom of ribose) with hydrogen, and creating a methyl substitution on 5-carbon atom of all uracil bases. Finally, the 3D DNA structures were optimized by steepest descent energy minimization method using Molecular Operating Environment (MOE, 2016) molecular modeling package.
Simulation of DNA–CEA interaction model and prediction of binding ability
Firstly, CEA crystal structure (code: 2QSQ) with a resolution of 1.95 Å, which was produced by Korotkova et al.,43 was obtained from the Protein Data Bank (https://www.rcsb.org) (Fig. S1, ESI†). Then ZDOCK online server (version 3.0.2, http://zdock.umassmed.edu)44 was used to simulate the docking between CEA and DNA structures, which were set as receptor and ligand respectively. As an automated tool, ZDOCK program searches all possible binding modes in the translational and rotational space between CEA and DNA, and evaluates each pose using an energy-based shape complementary scoring function. Each docking pose was ranked according to the ZDOCK scores. The pose with the highest ZDOCK score suggests that it is the best model for the aptamer and target interaction. Higher ZDOCK score means higher binding ability. For comparisons, the docking models and the binding abilities of the selected DNA aptamers to some interference proteins were also simulated and predicted.
Determination of the affinities of DNA aptamers to CEA
BLI is a label-free and real-time optical analysis technique that utilizes fiber-optic biosensors for measuring interactions between biomolecules.45,46 The working principle of BLI assay is described in ESI.† The assay procedure was based on that reported by Lou et al.47 It includes five steps: baseline 1; loading; baseline 2; association; and dissociation. All steps were performed at 30 °C under shaking at 1000 rpm in a 96-well plate containing 200 μL sample or buffer in each well. A preliminary experiment was firstly carried out to verify and decide the BLI assay conditions (Fig. S2, ESI†). Based on the preliminary experimental results, the conditions of the BLI affinity assay were set as followed: SSA sensor tips were pre-wetted in 200 μL loading buffer for 10 min followed by ‘baseline 1’ step with loading buffer for 100 s; ‘loading’ with 200 nM Biotin-TEG moiety modified DNA aptamer (shown in Table 1) in loading buffer for 200 s; ‘baseline 2’ step for 100 s with association buffer; ‘association’ of various concentrations (4.55–181.82 nM) of CEA in association buffer for 400 s; ‘dissociation’ with dissociation buffer for 1500 s. The designed plate map is schematically shown in Fig. S3.† The detailed experimental procedure is described in the ESI.† Except for the P sequence, three DNA mutants were tested, including P-ATG, GAC-P, and P-GTG, which have higher ZDOCK scores than P. A SSA sensor tip with a blank loading and association with buffers was tested as control. A 1
:
1 binding mode with mass transfer fitting was used to analyze the kinetic data.
Verification of the selected DNA aptamers in aptasensor application
To further verify the DNA aptamers selected by the in silico screening approach, the analytical performances of our preciously developed electrochemical CEA aptasensors21 fabricated from the selected aptamers were evaluated. The detailed biosensor fabrication procedure is described in the ESI.† Briefly, the DNA aptamers with an alkylthiol moiety at their one end (HS-P-ATG and HS-GAC-P, Table 1) were immobilized onto the cleaned gold electrode surface through formation of Au–S bond, by applying a steady potential of +0.4 V at the electrode for 500 s in the aptamer solution. After the electrode was cleaned with the immobilization buffer, it was passivated with 6-mercapto-1-hexanol (MCH). Then standard CEA samples in protein-dissolving buffer were added onto the passivated electrode surface, followed by keeping the electrode at 37 °C for 1 h under gentle shaking. After being rinsed thoroughly with a washing buffer, the electrode surface was covered and incubated with 100 μL 0.5 mg mL−1 ConA solution in the ConA activating buffer at 30 °C for 3 h. Finally, the electrode surface was dropped and incubated with 100 μL 5 μg mL−1 HRP solution at 30 °C for 2 h. The resulted sandwich aptasensors were washed with the washing buffer and then immersed into a detection buffer, in the presence of 2.0 mM H2Q and 2.5 mM H2O2 at room temperature. After 4 min of catalytic reaction, DPV measurement was performed. To investigate the detection specificity, 100 μL of interfering proteins, including BSA, HSA, γ-globulin, AFP, and CRP were detected instead of CEA with the aptasensors.
Results and discussion
Simulation of DNA–CEA interaction model and binding ability
Through the nucleotide base substitution and addition strategies, totally 250 DNA mutant sequences were generated from the P sequence. Their secondary structures were obtained with the Mfold web server, and then converted to 3D structures with the RNA Composer and Write program. To simulate their interaction model and binding ability with CEA, ZDOCK program was applied. All possible poses of DNA–CEA structural interactions were investigated. Each pose was evaluated using an energy-based shape complementary scoring function without changing the 3D structures of DNA and the crystal structure of CEA. The top five ZDOCK scores for each DNA sequence were analyzed. Fourteen DNA mutants exhibited higher mean ZDOCK scores than the P sequence (Fig. 1). Among them, the DNA mutants P-ATG and GAC-P showed significantly higher ZDOCK scores than P. In contrast, a random sequence, which was designed as a negative control, exhibited significantly lower ZDOCK score than P. Their sequences, dot-bracket format of secondary structures, and mean value of top five ZDOCK scores are listed in Table S1 (ESI†). The DNA–CEA interaction models (i.e. the pose with the highest ZDOCK score) of the top 3 DNA mutants (i.e. P-ATG, GAC-P, and P-GTG) and P are shown in Fig. 2. The interaction models of other 11 DNA mutants are shown in Fig. S4.† The structural domains and the amino acid residues of CEA in the binding interface were analyzed (Table S2, ESI†). It is interesting that we found that except for P-ATG, all the other DNA mutants as well as the P sequence bind with some turns and their nearby β-sheets on CEA. On the contrary, P-ATG binds with some α-helices and their nearby β-sheets on CEA. The knowledge about the CEA domains bound with these DNA aptamers could be used for rational design and fabrication of sandwich CEA aptasensors, for which recognition elements that binds with different CEA domains could be used.
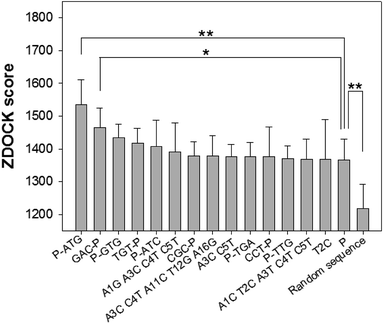 |
| Fig. 1 Histograms of the top 5 ZDOCK binding scores of various DNA sequences with CEA, obtained by using online ZDOCK automatic docking server. ** and * represents that the p value is less than 0.01 and 0.05, respectively, in the t-test. n = 5. | |
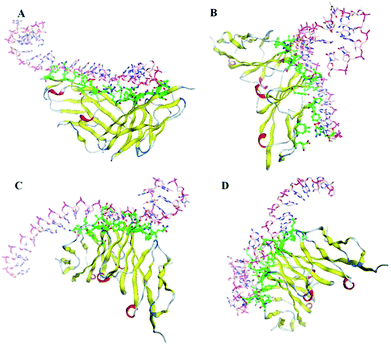 |
| Fig. 2 Structural images of DNA–CEA docking models with the highest ZDOCK docking score for each DNA mutant sequence. The green tags indicate the binding interface on the receptor (i.e. CEA) structure. (A) CEA/P-ATG, (B) CEA/GAC-P, (C) CEA/P-GTG, and (D) CEA/P. | |
The ZDOCK scores of the top 2 DNA mutants (i.e. P-ATG and GAC-P) to the interference proteins (HSA, γ-globulin, AFP, and CRP) are significantly smaller than those to the CEA target (Fig. S5†), suggesting high binding specificity of the DNA aptamers to CEA. The DNA–protein interaction models between the top 2 DNA mutants and each of interference proteins are shown in Fig. S6.†
Affinity determination
To verify the DNA aptamers selected by the in silico approach, the affinities of the 3 top ZDOCK score DNA mutants (i.e. P-ATG, GAC-P, and P-GTG) and P sequence to CEA were determined by using BLI technique. Fig. 3 shows the sensorgrams of the DNA mutants (Fig. 3A–C, for PATG, GAC-P, and P-GTG respectively) and P (Fig. 3D) in response to a series of different concentrations of CEA. The steady-state data, i.e. the response signals at equilibrium (denoted as Req) were analyzed. The plot of Req versus CEA concentration (denoted as CCEA) is shown in inset I in Fig. 3. The signal increased monotonically with the increase of CEA concentration from 4.55 nM to 45.45 nM, and then reached to a plateau, following the Michaelis–Menten kinetics. A linear 1/Req (nm−1) versus 1/CCEA (nM−1) plot was obtained for each DNA sequence (inset II in Fig. 3). The linear regression equations for the DNA mutant P-ATG and GAC-P are 1/Req = 5.32 + 24.57 × 1/CCEA (R = 0.9883), and 1/Req = 5.74 + 22.56 × 1/CCEA (R = 0.9857), respectively. Those for the DNA mutant P-GTG, and the aptamer P are listed in the ESI.† By using the Lineweaver–Burk equation (eqn (1)), the apparent Michaelis–Menten constant (i.e. the dissociation constant, Kd) was obtained. |
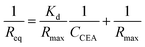 | (1) |
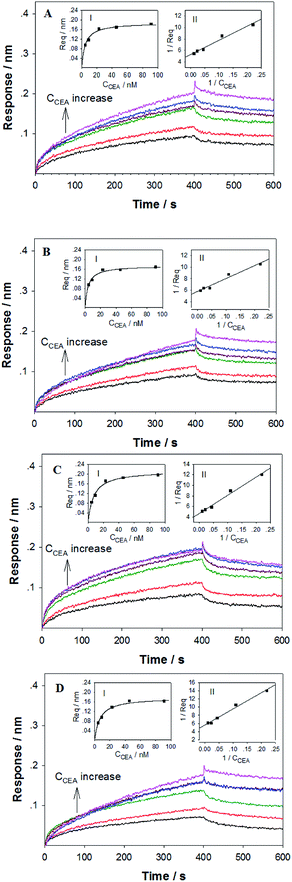 |
| Fig. 3 Sensorgrams of biotinylated TEG moiety modified (A) P-ATG, (B) GAC-P, (C) P-GTG and (D) P sequences binding to various concentrations of CEA using SSA sensors on Octet Red 96. CEA concentration was increased stepwisely from 4.55 nM, to 9.10 nM, 22.73 nM, 45.45 nM, 91.01 nM and finally 181.82 nM. Inset I: the plot of Req versus CCEA. Inset II: the plot of 1/Req versus 1/CCEA. | |
The Kd value for P-ATG, GAC-P, P-GTG, and P was determined to be 4.62 nM, 3.93 nM, 7.33 nM, and 6.95 nM, respectively. The DNA mutant sequences P-ATG and GAC-P exhibited obviously higher affinity to CEA than P. The BLI experimental results are in agreement with the bioinformatic simulation results, suggesting the feasibility of the developed in silico approach for post-SELEX screening, selection, and modification of DNA aptamers.
Verification of the selected DNA aptamers in aptasensor application
The analytical performances of our electrochemical sandwich aptasensors21 fabricated from the in silico selected DNA aptamers (i.e. P-ATG and GAC-P) were investigated. Firstly, the CEA detection sensitivity was evaluated. The DPV curves of the P-ATG aptasensor and the GAC-P aptasensor in response to various CEA concentrations are illustrated in Fig. 4A and B, respectively. The DPV peak current (Ip) increment (ΔIp = Ip − Ip0) in response to CEA compared with control (0 ng mL−1 CEA, Ip0) were taken as the CEA detection signal. For both the P-ATG aptasensor and the GAC-P aptasensor, the ΔIp values increased monotonically and linearly with the CEA concentration (CCEA) ranging from 5 ng mL−1 to 50 ng mL−1 (inset in Fig. 4). The P-ATG aptasensor followed a linear calibration equation of ΔIp (μA) = 0.74 + 0.46 × CCEA (ng mL−1) (R = 0.9959), and exhibited a limit of detection (LOD) of 1.5 ng mL−1 (calculated based on 3σ rule). In contrast, the linear calibration equation for the GAC-P aptasensor was ΔIp (μA) = 0.35 + 0.58 × CCEA (ng mL−1) (R = 0.9963), with a LOD value of 1.2 ng mL−1. The LOD values of the two in silicon selected DNA mutants based aptasensors are obviously superior than that based on P (3.4 ng mL−1), indicating that the two DNA mutant aptasensors possess obviously higher CEA detection sensitivity. The aptasensor application results are consistent with the bioinformatic simulation results and the BLI experimental results.
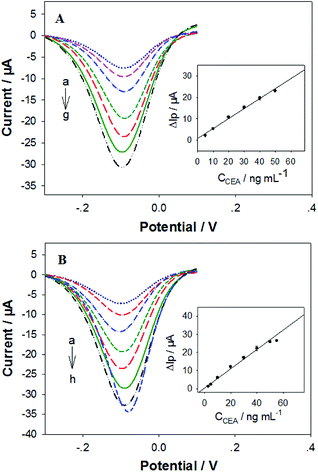 |
| Fig. 4 DPV curves of the aptasensors fabricated from (A) P-ATG, and (B) GAC-P, in response to (a) 0, (b) 5, (c) 10, (d) 20, (e) 30, (f) 40, (g) 50, and (h) 55 ng mL−1 CEA. Inset: the plot of the corresponding ΔIp values versus CCEA. n = 3. | |
The aptasensors fabricated from the in silico selected aptamer P-ATG (Fig. 5A) and GAC-P (Fig. 5B) also exhibited high CEA detection specificity, similar to that from the aptamer P. The interference protein concentrations used in the specificity experiment were referred to their physiological levels.23 Compared with the strong responses towards CEA, no obvious responses were observed towards all the interfering proteins tested, including BSA, HSA, γ-globulin, AFP, and CRP, being consistent with the bioinformatic simulation results (Fig. S5†).
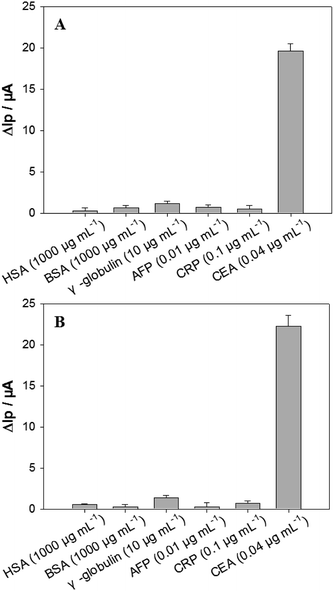 |
| Fig. 5 The DPV ΔIp values of the aptasensors fabricated from (A) P-ATG, and (B) GAC-P in response to CEA and interfering proteins including HSA, BSA, γ-globulin, AFP and CRP. n = 3–6. | |
It may be noted that the ranks of the BLI affinity and the aptasensor sensitivity of the selected aptamer P-ATG and GAC-P were reversed compared to that of the ZDOCK score. The BLI affinity differences as well as the aptasensor sensitivity differences between the aptamer P-ATG and GAC-P were very slight, in contrast to the obvious difference between their ZDOCK scores. It has been reported that a number of factors, like viscosity, pH, and ionic strength can influence protein–protein interaction kinetics.48 In this study, the ZDOCK scoring function did not take the buffer conditions into calculation, which may cause the slight variation from the real situation. However, this slight variation did not affect the feasibility of the developed in silico approach for post-SELEX screening, selection, and modification of DNA aptamers.
Conclusions
An in silico post-SELEX screening approach has been developed and used for selecting DNA mutants derived from the parent aptamer P against CEA. Two DNA mutants (i.e. P-ATG and GAC-P) exhibiting significantly higher binding ability to CEA than the parent DNA aptamer have been identified in the bioinformatic simulation process. P-ATG and GAC-P binds with different CEA structural domains. The in silico selected aptamer P-ATG and GAC-P exhibited obviously higher CEA affinity than P in BLI affinity determination experiment. The newly found aptamer P-ATG and GAC-P performed much better in fabrication of aptasensors, showing obviously higher CEA detection sensitivity than P, without compromising the CEA detection specificity. The developed in silico post-SELEX screening approach should be applicable for obtaining even higher affinity DNA aptamers against CEA, and may be used as a general approach for acquisition of high affinity DNA aptamers against other interested protein targets. The selected high affinity DNA aptamer sequences, and the knowledge about the CEA domains bound with these aptamers could be used for future CEA aptasensor design and fabrication, promisingly applicable for early cancer diagnosis.
Conflicts of interest
There are no conflicts to declare.
Acknowledgements
This work was supported by Plan for Scientific Innovation Talent of Henan Province to H. F. Cui (Grant number 154200510007), the National Natural Science Foundation of China (Grant number NSFC 21345007), the Provincial Natural Science Foundation of Henan (Grant number 182300410314), and the Henan Open-up and Collaboration Program of Science and Technology (Grant number 132106000070).
Notes and references
- N. Zamcheck and E. W. Martin, Cancer, 1981, 47, 1620–1630 CrossRef CAS PubMed.
- M. Grunnet and J. B. Sorensen, Lung Cancer, 2012, 76, 138–143 CrossRef CAS PubMed.
- F. Naghibalhossaini and P. Ebadi, Cancer Lett., 2006, 234, 158–167 CrossRef CAS PubMed.
- A. M. Steward, D. Nixon, N. Zamcheck and A. Aisenberg, Cancer, 1974, 33, 1246–1252 CrossRef CAS.
- A. Malkin, J. A. Kellen, G. M. Lickrish and R. S. Bush, Cancer, 1978, 42, 1452–1456 CrossRef CAS.
- P. M. Drake, W. Cho, B. Li, A. Prakobphol, E. Johansen, N. L. Anderson, F. E. Regnier, B. W. Gibso and S. J. Fisher, Clin. Chem., 2010, 56, 223–236 CrossRef CAS PubMed.
- L. Chen, Z. Zhang, P. Zhang, X. Zhang and A. Fu, Sens. Actuators, B, 2011, 155, 557–561 CrossRef CAS.
- J. Gao, Z. Guo, F. Su, L. Gao, X. Pang, W. Cao, B. Du and Q. Wei, Biosens. Bioelectron., 2015, 63, 465–471 CrossRef CAS PubMed.
- A. D. Ellington and J. W. Szostak, Nature, 1990, 346, 818–822 CrossRef CAS PubMed.
- C. Tuerk and L. Gold, Science, 1990, 249, 505–510 CrossRef CAS PubMed.
- H. Sun, X. Zhu, P. Y. Lu, R. R. Rosato, W. Tan and Y. Zu, Mol. Ther.–Nucleic Acids, 2014, 3, e182 CrossRef CAS PubMed.
- K. H. Lee and H. Q. Zeng, Anal. Chem., 2017, 89, 12743–12748 CrossRef CAS PubMed.
- S. Zhang, L. Ma, K. Ma, B. Xu, L. J. Liu and W. J. Tian, ACS Omega, 2018, 3, 12886–12892 CrossRef CAS PubMed.
- H. Xu, K. Gorgy, C. Gondran, A. Le Goff, N. Spinelli, C. Lopez, E. Defrancq and S. Cosnier, Biosens. Bioelectron., 2013, 41, 90–95 CrossRef CAS PubMed.
- H. Ilkhani, M. Sarparast, A. Noori, S. Zahra Bathaie and M. F. Mousavi, Biosens. Bioelectron., 2015, 74, 491–497 CrossRef CAS PubMed.
- N. Arroyo-Currás, K. Scida, K. L. Ploense, T. E. Kippin and K. W. Plaxco, Anal. Chem., 2017, 89, 12185–12191 CrossRef PubMed.
- K. H. Lee and H. Zeng, Anal. Chem., 2017, 89, 12743–12748 CrossRef CAS PubMed.
- Q. T. Zhou, X. B. Sun, X. L. Xia, Z. Fan, Z. F. Lou, S. W. Zhou, E. Shakhnovich and H. J. Liang, J. Phys. Chem. Lett., 2017, 8, 407–414 CrossRef CAS PubMed.
- T. Fukaya, K. Abe, N. Savory, K. Tsukakoshi, W. Yoshida, S. Ferri, K. Sode and K. Ikebukuro, J. Biotechnol., 2015, 212, 99–105 CrossRef CAS PubMed.
- L. Smith, US Pat. Appl. Publ., US 20130101506 A1, 2013.
- Q. L. Wang, H. F. Cui, X. J. Song, S. F. Fan, L. L. Chen, M. M. Li and Z. Y. Li, Sens. Actuators, B, 2018, 260, 48–54 CrossRef CAS.
- H. Cheng, L. Xu, H. Zhang, A. Yu and G. Lai, Analyst, 2016, 141, 4381–4387 RSC.
- H. Quan, C. Zuo, T. Li, Y. Liu, M. Li, M. Zhong, Y. Zhang, H. Qi and M. Yang, Electrochim. Acta, 2015, 176, 893–897 CrossRef CAS.
- R. H. Fletcher, Ann. Intern. Med., 1986, 104, 66–73 CrossRef CAS PubMed.
- N. Kim, H. H. Gan and T. Schlick, RNA, 2007, 13, 478–492 CrossRef CAS PubMed.
- A. Bini, M. Mascini and A. P. Turner, Biosens. Bioelectron., 2011, 26, 4411–4416 CrossRef CAS PubMed.
- N. Savory, K. Abe, K. Sode and K. Ikebukuro, Biosens. Bioelectron., 2010, 26, 1386–1391 CrossRef CAS PubMed.
- M. Heiat, A. Najafi, R. Ranjbar, A. M. Latifi and M. J. Rasaee, J. Biotechnol., 2016, 230, 34–39 CrossRef CAS PubMed.
- R. Rajamani and A. C. Good, Curr. Opin. Drug Discovery Dev., 2007, 10, 308–315 CAS.
- K. K. Alam, J. L. Chang and D. H. Burke, Mol. Ther.–Nucleic Acids, 2015, 4, e230 CrossRef CAS PubMed.
- J. Hoinka, E. Zotenko, A. Friedman, Z. E. Sauna and T. M. Przytycka, Bioinformatics, 2012, 28, i215–i223 CrossRef PubMed.
- P. C. Anderson and S. Mecozzi, Nucleic Acids Res., 2005, 33, 6992–6999 CrossRef CAS PubMed.
- M. Mascini, M. Sergi, D. Monti, M. Del Carlo and D. Compagnone, Anal. Chem., 2008, 80, 9150–9156 CrossRef CAS PubMed.
- M. Mascini, A. Macagnano, D. Monti, M. Del Carlo, R. Paolesse, B. Chen, P. Warner, A. D'Amico, C. Di Natale and D. Compagnone, Biosens. Bioelectron., 2004, 20, 1203–1210 CrossRef CAS PubMed.
- I. Chianella, M. Lotierzo, S. A. Piletsky, I. E. Tothill, B. Chen, K. Karim and A. P. Turner, Anal. Chem., 2002, 74, 1288–1293 CrossRef CAS PubMed.
- E. V. Piletska, N. W. Turner, A. P. Turner and S. A. Piletsky, J. Controlled Release, 2005, 108, 132–139 CrossRef CAS PubMed.
- R. Ahirwar, S. Nahar, S. Aggarwal, S. Ramachandran, S. Maiti and P. Nahar, Sci. Rep., 2016, 6, 21285 CrossRef CAS PubMed.
- P. C. Hsieh, H. T. Lin, W. Y. Chen, P. J. Tsai and W. P. Hu, BioMed Res. Int., 2017, 2017, 5041683 Search PubMed.
- L. L. Dong, Q. W. Tan, W. Ye, D. L. Liu, H. F. Chen, H. W. Hu, D. Wen, Y. Liu, Y. Cao, J. W. Kang, J. Fan, W. Guo and W. Z. Wu, Sci. Rep., 2015, 5, 15552 CrossRef CAS PubMed.
- R. Chen, L. Li and Z. Weng, Proteins, 2003, 52, 80–87 CrossRef CAS PubMed.
- M. Zuker, Nucleic Acids Res., 2003, 31, 3406–3415 CrossRef CAS PubMed.
- M. Popenda, M. Szachniuk, M. Antczak, K. J. Purzycka, P. Lukasiak, N. Bartol, J. Blazewicz and R. W. Adamiak, Nucleic Acids Res., 2012, 40, e112 CrossRef CAS PubMed.
- N. Korotkova, Y. Yang, I. Le Trong, E. Cota, B. Demeler, J. Marchant, W. E. Thomas, R. E. Stenkamp, S. L. Moseley and S. Matthews, Mol. Microbiol., 2008, 67, 420–434 CrossRef CAS PubMed.
- B. G. Pierce, K. Wiehe, H. Hwang, B. H. Kim, T. Vreven and Z. Weng, Bioinformatics, 2014, 30, 1771–1773 CrossRef CAS PubMed.
- G. L. Ciesielski, V. P. Hytonen and L. S. Kaguni, Methods Mol. Biol., 2016, 1351, 223–231 CrossRef CAS PubMed.
- D. Verzijl, T. Riedl, P. Parren and A. F. Gerritsen, Biosens. Bioelectron., 2017, 87, 388–395 CrossRef CAS PubMed.
- X. Lou, M. Egli and X. Yang, Curr. Protoc. Nucleic Acid Chem., 2016, 67, 1–15 CrossRef PubMed.
- G. Schreiber, Curr. Opin. Struct. Biol., 2002, 12, 41–47 CrossRef CAS PubMed.
Footnote |
† Electronic supplementary information (ESI) available: Additional information and figures. See DOI: 10.1039/c8ra10163a |
|
This journal is © The Royal Society of Chemistry 2019 |
Click here to see how this site uses Cookies. View our privacy policy here.