DOI:
10.1039/C9RA01975H
(Paper)
RSC Adv., 2019,
9, 19261-19270
Evaluation of anti-EGFR-iRGD recombinant protein with GOLD nanoparticles: synergistic effect on antitumor efficiency using optimized deep neural networks†
Received
14th March 2019
, Accepted 13th June 2019
First published on 19th June 2019
Abstract
The epidermal growth factor receptor, also known as EGFR, is a tyrosine kinase receptor commonly found in epithelial tumors. As part of the first target for cancer treatment, EGFR has been the subject of intense research for more than 20 years; as a result, there are a number of anti-EGFR agents currently available. More recently, with our basic understanding of mechanisms related to receptor activation and function, both the secondary and primary forms of EGFR somatic mutations have led to the discovery of new anti-EGFR agents aimed at providing new insights into the clinical targeting of this receptor and possibly acting as an ideal model for developing strategies to target other types of receptors. In this study, we use genomic pattern to prove that EGFR is most frequently altered in GBM, glioma and astrocytoma; and analysed the prognostic potentiality of EGFR in glioma, which is a major type of brain tumor. Further we proposed a new screening technique for EGFR inhibitors by employing an in silico optimized deep neural network approach. This method was applied to screen a nanoparticle (NP) library, and it was concluded that gold NPs (AuNPs) induced significant inhibition of EGFR compared with other selected NPs. These findings were further analyzed by molecular docking, systems biology, time course simulations and synthetic biology (biological circuits), revealing that anti-EGFR-iRGD and AuNP showed potential inhibition against tumors caused by EGFR.
1. Introduction
This study merges computer science, mathematics, statistics and biology, among other fields.1 Currently, bioinformatics tools and techniques are being used extensively to gain an in-depth insight into the molecular mechanisms of human diseases.2–5
Epidermal growth factor receptor (EGFR), also known as ErbB-1 and human HER1, is a transmembrane protein that functions as a receptor for members of the epidermal growth factor (EGF) family of extracellular protein ligands.6 ErbB receptors are a subfamily of four closely related tyrosine kinases, namely, EGFR HER1 (ErbB-1), HER2 (ErbB-2), HER3 (ErbB-3) and HER4 (ErbB-4).7 ErbB receptors are activated by binding with specific ligands, including EGF and transforming growth factor α (TGFα).8 The tyrosine kinases (ErbBs) play crucial roles in the regulation of cell differentiation, proliferation, migration and survival.9 Structure-based studies have been carried out for the regulation of EGFRs.10 Mutations in the EGFR coding gene affect its expression or activity, which may lead to several cancers.11 EGFR tests and the presence of cancer have been known to have a relationship with each other.12,13 Overexpression of human EGFR tyrosine kinases is linked with the development and progression of many types of tumors in different body parts,14 while poor signaling is linked with neurodegenerative diseases, such as Alzheimer's disease.15
Elevated levels of EFGR are indicators of multiple types of cancer and are known to stimulate tumor growth.16 There are more than 10 types of cancers reported to show elevated levels of EGFR compared with normal tissues.17 EGFR can be treated as an effective prognostic indicator for head and neck, ovarian, cervical, bladder and esophageal cancers. Efforts have been made to interrupt EGFR signaling to prevent the growth of tumors that are expressing EGFR by blocking the extracellular domain binding site of EGFR or by inhibiting intracellular tyrosine kinase activity.18,19
A nanoparticle (NP) is a particle whose dimensions are measured in nanometers. These particles are present from both natural and manmade sources. As NPs are very small, they possess certain unique characteristics that enable their application in various fields of medicine, catalysis and engineering. Over the years and due to the greater emphasis placed on developing gold NP technology, NPs have been used in high-end applications such as organic photovoltaics, therapeutic agents and sensory probes.20 Certain physical properties such as the optical and electronic properties can be fine-tuned depending on the size, aggregation state, and shape of the gold NP. All of these customizable physical properties make gold NPs our first choice for research.21–24 The field of systems biology employs techniques such as metabolic networks or cell signaling networks.25,26
In this study we found EGFR is majorly located in brain. The major genomic alternation types are mutation, amplification and deep deletion, and found the prognosis of EGFR-mutated glioma patients are significantly worse than not mutated ones (p < 0.0001); where the survival rates of patients with higher EGFR expression are significantly lower than those of lower EGFR expression (p < 0.0001); which shown that mutation and expression of EGFR can be treated as independent prognostic indicator for glioma patients; with the help of genomic pipeline we proposed EGFR inhibitors using deep learning approach and further validated by molecular docking, systems biology and synthetic biology technique that anti-EGFR-iRGD and AuNP complex shown lead inhibition against tumor.
2. Methodology
2.1. Pan-cancer genomic and transcriptomic analysis of EGFR
In order to depict the genomic pattern EGFR in pan-cancer manner, we retrieved the mutational information from cBioPortal of all the available cohorts which are provide with both mutational and copy number variation data. We excluded the cancer type of which the genomic alternation rate of EGFR is less than 5% and the sample size is below 50. We utilized the GEPIA to analysis the expression profile of EGFR among 33 cancer types in TCGA cohorts and paired normal tissues in GTEx datasets in pan-cancer and interactive manner. The expression values of EGFR, which are quantified as log2 transformed transcripts per million (TPM), of cancers were compared to those of corresponding normal tissues. We used the limma as differential expression detection backend with the threshold of log2 fold-change > 1 and q-value < 0.05 to identify the cancer types with altered EGFR expression in comparison of paired normal samples.
2.2. Survival analysis of EGFR in brain tumor
In order to measure the prognostic potential of EGFR in brain tumor. We used the R package “curatedTCGAData” to retrieve the mutational, expressional and clinical information of EGFR in LGG (Low Grade Glioma) and GBM (glioblastoma multiforme) cohorts. We combined the two datasets into one merged dataset simply stands for gliomata in brain. The patients in the merged data set were stratified into “high” and “low” based on the expression of EGFR using maximally selected rank statistics. Furthermore, these patients were also grouped by the EGFR mutational status. We used Kaplan–Meier method to estimate the survival probability and we used log-rank test to evaluate the significance between two groups. All the survival analysis and visualization were performed by R package “survminer”.
2.3. Virtual screening for potential nanoparticle against anti-EGFR-iRGD by deep neural network approach
The deep neural network algorithm was concluded to be advantageous, as it screened the potential inhibitors (NP's) based on the arbitrary configurations of the NPs, such as size, shape, molecular weight, hydrogen bond donor, hydrogen bond acceptor, Monoisotopic Mass (MM), Exact Mass (EM), Formal Charge (FC), Heavy Atom Count (HAC), Defined Atom Stereocenter Count (DASC), Undefined Atom Stereocenter Count (UASC), Defined Bond, Stereocenter Count (DBSC), Undefined Bond Stereocenter Count (UBSC), Isotope Atom Count (IAC), covalently-bonded unit. Herein, we employed an approach that computes molecular fingerprint vectors with differentiable neural networks whose input represents the NPs, where vertices represent individual atoms and edges represent bonds. The bottom lower layers of the network are applied to each atom and its neighboring entities. We applied a 70/30 ratio for training and testing a dataset of potential NPs. The proposed deep neural network algorithm was applied for screening the PubChem chemical compound library (Table 1).
Table 1 Nanoparticles library that were used for training and testing of NP dataset to predict binding affinity with EGFR with different NP, where different columns indicate the feature descriptors of NPa
Name |
MW (g mol−1) |
HBDC |
HBAC |
MM (g mol−1) |
EM (g mol−1) |
FC |
HAC |
DASC |
UASC |
DBSC |
UBSC |
IAC |
CBUC |
Note: abbreviation used Molecular Weight (MW), Hydrogen Bond Donor Count (HBDC), Hydrogen Bond Acceptor Count (HBAC), Monoisotopic Mass (MM), Exact Mass (EM), Formal Charge (FC), Heavy Atom Count (HAC), Defined Atom Stereocenter Count (DASC), Undefined Atom Stereocenter Count (UASC), Defined Bond Stereocenter Count (DBSC), Undefined Bond Stereocenter Count (UBSC), Isotope Atom Count (IAC), Covalently-Bonded Unit Count (CBUC), TRUE (T).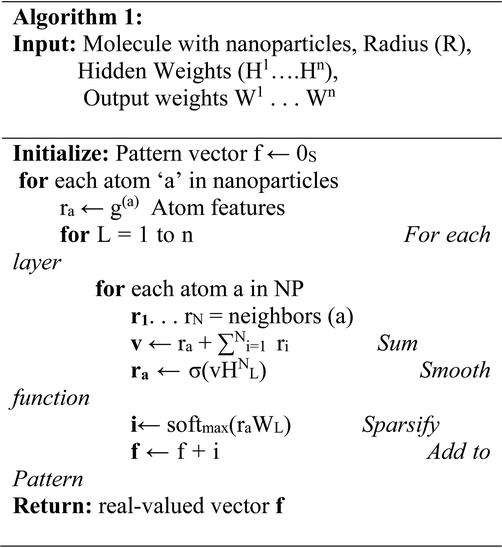 |
Γ-Aluminium oxide |
101.96 |
0 |
3 |
101.948 |
101.948 |
0 |
5 |
0 |
0 |
0 |
0 |
0 |
5 |
Zirconium(IV) oxide-yttria stabilized |
349.031 |
0 |
5 |
347.691 |
347.691 |
0 |
8 |
0 |
0 |
0 |
0 |
0 |
2 |
Zirconium dioxide |
275.248 |
0 |
5 |
273.874 |
273.874 |
2 |
11 |
0 |
0 |
0 |
0 |
0 |
2 |
Zinc oxide |
81.379 |
0 |
1 |
79.924 |
79.924 |
0 |
2 |
0 |
0 |
0 |
0 |
0 |
1 |
Zinc |
65.38 |
0 |
0 |
63.929 |
63.929 |
0 |
1 |
0 |
0 |
0 |
0 |
0 |
1 |
Ytterbium trifluoride |
230.049 |
0 |
3 |
230.934 |
230.934 |
0 |
4 |
0 |
0 |
0 |
0 |
0 |
1 |
Tungsten disulfide |
247.96 |
0 |
2 |
247.895 |
247.895 |
−4 |
3 |
0 |
0 |
0 |
0 |
0 |
3 |
Tungsten |
183.84 |
0 |
0 |
183.951 |
183.951 |
0 |
1 |
0 |
0 |
0 |
0 |
0 |
1 |
Titanium dioxide |
79.865 |
0 |
2 |
79.938 |
79.938 |
0 |
3 |
0 |
0 |
0 |
0 |
0 |
1 |
Titanium |
47.867 |
0 |
0 |
47.948 |
47.948 |
0 |
1 |
0 |
0 |
0 |
0 |
0 |
1 |
Sodium silicate |
122.062 |
0 |
3 |
121.941 |
121.941 |
0 |
6 |
0 |
0 |
0 |
0 |
0 |
3 |
Silver |
107.868 |
0 |
0 |
106.905 |
106.905 |
0 |
1 |
0 |
0 |
0 |
0 |
0 |
1 |
Silicon dioxide |
60.083 |
0 |
2 |
59.967 |
59.967 |
0 |
3 |
0 |
0 |
0 |
0 |
0 |
1 |
Rhodium |
102.906 |
0 |
0 |
102.905 |
102.905 |
0 |
1 |
0 |
0 |
0 |
0 |
0 |
1 |
Potassium |
39.098 |
0 |
0 |
38.964 |
38.964 |
0 |
1 |
0 |
0 |
0 |
0 |
0 |
1 |
Platinum |
195.084 |
0 |
0 |
194.965 |
194.965 |
0 |
1 |
0 |
0 |
0 |
0 |
0 |
1 |
Phosphorus |
30.974 |
0 |
0 |
30.974 |
30.974 |
0 |
1 |
0 |
0 |
0 |
0 |
0 |
1 |
Palladium |
106.42 |
0 |
0 |
105.903 |
105.903 |
0 |
1 |
0 |
0 |
0 |
0 |
0 |
1 |
Nickel(II) oxide |
74.692 |
0 |
1 |
73.93 |
73.93 |
0 |
2 |
0 |
0 |
0 |
0 |
0 |
1 |
Molybdenum disulfide |
160.07 |
0 |
2 |
161.85 |
161.85 |
0 |
3 |
0 |
0 |
0 |
0 |
0 |
1 |
Molybdenum |
95.95 |
0 |
0 |
97.905 |
97.905 |
0 |
1 |
0 |
0 |
0 |
0 |
0 |
1 |
Manganese |
54.938 |
0 |
0 |
54.938 |
54.938 |
0 |
1 |
0 |
0 |
0 |
0 |
0 |
1 |
Kojic acid |
142.11 |
2 |
4 |
142.027 |
142.027 |
T |
0 |
10 |
0 |
0 |
0 |
0 |
0 |
Iron |
55.845 |
0 |
0 |
55.935 |
55.935 |
0 |
1 |
0 |
0 |
0 |
0 |
0 |
1 |
Hydroxy acid |
195.174 |
3 |
4 |
195.053 |
195.053 |
T |
0 |
14 |
0 |
1 |
0 |
0 |
0 |
Graphite |
12.011 |
0 |
0 |
12 |
12 |
T |
0 |
1 |
0 |
0 |
0 |
0 |
0 |
Gold (Au) |
196.967 |
0 |
0 |
196.967 |
196.967 |
0 |
1 |
0 |
0 |
0 |
0 |
0 |
1 |
Copper sulfide |
95.606 |
0 |
1 |
94.902 |
94.902 |
0 |
2 |
0 |
0 |
0 |
0 |
0 |
2 |
Copper |
63.546 |
0 |
0 |
62.93 |
62.93 |
0 |
1 |
0 |
0 |
0 |
0 |
0 |
1 |
Cobalt oxide |
240.796 |
0 |
4 |
240.779 |
240.779 |
0 |
7 |
0 |
0 |
0 |
0 |
0 |
7 |
Cerium(IV) oxide |
172.114 |
0 |
2 |
171.895 |
171.895 |
0 |
3 |
0 |
0 |
0 |
0 |
0 |
1 |
Carbonmanganese oxide |
86.936 |
0 |
2 |
86.928 |
86.928 |
0 |
3 |
0 |
0 |
0 |
0 |
0 |
1 |
Carbon |
12.011 |
0 |
0 |
12 |
12 |
T |
0 |
1 |
0 |
0 |
0 |
0 |
0 |
Calcium hydroxide |
150.19 |
1 |
2 |
149.999 |
149.999 |
0 |
9 |
0 |
0 |
0 |
0 |
0 |
3 |
Calcium carbonate |
100.086 |
0 |
3 |
99.947 |
99.947 |
0 |
5 |
0 |
0 |
0 |
0 |
0 |
2 |
Calcium silicate hydrate |
134.175 |
1 |
4 |
133.935 |
133.935 |
0 |
6 |
0 |
0 |
0 |
0 |
0 |
3 |
Calcium |
40.078 |
0 |
0 |
39.963 |
39.963 |
0 |
1 |
0 |
0 |
0 |
0 |
0 |
1 |
Boron nitride |
24.817 |
0 |
1 |
25.012 |
25.012 |
0 |
2 |
0 |
0 |
0 |
0 |
0 |
1 |
Boron |
10.81 |
0 |
0 |
11.009 |
11.009 |
0 |
1 |
0 |
0 |
0 |
0 |
0 |
1 |
Barium strontium titanate |
336.81 |
0 |
4 |
337.738 |
337.738 |
0 |
7 |
0 |
0 |
0 |
0 |
0 |
7 |
Aluminum |
26.982 |
0 |
0 |
26.982 |
26.982 |
0 |
1 |
0 |
0 |
0 |
0 |
0 |
1 |
Aluminium oxide |
101.96 |
0 |
3 |
101.948 |
101.948 |
0 |
5 |
0 |
0 |
0 |
0 |
0 |
5 |
2.4. Molecular modelling
Molecular docking was employed specifically using the docking programs for anti-EGFR and GOLD (CID_23985)27 to successfully predict the predominant binding modes between them. The respective chemical structures were retrieved from the PubChem database, and the protein structure was collected from the RCSB-PDB databank.28 We then performed the docking operation for the NPs (GOLD) with the help of the AutoDock4.2 programs. Herein, the addition of hydrogen atoms with Kollman and Gasteiger charges yielded the preparation of the EGFR structure.29
2.5. Biochemical pathway designing for EGFR
As part of the step involving the design and execution of the EGFR's in silico biochemical pathway, we carried out the necessary literature reviews to gather the available information regarding EGFRs. We further acquired information on cancer-related topics, such as genes, truncating proteins, generic proteins, the role of GOLD, ions, and other molecules as shown in Fig. S1 (ESI†), the complete EGFR pathway can be seen, showing its interactions with the other entities. This figure was further created using the System's Biology Workbench to show the result of the interacting molecules in the presence of gold NPs. Furthermore, the pathway nodes are a representation of the entities, and the edges are a representation of the connectivity involved in one node to another that are very close to each other. The inhibition of EGFR on the biochemical pathway as well as the GOLD interactions are further constructed as well. Additionally, the participating GOLD is included to complete the biochemical pathway.
2.6. Time course simulation studies for EGFR
To conduct pharmacokinetics studies in the presence of GOLD NPs (GOLD), every entity value is assigned according to the concentration; here a concentration of 0.45 μmol is assigned to GOLD and therapeutic NP's dose.30 A simulation of the EGFR biochemical pathway was carried out with respect to the EGFR transition time in presence of GOLD NP.
2.7. Boolean network analysis using synthetic biology
Biological based Boolean circuit for the EGFR was structured by utilizing our own java script. It portrays the communications and reenactment as far as rationale circuit for EGFR with potential nano-sedate (GOLD) and other connecting substances engaged with disease. This rationale circuit was built dependent on the purposed finish biochemical pathway of malignancy, wherein input esteems were allocated as 1 and 0 or TRUE and FALSE utilizing fundamental information and yield all-inclusive table.
3. Results and discussion
There is renewed interest in targeting tumors and penetrating cancer cells to achieve the therapeutic purposes of drugs. Red blood cells are readily available and can act as biocompatible long-circulating intravascular carriers that are favorable toward chemical modifications, drug loading and reinjection. The goal of this research is to develop a tumor-targeting biocompatible drug delivery system for the delivery of antitumor drugs.
3.1. Pan-cancer genomic and transcriptomic profile of EGFR
We firstly analyzed the EGFR mutational and expressional profile in pan-cancer manner. Among all the TCGA cohorts, we found LGG, GBM, KIRC (kidney renal clear cell carcinoma) and THYM (thymoma and thymic carcinoma) show significant up-regulated expression of EGFR compared to normal tissues (Fig. 1A). As for the genomic pattern, we found EGFR is most frequently altered in GBM, glioma and astrocytoma (Fig. 1B), all of them are majorly located in brain. The major genomic alternation types are mutation, amplification and deep deletion.
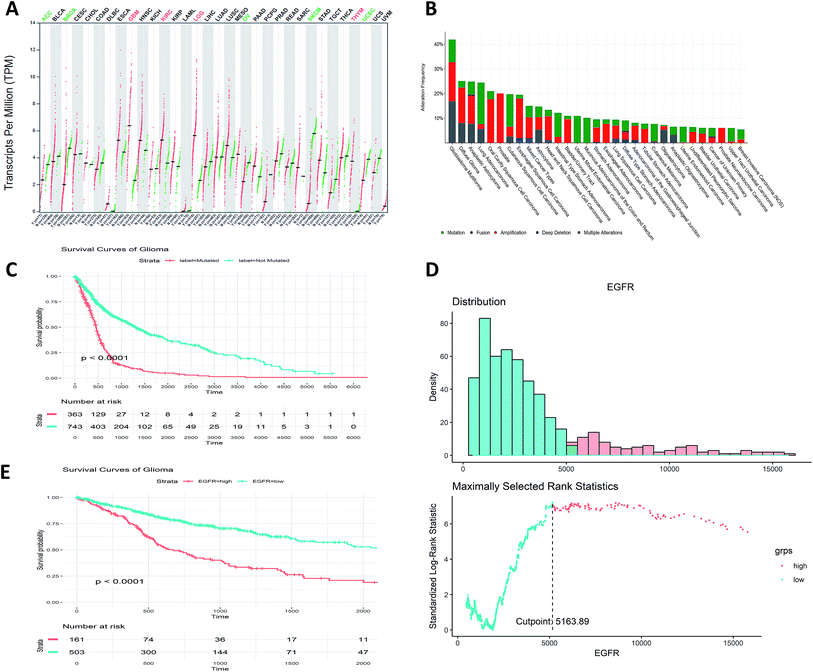 |
| Fig. 1 Pan-cancer genomic and transcriptomic profile of EGFR and KM plots. (A) Pan-cancer expressional profile of EGFR. “T” stands for tumor tissue and “N” stands for paired normal tissue. The expression abundance is measured by log-normalized transcripts per million (TPM). The green color of cancer type means that the expression of EGFR is significantly down-regulated in cancer tissue compared to paired normal tissue. The red color of cancer type means that the expression of EGFR is significantly up-regulated in cancer tissue compared to paired normal tissue. (B) Pan-cancer genomic alternation rate of EGFR. (C) KM plot for the EGFR mutation groups. (D) Plot for cut point determination of EGFR expression value. The optimal cut point is the expression value with the highest standardized log-rank statistics. (E) KM plots for the EGFR groups based on its expression. | |
3.2. EGFR can be an independent prognostic biomarker for gliomata
Further analysis of the prognostic potentiality of EGFR in glioma, which is a major type of brain tumor, comprising about 80% of all the malignancies in brain. Grouped by the mutational status (EGFR-mutated and EGFR-not mutated), we found the prognosis of EGFR-mutated glioma patients are significantly worse than not mutated ones (p < 0.0001, Fig. 1C). Stratified by the expression values of EGFR by the maximally selected rank statistics (Fig. 1D), we found the survival rates of patients with higher EGFR expression are significantly lower than those of lower EGFR expression (p < 0.0001, Fig. 1E). These results show that the mutation and the expression status of EGFR can be treated as independent prognostic indicator for glioma patients.
3.3. Virtual screening using deep learning approach
The deep learning approach allows prediction from one point of a structure to the other end, where the inputs are the NPs and their stereo chemical properties, such as size, shape, molecular weight, hydrogen bond donor, hydrogen bond acceptor, Monoisotopic Mass (MM), Exact Mass (EM), Formal Charge (FC), Heavy Atom Count (HAC), Defined Atom Stereocenter Count (DASC), Undefined Atom Stereocenter Count (UASC), Defined Bond Stereocenter Count (DBSC), Undefined Bond Stereocenter Count (UBSC), Isotope Atom Count (IAC), covalently-bonded unit. We used PERL script to train and test our dataset, where the dataset contains NPs with unique features, such as arbitrary configurations of the NP. Here, we showed an algorithm of the proposed features that is more interpretable and has a better predictive performance compared to wet-lab data. Following screening of the whole PubChem compound library (Table 1), we concluded that GOLD was a potential inhibitor against the EGFR protein, and we then further analyzed the screened AuNP.
3.4. Molecular modelling for validation of deep learning approach
Molecular docking was employed specifically using the docking programs for anti-EGFR and GOLD (CID_23985) to successfully predict the predominant binding modes between them where observed docking energy was −3.5 kcal mole−1, and the compound was bound with ASN845 with a distance of 2.1 Å from the metal–donor interaction with gold shown in Fig. 2. For validation of anti-EGFR-iRGD and GOLD complex we did literature survey to cross-validate in previous studies many nanoparticles were identified as a potential carrier for anti-EGFR.30–34
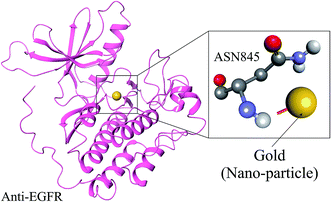 |
| Fig. 2 Anti-EGFR-IRGD docking pose with AuNP, where upper compartments represents the interaction with AuNP with molecular interaction view and lower compartment represents the AuNP interaction with anti-EGFR-iRGD. | |
3.5. Biochemical pathway designing for anti-EGFR-iRGD
As part of the study, anti-EGFR-iRGD NPs measuring 100 nm in size were constructed. We used a lipid insertion method to successfully functionalize these NPs without resorting to direct chemical conjugation. The process was successful because it was highly targeted and it further augmented the cytotoxic effects toward nontargeted RBC-PTX along with the interesting combination of anti-EGFR-iRGD and RBC-PTX. Part of our results revealed that anti-EGFR-iRGD was able to successfully penetrate into the tumor, as shown in Fig. S1 (ESI†).
The anti-EGFR-iRGD belongs to a group of tyrosine kinase receptors, and this group also includes erbB2/HER-2, erbB3/HER-3, and erbB4/HER-4. These receptors attach to the cytoplasmic membrane, and they share a similar structure composed of an extracellular ligand-binding domain, along with a short hydrophobic transmembrane region and an intracytoplasmic tyrosine kinase domain. Overexpression of EGFRs is common in cancer cases and can occur via NP-dependent and NP-independent mechanisms. NPs attach to EGFRs, as well as EGF, further transforming growth factor-α. The binding of NPs to the receptor is known to induce a conformational change in the receptor ectodomain that enables dimerization as well as autophosphorylation of tyrosine residues in the host with the COOH-terminal tail belonging to the receptor.
The activation of the NP-independent receptor also occurs in tumors that display certain forms of HER as well as EGFRs with a deletion in the extracellular domain; this further results in the activation of the constitutive receptor. Overexpression of the urokinase-type plasminogen activator receptor results in the activation of GOLD-independent anti-EGFR-iRGD by means of α5β1 integrin. Finally, cellular stresses, such as radiation, contribute toward ligand-independent receptor activation. These stresses are known to antagonize the activities related to the kinase receptor, further shifting the equilibrium of basal phosphorylation to a more activated state. The phosphorylation of key tyrosine residues within the COOH-terminal portion of the anti-EGFR-iRGD results from activation of the receptor. This process creates certain docking sites for cytoplasmic proteins that contain, for example, Src homology 2 and phosphotyrosine-binding domains. These sets of proteins cling to targeted phosphotyrosine residues and further boost intracellular signalling through numerous pathways.35,36
3.6. Ras/Raf/mitogen-activated protein kinase pathway
The Ras/Raf/mitogen-activated protein kinase pathway is very important and is responsible for regulating cell proliferation and survival. Once the anti-EGFR-iRGD is phosphorylated, the complex that was created by the adaptor protein Grb2 and Sos finally attach directly or by means of an association with Shc, which is an adaptor molecule. This attachment is performed at certain docking sites located on the receptor. This type of interaction leads to a conformational modification of Sos, which can then recruit Ras-GDP, resulting in the activation of Ras.36 Ras-GTP activates Raf-1 by means of certain midway steps, leading to the phosphorylation of mitogen-activated protegolin kinases (MAPKs) extracellular signal-regulated kinases 1 and 2. The activated MAPKs are transported to the nucleus region, where they are involved in the phosphorylation of specific transcription factors and cell proliferation, as shown in Fig. S1 (ESI†).
3.7. Phosphatidylinositol 3-kinase/Akt pathway
The phosphatidylinositol 3-kinase/Akt pathway is highly involved in certain processes, such as cell growth, apoptosis resistance, invasion, and migration. The beginning of the pathway is responsible for driving EGFR-dependent PI3K activation and consists of the dimerization of receptors with HER-3, as shown in Fig. S1 (ESI†). There are few docking sites for p85 on EGFR, while the number of docking sites for p85 is plentiful on HER-3. Therefore, alternatively, the p85 subunit can coordinate with EGFR by means of a docking protein called Gab-1.
3.8. Phospholipase Cγ
Phospholipase Cγ is best known to interact directly with EGFR, and it is activated and hydrolyzed by phosphatidylinositol 4,5-diphosphate to produce inositol 1,3,5-triphosphate. The latter is responsible for the release of intracellular calcium, along with other proteins, such as 1,2-diacylglycerol. As a result of the activation of protein kinase C, the terminal kinase activation of MAPK and the c-Jun NH2-terminal can occur.
3.9. Signal transducers and activators of transcription pathway
Signal transducers and activators of transcription, also known as STAT proteins, are known for the great number of interactions with phosphotyrosine residues by means of Src homology 2 domains. Upon dimerization, these proteins translocate to the nucleus and initiate the expression of targeted genes. The constitutive activation of STAT proteins and, most importantly, that of STAT 3 proteins are known to occur in a number of tumor-derived primary cancers and cell lines. Penetration of the STAT3 protein is known to result in augmented activity of membrane-associated tyrosine kinases—notably that of EGFR and HER-2, as well as the platelet-derived growth factor receptor.
3.10. Src kinase pathways
As an integral member of a nine-gene family, Src plays a very important role in regulating cell proliferation, migration, adhesion, angiogenesis, and immune function. It is located in the cytosol and is known to lead activation of a series of substrates, which includes focal adhesion kinase, PI3K, and STAT proteins. Src is known to operate independently, but it does coordinate with the signaling of certain other receptors tyrosine kinases. This form of interaction between the EGFR and Src is very complex in nature. While Src does act as a signal transducer, it also acts as an EGFR activation enhancer.
3.11. Time course simulation studies for anti-EGFR-iRGD
To conduct pharmacokinetics studies in the presence of GOLD NPs (GOLD), every entity value is assigned according to the concentration; here a concentration of 0.45 μmol is assigned to GOLD. A simulation of the pharmacokinetics was then carried out with respect to the anti-EGFR-iRGD transition time as well as that of the whole biochemical pathway due to the presence of both the GOLD NP and the targeted anti-EGFR-iRGD. Simultaneously, an investigation was performed in the absence and presence of the NPs. The major prediction of this study is that by examining the interaction of the NPs with a systems biology approach, we can determine the role of certain entities involved directly or indirectly in anti-EGFR-iRGD expression following changes in the biochemical pathway of cancer. Fig. S2 (ESI†) shows the Time Course Simulation, which are indicators of the entity's concentration over time, in assorted colors. The transition time is represented on the X-axis while the concentration value of the entities is represented on the Y-axis.
The initial proposal concerning the targeting of anti-EGFR-iRGD in cancer was mostly based on the notion that the receptor was commonly overexpressed in epithelial tumors combined with the preclinical activity of anti-EGFRs. Fig. S3 (ESI†) shows the stochastics simulation, which are indicators of the entity's concentration over time, in assorted colors. The transition time is represented on the X-axis while the concentration value of the entities is represented on the Y-axis.
3.12. Boolean network analysis using synthetic biology
Biological based Boolean circuit for the anti-EGFR-iRGD was structured by utilizing our own java content. Utilizing DNA computing approach, exchanging science can be utilized to build fake Boolean system which can navigate entire administrative component. It depends on biological circuit silicon chip actualized on A, C, T and G units that enables life forms to detect and control the administrative frameworks. Organic circuit approach can dissect circuit conduct and actualize into creating RNA based post-transcriptional and translational control frameworks. Biological circuits group and switch between cell states and in addition circuits to constantly adjust the states for proficient creation. At the point when the flag is “off”, the circuit is “off” and when flag is “on”, the circuit winds up responsive in a log-direct design. Here, we built the biological circuit for anti-EGFR-iRGD and endeavoured to examine the role in disease with NP and without NP. The development of first component for the anti-EGFR-iRGD with GOLD NP was to restrain the direction of malignancy instrument in milliseconds to nanoseconds, despite the fact that turn of system changes may be all the more quickly. The lines in figure speak to the 0 and 1 supply for each connecting particles, entire circuits are mix of essential rationale GATE. Blue delineate the dynamic shape and red show inert type of biological circuits shown in Fig. 3.
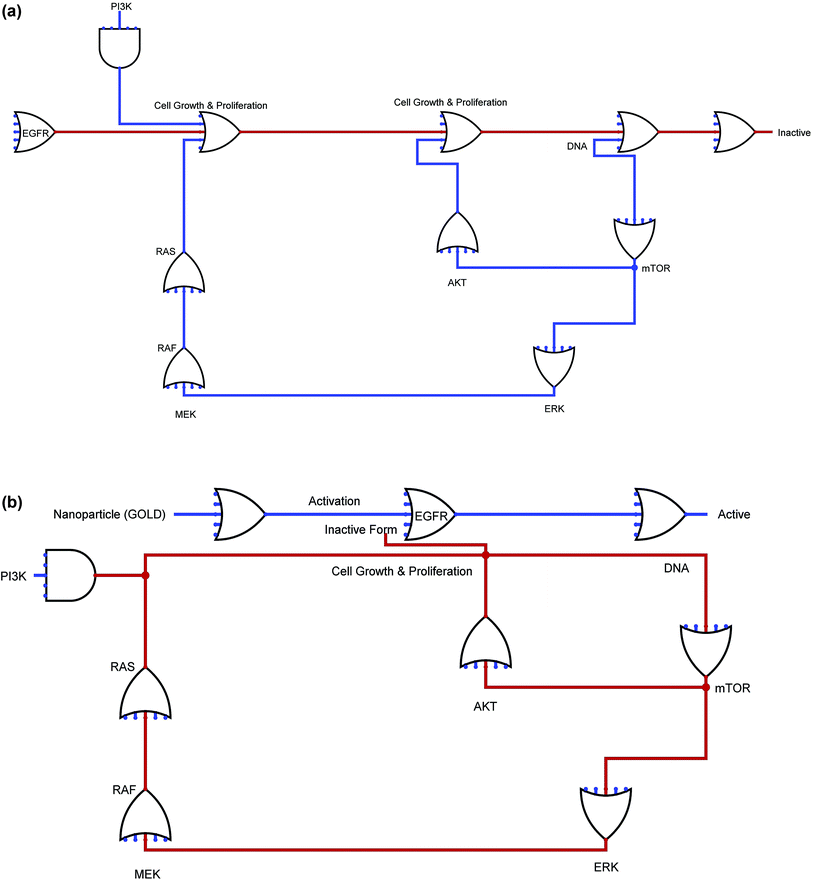 |
| Fig. 3 Figure depicts the anti-EGFR-iRGD endeavoured with NP (b) and without NP (a), anti-EGFR-iRGD with GOLD NP was to restrain the direction of malignancy where lines in figure speak to the 0 and 1 supply for each connecting entities. Blue delineate the dynamic shape and red show inert type of biological circuits. | |
4. Conclusions
Based on our research and observations, we formulated a novel anti-EGFR-iRGD-decorated erythrocyte membrane-sourced NP whose function is to be involved in targeting or drug delivery in a greatly enhanced approach to target tumors and exert antitumor effects. Anti-EGFR-iRGD-RBC-PTX is a great representation of a possible operational nanomedicine to treat gastric cancer. We use genomic pattern of EGFR from TCGA data to retrieve the mutational, expressional and clinical information; and measure the prognostic of EGFR in brain tumor. Further we were estimate the survival probability to evaluate the significance between TCGA groups. The proposed algorithm was executed for the screening of potential NPs from libraries. This approach was used to evaluate GOLD as a potential inhibitor of the anti-EGFR-iRGD protein. Furthermore, the synergistic effect of the AuNPs against the anti-EGFR-iRGD protein significantly induced the inactivity of the target protein. These results were further validated by computational approaches, such as systems biology and synthetic biology (Biological circuits). Additionally, the proposed biological routes can be developed to provide targets for sensors, which may be advantageous to further study the extracellular and intracellular signals released for the biological regulatory pathway of anti-EGFR-iRGD. This also opens up a new possibility in tumor therapy by employing synergistic inhibition of nanoparticles, such as AuNP. Cancer is a formidable disease that can only be cured with the help of the right type of anticancer medicines. Drug targeting is one of the most important aspects of the entire process. The effective delivery of drugs means fighting cancer in the affected regions. As part of our research, we created an effective drug delivery pathway that ensured a smooth and seamless delivery of anticancer drugs to the targeted tumor site, revealing the success of anti-EGFRs.
Authors contributions
Conceptualization: ACK; data curation: ACK; formal analysis: ACK, AK, XW; funding acquisition: DQW; investigation: DQW, ACK; methodology: ACK; project administration: DQW; resources: ACK; supervision: DQW; validation: DQW, ACK; writing-original draft: ACK; writing-review draft: ACK, AK, SPS, YLS, CTP, YJW, XW, DQW.
Funding
This work is supported by the grants from the Key Research Area Grant 2016YFA0501703 of the Ministry of Science and Technology of China, the National Natural Science Foundation of China (Contract no. 61832019, 61503244), the State Key Lab of Microbial Metabolism and Joint Research Funds for Medical and Engineering and Scientific Research at Shanghai Jiao Tong University (YG2017ZD14).
Conflicts of interest
We declare no competing interests.
Acknowledgements
The simulations in this work were supported by the Center for High Performance Computing, Shanghai Jiao Tong University.
References
- R. C. Taylor, BMC Bioinf., 2010, 11(suppl. 12), S1, DOI:10.1186/1471-2105-11-S12-S1.
- A. C. Kaushik and S. Sahi, Neural Comput. Appl., 2017, 28(11), 3385–3391, DOI:10.1007/s00521-016-2252-5.
- L. A. Torre, F. Bray, R. L. Siegel, J. Ferlay, J. Lortet-Tieulent and A. Jemal, Ca-Cancer J. Clin., 2015, 65(2), 87–108, DOI:10.3322/caac.21262.
- A. C. Kaushik and S. Sahi, Neural Comput. Appl., 2018, 29(4), 985–992, DOI:10.1007/s00521-016-2502-6.
- M. K. Ruhland, L. M. Coussens and S. A. Stewart, Biochim. Biophys. Acta, 2016, 1865(1), 14–22, DOI:10.1016/j.bbcan.2015.10.001.
- R. S. Herbst, Int. J. Radiat. Oncol., Biol., Phys., 2004, 59(suppl. 2), 21–26 CrossRef CAS PubMed.
- M. J. Wieduwilt and M. M. Moasser, Cell. Mol. Life Sci., 2008, 65(10), 1566–1584, DOI:10.1007/s00018-008-7440-8.
- E. Purba, E. Saita and I. Maruyama, Cells, 2017, 6(2), E13, DOI:10.3390/cells6020013.
- M. A. Lemmon and J. Schlessinger, Cell, 2010, 141(7), 1117–1134, DOI:10.1016/j.cell.2010.06.011.
- K. M. Ferguson, Annu. Rev. Biophys., 2008, 37, 353–373, DOI:10.1146/annurev.biophys.37.032807.125829.
- H. Zhang, A. Berezov, Q. Wang, G. Zhang, J. Drebin, R. Murali and M. I. Greene, J. Clin. Invest., 2007, 117(8), 2051–2058 CrossRef CAS PubMed.
- S. P. Singh, S. Urooj and A. Lay-Ekuakille, IEEE Sens. J., 2016, 16, 4847–4855 CAS.
- A. C. Kaushik, X. Mao, Y. Li, D. Q. Wei and S. Sahi, J. Mater. Res. DOI:10.1557/jmr.2018.453.
- P. Seshacharyulu, M. P. Ponnusamy, D. Haridas, M. Jain, A. K. Ganti and S. K. Batra, Expert Opin. Ther. Targets, 2012, 16(1), 15–31, DOI:10.1517/14728222.2011.648617.
- O. Shafi, BMC Neurol., 2016, 16(1), 236, DOI:10.1186/s12883-016-0765-2.
- H. Masuda, D. Zhang, C. Bartholomeusz, H. Doihara, G. N. Hortobagyi and N. T. Ueno, Breast Cancer Res. Treat., 2012, 136(2), 331–345 CrossRef CAS PubMed.
- S. T. Chien, A. Kumar, S. Pandey, C. K. Yen, S. Y. Wang, Z. H. Wen, A. C. Kaushik, Y. L. Shiue and C. T. Pan, Curr. Pharm. Des. DOI:10.2174/1381612824666181112104921.
- A. C. Kaushik, A. Kumar, V. D. Dwivedi, S. Bharadwaj, S. Kumar, K. Bharti, P. Kumar, R. K. Chaudhary and S. K. Mishra, Mol. Neurobiol., 2018, 55(4), 3224–3236, DOI:10.1007/s12035-017-0546-y.
- K. N. Sugahara, T. Teesalu, P. P. Karmali, V. R. Kotamraju, L. Agemy, D. R. Greenwald and E. Ruoslahti, Science, 2010, 328(5981), 1031–1035, DOI:10.1007/s13398-014-0173-7.2.
- D. Huang, F. Liao, S. Molesa, D. Redinger and V. Subramanian, J. Electrochem. Soc., 2003, 150(7), G412–G417, DOI:10.1149/1.1582466.
- C. Ricordel, M. Labalette-Tiercin, A. Lespagnol, M. Kerjouan, C. Dugast, J. Mosser, B. Desrues and H. Léna, Lung Cancer, 2015, 87(1), 80–84, DOI:10.1016/j.lungcan.2014.11.005.
- L. G. Ferreira, R. N. Dos Santos, G. Oliva and A. D. Andricopulo, Molecules, 2015, 20(7), 13384–13421, DOI:10.3390/molecules200713384.
- D. K. Johnson and J. Karanicolas, J. Chem. Inf. Model., 2016, 56(2), 399–411, DOI:10.1021/acs.jcim.5b00572.
- Z. Ji, K. Yan, W. Li, H. Hu and X. Zhu, BioMed Res. Int., 2017, 2017, 5958321, DOI:10.1155/2017/5958321.
- L. Lazzerini-Ospri, P. Stano, P. L. Luisi and R. Marangoni, BMC Bioinf., 2012, 13(suppl. 4), S9, DOI:10.1186/1471-2105-13-S4-S9.
- Y. Vugmeyster, World J. Biol. Chem., 2012, 3(4), 73–92, DOI:10.4331/wjbc.v3.i4.73.
- A. C. Kaushik, S. Bharadwaj, S. Kumar and D. Q. Wei, Sci. Rep., 2018, 8(1), 9169, DOI:10.1038/s41598-018-27580-1.
- P. W. Rose, C. Bi, W. F. Bluhm, C. H. Christie, D. Dimitropoulos, S. Dutta, R. K. Green, D. S. Goodsell, A. Prlić, M. Quesada, G. B. Quinn, A. G. Ramos, J. D. Westbrook, J. Young, C. Zardecki, H. M. Berman and P. E. Bourne, Nucleic Acids Res., 2013, 41, D475–D482, DOI:10.1093/nar/gks1200.
- G. M. Morris, R. Huey, W. Lindstrom, M. F. Sanner, R. K. Belew, D. S. Goodsell and A. J. Olson, J. Comput. Chem., 2009, 30(16), 2785–2791, DOI:10.1002/jcc.21256.
- X. Liu, J. Jiang, Y. Ji, J. Lu, R. Chan and H. Meng, Mol. Syst. Des. Eng., 2017, 2, 370–379 RSC.
- K. Wang, X. Zhang, Y. Liu, C. Liu, B. Jiang and Y. Jiang, Biomaterials, 2014, 35, 8735–8747 CrossRef CAS PubMed.
- C. Liu, S. Yao, X. Li, F. Wang and Y. Jiang, J. Drug Targeting, 2017, 25, 235–246 CrossRef CAS PubMed.
- Z. Zhang, H. Qian, J. Huang, H. Sha, H. Zhang, L. Yu, B. Liu, D. Hua and X. Qian, Int. J. Nanomed., 2018, 13, 4961–4975 CrossRef CAS PubMed.
- Z. Zhang, H. Qian, J. Huang, H. Sha, H. Zhang, L. Yu, B. Liu, D. Hua and X. Qian, Int. J. Nanomed., 2018, 13, 4961–4975, DOI:10.2147/IJN.S170148.
- N. L. Cianciola, D. Crooks, A. H. Shah and C. Carlin, J. Virol., 2007, 81(19), 10437–10450, DOI:10.1128/JVI.00399-07.
- M. Scaltriti and J. Baselga, Clin. Cancer Res., 2006, 12(18), 5268–5272 CrossRef CAS PubMed.
Footnotes |
† Electronic supplementary information (ESI) available. See DOI: 10.1039/c9ra01975h |
‡ These authors contributed equally. |
|
This journal is © The Royal Society of Chemistry 2019 |
Click here to see how this site uses Cookies. View our privacy policy here.