DOI:
10.1039/C9RA03479J
(Paper)
RSC Adv., 2019,
9, 21893-21902
Accumulation, temporal variation, source apportionment and risk assessment of heavy metals in agricultural soils from the middle reaches of Fenhe River basin, North China
Received
9th May 2019
, Accepted 9th July 2019
First published on 15th July 2019
Abstract
The Fenhe River basin is the main agricultural and industrial developed area in Shanxi province, China. In recent years, agricultural non-point source pollution in the Fenhe River basin intensified, threatening soil quality and safety in the area. Accumulation of eight heavy metals (HMs) including chromium (Cr), nickel (Ni), copper (Cu), zinc (Zn), arsenic (As), cadmium (Cd), lead (Pb) and mercury (Hg) has been detected in soil samples from 50 agricultural sites (0–20 cm) from the middle reaches of the Fenhe River basin. The ecological and human health risk and potential sources of the eight HMs were investigated. In addition, the human health and ecological risks imposed by the possible sources of the eight HMs were quantitatively apportioned. The enrichment factor (EF) values of Cr, Ni, Cu, Pb and Zn were lower than 2, indicating minimal enrichment, while values for As, Cd and Hg were between 2 and 5, exhibiting moderate enrichment. Temporal variation analysis suggested that most HMs in the study area exhibited low concentrations after 2015, except As. The potential ecological risk index was 174.09, indicating low ecological risk. The total hazard index and cancer risk values were 0.395 and 5.35 × 10−4 for adults and 2.75 and 3.63 × 10−4 for children, indicating the accepted standard levels were exceeded for non-carcinogenic risk for children and carcinogenic risks for both adults and children. Four potential sources were identified: (1) natural sources, (2) farming activities, (3) coal combustion, and (4) exhaust emissions. Natural sources represented the largest contributor to ecological risk, accounting for 57.42% of the total. Coal combustion was the major contributor to human health risks, accounting for 43.27% and 43.73% of the total non-carcinogenic risk and carcinogenic risk for adults, respectively, and 42.72% and 43.88% for children, respectively.
Introduction
In recent decades, large quantities of pollutants have been discharged into agricultural soil during rapid agricultural intensification, industrialization and urbanization.1,2 Among these pollutants, heavy metal (HM) pollution in agricultural soils has received increasing attention, not only because of the high toxicity of HMs to plants and microorganisms,3 but also because of the persistence of HMs in ecosystems.4,5 For example, HMs can destroy microorganism population structure even at low levels.6 HMs are harmful to metabolism and intelligence in humans if the amount of inhalation exceeds the values recommended by the World Health Organization.7,8
Ecological and human health risk evaluation methods have been widely used to assess the potential risks of HMs to ecosystems and human beings.9 Ecological risk methods have been developed based on the toxicity variance and comprehensive effect of multiple contaminants, such as potential ecological risk index (RI),10,11 soil environment quality standard,10 geoaccumulation index,12 pollution load index13 and enrichment factor (EF).14 The health risk caused by HMs is usually evaluated by hazard quotient and cancer risk.8,15 A combination of these ecological and health risk assessment methods can be used to produce a suitably accurate and comprehensive evaluation for HMs in agricultural soils.
In addition to risk assessment, identification of HM sources is also important as the information can be used to effectively control HM pollution. In agricultural soil, natural and anthropogenic sources have been identified as the main two pathways of HM contamination.16,17 The main natural source of HMs is their release into the soil during rock weathering.18 Anthropogenic sources are the important aspect of soil HM pollution and may include mining,19 fossil fuel combustion,20 waste disposal,21 sewage irrigation,22 urban effluent,23 vehicle exhausts,24 atmospheric deposition,10 pesticides and fertilizer application.25,26 For example, the long-term use of pesticides and chemical fertilizers has resulted in accumulation of several metals, such as nickel (Ni), copper (Cu), zinc (Zn) and cadmium (Cd). Quantitative apportioning of sources could help us to understand the contribution of each source and implement effective control measures to reduce further entry of HMs into soil.27 Different sources may have different risks and toxicities of released HMs to soil break because of the different composition of HMs in the source. Thus, the major contributor to HM concentration may not be the largest contributor to ecological and health risk, and thus analysis of the ecological and human health risk following exposure to each source is needed. Previous studies have only focused on the contribution of HM sources to health risk.28–35 However, exposure of both ecosystem and human beings to HMs can be harmful,36,37 and thus evaluation of the contributions of different HM sources to ecological and human health risks is important but has rarely been explored in previous studies.
The Fenhe River basin covers a quarter of the total area of Shanxi province, and is the main agricultural and industrial developed area of the province. This area accounts for 64% of the agricultural output of the whole province. As an important heavy industrial area in China, the four basic industries in Shanxi province are those of machinery, metallurgy, coal and chemical. Currently, the problem of agricultural non-point pollution in Fenhe River basin has become serious,38 and threatens the soil quality of the Fenhe River basin and the safety of the surrounding environment. The pollution, especially the agricultural soil pollution, has attracted considerable attention. The objectives of the present study are as follows: (1) to investigate the accumulation and temporal variation of the eight HMs in agricultural soils from the middle reaches of the Fenhe River basin; (2) to assess ecological and human health risks of these HMs in the investigated area using RI and the carcinogenic and non-carcinogenic risk assessment method; (3) to identify potential sources of the HMs by cluster analysis and principal component analysis; and (4) to analyse the ecological and human health risks caused by the possible sources using factor analysis-multiple linear regression (FA-MLR).
Materials and methods
Sampling collection and analysis
The study area is located in the middle reaches of Fenhe River basin (Taiyuan city to Huozhou county of Linfen district), Shanxi province, China (Fig. 1). Fifty agricultural soil samples (0–20 cm sampling depth) were collected in July 2017 during the crop-growing season. Each sample was bulked from five subsamples, which were randomly taken approximately 20 m apart. All the soil samples were packed into self-styled bags for return to the laboratory. Soil samples were air-dried prior to further analysis.
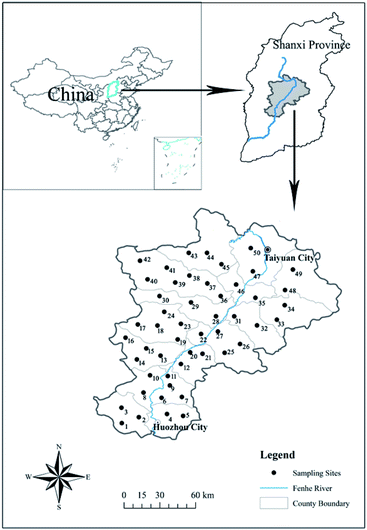 |
| Fig. 1 Sketch map of sampling sites. | |
To measure the HM concentrations, samples were weighed and sieved through a 0.15 mm sieve. First, 0.10–0.15 g of the sieved soil samples was transferred into Teflon tubes, and then completely digested by HNO3–HCl–HF in a microwave digestion meter. After digestion, the solutions cooled to ambient temperature and then the solution was moved to a volumetric flask and deionized water was added to dilute the solution to 50 ml. The mercury (Hg) content was analysed by atomic fluorescence spectrometry (FS5, Edinburgh instruments, UK) and the chromium (Cr), Ni, Cu, Zn, arsenic (As), Cd and lead (Pb) contents were detected by inductively coupled plasma mass spectrometry (ICP-MS iCAP QC, Thermo, USA). In order to determine the contents of elements of Mn, Fe and Al, another 0.10–0.15 g soil samples were digested by HNO3–HClO4–HF, after digestion, concentrations of Mn, Fe and Al were detected by ICP-MS (iCAP QC, Thermo, USA).
Standard substances (GBW07401) and reagent blanks were used for quality assurance and quality control after every tenth sample. Recoveries of the standard substances were 90–113% for eight metals and reference elements, which meet the experiment requirements. All digestions and metal analyses were performed in triplicate. The limit of detection (LOD) and limit of quantification (LOQ) were defined as 3 times and 10 times the standard deviation of 15 blank measurements. The LODs for Cr, Ni, Cu, Zn, As, Cd, Hg and Pb were 0.040 mg kg−1, 0.019 mg kg−1, 0.022 mg kg−1, 0.030 mg kg−1, 0.012 mg kg−1, 0.100 μg kg−1, 0.040 μg kg−1 and 0.020 mg kg−1, respectively. The LOQs for Cr, Ni, Cu, Zn, As, Cd, Hg and Pb were 0.130 mg kg−1, 0.061 mg kg−1, 0.072 mg kg−1, 0.100 mg kg−1, 0.040 mg kg−1, 0.130 μg kg−1, 0.020 μg kg−1 and 0.070 mg kg−1, respectively. Heavy metals and Mn, Fe, Al standard solutions (Sinopharm Chemical Reagent, Beijing, China) were used to construct the standard curves, correlation coefficient of standard curve range from 0.9990–0.9999.
Enrichment factor (EF) method
EF is a common parameter for assessing the HM enrichment level and evaluating the degree of anthropogenic influence on HMs.39 The EFs are calculated based on a reference element for normalization.40,41 Usually, stable elements that have relatively high contents in the crust (e.g., Mn, Ti, Al and Fe) are chosen as ref. 42 and 43. Because of the relatively high concentration and stability in the study area, Mn was selected as the reference element in the current study (Table 1).28,44 The EFs were determined through eqn (1): |
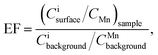 | (1) |
where, Cisurface and CMn are the concentrations of the eight HMs and Mn in agricultural soil (Table 1), and Cibackground and CMnbackground are the background values of the eight HMs and Mn in the soil of Shanxi province (Table 1). The EF values were classified into five grades, presented in Table 2.45
Table 1 Concentrations of elements (g kg−1 for Fe and Al, mg kg−1for other elements) in agricultural soils of the middle reaches of Fenhe River basina
|
Cr |
Ni |
Cu |
Zn |
As |
Cd |
Pb |
Hg |
Fe |
Mn |
Al |
Range – the difference between the maximum and the minimum; S.D. – standard deviation; CV – coefficient of variation; BV – the background values of soils in Shanxi province.46 Standard values for heavy metals in agricultural soil in China, 1995.47 |
Max |
96.58 |
45.57 |
40.34 |
136.32 |
68.00 |
0.39 |
37.33 |
0.13 |
1.89 |
684.37 |
15.27 |
Min |
38.25 |
19.98 |
13.99 |
48.76 |
9.10 |
0.09 |
14.70 |
0.02 |
0.75 |
248.26 |
2.34 |
Mean |
51.49 |
27.53 |
21.53 |
72.32 |
15.81 |
0.19 |
22.09 |
0.05 |
1.25 |
428.93 |
6.18 |
Median |
50.58 |
26.52 |
21.09 |
69.13 |
14.13 |
0.19 |
22.14 |
0.04 |
1.24 |
393.39 |
5.73 |
S.D. |
10.15 |
5.88 |
5.32 |
17.56 |
8.41 |
0.06 |
3.88 |
0.03 |
0.36 |
115.98 |
2.68 |
CV |
0.20 |
0.21 |
0.25 |
0.24 |
0.53 |
0.32 |
0.18 |
0.60 |
0.29 |
0.27 |
0.43 |
BV |
55.30 |
29.90 |
22.90 |
63.50 |
9.10 |
0.10 |
14.70 |
0.02 |
3.00 |
532 |
6.40 |
Grade Ib |
90.00 |
40.00 |
150.00 |
100.00 |
NA |
0.20 |
35.00 |
0.15 |
NA |
NA |
NA |
Grade IIb |
250.00 |
40.00 |
150.00 |
200.00 |
NA |
0.30 |
250.00 |
0.30 |
NA |
NA |
NA |
Table 2 Classification of enrichment factor (EF) and potential ecological risk (RI) index
Index |
Category |
Risk level for EF |
EF45 |
EF < 2 |
Minimal enrichment |
2 ≤ EF < 5 |
Moderate enrichment |
5 ≤ EF < 20 |
Significant enrichment |
20 ≤ EF < 40 |
Very high enrichment |
EF > 40 |
Extremely high enrichment |
|
Category |
Risk level for Eif |
Category |
Risk level for RI |
RI14 |
Eif < 40 |
Low risk |
RI < 150 |
Low risk |
40 ≤ Eif < 80 |
Moderate risk |
150 ≤ RI < 300 |
Moderate risk |
80 ≤ Eif < 160 |
Considerable risk |
300 ≤ RI < 600 |
Considerable risk |
160 ≤ Eif < 320 |
High risk |
RI ≥ 600 |
High risk |
Eif ≥ 320 |
Very high risk |
Ecological risk assessment method
The RI is a comprehensive method to evaluate the multiple elements in soil because it considers both the background values and toxicity of the HMs.48 The RI was defined using eqn (2)–(4):14 |
Cif = Cisurface/Cibackground,
| (2) |
|
 | (4) |
where Cif is the contamination factor; Tif is the toxic response factor, taking values of 40, 30, 10, 5, 5, 5, 2 and 1 for Hg, Cd, As, Ni, Cu, Pb, Cr and Zn, respectively.14 Categories of Eif and RI are presented in Table 2.14
Human health risk assessment method
Human health risk was applied to evaluate the possible risk of the HMs, including non-carcinogenic risk and carcinogenic risk.49 According to the model, average daily intake (ADI) caused through exposure pathways for Cr, Ni, Cu, Zn, As, Cd and Pb: direct soil ingestion, inhalation through soil vapour and dermal contact,50 ADI were determined through eqn (5)–(7): |
 | (5) |
|
 | (6) |
|
 | (7) |
where RIngestion is the ingestion rate (50 mg per day for adults and 87 mg per day for children);51,52 BW is the average body weight (64 kg for adults and 22.3 kg for children); ED is the exposure duration (24 years for adults and 2 years for children); ExF is the exposure frequency (350 days year−1);50 AT average life span (ED × 365 days);50 RInhalation is the inhalation rate (16.1 m3 per day for adults and 8.5 m3 per day for children); PEF is the particle emission factor (1.32 × 109 m3 kg−1);50 SA is the skin area available for soil contact (5700 cm2 per day for adults and 2800 cm2 per day for children);50 AF is the skin adherence factor (2 × 10−7 kg cm−2 for adults and 10−6 kg cm−2 for children);50 and ABF is the dermal adsorption factor (0.1).53
Mercury is appreciably volatile even at room temperature and health risk exposure to soil Hg can occur not only through the three pathways mentioned above, but also through inhalation of Hg vapor (ADIvapor), which can be calculated as eqn (8):54,55
|
 | (8) |
where VF is the volatilization factor (32
![[thin space (1/6-em)]](https://www.rsc.org/images/entities/char_2009.gif)
675.6 m
3 kg
−1).
54
The non-carcinogenic risk (HI) was then calculated via eqn (9) and (10):53
|
 | (9) |
where HQ
i is the non-carcinogenic risk of one exposure pathway
15 and R
fD is the recommended dose (
Table 3).
56 If HI > 1, the HMs are likely to contribute to non-carcinogenic risks, where as HI < 1 indicates no non-carcinogenic risk.
15
Table 3 Reference dose (RfD; mg per kg per day) and cancer slope factor (CSF0; kg day mg−1) of HMs
metals |
Ingestion RfD61–63 |
Dermal RfD61–63 |
Inhalation RfD61–63 |
Ingestion CSF061–63 |
Dermal CSF061–63 |
Inhalation CSF061–63 |
NA means not available. |
Cu |
0.04 |
0.012 |
0.012 |
NAa |
NA |
NA |
Zn |
0.30 |
0.06 |
0.30 |
NA |
NA |
NA |
Cr |
0.003 |
0.003 |
0.000029 |
0.50 |
2.00 |
4.20 |
Ni |
0.02 |
0.0054 |
0.0206 |
1.70 |
4.25 |
0.90 |
As |
0.0003 |
0.00012 |
0.0003 |
1.50 |
3.66 |
1.51 |
Cd |
0.001 |
0.00003 |
0.000057 |
NA |
NA |
6.30 |
Pb |
0.0015 (adults) |
0.00053 |
0.0035 |
NA |
NA |
NA |
0.00005 (children) |
Hg |
0.00016 |
0.000096 |
0.00011 |
NA |
NA |
NA |
The carcinogenic risk (CR) was defined using eqn (10) and (11):57
where CSF
0 is the carcinogenic slope factor for each metal (
Table 3).
56 A CR higher than 10
−4 indicates a potential carcinogenic risk, a CR value between 10
−6 and 10
−4 means an acceptable or tolerable carcinogenic risk, and a CR value lower than 10
−6 indicates no carcinogenic risk.
56
Source apportionment methods
Principal component analysis (PCA) and cluster analysis (CA), two traditional statistical methods, were used to determine potential sources of the eight HMs. The two models for source identification have proven to be useful tools and are used widely for source apportionment of pollutants in sediments,18 soils,40,41,58 dusts20 and air.59 Correlation between HMs in factors can be obtained by factor analysis. The rotation of principal components was implemented following to the varimax method. The principal components were chosen based on the standard of cumulative variance greater than 80%, with eigenvalues greater than 1.0.60 CA involves grouping sets of objects into multiple classes composed of similar objects. Each group is likely to represent a single source type. To ensure that the data conformed to the normal distribution, a logarithmic transformation was carried out before PCA and CA.41 Multiple linear regression method with factor analysis (FA-MLR) based on PCA was used to quantify the source contributions to ecological and human risks. The extracted principal components were taken as variables and RI, HI and HQ values were taken as independent variables for regression analysis. The contribution of each principal component to RI, HI and HQ was then calculated. SPSS software v.23.0 was used for the PCA, CA and FA-MLR.
Results and discussion
Accumulation of HMs in agricultural soils
The HM concentrations in agricultural soils from the investigated area are presented in Table 1. The average concentrations of Zn, Cr, Ni, Pb, Cu, As, Cd and Hg were 72.32, 51.45, 27.53, 22.09, 21.53, 15.81, 0.19 and 0.05 mg kg−1, respectively. Zinc, Cd, Pb, Hg and As showed mean values greater than their corresponding background values for Shanxi province (Table 1), especially for Cd and Hg, which were 2 and 2.5 times greater than their corresponding background values. However, concentrations of Cr exceeded the background values for Shanxi province at several sampling sites (Table 1). Fortunately, the average concentrations of the eight HMs were all lower than the Chinese soil quality standard (Grade II), which could be employed to protect agricultural production and human health.
Comparison with studies from other regions in China as presented in Table 4 revealed that HMs in our study area had low levels. These findings indicate that long-term industrialization and agricultural intensification have resulted in the enrichment of HMs in agricultural soils. For example, developed regions in China (Nanjing, Shenzhen, Tianjin and Hunan provinces) had high levels of Cr, Zn, Cd, Pb, Hg and As. Table 5 presents the temporal variation of concentrations for eight HMs from 2003 to 2017 in the middle reaches of the Fenhe River basin. The contents of most HMs (except As) exhibited an increasing trend before 2015 (Table 5). Lower values of HMs (except As) were observed in 2017, which may indicate benefits from the government's curb on environmental pollution on the Fenhe River basin, such as changed from coal combustion to gas heating, sewage purification and vehicle restrictions. There was a slightly higher concentration of As in 2017 than it used to be, indicating that a source of As in the study area is not well controlled.
Table 4 Average concentrations of heavy metals in the agricultural soils in China (mg kg−1)
Region |
Cr |
Ni |
Cu |
Zn |
As |
Cd |
Pb |
Hg |
Reference |
Sihui city, Pearl River Delta |
|
14.7 |
16.6 |
|
12.0 |
|
31.2 |
0.13 |
64 |
Shunde city, Pearl River Delta |
|
35.4 |
44.2 |
|
21.6 |
|
48.3 |
0.45 |
64 |
Baiyin city, Gansu province |
49.52 |
33.61 |
59.64 |
279 |
|
|
37.48 |
|
65 |
Hunan province |
74.96 |
26.83 |
38.85 |
147.28 |
21.05 |
0.85 |
56.06 |
0.25 |
66 |
Tianjin city |
91.21 |
64.19 |
41.17 |
276.41 |
17.72 |
0.85 |
41.56 |
1.72 |
67 |
Chongqing city |
75.89 |
35.57 |
27.08 |
88.53 |
6.30 |
0.34 |
28.06 |
0.08 |
68 |
Gongzhuling, Jilin province |
53.04 |
27.16 |
19.61 |
57.82 |
|
0.11 |
28.34 |
|
69 |
Hexi Corridor |
97.51 |
47.42 |
35.20 |
|
75.34 |
|
5.54 |
|
70 |
Nan Jing, Jiangsu province |
97 |
|
44.8 |
119 |
11.2 |
0.31 |
35.6 |
0.08 |
33 |
Shenzhen city |
49.70 |
|
69.87 |
323.47 |
|
8.74 |
121.54 |
|
71 |
The middle reaches of Fenhe River basin |
51.45 |
27.53 |
21.53 |
72.32 |
15.81 |
0.19 |
22.09 |
0.05 |
This study |
Average of China |
61 |
27 |
23 |
74 |
11 |
0.097 |
27 |
— |
72 |
Table 5 HMs concentrations in agricultural soils of the study area from 2003 to 2017 (mg kg−1)
Time |
Cr |
Ni |
Cu |
Zn |
As |
Cd |
Pb |
Hg |
Reference |
2003 |
|
|
|
|
|
0.27 |
26.84 |
|
73 |
2006 |
57.84 |
|
|
|
|
|
|
0.087 |
74 |
2010 |
74.19 |
31.35 |
26.38 |
230.4 |
11.64 |
0.30 |
20.30 |
0.091 |
75 and 76 |
2011 |
75.4 |
26.6 |
21.8 |
60.6 |
10.7 |
0.11 |
|
0.041 |
77 |
2012 |
71 |
32 |
30 |
82 |
14 |
0.2 |
23 |
0.13 |
58 |
2013 |
74.1 |
29.74 |
32.11 |
90.76 |
10.7 |
0.25 |
27.87 |
0.09 |
78 |
2014 |
31.57 |
26.22 |
30.91 |
71.33 |
9.5 |
0.33 |
34.46 |
0.11 |
79 |
2015 |
80.88 |
39.65 |
28.54 |
101.7 |
10.35 |
0.77 |
1099 |
0.13 |
80,81 |
2017 |
51.45 |
27.53 |
21.53 |
72.32 |
15.81 |
0.19 |
22.09 |
0.05 |
This study |
Because the EF provides standardized values, it can be used to easily compare pollution levels between different HMs. According to the enrichment categories recognized based on the EF values, As, Cd and Hg showed moderate enrichment, with EF values of 2.37, 2.56 and 3.23, respectively (Table 6). However, Cr, Ni, Cu, Zn and Pb exhibited minimal enrichment with EF values lower than 2 (Table 6). In addition, EF values can be used in the assessment of HM sources. An EF value < 1.5 suggests that the HM may be from natural sources, whereas EF values > 1.5 suggest HM contamination from anthropogenic sources.64 In our study, EF values for Zn, As, Cd, Hg and Pb exceeded 1.5, suggesting that these HMs were mainly from anthropogenic activities. Although the mean EF values were lower than 1.5, EF values of 30% of all sampling sites for Cr exceeded 1.5 (Table 6), and thus Cr in soil of these sampling sites was probably influenced by human beings.
Table 6 Data characteristic of EF values
|
EF values |
Numbers of sampling sites of EF values in different categories |
EF < 2 |
2 ≤ EF < 5 |
5 ≤ EF < 20 |
EF > 1.5 |
Mean |
Range |
N |
% of the total |
N |
% of the total |
N |
% of the total |
N |
% of the total |
Cr |
1.21 |
0.71–2.25 |
48 |
96 |
2 |
4 |
0 |
0 |
15 |
30 |
Ni |
1.24 |
0.67–2.61 |
47 |
94 |
3 |
6 |
0 |
0 |
2 |
4 |
Cu |
1.26 |
0.62–2.72 |
44 |
88 |
6 |
12 |
0 |
0 |
5 |
10 |
Zn |
1.51 |
0.71–2.95 |
42 |
84 |
8 |
16 |
0 |
0 |
27 |
54 |
As |
2.37 |
1.02–13.50 |
23 |
46 |
26 |
52 |
1 |
2 |
40 |
80 |
Cd |
2.56 |
0.88–5.99 |
17 |
34 |
30 |
60 |
3 |
6 |
41 |
82 |
Pb |
1.99 |
1.02–3.92 |
27 |
54 |
23 |
46 |
0 |
0 |
40 |
80 |
Hg |
3.23 |
0.75–8.73 |
16 |
32 |
28 |
56 |
6 |
12 |
45 |
90 |
Ecological and health risk assessment
The mean Eif values for the eight HMs decreased in the following order (Fig. 2): Hg (80.87) > Cd (56.03) > As (17.37) > Pb (7.51) > Cu (4.70) > Ni (4.60) > Cr (1.86) > Zn (1.14) (Fig. 2). According to Table 2, As, Pb, Cu, Ni, Cr and Zn exhibited a low ecological risk to the environment, with Eif values lower than 40. However, the Eif values for Cd exceeded 40 and Hg exceeded 80, indicating Cd and Hg had moderate and considerable ecological risks. According to Fig. 2, Cd and Hg were the two main HMs causing ecological risk in agricultural soils from the middle reaches of Fenhe River basin. The RI value ranged from 88.22 to 387.70, with an average value of 174.09, representing moderate ecological risks. The Eif values of Cd and Hg contributed substantially to the RI value, which was consistent with previous studies for agricultural soil in other areas of China.10
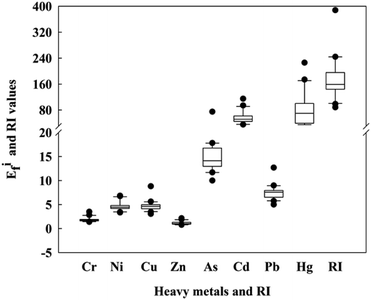 |
| Fig. 2 Potential ecological risk index (RI) of heavy metals in agricultural soils from the middle reaches of Fenhe River basin. | |
The potential human health risk for adults (aged >18) and children (aged 1–18) for the eight HMs through the three pathways (ingestion, inhalation and dermal) are shown in Table 7. The total HI values were 0.395 and 2.75 for adults and children, respectively. Values for children greater than 1 indicate a non-carcinogenic risk for exposure to HMs in the study area. The HQ values presented a decreasing trend for both adults and children as follows: As > Pb > Cr > Cd > Ni > Hg > Cu > Zn. Arsenic and Pb were the main metals associated with human non-carcinogenic risks in the study area. Except for Hg, direct dermal contact was the main exposure pathways for most HMs, representing about 68.8–98.7% of the corresponding total HI values for adults and 76.1–99.1% for children. Dermal contact was also the primary exposure pathway of Hg for children, whereas the non-carcinogenic risk posed to adults was dominated by inhalation of Hg vapor. It should be noted that inhalation of Hg vapor has not been considered in most studies, which may cause the non-carcinogenic risk to have been under estimated.28,33,82 The order of contributions of the three pathways was the same as in previous studies.28,55,82,83 It is worth noting that children were 6.96 times more likely to experience non-carcinogenic effects than adults, which is probably because of the direct hand-to-mouth contact and higher exposure per unit of body weight in children.
Table 7 Non-carcinogenic risks and carcinogenic risks results of each metal
|
Non-carcinogenic risks for adults (aged >18) |
Carcinogenic risks for adults (aged >18) |
HQIngeastion |
HQInhalation |
HQDermal |
HQvapor |
HI |
CRIngestion |
CRInhalation |
CRDermal |
CR |
Cr |
1.28 × 10−2 |
4.28 × 10−4 |
2.93 × 10−2 |
— |
4.26 × 10−2 |
1.93 × 10−5 |
5.21 × 10−8 |
1.76 × 10−4 |
1.95 × 10−4 |
Ni |
1.03 × 10−3 |
3.22 × 10−7 |
8.71 × 10−3 |
— |
9.74 × 10−3 |
3.51 × 10−5 |
5.98 × 10−9 |
2.00 × 10−4 |
2.35 × 10−4 |
Cu |
4.03 × 10−4 |
4.33 × 10−7 |
3.06 × 10−3 |
— |
3.47 × 10−3 |
— |
— |
— |
|
Zn |
1.81 × 10−4 |
5.81 × 10−8 |
2.06 × 10−3 |
— |
2.24 × 10−3 |
— |
— |
— |
|
As |
3.57 × 10−2 |
1.15 × 10−5 |
0.204 |
— |
0.239 |
1.61 × 10−5 |
5.18 × 10−9 |
8.94 × 10−5 |
1.06 × 10−4 |
Cd |
1.43 × 10−4 |
8.06 × 10−7 |
1.08 × 10−2 |
— |
1.10 × 10−2 |
— |
2.90 × 10−10 |
— |
2.90 × 10−10 |
Pb |
1.18 × 10−2 |
1.52 × 10−6 |
7.12 × 10−2 |
— |
8.30 × 10−2 |
— |
— |
— |
— |
Hg |
2.18 × 10−4 |
1.02 × 10−7 |
8.27 × 10−4 |
3.12 × 10−3 |
4.17 × 10−3 |
— |
— |
— |
— |
Total |
6.24 × 10−2 |
4.43 × 10−4 |
0.330 |
3.12 × 10−3 |
0.395 |
7.04 × 10−5 |
6.36 × 10−8 |
4.65 × 10−4 |
5.35 × 10−4 |
|
Non-carcinogenic risks for children (aged 1–18) |
Carcinogenic risks for children (aged 1–18) |
HQIngeastion |
HQInhalation |
HQDermal |
HQvapor |
HI |
CRIngestion |
CRInhalation |
CRDermal |
CR |
Cr |
6.42 × 10−2 |
8.56 × 10−4 |
0.206 |
— |
0.271 |
9.62 × 10−5 |
1.04 × 10−7 |
1.24 × 10−3 |
1.95 × 10−4 |
Ni |
5.15 × 10−3 |
6.45 × 10−7 |
6.14 × 10−2 |
— |
6.65 × 10−2 |
1.75 × 10−4 |
1.20 × 10−8 |
1.41 × 10−3 |
2.35 × 10−4 |
Cu |
2.01 × 10−3 |
8.66 × 10−7 |
2.16 × 10−2 |
— |
2.36 × 10−2 |
— |
— |
— |
— |
Zn |
9.02 × 10−4 |
1.16 × 10−7 |
1.45 × 10−2 |
— |
1.54 × 10−2 |
— |
— |
— |
— |
As |
0.178 |
2.30 × 10−5 |
1.44 |
— |
1.61 |
8.03 × 10−5 |
1.04 × 10−8 |
6.31 × 10−4 |
1.06 × 10−4 |
Cd |
7.13 × 10−4 |
1.16 × 10−6 |
7.65 × 10−2 |
— |
7.72 × 10−2 |
— |
5.79 × 10−10 |
— |
2.90 × 10−10 |
Pb |
0.165 |
3.04 × 10−6 |
0.502 |
— |
0.667 |
— |
— |
— |
— |
Hg |
1.09 × 10−3 |
2.04 × 10−7 |
5.83 × 10−3 |
4.73 × 10−3 |
1.17 × 10−2 |
— |
— |
— |
— |
Total |
0.311 |
8.85 × 10−4 |
2.32 |
4.73 × 10−3 |
2.75 |
3.52 × 10−4 |
1.27 × 10−7 |
3.28 × 10−3 |
3.63 × 10−3 |
The CR exposures for adults and children to Cr, Ni, As and Cd were assessed and the results are presented in Table 7. The values for Cu, Zn, Pb and Hg were excluded because of the lack of CSF0 values. The total CR values for adults and children were 5.35 × 10−4 and 3.63 × 10−4, respectively, which were both higher than the maximum tolerable or acceptable risk (1 × 10−4). Similar to the non-carcinogenic risk, children had a higher carcinogenic risk than adults. The CR values for adults and children for the four metals decreased in the order Ni > Cr > As > Cd. Nickel had the highest CR value and Cd had the lowest, which is different from the trend of HI values. The single CR value for each of Ni, Cr and As exceeded 1 × 10−4, indicating an unacceptable carcinogenic risk. However, Cd showed no carcinogenic risk to the public, because its CR value was less than 1 × 10−6. Although Cr and Ni had mean concentrations less than their corresponding background values and low ecological risks, they could still cause carcinogenic risks. This suggests that specific metals with low concentration should also receive more attention because the health risk posed by them cannot be ignored.
Source identification of heavy metals in agricultural soils
There were no obvious point pollution sources in the investigated region, thus HMs may originate from sources such as chemical fertilizers and pesticides, atmospheric deposition and sewage irrigation.
Table 8 shows the PCA results for the HM concentrations. Four main components with eigenvalue > 1.0 were selected, which explained 81.42% of the total variance, indicating that the eight HMs can be presented by four factors. Factor 1 was highly correlated with Cr, Ni, Cu and Zn, and presented 42.06% of the total variance; Factor 2, representing 15.50% of the total variance, was positively correlated with Cr and Zn; Factor 3, explaining 12.37% of the total variance, had high loadings of As; and Factor 4, explaining for 10.17% of the total variance, and was highly associated with Cd, Pb and Hg.
Table 8 Principle component analysis results of eight HMs in agricultural soils of the middle reaches of Fenhe River basin
Elements |
Component |
1 |
2 |
3 |
4 |
Cr |
0.66 |
0.51 |
−0.5 |
−0.24 |
Ni |
0.57 |
−0.30 |
0.27 |
−0.44 |
Cu |
0.63 |
−0.21 |
0.22 |
0.37 |
Zn |
0.74 |
0.53 |
−0.16 |
0.10 |
As |
0.17 |
0.34 |
0.62 |
0.16 |
Cd |
0.48 |
−0.27 |
0.26 |
0.52 |
Pb |
−0.31 |
−0.05 |
−0.05 |
0.81 |
Hg |
0.48 |
−0.22 |
−0.22 |
0.65 |
Eigenvalue |
2.92 |
2.41 |
1.29 |
1.01 |
% of variance |
42.06 |
15.50 |
12.37 |
10.17 |
% of accumulative |
42.06 |
62.00 |
75.75 |
81.42 |
CA was carried out to confirm the sources of HMs and shown as a dendrogram in Fig. 3. Fig. 3 showed that the eight HMs were clustered into four groups, which was consistent with the PCA results: (1) Cd, Hg and Pb; (2) As; (3) Cr, Ni, Cu and Zn; (4) Cr and Zn.
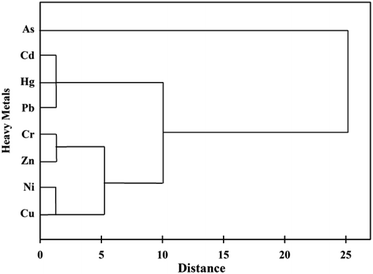 |
| Fig. 3 Dendrogram results of cluster analysis based on concentrations for eight heavy metals. | |
According to the PCA and CA results, four main factors contributed to the accumulation of the eight HMs. The first factor grouped Cr, Ni, Cu and Zn, which can be called the natural factor because considerable research has reported that Cr and Zn derived from weathering of parent materials.18 The Cr, Ni and Cu concentrations were lower than the background values for Shanxi province.
The second factor was highly associated with Cu, Zn, Cd and Hg. In China, irrigation sewage consistently contains high levels of Cr and Zn.22 The sewage, including domestic sewage and industrial sewage (such as effluents from thermal power plants, chemical plants and paper mills) is discharged into Fenhe River, which is used to irrigate farmland. According to statistical data from the government, Fenhe River accepted 335.41 million tons of sewage every year, including 117.96 million tons of industrial sewage, 81.17 million tons of domestic sewage and 136.28 million tons of mixed sewage.84 Thus, sewage irrigation can be designated as the major source of HM pollution of Cr and Zn in agricultural soil. Usually, fertilizers contain high levels of Cr and Zn, especially animal manures.85 The Cr and Zn contents in fowl manure were 1.18–314.28 mg kg−1 and 56.38–981.56 mg kg−1, respectively.85 Therefore, another important source presented in Factor 2 is likely to be the application of livestock and poultry manure. According to the China Statistical Yearbook 2017,86 the amount of chemical fertilizers applied in Shanxi province in 2015 was as high as 112.00 million tons, and has exhibited a growing trend between 2003 and 2017. Long-term and excessive use of fertilizers has resulted in the accumulation of Cr and Zn in agricultural soils. Although the overall average Cr concentration in soil of the studied area was lower than the background value, concentrations of Cr in 12 of the sampling sites were higher than the background values. In addition, EF values of Cr exceeded 1.5 in 30% of the total sampling sites (Table 6), indicating Cr in some sampling sites comes from anthropogenic sources. Thus, farming activities including sewage irrigation and chemical fertilizers were represented by the second factor.
The third factor was mainly related to As concentrations. Arsenic is easily generated during coal combustion.87 As China's top province for coal production, coal is also the main fuel in Shanxi province. The amount of coal consumed in 2016 was 356.21 million tons according to the China Statistical Yearbook 2016.88 Fly ash containing As could be produced by coal combustion, introduced into the atmosphere, and deposited onto agricultural soils.89 Therefore, coal combustion source is likely to be represented by the third factor.
The last factor mainly included Cd, Pb and Hg. Cd, Pb and Hg are the dominant HM components of vehicle tail gas emissions.90 The study area is within an area of rapid agricultural development, which involved rapid agricultural mechanization in which agricultural machinery powered by gasoline and diesel were used to improve agricultural productivity. Exhaust emissions from agricultural machinery entering the agricultural soil through atmospheric deposition could result in soil Pb and Hg accumulation. It is also worth mentioning that vehicle exhaust also contributed to the increase of Pb and Hg contents in agricultural soil. Therefore, the final factor was related to exhaust emissions.
Source apportionment of ecological and human health risks
Because the eight HMs derived from various sources that are likely to exhibit different ecological and health risks, the source apportionment to ecological and health risk is important for environment management. After identifying the possible sources of the eight HMs, source contributions for HM ecological and human health risks were computed using FA-MLR. FA-MLR is a practical method for quantifying contributions from different sources.91 The multiple correlation coefficients R2 for RI, HQ and CR were between 0.83 and 0.99 and the ratio between the estimated and observed values (E/O) was around 1.0 for RI, HQ and CR, indicating that results gathered from FA-MLR are acceptable.92
Estimated values and contribution source apportionment of ecological and health risks are exhibited in Table 9 and Fig. 4. For ecological risk assessment, Factors 1–4 accounted for 57.42%, 5.57%, 9.01% and 28.00% of the RI, respectively. Source apportionment of health risk assessment showed that for adults, Factors 1–4 accounted for 13.65%, 15.47%, 43.27% and 27.61% of the non-carcinogenic risks, respectively, and 22.82%, 26.44%, 43.73% and 7.01% of the CR, respectively. For children, Factors 1–4 accounted for 13.86%, 13.80%, 42.72% and 29.62% of the HQ, respectively, and 22.48%, 26.51%, 43.88% and 7.13% of the carcinogenic risks, respectively. Farming activities, which also represented the main factor explaining the total variance (42.06%) caused the greatest RI. Factor 3, coal combustion, which was only third in interpretation of the total variance (12.37%), was the major contributor to CR and HQ. Thus, human health and the ecological environment are unlikely to be protected if the pollution source emissions cannot be controlled. Fortunately, the method for heating in some regions of Shanxi province has changed from coal combustion to gas heating, which should reduce the emission of HMs. In addition, some biological control measures have been taken in agriculture in the Fenhe River basin to reduce the use of fertilizers and pesticides.
Table 9 Estimate values of ecological, non-carcinogenic and carcinogenic risks for the apportioned sources
|
Farming activities |
Natural source |
Coal combustion |
Exhaust emission |
Total risk |
HI for adults |
5.37 × 10−2 |
6.12 × 10−2 |
1.71 × 10−1 |
1.09 × 10−1 |
3.95 × 10−1 |
HI for children |
3.67 × 10−1 |
3.64 × 10−1 |
1.13 |
7.81 × 10−1 |
2.64 |
CR for adults |
1.22 × 10−4 |
1.41 × 10−4 |
2.34 × 10−4 |
3.75 × 10−5 |
5.35 × 10−4 |
CR for children |
8.16 × 10−4 |
9.60 × 10−4 |
1.59 × 10−3 |
2.59 × 10−4 |
3.63 × 10−3 |
RI |
99.93 |
9.75 |
15.67 |
48.75 |
174.09 |
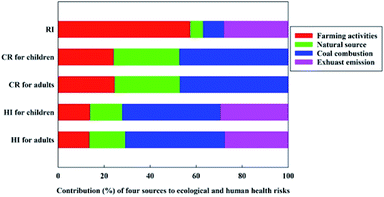 |
| Fig. 4 Source apportionment of ecological and human health risks. | |
Conclusions
The Zn, Cd, Pb, Hg and As in agricultural soil from the middle reaches of Fenhe River basin showed enriched values that exceeded their corresponding background values in soil of Shanxi province. Temporal variation indicated that HM contents (except for As) have tended to decrease over the last few years. On the basis of EF values, HMs from the study area exhibited mineral and moderate enrichment. Chromium and Hg were the main metals causing ecological risk, with Eif values higher than other metals. The RI value was 174.09, indicating that HMs in agricultural soil posed a moderate ecological risk to the ecosystem. The CR and HQ for exposure of both adults and children to HMs exceeded the accepted standard levels. Four different sources (farming activities, natural sources, coal combustion and exhaust emissions) for HMs in agricultural soils were identified via PCA and CA. Source apportionment through FA-MLR suggested that natural sources were the largest contributor to ecological risk, whereas coal combustion was the main source causing human non-carcinogenic and cancer risks. The results of this study can provide some information on environmental management and evaluation of HM pollution in developed areas of agriculture and industry.
Conflicts of interest
The authors declare no competing financial interests.
Acknowledgements
This project was supported by Applied Basic Research of Shanxi province of China (No. 201801D221042), Science and Technology Innovation Fund of Shanxi Agricultural University (No. 2016YJ13), and National Natural Science Foundation of China (No. 31500523).
References
- F. Zang, S. Wang, Z. Nan, J. Ma, Q. Zhang, Y. Chen and Y. Li, Geoderma, 2017, 305, 188–196 CrossRef CAS.
- F. J. Zhao, Y. Ma, Y. G. Zhu, Z. Tang and S. P. Mcgrath, Environ. Sci. Technol., 2015, 49, 750 CrossRef CAS.
- S. R. Smith, Environ. Int., 2009, 35, 142–156 CrossRef CAS.
- D. S. Manta, M. Angelone, A. Bellanca, R. Neri and M. Sprovieri, Sci. Total Environ., 2002, 300, 229–243 CrossRef CAS.
- T. T. Al-Rashdi and H. Sulaiman, APCBEE Proc., 2013, 5, 271–278 CrossRef CAS.
- A. Lenart-Boroń and P. Boroń, Environmental Risk Assessment of Soil Contamination, ed. M. C. Hernandez-Soriano, 2014 Search PubMed.
- A. Kabatapendias, Trace Elements in Soils and Plants, CRC Press, 3rd edn, 2000 Search PubMed.
- N. Zheng, J. Liu, Q. Wang and Z. Liang, Sci. Total Environ., 2010, 408, 726–733 CrossRef CAS PubMed.
- G. Shi, Z. Chen, S. Xu, Z. Ju, W. Li, C. Bi and J. Teng, Environ. Pollut., 2008, 156, 251–260 CrossRef CAS.
- M. Liu, Y. Yang, X. Yun, M. Zhang and J. Wang, Environ. Earth Sci., 2015, 74, 5001–5008 CrossRef CAS.
- H. Li, H. Ji, C. Shi, Y. Gao, Y. Zhang, X. Xu, H. Ding, L. Tang and Y. Xing, Chemosphere, 2017, 172, 505–515 CrossRef CAS.
- A. E. Azhari, A. Rhoujjati, M. L. E. Hachimi and J. P. Ambrosi, Ecotoxicol. Environ. Saf., 2017, 144, 464–474 CrossRef.
- M. B. Hossain, T. B. Shanta, A. S. S. Ahmed, M. K. Hossain and S. A. Semme, Mar. Pollut. Bull., 2019, 140, 255–261 CrossRef CAS.
- L. Hakanson, Water Res., 1980, 14, 975–1001 CrossRef.
- P. Gao, S. Liu, W. Ye, N. Lin, P. Meng, Y. Feng, Z. Zhang, F. Cui, B. Lu and B. Xing, Sci. Total Environ., 2015, 508, 37–45 CrossRef CAS.
- Y. Ouyang, J. Higman, J. Thompson, T. O'Toole and D. Campbell, J. Contam. Hydrol., 2002, 54, 19–35 CrossRef CAS.
- M. S. Choi, H. I. Yi, S. Y. Yang, C. B. Lee and H. J. Cha, Mar. Chem., 2007, 107, 255–274 CrossRef CAS.
- M. Liu, Y. Yang, X. Yun, M. Zhang, Q. X. Li and J. Wang, Ecotoxicology, 2014, 23, 92–101 CrossRef CAS.
- A. El Azhari, A. Rhoujjati, M. L. El Hachimi and J.-p. Ambrosi, Ecotoxicol. Environ. Saf., 2017, 144, 464–474 CrossRef CAS.
- C. Men, R. Liu, F. Xu, Q. Wang, L. Guo and Z. Shen, Sci. Total Environ., 2018, 612, 138–147 CrossRef CAS.
- Y. Chen, Y. Liu, Y. Li, Y. Wu, Y. Chen, G. Zeng, J. Zhang and H. Li, Bioresour. Technol., 2017, 243, 347–355 CrossRef CAS.
- Y. Liu, H. Wang, X. Li and J. Li, Pedosphere, 2015, 25, 901–909 CrossRef.
- F. Xu, Z. Liu, Y. Cao, L. Qiu, J. Feng, F. Xu and X. Tian, Catena, 2017, 150, 9–16 CrossRef CAS.
- H.-H. Li, L.-J. Chen, L. Yu, Z.-B. Guo, C.-Q. Shan, J.-Q. Lin, Y.-G. Gu, Z.-B. Yang, Y.-X. Yang, J.-R. Shao, X.-M. Zhu and Z. Cheng, Sci. Total Environ., 2017, 586, 1076–1084 CrossRef CAS.
- P. Liu, H. Zhao, L. Wang, Z. Liu, J. Wei, Y. Wang, L. Jiang, L. Dong and Y. Zhang, Agric. Sci. China, 2011, 10, 109–119 CrossRef CAS.
- S. S. Huang, Q. L. Liao, M. Hua, X. M. Wu, K. S. Bi, C. Y. Yan, B. Chen and X. Y. Zhang, Chemosphere, 2007, 67, 2148–2155 CrossRef CAS.
- G. Liu, T. Li, X. Liu, H. Jing, A. Wang and R. Li, J. Geochem. Explor., 2013, 132, 156–163 CrossRef CAS.
- Y. Jiang, S. Chao, J. Liu, Y. Yang, Y. Chen, A. Zhang and H. Cao, Chemosphere, 2016, 168, 1658–1668 CrossRef.
- X. Liu, L. Zhong, J. Meng, F. Wang, J. Zhang, Y. Zhi, L. Zeng, X. Tang and J. Xu, Environ. Pollut., 2018, 239, 308–317 CrossRef CAS.
- A. C. Mateos, A. C. Amarillo, H. A. Carreras and C. M. González, Environ. Res., 2018, 161, 370–380 CrossRef CAS.
- M. Briki, Y. Zhu, Y. Gao, M. Shao, H. Ding and H. Ji, Environ. Monit. Assess., 2017, 189, 458 CrossRef.
- H. Huang, C. Lin, R. Yu, Y. Yan, G. Hu and H. Li, RSC Adv., 2019, 9, 14736–14744 RSC.
- Y. Huang, Q. Chen, M. Deng, J. Japenga, T. Li, X. Yang and Z. He, J. Environ. Manage., 2018, 207, 159–168 CrossRef CAS.
- H. H. Li, L. J. Chen, L. Yu, Z. B. Guo, C. Q. Shan, J. Q. Lin, Y. G. Gu, Z. B. Yang, Y. X. Yang and J. R. Shao, Sci. Total Environ., 2017, 586, 1076–1084 CrossRef CAS PubMed.
- R. Xiao, S. Wang, R. Li, J. Wang and Z. Zhang, Ecotoxicol. Environ. Saf., 2017, 141, 17–24 CrossRef CAS.
- G. Tóth, T. Hermann, M. R. D. Silva and L. Montanarella, Environ. Int., 2016, 88, 299–309 CrossRef.
- T. A. Adagunodo, L. A. Sunmonu and M. E. Emetere, Data Brief, 2018, 18 Search PubMed.
- L. Wang, Master thesis, Shanxi Agricultural University, 2018.
- K. Loska, D. Wiechuła and I. Korus, Environ. Int., 2004, 30, 159–165 CrossRef CAS.
- L. Cai, Z. Xu, B. Peng, H. Mei, D. Lei, L. Chen, Y. Zhou and Y. G. Zhu, J. Geochem. Explor., 2015, 148, 189–195 CrossRef CAS.
- S. Wang, L.-M. Cai, H.-H. Wen, J. Luo, Q.-S. Wang and X. Liu, Sci. Total Environ., 2019, 655, 92–101 CrossRef CAS.
- S. Andrews and R. A. Sutherland, Sci. Total Environ., 2004, 324, 173–182 CrossRef CAS.
- C. Y. Hsu, H. C. Chiang, S. L. Lin, M. J. Chen, T. Y. Lin and Y. C. Chen, Sci. Total Environ., 2015, 541, 1139 CrossRef PubMed.
- Y. Tasdemir and C. Kural, Environ. Pollut., 2005, 138, 462–472 CrossRef CAS.
- R. A. Sutherland, Environ. Geol., 2000, 39, 611–627 CrossRef CAS.
- CNEMC (China National Environmental Monitoring Center), Soil Elements Background Values in China (in Chinese), China Environmental Science Press, Beijing, 1990 Search PubMed.
- CEPA (Chinese Environmental protection Administrition), Environmental quality standard for soils (GB-1995), 1995 Search PubMed.
- J. Kowalska, R. Mazurek, M. Gąsiorek, M. Setlak, T. Zaleski and J. Waroszewski, Environ. Pollut., 2016, 218, 1023–1036 CrossRef CAS.
- M. A. Khairy, A. O. Barakat, A. R. Mostafa and T. L. Wade, Microchem. J., 2011, 97, 234–242 CrossRef CAS.
- USEPA (United States Environmental Protection Agency), Risk Assessment Guidance for Superfund Volume I, Human Health Evaluation Manual (Part A), Washington, DC, 1989 Search PubMed.
- X. Duan, X. Zhao, B. Wang, Y. Chen and S. Cao, Highlights of the Chinese Exposure Factors Handbook (Adults), China environment press, Beijing, 2015 Search PubMed.
- X. Duan, Highlight of Chinese Children's Exposure Factors Handbook, China environment press, Beijing, 2016 Search PubMed.
- H. Li, X. Qian, W. Hu, Y. Wang and H. Gao, Sci. Total Environ., 2013, 456–457, 212–221 CrossRef CAS.
- USEPA (United States Environmental Protection Agency), Supplemental guidance for developing soil screening levels for superfund sites, 2001 Search PubMed.
- F. Fang, H. Wang and Y. Lin, Environ. Monit. Assess., 2011, 179, 255–265 CrossRef CAS.
- H. Chen, Y. Teng, S. Lu, Y. Wang and J. Wang, Sci. Total Environ., 2015, 512–513, 143–153 CrossRef CAS.
- S. Dehghani, F. Moore, B. Keshavarzi and B. A. Hale, Ecotoxicol. Environ. Saf., 2017, 136, 92–103 CrossRef CAS.
- L. B. Pan, J. Ma, X. L. Wang and H. Hou, Chemosphere, 2016, 148, 248–254 CrossRef CAS.
- M. Manousakas, H. Papaefthymiou, E. Diapouli, A. Migliori, A. Karydas, I. B. Radović and K. Eleftheriadis, Sci. Total Environ., 2017, 574, 155–164 CrossRef CAS.
- T. Kowalkowski, R. Zbytniewski, J. Szpejna and B. Buszewski, Water Res., 2006, 40, 744–752 CrossRef CAS.
- W. H. Organization, Guidelines for Drinking Water Quality, vol. I. Recommendations, WHO, Geneva, 1993 Search PubMed.
- United States Department of Energy, RAIS: Risk Assessment Information System, 2004 Search PubMed.
- EFSA (European Food Safety Authority), EFSA J., 2010, 8, 1570 CrossRef.
- J. Zhang, H. Li, Y. Zhou, L. Dou, L. Cai, L. Mo and J. You, Environ. Pollut., 2018, 235, 710–719 CrossRef CAS.
- C. Cao, Q. Zhang, Z.-B. Ma, X.-M. Wang, H. Chen and J.-J. Wang, Geoderma, 2018, 328, 1–9 CrossRef CAS.
- X. Li, Z. Zhao, Y. Yuan, X. Wang and X. Li, RSC Adv., 2018, 8, 10665–10672 RSC.
- W. Meng, Z. Wang, B. Hu, Z. Wang, H. Li and R. C. Goodman, Agric. Water Manag., 2016, 171, 153–161 CrossRef.
- S. Li and Z. Jia, Catena, 2018, 163, 414–423 CrossRef CAS.
- J. Zhang, Y. Wang, J. Liu, Q. Liu and Q. Zhou, J. Soils Sediments, 2016, 16, 634–644 CrossRef CAS.
- Q. Guan, F. Wang, C. Xu, N. Pan, J. Lin, R. Zhao, Y. Yang and H. Luo, Chemosphere, 2017, 193, 189–197 CrossRef.
- J. Wu, J. Song, W. Li and M. Zheng, Environ. Sci. Pollut. Res., 2016, 23, 1428 CrossRef CAS.
- L. Luo, Y. Ma, S. Zhang, D. Wei and Y. G. Zhu, J. Environ. Manage., 2009, 90, 2524–2530 CrossRef CAS.
- N. Zhang, B. Li and K. Hu, Acta Pedol. Sin., 2003, 40, 151–154 CAS.
- Y. Liu, H. Zhang and J. Yin, Trans. CSAE, 2008, 24, 57–60 Search PubMed.
- D. Wang, X. Wu, Z. Zhang, D. Yang and L. Yang, Journal of Shanxi Agricultural Sciences, 2015, 43, 1290–1296 Search PubMed.
- J. Li, H. Zhang and W. Shi, Energy Environ. Sci., 2013, 34, 116–120 Search PubMed.
- H. Zhang, G. Liu, W. Shi and J. Li, Bull. Environ. Contam. Toxicol., 2014, 93, 182–186 CrossRef CAS.
- S. Bi, Y. Yang, C. Xu, Y. Zhang, X. Zhang and X. Zhang, Mar. Pollut. Bull., 2017, 121, 357–366 CrossRef CAS.
- M. Dong, Y. Gao and Z. Yongqing, Journal of Henan Agricultural Sciences, 2014, 43, 72–78 Search PubMed.
- X. Guo, Y. Wang, X. Cao and T. Zhang, Bulletin of Soil and Water Conservation, 2018, 38, 53–57 Search PubMed.
- G. Ding, G. Fan and Y. Zhang, Journal of Irrigation and Drainage, 2015, 34, 53–55 Search PubMed.
- H. Chen, Y. Teng, S. Lu, Y. Wang, J. Wu and J. Wang, Chemosphere, 2016, 144, 1002–1011 CrossRef CAS PubMed.
- M. Chabukdhara and A. K. Nema, Ecotoxicol. Environ. Saf., 2013, 87, 57–64 CrossRef CAS PubMed.
- Shanxi Provincial Bureau of Statistics, Shanxi Statistical Yearbook 2017, China Statistics Press, Beijing, 2018 Search PubMed.
- H. Hao, W. Chen, Y. Lv and Z. Liao, Hunan Agric. Sci., 2018, 50–54 Search PubMed.
- National Bureau of Statistics, China Statistical Yearbook 2016, Statistic Press, Beijing, 2018 Search PubMed.
- J. Wang, S. Li, X. Cui, H. Li, X. Qian, C. Wang and Y. Sun, Ecotoxicol. Environ. Saf., 2016, 128, 161–170 CrossRef CAS.
- National Bureau of Statistics, China Statistical Yearbook 2016, Statistic Press, Beijing, 2017 Search PubMed.
- R. Raja, A. K. Nayak, K. S. Rao, C. Puree, M. Shahid, B. B. Panda, A. Kumar, R. Tripathi, P. Bhattacharyya and M. J. Baig, Bull. Environ. Contam. Toxicol., 2014, 93, 106–112 CrossRef CAS.
- L. D. Sabin, J. H. Lim, K. D. Stolzenbach and K. C. Schiff, Environ. Toxicol. Chem., 2010, 25, 2334–2341 CrossRef.
- H. Pekey, D. Karakaş and M. Bakoglu, Mar. Pollut. Bull., 2004, 49, 809–818 CrossRef CAS.
- F. Zhou, H. Guo and L. Liu, Environ. Geol., 2007, 53, 295–305 CrossRef CAS.
|
This journal is © The Royal Society of Chemistry 2019 |
Click here to see how this site uses Cookies. View our privacy policy here.