DOI:
10.1039/C9RA03912K
(Paper)
RSC Adv., 2019,
9, 33354-33367
An integrated metabolomics and 16S rRNA gene sequencing approach exploring the molecular pathways and potential targets behind the effects of Radix Scrophulariae†
Received
24th May 2019
, Accepted 8th October 2019
First published on 17th October 2019
Abstract
To assess the impact of the caecal microbiota on faecal metabolic phenotypes in the presence of Radix Scrophulariae (Chinese name: Xuanshen), an integrated approach involving 16S rRNA gene sequencing combined with ultrahigh-performance liquid chromatography/time-of-flight mass spectrometry (UHPLC/TOF-MS)-based faecal metabolomics was applied to Radix Scrophulariae-treated rats. Interestingly, Radix Scrophulariae led to significant gut microbiota changes at the phylum and genus levels in treated rats compared to control rats. Additionally, distinct changes in faecal metabolites, including linoleic acid (LA), guanosine, inosine, hypoxanthine, xanthine, 4-hydroxycinnamic acid, cholic acid, N-acetyl-D-glucosamine, L-urobilinogen and uridine, were observed in Radix Scrophulariae-treated rats. Of these, seven metabolites were up-regulated, and the remaining three metabolites were down-regulated. Moreover, there were substantial associations between altered levels of gut microbiota genera and discrepant levels of faecal metabolites, particularly for compounds involved in LA and purine metabolism. These results demonstrated that the gut microbiota is altered in association with faecal metabolism following treatment with Radix Scrophulariae. Our findings suggest that further application of this 16S rRNA gene sequencing and UHPLC/TOF-MS-based metabolomics approach will facilitate the assessment of the pharmacological action of Radix Scrophulariae and thus expand the scope of this herb.
1. Introduction
Radix Scrophulariae, the dried root of Scrophularia ningpoensis Hemsl., belongs to the Scrophulariaceae family and has been used in traditional Chinese medicine (TCM) for thousands of years. In the Pharmacopoeia of the People's Republic of China (2015 edition), the species' traditional functions include the treatment of febrile diseases, constipation, hot eyes, pharyngalgia, diphtheria, and scrofula. Modern pharmacological research has shown that Radix Scrophulariae inhibits ventricular remodelling,1–4 hypoxia-induced microglial activation and neurotoxicity,5 hypertension and attenuating arteriosclerosis,6 proliferation, apoptosis induction in cancer cell lines,7 and antioxidative activity,8 among other actions. TCM has always been noted for its multi-component and multi-targeted action mechanisms, and the gut microbiota is one of its targets. Many TCM substances are likely to exert therapeutic functions by altering the structure and metabolism of the gut flora. TCM has a major influence on the human microbiota and is suggested to possess broader prospects for gut flora research. Following prolonged oral drug use, the gut microbiota and metabolites may be disturbed after reaching the gastrointestinal tract.9 In rats, the caecum is the largest section of the gastrointestinal tract and contains many microbes per gram that support the digestion of food under appropriate conditions. The caecal microbiota is essential for the maintenance of normal rat physiology. However, few studies have investigated variations in the caecal microbiota and faecal metabolic phenotypes associated with Radix Scrophulariae. The intestinal flora may not only affect the pharmacological effects of Radix Scrophulariae but also be critical for effective clinical application.
Thus, we first conducted high-throughput 16S rRNA gene sequencing to investigate changes in the structure and function of the caecal microbiota in rats after treatment with Radix Scrophulariae. In the context of the relationship between the gut microbiota and host metabolic phenotypes, it is possible to address the question of how microbial communities affect metabolic functions using non-targeted metabolomics. Faecal metabolomics reveal not only the relationship between genes and phenotypes and permit the monitoring and inference of gene function but also a symbiotic relationship between the host and intestinal flora. Therefore, ultrahigh-performance liquid chromatography/time-of-flight mass spectrometry coupled with pattern recognition detection methods was performed to investigate potential metabolites and metabolic pathways involved in Radix Scrophulariae usage. Reports describing faecal metabolic profiles are scarce, and no information is available regarding changes in faecal metabolic phenotypes associated with Radix Scrophulariae. Thus, it is very important to assess alterations in gut microbiota-related faecal metabolic phenotypes to further understand the pharmacological effect of Radix Scrophulariae.
In this study, normal rats were treated with Radix Scrophulariae to explore the role of the gut microbiota and the underlying metabolic mechanisms associated with Radix Scrophulariae use. Both 16S rRNA gene sequencing and UHPLC/TOF-MS-based faecal metabolomics10 were applied to analyse the gut microbiota and faecal metabolic phenotype changes in Radix Scrophulariae-treated rats.
2. Materials and methods
2.1. Reagents and biochemicals
HPLC-grade acetonitrile was purchased from Fisher Scientific (Pittsburgh, PA). Purified water (18.2 MΩ) was prepared using a Milli-Q water purification system (Millipore, USA). Formic acid, ammonium formate and leucine-enkephalin were purchased from Sigma-Aldrich (St. Louis, MO, USA). Agarose (75510-019) and a Quant-iTPicoGreen dsDNA Assay Kit were purchased from Invitrogen (Shanghai, China). Ethidium bromide stock solution (EX328-5 mL) was purchased from Biological Engineering Co., Ltd. (Shanghai). A DNA Isolation Kit (12888) was obtained from MoBioPowerSoil®. A DNA Gel Extraction kit was obtained from AxygenAxy Prep. A DNA LT Sample Prep Kit (FC-121-4001 or FC-121-4002) was obtained from TruSeq Nano. A Quant-iTPicoGreen dsDNA Assay Kit (P7589) was purchased from Invitrogen. An Agilent High Sensitivity DNA Kit (5067-4626) was purchased from Agilent. A MiSeq Reagent Kit v2 (600-cycles-PE) (MS-102-3003) was acquired from Illumina.
2.2. Plant material and extract preparation
The natural medicinal materials in the root of Scrophularia ningpoensis Hemsl. Radix Scrophulariae were acquired from the Heilongjiang Province Drug Company (Harbin, PR China). The voucher specimen (hlj-20120623012) of the herb was authenticated by Prof. Zhenyue Wang of the Department of Resources and Development of TCM at Heilongjiang University of Traditional Chinese Medicine, and it met the standards of the “Pharmacopoeia of the People's Republic of China (2015 edition)”, page 117.
Two kilograms of dried Radix Scrophulariae roots were crushed and extracted with petroleum ether (1.2 L × 2) for 12 h per treatment, and the combined extract solutions were concentrated in vacuo to give 8.5 g of fatty oil extracts of Radix Scrophulariae. The drug residue was extracted with 10 and 8 volumes of boiling water under reflux condensation for 1.5 h each. The combined extracts were freeze-dried to yield a residue of 1.014 kg (50.7%), which constituted the aqueous extract of Radix Scrophulariae. The fingerprints of this aqueous extract were determined by performing UHPLC (Fig. 1). A Waters 2695 Acquity™ UHPLC (consisting of a vacuum degasser, auto sampler, binary pump, PAD-DAD detector and oven) was equipped with a Spursil™ C18 column (250 mm × 4.6 mm, i.d. 5 μm, Waters Corp., Milford, USA). The mobile phase was composed of acetonitrile (A) and water (B) with 0.17% acetic acid. The flow rate was set at 0.8 mL min−1. The gradient elution conditions were: 1% A, 0–5 min; 1–2% A, 5–6 min; 2–5% A, 6–20 min; 5–30% A, 20–40 min; and 30–60% A, 40–55 min. The detection wavelength was 190–600 nm. The column temperature was set to 30 °C.
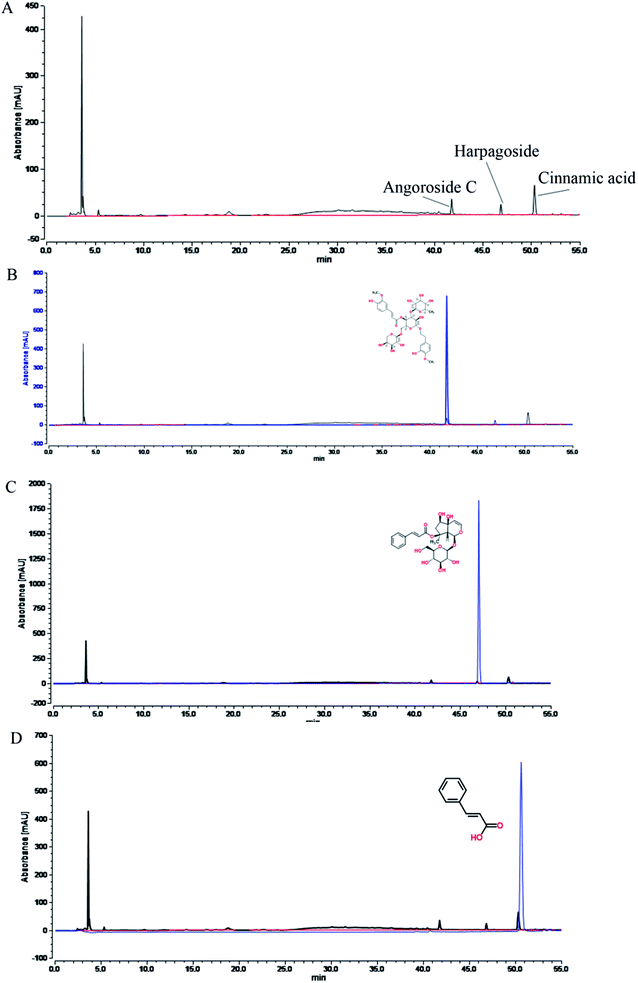 |
| Fig. 1 Fingerprint chromatograms of the aqueous extract of Radix Scrophulariae, which include (A), angoroside C (B), harpagoside (C) and cinnamic acid (D). | |
2.3. Rats and treatments
Thirty male Sprague-Dawley rats (healthy male rats), weighing 200 ± 20 g, were purchased from the Liaoning Changsheng Biotechnology Co., Ltd. (PR China) (animal certificate no.: SCXK [Liao] 2015-0001). Rats were given a standard diet and free access to water and kept in metabolic cages (one animal per cage) with constant temperature (21–23 °C) and humidity (40–50%) in controlled rooms and a 12 h/12 h light/dark cycle. After acclimatization for two weeks, rats were used for experiments. This study was performed in strict accordance with the recommendations of the Guide for the Care and Use of Laboratory Animals of the National Institutes of Health. The protocol was approved by the Committee on the Ethics of Animal Experiments of the College of Pharmacy of Heilongjiang University of Chinese Medicine.
Twenty rats were randomly divided into two groups: a control group and an aqueous extract of Radix Scrophulariaegroup (n = 10 per group). Rats in the Radix Scrophulariae group received the aqueous Radix Scrophulariae extract (1350 mg of crude drug per kg, i.g.) once per day for 15 days, and the control group rats received the same volume of 0.9% saline once daily for 15 days.
2.4. Faecal metabolomics
2.4.1 Faecal sample preparation. Faecal pellets were obtained from each rat in the Radix Scrophulariae and control groups after baseline and the 1st, 3rd, 5th, 7th, 9th, 11th, 13th and 15th days post-treatment. Then, 300 mg of freeze-dried faecal pellets were dissolved in 2.7 mL of chilled methanol and vortexed for 3 min followed by ultrasonication for 15 min. The faecal suspension was centrifuged twice at 15
000g for 15 min at 4 °C. Supernatants were obtained and stored at 4 °C for UHPLC/TOF-MS analysis.
2.4.2 UHPLC/TOF-MS analysis. A Waters Acquity™ UHPLC (consisting of a vacuum degasser, autosampler, binary pump, photodiode array detector, and oven) was equipped with an ACQUITY UHPLC® BEH C18 column (2.1 mm × 50 mm, i.d. 1.7 μm, Waters Corp). The analytical column was maintained at a temperature of 40 °C, and the mobile phase consisted of acetonitrile (A) and water (B), each containing 0.05% formic acid. A solvent gradient system was used: 2–100% A for 10 min; 100% A from 10 to 12 min; 100–2% A from 12–13 min; and 2% A for 2 min. The flow rate was 0.4 mL min−1, and the injection volume was 2 μL. The eluent was introduced to the MS directly without a split.MS analysis was performed on a TOF analyser in the SYNAPT HDMS system (Waters Corporation) in positive ion mode (capillary voltage, 1300 V; sample cone voltage, 60 V; source temperature, 110 °C; desolvation temperature, 350 °C; desolvation gas flow, 750 L h; cone gas flow, 20 L h−1) and negative ion mode (capillary voltage, 1500 V; sample cone voltage, 70 V; source temperature, 110 °C; desolvation temperature, 350 °C; desolvation gas flow, 750 L h; cone gas flow, 20 L h−1). MS data were collected in full scan mode from m/z 100–1500. All data were acquired using an independent reference lock mass via the LockSpray™ interface to ensure accuracy and reproducibility during MS analysis. Leucine-encephalin was used as the reference ion for the positive ion mode ([M + H]+ = 556.2771) and the negative ion mode ([M − H]− = 554.2615) at a concentration of 1 ng mL−1 under a flow rate of 30 μL min−1. The data were collected in centroid mode, and the Lock Spray frequency was set at 15 s and averaged over five scans for correction.
2.4.3 Data processing and multivariate analysis. The UPLC-TOF/MS system provides precise molecular mass data for the mass spectrum peaks corresponding to different ions. These data are first used to determine the molecular weight of metabolites. After samples were tested, data were imported into Progenesis QIV3.0.1 (Waters Corporation, 2015) which was used to perform alignment and peak picking. Our research team developed a metabolomic data calibration tool (MDCT) based on Pooled Quality Control (PQC) strategy for calibration and integration of metabolomics data. This strategy greatly improves the quality of PQC by properly pre-processing the mixed sample quality control. The corrected data set can be used directly for subsequent statistical analysis.Confirmation of the compounds was performed by the parameters, including “Score”, “Fragmentation score” and “Isotope similarity” given by Progenesis QI. “Score” ranging from 0 to 100, is used to quantify the reliability of each identity. According to the score results of the reference standards, the threshold was set at 35.0. “Fragmentation score” represents the matching degree between the theoretical fragments and the measured ones. The fragmentation score of 0 indicates no match occurs or the compound generates no fragments. “Isotope similarity” is calculated by comparison of the measured isotope distribution of a precursor ion with the theoretical. Databases such as HMDB (http://www.hmdb.ca/) version 4.0 and MassBank (http://www.massbank.jp/) system version 2.0 were used for confirmation.11,12 Metabolic pathway analysis was performed with Metabo-Analyst Pathway Analysis (http://www.metaboanalyst.ca/Metabo-Analyst/) and Cytoscape software (version 3.4.0) based on database sources, including KEGG (http://www.genome.jp/kegg/), SMPDB (http://www.smpdb.ca/), Progenesis Metascope, and HMDB, to identify, analyse and visualize the affected metabolic pathways. A relative mass error of 5 ppm was used for theoretical fragmentation. The normalized data were exported to Ezinfo 2.0 software for orthogonal projection to perform principal component analysis (PCA), orthogonal to the partial least squares-discriminate analysis (OPLS-DA) and 2D-PCA. PCA and 2D-PCA was applied to assess whether the Radix Scrophulariae group and normal controls were separated. OPLS-DA was employed to characterize metabolic perturbation induced by Radix Scrophulariae. In the OPLS-DA model, variable importance (VIP) > 1 and standard S plots were generated to select potential biomarkers for Radix Scrophulariae treatment. Student's t-test was used for statistical analysis to evaluate significant differences between potential intervention targets. An analysis of variance (ANOVA) p-value ≤ 0.05 indicated that the effects of potential biomarkers in different experimental groups were significant. A multivariate data matrix consisting the retention time, m/z, ANOVA (p), compound ID, adducts, score, fragmentation score, isotope similarity, theoretical isotope distribution, q value, max fold change and intensity (via the peak area normalized to protein concentration) for each ion was analysed using Ezinfo software (Waters).
2.5. 16S rRNA microbial diversity analysis
2.5.1 Caecal sample preparation. An additional 5 rats, except for those in the control group (0.9% saline, i.g., n = 5), were treated with the aqueous extract of Radix Scrophulariae (1350 mg of crude drug per kg, n = 5) daily for 11 consecutive days at 1 mL/100 g body weight. The rats were subjected to intraperitoneal anaesthesia with 1% sodium pentobarbital (0.15 mL/100 g body weight), and all efforts were made to minimize suffering. The contents of the caecum were collected 24 h after the 11th day following administration. A total of 10 content samples were immediately frozen in liquid nitrogen and stored at −80 °C until further use.
2.5.2 Data analysis. Total microbial genomic DNA samples were extracted using the DNeasyPowerSoil Kit (QIAGEN, Inc., Netherlands), following the manufacturer's instructions, and stored at −20 °C prior to further analysis. The quantity and quality of extracted DNAs were measured using a NanoDrop ND-1000 spectrophotometer (Thermo Fisher Scientific, Waltham, MA, USA) and agarose gel electrophoresis, respectively.PCR amplification of the bacterial 16S rRNA gene V4 region was performed using the forward primer, 520F (5′-barcode + AYTGGGYDTAAAGNG-3′), and the reverse primer, 802R (5′-TACNVGGGTATCTAATCC-3′). Sample-specific 7-bp barcodes were incorporated into the primers for multiplex sequencing. The PCR components contained 5 μL of Q5 reaction buffer (5×), 5 μL of Q5 High-Fidelity GC buffer (5×), 0.25 μL of Q5 High-Fidelity DNA Polymerase (5 U per μL), 2 μL (2.5 mM) of dNTPs, 1 μL (10 μM) of each forward and reverse primer, 2 μL of DNA template, and 8.75 μL of ddH2O. Thermal cycling consisted of initial denaturation at 98 °C for 4 min, followed by 25 cycles consisting of denaturation at 98 °C for 30 s, annealing at 50 °C for 30 s, and extension at 72 °C for 30 s, with a final extension of 5 min at 72 °C. PCR amplicons were purified with Agencourt AMPure Beads (Beckman Coulter, Indianapolis, IN) and quantified using the PicoGreen dsDNA Assay Kit (Invitrogen, Carlsbad, CA, USA). After the individual quantification step, amplicons were pooled in equal amounts, and paired-end 2 × 300 bp sequencing was performed using the Illumina MiSeq platform with MiSeq Reagent Kit v3 at Shanghai Personal Biotechnology Co., Ltd. (Shanghai, China).
The Quantitative Insights Into Microbial Ecology (QIIME, v1.8.0) pipeline was employed to process the sequencing data, as previously described.13 Briefly, raw sequencing reads with exact matches to the barcodes were assigned to respective samples and identified as valid sequences. The low-quality sequences were filtered through the following criteria:14,15 sequences that had a length of <150 bp, sequences that had average Phred scores of <20, sequences that contained ambiguous bases, and sequences that contained mononucleotide repeats of >8 bp. Paired-end reads were assembled using FLASH.16 After chimaera detection, the remaining high-quality sequences were clustered into operational taxonomic units (OTUs) at 97% sequence identity by UCLUST.17 A representative sequence was selected from each OTU using default parameters. OTU taxonomic classification was conducted by BLAST searching of the representative sequences set against the Greengenes Database18 using the best hit.18
An OTU table was further generated to record the abundance of each OTU in each sample and the taxonomy of these OTUs. OTUs containing less than 0.001% of total sequences across all samples were discarded. To minimize the difference of sequencing depth across samples, an averaged, rounded rarefied OTU table was generated by averaging 100 evenly resampled OTU subsets under the 90% of the minimum sequencing depth for further analysis.
Sequence data analyses were mainly performed using QIIME and R packages (v3.2.0). OTU-level alpha diversity indices, such as the Chao1 richness estimator, abundance-based coverage estimator (ACE) metric, Shannon diversity index, and Simpson index, were calculated using the OTU table in QIIME. OTU-level ranked abundance curves were generated to compare the richness and evenness of OTUs among samples. Beta diversity analysis was performed to investigate the structural variation of microbial communities across samples using UniFrac distance metrics19,20 and visualized via principal coordinate analysis (PCoA), nonmetric multidimensional scaling (NMDS) and unweighted pair-group method with arithmetic means (UPGMA) hierarchical clustering.21
Differences in the UniFrac distances for pairwise comparisons among groups were determined using Student's t-test and the Monte Carlo permutation test with 1000 permutations and visualized through box-and-whiskers plots. PCA was also conducted based on the genus-level compositional profiles.21 The significance of the differentiation of microbiota structures amongst the groups was assessed by permutational multivariate analysis of variance (PERMANOVA) and analysis of similarities ANOSIM22,23 evaluations using the R package “vegan”. The taxonomy compositions and abundances were visualized using MEGAN24 and GraPhlAn.25 A Venn diagram was generated to visualize the shared and unique OTUs among samples or groups using the R package “VennDiagram” based on the occurrence of OTUs across samples/groups regardless of their relative abundance.26 Taxa abundances at the phylum, class, order, family, genus and species levels were statistically compared among samples or groups by Metastats,27 and visualized as violin plots. Linear discriminant analysis effect size (LEf Se) analysis was performed to detect differentially abundant taxa across groups using the default parameters.28 Partial least squares discriminant analysis (PLS-DA) was also introduced as a supervised model to reveal the microbiota variation among groups using the “plsda” function in the R package “mixOmics”.29 Random forest analysis was applied to discriminate the samples from different groups using the R package “randomForest” with 1000 trees all the default settings.30 The generalization error was estimated using 10-fold cross-validation. The expected “baseline” error was also included, which was obtained by a classifier that simply predicts the most common category label. Co-occurrence analysis was performed by calculating Spearman's rank correlations between the predominant taxa. Correlations with |RHO| > 0.6 and p < 0.01 were visualized as a co-occurrence network using Cytoscape.31 Microbial functions were predicted by phylogenetic investigation of communities by reconstruction of unobserved states (PICRUSt), based on high-quality sequences.32
3. Results
3.1. Faecal metabolic profiling of rats treated with Radix Scrophulariae
3.1.1 Trajectory changes over time in normal rats treated with Radix Scrophulariae. PCA was first performed to investigate trajectory changes over time in normal rats treated with Radix Scrophulariae (Fig. 2A). The metabolic phenotype of the faecal supernatant on the first day was similar to that on day 0. Beginning on the third day, the metabolic phenotype of rat faeces gradually deviated from that of day 0, such that the greatest distance was observed between the 11th day and day 0. Interestingly, the distance observed by the 13th day gradually reached that of day 0, and the metabolic phenotypes on the 15th day mostly overlapped with those of day 0, which indicated that the rats given Radix Scrophulariae for 15 days had become resistant to the herb. Next, 2D-PCA was performed to sharpen the already established (weak) separation between the groups identified by PCA to investigate the effects of treating normal rats with Radix Scrophulariae for eleven days (Fig. 2B and C). The results indicated that the samples separated into two blocks, thus improving our efforts to discover potential targets and suggesting that biochemical agitation was significant in the Radix Scrophulariae-treated group.
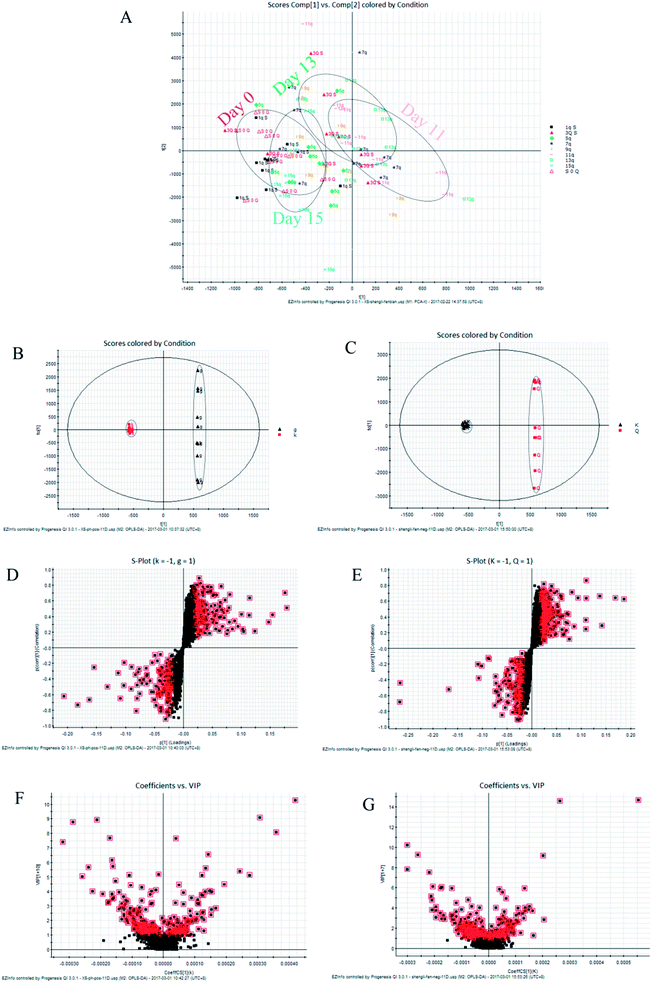 |
| Fig. 2 (A) PCA plot of the faecal metabolic trajectory of rats at different time points before and after the administration of Radix Scrophulariae. A 2D-PCA plot based on data from the control and Radix Scrophulariae groups at day 11 in positive (B) and negative (C) ion mode. S and VIP plots of faecal samples from the control and Radix Scrophulariae groups in both positive (D and F) and negative (E and G) ion modes. K represents the control group; g represents the Radix Scrophulariae group. The points in Fig. 2A represent control and Radix Scrophulariae group samples. Q and g in Fig. 2B represent the Radix Scrophulariae group, and k in Fig. 2B represents the control group. | |
3.1.2 Multivariate data analysis. S plots is a tool for visualizing the covariance and correlation between metabolites and model categories. Those ions that deviate from the origin helped show that the two groups were significantly clustered. S-plots correspond to VIP statistics. VIP values represent the effect of metabolites on clustering. VIP > 1 indicates that these variables have an above-average effect on clustering. VIP values > 1 in the S and VIP plots (Fig. 2D–G) were far from the origin, and p ≤ 0.05 was selected for further chemical structure identification. Ten potential biomarkers were screened from the faecal samples, of which seven metabolites were upregulated by Radix Scrophulariae and three were downregulated by comparing the peak areas of these potential biomarkers (Fig. 3A and B and Table S1†).
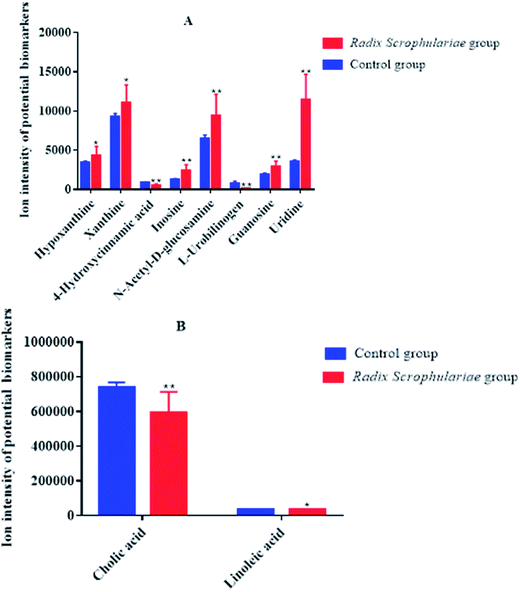 |
| Fig. 3 Ion intensity of potential biomarkers in different faecal sample groups (A and B). Data represent the mean ± SE of each group (n = 10 in each group). *Altered trend compared to the control group, p < 0.05; **altered trend compared to the control group, p < 0.01. | |
Ten metabolites were used as potential biomarkers for control rats. The pathways were enriched and analysed using MetaPA recognition to ensure that metabolic pathways were associated with metabolites. The critical value for each metabolic pathway was set to 0.05. If the value was greater than 0.05, then the pathway was chosen as a key metabolic pathway (Fig. 4 and Table S2†). Further analysis of pathways and networks influenced by Radix Scrophulariae was performed using MetaboAnalyst and Cytoscape software (Fig. 4B), which revealed that the potential intervention targets in the faecal samples were primarily responsible for LA and purine metabolism. Among them, the potential biomarker involved in LA metabolism was LA, and the markers involved in purine metabolism were guanosine, inosine, hypoxanthine and xanthine.
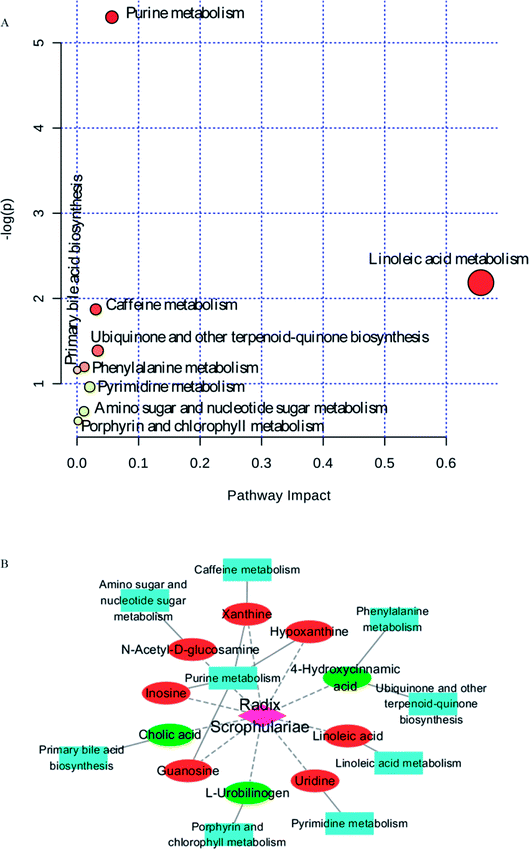 |
| Fig. 4 Pathway analysis of metabolites regulating metabolism and metabolic pathway analysis of potential intervention targets of Radix Scrophulariae. Elliptical nodes represent pathways, rectangle nodes represent metabolites (red: upregulation, green: downregulation), and diamond nodes represent Radix Scrophulariae. Black edges represent the relationships between the metabolites and pathways, and red edges represent possible relationships between Radix Scrophulariae and metabolites. | |
3.2. Gut microbiota changes in rats treated with Radix Scrophulariae
3.2.1 Bacterial community structure. Based on 97% species similarity, 1598 OTUs were present in the control group at the phylum level, 1598 at the class level, 1597 at the order level, 1206 at the family level, 597 at the genus level and 78 at the species level (Fig. 5A). In the Radix Scrophulariae group, there were 1487 OTUs at the phylum level, 1487 at the class level, 1486 at the order level, 1120 at the family level, 542 at the genus level, and 62 at the species level (Fig. 5A). At the phylum level, the caecal bacteria in the control group belonged to 1598 OTUs, of which 1357 overlapped with the Radix Scrophulariae group, 241 were unique to the control group, and 130 were in the Radix Scrophulariae group (Fig. 5B). The Radix Scrophulariae group contained a fewer total OTUs than the control group, indicating that Radix Scrophulariae reduces the number of OTUs in the caecal microbiota of healthy rats.
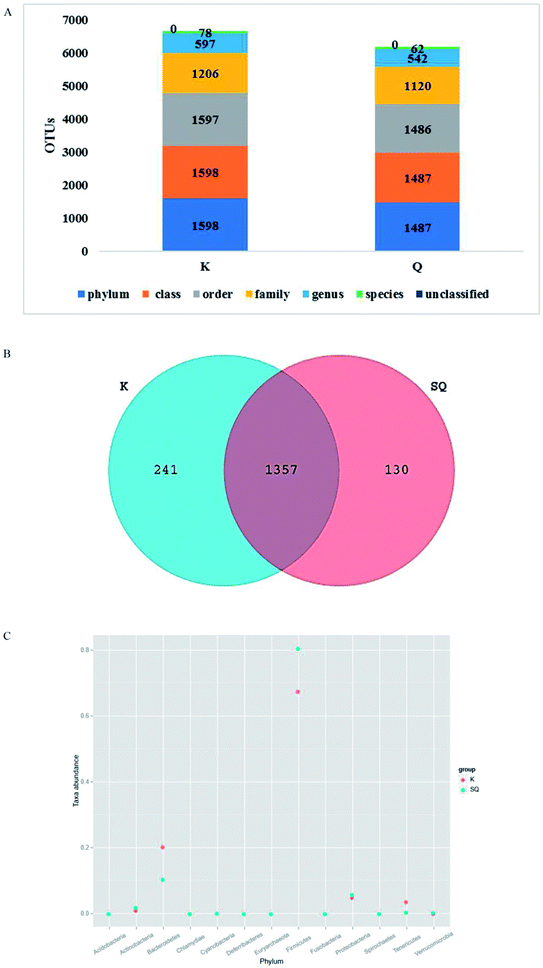 |
| Fig. 5 OTUs of all levels (A and B) are represented by a Venn diagram of the OTUs at the phylum level and a bar chart of the taxon abundance at the phylum level (C). Each ellipse represents a group, and overlapping regions of the ellipses indicate that OTUs are shared by groups. The number in each block indicates the number of OTUs contained in each group or the number of unique OTUs. k represents the control group, and SQ represents the Radix Scrophulariae group. | |
3.2.2 Taxonomic analysis of caecal flora. At the phylum level, the caecal bacteria in the control and Radix Scrophulariae groups belonged to 13 phyla, of which Radix Scrophulariae reduced Bacteroidetes by 60% (from 20 to 12%) and increased Firmicutes from 68 to 81%. Radix Scrophulariae decreased the remaining phyla from 12 to 9%; of these, Radix Scrophulariae increased Actinobacteria by 67% (from 1 to 3%) and Proteobacteria by 20% (from 5 to 6%) (Fig. 5C).At the genus level, the Prevotella levels decreased the most, followed by Ruminococcus. The remaining nine groups showed minor decreases: Corynebacterium, Dehalobacterium, Brevundimonas, Rhodococcus, Enhydrobacter, Neisseria, Flavobacterium, Acinetobacter and Anaerobiospirillum. The most interesting Radix Scrophulariae groups demonstrating significant increases were Butyricimonas, Rothia, Lactococcus, Geobacillus, Burkholderia, Odoribacter, Zea and Sediminibacterium (Table S3†).
3.3. Correlation between gut microbiota and faecal metabolic phenotypes
To explore the functional relationship between altered gut microbiota and disturbed faecal metabolites, we formulated three correlation matrices based on Pearson's correlation coefficients. A clear correlation (r > 0.5 or r < −0.5, p < 0.05) was observed between the gut microbiota and faecal metabolic phenotypes (Fig. 6A). In particular, correlations were found between gut microbiota-related faecal metabolites and specific gut bacteria (∣r∣ > 0.8): the main pathways were purine and LA metabolism (Fig. 6B and C). The metabolites involved in purine metabolism were guanosine, hypoxanthine, xanthine and inosine. LA was primarily involved in LA metabolism. Guanosine displayed strong negative correlations with Burkholderia and Anaerobiospirillum. Hypoxanthine displayed strong positive correlations with Rhodococcus and Lactococcus but negative correlations with Odoribacter and Facklamia. Xanthine displayed a strong positive correlation with Neisseria and a negative correlation with Lactobacillus. However, Neisseria, Corynebacterium, Ruminococcus, Enhydrobacter, Lactobacillus, Lactococcus, Acinetobacter and Sediminibacterium exhibited significantly positive correlations with inosine. LA displayed strong negative correlations with Lactobacillus and Lactococcus and positive correlations with Brevundimonas, Dehalobacterium, Enhydrobacter, Acinetobacter and Anaerobiospirillum. In summary, Radix Scrophulariae altered the structure and composition of the gut microbiota and substantially altered the faecal metabolic phenotype.
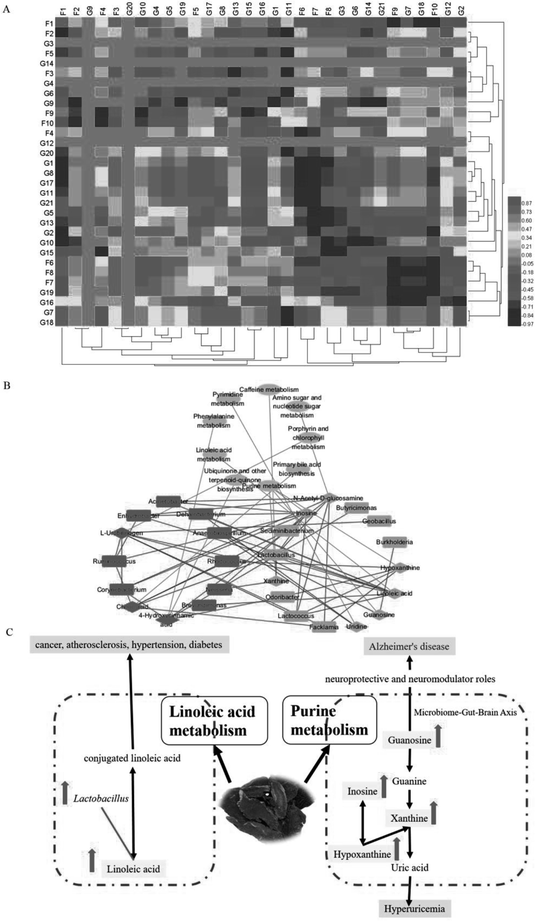 |
| Fig. 6 (A) Correlation of specific gut bacteria and gut microbiota-related faecal metabolites. (B) Metabolic pathway analysis of metabolites and genera in the Radix Scrophulariae-treated group. Diamond nodes represent metabolites, ellipse nodes represent pathways and round rectangles represent genera (red: upregulation, green: downregulation). Red edges represent positive correlations between metabolites and genera, while green edges represent negative correlations between metabolites and genera. (C) Correlation between gut microbiota genera, perturbed metabolites and the most relevant metabolic pathways. Pink rectangle nodes represent metabolites. The levels of potential biomarkers in the Radix Scrophulariae group compared to the control group were labelled with (↓) for downregulation and (↑) for upregulation. | |
4. Discussion
An integrated 16S rRNA gene sequencing and UHPLC/TOF-MS-based metabolomics approach10 was performed to explore the impact of Radix Scrophulariae on the gut microbiota and faecal metabolic phenotype. The results demonstrated significant differences in abundance among phyla and genera in the gut microbial community; meanwhile, the host metabolic profile also changed following Radix Scrophulariae treatment. Furthermore, the altered gut microbiota revealed significant correlations with faecal metabolites, suggesting that Radix Scrophulariae not only alters the gut microbiota but also affects the host faecal metabolic phenotypes. These findings may provide new insight into the novel pharmacological actions of Radix Scrophulariae and facilitate a greater understanding of the role of the gut microbiota and how it is modulated by this herb.
The results of this study revealed that the performances of both Bacteroidetes (12%) and Firmicutes (81%) were consistent with the theory that more than 90% of these phyla are present in the distal caecal flora.33 Moreover, variations in genera, including significantly attenuated levels of Butyricimonas, Rothia, Facklamia, Lactococcus, Geobacillus, Burkholderia, Odoribacter, Zea and Sediminibacterium, were observed in the Radix Scrophulariae-treated rats, and other genera were significantly reduced. To the best of our knowledge, however, these genera have not been reported in association with either human or animal models. Therefore, further studies are warranted to explore the roles of these bacteria in the pharmacological action of Radix Scrophulariae.
Recently, the metabolomics of faecal samples indicated a correlation between changes in metabolic phenotypes and the gut microbiota. The present study revealed that the faecal metabolic profile of Radix Scrophulariae rats was significantly different from that of normal controls. Additionally, a total of ten faecal metabolites, primarily involved in LA metabolism and purine metabolism, were identified as biomarkers of the pharmacological activity of Radix Scrophulariae.
In this study, LA levels and the number of Lactobacillus species present in the rat gut were upregulated by Radix Scrophulariae. Lactobacillus has previously been reported to convert LA into conjugated LA (CLA),34 an isomer of LA that has many beneficial effects on diseases, such as cancer, atherosclerosis, hypertension and diabetes.35 Lactobacillus demonstrates a variety of healthcare-related functions, including improved intestinal flora levels, reduced cancer risk,36 promotion of digestion, antiaging properties and disease prevention.37 Radix Scrophulariae likewise exerts anti-atherosclerosis,38 anticancer,7 and antioxidant8 effects and protects the cardiovascular system,2–4,6 indicating that Radix Scrophulariae may induce the combined effects of CLA and Lactobacillus to regulate intestinal microecology.
Guanosine, hypoxanthine, xanthine and inosine levels in the faecal supernatant were elevated by Radix Scrophulariae. Guanosine plays a neuroprotective role in the central nervous system by inhibiting glutamate neurotransmission.39 Equal numbers of neurons are present in the gut and the brain, and the neurotransmitters and metabolic pathways used by these two groups are nearly identical. Communication between the two centres is frequent, with a greater incidence of communication between neurons of the intestine to the brain than in the reverse direction. A “bacterial-gut-brain axis” has been observed between the intestine and brain that is responsible for this interaction.40 Radix Scrophulariae is useful for the treatment of Alzheimer's disease41 due to the involvement of the intestinal flora. Inosine is degraded to hypoxanthine by the purine nucleoside phosphorylase, oxidized to xanthine and eventually converted to uric acid, which is then excreted.42 The phenylpropanoid glycoside acteoside of Scrophularia ningpoensis has hypouricemic effects,43 possibly due to the Radix Scrophularia-mediated promotion of uric acid excretion through increased levels of inosine, hypoxanthine and xanthine.
The study of metabolic pathways and biaomarkers is crucial to elucidate the pathological mechanism44–50 and might provide insight into the characteristics for pharmacological effects.51–58 Gut microbiota is a functioning organ that can became signals for biological communication.59–62 Here, we observed a significant correlation between gut microbiota genera and faecal metabolites via Pearson's correlation analysis. Of particular interest, LA metabolism changes displayed strong positive correlations with Brevundimonas, Dehalobacterium, Enhydrobacter, Acinetobacter and Anaerobiospirillum species and negative correlations with Lactobacillus and Lactococcus. The purine metabolism of hypoxanthine displayed positive correlations with Rhodococcus and Lactococcus and negative correlations with Odoribacter and Facklamia species. Xanthine metabolism displayed a strong positive correlation with Neisseria species and a negative correlation with Lactobacillus species. Interestingly, Neisseria, Corynebacterium, Ruminococcus, Enhydrobacter, Lactobacillus, Lactococcus, Acinetobacter and Sediminibacterium exhibited significant positive correlations with inosine, while the purine metabolism of guanosine displayed strong negative correlations with Burkholderia and Anaerobiospirillum species. These findings suggest that the increased purine and LA metabolism are closely related to gut microbiota functions. Therefore, our results further confirmed that variations in the gut microbiota and faecal metabolic phenotypes are associated with the pharmacological activity of Radix Scrophulariae.
5. Conclusions
UHPLC/TOF-MS and 16S rRNA gene sequencing-based metabolomics were combined to assess the effects of Radix Scrophulariae on the gut microbiota and faecal metabolic phenotype. Our results showed that Radix Scrophulariae significantly altered not only the gut microbiota composition but also the faecal metabolic phenotype. In addition, correlation analysis revealed that certain altered gut microbiota genera were strongly correlated with altered faecal metabolites. Overall, these findings indicate that Radix Scrophulariae not only alters the gut microbiota at the abundance level but also alters host metabolic homeostasis. Therefore, regulated gut microbiota-related metabolites may be potential biomarkers for probing the functional effects of Radix Scrophulariae.
Conflicts of interest
The authors declare that they have no conflicts of interest.
Acknowledgements
We thank Personal Biotechnology Co., Ltd. (Shanghai, China) for their data analysis. The work was supported by the grants from the Major State Basic Research Development Program (973 Program) of China (project no. 2013CB531804) and the outstanding innovative talent support programs of Heilongjiang University of Chinese Medicine.
References
- C. Z. Chao, L. G. Wei and M. W. Xi, et al., Active components from Radix Scrophulariae inhibits the ventricular remodeling induced by hypertension in rats, SpringerPlus, 2016, 5, 358 CrossRef.
- L. G. Wei, X. C. Chang and Y. H. Xiao, et al., The effect of angoroside C on pressure overload-induced ventricular remodeling in rats, Phytomedicine, 2015, 22, 705–712 CrossRef.
- Y. H. Xiao, X. C. Chang and M. Z. Xue, et al., Effects of ethanolic extract from Radix Scrophulariae on ventricular remodeling in rats, Phytomedicine, 2012, 19, 193–205 CrossRef PubMed.
- G. Wei-Liang, C. Chang-Xun and W. Qi, et al., Effects of Chinese herb medicine Radix Scrophulariae on ventricular remodeling, Pharmazie, 2010, 65, 770–775 Search PubMed.
- S. Y. Sheu, Y. W. Hong and J. S. Sun, et al., Radix Scrophulariae extracts (harpagoside) suppresses hypoxia-induced microglial activation and neurotoxicity, BMC Complementary Altern. Med., 2015, 15, 324 CrossRef PubMed.
- C. Chen, C. X. Chen and X. M. Wu, et al., Effects of extracts of Radix Scrophulariae on blood pressure in spontaneously hypertensive rats and the underlying mechanisms, J. Chin. Integr. Med., 2012, 10, 1009 CrossRef.
- S. Xiao, E. Tolga and G. Henry Johannes, et al., Effects of Scrophularia ningpoensis Hemsl. on Inhibition of Proliferation, Apoptosis Induction and NF-κB Signaling of Immortalized and Cancer Cell Lines, Pharmaceuticals, 2012, 5, 189–208 CrossRef PubMed.
- Y. M. Li, Z. H. Han and S. H. Jiang, et al., Fast repairing of oxidized OH radical adducts of dAMP and dGMP by phenylpropanoid glycosides from Scrophularia ningpoensis Hemsl, Acta Pharmacol. Sin., 2000, 21, 1125–1128 CAS.
- C. C. Feng, A. H. Zhang, J. H. Miao, H. Sun and X. J. Wang, et al., Recent advances in understanding cross-talk between bile acids and gut microbiota, Open Journal of Proteomics and Genomics, 2018, 3(1), 024–034 Search PubMed.
- M. Yu, H. Jia and C. Zhou, et al., Variations in gut microbiota and fecal metabolic phenotype associated with depression by 16S rRNA gene sequencing and LC/MS-based metabolomics, J. Pharm. Biomed. Anal., 2017, 138, 231–239 CrossRef CAS PubMed.
- G. Paglia and G. Astarita, Metabolomics and lipidomics using traveling-wave ion mobility mass spectrometry, Nat. Protoc., 2017, 12, 797–813 CrossRef CAS.
- H. Fang, A. Zhang and J. Yu, et al., Insight into the metabolic mechanism of scoparone on biomarkers for inhibiting Yanghuang syndrome, Sci. Rep., 2016, 6, 37519 CrossRef CAS.
- J. G. Caporaso, J. Kuczynski and J. Stombaugh, et al., QIIME allows analysis of high-throughput community sequencing data, Nat. Methods, 2010, 7, 335–336 CrossRef CAS.
- S. R. Gill, P. Mihai and R. T. Deboy, et al., Metagenomic analysis of the human distal gut microbiome, Science, 2006, 312, 1355–1359 CrossRef CAS.
- H. Chen and W. Jiang, Application of high-throughput sequencing in understanding human oral microbiome related with health and disease, Front. Microbiol., 2014, 5, 508 Search PubMed.
- M. Tanja and S. L. Salzberg, FLASH: fast length adjustment of short reads to improve genome assemblies, Bioinformatics, 2011, 27, 2957–2963 CrossRef.
- R. C. Edgar, Search and clustering orders of magnitude faster than BLAST, Bioinformatics, 2010, 26, 2460 CrossRef CAS.
- T. Z. Desantis, P. Hugenholtz and N. Larsen, et al., Greengenes, a chimera-checked 16S rRNA gene database and workbench compatible with ARB, Appl. Environ. Microbiol., 2006, 72, 5069–5072 CrossRef CAS.
- L. Catherine and K. Rob, UniFrac: a new phylogenetic method for comparing microbial communities, Appl. Environ. Microbiol., 2005, 71, 8228–8235 CrossRef.
- C. A. Lozupone, H. Micah and S. T. Kelley, et al., Quantitative and qualitative beta diversity measures lead to different insights into factors that structure microbial communities, Appl. Environ. Microbiol., 2007, 73, 1576–1585 CrossRef CAS.
- R. Alban, Multivariate analyses in microbial ecology, FEMS Microbiol. Ecol., 2010, 62, 142–160 Search PubMed.
- K. Voigt, S. Bojanowski and S. Taché, et al., Home visits in primary care: contents and organisation in daily practice. Study protocol of a cross-sectional study, BMJ Open, 2016, 6, e008209 CrossRef.
- G. Karagkounis, G. Cai and P. T. Johnson, et al., The Role of Neoadjuvant Therapy in Characterizing Indeterminate Lung Lesions in Patients with Resectable Colorectal Liver Metastases, Ann. Surg. Oncol., 2015, 22, 2201–2208 CrossRef.
- D. H. Huson, S. Mitra and H.-J. Ruscheweyh, et al., Integrative analysis of environmental sequences using MEGAN4, Genome Res., 2011, 21, 1552–1560 CrossRef CAS.
- F. Asnicar, G. Weingart and T. L. Tickle, et al., Compact graphical representation of phylogenetic data and metadata with GraPhlAn, PeerJ, 2015, 3, e1029 CrossRef.
- E. Zaura, B. J. Keijser and S. M. Huse, et al., Defining the healthy “core microbiome” of oral microbial communities, BMC Microbiol., 2009, 9, 259 CrossRef.
- J. R. White, N. Nagarajan and M. Pop, et al., Statistical Methods for Detecting Differentially Abundant Features in Clinical Metagenomic Samples, PLoS Comput. Biol., 2009, 5, e1000352 CrossRef.
- N. Segata, J. Izard and L. Waldron, et al., Metagenomic biomarker discovery and explanation, Genome Biol., 2011, 12, R60 CrossRef.
- F. Rohart, B. Gautier and A. Singh, et al., mixOmics: An R package for ‘omics feature selection and multiple data integration, PLoS Comput. Biol., 2017, 13, e1005752 CrossRef.
- Q. Novi and G. Zoubin, A Very Simple Safe-Bayesian Random Forest, IEEE Trans. Pattern Anal. Mach. Intell., 2015, 37, 1297–1303 Search PubMed.
- P. Shannon, Cytoscape: A Software Environment for Integrated Models of Biomolecular Interaction Networks, Genome Res., 2003, 13, 2498 CrossRef CAS.
- M. G. I. Langille, Z. Jesse and C. J Gregory, et al., Predictive functional profiling of microbial communities using 16S rRNA marker gene sequences, Nat. Biotechnol., 2013, 31, 814 CrossRef CAS.
- J. Carlson, T. Gould and J. Slavin, Invitro analysis of partially hydrolyzed guar gum fermentation on identified gut microbiota, Anaerobe, 2016, 42, 60–66 CrossRef CAS.
- J. Ortega-Anaya and A. Hernández-Santoyo, Production of bioactive conjugated linoleic acid by the multifunctional enolase from Lactobacillus plantarum, Int. J. Biol. Macromol., 2016, 91, 524–535 CrossRef CAS.
- X. Li, Y. Han, A. Zhang, J. Miao and H. Sun, et al., Mechanistic and Therapeutic Advances in Colon Cancer: A Systematic Review, Open Journal of Proteomics and Genomics, 2019, 4(1), 001–012 CrossRef.
- M. A. Zulet, A. Marti and M. D. Parra, et al., Inflammation and conjugated linoleic acid: mechanisms of action and implications for human health, J. Physiol. Biochem., 2005, 61(3), 483–494 CrossRef CAS PubMed.
- M. Urbańska, D. Gieruszczak-Białek and H. Szymański, et al., Effectiveness of Lactobacillus reuteri DSM 17938 for the Prevention of Nosocomial Diarrhea in Children: A Randomized, Double-blind, Placebo-controlled Trial, Pediatr. Infect. Dis. J., 2016, 35, 142 CrossRef.
- P. Li, L. Ming and X. Ying-Zhi, et al., Effect of Si-Miao-Yong-An on the stability of atherosclerotic plaque in a diet-induced rabbit model, J. Ethnopharmacol., 2012, 143, 241–248 CrossRef.
- D. Lanznaster, J. M. Mack and V. Coelho, et al., Guanosine Prevents Anhedonic-Like Behavior and Impairment in Hippocampal Glutamate Transport Following Amyloid-β 1–40 Administration in Mice, Mol. Neurobiol., 2017, 54, 1–15 CrossRef.
- T. G. Dinan and J. F. Cryan, The Microbiome-Gut-Brain Axis in Health and Disease, Gastroenterol. Clin. N. Am., 2017, 46, 77 CrossRef.
- S. Wei, Potential therapeutic action of natural products from traditional Chinese medicine on Alzheimer's disease animal models targeting neurotrophic factors, Fundam. Clin. Pharmacol., 2016, 30, 490–501 CrossRef CAS.
- O. Jiro, K. Kaori and S. Shunichi, et al., Reactive oxygen species derived from xanthine oxidase interrupt dimerization of breast cancer resistance protein, resulting in suppression of uric acid excretion to the intestinal lumen, Biochem. Pharmacol., 2015, 97, 89–98 CrossRef.
- G. H. Cai, J. S. Yan and J. Zhang, et al., Hypouricemic Effects of Phenylpropanoid Glycosides Acteoside of Scrophularia ningpoensis on Serum Uric Acid Levels in Potassium Oxonate-Pretreated Mice, Am. J. Chin. Med., 2008, 36, 149–157 CrossRef.
- X. Li, A. Zhang and H. Sun, et al., Metabolic characterization and pathway analysis of berberine protects against prostate cancer, Oncotarget, 2017, 8, 65022–65041 Search PubMed.
- A. Zhang, G. Yan and Y. Han, et al., Metabolomics approaches and applications in prostate cancer research, Appl. Biochem. Biotechnol., 2014, 174, 6–12 CrossRef CAS.
- H. Zhang, A. Zhang and J. Miao, et al., Targeting regulation of tryptophan metabolism for colorectal cancer therapy: a systematic review, RSC Adv., 2019, 9, 3072–3080 RSC.
- H. Sun, A. H. Zhang and S. B. Liu, et al., Cell metabolomics identify regulatory pathways and targets of magnoline against prostate cancer, J. Chromatogr. B: Anal. Technol. Biomed. Life Sci., 2018, 1102–1103, 143–151 CrossRef CAS.
- X. J. Wang, J. L. Ren and A. H. Zhang, et al., Novel applications of mass spectrometry-based metabolomics in herbal medicines and its active ingredients: current evidence, Mass Spectrom. Rev., 2019, 9999, 1–23 Search PubMed.
- J. Xie, A. H. Zhang and S. Qiu, et al., Identification of the perturbed metabolic pathways associating with prostate cancer cells and anticancer affects of obacunone, J. Proteomics, 2019, 206, 103447 CrossRef CAS.
- Y. Nan, X. Zhou and Q. Liu, et al., Serum metabolomics strategy for understanding pharmacological effects of ShenQi pill acting on kidney yang deficiency syndrome, J. Chromatogr. B: Anal. Technol. Biomed. Life Sci., 2016, 1026, 217–226 CrossRef CAS.
- Y. Zhang, P. Liu and Y. Li, et al., Exploration of metabolite signatures using high-throughput mass spectrometry coupled with multivariate data analysis, RSC Adv., 2017, 7, 6780–6787 RSC.
- X. Liu, A. Zhang and H. Fang, et al., Serum metabolomics strategy for understanding the therapeutic effects of Yin-Chen-Hao-Tang against Yanghuang syndrome, RSC Adv., 2018, 8, 7403–7413 RSC.
- H. Sun, H. L. Zhang and A. H. Zhang, et al., Network pharmacology combined with functional metabolomics discover bile acid metabolism as a promising target for mirabilite against colorectal cancer, RSC Adv., 2018, 8, 30061–30070 RSC.
- H. Fang, A. H. Zhang and H. Sun, et al., High-throughput metabolomics screen coupled with multivariate statistical analysis identifies therapeutic targets in alcoholic liver disease rats using liquid chromatography-mass spectrometry, J. Chromatogr. B: Anal. Technol. Biomed. Life Sci., 2019, 1109, 112–120 CrossRef CAS.
- A. H. Zhang, H. Sun and G. L. Yan, et al., Chinmedomics: A Powerful Approach Integrating Metabolomics with Serum Pharmacochemistry to Evaluate the Efficacy of Traditional Chinese Medicine, Engineering, 2019, 5, 60–68 CrossRef CAS.
- H. Sun, A. H. Zhang and Q. Song, et al., Functional metabolomics discover pentose and glucuronate interconversion pathways as promising targets for Yang Huang syndrome treatment with Yinchenhao Tang, RSC Adv., 2018, 8, 36831–36839 RSC.
- L. Wang, H. Dong and A. H. Zhang, et al., Exploring the detoxification effects and mechanism of Caowu in prescription using liquid chromatography-high-resolution mass spectrometry-based metabolomics, Open Journal of Proteomics and Genomics, 2018, 3(1), 011–023 Search PubMed.
- X. N. Li, A. Zhang and M. Wang, et al., Screening the active compounds of Phellodendri Amurensis cortex for treating prostate cancer by high-throughput chinmedomics, Sci. Rep., 2017, 7, 46234 CrossRef CAS.
- X. Wang, A. Zhang and J. Miao, et al., Gut microbiota as important modulator of metabolism in health and disease, RSC Adv., 2018, 8, 42380–42389 RSC.
- H. L. Zhang, A. H. Zhang and X. H. Zhou, et al., High-throughput lipidomics reveal mirabilite regulating lipid metabolism as anticancer therapeutics, RSC Adv., 2018, 8(62), 35600–35610 RSC.
- Y. Zhao, H. Lv and S. Qiu, et al., Plasma metabolic profiling and novel metabolite biomarkers for diagnosing prostate cancer, RSC Adv., 2017, 7(48), 30060–30069 RSC.
- C. C. Feng, A. H. Zhang and J. H. Miao, et al., Recent advances in understanding cross-talk between Bile Acids and Gut Microbiota, Open Journal of Proteomics and Genomics, 2018, 3(1), 024–034 Search PubMed.
Footnotes |
† Electronic supplementary information (ESI) available. See DOI: 10.1039/c9ra03912k |
‡ Joint first authors. These authors contributed equally to this work. |
|
This journal is © The Royal Society of Chemistry 2019 |