DOI:
10.1039/C9RA05046A
(Paper)
RSC Adv., 2019,
9, 35969-35975
Effects of Bailing capsule on diabetic nephropathy based on UPLC-MS urine metabolomics
Received
3rd July 2019
, Accepted 23rd October 2019
First published on 5th November 2019
Abstract
Diabetic nephropathy (DN) is one of the most common microvascular diabetes complications and has become a threat to human health. Bailing capsules (BLCs), containing fermentation products of Cordyceps sinensis, have been commonly used for treatment of renal dysfunction, such as DN. However, mechanisms underlying the protective effects of BLC remain largely obscure and await more investigation. In this study, UPLC-MS-based comprehensive metabolomics along with pattern recognition was applied to explore the urine metabolic alteration of DN as well as therapeutic mechanisms of BLC. Nineteen differentially expressed endogenous metabolites were identified related to DN, which were involved in the perturbations of tyrosine metabolism, tryptophan metabolism, glycine metabolism, purine metabolism, glutamine metabolism, phenylalanine metabolism, histidine metabolism and TCA cycle metabolism pathways. After drug intervention, most of the biomarkers exhibited a certain extent towards normal levels (P < 0.05), which indicated that BLC was an effective drug for treating DN and might play its therapeutic role by retrieving abnormal metabolism pathways. The data obtained in this research may pave the way for further exploration of DN and provide key clues to understand the protective effect of BLC.
1. Introduction
Diabetic nephropathy (DN), considered as one of the most common microvascular diabetes complications, has become a threat to human health, affecting roughly one third of all diabetic individuals.1–3 In clinical practice, proteinuria and the estimated glomerular filtration rate (eGFR) are the gold standard for diagnosis and staging the degree of DN, reflecting both glomerular lesions and tubular injuries.4 Evidence indicated that the progression of DN is related to hypertension, dyslipidemia, hyperglycemia and genetic predisposition.1 However, due to the complexity of DN, the mechanism of DN is not fully understood and there is still lack of treatment that can cure or prevent DN.5,6 Therefore, it is a challenge to uncover effective treatment approaches that can specifically target the progression of DN and reveal its mechanism.
Cordyceps sinensis, also known as ‘Dong Chong Xia Cao’ (winter worm, summer grass), is a precious parasitic complex of caterpillar and fungus and one of the most valued traditional Chinese medicine.7 Since the Qing dynasty, it has been widely used as a health care product to replenish the kidney and soothe the lung for centuries.8 Recent pharmacological researches showed that Cordyceps sinensis has antioxidation activity, immunomodulating effect, anti-mutation effect and antitumour effect.9,10 Previous study also demonstrated that Cordyceps could protect against chronic kidney disease progression by affecting the TLR4/NF-κB lipid and redox signaling pathway.11 Bailing capsule (BLC), containing fermentation products of Cordyceps sinensis, has been commonly used for treatment of renal dysfunction, such as DN. However, mechanisms underlying the protective of BLC remain largely obscure and await more investigation.
Metabolomics is a modern strategy focused on low molecular weight endogenous metabolites to capture comprehensive biochemical events in biological systems by multiple analytical techniques.3,12,13 It is a powerful tool that can not only systematically search for key biomarkers for monitoring and diagnosing disease, but also offer insights into the mechanisms of pharmaceutical effects.14–17 Therefore it is beneficial to explore heterogeneous metabolic disorders such as DN and estimate the therapeutic effect of drugs.
In the present work, we performed a metabolomics study based on ultra performance liquid chromatography-mass spectrometry (UPLC-MS) to discriminate the potential biomarkers of DN. Then, we investigated the underlying therapeutic mechanisms of BLC on DN.
2. Materials and methods
2.1. Study populations
The study protocol was approved by the Institutional Ethics Committee of the 8th Medical Center of Chinese PLA General Hospital and followed the tenets of the Declaration of Helsinki. With informed consent obtaining from all patients, we recruited 48 type 2 diabetes participants for monitoring renal function change. The following two groups were defined according to the clinical course and urinary albuminuria levels: 16 patients without albuminuria (control, albumin-to-creatinine (ACR) ratio < 30 mg g−1) and 32 patients with microalbuminuria (DN; 30 < ACR < 300 mg g−1). Sixteen DN patients enrolled in the study were assigned to receive BLC treatment for 24 weeks as DN + BLC group. Sixteen clinically group-matched (age, sex, BMI, creatinine, baseline albuminuria status) DN subjects were selected as DN group. All patients received routine diabetes therapy scheme and diabetic diet. All urine samples were derived from 24 h urine collections and centrifuged (5
000g for 30 min at 4 °C) to remove cell debris. The sample was then kept at −80 °C for long-term storage.
2.2. Reagents and materials
HPLC-grade acetonitrile (ACN) and methanol were purchased from Merck (Darmstadt, Germany). All other reagents were purchased from Sigma-Aldrich (St. Louis, MO). Ultrapure water was purified with a Milli-Q purification system (Millipore, Billerica, MA). BLC was purchased from Huadong Medicine Company (Hangzhou, Zhejiang, China).
2.3. Sample preparation
To precipitate protein, four times of methanol (containing 0.01 mg mL−1 L-2-chlorophenylalanine as internal standard) was added to 100 μL aliquots of urine in 1.5 mL centrifuge tube. The mixture was vortexed vigorously for 1 min and then centrifuged at 14
000g for 15 min at 4 °C. After transferred to autosampler vial kept, 4 μL of supernatant was injected for UPLC-MS analysis. Quality control (QC) was a mixture by pooling 10 μL of each sample.
2.4. Data acquisition
Chromatographic analysis was performed on Agilent 1290 Infinity UPLC System (Palo Alto, California, USA). A Waters XSelect HSS T3 Column (2.1 mm × 100 mm, 2.5 μm, Milford, USA) was used for separation. The mobile phase consisted of solvent A (0.1% formic acid in water, v/v) and solvent B (0.1% formic acid in acetonitrile, v/v). The gradient for chromatographic analysis was as follows: 0–2 min, 3% B; 2–16 min, 3–30% B; 16–18 min, 30–95% B; 18–19.5 min, 95% B; 19.5–20 min at 95–3% B and subsequently re-equilibrated for 4 min. The flow rate was 0.4 mL min−1.
The mass range was set at m/z 50–1
000 at 1 Hz in positive mode. The parameters were employed as follows: capillary voltage of 3.5 kV and nebulizer pressure of 45 psig. The gas temperature was set to 350 °C with drying gas flow of 11 L min−1. The fragmentor voltage and skimmer voltage were set at 120 V and 60 V, respectively. The MS/MS spectra of metabolites were obtained at 0.5 spectra per s, with a medium isolation window (∼4 m/z) and collision energy range from 10–30 eV.
2.5. Data processing
The LC-MS data was converted to mzData formats via Agilent MassHunter Qualitative software. The program XCMS was applied so as to nonlinear alignment of the data and automatic integrate and extract the peak intensities. The variables presenting in at least 80% of either group were extracted.18 For further analysis, the data were normalized to the sum of all peak areas of each sample by MATLAB 7.0 software (The MathWorks, Inc, USA).
2.6. Metabolomic data analysis
To detect the potential biomarkers from different groups, the processed data were subject to partial least-squares discriminant analysis (PLS-DA) and orthogonal partial least-squares discriminant analysis (OPLS-DA) using SIMCA-P 11.0 (Umetrics, Sweden). As the variable importance in the projection (VIP) value obtained from the OPLS-DA model indicated the contribution to the classification, metabolites with VIP values were larger than 1.0 were selected for further Student's t test. The quality of the OPLS-DA models was indicated by three parameters (R2X, R2Y, and Q2Y), in which they were employed to quantify the goodness-of-fit and to assess model predictability, respectively.19,20
2.7. Identification of biomarker metabolites
The potential biomarkers were first identified by searching the experimental accurate mass and tandem mass spectra (MS/MS) in open-source database, such as METLIN (http://www.metlin.scripps.edu), HMDB (http://www.hmdb.ca), the mass bank web site (http://www.massbank.jp/en/database.html) and NIST database. Then, MS/MS and retention time (RT) of the potential biomarkers were further compared with those of reference standards.
2.8. Statistical analysis
The differences between groups were analyzed by One-way ANOVA with Tukey post hoc test on SPSS software. P values < 0.05 were regarded as statistically significant.
3. Results
3.1. Study population and samples
A total of 16 type 2 diabetes controls and 32 DN patients from the 8th Medical Center of Chinese PLA General Hospital were screened. According to the clinical and demographic characteristics, the groups were well balanced (Table 1). We observed no significant difference in age, sex, BMI, creatinine, systolic BP, diastolic BP and HbA1c (%) among three groups. The DN group showed significantly lower eGFR, higher albuminuria, and higher urine ACR compared with the control group (p < 0.05), while there was no significant difference in eGFR between the DN and DN + BLC group. Despite matching for baseline albuminuria stage, a significant difference in albuminuria levels between DN + BLC group and DN group was observed, suggesting that BLC had a protective effect in DN. In addition, urine albumin to creatinine ratio (ACR) was statistically lower in DN + BLC subjects than in DN group (p < 0.01), which also indicating that BLC had a therapeutic influence in DN (Table 1).
Table 1 Clinical characteristics in 48 patients with type 2 diabetes mellitusa
|
Control |
DN |
DN + BLC |
DN, diabetic nephropathy; BLC, Bailing capsule; eGFR, estimated glomerular filtration rate. ACR: albumin to creatinine ratio. Data reported as mean ± standard deviation (SD). Significantly altered compared to control group. Significantly (p < 0.05) altered compared to DN group. |
Number |
16 |
16 |
16 |
Male/female |
3/13 |
4/12 |
4/12 |
Age (years) |
59 ± 7 |
60 ± 6 |
61 ± 6 |
BMI (kg m−2) |
28 ± 4 |
28 ± 4 |
28 ± 4 |
Creatinine (mg dL−1) |
0.8 ± 0.1 |
0.9 ± 0.1 |
0.8 ± 0.1 |
Systolic BP (mmHg) |
119 ± 10 |
127 ± 19 |
124 ± 17 |
Diastolic BP (mmHg) |
74 ± 11 |
79 ± 13 |
77 ± 12 |
HbA1c (%) |
6.2 ± 0.8 |
6.7 ± 1.1 |
6.5 ± 1.0 |
Diabetes duration (years) |
10 ± 3 |
15 ± 4 |
14 ± 3 |
eGFR (mL min−1) |
93 ± 21 |
79 ± 20b |
88 ± 23 |
Albuminuria (mg L−1) |
0.7 ± 0.4 |
8 ± 5b |
4 ± 3c |
Urine ACR (mg g−1) |
5 ± 2 |
108 ± 46b |
44 ± 22c |
3.2. Metabolic profiles of controls and DN patients
OPLS-DA method was performed to achieve maximum separation between groups and screen out differential metabolites from a huge amount of signals. Score plot of the supervised OPLS-DA model (Fig. 1) displayed that DN group and control group were entirely isolated and distinctly separated in the first dimension, indicating that there were significant metabolic abnormalities in the DN patients. The goodness-of-fit and model predictability of the OPLS-DA models was quantified by three parameters (R2X, R2Y, and Q2Y). The performance statistics of R2X, R2Y and predictive parameter Q2Y were 0.617, 0.992 and 0.708 respectively, indicating that the model was valid and prediction.
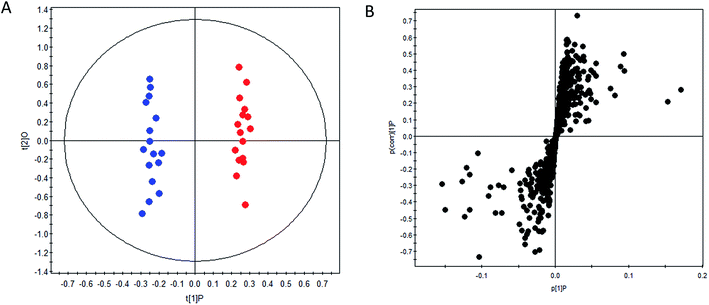 |
| Fig. 1 OPLS-DA scores plot (A) and S-plot (B) of control group ( ) and DN group ( ). | |
The corresponding S-plots and VIPs of OPLS-DA model were then created to screen out the potential metabolites that were responsible for the differences. Based on VIP threshold (VIP > 1) in OPLS-DA model and Student's t test (p < 0.05), nineteen differentially expressed metabolites enabling the separation between control group and DN group, were marked as potential biomarkers. The detailed information of these biomarkers summarized in Table 2.
Table 2 Metabolites discriminating DN and type 2 diabetes subjects by UPLC-MS analysisa
Metabolites |
Trendb |
Related pathway |
DN vs. control |
DN + BLC vs. DN |
P valuec |
FC |
P valuec |
FC |
Abbreviations: DN, diabetic nephropathy; BLC, Bailing capsule; FC, fold change. Arrow (↑) indicates relative increase in signal. Arrow (↓) indicates relative decrease in signal. The P value was calculated by one-way ANOVA and then by the Tukey post hoc test for comparisons of multiple groups. |
5-Methoxytryptophan |
↓ |
Tryptophan metabolism |
5.79 × 10−3 |
0.46 |
4.82 × 10−3 |
2.70 |
p-Hydroxyphenylacetic acid |
↑ |
Phenylalanine metabolism |
8.01 × 10−4 |
3.89 |
8.24 × 10−2 |
0.65 |
5-Hydroxy-6-methoxyindole glucuronide |
↑ |
Tryptophan metabolism |
8.09 × 10−3 |
2.22 |
3.87 × 10−3 |
0.33 |
2-Phenylglycine |
↑ |
Glycine metabolism |
5.36 × 10−3 |
2.69 |
4.79 × 10−2 |
0.49 |
Indoleacetyl glutamine |
↑ |
Tryptophan metabolism |
4.06 × 10−5 |
3.60 |
5.53 × 10−2 |
0.75 |
Indoxyl glucuronide |
↑ |
Tryptophan metabolism |
2.75 × 10−2 |
2.72 |
5.47 × 10−3 |
0.50 |
L-Histidine |
↓ |
Histidine metabolism |
6.80 × 10−3 |
0.38 |
6.10 × 10−3 |
3.04 |
Uric acid |
↑ |
Purine metabolism |
3.32 × 10−4 |
2.87 |
2.75 × 10−2 |
0.39 |
2-Phenylacetamide |
↑ |
Phenylalanine metabolism |
3.82 × 10−2 |
2.01 |
9.16 × 10−2 |
0.82 |
Phenyl glucuronide |
↑ |
|
8.31 × 10−3 |
2.50 |
1.00 × 10−2 |
0.47 |
Purine |
↑ |
Purine metabolism |
3.90 × 10−2 |
1.86 |
8.88 × 10−2 |
0.59 |
L-Tryptophan |
↓ |
Tryptophan metabolism |
3.68 × 10−2 |
0.47 |
1.77 × 10−2 |
1.73 |
Hippuric acid |
↑ |
Glycine metabolism |
7.22 × 10−5 |
2.91 |
6.79 × 10−3 |
0.64 |
Phenylacetylglutamine |
↑ |
Glutamine metabolism |
3.79 × 10−2 |
2.01 |
9.53 × 10−2 |
0.70 |
4-Hydroxyhippuric acid |
↑ |
Glycine metabolism |
5.81 × 10−3 |
2.52 |
4.38 × 10−2 |
0.53 |
Indoxylsulfuric acid |
↑ |
Tryptophan metabolism |
4.29 × 10−2 |
2.14 |
2.26 × 10−2 |
0.62 |
p-Cresol sulfate |
↑ |
Tyrosine metabolism |
1.93 × 10−2 |
1.56 |
3.10 × 10−2 |
0.55 |
p-Cresol glucuronide |
↑ |
Tyrosine metabolism |
3.92 × 10−3 |
3.44 |
1.45 × 10−2 |
0.48 |
Citric acid |
↓ |
TCA cycle |
9.53 × 10−3 |
0.47 |
1.47 × 10−2 |
2.25 |
3.3. Effect of BLC treatment
The therapeutic effect of BLC in DN was confirmed as exhibited in the clinical characteristics alteration, including albuminuria and urine ACR. To further reveal the mechanisms of BLC, 19 significantly altered metabolites connected with DN were regarded as the monitoring indicators to establish model for analysis. This newly built PLS-DA model was established to further uncover the metabolic alterations between these groups. The data of the PLS-DA model showed that R2 Y = 0.801 and Q2 (cum) = 0.739, which suggested the model was robust and reliable. As shown in Fig. 2, a distinct separation of the DN group and control group was obtained, while the BLC treatment group had the tendency to resume to the control group, demonstrating that BLC exhibited a certain extent of treatment effect on DN. The results suggested that the metabolic profiling was ameliorated with the BLC therapy, which could modify the dysregulated metabolites caused by DN and return them to normal levels.
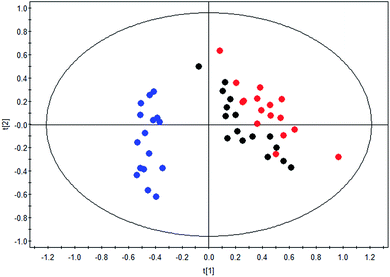 |
| Fig. 2 PLS-DA scores plot of nineteen metabolites from control group ( ), DN group ( ) and DN + BLC group ( ). | |
Furthermore, one-way ANOVA analysis was accomplished among the three groups to evaluate the recovery situation of the altered metabolites by treatment with BLC (Table 2). The relative peak intensity of the 19 potential biomarkers is shown in Fig. 3. Following BLC treatment, the levels of fourteen metabolites including 5-methoxytryptophan, 5-hydroxy-6-methoxyindole glucuronide, 2-phenylglycine, indoxyl glucuronide, L-histidine, uric acid, phenyl glucuronide, L-tryptophan, hippuric acid, 4-hydroxyhippuric acid, indoxylsulfuric acid, p-cresol sulfate, p-cresol glucuronide and citric acid were obviously improved in DN + BLC group in comparison with DN group. The other five metabolites were also ameliorated to some degrees. Then, overview of the metabolites and major metabolic pathways changes in DN was built by exploring KEGG PATHWAY Database (Fig. 4). All the results above indicated that the protective effect of BLC on DN may act by partially resuming the disequilibrium of tyrosine metabolism, tryptophan metabolism, glycine metabolism, purine metabolism, glutamine metabolism, phenylalanine metabolism, histidine metabolism and TCA cycle metabolism pathways.
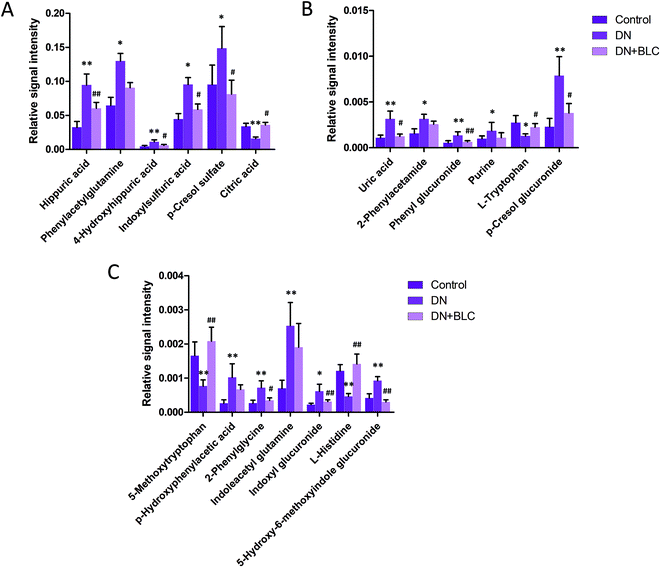 |
| Fig. 3 Bar plots show UPLC-MS relative signal intensities for candidate metabolites (A–C) in control, DN and DN + BLC groups. Data are expressed as mean ± S.D. (DN vs. control group, *p < 0.05, **p < 0.01; DN + BLC vs. DN group, #p < 0.05, ##p < 0.01). | |
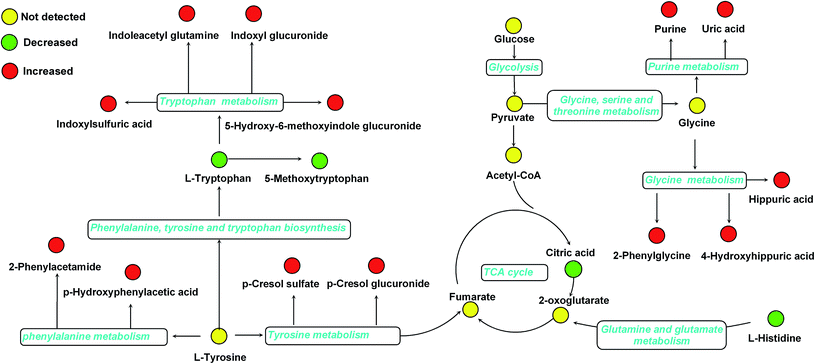 |
| Fig. 4 Structure of metabolism pathway networks involved in DN patients. The metabolites are shown in color: red represents increased metabolite, green represents decreased metabolites compared to controls and yellow represents no detected metabolites, respectively. The blue italic words are pathway's names. | |
4. Discussion
The local and traditional uses of Cordyceps sinensis are replenishing the kidney and soothing the lung.8 Recent pharmacological stated that it possesses widely pharmacological activities, including kidney protection, immunoregulation, antioxidation and anti-cancer.9,10 Bailing capsule (BLC), containing fermentation products of Cordyceps sinensis, has been commonly used for treatment of renal dysfunction.11 But the mechanisms underlying the protective of BLC are still largely unknown. In our study, metabolomics investigation combined with OPLS-DA analysis was used to discriminate the potential biomarkers of DN and investigate the underlying therapeutic mechanisms of BLC. Nineteen potential biomarkers were identified, which were associated with tyrosine metabolism, tryptophan metabolism, glycine metabolism, purine metabolism, glutamine metabolism, phenylalanine metabolism, histidine metabolism and TCA cycle metabolism pathways. Based on the alteration of biomarkers after BLC treatment, it was confirmed that BLC might play its therapeutic role by retrieving abnormal metabolism pathways.
A distinct tryptophan metabolism was observed in DN group, in which six metabolites were significantly altered. Among them, L-tryptophan and 5-methoxytryptophan were significantly decreased in DN patients compared to the controls, while, 5-hydroxy-6-methoxyindole glucuronide, indoleacetyl glutamine, indoxyl glucuronide and indoxylsulfuric acid were significantly elevated. Increasing evidence showed that disturbed tryptophan metabolism was associated with the decline in eGFR and tryptophan related metabolites could be potential prognostic markers for DN.21–23 The present investigation added new evidence that tryptophan metabolism is a key metabolic pathway in the progression of DN. As a rate-limiting enzyme in tryptophan catabolism, indoleamine 2,3-dioxygenase was a key player in immunoregulatory of chronic inflammation and renal injury.4,24 It was reported that indoleamine 2,3-dioxygenase activity was induced in patients with type 2 DN, which may lead to the decreased level of tryptophan and increased level of oxidation metabolites of tryptophan in DN. Meanwhile, it has been noticed that several glucuronides, such as 5-hydroxy-6-methoxyindole glucuronide, indoxyl glucuronide, phenyl glucuronide and p-cresol glucuronide, were significantly upregulated in the patients with DN, which was in accordance with previous research.25 Since glucuronidation could assist the excretion of toxic substances, the elevated glucuronides might reflect the process of toxins elimination when kidney was injured. After BLC treatment, five of the six tryptophan metabolites and all glucuronides were dramatically revised and restored to normal levels, which indicated that BLC may play its protective role in DN through rebalancing tryptophan metabolism and reducing glucuronides.
In our differentially expressed metabolites, p-cresol sulfate and p-cresol glucuronide belonging to the tyrosine metabolism were remarkably elevated in patients with DN. It was reported that p-cresol sulfate was inversely related to eGFR in a large population base cohort with minimal renal function decline and could be early markers of renal function decline,26 which indirectly supported our results. Cresols are generated endogenously from tyrosine metabolism by microflora in colon.27 Obvious imbalanced tyrosine and tryptophan metabolism indicated that microbial metabolism of amino acids in intestinal flora played an important role in DN. In DN + BLC group, both of the cresols were significantly decreased and had the tendency to resume to normal levels, which suggested that BLC may relieve DN by retrieving the gut microbial and repairing abnormal tyrosine metabolism pathway.
Interestingly, several uremic toxins, including hippuric acid, 4-hydroxyhippuric acid, indoxylsulfuric acid and p-cresol sulfate, were significantly upregulated in DN patients. Earlier finding showed that serum uremic toxins have a significant role in the vascular disease and higher mortality observed in chronic kidney disease patients.28 Moreover, a recent research demonstrated that uremic toxins, such as indoxyl sulfate and p-cresol sulfate, directly promote vascular calcification via activation of inflammation and coagulation pathways and were strongly associated with impaired glucose homeostasis,29 which partially explained the phenomenon of elevated uremic toxins observed in our study. In general, main elimination pathway of uremic toxins was via urinary excretion with active tubular secretion.30 However, when DN occurred and renal function was compromised, elimination of uremic toxins became impaired, which lead to increased levels of uremic toxins in urine. After BLC administration, the levels of all abnormal uremic toxins remarkably declined and exhibited a certain degree of normalization, which indicated that reducing accumulation of uremic toxins may play an important role in BLC's therapeutic effects.
The levels of uric acid and purine were found to be significantly higher in urine of DN patients, which were mapped into purine metabolism. As final enzymatic product in the degradation of purine nucleosides, it has been reported uric acid is involved in various stages of DN onset and progression.31 In the meantime, there is evidence that purine metabolism related metabolites including xanthine, xanthosine, guanosine and hypoxanthine were upregulated in DN and diabetic patients,32,33 which was in agreement with our results. It was also reported that an overactivated purine metabolism was observed in DN and it could be normalized by the specific mitochondrial complex-1 inhibitor, which supported our results.34 Purine metabolism has been shown to increase the activation of mitochondrial defense mechanisms under oxidative stress conditions, such as diabetes and renal injury.33 In addition, a recent study found that the overactivation of mitochondrial activity in the kidney could contribute to metabolic disorders and the pathogenesis of early DN. Therefore, the increased uric acid and purine in DN patients may be related to mitochondrial antioxidant defense processes. It was observed that concentration of uric acid and purine return to normal after BLC treatment, which suggested that BLC may attenuate DN by rebalancing the purine metabolism pathway.
5. Conclusion
In summary, comprehensive metabolomics along with pattern recognition was applied to explore the metabolic alteration of DN as well as therapeutic mechanisms of BLC. Nineteen differentially expressed endogenous metabolites were identified related to DN, which were involved in the perturbations of tyrosine metabolism, tryptophan metabolism, glycine metabolism, purine metabolism, glutamine metabolism, phenylalanine metabolism, histidine metabolism and TCA cycle metabolism. After drug intervention, most of the biomarkers exhibited a certain extent towards normal levels (P < 0.05), which indicated that BLC was an effective drug for treating DN and might play its therapeutic role by retrieving abnormal metabolism pathways. The data obtained in this research may pave the way for further exploration of DN and provide key clues to understand the protective effect of BLC. In the future, we will try to discover the underlying mechanism regarding how BLC regulates the disordered metabolism pathways.
Conflicts of interest
The authors declare no conflict of interest.
Acknowledgements
This research was supported by National Natural Science Foundation of China (81800660, 81770745, 81570679, 81200547) and Beijing NOVA program (Z161100004916141).
References
- M. Lv, Z. Chen, G. Hu and Q. Li, Drug Discovery Today, 2015, 20, 332–346 CrossRef CAS.
- X. Xiang, H.-D. Cai, S.-l. Su, X.-x. Dai, Y. Zhu, J.-m. Guo, H. Yan, S. Guo, W. Gu, D.-w. Qian, Z.-s. Tang and J.-a. Duan, Pharmacol. Res., 2019, 139, 26–40 CrossRef CAS.
- M. J. Pena, H. J. L. Heerspink, M. E. Hellemons, T. Friedrich, G. Dallmann, M. Lajer, S. J. L. Bakker, R. T. Gansevoort, P. Rossing, D. Zeeuw and S. S. Roscioni, Diabetic Med., 2014, 31, 1138–1147 CrossRef CAS.
- Y. Liu, X. Chen, Y. Liu, T. Chen, Q. Zhang, H. Zhang, Z. Zhu, Y. Chai and J. Zhang, Chem.-Biol. Interact., 2019, 108815, DOI:10.1016/j.cbi.2019.108815.
- J. Brenneman, J. Hill and S. Pullen, Bioorg. Med. Chem. Lett., 2016, 26, 4394–4402 CrossRef CAS.
- K. M. Sas, A. Karnovsky, G. Michailidis and S. Pennathur, Diabetes, 2015, 64, 718–732 CrossRef CAS.
- X. Li, F. Wang, Q. Liu, Q. Li, Z. Qian, X. Zhang, K. Li, W. Li and C. Dong, BMC Genomics, 2019, 20, 337 CrossRef.
- H. Zhang, Y. Li, J. Mi, M. Zhang, Y. Wang, Z. Jiang and P. Hu, Molecules, 2017, 22, 1800 CrossRef.
- M. G. Shashidhar, P. Giridhar, K. Udaya Sankar and B. Manohar, J. Funct. Foods, 2013, 5, 1013–1030 CrossRef CAS.
- X. Zhou, Z. Gong, Y. Su, J. Lin and K. Tang, J. Pharm. Pharmacol., 2009, 61, 279–291 CrossRef CAS.
- T. Sun, W. Dong, G. Jiang, J. Yang, J. Liu, L. Zhao and P. Ma, Oxid. Med. Cell. Longevity, 2019, 2019, 16 Search PubMed.
- Y. Liu, Z. Hong, G. Tan, X. Dong, G. Yang, L. Zhao, X. Chen, Z. Zhu, Z. Lou, B. Qian, G. Zhang and Y. Chai, Int. J. Cancer, 2014, 135, 658–668 CrossRef CAS.
- R. O. Bahado-Singh, R. Akolekar, R. Mandal, E. Dong, J. Xia, M. Kruger, D. S. Wishart and K. Nicolaides, Am. J. Obstet. Gynecol., 2013, 208, 371 Search PubMed.
- Y.-H. You, T. Quach, R. Saito, J. Pham and K. Sharma, J. Am. Soc. Nephrol., 2016, 27, 466–481 CrossRef CAS.
- K. Sharma, B. Karl, A. V. Mathew, J. A. Gangoiti, C. L. Wassel, R. Saito, M. Pu, S. Sharma, Y.-H. You, L. Wang, M. Diamond-Stanic, M. T. Lindenmeyer, C. Forsblom, W. Wu, J. H. Ix, T. Ideker, J. B. Kopp, S. K. Nigam, C. D. Cohen, P.-H. Groop, B. A. Barshop, L. Natarajan, W. L. Nyhan and R. K. Naviaux, J. Am. Soc. Nephrol., 2013, 24, 1901–1912 CrossRef CAS.
- Y. Liu, S. Lu, L. Zhao, X. Dong, Z. Zhu, Y. Jin, H. Chen, F. Lu, Z. Hong and Y. Chai, Anal. Bioanal. Chem., 2018, 410, 3325–3335 CrossRef CAS.
- M. Zhang, Y. Liu, M. Liu, B. Liu, N. Li, X. Dong, Z. Hong and Y. Chai, Metabolomics, 2019, 15, 13 CrossRef.
- S. Bijlsma, I. Bobeldijk, E. R. Verheij, R. Ramaker, S. Kochhar, I. A. Macdonald, B. van Ommen and A. K. Smilde, Anal. Chem., 2005, 78, 567–574 CrossRef.
- S. Wiklund, E. Johansson, L. Sjöström, E. J. Mellerowicz, U. Edlund, J. P. Shockcor, J. Gottfries, T. Moritz and J. Trygg, Anal. Chem., 2008, 80, 115–122 CrossRef CAS.
- E. S. Ong, L. Zou, S. Li, P. Y. Cheah, K. W. Eu and C. N. Ong, Mol. Cell. Proteomics, 2010 DOI:10.1074/mcp.m900551-mcp200.
- C. A. Chou, C. N. Lin, D. T. Y. Chiu, I. W. Chen and S. T. Chen, J. Diabetes Invest., 2018, 9, 366–374 CrossRef CAS.
- F. M. van der Kloet, F. W. A. Tempels, N. Ismail, R. van der Heijden, P. T. Kasper, M. Rojas-Cherto, R. van Doorn, G. Spijksma, M. Koek, J. van der Greef, V. P. Mäkinen, C. Forsblom, H. Holthöfer, P. H. Groop, T. H. Reijmers and T. Hankemeier, Metabolomics, 2012, 8, 109–119 CrossRef CAS.
- E. P. Rhee, A. Souza, L. Farrell, M. R. Pollak, G. D. Lewis, D. J. R. Steele, R. Thadhani, C. B. Clish, A. Greka and R. E. Gerszten, J. Am. Soc. Nephrol., 2010, 21, 1041–2051 CrossRef CAS.
- P. Puccetti and U. Grohmann, Nat. Rev. Immunol., 2007, 7, 817 CrossRef CAS.
- T. Zhao, H. Zhang, T. Zhao, X. Zhang, J. Lu, T. Yin, Q. Liang, Y. Wang, G. Luo, H. Lan and P. Li, J. Pharm. Biomed. Anal., 2012, 60, 32–43 CrossRef CAS.
- C. Barrios, M. Beaumont, T. Pallister, J. Villar, J. K. Goodrich, A. Clark, J. Pascual, R. E. Ley, T. D. Spector, J. T. Bell and C. Menni, PLoS One, 2015, 10, e0134311 CrossRef.
- K. K. Pasikanti, K. Esuvaranathan, Y. Hong, P. C. Ho, R. Mahendran, L. Raman Nee Mani, E. Chiong and E. C. Y. Chan, J. Proteome Res., 2013, 12, 3865–3873 CrossRef CAS.
- F. C. Barreto, D. V. Barreto, S. Liabeuf, N. Meert, G. Glorieux, M. Temmar, G. Choukroun, R. Vanholder and Z. A. Massy, Clin. J. Am. Soc. Nephrol., 2009, 4, 1551–1558 CrossRef CAS.
- B. Opdebeeck, S. Maudsley, A. Azmi, A. De Maré, W. De Leger, B. Meijers, A. Verhulst, P. Evenepoel, P. C. D'Haese and E. Neven, J. Am. Soc. Nephrol., 2019, 30, 751–766 CrossRef CAS PubMed.
- Y. Tsutsumi, T. Deguchi, M. Takano, A. Takadate, W. E. Lindup and M. Otagiri, J. Pharmacol. Exp. Ther., 2002, 303, 880–887 CrossRef CAS.
- D. I. Jalal, D. M. Maahs, P. Hovind and T. Nakagawa, Semin. Nephrol., 2011, 31, 459–465 CrossRef CAS.
- S. Kalim, C. B. Clish, J. J. Deferio, G. Ortiz, A. S. Moffet, R. E. Gerszten, R. Thadhani and E. P. Rhee, BMC Nephrol., 2015, 16, 98 CrossRef.
- C.-J. Chen, W.-L. Liao, C.-T. Chang, Y.-N. Lin and F.-J. Tsai, J. Proteome Res., 2018, 17, 3997–4007 CrossRef CAS.
- M. Wu, S. Li, X. Yu, W. Chen, H. Ma, C. Shao, Y. Zhang, A. Zhang, S. Huang and Z. Jia, Am. J. Physiol. Renal. Physiol., 2019, 317, F593–F605 CrossRef CAS.
Footnote |
† These authors contributed equally to this work. |
|
This journal is © The Royal Society of Chemistry 2019 |
Click here to see how this site uses Cookies. View our privacy policy here.