DOI:
10.1039/C9RA06146K
(Paper)
RSC Adv., 2019,
9, 31413-31424
Screening of molecular targets and construction of a ceRNA network for oxaliplatin resistance in colorectal cancer†
Received
7th August 2019
, Accepted 24th September 2019
First published on 2nd October 2019
Abstract
Oxaliplatin resistance reduces the efficacy of chemotherapy for colorectal cancer (CRC). This study aimed to screen molecular targets of oxaliplatin resistance in CRC to construct a ceRNA network. The differentially expressed mRNA and lncRNA between the oxaliplatin-resistant and oxaliplatin-sensitive colon cancer cell lines was determined using RNA sequencing data (no. GSE42387) from the NCBI GEO database. Gene Ontology BP (biological process) and KEGG pathway enrichment analyses were used to analyze the function and pathway enrichment of the differentially expressed mRNA and lncRNA. The lnCeDB and starBase v2.0 were used to predict miRNA, and Cytoscape software was used to build a ceRNA network. The top 5 mRNA, miRNAs, and lncRNAs with high degrees of connectivity in the ceRNA network were validated by qPCR. TCGA colon cancer clinical data was used to perform a survival analysis of patients with differential mRNA and lncRNA expression. Between the two groups, 2515 mRNAs and 23 lncRNAs were differentially expressed. We constructed a ceRNA network containing 503 lncRNA–miRNA–mRNA regulatory pairs, 210 lncRNA–miRNA pairs, 382 miRNA–mRNA pairs, and 212 mRNA co-expression pairs. The differentially expressed lncRNA, miRNA and mRNA were verified by qPCR. One lncRNA (HOTAIR) and 14 mRNAs significantly correlated with patient prognosis. The discovery of differentially expressed genes and the construction of ceRNA networks will provide important resources for the search for therapeutic targets of oxaliplatin resistance. Moreover, this resource will aid the discovery of the mechanisms behind this type of drug resistance.
1 Introduction
New cases of colorectal cancer (CRC) and CRC-related deaths are expected to increase by 60% in the next few decades, and more than 2.2 million new cases and 1.1 million deaths are expected by 2030.1 The standard of care for CRC involves treatment with oxaliplatin, via chemotherapy regimens such as FOLFOX and CapeOX, as the first-line therapy for CRC. The median survival of patients with CRC receiving oxaliplatin significantly improves according to NCCN guidelines.2 Although a variety of small molecule targeted drugs are widely used in clinical practice, oxaliplatin, a third-generation platinum drug, has not yet been challenged as the first-line therapy for CRC.3,4
Oxaliplatin is as an alkylating agent with the molecular formula 1,2-diaminocyclohexane (DACH), and can form DACH–DNA adducts and produce inter- or intra-chain crosslinks. This prevents the separation of DNA strands during transcription and translation, inducing cancer cell apoptosis.5–8
Cancer cells can develop resistance to oxaliplatin. Oxaliplatin resistance can be divided into two categories: congenital drug resistance and acquired drug resistance.9 Congenital resistance is the insensitivity of patients to oxaliplatin due to factors present at birth, and acquired resistance refers to the repeated treatment with oxaliplatin, leading to the gradual development of resistance in patients.
Current studies suggest that oxaliplatin resistance in CRC may be related to the following mechanisms: (i) DNA adduct formation, the mechanism of oxaliplatin's anticancer activity, can be repaired by enhanced DNA repair capacity (DRC) and increased DNA-like adducts.10,11 (ii) The overexpression of transporters increases the excretion of oxaliplatin from the cells, reducing the intracellular drug concentration to reduce oxaliplatin's cytotoxic effects.12,13 Copper transporters are involved in the accumulation of oxaliplatin,14 and the expression levels of organic cation transporters (OCT) positively correlate with oxaliplatin absorption and resistance.15,16 ABC transporters, such as multidrug resistance proteins (MRPs) and sodium potassium ATPases, are also involved in oxaliplatin drug resistance.17–20 (iii) Glutathione (GSH)-mediated oxaliplatin effusion reduces the amount of oxaliplatin in cancer cells. In this mechanism, oxaliplatin reaches the cytoplasm and forms a hydrate, which reacts with mercapto-containing molecules such as glutathione.21,22
In recent years, gene engineering technology, such as antisense oligodeoxyribonucleotides (AOD), RNA interference (RNAi) and antisense RNA technology, has been applied to reverse drug resistance in cancer. The molecular targets and pathways that induce oxaliplatin resistance are the prerequisites for the application of genetic engineering technology. Therefore, the present study identified oxaliplatin resistance-associated RNAs by screening the transcriptome of oxaliplatin resistant cell lines. The differentially expressed genes obtained by bioinformatics analysis were further validated to explore the molecular mediators of oxaliplatin resistance.
The ceRNA (competing endogenous RNA) hypothesis is a novel theory of RNA interactions, which states that ceRNA can bind to microRNA via microRNA response elements (MREs) and thus influence molecular signaling pathways.23 Recent evidence has highlighted the crucial regulatory roles of ceRNA networks in colorectal cancer.24,25 Many studies have shown that multiple lncRNAs, such as CACS15,26 KCNQ1OT1,27 and BLACAT1,28 and multiple miRNAs, such as miR-625-3p,29 miR-34a,30 miR-483-3p,31 and miR-122,32 are associated with oxaliplatin resistance. In this study, the ceRNA network was constructed based on RNA information obtained from bioinformatics analyses. The construction of this ceRNA network may lead to the identification of new players in oxaliplatin resistance mechanisms for future studies.
2 Materials and methods
2.1. Screening and analysis of oxaliplatin resistance genes in colon cancer cell lines
2.2.1. Data acquisition and RNA re-annotation.
RNA data (no. GSE42387) (Homo sapiens) from the NCBI GEO database (Gene Expression Omnibus, GEO, http://www.ncbi.nlm.nih.gov/geo/) was acquired (data download time: March 2019). This data set contains three oxaliplatin-sensitive lines (HCT116, HT29, and LoVo) and oxaliplatin-resistant CRC cell lines. The oxaliplatin-resistant cell lines were generated by treating cells with oxaliplatin for 9 months. The treatment of each cell line was repeated 3 times for a total of 18 samples. The whole genome from all samples was tested using Agilent-014850 Whole Human Genome Microarray 4x44K G4112F (Agilent Systematic Name, collapsed probe version). The GSE42387_series_matrix.txt was downloaded and the preprocessed and the quantile normalized gene expression matrix file was acquired. The platform annotation file was downloaded to match the probe and gene symbol. The mean value of different probes was presented as the final expression value of the targeted gene if different probes mapped to the same gene. The RNA described as “protein_coding” was labeled as mRNA, and the RNA described as “antisense”, “sense_intronic”, “lincRNA”, “sense_overlapping”, or “processed_transcript” was labeled as lncRNA according to the human gene annotation files (Release 29) provided by GENCODE.
2.2.2. Differential RNA analysis.
The differential mRNA and lncRNA expression in oxaliplatin-resistant and oxaliplatin-sensitive CRC cell lines was analyzed using the classic bayesian method from the limma packet33 (Version 3.10.3, http://www.bioconductor.org/packages/2.9/bioc/html/limma.html). A p-value < 0.05 and |log
FC| > 0.585 (1.5-fold change) were used as the threshold for differential RNA expression.
2.2.3. Functional enrichment and pathway analyses of differential mRNA expression.
The DAVID34 (Version6.8, https://david-d.ncifcrf.gov/) was used to perform the Gene Ontology BP (biological process)35 functional annotation and KEGG36 pathway enrichment analysis involving in the differential RNA expression between oxaliplatin-resistant and oxaliplatin-sensitive CRC cell lines. A p-value < 0.05 and enrichment count ≥ 5 were considered as significant enrichment results.
2.2.4. Co-expression analysis of lncRNA and mRNA and functional prediction of lncRNA.
The Pearson correlation test was used to analyze the differential lncRNA and mRNA expression between oxaliplatin-resistant and oxaliplatin-sensitive CRC cell lines. The synergistic relationship between the expression of lncRNA and mRNA was used for construction of the ceRNA network. The related pairs with r > 0.7 and p-value < 0.05 were screened. This co-expression analysis of lncRNA and mRNA suggested that mRNA was a potential target of lncRNA. The KEGG pathway enrichment analysis was conducted on the mRNAs corresponding to lncRNAs using the R Package Cluster Profiler37 (version 3.8.1, http://bioconductor.org/packages/release/bioc/html/clusterProfiler.html), and the results of the enrichment analysis were used to predict the function of lncRNA. A p-value < 0.05 was considered to indicate a significant enrichment.
2.2. miRNA prediction and construction of the ceRNA network
Based on the above lncRNA–mRNA relationship pairs, the lncRNA–miRNA relationship pairs were further predicted using lnCeDB38 and starBase v2.0 (ref. 39) online databases. The miRNA–mRNA relationship pairs were predicted using miRWalk2.0 (ref. 40) (http://zmf.umm.uni-heidelberg.de/apps/zmf/mirwalk2/) and six databases including miRWalk, miRanda, miRDB, PITA, RNA22, and Targetscan. The lncRNA–miRNA–mRNA complexes regulated by the same miRNA were screened based on the above mRNA–miRNA and lncRNA–miRNA pairs (correlation coefficient > 0.7). The ceRNA network was built using the Cytoscape software (version 3.4.0, http://chianti.ucsd.edu/cytoscape-3.4.0/).41 The lncRNA and mRNA regulated by the same miRNA in the ceRNA network were considered as ceRNA. The connectivity of each node in the ceRNA network was analyzed using CytoNCA42 (Version 2.1.6, http://apps.cytoscape.org/apps/cytonca).
2.3. Verification of oxaliplatin resistance genes in colon cancer cell lines
2.3.1. Cell culture.
The human colorectal cancer cell lines HCT-116, HCT-8 (obtained from the Cell Bank of Type Culture Collection of Chinese Academy of Sciences, Shanghai, China), and oxaliplatin-resistant cell lines HCT-116/L and HCT-8/L (Shanghai Kongene Biotechnology Co. Ltd., Shanghai, China) were cultured in RPMI-1640 (Gibco; Thermo Fisher Scientific, Inc., Waltham, MA, USA) mixed with 10% heat-inactivated fetal bovine serum (FBS; Gibco, Thermo Fisher Scientific, Inc.). The cells were incubated in a humidified incubator (Sanyo XD-101; Sanyo Electric Co., Ltd., Osaka, Japan) at 37 °C and 5% CO2.
2.3.2. qPCR analysis.
The qRT-PCR assays were performed using HCT-116, HCT-8, HCT-116/L, and HCT-8/L cell lines. Total RNA was extracted from the cells using TRIzol reagent (Invitrogen; Thermo Fisher Scientific, Inc).
(i) qPCR analysis of mRNA: the cDNA was synthesized using the cDNA synthesis kit (RR047A, TAKARA BIO INC. Japan) in a reaction containing 5× gDNA Eraser Buffer (2 μl), gDNA Eraser (1 μl), RNA (1 μg), 5× primer Script Buffer (4 μl), RT Enzyme Mix 1 (1 μl), RT primers (1 μl), and nuclease-free water (11 μl) according to the manufacturer's instructions. The primers and thermocycling conditions are shown in ESI 1.† PCR reactions were performed in 20 μl containing SYBR Green Mix (10 μl), Forward Primer Mix (1 μl), Reverse Primer Mix (1 μl), nuclease-free water (3 μl) and cDNA (5 μl).
(ii) qPCR analysis of microRNA: the cDNA was synthesized using a cDNA synthesis kit (KR211; Tiangen Biotech Co., Ltd, Beijing, CA) in reactions containing 2× miRNA RT Reaction Buffer (10 μl), miRNA RT Enzyme Mix I (2 μl), RNA (1 μg) and nuclease-free water (7 μl) according to the manufacturer's instructions. The primers and thermocycling conditions are shown in ESI 1.† PCR reactions were performed in 20 μl total (FP411; TIANGEN biotech CO., LTD, Beijing, CA.) containing 2× miR Cute Mix (10 μl), Forward Primer Mix (1 μl), Reverse Primer Mix (1 μl), nuclease-free water (3 μl) and cDNA (5 μl).
(iii) qPCR analysis of lncRNA: the cDNA was synthesized with a cDNA synthesis kit (RR047A, TAKARA BIO INC. Japan) in reactions containing 5× gDNA Eraser Buffer (2 μl), gDNA Eraser (1 μl), RNA (1 μg) and 5× primer Script Buffer (4 μl), RT Enzyme Mix 1 (1 μl), RT primers (1 μl) and nuclease-free water (10 μl) according to the manufacturer's protocol. The primers and thermocycling conditions are shown in ESI 1.† PCR reactions were performed in 20 μl total volume containing SYBR Green Mix (10 μl), Forward Primer Mix (1 μl), Reverse Primer Mix (1 μl), nuclease-free water (3 μl) and cDNA (5 μl).
PCR reactions were performed using the FTC-3000 real-time fluorescence quantitative PCR system (Funglyn Biotech, Inc., Ontario, Canada). The experiment was repeated three times. The relative quantification was calculated by the 2−ΔΔCt method with the reference gene and control cells were used as the baseline.43 The 18s RNA was used as a reference gene for detecting mRNA and lncRNA, and U6 was used as a reference gene for detecting miRNA.
2.4. Survival analysis of oxaliplatin resistance genes
Data for survival analysis was acquired from the UCSC database44 (http://xena.ucsc.edu/). Survival information of patients with colon cancer (COAD) and gene expression RNAseq profiles (FPKM) were matched one-to-one. Finally, 435 cancer samples with survival information were obtained (data download time: March 2019). The TCGA colon cancer clinical data from the UCSC database and clinical information related to prognosis, including overall survival (OS) and OS status data, were sorted and analyzed. The mRNA and lncRNA from the above ceRNA network were identified as candidate genes. The expression level of candidate genes was screened from TCGA. Clinical samples were divided into two groups of high expression and low expression according to their median expression value. The log-rank statistical test was used to analyze the relationship between candidate genes and prognosis. The threshold was set as p-value < 0.05. A Kaplan–Meier survival curve was then plotted.
3 Results
3.1. Differential expression of mRNA and lncRNA
A total of 16
571 individual mRNAs and 245 lncRNAs were annotated. The differences in mRNA and lncRNA expression between oxaliplatin-resistant and oxaliplatin-sensitive colon cancer cell lines were analyzed. The analysis identified 2515 differentially expressed mRNAs and 23 differentially expressed lncRNAs.
The amount of differentially expressed mRNA and lncRNA is shown in Table 1. The VEEN chart in panels (A) and (B) of Fig. 1 show the amount of differentially expressed mRNA and lncRNA, respectively, among the three colon cancer cell lines. The heatmaps in panels (C) and (D) of Fig. 1 show the relative expression level of differential mRNA and lncRNA, respectively, among the three colon cancer cell lines. The results suggest that the expression of the following lncRNAs was significantly different: MIAT, ZNF503-AS1, EMX2OS, TTY15, DIO3OS, TP53TG1, MCF2L-AS1, MIRLET7BHG, CRNDE, HNF1A-AS1, HOTAIR, HOTAIRM1, TUG1, LINCO0239, LNC00346, C8orf31, SPATA13, LINC00326, LINC00467, ELFN2, H19, and SFTA1P.
Table 1 Amount of differentially expressed mRNA and lncRNA
Cell lines |
Type |
mRNA |
lncRNA |
HCT116 |
Up |
382 |
4 |
Down |
293 |
4 |
Total |
675 |
8 |
HT29 |
Up |
589 |
2 |
Down |
682 |
5 |
Total |
1271 |
7 |
LoVo |
Up |
484 |
2 |
Down |
559 |
6 |
Total |
1043 |
8 |
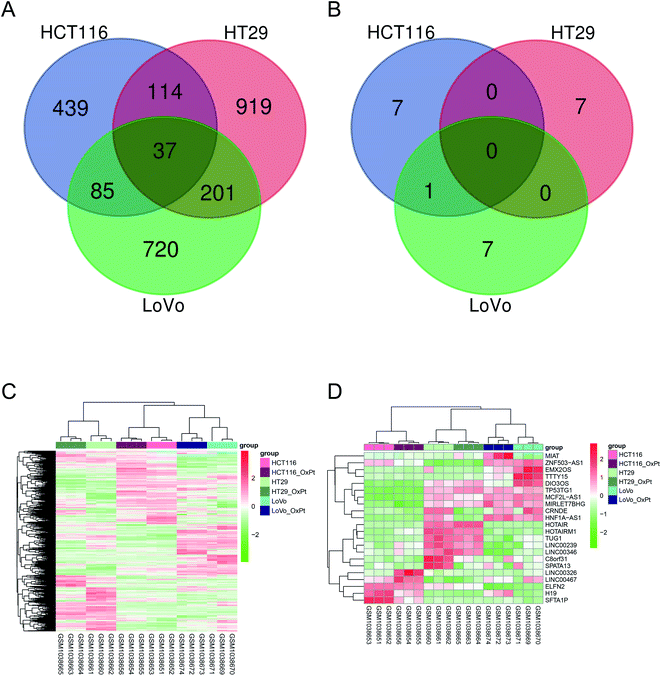 |
| Fig. 1 Differentially expressed mRNAs and lncRNAs for oxaliplatin resistance. This figure shows the differentially expressed mRNA and lncRNA between oxaliplatin-resistant and oxaliplatin-sensitive colon cancer cell lines. Panels (A) and (B) show the amount of differentially expressed mRNA and lncRNA, respectively, among the three colon cancer cell lines. Panels (C) and (D) show the relative expression of the differentially expressed mRNA and lncRNA, respectively, among the three colon cancer cell lines. | |
3.2. Functional and pathway enrichment analysis of differentially expressed RNAs
GO BP functional enrichment and KEGG pathway enrichment analyses were carried out on the 2515 differentially expressed mRNAs. The results showed that 228 GO BPs and 39 KEGG pathways differed significantly. Panel (A) of Fig. 2 shows the GO BP and KEGG pathway ranked by the top 10 p-values. The results suggested that a large number of genes were enriched in metabolic pathways and oxidation–reduction processes.
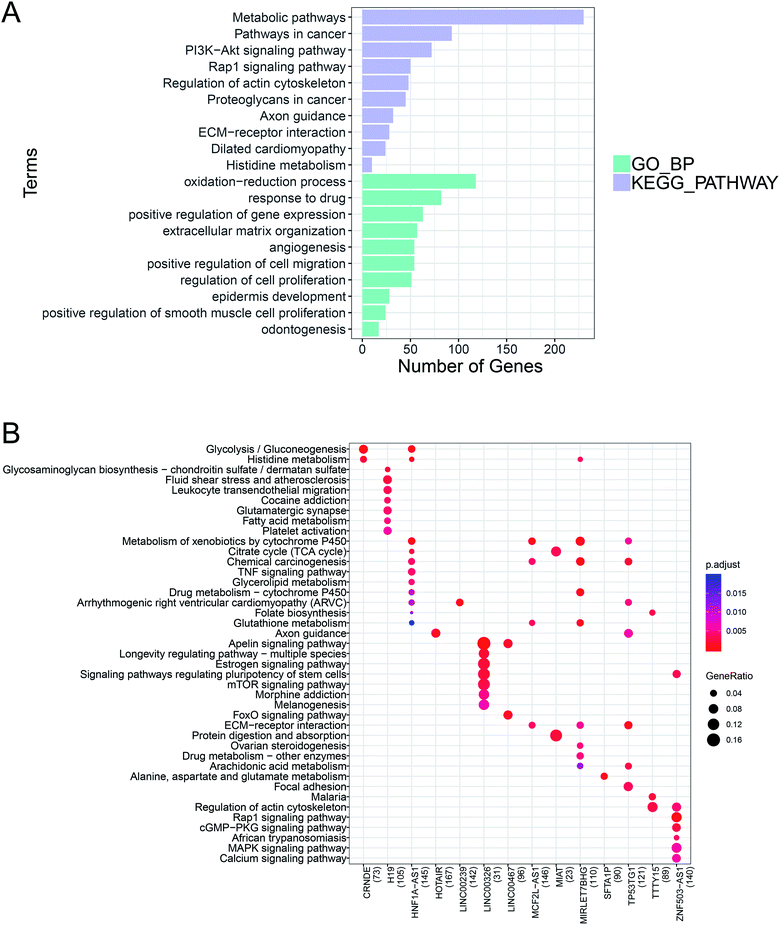 |
| Fig. 2 Functional enrichment and pathway analyses of differentially expressed RNAs. The GO BP functional enrichment and KEGG pathway enrichment analyses were performed on the differentially expressed mRNAs. Panel (A) shows the top 10 p-values from the GO BP and KEGG pathway analyses. Green represents GO BP and light purple represents the KEGG pathway analysis. The bar length indicates the number of enriched RNAs. Based on the lncRNA–mRNA co-expression pairs, the KEGG pathway enrichment analysis was conducted on the mRNA of the lncRNA target gene to predict the function of the lncRNA. Panel (B) shows the KEGG pathways of 14 lncRNAs. Blue to red indicates a decrease in p-value significance, and the bubble size indicates the proportion of enriched RNAs. | |
According to the set threshold, a total of 5188 pairs of co-expression pairs, including 1824 mRNAs and 20 lncRNAs, were screened. Based on these lncRNA–mRNA pairs, the KEGG pathway enrichment analysis was conducted on the mRNA as the target gene of the corresponding lncRNA to predict its function. Panel (B) of Fig. 2 shows the KEGG pathways with the 14 lncRNAs.
3.3. miRNA prediction and construction of the ceRNA network
Prediction of miRNA was obtained based on the 20 lncRNAs in the 821 lncRNA–mRNA pairs using lnCeDB and starBase v2.0. A total of 821 lncRNA–miRNA pairs including 487 miRNAs and 16 lncRNAs were obtained, and 4387 miRNA–mRNA pairs including 592 miRNAs and 567 mRNAs were obtained using miRWalk2.0. Finally, 459 lncRNA–miRNA–mRNA regulatory pairs including 138 miRNAs, 15 lncRNAs and 147 mRNAs were obtained by screening the mRNAs and lncRNAs that were regulated by the same miRNA. These lncRNA–miRNA–mRNA regulatory complexes were used to construct the ceRNA network. As shown in Fig. 3, the ceRNA network contains a total of 503 lncRNA–miRNA–mRNA regulatory complexes, 210 lncRNA–miRNA pairs, 382 miRNA–mRNA pairs, and 212 mRNA co-expression pairs. The top 5 mRNAs, miRNAs and lncRNAs with the highest degrees of connectivity are listed in Table 2 by the degree of connectivity at each node in the above-mentioned ceRNA network.
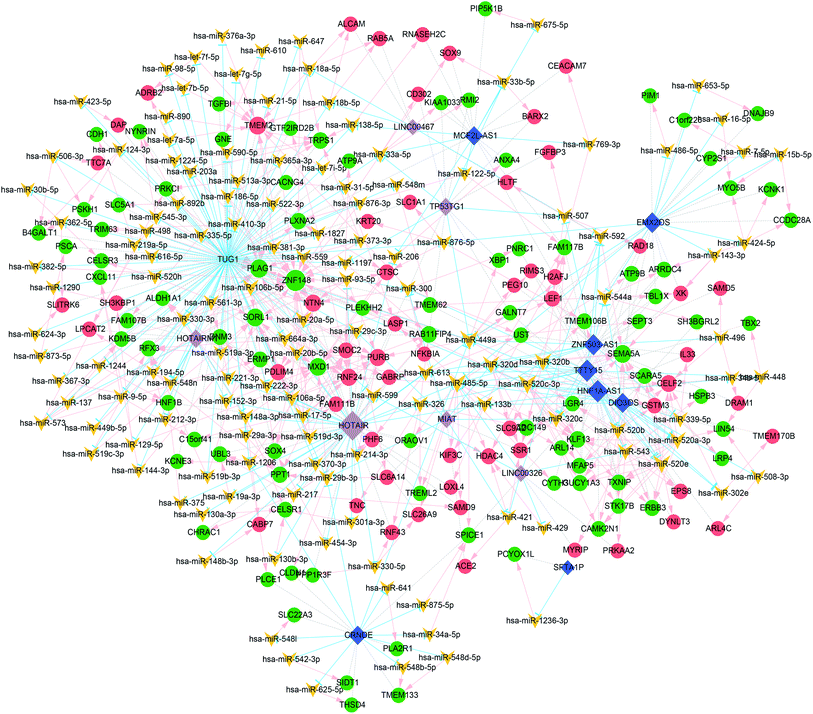 |
| Fig. 3 CeRNA network for oxaliplatin resistance in CRC. The red and green circles represent up-regulated mRNA, and the green circle represents downregulated and upregulated mRNA. The light purple and dark blue diamonds represent up- and down-regulated lncRNAs, respectively. The blue T-shaped line, pink arrow, and grey dotted line represent the regulatory relationship between lncRNA–miRNA, the regulatory relationship between miRNA–mRNA, and the co-expression relationship between mRNAs and lncRNAs, respectively. | |
Table 2 The connectivity degree analysis of each node in the ceRNA network (TOP 5)
Node |
Connectivity degree |
Type |
TUG1 |
128 |
lncRNA_down |
HOTAIR |
61 |
lncRNA_up |
HNF1A-AS1 |
32 |
lncRNA_down |
TTTY15 |
31 |
lncRNA_down |
ZNF503-AS1 |
27 |
lncRNA_down |
ZNF148 |
26 |
mRNA_down |
PLAG1 |
15 |
mRNA_down |
SEMA5A |
13 |
mRNA_down |
SORL1 |
13 |
mRNA_down |
TMEM2 |
13 |
mRNA_up |
hsa-miR-449a |
18 |
miRNA |
hsa-miR-485-5p |
17 |
miRNA |
hsa-miR-520c-3p |
17 |
miRNA |
hsa-miR-520e |
15 |
miRNA |
hsa-miR-520b |
14 |
miRNA |
Because the ceRNA network was too large with many miRNAs, the miRNAs with more than 10 degrees of connectivity were further screened to construct a more concise ceRNA network. As shown in Fig. 4, the concise ceRNA network contains a total of 156 lncRNA–miRNA–mRNA regulatory complexes, 40 lncRNA–miRNA pairs, 111 miRNA–mRNA pairs, and 67 mRNA co-expression pairs.
 |
| Fig. 4 Concise ceRNA network for oxaliplatin resistance in CRC. The miRNAs with more than 10 degrees of connectivity were used for the construction of the concise ceRNA network. The red and green circles represent up- and down-regulated mRNAs, respectively. The light purple and dark blue diamonds represent upregulated and downregulated lncRNAs, respectively. The blue T-shaped line, the pink arrow, and grey dotted line represent the regulatory relationship between lncRNA–miRNA, the regulatory relationship between miRNA–mRNA, and the co-expression relationship between mRNAs and lncRNAs, respectively. | |
3.4. Verification of RNA in key nodes
The HCT-116 and HCT-8 cell lines were used as controls, and the cell lines HCT-116/L and HCT-8/L were used as the oxaliplatin resistant lines. The top 5 mRNAs, miRNAs and lncRNAs with the highest degrees of connectivity were verified by PCR. As shown in Fig. 5, the results showed that the expression of lncRNAs TUG1, HOTAIR, and HNF1A-AS1; miRNAs hsa-miR-449a and hsa-miR-520c-3p; and mRNA PLAG1, SEMA5A, SORL1, and TMEM2 differed significantly between the oxaliplatin resistant group and the control group.
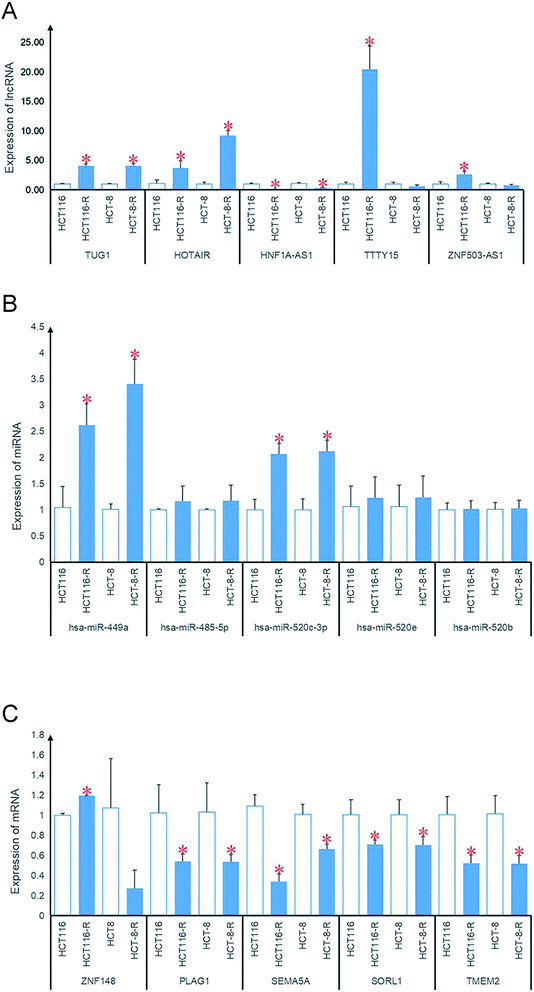 |
| Fig. 5 Verification of RNAs in key nodes. The HCT-116 and HCT-8 cell lines were used as controls, and the oxaliplatin-resistant cell lines HCT-116/L and HCT-8/L were used as the oxaliplatin resistant group. The top 5 mRNAs, miRNAs and lncRNAs with high degrees of connectivity were verified by PCR. * on the bar means p < 0.05 (Student's t-test). | |
3.5. Survival analysis of oxaliplatin resistance genes
All mRNAs and lncRNAs in the ceRNA network were used as candidate genes for survival analysis. The mRNA and lncRNA expression levels and patient survival information from TCGA were used to plot the KM survival curve. The samples were divided into high risk and low risk groups according to the median expression value of each gene. The KM survival curve in Fig. 6 shows that some RNAs significantly correlate with prognosis, including one lncRNA (HOTAIR) and 14 mRNAs (ARL4C, CACNG4, CCDC149, GALNT7, LPCAT2, PDLIM4, PLAG1, PPP1R3F, RAB11FIP4, RIMS3, SSR1, TREML2, TTC7A, and UST).
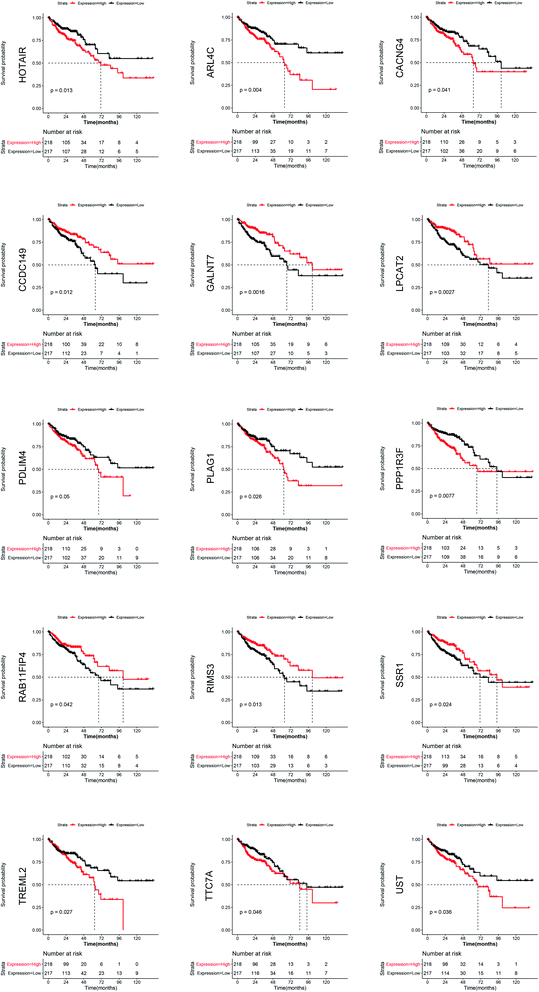 |
| Fig. 6 Survival curve with CRC containing differentially expressed RNA involved in oxaliplatin resistance. The mRNA and lncRNA expression levels and patient survival information from TCGA colon cancer data were used to plot the KM survival curve. All mRNAs and lncRNAs in the ceRNA network were used as candidate genes for the survival analysis. The figure shows that the RNAs significantly correlate with prognosis including one lncRNA (HOTAIR) and 14 mRNAs (ARL4C, CACNG4, CCDC149, GALNT7, LPCAT2, PDLIM4, PLAG1, PPP1R3F, RAB11FIP4, RIMS3, SSR1, TREML2, TTC7A, and UST) (p-value < 0.05). | |
4 Discussion
In the present study, the 2515 mRNAs and 23 lncRNAs that were differentially expressed between the oxaliplatin-resistant and oxaliplatin-sensitive CRC cell lines were examined. A ceRNA network containing 503 lncRNA–miRNA–mRNA regulatory pairs, 210 lncRNA–miRNA pairs, 382 miRNA–mRNA pairs, and 212 mRNA co-expression pairs was constructed. The mRNAs, miRNAs, and lncRNAs with high degrees of connectivity in the ceRNA network were validated. KM survival curves were constructed to display one lncRNA (HOTAIR) and 14 mRNAs, which correlated with prognosis.
FOLFOX and XELOX chemotherapy regimens are recommended by NCCN guidelines for postoperative adjuvant chemotherapy for CRC.45 However, the presence of oxaliplatin resistant cell populations has not been studied in clinical practice. Oxaliplatin is often combined with fluorouracil or capecitabine for the treatment of CRC. Patients with poor prognosis or rapidly progressing tumors make it difficult to judge the change in prognosis caused by oxaliplatin resistance. It is impossible to conduct clinical research on oxaliplatin resistance in the treatment of CRC with oxaliplatin alone because it is always combined with chemotherapy. Thus, screening for genes required for drug resistance to elucidate the molecular mechanisms of oxaliplatin resistance has become the only alternative to improve the prognosis of patients using oxaliplatin.
In recent years, targeted drugs such as bevacizumab, cetuximab, and immunotherapies such as immune checkpoint inhibitors PD1/PDL1 have been applied in the treatment of advanced CRC.46–48 These therapies have significantly improved the survival time of patients. However, efficacy is significantly improved when these targeted drugs are combined with oxaliplatin chemotherapy compared to treatment with targeted drugs alone. Therefore, examining oxaliplatin resistance genes should not be ignored for use in second-line treatment of CRC.
In the present study, the RNAs that were differentially expressed between the oxaliplatin-resistant and oxaliplatin-sensitive colon cancer cell lines were analyzed. Subsequently, the relationship between these genes and the prognosis of patients with CRC was analyzed, which revealed that lncRNA HOTAIR and 14 mRNAs (ARL4C, CACNG4, CCDC149, GALNT7, LPCAT2, PDLIM4, PLAG1, PPP1R3F, RAB11FIP4, RIMS3, SSR1, TREML2, TTC7A, and UST) are associated with prognosis. The chemotherapy regimen containing oxaliplatin is used in postoperative adjuvant chemotherapy and advanced chemotherapy for CRC. Whether the differential expression of these oxaliplatin-resistance-associated genes truly leads to oxaliplatin resistance, thus affecting the prognosis of patients with CRC, is a topic worthy of discussion.
The non-coding and coding regions of DNA interact with and regulate one another. This very complex network system has not yet been recognized.49,50 Elucidating these molecular interactions has become urgent in the post-genomic era. The ceRNA network constructed here, containing 503 lncRNA–miRNA–mRNA regulatory pairs, 210 lncRNA–miRNA pairs, 382 miRNA–mRNA pairs, and 212 mRNA co-expression pairs, will provide guidance for follow-up studies on the molecular mechanism of oxaliplatin resistance.
The main limitation of this study is that cancer heterogeneity and diversity cannot be recapitulated by a single tumor cell clone propagated in vitro. Thus, further clinical validation of the genes identified here involved in oxaliplatin resistance will aid in the development of personalized therapies. Additionally, the ceRNA network should be further verified with in vitro and in vivo experimental studies.
Funding
This work was supported by the Science Technology Projects of Zhejiang Province (No. 2017C33207).
Conflicts of interest
The authors declare that no conflicts of interest exist.
Abbreviation
CRC | Colorectal cancer |
KEGG | Kyoto encyclopedia of genes and genomes |
RNA | Ribonucleic acid |
mRNA | Messenger ribonucleic acid |
miRNA | Micro ribonucleic acid |
lncRNA | Long non-coding ribonucleic acid |
BP | Biological process |
ceRNA | Competing endogenous ribonucleic acid |
K–M | Kaplan–Meier |
PCR | Polymerase chain reaction |
NCCN | The National Comprehensive Cancer Network |
DACH | Diaminocyclohexane |
DRC | Deoxyribonucleic acid repair capacity |
OCT | Organic cation transporters |
MRP | Multidrug resistant related proteins |
GSH | Glutathione |
AOD | Antisense oligodeoxyribonucleotide |
RNAi | Ribonucleic acid interference |
MREs | MicroRNA response elements |
GEO | Gene expression omnibus |
cDNA | Complementary deoxyribonucleic acid |
FPKM | Fragments per kilobase million |
OS | Overall survival |
PD-1 | Programmed cell death protein 1 |
Acknowledgements
The authors gratefully acknowledge the multiple databases, which made the data available.
References
- M. Arnold, M. S. Sierra and M. Laversanne,
et al., Global patterns and trends in colorectal cancer incidence and mortality, Gut, 2016, 66(4), 683–691 CrossRef.
- A. B. Benson, A. P. Venook and M. M. Al-Hawary,
et al., NCCN Guidelines Insights: Colon Cancer, Version 2.2018, J. Natl. Compr. Cancer Network, 2018, 16(4), 359–369 CrossRef.
- L. S. Rosen, I. A. Jacobs and R. L. Burkes, Bevacizumab in Colorectal Cancer: Current Role in Treatment and the Potential of Biosimilars, Target. Oncol., 2017, 12(5), 599–610 CrossRef.
- A. P. Venook, D. Niedzwiecki and H. J. Lenz,
et al., Effect of First-Line Chemotherapy Combined With Cetuximab or Bevacizumab on Overall Survival in Patients With KRAS Wild-Type Advanced or Metastatic Colorectal Cancer: A Randomized Clinical Trial, J. Am. Med. Assoc., 2017, 317(23), 2392–2401 CrossRef CAS.
- S. V. Hato, A. Khong and I. J. M. D. Vries,
et al., Molecular Pathways: The Immunogenic Effects of Platinum-Based Chemotherapeutics, Clin. Cancer Res., 2014, 20(11), 2831–2837 CrossRef CAS.
- L. Kelland, The resurgence of platinum-based cancer chemotherapy, Nat. Rev. Cancer, 2007, 7(8), 573–584 CrossRef CAS.
- H. Y. Chen, H. L. Cheng and Y. H. Lee,
et al., Tumor-associated NADH oxidase (tNOX)-NAD+-sirtuin 1 axis contributes to oxaliplatin-induced apoptosis of gastric cancer cells, Oncotarget, 2017, 8(9), 15338–15348 Search PubMed.
- H. Ouzon-Shubeita, M. Baker and M. C. Koag,
et al., Structural basis for the bypass of the major oxaliplatin-DNA adducts by human DNA polymerase eta, Biochem. J., 2019, 476(4), 747–758 CrossRef CAS.
- N. F. Jensen, J. Stenvang and M. K. Beck,
et al., Establishment and characterization of models of chemotherapy resistance in colorectal cancer: Towards a predictive signature of chemoresistance, Mol. Oncol., 2015, 9(6), 1169–1185 CrossRef CAS.
- J. Slyskova, M. Sabatella and C. Ribeiro-Silva,
et al., Base and nucleotide excision repair facilitate resolution of platinum drugs-induced transcription blockage, Nucleic Acids Res., 2018, 46(18), 9537–9549 CrossRef CAS.
- E. Martinezbalibrea, A. Martínezcardús and A. Ginés,
et al., Tumor-Related Molecular Mechanisms of Oxaliplatin Resistance, Mol. Cancer Ther., 2015, 14(8), 1767–1776 CrossRef CAS.
- K. M. Redmond, T. R. Wilson and P. G. Johnston,
et al., Resistance mechanisms to cancer chemotherapy, Front. Biosci., 2008, 13(5), 5138–5154 CrossRef CAS.
- D. Theile, S. Grebhardt and W. E. Haefeli,
et al., Involvement of drug transporters in the synergistic action of FOLFOX combination chemotherapy, Biochem. Pharmacol., 2009, 78(11), 1366–1373 CrossRef CAS.
- S. B. Howell, R. Safaei and C. A. Larson,
et al., Copper Transporters and the Cellular Pharmacology of the Platinum-Containing Cancer Drugs, Mol. Pharmacol., 2010, 77(6), 887–894 CrossRef CAS.
- I. Buss, A. Hamacher and N. Sarin,
et al., Relevance of copper transporter 1 and organic cation transporters 1-3 for oxaliplatin uptake and drug resistance in colorectal cancer cells, Metallomics, 2018, 10(3), 414–425 RSC.
- T. Oguri, E. Kunii and S. Fukuda,
et al., Organic cation transporter 6 directly confers resistance to anticancer platinum drugs, Biomed. Rep., 2016, 5(5), 639–643 CrossRef CAS.
- Y. Dong, Z. Wang and G. F. Xie,
et al., Pregnane X receptor is associated with unfavorable survival and induces chemotherapeutic resistance by transcriptional activating multidrug resistance-related protein 3 in colorectal cancer, Mol. Cancer, 2017, 16(1), 71 CrossRef PubMed.
- M. C. Chen, H. H. Hsu and Y. Y. Chu,
et al., Lupeol alters ER stress-signaling pathway by downregulating ABCG2 expression to induce Oxaliplatin-resistant LoVo colorectal cancer cell apoptosis, Environ. Toxicol., 2018, 33(5), 587–593 CrossRef CAS.
- R. Tummala, D. Wolle and S. P. Barwe,
et al., Expression of Na,K-ATPase-β1subunit increases uptake and sensitizes carcinoma cells to oxaliplatin, Cancer Chemother. Pharmacol., 2009, 64(6), 1187–1194 CrossRef CAS.
- Z. Wang, L. Zhang and Z. Ni,
et al., Resveratrol induces AMPK-dependent MDR1 inhibition in colorectal cancer HCT116/L-OHP cells by preventing activation of NF-κB signaling and suppressing cAMP-responsive element transcriptional activity, Tumor Biol., 2015, 36(12), 9499–9510 CrossRef CAS.
- M. Ghanbarian, A. Afgar and R. Yadegarazari,
et al., Through oxaliplatin resistance induction in colorectal cancer cells, increasing ABCB1 level accompanies decreasing level of miR-302c-5p, miR-3664-5p and miR-129-5p, Biomed. Pharmacother., 2018, 108, 1070–1080 CrossRef CAS.
- X. T. Fu, K. Song and J. Zhou,
et al., Autophagy activation contributes to glutathione transferase Mu 1-mediated chemoresistance in hepatocellular carcinoma, Oncol. Lett., 2018, 16(1), 346–352 Search PubMed.
- H. Shuwen, Z. Qing and Z. Yan,
et al., Competitive endogenous RNA in colorectal cancer: A systematic review, Gene, 2018, 645, 157–162 CrossRef.
- B. Neve, N. Jonckheere and A. Vincent,
et al., Epigenetic Regulation by lncRNAs: An Overview Focused on UCA1 in Colorectal Cancer, Cancers, 2018, 10(11), pii: E440 CrossRef.
- R. Abdollahzadeh, A. Daraei and Y. Mansoori,
et al., Competing endogenous RNA (ceRNA) cross talk and language in ceRNA regulatory networks: A new look at hallmarks of breast cancer, J. Cell. Physiol., 2019, 234(7), 10080–10100 CrossRef CAS.
- R. Gao, C. Fang and J. Xu,
et al., LncRNA CACS15 contributes to oxaliplatin resistance in colorectal cancer by positively regulating ABCC1 through sponging miR-145, Arch. Biochem. Biophys., 2019, 663, 183–191 CrossRef CAS.
- H. Hu, L. Yang and L. Li,
et al., Long non-coding RNA KCNQ1OT1 modulates oxaliplatin resistance in hepatocellular carcinoma through miR-7-5p/ABCC1 axis, Biochem. Biophys. Res. Commun., 2018, 503(4), 2400–2406 CrossRef CAS.
- X. Wu, Y. Zheng and B. Han,
et al., Long noncoding RNA BLACAT1 modulates ABCB1 to promote oxaliplatin resistance of gastric cancer via sponging miR-361, Biomed. Pharmacother., 2018, 99, 832 CrossRef CAS.
- M. H. Rasmussen, I. Lyskjær and R. R. Jersiechristensen,
et al., miR-625-3p regulates oxaliplatin resistance by targeting MAP2K6-p38 signalling in human colorectal adenocarcinoma cells, Nat. Commun., 2016, 7, 12436 CrossRef CAS.
- C. Sun, F. J. Wang and H. G. Zhang,
et al., miR-34a mediates oxaliplatin resistance of colorectal cancer cells by inhibiting macroautophagy via transforming growth factor-beta/Smad4 pathway, World J. Gastroenterol., 2017, 23(10), 1816–1827 CrossRef CAS.
- H. Liang, Y. Xu and Q. Zhang,
et al., MiR-483-3p regulates oxaliplatin resistance by targeting FAM171B in human colorectal cancer cells, Artif. Cells, Nanomed., Biotechnol., 2019, 47(1), 725–736 CrossRef CAS.
- F. Cao and L. X. Yin, miR-122 enhances sensitivity of hepatocellular carcinoma to oxaliplatin via inhibiting MDR1 by targeting Wnt/beta-catenin pathway, Exp. Mol. Pathol., 2019, 106, 34–43 CrossRef CAS.
-
G. K. Smyth, limma: Linear Models for Microarray Data, Bioinformatics & Computational Biology Solutions Using R & Bioconductor, 2011, pp. 397–420 Search PubMed.
- D. W. Huang, B. T. Sherman and R. A. Lempicki, Systematic and integrative analysis of large gene lists using DAVID bioinformatics resources, Nat. Protoc., 2009, 4(1), 44–57 CrossRef CAS.
- M. Ashburner, C. A. Ball and J. A. Blake,
et al., Gene ontology: tool for the unification of biology. The Gene Ontology Consortium, Nat. Genet., 2000, 25(1), 25–29 CrossRef CAS.
- J. Wixon and D. Kell, The Kyoto encyclopedia of genes and genomes–KEGG, Yeast, 2000, 17(1), 48–55 CAS.
- G. Yu, L. G. Wang and Y. Han,
et al., clusterProfiler: an R Package for Comparing Biological Themes Among Gene Clusters, OMICS: J. Integr. Biol., 2012, 16(5), 284–287 CrossRef CAS.
- D. Shaoli, G. Suman and S. Rituparno,
et al., lnCeDB: Database of Human Long Noncoding RNA Acting as Competing Endogenous RNA, PLoS One, 2014, 9(6), e98965 CrossRef.
- J. H. Li, S. Liu and H. Zhou,
et al., starBase v2.0: decoding miRNA-ceRNA, miRNA-ncRNA and protein-RNA interaction networks from large-scale CLIP-Seq data, Nucleic Acids Res., 2014, 42, D92 CrossRef CAS.
- H. Dweep and N. Gretz, miRWalk2.0: a comprehensive atlas of microRNA-target interactions, Nat. Methods, 2015, 12(8), 697 CrossRef CAS.
-
P. Shannon, A. Markiel, O. Ozier, et al., Cytoscape: A Software Environment for Integrated Models of Biomolecular Interaction Networks//Contributions to the history of economic thought, Routledge, 2003 Search PubMed.
- Y. Tang, M. Li and J. Wang,
et al., CytoNCA: a cytoscape plugin for centrality analysis and evaluation of protein interaction networks, BioSystems, 2015, 127, 67–72 CrossRef CAS.
- K. J. Livak and T. D. Schmittgen, Analysis of relative gene expression data using real-time quantitative PCR and the 2−ΔΔCt method, Methods, 2001, 25, 402–408 CrossRef CAS.
- C. Tyner, G. P. Barber and J. Casper,
et al., The UCSC Genome Browser database: 2017 update, Nucleic Acids Res., 2016, 45(1), 626–634 Search PubMed.
- K. Okada, S. Sadahiro and G. Saito,
et al., Postoperative Adjuvant Chemotherapy for Stage III Colon Cancer–Drug Selection, Tolerability, and Safety in Clinical Practice, Gan to Kagaku Ryoho, 2016, 43(5), 587–592 Search PubMed.
- Wen-Cong, Y.-P. Che and L. Ding,
et al., Efficacy and Toxicity of Addition of Bevacizumab to Chemotherapy in Patients with Metastatic Colorectal Cancer, Comb. Chem. High Throughput Screening, 2018, 21, 718–724 Search PubMed.
- Karuna, K. Stadler Zsofia and C. Andrea,
et al., Immunotherapy in colorectal cancer: rationale, challenges and potential, Nat. Rev. Gastroenterol. Hepatol., 2019, 16, 361–375 CrossRef.
- Neda, S. Arash and G. Kiarash,
et al., PD-1/PD-L1 blockade as a novel treatment for colorectal cancer, Biomed. Pharmacother., 2019, 110, 312–318 CrossRef.
- M. N. Timofeeva, B. Kinnersley and S. M. Farrington,
et al., Recurrent Coding Sequence Variation Explains Only A Small Fraction of the Genetic Architecture of Colorectal Cancer, Sci. Rep., 2015, 5(40), 16286 CrossRef CAS.
- Anna, T.-S. Alicja and K. Ryszard,
et al., RNA-protein interactions: disorder, moonlighting and junk contribute to eukaryotic complexity, Open Biol., 2019, 9, 190096 CrossRef.
Footnote |
† Electronic supplementary information (ESI) available. See DOI: 10.1039/c9ra06146k |
|
This journal is © The Royal Society of Chemistry 2019 |
Click here to see how this site uses Cookies. View our privacy policy here.