DOI:
10.1039/C9RA06697G
(Review Article)
RSC Adv., 2019,
9, 37245-37257
Metabolomics biotechnology, applications, and future trends: a systematic review
Received
25th August 2019
, Accepted 3rd November 2019
First published on 14th November 2019
Abstract
Given the highly increased incidence of human diseases, a better understanding of the related mechanisms regarding endogenous metabolism is urgently needed. Mass spectrometry-based metabolomics has been used in a variety of disease research areas. However, the deep research of metabolites remains a difficult and lengthy process. Fortunately, mass spectrometry is considered to be a universal tool with high specificity and sensitivity and is widely used around the world. Mass spectrometry technology has been applied to various basic disciplines, providing technical support for the discovery and identification of endogenous substances in living organisms. The combination of metabolomics and mass spectrometry is of great significance for the discovery and identification of metabolite biomarkers. The mass spectrometry tool could further improve and develop the exploratory research of the life sciences. This mini review discusses metabolomics biotechnology with a focus on recent applications of metabolomics as a powerful tool to elucidate metabolic disturbances and the related mechanisms of diseases.
1. Introduction
With the advancement of science and technology, scientists have found that simply studying a certain direction cannot explain all biomedical problems, so it was proposed to apply the concept of omics from a holistic perspective. The birth of genomics, metabolomics, proteomics, lipidomics and transcriptomics has provided a new approach to exploring the pathogenesis of human disease. The application of metabolomics as an important method for studying modern life sciences is closely related to the latest developments in science and technology (Fig. 1). Metabolomics, as an important part of systems biology, mainly analyzes substances such as blood, urine, and feces, and then studies small molecule metabolites of various metabolic pathway matrices and products.1 Techniques involved in metabolomics methods include nuclear magnetic resonance, mass spectrometry (MS), and chromatography. Mass spectrometry-based metabolomics plays an important role in clinical research, disease treatment, drug characterization, animal and plant research, agricultural research, and nutrition.2 In recent years, a large number of domestic and foreign scholars have concentrated on the research of metabolomics, and based on their important position in the field of science, the metabolomics techniques and methods have been optimized.3 Understanding the research status and achievements of different scholars in this field, and interpreting the imperfect areas in this field, will lay a foundation for further study of metabolomics and elaboration of metabolic mechanisms. We will discuss this from the perspectives of metabolomics research and the application of MS.
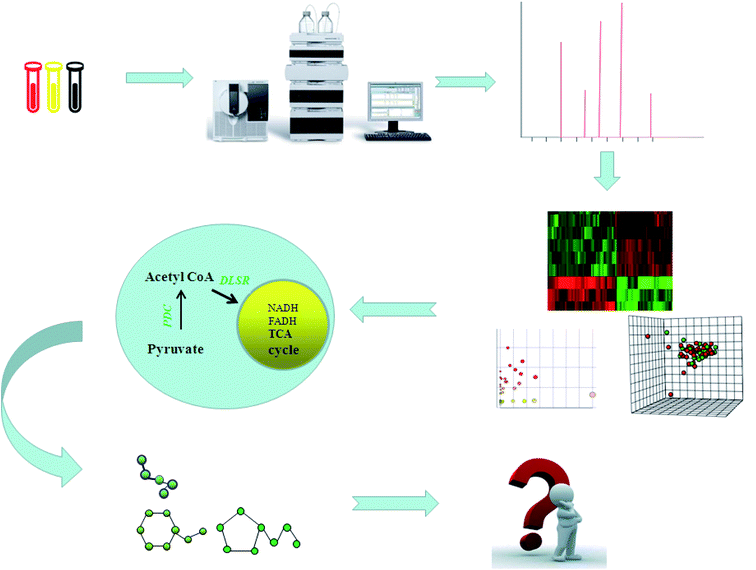 |
| Fig. 1 A flow chart of metabolomics analysis technology. | |
2. The metabolomics method
In 1999, Professor Jeremy Nicholson first proposed the concept of metabolomics.4 Using the same idea as genomics and proteomics, metabolomics is a way to quantitatively analyze all metabolites in organisms and to find the relative relationship between metabolites and physiological and pathological changes. As an integral part of systems biology, most of the research objects in metabolomics are small molecules with a relative molecular weight of less than 1000. Advanced analytical techniques combined with pattern recognition and expert systems are the basic methods of metabolomics research.5 The research process includes sample preparation, sample analysis, data analysis and metabolic pathway analysis. Biological samples analyzed mainly include blood, urine, feces and the like. Sample preparation is generally composed of sample extraction, compound pretreatment and separation. The quality of the obtained sample is critical to the success of the experiment. Often, a large amount of pretreatment is required before the sample is analyzed. Pretreatment is an important aspect of sample preparation. In short, sample analysis in sample preparation includes sample source management, sample storage, and sample extraction to ensure that the metabolic information does not change due to sample processing. Different analyses have distinct requirements for sample processing. The information of the sample may change with the environment in which it is used. For example, when analyzing samples by NMR, there are certain requirements for the pH and ionic strength of the sample, which are different when mass spectrometry is utilized to study metabolism. In the case of the samples, macromolecules should be eliminated and small molecules should be recovered as completely as possible.
Analytical tools for metabolomics research include nuclear magnetic resonance spectroscopy (NMR), gas chromatography-mass spectrometry (GC-MS), and liquid chromatography-mass spectrometry (LC-MS). Among them, the NMR technique generally uses a hydrogen spectrum (1H NMR), a carbon spectrum (13C NMR), or a phosphorus spectrum (31P NMR), of which 1H NMR is the most widely used.6–9 Metabolomics research is still a complete explanation of the data. Because the original map of metabolomics research is complex and the data volume is large, it cannot be analyzed by conventional data processing methods, so metabolomics analysis involves a special analysis with three steps: data extraction, data preprocessing and pattern recognition. Data extraction is the visualization of the map; data preprocessing includes noise filtering, overlapping the peak resolution, peak alignment, peak matching, normalization, etc.; pattern recognition can be conducted using a supervised mode or an unsupervised mode. Unsupervised models include principal component analysis (PCA), cluster analysis (HCA) and nonlinear mapping (NLM); supervisory models include artificial neural networks (ANN), partial least squares discriminant analysis (PLS-DA), positive and negative minimum two-square discriminant analysis (OPLS-DA), etc.10–12 Metabolomics needs to process a large amount of raw data and extract the potential information in the data through statistical processing and explain the biological merit contained in the data, which will be the key to metabolomics research. The use of statistical means to deeply explore the information in metabolic samples will strongly promote the development of metabolomics technology.
Metabolomics studies investigate endogenous small molecule compounds, including sugars, organic acids, amino acids, lipids, and the like. The goal of metabolomics is to discover the affected small molecules and ultimately apply them to certain related diseases. As an application-driven emerging science, metabolomics technology has been widely used in medical-related fields, with significant impacts on life, food and plant sciences, drug development, toxicology, environmental science, and medicine.
3. Mass spectrometry technology
In 1919, British physicists successfully studied the world's first mass spectrometer. By the middle of the 20th century, mass spectrometers had become analytical tools used by chemists and they are increasingly used for organic analysis. Compared with general analytical methods, mass spectrometry is an analytical method for measuring the mass-to-charge ratio of ions. It provides abundant structural information in one analysis and plays a key role in the identification of organic molecules. It can quickly and extremely accurately determine the molecular weight of biomacromolecules, enabling proteomics research from protein identification to advanced structural studies and investigations into the interactions between various proteins. With the application of mass spectrometry technology, mass spectrometry technology is gradually improving, and the combination of separation technology and mass spectrometry is a breakthrough in scientific separation methods.13,14 Because of their high sensitivity, low sample consumption, fast analysis, and advantages of separation and identification, mass spectrometers are widely used in chemistry, the environment, energy, medicine, sports medicine, criminal science and technology, life sciences, materials science and pharmaceuticals.
In the 21st century, the development of modern science and technology poses new challenges to analytical testing techniques. Unlike classical chemical analysis methods and traditional instrumental analysis methods, in situ, real-time, online, non-destructive, high-throughput, high-sensitivity, high-selectivity, and low-loss are always the goals pursued by analysts in modern analytical science. Among the many analytical test methods, the MS method is considered to be a method with high specificity and high sensitivity and is widely used. Electrospray desorption ionization technology, corona discharge real-time direct analysis ionization technology and electrospray ionization technology have met the needs of the times, meeting the requirements of scientific and technological development, and have opened a window for rapid MS analysis of complex samples.15–17
4. Recent advances and applications
The number of MS-based metabolomics studies has increased. MS-based metabolomics methods have been used to study the effects of drugs, toxins, and various diseases on metabolites. MS-based metabolomics methods have been used to study many diseases, including breast cancer, kidney cancer, cardiovascular disease, bladder cancer, esophageal cancer and gastric cancer, kidney disease, natural metabolic errors, toxicological effects, and nutrition.18–24
4.1 Disease diagnosis
Metabolomics research focuses on the commonality of specific components, and ultimately involves studying the commonalities, properties, and laws of each of the metabolic components. Metabolomics is more closely linked to physiology than genomics and proteomics. The disease causes changes in the pathophysiological process of the body, which eventually causes corresponding changes in metabolites. By analyzing certain metabolites and comparing them with normal human metabolites, the biomarkers of the disease are sought. Metabolomics methods will serve as a new approach to disease diagnosis. Johno, H. et al. used electrostatic ionization mass spectrometry to establish a diagnostic method for automatic atherosclerotic disease detection. Cholesterol sulfate and phospholipids are considered to be new biomarkers of arteriosclerosis.25 Tavares, G. et al. performed metabolomics on plasma from 56 DKD patients. Their results indicate that lower levels of 1,5-anhydroglucitol, norvaline and L-aspartic acid are associated with the progression of macroalbuminuric DKD. Inverse biomarkers of hyperglycemia could be explored as a new tool in DKD.26 Hao, M. et al. used a combination of plasma metabolomics and network pharmacology to elucidate the mechanism of Yishu in treating blood stasis from a metabolic point of view. It was found that the improvement of blood stasis by Curcuma wenyujin rhizomes was mainly related to lipid metabolism and amino acid metabolism.27
Nowadays, people's understanding of diseases is shallow, and people often do not know that well after illness, many diseases can lurk in the patient's body, and many can be lurking for several years. Some diseases do not immediately damage the body, and most patients tend to have a neglected attitude. This can lead to the patient missing the best treatment period, making the treatment more difficult. Understanding the causes of diseases and their influencing factors through mass spectrometry-based metabolomics methods is of great significance for understanding the mechanism of action of diseases. Using LC-MS/MS analysis, Abdulwahab, R. A. et al. revealed new associations between 37 proteins and T2DM, as well as significant up-regulation of immunoglobulin.28 Using UPLC-QTOF/MS and multivariate statistical analysis, Yang, G. et al. found that there were 33 distinct metabolites in the urine of femoral head necrosis patients and healthy people.29 Lin, S. et al. used gas chromatography-MS to detect plasma metabolites, and found that the levels of alanine, aspartic acid and carbamate were significantly different among all groups of patients with respiratory distress classified from mild to severe.30
Understanding the causes of diseases and their influencing factors is of great significance for understanding diseases. The application of mass spectrometry-based metabolomics methods in disease diagnosis will be explained through the following examples. Øvrehus, M. A. et al. found that early hypertensive nephrosclerosis showed disturbances in dopamine intrarenal biosynthesis.31 Barbosa-Breda, J. et al. have conducted many studies on glaucoma patients and compared them with healthy people by MS. Significant differences were found in metabolic pathways such as palmitoyl carnitine, sphingolipid, vitamin D-related compounds and steroid precursors.32 Dong, S. et al. used LC/MS as a biomarker for nonalcoholic fatty liver disease and nonalcoholic steatohepatitis, and found significant differences in 31 metabolites between the two groups.33 Based on the metabolomic method of GC-MS, Wang, D. et al. found that the changes of acute ischemic stroke metabolites in serum were mainly related to amino acid-related metabolism.34 Li, Q. et al. used GC-MS to analyze changes in urine metabolism in gout patients. It was found that there are 30 different metabolites in the urine of patients with gout, mainly involving metabolic pathways such as amino acid metabolism, lipid metabolism and the tricarboxylic acid cycle. The discovery of differential metabolites provides a new idea for the diagnosis of gout disease.35 Sun, H. et al. used UPLC/MS to perform metabolomics analysis of ischemic stroke and found significant changes in 12 metabolites, mainly related to heme and fatty acid metabolism.36 Lu, H. et al. used HPLC-MS to study the effects of hydrogen on serum and urine metabolites in a kidney injury model. It was found that the differential metabolites were mainly related to amino acid metabolism, fatty acid metabolism and phospholipid metabolism.37
Biomarkers have a very wide range of uses during research processes. They can be utilized for disease diagnosis, judging disease stage or evaluating new drugs. Understanding the impact of metabolomics techniques on biomarkers is important for understanding biomarkers. The following examples will illustrate the application of metabolomics techniques in the discovery of disease biomarkers from different directions, laying the foundation for the close integration of metabolomics and clinical medicine. Zhao, H. et al. used UPLC-Q-TOF/MS to identify different metabolites of potential biomarkers in the urine of patients with essential hypertension. The metabolic pathways involved were amino acid metabolism, fatty acid metabolism, steroid hormones, biosynthesis and oxidative stress.38 Dittharot, K. et al. used GC/MS to study the urinary metabolomics characteristics of patients with chronic hepatitis B. Compared with normal individuals, 12 differential metabolites were found, mainly related to fatty acid, amino acid, bile acid and intestinal microbial metabolic pathways.39 Zhao, H. et al. used LC-MS-based metabolomics to study potential biomarkers of Parkinson's disease and identified 17 significant metabolites, mainly related to tyrosine biosynthesis, glycerophospholipid metabolism, carnitine metabolism and bile acids.40 Li, X. Z. et al. used UPLC-QTOF-MS to study the pathogenesis of oral lichen planus and identified 12 regulated metabolites, which were presumed to be potential biomarkers.41 UPLC/MS was used to analyze urine samples from patients with acute coronary syndrome and healthy controls. Wang, Y. et al. found that fatty acid metabolism, fatty acid β-oxidative metabolism, amino acid metabolism and TCA cycle play an important role in acute coronary syndrome.42 Based on the metabolomics method of gas chromatography-MS, Wei, J. et al. studied the metabolic characteristics of systemic lupus erythematosus and found that it is mainly related to energy metabolism and amino acid metabolism pathways.43 Metabolomics technology is of great significance in the diagnosis of diseases, but this diagnosis is still one-sided. It should be closely integrated with modern medical technology to better understand the disease, understand the pathogenesis of the disease, and add protection to the health of patients.
4.2 Cancer research
Cancer is still a major problem, with tens of millions of people dying of cancer every year; therefore, how to treat and conquer cancer is a contemporary issue of the times. Understanding the causes of cancer and its influencing factors is important for understanding cancer. Because cancer is affected by many factors, such as environment, irrational diet, and genetic factors, researchers use metabolomics to describe the effects of various factors on cancer. For example, Yang, W. et al. used MS to explore the metabolic changes of ovarian cancer and identified 18 metabolites closely related to ovarian cancer.44 An, Y. et al. used LC/MS to study the metabolic changes of pancreatic cancer tissues and found that seven metabolites may be potential biomarkers.45 Jasbi, P. et al. used LC-MS/MS to identify metabolic markers of breast cancer and found that the affected metabolic pathways are closely related to tumor growth, metastasis and immune escape mechanisms.46 Fan, X. et al. used a combination of LC-MS and GC-MS to study the effect of Macranthoidin B on the total metabolites of colorectal cancer cells. It was found to inhibit colorectal cancer cell proliferation by inducing reactive oxygen species-mediated apoptosis.47 The problem of cancer has been plaguing humans, and the number of patients dying from cancer is increasing every year. An understanding of cancer mechanisms and appropriate treatments are urgently needed, and emerging technologies will face the enormous challenges of cancer, so there is a long way to go. Although metabolomics technology is used to discover the mechanism of action in cancer, cancer is a special disease that is difficult to cure, and clinical samples of cancer patients are difficult to obtain. Therefore, further development has been hindered.
4.3 Metabolic mechanism
Clearly clarifying the metabolic mechanism of a disease plays a crucial role in the treatment of the disease. Because the occurrence of diseases is affected by many factors, such as environmental and genetic factors, researchers use metabolomics techniques to describe the effects of various factors on disease mechanisms. Studies have shown that breviscapine plays a therapeutic role in mice with Alzheimer's disease caused by amyloid β1-42, and found that breviscapine mainly improves the metabolism of phospholipids by regulating the level of serotonin and so on. For the purpose of studying learning and memory impairment,48 Zhao, G. et al. investigated the hypoglycemic effect of Crassostrea gigas polysaccharides by UPLC-Q-TOF-MS. 19 metabolites were used as potential therapeutic biomarkers, mainly involved in amino acid metabolism, carbohydrate metabolism and purine metabolism.49 Ji, H. et al. used UPLC-ESI-QTOF-MS technology to find that aloe emodin has a good therapeutic effect on HFD-induced hyperlipidemia by regulating metabolic disorders.50 The metabolomics method is also used to study diseases such as arthritis. Pang, Z. et al. found that methotrexate can inhibit the development of arthritis by inhibiting the activation of NF-kappa B and NLRP3/caspase-1 pathways and regulating inflammatory-related metabolic networks.51 A disease is caused by the disorder of the action mechanism in the living body. However, different diseases may be influenced by multiple mechanisms of action. Therefore, researchers discuss the mechanism of action of the disease in order to find a new method for disease treatment. Rodrigues, D. et al. found that a GC-MS metabolomics-based approach for analysis of volatile organic compounds is a valuable strategy for identifying new and specific biomarkers that may improve bladder cancer diagnosis.52 Zhou, P. et al. have used ultra-performance liquid chromatography-mass spectrometry to characterize the fecal metabolome of Clostridium difficile positive and negative diarrhea and healthy control stool samples. They found that Clostridium difficile diarrhea has obvious fecal metabolome characteristics.53 Shou, Q. et al. applied metabolomics techniques to investigate the mechanism of paeoniflorin in the treatment of allergic asthma. They found that paeoniflorin can treat allergic asthma by regulating fatty acid metabolism, inflammation and system-level adhesion.54
Disorders of metabolic mechanisms are one of the crucial factors affecting a disease. The biological mechanism of the body in a normal state is in a relatively balanced state. The occurrence of a disease is tantamount to disruption of this relative equilibrium state. Studies have demonstrated that angiotensin-(1–7) can improve angiotensin II-stimulated metabolic perturbations associated with cardiac fibroblast activation. Pyroglutamic acid and arachidonic acid may be potential biomarkers for monitoring angiotensin-(1–7) anti-fibrotic effects.55 Matsutomo, T. et al. found that a single dose of S-1-propenylcysteine (S1PC) exerted an antihypertensive effect. They explored the potential mechanism of the antihypertensive effect of S1PC in spontaneously hypertensive rats using a metabolomic approach and found that S1PC alters histidine metabolism and consequently exerts an antihypertensive effect via the central histamine H3 receptor.56,57 The homeostasis of the metabolic mechanism depends not only on the stability of the internal environment, but also on individual differences. How to study the mechanism of action will be an important point to promote advances in medicine and biology.
4.4 Drug efficacy and screening
Traditional Chinese medicine theory and western medical theory are essentially different in nature, and applying metabolomics technology to understand the development process of a disease, and the theory of the holistic view, system view and dialectical view of Chinese medicine are inconsistent with each other, giving Chinese medicine a complicated theoretical system. Research provides new ideas and methods. The application of metabolomics technology in the field of traditional Chinese medicine is increasing, and it has gradually penetrated into various fields of traditional Chinese medicine research, such as the study of drugs, mechanisms of action and compatibility.
The research on traditional Chinese medicine is fairly divided into unit drug research and Chinese medicine compound research. The study of single-flavored drugs pays heed to the specific pharmacological effects, toxic and side effects, and metabolic mechanisms of the drugs; the research of the compound mainly studies the compatibility rules and metabolic pathways. Li, C. R. et al. found that the process of different treatments of the drug could be distinguished by metabolic methods.58 Bao, J. et al. used UPLC/MS-based metabolomics to rapidly identify anticancer compounds in Forsythia, and found that betulinic acid is the most effective anticancer compound in Forsythia suspensa.59 Yuan, A. et al. used UPLC-Q-TOF-MS/MS to study the anti-inflammatory mechanism of Forsythia extracts and Forsythia oil, and found that they exert anti-inflammatory effects by acting on different metabolic pathways.60 Zhuang, Y. et al. used UHPLC/MS to study the potential mechanism of Xanthii Fructus for allergic rhinitis. Twenty-seven potential biomarkers were discovered, mainly involved in metabolic pathways such as glycerophospholipid metabolism and branched-chain amino acid metabolism.61 Wang, Y. et al. found that gross saponins of Tribulus terrestris fruit can reverse middle cerebral artery occlusion-induced serum metabolic deviations by regulating multiple metabolic pathways.62 Zhou, J. et al. found that gastrointestinal injury was mainly related to vitamin B6 metabolism, phenylalanine metabolism, arachidonic acid metabolism, and taurine. Fructus Gardenia affects the normal functions of the gastrointestinal tract via regulating a variety of metabolic pathways to an abnormal state.63 It has become a mission of the times to develop and carry forward the traditional Chinese medicine culture, to understand the effect and mechanism of the efficacy of traditional Chinese medicine, and to recognize the role of traditional Chinese medicine more clearly, for it to be recognized at home and abroad.
Today, Chinese medicine and western medicine develop together. At the same time, they also pay attention to each other's treatment methods. The difference between Chinese medicine and western medicine is that western medicine has a single component, whereas traditional Chinese medicine has complex components, and the system plays a role in treating diseases. The sole component of western medicine has obvious effects on the treatment of diseases, but long-term use of western medicine alone will cause certain damage to the patient's body. Therefore, it is especially important to examine the metabolic mechanism of western medicine. Metabolomics research based on MS has paved the way for drug research.64 Liu, Z. et al. used GC-MS/MS to determine 9 common sulfonates in drugs. These alkyl sulfonates have been successfully used for the determination of capecitabine and imatinib mesylate, and can be further applied to other drugs.65
Metabolomics has a wide range of applications in animal model validation, drug screening, drug efficacy and toxicity evaluation, and clinical evaluation. Based on the metabolomics method of ultra-performance liquid chromatography combined with quadrupole time-of-flight mass spectrometry, the acute and chronic hepatotoxicity of gourd extract on mice was investigated.66 Kim, J. H. et al. used liquid chromatography-mass spectrometry-based metabolomics to assess the metabolic characteristics of ketoconazole in mice and humans and to determine the underlying mechanisms of its hepatotoxicity.67 Yan, H. et al. used HPLC-TOF-MS to investigate the toxicity of brodifacoum, and found that it mainly played a toxic role by disturbing metabolic pathways such as the tricarboxylic acid cycle and glycolysis.68 Wang, Z. et al. used UPLC-MS to study the hepatotoxicity of the sheath Genkwa Flos, and found that aminoglycan-1-phosphate metabolism and fatty acid metabolism disorders may be the key factors leading to liver injury.69 Zhao, D. S. et al. used gas chromatography-mass spectrometry to study the hepatotoxicity of Dioscorea bulbifera rhizomes, and found that they regulate amino acids, bile acids and energy metabolism to affect the physiological and biological functions of the liver.70 While the drug is treating the disease, it will also bring different degrees of damage to the body, mainly in terms of liver function and kidney function damage, etc. How to use metabolomics to determine the tissues and organs and their parts affected by drug toxicity, speculative drugs and the mechanism of action is critical to identifying potential biomarkers associated with toxicity. Liu, S. Y. et al. discovered that the protection mechanism in anti-ischemic cerebral reperfusion damage of Kudiezi injection was possibly related to the biosynthesis of phenylalanine, tyrosine, and tryptophan.71 Zhu, B. et al. studied the mechanism of Schisandra polysaccharide treatment of Alzheimer's disease, and found that it treats AD by reducing the degree of phosphorylation of tau protein, deposition of Aβ and oxidative damage.72
4.5 Nutrition
The application of metabolomics in the field of nutrition is called nutritional metabolomics, and refers to the systematic study of the interaction between diet and organism metabolism using metabolomics in different health and disease states of organisms. Vegetables and fruits are full of trace elements required by the human body, which can supplement the trace elements required by the human body. There are many factors affecting the content of trace elements in fruit. These include geographical factors and biological stress. Characteristics of metabolomics in nutrition can be described by a number of examples. Among them, Zhao, L. et al. used 1H NMR and GC-MS techniques to examine the changes of cucumber fruit metabolites under nano-Cu stress. They found that nano-copper exposure had an effect on the metabolic profile of fruit metabolites.73 Wang, Y. Q. et al. used a UHPLC-MS/MS-based metabolomics strategy to study the nutrient profiles of Chinese kale. It was found that the contents of components in different cabbage varieties were different.74 Rahman, M. M. et al. used LC-MS/MS to analyze dinitrofuran and its three metabolites in plums, which laid a foundation for the study of plum components, etc.75 Alolga, R. N. et al. used Q/TOF-MS technology to explore the difference in secondary metabolites and heavy metal content in dried ginger from different habitats. It is speculated that abiotic stress due to metals may lead to differences in metabolite abundance.76 Ramírez-Acosta, S. et al. used GC-MS technology to study the metabolic changes of strawberry fruit caused by controlled atmosphere treatment, and found that several metabolites related to it were mainly biologically active compounds.77 Kim, G. D. et al. used LC-MS/MS to identify caffeic acid derivatives and Danshensu as the main anti-inflammatory substances in the leaves of kiwi fruit plants.78
However, it is not only vegetables and fruits that provide nutrients, and many other substances provide essential proteins, lipids and sugars to the body. A study found that major royal jelly proteins improve spatial memory in aged rats by affecting niacin and nicotine metabolism, cysteine–taurine metabolism, and energy metabolism pathways.79 Wang, D. et al. used a new metabolomics strategy to detect the content of different metabolites in black sesame and white sesame, and found differences in the activity of phenylpropanol biosynthesis, tyrosine metabolism and riboflavin metabolism.80
Gil-Solsona, R. et al. used a metabolomic model to identify sensitive and robust biomarkers of malnutrition in cultured fish.81 Moreira, V. et al. used a metabolomic method based on MS to assess the effect of orange juice on blood lipid levels in healthy volunteers after two weeks of drinking orange juice. They found that orange juice intake may affect fatty acid β-oxidation through the mitochondria and peroxidase pathways.82 Saa, D. L. T. et al. used gas chromatography-mass spectrometry to study the volatile components of different baking products and explore ways to improve the quality of bread, providing new ideas for further study of nutrients in food.83 Xu, L. et al. used a UPLC-QTOF-based non-target metabolomics method to study the difference in coffee brewed by boiling. Nine potential biomarkers were identified.84
Liao, Y. et al. studied the metabolic difference of different beef cattle under heat stress by gas chromatography-mass spectrometry and found that Xuanhan yellow cattle have higher glycolytic activity and possess a stronger antioxidant defense system.85 Arapitsas, P. et al. used LC-MS to study the effects of storage conditions on the composition of wine. It was found that the storage conditions had significant effects on the metabolites, such as phenols and vitamins in red wine.86 At present, the world is facing the double problem of nutritional deficiencies and overnutrition. The prevalence of nutrition-related chronic diseases is increasing year by year. Nutritional metabolic diseases such as diabetes, high blood pressure and obesity are serious threats to national health. Studies have shown that personalized diets can change the metabolism of living organisms, which in turn affects the health status of living organisms.
4.6 Agronomy and plant biology
Metabolomics based on MS is increasingly used in different scientific fields, especially agronomy and plant biology, to understand the behavior of plants under different stress conditions.87 We have summarized several interesting examples to illustrate the application of metabolomics techniques in agriculture and the biotechnology industry. In the process of plant growth, a large number of elements are often required, and it is essential to explore the influence of elements on the growth laws of plants. Xu, S. et al. analyzed the metabolic characteristics of leaves and roots of soybean seedlings cultured under normal and excessive Mo conditions by UPLC-MS/MS. They speculated that organic compounds such as 2-oxo-arginine, L-nicotine, gluconic acid, D-gluconic acid and citric acid play an important role in the chelation of molybdenum and reducing its toxicity.88 Ghosson, H. et al. used metabolomics based on MS to investigate the effects of sulfur deficiency on metabolites, which laid the foundation for agricultural research. This is helpful to the management of fertilization in agriculture.89 Yang, N. et al. studied the metabolic responses of magnesium deficiency in soybean leaves and roots. It was found that there were significant differences in carbon and nitrogen metabolic responses between leaves and roots under magnesium deficiency.90
Nitrogen plays an important role in plant growth. Nitrogen is the major component of protein. Protein is the most basic substance that makes up plants. Nitrogen is also an important element in chlorophyll. Allwood, J. W. et al. studied the yield, grain quality and response of grain metabolites to increasing nitrogen application and found that nitrogen application had a significant effect on grain yield.91 Zhang, Y. et al. performed metabonomic analysis on two wheat varieties with different induced nitrogen levels, and found that flavonoids and their related derivatives were considered as potential biomarkers under low nitrogen stress.92 Herbicide residues are one of the important issues of concern in modern agriculture. How to control herbicide residues within a reasonable range is not only an important way to increase production, but also a safety guarantee for consumers. Du, P. et al. used UPLC and triple tandem quadrupole mass spectrometry to determine the content of sulfonylurea and its metabolites in rice and discussed the problem of herbicide residues.93
In addition to their significant application in agriculture, the plant and biological aspects still have a very wide range of applications. For example, an LC-MS-based metabolomics method was optimized for cyanobacterium Synechocystis sp. PCC 6803, and Pei, G. et al. detected 24 important carbohydrates and important metabolites related to energy metabolism.94 Mibei, E. K. and others used gas chromatography-mass metabolomics methods to characterize the effects of drought stress on metabolites in different growth stages of eggplants. Drought stress was found to have a significant effect on metabolites such as amino acids, sugars and organic acids.95 Qian, Y. et al. used UPLC/MS to investigate the effect of in vitro sucrose on the quality components of tea plants, and found that sucrose regulates polyphenol biosynthesis in tea plants by changing the expression of transcription factor genes and pathway genes.96 Not only this, but also the application trend in the field of agriculture and plant biology has become increasingly prominent. How to combine metabolomics technology with agriculture and plant biology will be key in the field of agriculture and plant biology. Metabolomics technology is widely used in crop information measurement, disease diagnosis, etc., and contributes to increasing agricultural income. The mutual integration of the two has opened up a new direction for the development of the discipline of metabolomics.
5. Limitations
Some analytical techniques have not been able to achieve comprehensive qualitative and quantitative analysis of all metabolites in all metabolic pathways in living organisms. There are limitations in metabolomics research and different analytical methods can achieve unusual results.97–102 At the same time, the testing instruments and equipment required for conducting metabolomics research are expensive, the required expertise of the operators are high, and there are certain restrictions on the promotion and application of metabolomics technology. Secondly, some non-experimental factors in the study can cause large interference with the results. Experiments have shown that various physiological factors, such as gender, age, diet, healthy animal strains, temperature and other stimuli, and even changes in climatic environment can affect the metabolites and metabolic profiles of organisms, thus complicating data analysis.103–110 At the same time, in metabolomics data analysis, although there are several commonly used databases, the number of collected metabolites needs to be improved. Many of the metabolites found during the research process are currently not confirmed by the database. Therefore, the metabolomics database needs to be further improved; again, in the process of metabolomics data analysis, statistical analysis methods such as principal component analysis and partial least squares are commonly used. Most of these methods are applied to linear data sets, and how to use them for nonlinear data is an outstanding issue. Effective extraction of metabolites is also a problem faced by metabolomics.111–119 This requires the latest research results in the fields of mathematics and statistics to be fully borrowed and absorbed into metabolomics research.120–125 Perfection of metabolomics still requires extensive effort. How to integrate traditional Chinese medicine theory with metabolomics has become a topic of the time.
6. Future perspectives
Metabolomics technology will be combined with more emerging technologies to provide a guarantee for the development of metabolomics. Metabolomics based on MS will be more perfect, and all kinds of combined techniques, such as LC/MS and GC/MS, will provide a scientific basis for solving the problem of metabolite research and elucidating metabolic pathways. Mass spectrometry continues to play a leading role as an important analytical tool in the field of metabolomics. Advances in this field have enabled the discovery of many putative disease biomarkers and provided insight into the pathogenesis of various diseases.126–129 Numerous studies have also been tested, including early disease detection, treatment prediction and prognosis, monitoring treatment and recurrence testing.130–139 Given the extreme complexity of biological mixtures, most MS analytical methods involve the use of LC, GC or CE. However, rapidly growing applications using evolving separation methods and protocols offer opportunities and challenges. Clearly, developments in chromatographic methods have been effective in separating metabolites and increasing the number of metabolites detected.140,141 However, one major challenge is the inability to compare and correlate the results of these studies, which are performed on the same or similar samples obtained by independent research groups. This is the main bottleneck in the development of this field. Other factors such as sample preparation, sample matrix and residual effects can also lead to data variability. To overcome these challenges, it is necessary to shift from the conventionally used relative metabolite concentration measurements to a more reliable absolute concentration determination, which will be independent of the analytic platform, method and protocol used. This method is not straightforward for MS, but it is very important.
7. Conclusion
Since the birth of metabolomics, its research has made great progress. As an emerging research technique, it also faces the challenges of methods and applications. Methodologically, the analytical instruments, analytical techniques, and data acquisition and data analysis require further improvement. From the application point of view, although a large number of important landmark metabolites related to genetic variation or physiological and pathological changes have been obtained, it is a huge challenge to establish a clinical predictive diagnostic expert system to achieve diagnostic rationalization. A challenge is accompanied by opportunities, so the challenges currently encountered in metabolomics are opportunities for future development. It is believed that with the continuous optimization and improvement of metabolomics research methods, drug safety evaluation will be more accurate and efficient, and the understanding of disease processes will be more comprehensive, and it may even become a powerful means to guide human health and monitor the human environment.
Conflicts of interest
There are no conflicts to declare.
Acknowledgements
This work was supported by grants from the Key Program of Natural Science Foundation of State (Grant No. 81830110, 8181101160, 81973745, 81903818, 81903847, 81430093, 81673586, 81703685, 81302905, 81503386, 81373930), National Key Research and Development Program of China (2018YFC1706103), National Key Subject of Drug Innovation (Grant No. 2015ZX09101043-005, 2015ZX09101043-011), TCM State Administration Subject of Public Welfare (Grant No. 2015468004), Major Projects of Application Technology Research and Development Plan in Heilongjiang Province (GX16C003), Natural Science Foundation of Heilongjiang Province (YQ2019H030, LH2019H056, H2016056), Chinese Postdoctoral Science Foundation (2017M621319b), University Nursing Program for Young Scholars with Creative Talents in Heilongjiang Province (UNPYSCT-2015118, UNPYSCT-2016213, UNPYSCT-2016212), Young Talent Lift Engineering Project of China Association of Traditional Chinese Medicine (QNRC2-B06), Foundation of Heilongjiang University of Chinese Medicine (2018jc01, 2018bs02, 201809), and Heilongjiang Touyan Innovation Team Program.
References
- R. Ju, X. Liu and F. Zheng, et al., Removal of false positive features to generate authentic peak table for high-resolution mass spectrometry-based metabolomics study, Anal. Chim. Acta, 2019, 1067, 79–87 CrossRef CAS PubMed.
- X. Wu, X. Sun and C. Zhao, et al., Exploring the pharmacological effects and potential targets of paeoniflorin on the endometriosis of cold coagulation and blood stasis model rats by ultra-performance liquid chromatography tandem mass spectrometry with a pattern recognition approach, RSC Adv., 2019, 9(36), 20796–20805 RSC.
- A. Amberg, B. Riefke and G. Schlotterbeck, et al., NMR and MS Methods for Metabolomics, Methods Mol. Biol., 2017, 1641, 229–258 CrossRef CAS PubMed.
- J. K. Nicholson, J. C. Lindon and E. Holmes, ‘Metabonomics’: Understanding the metabolic responses of living systems to pathophysiological stimuli via multivariate statistical analysis of biological NMR spectroscopic data, Xenobiotica, 1999, 29(11), 1181 CrossRef CAS PubMed.
- T. Andrew Clayton, J. C. Lindon and O. Cloarec, et al., Pharmaco-metabonomic phenotyping and personalized drug treatment, Nature, 2006, 440(7087), 1073–1077 CrossRef PubMed.
- A. Zhang, Z. Ma and H. Sun, et al., High-Throughput Metabolomics Evaluate the Efficacy of Total Lignans From Acanthophanax Senticosus Stem Against Ovariectomized Osteoporosis Rat, Front. Pharmacol., 2019, 10, 553 CrossRef PubMed.
- A. Zhang, H. Sun and P. Wang, et al., Salivary proteomics in biomedical research, Clin. Chim. Acta, 2013, 415, 261–265 CrossRef CAS PubMed.
- H. Dong, A. Zhang and H. Sun, et al., Ingenuity pathways analysis of urine metabolomics phenotypes toxicity of Chuanwu in Wistar rats by UPLC-Q-TOF-HDMS coupled with pattern recognition methods, Mol. BioSyst., 2012, 8(4), 1206–1221 RSC.
- C. K. Larive, G. A. Barding Jr and M. M. Dinges, NMR spectroscopy for metabolomics and metabolic profiling, Anal. Chem., 2015, 87(1), 133–146 CrossRef CAS PubMed.
- A. Zhang, H. Sun and G. Yan, et al., Mass spectrometry-based metabolomics: applications to biomarker and metabolic pathway research, Biomed. Chromatogr., 2016, 30(1), 7–12 CrossRef PubMed.
- A. Zhang, H. Sun and P. Wang, et al., Modern analytical techniques in metabolomics analysis, Analyst, 2012, 137(2), 293–300 RSC.
- X. Wang, X. Gao and A. Zhang, et al., High-throughput metabolomics for evaluating the efficacy and discovering the metabolic mechanism of Luozhen capsules from the excessive liver-fire syndrome of hypertension, RSC Adv., 2019, 9(55), 32141–32153 RSC.
- H. Sun, X. Li and A. Zhang, et al., Exploring potential biomarkers of coronary heart disease treated by Jing Zhi Guan Xin Pian using high-throughput metabolomics, RSC Adv., 2019, 9(20), 11420–11432 RSC.
- H. Sun, M. Wang and A. Zhang, et al., UPLC-Q-TOF-HDMS Analysis of Constituents in the Root of Two Kinds of Aconitum Using a Metabolomics Approach, Phytochem. Anal., 2013, 24(3), 263–276 CrossRef CAS PubMed.
- A. García, J. Godzien and Á. López-Gonzálvez, et al., Capillary electrophoresis mass spectrometry as a tool for untargeted metabolomics, Bioanalysis, 2017, 9(1), 99–130 CrossRef PubMed.
- A. Zhang, H. Sun and X. Wang, Potentiating therapeutic effects by enhancing synergism based on active constituents from traditional medicine, Phytother. Res., 2014, 28(4), 526–533 CrossRef PubMed.
- T. Mairinger, T. J. Causon and S. Hann, The potential of ion mobility-mass spectrometry for non-targeted metabolomics, Curr. Opin. Chem. Biol., 2018, 42, 9–15 CrossRef CAS PubMed.
- A. Zhang, H. Sun and S. Qiu, et al., NMR-based metabolomics coupled with pattern recognition methods in biomarker discovery and disease diagnosis, Magn. Reson. Chem., 2013, 51(9), 549–556 CrossRef CAS PubMed.
- C. C. Feng, A. H. Zhang and J. H. Miao, et al., Recent advances in understanding cross-talk between Bile Acids and Gut Microbiota, Open Journal of Proteomics and Genomics, 2018, 3(1), 024–034 Search PubMed.
- A. Zhang, H. Sun and S. Qiu, et al., Metabolomics in noninvasive breast cancer, Clin. Chim. Acta, 2013, 424, 3–7 CrossRef CAS PubMed.
- A. Zhang, S. Qiu and H. Xu, et al., Metabolomics in diabetes, Clin. Chim. Acta, 2014, 429, 106–110 CrossRef CAS PubMed.
- G. Yan, A. Zhang and H. Sun, et al., An effective method for determining the ingredients of Shuanghuanglian formula in blood samples using high-resolution LC–MS coupled with background subtraction and a multiple data processing approach, J. Sep. Sci., 2013, 36(19), 3191–3199 CAS.
- A. Zhang, H. Sun and G. Yan, et al., Serum proteomics in biomedical research: a systematic review, Appl. Biochem. Biotechnol., 2013, 170(4), 774–786 CrossRef CAS PubMed.
- X. Li, Y. Han and A. Zhang, et al., Mechanistic and Therapeutic Advances in Colon Cancer: A Systematic Review, Open Journal of Proteomics and Genomics, 2019, 4(1), 001–012 CrossRef.
- H. Johno, K. Yoshimura and Y. Mori, et al., Detection of potential new biomarkers of atherosclerosis by probe electrospray ionization mass spectrometry, Metabolomics, 2018, 14(4), 38 CrossRef PubMed.
- G. Tavares, G. Venturini and K. Padilha, et al., 1,5-Anhydroglucitol predicts CKD progression in macroalbuminuric diabetic kidney disease: results from non-targeted metabolomics, Metabolomics, 2018, 14(4), 39 CrossRef PubMed.
- M. Hao, D. Ji, L. Li and L. Su, et al., Mechanism of Curcuma wenyujin Rhizoma on Acute Blood Stasis in Rats Based on a UPLC-Q/TOF-MS Metabolomics and Network Approach, Molecules, 2018, 24(1), E82 CrossRef PubMed.
- R. A. Abdulwahab, A. Alaiya and Z. Shinwari, et al., LC-MS/MS proteomic analysis revealed novel associations of 37 proteins with T2DM and notable upregulation of immunoglobulins, Int. J. Mol. Med., 2019, 43(5), 2118–2132 CAS.
- G. Yang, G. Zhao and J. Zhang, et al., Global urinary metabolic profiling of the osteonecrosis of the femoral head based on UPLC-QTOF/MS, Metabolomics, 2019, 15(3), 26 CrossRef PubMed.
- S. Lin, X. Yue and H. Wu, et al., Explore potential plasma biomarkers of acute respiratory distress syndrome (ARDS) using GC-MS metabolomics analysis, Clin. Biochem., 2019, 66, 49–56 CrossRef CAS PubMed.
- M. A. Øvrehus, P. Bruheim, W. Ju and L. R. Zelnick, et al., Gene Expression Studies and Targeted Metabolomics Reveal Disturbed Serine, Methionine, and Tyrosine Metabolism in Early Hypertensive Nephrosclerosis, Kidney International Reports, 2018, 4(2), 321–333 CrossRef PubMed.
- J. Barbosa-Breda, U. Himmelreich and B. Ghesquière, et al., Clinical Metabolomics and Glaucoma, Ophthalmic Res., 2018, 59(1), 1–6 CrossRef CAS PubMed.
- S. Dong, Z. Y. Zhan and H. Y. Cao, et al., Urinary metabolomics analysis identifies key biomarkers of different stages of nonalcoholic fatty liver disease, World J. Gastroenterol., 2017, 23(15), 2771–2784 CrossRef CAS PubMed.
- D. Wang, J. Kong and J. Wu, et al., GC-MS-based metabolomics identifies an amino acid signature of acute ischemic stroke, Neurosci. Lett., 2017, 642, 7–13 CrossRef CAS PubMed.
- Q. Li, S. Wei and D. Wu, et al., Urinary Metabolomics Study of Patients with Gout Using Gas Chromatography-Mass Spectrometry, BioMed Res. Int., 2018, 2018, 3461572 Search PubMed.
- H. Sun, J. Zhao and D. Zhong, et al., Potential serum biomarkers and metabonomic profiling of serum in ischemic stroke patients using UPLC/Q-TOF MS/MS, PLoS One, 2017, 12(12), e0189009 CrossRef PubMed.
- H. Lu, J. Ding and W. Liu, et al., UPLC/MS-Based Metabolomics Investigation of the Protective Effect of Hydrogen Gas Inhalation on Mice with Calcium Oxalate-Induced Renal Injury, Biol. Pharm. Bull., 2018, 41(11), 1652–1658 CrossRef CAS PubMed.
- H. Zhao, Y. Liu and Z. Li, et al., Identification of essential hypertension biomarkers in human urine by non-targeted metabolomics based on UPLC-Q-TOF/MS. Clinica Chimica Acta, 486, 192–198, Clin. Chim. Acta, 2018, 486, 192–198 CrossRef CAS PubMed.
- K. Dittharot, P. Jittorntam and P. Wilairat, et al., Urinary Metabolomic Profiling in Chronic Hepatitis B Viral Infection
Using Gas Chromatography/Mass Spectrometry, Asian Pac. J. Cancer Prev., 2018, 19(3), 741–748 CAS.
- H. Zhao, C. Wang and N. Zhao, et al., Potential biomarkers of Parkinson's disease revealed by plasma metabolic profiling, J Chromatogr B Analyt Technol Biomed Life Sci, 2018, 1081–1082, 101–108 CrossRef CAS PubMed.
- X. Z. Li, X. Y. Yang and Y. Wang, et al., Urine metabolic profiling for the pathogenesis research of erosive oral lichen planus, Arch. Oral Biol., 2017, 73, 206–213 CrossRef CAS PubMed.
- Y. Wang, W. Sun and J. Zheng, et al., Urinary metabonomic study of patients with acute coronary syndrome using UPLC-QTOF/MS, J Chromatogr B Analyt Technol Biomed Life Sci, 2018, 1100–1101, 122–130 CrossRef CAS PubMed.
- J. Wei, J. Gao and X. Ding, Exploring the mechanism of Jieduquyuziyin prescription on systemic lupus erythematosus by GC-MS-based urine metabolomics, Biomed. Chromatogr., 2018, 32(2), e4087 CrossRef PubMed.
- W. Yang, T. Mu and J. Jiang, et al., Identification of Potential Biomarkers and Metabolic Profiling of Serum in Ovarian Cancer Patients Using UPLC/Q-TOF MS, Cell. Physiol. Biochem., 2018, 51(3), 1134–1148 CrossRef CAS PubMed.
- Y. An, H. Cai and Y. Yang, et al., Identification of ENTPD8 and cytidine in pancreatic cancer by metabolomic and transcriptomic conjoint analysis, Cancer Sci., 2018, 109(9), 2811–2821 CrossRef CAS PubMed.
- P. Jasbi, D. Wang and S. L. Cheng, et al., Breast cancer detection using targeted plasma metabolomics, J Chromatogr B Analyt Technol Biomed Life Sci, 2019, 1105, 26–37 CrossRef CAS PubMed.
- X. Fan, J. Rao and Z. Zhang, et al., Macranthoidin B Modulates Key Metabolic Pathways to Enhance ROS Generation and Induce Cytotoxicity and Apoptosis in Colorectal Cancer, Cell. Physiol. Biochem., 2018, 46(4), 1317–1330 CrossRef CAS PubMed.
- H. Xia, L. Wu and M. Chu, et al., Effects of breviscapine on amyloid beta 1-42 induced Alzheimer's disease mice: A HPLC-QTOF-MS based plasma metabonomics study, J Chromatogr B Analyt Technol Biomed Life Sci, 2017, 1057, 92–100 CrossRef CAS PubMed.
- G. Zhao, X. Hou and X. Li, et al., Metabolomics analysis of alloxan-induced diabetes in mice using UPLC-Q-TOF-MS after Crassostrea gigas polysaccharide treatment, Int. J. Biol. Macromol., 2018, 108, 550–557 CrossRef CAS PubMed.
- H. Ji, Y. Liu and F. He, et al., LC-MS based urinary metabolomics study of the intervention effect of aloe-emodin on hyperlipidemia rats, J. Pharm. Biomed. Anal., 2018, 156, 104–115 CrossRef CAS PubMed.
- Z. Pang, G. Wang and N. Ran, et al., Inhibitory Effect of Methotrexate on Rheumatoid Arthritis Inflammation and Comprehensive Metabolomics Analysis Using Ultra-Performance Liquid Chromatography-Quadrupole Time of Flight-Mass Spectrometry (UPLC-Q/TOF-MS), Int. J. Mol. Sci., 2018, 19(10), E2894 CrossRef PubMed.
- D. Rodrigues, J. Pinto and A. M. Araújo, et al., Volatile metabolomic signature of bladder cancer cell lines based on gas chromatography-mass spectrometry, Metabolomics, 2018, 14(5), 62 CrossRef PubMed.
- P. Zhou, N. Zhou and L. Shao, et al., Diagnosis of Clostridium difficile infection using an UPLC-MS based metabolomics method, Metabolomics, 2018, 14(8), 102 CrossRef PubMed.
- Q. Shou, L. Jin and J. Lang, et al., Integration of Metabolomics and Transcriptomics Reveals the Therapeutic Mechanism Underlying Paeoniflorin for the Treatment of Allergic Asthma, Front. Pharmacol., 2019, 9, 1531 CrossRef PubMed.
- Y. L. Chen, J. Fan and L. Cao, et al., Unique mechanistic insights into the beneficial effects of angiotensin-(1–7) on the prevention of cardiac fibrosis: A metabolomic analysis of primary cardiac fibroblasts, Exp. Cell Res., 2019, 378(2), 158–170 CrossRef CAS PubMed.
- T. Matsutomo, M. Ushijima and K. Kunimura, et al., Metabolomic study reveals the acute hypotensive effect of S-1-propenylcysteine accompanied by alteration of the plasma histidine level in spontaneously hypertensive rats, J. Pharm. Biomed. Anal., 2019, 168, 148–154 CrossRef CAS PubMed.
- X. Yan, Y. Zhuo and X. Bian, et al., Integrated Proteomics, Biological Functional Assessments, and Metabolomics Reveal Toosendanin-Induced Hepatic Energy Metabolic Disorders, Chem. Res. Toxicol., 2019, 32(4), 668–680 Search PubMed.
- C.-R. Li, M.-N. Li and H. Yang, et al., Rapid characterization of chemical markers for discrimination of Moutan Cortex and its processed products by direct injection-based mass spectrometry profiling and metabolomic method, Phytomedicine, 2018, 45, 76–83 CrossRef CAS PubMed.
- J. Bao, R. B. Ding and X. Jia, et al., Fast identification of anticancer constituents in Forsythiae Fructus based on metabolomicsapproaches, J. Pharm. Biomed. Anal., 2018, 154, 312–320 CrossRef CAS PubMed.
- A. Yuan, L. Gong and L. Luo, et al., Revealing anti-inflammation mechanism of water-extract and oil of forsythiae fructus on carrageenan-Induced edema rats by serum metabolomics, Biomed. Pharmacother., 2017, 95, 929–937 CrossRef CAS PubMed.
- Y. Zhuang, K. Qin and B. Yu, et al., A metabolomics research based on UHPLC-ESI-Q-TOF-MS coupled with metabolic pathway analysis: Treatment effects of stir-frying Xanthii Fructus on allergic rhinitis in mice model, Biomed. Chromatogr., 2018, 32(12), e4352 CrossRef PubMed.
- Y. Wang, H. Zhao and Y. Liu, et al., GC-MS-Based Metabolomics to Reveal the Protective Effect of Gross Saponins of Tribulus terrestris Fruit against Ischemic Stroke in Rat, Molecules, 2019, 24(4), E793 CrossRef PubMed.
- J. Zhou, N. Yao and S. Wang, et al., Fructus Gardeniae-induced gastrointestinal injury was associated with the inflammatory response mediated by the disturbance of vitamin B6, phenylalanine, arachidonic acid, taurine and hypotaurine metabolism, J. Ethnopharmacol., 2019, 235, 47–55 CrossRef CAS PubMed.
- A. F. Nassar, T. Wu and S. F. Nassar, et al., UPLC-MS for metabolomics: a giant step forward in support of pharmaceutical research, Drug Discovery Today, 2017, 22(2), 463–470 CrossRef CAS PubMed.
- Z. Liu, H. Fan and Y. Zhou, et al., Development and validation of a sensitive method for alkyl sulfonate genotoxic impurities determination in drug substances using gas chromatography coupled to triple quadrupole mass spectrometry, J. Pharm. Biomed. Anal., 2019, 168, 23–29 CrossRef CAS PubMed.
- Z. Wei, Q. Qian and X. Dong, et al., Metabolomic approach to understand the acute and chronic hepatotoxicity of Veratrum nigrum extract in mice based on ultra-high-performance liquid chromatography coupled with quadrupole time-of-flight mass spectrometry, Toxicol. Mech. Methods, 2017, 27(9), 687–696 CrossRef CAS PubMed.
- J.-H. Kim, W.-G. Choi and S. Lee, et al., Revisiting the Metabolism and Bioactivation of Ketoconazole in Human and Mouse Using Liquid Chromatography-Mass Spectrometry-Based Metabolomics, Int. J. Mol. Sci., 2017, 18(3), E621 CrossRef PubMed.
- H. Yan, X. Y. Zhuo and B. H. Shen, et al., Evaluation of Brodifacoum-induced Toxicity by Metabonomics ApproachBased on HPLC-TOF-MS, Fayixue Zazhi, 2017, 33(3), 247–251 CAS.
- Z. Wang, Y. Zhang and Q. Liu, et al., Investigation of the mechanisms of Genkwa Flos hepatotoxicity by a cell metabolomics strategy combined with serum pharmacology in HL-7702 liver cells, Xenobiotica, 2019, 49(2), 216–226 CrossRef CAS PubMed.
- D. S. Zhao, L. L. Jiang and Y. X. Fan, et al., Investigation of Dioscorea bulbifera Rhizome-Induced Hepatotoxicity in Rats by a Multisample Integrated Metabolomics Approach, Chem. Res. Toxicol., 2017, 30(10), 1865–1873 Search PubMed.
- S. Y. Liu, W. Cai and F. Wang, et al., UHPLC-LTQ-Orbitrap-based metabolomics coupled with metabolomics pathway analysis method for exploring the protection mechanism of Kudiezi injection in a rat anti-ischemic cerebral reperfusion damage model, Chin. J. Nat. Med., 2017, 15(12), 955–960 Search PubMed.
- B. Zhu, H. Cao and L. Sun, et al., Metabolomics-based mechanisms exploration of Huang-Lian Jie-Du decoction on cerebral ischemia via UPLC-Q-TOF/MS analysis on rat serum, J. Ethnopharmacol., 2018, 216, 147–156 CrossRef CAS PubMed.
- L. Zhao, J. Hu, Y. Huang and H. Wang, et al., A. 1H NMR and GC-MS based metabolomics reveal nano-Cu altered cucumber (Cucumis sativus) fruit nutritional supply, Plant Physiol. Biochem., 2017, 110, 138–146 CrossRef CAS PubMed.
- Y. Q. Wang, L. P. Hu and G. M. Liu, et al., Evaluation of the Nutritional Quality of Chinese Kale (Brassica alboglabra Bailey) Using UHPLC-Quadrupole-Orbitrap MS/MS-Based Metabolomics, Molecules, 2017, 22(8), E1262 CrossRef PubMed.
- M. M. Rahman, A. M. Abd El-Aty and M. H. Kabir, et al., A quick and effective methodology for analyzing dinotefuran and its highly polar metabolites in plum using liquid chromatography-tandem mass spectrometry, Food Chem., 2018, 239, 1235–1243 CrossRef CAS PubMed.
- R. N. Alolga and M. A. S. C. Chavez, et al., Untargeted UPLC-Q/TOF-MS-based metabolomics and inductively coupled plasma optical emission spectroscopic analysis reveal differences in the quality of ginger from two provinces in Zambia, J. Pharm. Pharmacol., 2018, 70(9), 1262–1271 CrossRef CAS PubMed.
- S. Ramírez-Acosta, A. Arias-Borrego and J. L. Gómez-Ariza, et al., Metabolomic study of bioactive compounds in strawberries preserved under controlled atmosphere based on GC-MS and DI-ESI-QqQ-TOF-MS, Phytochem. Anal., 2019, 30(2), 198–207 CrossRef PubMed.
- G. D. Kim, J. Y. Lee and J. H. Auh, Metabolomic Screening of Anti-Inflammatory Compounds from the Leaves of Actinidia arguta(Hardy Kiwi), Foods, 2019, 8(2), E47 CrossRef PubMed.
- D. Chen, F. Liu, J.-B. Wan, C. Q. Lai and L. R. Shen, et al., Effect of Major Royal Jelly Proteins on Spatial Memory in Aged Rats: Metabolomics Analysis in Urine, J. Agric. Food Chem., 2017, 65(15), 3151–3159 CrossRef CAS PubMed.
- D. Wang, L. Zhang and X. Huang, et al., Identification of Nutritional Components in Black Sesame Determined by Widely Targeted Metabolomics and Traditional Chinese Medicines, Molecules, 2018, 23(5), E1180 CrossRef PubMed.
- R. Gil-Solsona, J. Nácher-Mestre and L. Lacalle-Bergeron, et al., Untargeted metabolomics approach for unraveling robust biomarkers of nutritional status in fasted gilthead sea bream (Sparus aurata), PeerJ, 2017, 5, e2920 CrossRef PubMed.
- V. Moreira, E. Brasili, J. Fiamoncini and F. Marini, et al., Orange juice affects acylcarnitine metabolism in healthy volunteers as revealed by a mass-spectrometry based metabolomics approach, Food Res. Int., 2018, 107, 346–352 CrossRef CAS PubMed.
- D. L. T. Saa, L. Nissen and A. Gianotti, Metabolomic approach to study the impact of flour type and fermentation process on volatile profile of bakery products, Food Res. Int., 2019, 119, 510–516 CrossRef CAS PubMed.
- L. Xu, F. Lao, Z. Xu and X. Wang, et al., Use of Liquid Chromatography Quadrupole Time-of-Flight Mass Spectrometry and Metabolomic Approach to Discriminate Coffee Brewed by Different Methods, Food Chem., 2019, 286, 106–112 CrossRef CAS PubMed.
- Y. Liao, R. Hu, Z. Wang and Q. Peng, et al., Metabolomics Profiling of Serum and Urine in Three Beef Cattle Breeds Revealed Different Levels of Tolerance to Heat Stress, J. Agric. Food Chem., 2018, 66(26), 6926–6935 CrossRef CAS PubMed.
- P. Arapitsas, A. D. Corte, H. Gika and L. Narduzzi, et al., Studying the effect of storage conditions on the metabolite content of red wine using HILIC LC-MS based metabolomics, Food Chem., 2016, 197, 1331–1340 CrossRef CAS PubMed.
- R. M. do Prado, C. Porto, E. Nunes and C. L. de Aguiar, et al., Metabolomics and Agriculture: What Can Be Done?, mSystems, 2018, 3(2), e00156-17 CrossRef PubMed.
- S. Xu, C. Hu, S. Hussain and Q. Tan, et al., Metabolomics analysis reveals potential mechanisms of tolerance to excess molybdenum in soybean seedlings, Ecotoxicol. Environ. Saf., 2018, 164, 589–596 CrossRef CAS PubMed.
- H. Ghosson, A. Schwarzenberg and F. Jamois, et al., Simultaneous untargeted and targeted metabolomics profiling of underivatized primary metabolites in sulfur-deficient barley by ultra-high performance liquid chromatography-quadrupole/time-of-flight mass spectrometry, Plant Methods, 2018, 14, 62 CrossRef PubMed.
- N. Yang, J. Jiang and H. Xie, et al., Metabolomics Reveals Distinct Carbon and Nitrogen Metabolic Responses to Magnesium Deficiency in Leaves and Roots of Soybean [Glycine max (Linn.) Merr.], Front. Plant Sci., 2017, 8, 2091 CrossRef PubMed.
- J. W. Allwood, Y. Xu, P. Martinez-Martin and C. Howarth, et al., Rapid UHPLC-MS metabolite profiling and phenotypic assays reveal genotypic impacts of nitrogen supplementation in oats, Metabolomics, 2019, 15(3), 42 CrossRef PubMed.
- Y. Zhang, X. M. Ma and X. C. Wang, et al., UPLC-QTOF analysis reveals metabolomic changes in the flag leaf of wheat (Triticum aestivum L.) under low-nitrogen stress, Plant Physiol. Biochem., 2017, 111, 30–38 CrossRef CAS PubMed.
- P. Du, X. Wu and J. Xu, et al., Determination and dissipation of mesotrione and its metabolites in rice using UPLC and triple-quadrupole tandem mass spectrometry, Food Chem., 2017, 229, 260–267 CrossRef CAS PubMed.
- G. Pei, X. Niu and Y. Zhou, et al., Crosstalk of two-component signal transduction systems in regulating central carbohydrate and energy metabolism during autotrophic and photomixotrophic growth of Synechocystis sp. PCC6803, Integr. Biol., 2017, 9(5), 485–496 CrossRef CAS PubMed.
- E. K. Mibei, W. O. Owino and J. Ambuko, et al., Metabolomic analyses to evaluate the effect of drought stress on selected African Eggplant accessions, J. Sci. Food Agric., 2018, 98(1), 205–216 CrossRef CAS PubMed.
- Y. Qian, S. Zhang and S. Yao, et al., Effects of vitro sucrose on quality components of tea plants (Camellia sinensis) based on transcriptomic and metabolic analysis, BMC Plant Biol., 2018, 18(1), 121 CrossRef PubMed.
- J. P. Koelmel, C. Z. Ulmer and C. M. Jones, et al., Common cases of improper lipid annotation using high-resolution tandem mass spectrometry data an corresponding limitations in biological interpretation, Biochim. Biophys. Acta, Mol. Cell Biol. Lipids, 2017, 1862(8), 766–770 CrossRef CAS PubMed.
- P. M. Vaysse, R. M. A. Heeren and T. Porta, et al., Mass spectrometry imaging for clinical research-latest developments, applications, and currentlimitations, Analyst, 2017, 142(15), 2690–2712 RSC.
- Y. F. Li, S. Qiu and L. J. Gao, et al., Metabolomic estimation of the diagnosis of hepatocellular carcinoma based on ultrahigh performance liquid chromatography coupled with time-of-flight mass spectrometry, RSC Adv., 2018, 8(17), 9375–9382 RSC.
- H. Wang, G. Yan and A. Zhang, et al., Rapid discovery and global characterization of chemical constituents and rats metabolites of Phellodendri amurensis cortex by ultra-performance liquid chromatography-electrospray ionization/quadrupole-time-of-flight mass spectrometry coupled with pattern recognition approach, Analyst, 2013, 138(11), 3303–3312 RSC.
- X. Wang, J. Li and A. H. Zhang, Urine metabolic phenotypes analysis of extrahepatic cholangiocarcinoma disease using ultra-high performance liquid chromatography-mass spectrometry, RSC Adv., 2016, 6(67), 63049–63057 RSC.
- A. Zhang, H. Sun and G. Yan, et al., Metabolomics study of type 2 diabetes using ultra-performance LC-ESI/quadrupole-TOF high-definition MS coupled with pattern recognition methods, J. Physiol. Biochem., 2014, 70(1), 117–128 CrossRef CAS PubMed.
- A. Zhang, H. Sun and S. Qiu, et al., Metabolomics insights into pathophysiological mechanisms of nephrology, Int. Urol. Nephrol., 2014, 46(5), 1025–1030 CrossRef CAS PubMed.
- H. Sun, A. H. Zhang and Q. Song, et al., Functional metabolomics discover pentose and glucuronate interconversion pathways as promising targets for Yang Huang syndrome treatment with Yinchenhao Tang, RSC Adv., 2018, 8, 36831–36839 RSC.
- A. H. Zhang, J. B. Yu and H. Sun, et al., Identifying quality-markers from Shengmai San protects against transgenic mouse model of Alzheimer's disease using chinmedomics approach, Phytomedicine, 2018, 45, 84–92 CrossRef CAS PubMed.
- A. Zhang, H. Sun and H. Xu, et al., Cell metabolomics, OMICS, 2013, 17(10), 495–501 CrossRef CAS PubMed.
- X. Wang, A. Zhang and X. Zhou, et al., An integrated chinmedomics strategy for discovery of effective constituents from traditional herbal medicine, Sci. Rep., 2016, 6, 18997 CrossRef CAS PubMed.
- H. Fang, A. H. Zhang and H. Sun, et al., High-throughput metabolomics screen coupled with multivariate statistical analysis identifies therapeutic targets in alcoholic liver disease rats using liquid chromatography-mass spectrometry, J Chromatogr B Analyt Technol Biomed Life Sci, 2019, 1109, 112–120 CrossRef CAS PubMed.
- H. Cao, A. Zhang and H. Zhang, et al., The application of metabolomics in traditional Chinese medicine opens up a dialogue between Chinese and Western medicine, Phytother. Res., 2015, 29(2), 159–166 CrossRef PubMed.
- X. Liu, A. Zhang and H. Fang, et al., Serum metabolomics strategy for understanding the therapeutic effects of Yin-Chen-Hao-Tang against Yanghuang syndrome, RSC Adv., 2018, 8, 7403–7413 RSC.
- Y. Zhao, H. Lv and S. Qiu, et al., Plasma metabolic profiling and novel metabolite biomarkers for diagnosing prostate cancer, RSC Adv., 2017, 7(48), 30060–30069 RSC.
- X. N. Li, A. Zhang and M. Wang, et al., Screening the active compounds of Phellodendri Amurensis cortex for treating prostate cancer by
high-throughput chinmedomics, Sci. Rep., 2017, 7, 46234 CrossRef CAS PubMed.
- A. Zhang, H. Sun and Y. Han, et al., Urinary metabolic biomarker and pathway study of hepatitis B virus infected patients based on UPLC-MS system, PLoS One, 2013, 8(5), e64381 CrossRef CAS PubMed.
- H. Sun, A. Zhang and X. Wang, Potential role of metabolomic approaches for Chinese medicine syndromes and herbal medicine, Phytother. Res., 2012, 26(10), 1466–1471 CAS.
- A. Zhang, H. Sun and P. Wang, et al., Salivary proteomics in biomedical research, Clin. Chim. Acta, 2013, 415, 261–265 CrossRef CAS PubMed.
- A. Zhang, H. Sun and G. Yan, et al., Metabolomics in diagnosis and biomarker discovery of colorectal cancer, Cancer Lett., 2014, 345(1), 17–20 CrossRef CAS PubMed.
- A. Zhang, H. Sun and Y. Han, et al., Exploratory urinary metabolic biomarkers and pathways using UPLC-Q-TOF-HDMS coupled with pattern recognition approach, Analyst, 2012, 137(18), 4200–4208 RSC.
- A. Zhang, H. Sun and G. Yan, et al., Mass spectrometry-based metabolomics: applications to biomarker and metabolic pathway research, Biomed. Chromatogr., 2016, 30(1), 7–12 CrossRef PubMed.
- H. Sun, A. Zhang and G. Yan, et al., Metabolomic analysis of key regulatory metabolites in hepatitis C virus-infected tree shrews, Mol. Cell. Proteomics, 2013, 12(3), 710–719 CrossRef CAS PubMed.
- A. Zhang, H. Sun and X. Wang, Potentiating therapeutic effects by enhancing synergism based on active constituents from traditional medicine, Phytother. Res., 2014, 28(4), 526–533 CrossRef PubMed.
- A. Zhang, H. Sun and P. Wang, et al., Future perspectives of personalized medicine in traditional Chinese medicine: a systems biology approach, Complement. Ther. Med., 2012, 20(1–2), 93–99 CrossRef PubMed.
- H. Chu, A. Zhang and Y. Han, et al., Metabolomics approach to explore the effects of Kai-Xin-San on Alzheimer's disease using UPLC/ESI-Q-TOF mass spectrometry, J. Chromatogr. B: Anal. Technol. Biomed. Life Sci., 2016, 1015, 50–61 CrossRef PubMed.
- A. Zhang, H. Sun and G. Yan, et al., Metabolomics for biomarker discovery: moving to the clinic, BioMed Res. Int., 2015, 2015, 354671 Search PubMed.
- A. Zhang, H. Sun and X. Wang, Emerging role and recent applications of metabolomics biomarkers in obesity disease research, RSC Adv., 2017, 7(25), 14966–14973 RSC.
- H. Sun, H. Wang and A. Zhang, et al., Berberine ameliorates nonbacterial prostatitis via multi-target metabolic network regulation, OMICS, 2015, 19(3), 186–195 CrossRef CAS PubMed.
- H. Xiong, A. H. Zhang and Q. Q. Zhao, et al., Discovery and screening quality-marker ingredients of Panax quinquefolius using chinmedomics approach, Phytomedicine, 2019, 152928 CrossRef PubMed.
- A. Zhang, H. Wang and H. Sun, et al., Metabolomics strategy reveals therapeutical assessment of limonin on nonbacterial prostatitis, Food Funct., 2015, 6(11), 3540–3549 RSC.
- H. L. Zhang, A. H. Zhang and X. H. Zhou, et al., High-throughput lipidomics reveal mirabilite regulating lipid metabolism as anticancer therapeutics, RSC Adv., 2018, 8(62), 35600–35610 RSC.
- H. Sun, A. Zhang and L. Yang, et al., High-throughput chinmedomics strategy for discovering the quality-markers and potential targets for Yinchenhao decoction, Phytomedicine, 2019, 54, 328–338 CrossRef CAS PubMed.
- Y. Zhang, P. Liu and Y. Li, et al., Exploration of metabolite signatures using high-throughput mass spectrometry coupled with multivariate data analysis, RSC Adv., 2017, 7, 6780–6787 RSC.
- L. Wang, H. Dong and A. H. Zhang, et al., Exploring the detoxification effects and mechanism of Caowu in prescription using liquid chromatography-high-resolution mass spectrometry-based metabolomics, Open Journal of Proteomics and Genomics, 2018, 3(1), 011–023 Search PubMed.
- A. H. Zhang, H. Sun and G. L. Yan, et al., Chinmedomics: A Powerful
Approach Integrating Metabolomics with Serum Pharmacochemistry to Evaluate the Efficacy of Traditional Chinese Medicine, Engineering, 2019, 5, 60–68 CrossRef CAS.
- H. Sun, H. L. Zhang and A. H. Zhang, et al., Network pharmacology combined with functional metabolomics discover bile acid metabolism as a promising target for mirabilite against colorectal cancer, RSC Adv., 2018, 8, 30061–30070 RSC.
- Q. Liang, H. Liu and X. Li, et al., High-throughput metabolomics analysis discovers salivary biomarkers for predicting mild cognitive impairment and Alzheimer's disease, RSC Adv., 2016, 6, 75499–75504 RSC.
- Y. Nan, X. Zhou and Q. Liu, et al., Serum metabolomics strategy for understanding pharmacological effects of ShenQi pill acting on kidney yang deficiency syndrome, J. Chromatogr. B: Anal. Technol. Biomed. Life Sci., 2016, 1026, 217–226 CrossRef CAS PubMed.
- J. Xie, A. H. Zhang and S. Qiu, Identification of the perturbed metabolic pathways associating with prostate cancer cells and anticancer affects of obacunone, J. Proteomics, 2019, 206, 103447 CrossRef CAS PubMed.
- X. J. Wang, J. L. Ren and A. H. Zhang, et al., Novel applications of mass spectrometry-based metabolomics in herbal medicines and its active ingredients: Current evidence, Mass Spectrom. Rev., 2019, 38(4–5), 380–402 CrossRef PubMed.
- H. Sun, A. H. Zhang and S. B. Liu, et al., Cell metabolomics identify regulatory pathways and targets of magnoline against prostate cancer, J Chromatogr B Analyt Technol Biomed Life Sci, 2018, 1102–1103, 143–151 CrossRef CAS PubMed.
- H. Zhang, A. Zhang and J. Miao, et al., Targeting regulation of tryptophan metabolism for colorectal cancer therapy: a systematic review, RSC Adv., 2019, 9, 3072–3080 RSC.
- X. Li, A. Zhang and H. Sun, et al., Metabolic characterization and pathway analysis of berberine protects against prostate cancer, Oncotarget, 2017, 8, 65022–65041 Search PubMed.
- H. Fang, A. Zhang and J. Yu, et al., Insight into the metabolic mechanism of scoparone on biomarkers for inhibiting Yanghuang syndrome, Sci. Rep., 2016, 6, 37519 CrossRef CAS PubMed.
|
This journal is © The Royal Society of Chemistry 2019 |
Click here to see how this site uses Cookies. View our privacy policy here.