DOI:
10.1039/C9RA08970E
(Paper)
RSC Adv., 2019,
9, 41088-41098
Network pharmacology and RNA sequencing studies on triterpenoid saponins from Bupleurum chinense for the treatment of breast cancer†
Received
31st October 2019
, Accepted 26th November 2019
First published on 12th December 2019
Abstract
Breast cancer remains the most commonly diagnosed malignancy among women, which is frequently associated with adverse side-effects and high metastasis. Bupleurum chinense DC. has been empirically and extensively used as the core prescription for more than half of Chinese formulations for the adjuvant therapy of breast cancer, and its biological activity against breast cancer has been proven by both in vitro and in vivo experiments. Saikosaponin compounds are the characteristic constituent of B. chinense, which exhibit significant cytotoxicity toward several cancer cells. However, the specific mechanisms of these compounds in the treatment of breast cancer have not been comprehensively understood. Therefore, we aimed to determine more potentially therapeutic targets and investigate the biological mechanisms of B. chinense. In the present study, we adopted network pharmacology and bioinformatics analysis to facilitate this requirement. Consequently, the network analysis revealed that saikosaponin-f (39), saikosaponin-d (14), saikosaponin-c (26), saikosaponin-h (54), saikosaponin-g (41), 3′′,6′′-O-diacetylsaikosaponin-d (20), 11α-methoxy-saikosaponin-f (40), and 6′′-O-acetylsaikosaponin-b1 (48) might play important roles in the treatment of breast cancer. In addition, the apoptosis regulator Bcl-2 (BCL-2), C-X-C chemokine receptor type 4 (CXCR4), probable ATP-dependent RNA helicase DDX5 (DDX5), protein kinase C alpha (PRKCA), and proto-oncogene tyrosine-protein kinase Src (SRC) were the potential therapeutic targets that exhibited intense interactions. Mechanistically, a gene enrichment analysis revealed that the action of B. chinense against breast cancer was achieved by the regulation of several biological signaling pathways, such as pathways in cancer, PI3K-Akt signaling pathway, EGFR tyrosine kinase inhibitor resistance, microRNAs in cancer, etc. More importantly, we verified that the predictions involving saikosaponin-d by the cytotoxicity assay, apoptosis analysis, and RNA sequencing methods were partly consistent with those obtained from the network pharmacology prediction.
1. Introduction
Breast cancer is the most common type of female malignancy and the leading cause of cancer deaths worldwide.1 Breast cancer is expected to account for 24.2% of all new cancer diagnoses and 15.0% of all cancer-related female deaths.2,3 Despite the fact that mammography and emerging adjuvant therapies have already been formulated (e.g., adjuvant chemotherapy and hormonal therapy in the 1980s and targeted therapies in the 1990s), the recurrence rate of breast cancer has significantly decreased, and the mortality rate is still not controlled.4,5 The prognosis of newly diagnosed breast cancer patients was determined according to the classification of breast cancer. According to the different patterns of gene expressions, there are at least four major breast cancer subtypes: lumen A, lumen B, HER2 overexpression, and triple-negative subtypes.6 Currently, the best strategy to treat breast cancer is the use of surgery, radiotherapy, chemotherapy, and endocrine therapy.7 However, the use of chemotherapy for breast cancer is largely limited due to its terrible side-effects and drug resistance. Therefore, there is an urgent need for more effective and less toxic treatments for the treatment of breast cancer. Traditional Chinese medicine (TCM), as an important component of Complementary and Alternative Medicine (CAM), may play a key role in the treatment of breast cancer.8 Recently, TCM has been increasingly used by clinicians and researchers because of its proven efficacy and fewer side-effects. Bupleurum chinense DC.—a perennial herbaceous plant—belonging to Bupleurum L. (Umbelliferae family) is the favorite medicinal herb in TCM, and it has been used in China for more than 2000 years.9 Traditionally, B. chinense is employed as the main treatment toward fever, cold and heat, chest fullness, liver qi hysteresis, irregular menstruation, etc.10,11 Furthermore, more than half the Chinese formulas used for the treatment of breast cancer involve the use of B. chinense as the core prescription, such as Xiao Chai Hu Tang, BuzhongYiQi decoction, Xiao Yao San, et al., which are ancient Chinese medicine formulas dating back to the first century.9–12 In addition, an increasing number of preclinical cancer studies have demonstrated that B. chinense induces certain anti-breast-cancer effects.13 Therefore, B. chinense has valuable medicinal value and requires more in-depth research and utilization. The major component of B. chinense is triterpenoid saponins, which are generally considered to be the primary active ingredients effective against breast cancer.14 However, the potential mechanisms of B. chinense for the anti-breast-cancer effect have rarely been investigated.
TCM has “multi-component, multi-target, and multi-path effects,” which ensure synergistic efficacy by modulating the biological network of the body.7 Therefore, it is relatively difficult to detect the mechanism of TCM by routine experimentation. Consequently, there is an urgent need for new and appropriate methods to systematically and comprehensively analyze the herbal mechanisms. Due to the rapid development of bioinformatics, network pharmacology has become an emerging method to systematically understand the molecular and pharmacological mechanisms of TCM.15 Unlike the earlier reductionist “one drug, one target” mechanism, network pharmacology involves the fact that many active ingredients interact with a wide variety of genes or proteins, highlighting the overall idea of TCM.16 Network pharmacology is a discipline that combines systems biology, omics, and computational biology. It clarifies the mechanism of drug action from a holistic perspective, with completeness, synergy, and dynamic characteristics.15 By analyzing the intervention of drugs on the disease networks, the “drug-target-disease” network was constructed, which provided a new strategy for drug development.17 Network pharmacology provides new ideas and perspectives for the study of complex Chinese medicine systems. Therefore, we used network pharmacology to explore the impact of B. chinense on breast cancer to understand its medical value. In addition, cytotoxicity assays, apoptosis analyses, and RNA sequencing approaches were adopted for investigating the mechanism of action underlying the effectiveness of B. chinense against breast cancer. Overall, the present study could provide scientific evidence supporting the application of B. chinense for breast cancer treatment, as well as verify the application of modern techniques in exploring the value of TCM.
2. Materials and methods
2.1 Reagent and materials
Saikosaponin d was isolated at our laboratory, and the spectroscopic data are listed in the ESI (Fig. S1 and S2†). Human breast cancer cell lines SK-BR-3 and MCF-7, and human normal breast cell line HBL-100 were obtained from the Cell Bank of Shanghai Institute of Cell Biology, Chinese Academy of Sciences. Phosphate-buffered saline (PBS), Dulbecco's Modified Eagle's Medium (DMEM), and fetal bovine serum were purchased from HyClone (Logan, Utah, USA). The Cell Counting Kit-8 (CCK-8) assay was purchased from Sigma-Aldrich (Dojindo, Kumamoto, Japan). Annexin V-FITC apoptosis solution was obtained from Nanjing Jiancheng Bioengineering Institute (Nanjing, China).
2.2 Database construction
2.2.1 Compounds' database construction. The 62 triterpenoids of B. chinense were collated from the literature and Traditional Chinese Medicine Systems Pharmacology (TCMSP, http://lsp.nwu.edu.cn/tcmsp.php) database, which were used to establish a library of compounds. The TCMSP database—a unique pharmacology platform—captures the relationships between drugs, targets, and diseases involving Chinese herbal medicines (Table S1†).18 Moreover, some of these were already isolated from B. chinense by our group. The 3D chemical structures of triterpenoids were obtained from the PubChem database of the National Center for Biotechnological Information (NCBI) (https://pubchem.ncbi.nlm.nih.gov/), and the data were plotted using the ChemDraw 14.0 software. These compounds with structural information were stored in the mol2 format for further analysis.
2.2.2 Target protein database construction. The candidate proteins involved in breast cancer were datamined from the literature and the following public database sources: PubMed database (http://www.pubmed.org), which is a free literature search platform; PubChem database (http://www.pubchem.org), which provides information about chemical substances and their biological activities as a public repository; DrugBank database (http://www.drugbank.ca/), a unique bioinformatics and chemical informatics resource; Potential Drug Target Database (http://www.dddc.ac.cn/pdtd/), an accessible protein database for drug target recognition; and Therapeutic Target Database (http://bidd.nus.edu.sg/group/ttd/), a database that provides information about the explored therapeutic protein, nucleic acid targets, targeted diseases, pathway information, and corresponding drugs for each of these targets. According to the determination of the protein–ligand complex and crystal structures, 54 protein targets were obtained (Tables 1 and S2†). The crystal structures of the target protein complexes containing the original ligands were downloaded from the Protein Data Bank (PDB) database (it is a free database consisting of the structural data of biological macromolecules). These proteins were used as the receptors in molecular docking experiments.
Table 1 Relevant information regarding the target proteins mentioned in this article
Protein names |
Gene names |
Protein codes |
Resolution |
Insulin-like growth factor 1 receptor |
IGF1R |
3NW7 |
2.11 Å |
Heat shock protein HSP 90-alpha |
HSP90AA1 |
4YKZ |
1.85 Å |
Phosphatidylinositol 4,5-bisphosphate 3-kinase catalytic subunit alpha |
PIK3CA |
4JPS |
2.2 Å |
Proto-oncogene tyrosine-protein kinase Src |
SRC |
4MXO |
2.105 Å |
Probable ATP-dependent RNA helicase DDX5 |
DDX5 |
3FE2 |
2.6 Å |
Hepatocyte growth factor receptor |
c-Met |
5EYD |
1.85 Å |
Prostaglandin G/H synthase 2 |
COX2 |
5KIR |
2.697 Å |
Neutrophil gelatinase-associated lipocalin |
LCN2 |
3DSZ |
2 Å |
Protein-glutamine gamma-glutamyltransferase 2 |
TGM2 |
4PYG |
2.8 Å |
Protein kinase C alpha |
PRKCA |
4RA4 |
2.63 Å |
Serine/threonine-protein kinase mTOR |
mTOR |
4JT5 |
3.45 Å |
Estrogen receptor |
ESR1 |
5FQV |
1.74 Å |
Disintegrin and metalloproteinase domain-containing protein 17 |
ADAM17 |
3LEA |
2 Å |
Receptor tyrosine-protein kinase erbB-2 |
ERBB2 |
3PP0 |
2.25 Å |
Tumor protein P53 |
TP53 |
5G4O |
1.48 Å |
Epidermal growth factor receptor |
EGFR |
5JEB |
3.298 Å |
Cadherin 1 |
CDH1 |
4ZTE |
2.13 Å |
Catenin beta 1 |
CTNNB1 |
3TX7 |
2.76 Å |
Fibroblast growth factor receptor 2 |
FGFR2 |
5EG3 |
2.606 Å |
Progesterone receptor |
PGR |
1SR7 |
1.46 Å |
Prostaglandin-endoperoxide synthase 2 |
PTGS2 |
5IKT |
2.451 Å |
Erb-B2 receptor tyrosine kinase 3 |
ERBB3 |
6OP9 |
2.501 Å |
Apoptosis regulator Bcl-2 |
BCL2 |
4LXD |
1.9 Å |
Aromatase |
CYP19A1 |
3EQM |
2.9 Å |
Proto-oncogene c-Abl |
ABL1 |
4ZOG |
2.3 Å |
Estrogen receptor beta |
ESR2 |
4ZI1 |
2.1 Å |
Vascular endothelial growth factor receptor 2 |
KDR |
3EWH |
1.6 Å |
Cytochrome P450 11B1 |
CYP11B1 |
6M7X |
2.095 Å |
PI3K delta |
PIK3CD |
5T8F |
2.91 Å |
Carbonic anhydrase IV |
CA4 |
3F7B |
2.05 Å |
Carbonic anhydrase II |
CA2 |
4PZH |
1.06 Å |
Urokinase-type plasminogen activator |
PLAU |
1SQT |
1.9 Å |
DNA topoisomerase I |
TOP1MT |
1T8I |
3 Å |
Insulin receptor |
INSR |
5HHW |
1.79 Å |
FL cytokine receptor |
FLT3 |
4XUF |
3.2 Å |
Protein kinase C gamma type |
PRKCG |
2UZP |
2 Å |
Androgen receptor |
AR |
5V8Q |
1.44 Å |
Mast/stem cell growth factor receptor |
KIT |
3G0E |
1.6 Å |
Tyrosine-protein kinase JAK2 |
JAK2 |
5UT6 |
1.645 Å |
FL cytokine receptor |
FLT3 |
4RT7 |
3.1 Å |
Cyclin-dependent kinase 6 |
CDK6 |
5L2T |
2.37 Å |
Dihydrofolate reductase |
DHFR |
4QJC |
1.62 Å |
Urokinase plasminogen activator surface receptor |
PLAUR |
3U74 |
2.39 Å |
Ephrin type-A receptor 2 |
EPHA2 |
5IA5 |
1.776 Å |
Dual specificity mitogen-activated protein kinase kinase 1 |
MAP2K1 |
5EYM |
2.7 Å |
C-X-C chemokine receptor type 4 |
CXCR4 |
3OE9 |
3.1 Å |
Cathepsin D |
CTSD |
4OD9 |
1.9 Å |
Ubiquitin-protein ligase E3 Mdm2 |
MDM2 |
5LAW |
1.64 Å |
Stromal cell-derived factor 1 |
CXCL12 |
4UAI |
1.9 Å |
Steryl-sulfatase |
STS |
1P49 |
2.6 Å |
Cytochrome P450 2C19 |
CYP2C19 |
4GQS |
2.87 Å |
Thymidine phosphorylase |
TYMP |
1UOU |
2.11 Å |
Steroid hormone receptor ERR1 |
ESRRA |
3K6P |
1.996 Å |
RAC-alpha serine/threonine-protein kinase |
AKT1 |
4GV1 |
1.49 Å |
2.3 Bioinformatics analysis
2.3.1 Molecular docking. One of the key technologies in network pharmacology is the molecular docking technology. Molecular docking is an important means for the virtual screening of drugs, which starts from known proteins and small-molecule compounds, and they are further identified by simulating the geometry and energy matching.19 In this study, molecular semiflexible docking used the Surflex-Dock plugin in the Sybyl-X software (version 2.0, TRIPOS, Inc.).The target proteins were modified using the Sybyl-X software, including hydrogenation, water removal, optimization, and repairing the amino acid residues. The target proteins were constructed in the docking pockets to verify the ligands.
2.3.2 Network construction and feature analysis. The molecular docking scores showed the level of binding of the compounds to the target proteins. The scores of the original ligands were set as the threshold. A higher score (greater than the threshold of 5.0) was considered to be active. To further explore the multicomponent and multiscale mechanisms of the action of B. chinense in treating breast cancer, two types of networks were constructed by a network analysis software, i.e., Cytoscape 3.6.1 (http://cytoscape.org/), including the compound-target (C-T) and compound-target-pathway (C-T-P) networks. Cytoscape 3.6.1 is fairly suitable for visualizing and analyzing the networks of intermolecular interactions, biological pathways, etc., as well as providing powerful data integration, analysis, and visualization functions to analyze complicated networks.20 The nodes in the network represented compounds, targets, and pathways, and the edges represented interactions between the targets and compounds. The key parameters of the network, such as “degree” and “betweenness”, were calculated using the network analyzer plugin in Cytoscape 3.6.1. These parameters were calculated to determine the topological characteristics. The higher the performance evaluation of the “degree” and “betweenness,” the more important was the node to the network. The “degree” was defined as the number of links to the nodes; the “betweenness” was defined as the number of shortest paths between the pairs of nodes, which run through the nodes.20
2.3.3 Gene ontology (GO) and pathway enrichment analyses. GO and Kyoto Encyclopedia of Genes and Genomes (KEGG) pathway enrichment analyses were performed using the Database for Annotation, Visualization, and Integrated Discovery (DAVID, version 6.8; https://david.ncifcrf.gov/) to classify the proteins. The DAVID database provided systematic, comprehensive biofunctional annotation information for large-scale genes or proteins, enabling the identification of the most significant enriched biological annotations.17,18 By importing the potential target proteins of B. chinense into the DAVID database, setting the select identifier to “OFFICIAL GENE SYMBOL”, setting the list type to “Gene List”, and defining the species as “Homo Sapiens”, the GO and KEGG pathway analyses were performed on the targets of B. chinense, and the results were saved. The threshold was set at P < 0.05 according to the number of targets involved.
2.4 Experimental validation
2.4.1 Cytotoxicity assay. The cytotoxicity tests involving saikosaponin-d were performed by using the standard CCK-8 assay.21 The SK-BR-3 and MCF-7 cells were cultured in a DMEM medium (HyClone, Logan, USA), supplemented with 10% FBS (Biological Industries, Kibbutz Beit Haemek, Israel), 1% penicillin (HyClone, Logan, USA), and streptomycin (HyClone, Logan, USA). Further, the HBL-100 cells were cultured in RPMI-1640 (Gibco, Carlsbad, USA), 10% FBS, and 1% penicillin in a humidified atmosphere containing 5% CO2 at 37 °C. Logarithmically growing cells were used in all the experiments. Briefly, SK-BR-3, MCF-7, and HBL-100 cells were inoculated into a 96-well flat-bottom microplate at a cell density of 5 × 103 cells per well. After incubation for 24 h, media containing increasing concentrations of the tested compounds (3.125, 6.25, 12.5, 25, 50, and 100 μM) were added to the cells, and the samples were incubated for 48 h. Further, 5-fluorouracil (Aladdin, Shanghai, China; 3.125, 6.25, 12.5, 25, 50, and 100 μM) was used as the positive control. After 48 h of incubation, the CCK-8 assay was performed for each well for 3 h, and the absorbance was measured at 450 nm using a microplate reader (Thermo, Waltham, USA). All the tests were conducted in triplicate.
2.4.2 Apoptosis analysis. An apoptotic analysis was carried out using an Annexin V-FITC apoptosis staining solution according to the manufacturer's instruction (Nanjing Jiancheng Bioengineering Institute, Nanjing, China). SK-BR-3 cells were treated with saikosaponin-d (2.5, 5, and 10 μM) for 48 h. After incubation at a density of 4 × 105 cells per well in 6-well plates, the cells were washed with PBS and stained in Annexin V-FITC/PI solution for 15 min. Thereafter, the cell samples were immediately analyzed by a Can60IIflow cytometer (Becton Dickinson, Franklin Lakes, USA).
2.4.3 Western blot analysis. The SK-BR-3 cells were seeded into 6-well plates for 24 h, and they were treated with different concentrations of saikosaponin-d (2.5, 5, and 10 μM) for 48 h. The cells were collected and lysed in RIPA buffer (Beyotime, Shanghai, China) containing 50 mM Tris (pH 7.4), 150 mM NaCl, 1% Triton X-100, 1% sodium deoxycholate, 0.1% SDS, and 1 mM PMSF at 4 °C for 30 min. Then, the samples were boiled for 10 min at 100 °C. Further, the cell lysates were centrifuged at 16
000 g for 10 min at 4 °C, and the protein concentration was determined using a BCA protein assay kit. An equal amount of protein (30 μg) was exposed to 10% SDS-PAGE gel and transferred to PVDF membranes (0.2 μm, Millipore) using an electroblotting apparatus (Bio-Rad, Hercules, CA). The membranes were blocked with 5% nonfat milk for 2 h at room temperature and then probed with primary antibodies against Bcl-2 and β-actin (Cell Signaling Technology, Beverly, USA) overnight at 4 °C. Then, the membranes were washed three times with PBS-T and incubated with HRP-conjugated secondary antibodies (Santa Cruz Biotechnology Inc., Dallas, TX, USA) at room temperature for 2 h. The protein bands were visualized by an ECL Reagent (Beyotime, Shanghai, China). The ImageJ software was used to calculate the intensities of protein bands.
2.4.4 RNA sequencing. Based on the results of the network-pharmacology-based analysis and cells experiments, we selected saikosaponin-d for further RNA sequencing assays.22 SK-BR-3 cells were firstly treated with 15 μM saikosaponin-d for 48 h. Then, the total RNA from the samples treated and not treated with saikosaponin-d was isolated using TRIzol (Invitrogen, Carlsbad, CA, USA) according to the manufacturer's protocol. The extracted total RNA quality was verified by an Agilent 2100 bioanalyzer (Agilent Technologies, Palo Alto, CA, USA), and detected by using RNase-free agarose gel electrophoresis. After the total RNA was extracted, eukaryotic mRNA was enriched by Oligo(dT) beads. In addition, prokaryotic mRNA was gathered by removing the rRNA using Ribo-Zero™ magnetic kit (Epicentre, Madison, WI, USA). The enriched mRNA was further fragmented into shorter fragments by a fragmentation buffer, and reverse-transcripted into cDNA with random primers. The second-strand cDNA was synthesized by DNA polymerase I, RNase H, dNTP, and buffer.
3. Results and discussion
3.1 C-T network analysis
It is well known that B. chinense may exert its therapeutic effects on breast cancer because of its multi-component and multi-target characteristics. Therefore, a better understanding of the key active compounds as well as the mechanisms of B. chinense is essential. The C-T network is shown in Fig. 1, which included 90 nodes (55 active compound nodes and 35 composite target nodes) and 233 edges. The interactions between the compounds and target proteins were also evaluated by degree—an important topological parameter. In this network, the compound nodes in the inner circle showed more interaction than the outer nodes. Based on the C-T network, it was determined that saikosaponin-f (39), saikosaponin-d (14), saikosaponin-c (26), saikosaponin-h (54), saikosaponin-g (41), 3′′,6′′-O-diacetylsaikosaponin-d (20), 11α-methoxy-saikosaponin-f (40), and 6′′-O-acetylsaikosaponin-b1 (48) might exhibit critical pharmacological activities in treating breast cancer by means of B. chinense (Fig. 2). In addition, saikosaponin-d (14) was already isolated in our earlier study (Fig. S1 and S2†). The structures of the representative compounds are listed in the ESI (Table S1†). The C-T network revealed that several target proteins could hit multiple compounds. According to our earlier studies, it was understood that saikosaponin-d suppresses MCF-7 cell proliferation through the estrogenic effect by regulating the estrogen receptors; this was consistent with the above prediction.23 As shown in Table 2, 62 candidate ingredients in this herb yielded 35 potential targets from the C-T network. For instance, apoptosis regulator Bcl-2 (BCL-2; PDB ID: 4LXD), C-X-C chemokine receptor type 4 (CXCR4; PDB ID: 3OE9), probable ATP-dependent RNA helicase DDX5 (DDX5; PDB ID: 3FE2), protein kinase C alpha (PRKCA; PDB ID: 4RA4), and proto-oncogene tyrosine-protein kinase Src (SRC; PDB ID: 4RA4) were regulated by a variety of ingredients, such as saikosaponin-f, saikosaponin-d, saikosaponin-c, saikosaponin-h, saikosaponin-g, 3′′,6′′-O-diacetylsaikosaponin-d, and so on. These proteins might be the hub targets of this network. Interestingly, some of these compounds have been validated to exhibit potent anticancer biological activities. In particular, the saikosaponin compounds with an epoxy bridge exhibit cytotoxic effects against several types of cancer cells.24 For instance, saikosaponin-d decreases the expression of B cell lymphoma 2 (Bcl-2) family of proteins, as well as suppresses the expression of COX-2. It has been reported that these proteins are involved in carcinogenesis.25 It has been considered that the BCL-2 gene blocks apoptosis and promotes tumor development. It is localized to the luminal cells of normal breasts, which are considered to be the origin of malignant breast diseases.26 Both antiapoptotic cell survival proteins and proapoptotic cell death proteins were discovered within the Bcl-2 family; they are considered to be the regulators of apoptosis.25 However, more than 15 different Bcl-2 family members have been reported in humans. More extensively studied members of this family comprise Bcl-2, Bcl-xL, Bax, Bak, and Bad.27 In addition, in the hepatoma carcinoma cells of Hep G2 and Hep 3B cells, saikosaponin-d inhibited cell-survival signaling by enhancing the expression of IkBa and reducing the level of NF-κB, further attenuating the amount of Bcl-XL (Bcl-XL also belongs to the BCL-2 family).28 CXCR4 belongs to the superfamily of seven-transmembrane domain heterotrimeric G protein-coupled receptors (GPCR) and plays a pivotal role in the metastatic spread of breast cancer.29 In addition, DDX5 is a prototypical member of the DEAD box family, which is involved in a broad spectrum of biological processes, including transcription, translation, precursor messenger RNA processing or alternative splicing, and microRNA (miRNA) processing.30 DDX5 is overexpressed in breast cancer and involved in the regulation of various tumorigenic factors, such as c-Myc, VEGF, c-jun, AKT, fra-1, MMP2, and MMP9.31 Therefore, DDX5 could be considered as a useful target for cancer therapy because of its critical function in tumor development. SRC is a membrane-associated nonreceptor tyrosine kinase belonging to the SRC family kinase group, which plays a crucial role in the coordination and facilitation of cell-signaling pathways. In animal models of breasts, the overexpression of SRC has been associated with increased propensity for metastases.32 Therefore, we can approximate the relationship between biologically active compounds and target proteins, and we can determine the potential pharmacological effects of B. chinense from the C-T network.
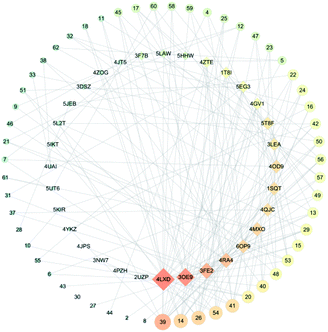 |
| Fig. 1 C-T network. Circular nodes represent compounds; the size denotes the number of interactions with the target proteins. Diamond-shaped nodes represent the target proteins. | |
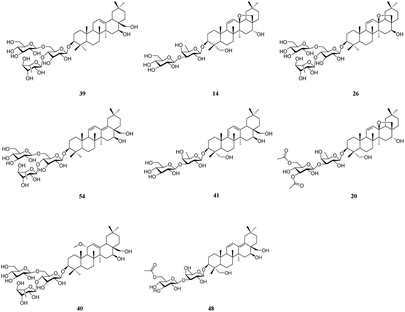 |
| Fig. 2 Top 8 potential active compounds of B. chinense. | |
Table 2 Features of the C-T network
Protein names |
Gene names |
Protein codes |
Degrees of target proteins |
Betweenness of target proteins |
Insulin-like growth factor 1 receptor |
IGF1R |
3NW7 |
1 |
0 |
Heat shock protein HSP 90-alpha |
HSP90AA1 |
4YKZ |
2 |
9.39 × 10−4 |
Phosphatidylinositol 4,5-bisphosphate 3-kinase catalytic subunit alpha |
PIK3CA |
4JPS |
1 |
0 |
Proto-oncogene tyrosine-protein kinase Src |
SRC |
4MXO |
10 |
0.08361207 |
Probable ATP-dependent RNA helicase DDX5 |
DDX5 |
3FE2 |
14 |
0.09386843 |
Prostaglandin G/H synthase 2 |
COX2 |
5KIR |
2 |
4.25 × 10−4 |
Neutrophil gelatinase-associated lipocalin |
LCN2 |
3DSZ |
3 |
0.00454735 |
Protein kinase C alpha |
PRKCA |
4RA4 |
12 |
0.07004885 |
Serine/threonine-protein kinase mTOR |
mTOR |
4JT5 |
3 |
0.00282128 |
Disintegrin and metalloproteinase domain-containing protein 17 |
ADAM17 |
3LEA |
8 |
0.01679009 |
Epidermal growth factor receptor |
EGFR |
5JEB |
3 |
0.00394928 |
Cadherin 1 |
CDH1 |
4ZTE |
5 |
0.02783261 |
Fibroblast growth factor receptor 2 |
FGFR2 |
5EG3 |
6 |
0.01547412 |
Prostaglandin-endoperoxide synthase 2 |
PTGS2 |
5IKT |
3 |
0.00170749 |
Erb-B2 receptor tyrosine kinase 3 |
ERBB3 |
6OP9 |
10 |
0.05911205 |
Apoptosis regulator Bcl-2 |
BCL2 |
4LXD |
26 |
0.2472 |
Proto-oncogene c-Abl |
ABL1 |
4ZOG |
3 |
0.00661283 |
PI3K delta |
PIK3CD |
5T8F |
8 |
0.02876791 |
Carbonic anhydrase IV |
CA4 |
3F7B |
4 |
0.00616844 |
Carbonic anhydrase II |
CA2 |
4PZH |
1 |
0 |
Urokinase-type plasminogen activator |
PLAU |
1SQT |
9 |
0.03517613 |
DNA topoisomerase I |
TOP1MT |
1T8I |
6 |
0.01743362 |
Insulin receptor |
INSR |
5HHW |
4 |
0.00569398 |
Protein kinase C gamma type |
PRKCG |
2UZP |
1 |
0 |
Tyrosine-protein kinase JAK2 |
JAK2 |
5UT6 |
2 |
0.00165432 |
Cyclin-dependent kinase 6 |
CDK6 |
5L2T |
3 |
0.00186753 |
Dihydrofolate reductase |
DHFR |
4QJC |
9 |
0.02963539 |
C-X-C chemokine receptor type 4 |
CXCR4 |
3OE9 |
18 |
0.11203831 |
Cathepsin D |
CTSD |
4OD9 |
9 |
0.03673176 |
Ubiquitin-protein ligase E3 Mdm2 |
MDM2 |
5LAW |
4 |
0.00974686 |
Stromal cell-derived factor 1 |
CXCL12 |
4UAI |
2 |
0.00127425 |
RAC-alpha serine/threonine-protein kinase |
AKT1 |
4GV1 |
6 |
0.01464801 |
3.2 GO and pathway enrichment analyses
To assess the functional characteristics of the potential targets and the signaling pathways at a systemic level, we performed functional enrichment analysis. All the enrichment pathways identified using this method represent biological regions that show statistically significant correlations with the B. chinense genome and require further investigation. The relationships among the potential targets were entered into the DAVID 6.8 database for GO enrichment analysis and associated KEGG pathways annotation, and they returned several breast-cancer-related biological processes. GO enrichment analysis covered three aspects of biological functions: cell component (CC), molecular function (MF), and biological process (BP). Fig. 3 shows a list of the top 10 significantly enriched GO terms (FDR < 0.01) in the abovementioned three aspects of biological functions of the potential targets. The GO enrichment analysis revealed that the targets of B. chinense were strongly correlated with the BP of signal transduction, protein autophosphorylation, phosphatidylinositol-mediated signaling, and so on. These targets are also involved in the CC, including the plasma membrane, cytoplasm, cytosol, and so on. The MFs include protein binding, ATP binding, protein tyrosine kinase activity, and so on. These results demonstrated that the use of B. chinense in treating breast cancer probably worked by engaging the abovementioned BPs, CCs, and MFs. The potential target proteins were inputted into KEGG (http://www.genome.jp/kegg/). The KEGG pathway annotation showed that 35 potential target genes were enriched and they involved 179 pathways (P ≤ 0.05). The top 10 pathways with the largest number of genes involved are shown in Fig. 3. The pathways in cancer (hsa05200) containing 16 genes is the highest; PI3K-Akt signaling pathway (hsa04151) with 13 genes is the second highest; EGFR tyrosine kinase inhibitor resistance (hsa01521) and miRNAs in cancer (hsa05206) yield 11 genes; proteoglycans in cancer (hsa05205) and Rap1 signaling pathway (hsa04015) included 10 genes. The details are shown in Fig. 3 and listed in Table S3.†
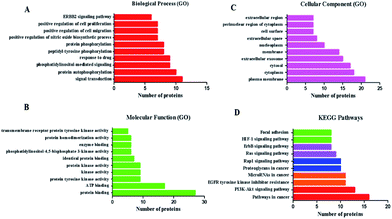 |
| Fig. 3 GO and KEGG pathways analyses by using the DAVID database. (A–C) GO analysis of the candidate targets. Data show the top 10 remarkably enriched items in the biological process (BP), cell component (CC), and molecular function (MF). (D) KEGG pathways of the target proteins. | |
3.3 C-T-P network analysis
Based on the above GO and pathway enrichment analyses, the C-T-P network was generated by connecting the compounds, corresponding targets, and potential pathways (Fig. 5). The C-T-P network was constructed to reflect a global view of the interactions between the targets and anti-breast-cancer-therapy-associated pathways. The C-T-P network is shown in Fig. 4, which includes 309 nodes (55 active compound nodes, 35 composite target nodes, and 179 pathway nodes) and 269 edges. Based on this network, the pathways in cancer (hsa05200), PI3K-Akt signaling pathway (hsa04151), EGFR tyrosine kinase inhibitor resistance (hsa01521), miRNAs in cancer (hsa05206), proteoglycans in cancer (hsa05205), and Rap1 signaling pathway (hsa04015) with several breast-cancer-associated targets might prove to be the key pathways in the development of breast cancer. As a part of the growth factor receptor (GFR) signaling, the PI3K-Akt pathway is a key mediator toward cell proliferation, survival, growth, and motility, which is affected by genetic aberrancies at different levels. The PI3K-Akt pathway is a crucial pathway for cancer development and represents a therapeutic target against breast cancer.33 More than 70% of breast tumors have molecular alterations in at least one component of the pathway.34 Numerous studies have proved that this pathway can be activated by losing PTEN, PIK3CA mutations, and mutations or other aberrations at the levels of PDK1, AKT1, AKT2, and p70S6 kinase.35 For triple-negative breast cancers, the upregulation of PI3K/AKT and the loss of PTEN is probably the most important mechanism of resistance.36 Based on earlier studies and our predictions received from the C-T-P network analysis, the PI3K-Akt signaling pathway could play an important role in the treatment of breast cancer. As predicted by the C-T-P network analysis, EGFR tyrosine kinase inhibitor resistance was also important in this network. EGFR, belonging to the human epidermal receptor (HER) family, is a transmembrane receptor tyrosine kinase (RTK), which is a crucial component of cell signal pathways.37 Tyrosine kinase inhibitors (TKIs) are applied to treat cancer-harboring EGFR mutations or aberrant activation of EGFR.38 EGFR mutations induce drug resistance, which are particularly mediated by the secondary mutation T790M and the synchronous activation of redundant kinases via bypass pathways. Some research has also reported that miRNAs would also be employed in treating resistance toward EGFR-TKIs in cancers.39 Recently, miRNAs in cancer have been widely investigated because of their potential role as targets for the treatment of cancer.40 Further, miRNAs are a major class of small noncoding RNA molecules (size: 17–25 nucleotides), which regulate gene expression by targeting mRNAs to post-transcriptionally modulate the gene expression.41 In addition, by binding to the 3′-untranslated region (3′-UTR) of the mRNA, miRNAs can also cause mRNA degradation or inhibit protein translation. Further, miRNAs have been known to regulate a majority of biological processes, including cell cycle, cell growth, apoptosis, cell differentiation, and stress response.42 Recent studies have established that miRNAs might be involved in cell proliferation, invasion, angiogenesis, and metastasis of breast cancer.43 Furthermore, miRNAs and their target genes are involved in the survival of breast cancer patients. Therefore, miRNA plays an important role in the occurrence and development of breast cancer, and it can be used as a potential biomarker for the early detection of breast cancer. Proteoglycans in cancer are also important in the C-T-P network. Proteoglycans are the key molecular effectors at the cell surface and pericellular microenvironments of breast cancer, which are markedly modified in the tumor microenvironment during tumor development and growth.44 Proteoglycans, a class of essential biological macromolecules, play a definite role in breast carcinogenesis; its expression levels are found to be different in malignant versus normal breast tissues.45 As powerful mediators, proteoglycans in cancer control a variety of processes, and they might reveal novel therapeutic modalities against cancer.44 Rap1 is a member of the Ras monomer G protein family and is recognized for its role in tumorigenesis and cancer progression.46 The Rap1 signaling pathway involves cell adhesion, migration, and survival, which may promote several processes associated with tumorigenesis and metastasis.47 In osteosarcoma cells, the inactivation of DOCK4 (Rap1 activator) makes cells more aggressive. In addition, the Rap1GAP expression is associated with the increased in vitro invasion of squamous cell carcinoma.48 On the other hand, Rap1GAP overexpression leads to a reduction in pancreatic cancer incidence and local invasion from the primary tumor site.47 Therefore, it is evident that the Rap1 signaling pathway can positively or negatively regulate the invasion and metastasis of breast cancer. After the establishment of these networks, we made a preliminary speculation on the material basis of saikosaponin compounds against breast cancer.
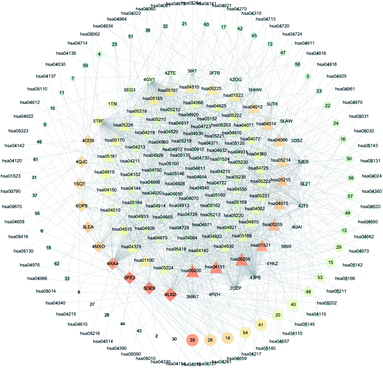 |
| Fig. 4 C-T-P network. Circular nodes represent the compounds that have effects on the intersection between the compounds and target proteins. Diamond-shaped nodes correspond to the target proteins. Triangular nodes correspond to pathways. Bigger and darker nodes represent closer association. Lines represent the relationships between the compounds, targets, and pathways. | |
3.4 In vitro validation
The network pharmacology concept could improve our understanding of herbal medicines. The network pharmacology analysis was applied to facilitate the exploration of active compounds in B. chinense, prediction of protein targets, and correlation between the signaling pathways. In order to verify the prediction results of network pharmacology, in vitro experiments involving the isolated compounds were performed. In addition, saikosaponin compounds isolated from the Bupleurum genus have demonstrated strong cytotoxic activities in various types of cancer cell lines, which inspired us to study the mechanisms of these compounds.12,13 Saikosaponin-d was one of the major constituents of B. chinense, and it has already been isolated by our group.13 Therefore, in order to verify the prediction results of the C-T and C-T-P network pharmacologies, we used saikosaponin-d for further in vitro validation. The cytotoxicity characteristics of saikosaponin-d and 5-fluorouracil against SK-BR-3 and MCF-7 cells were examined using the CCK-8 assay.21 As shown in Fig. 5A, saikosaponin-d showed cytotoxic activities toward SK-BR-3 cells with an IC50 value of 13.26 μM, which was comparable to that of 5-fluorouracil (17.25 μM). In addition, saikosaponin-d showed cytotoxic activities toward MCF-7 cells with an IC50 value of 15.84 μM. We used the normal breast HBL-100 cells to determine the cytotoxicity of saikosaponin-d. The results revealed that saikosaponin-d showed no cytotoxicity activity toward HBL-100 cells (IC50 > 80). Apoptosis is a typical form of programmed cell death, which plays an important role in the regulation of cancer development and progression.49 Annexin V-FITC/PI staining assay was used to evaluate the apoptotic effects of saikosaponin-d at concentrations of 2.5, 5, and 10 μM for 48 h. The percentage of apoptotic cells was 29.8% at 10 μM (Fig. 5B and C), which induced an accumulation of apoptotic cells as compared to that of 9.40% in the untreated control cells. To further study the mechanism of saikosaponin-d-induced apoptosis, the level of antiapoptotic protein Bcl-2 was evaluated by western blot analysis in SK-BR-3 cells. The results revealed that saikosaponin-d decreased the expression of Bcl-2 (Fig. 5D and E). These results suggested that saikosaponin-d could induce apoptotic cell death in SK-BR-3 cells. Taken together, these results proved that saikosaponin-d had a significant impact on the induction of apoptosis in SK-BR-3 cells. The RNA sequencing (RNA-seq) approach could be used to determine the genome-wide expression changes, reveal the full repertoire of alternative splice variants, and illustrate new transcripts.50 By using the RNA-seq approach, the effects of saikosaponin-d on SK-BR-3 cell line transcriptomes were evaluated. Subsequently, 198 upregulated genes and 41 downregulated expressed genes were found in SK-BR-3 cells after saikosaponin-d treatment (Fig. 6A and B). The KEGG analysis of the RNA sequencing results illustrated that multiple cancer-related pathways were significantly involved in the action mechanisms of breast cancer, including focal adhesion, fluid shear stress, and atherosclerosis, TNF signaling pathway, proteoglycans in cancer, leukocyte transendothelial migration, miRNAs in cancer, and rheumatoid arthritis (Fig. 6C). Here, the Y-axis denotes the enriched KEGG pathways of the target genes and the X-axis denotes the enrichment factor. The size of the dot indicates the number of target genes in the pathway, and the color of the dot exhibits the different ranges of Q values. Based on the KEGG analysis, some pathways such as focal adhesion, proteoglycans in cancer, and miRNAs in cancer were also predicated by the network pharmacology analysis (Fig. 6C). Therefore, the RNA-seq results could further verify the reliability of the results obtained from the network pharmacology prediction. In conclusion, the above cytotoxicity assay, Annexin V-FITC/PI staining analysis, and RNA-seq approach confirmed the activities of B. chinense and saikosaponin-d toward breast cancer, which were partly consistent with the results obtained from the network pharmacology prediction.
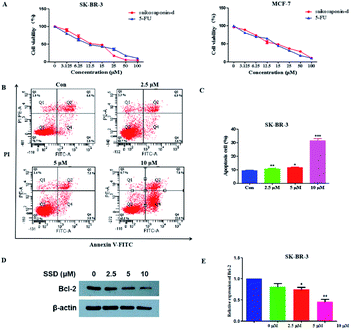 |
| Fig. 5 Cytotoxic activities and apoptosis induced by saikosaponin-d in SK-BR-3 and MCF-7 cells. (A) SK-BR-3 and MCF-7 cells were treated with various doses of saikosaponin-d and 5-Fu for 48 h, and the cell viabilities were determined by CCK-8 assays. (B) Flow cytometry analysis of Annexin V-FITC/PI staining in SK-BR-3 cells for 48 h. (C) Quantitative analyses of the apoptotic ratio in SK-BR-3 cells. (D) Apoptosis-associated Bcl-2 protein was detected by the western blot assay. (E) Relative levels of Bcl-2 were quantified by the ImageJ software. *p < 0.05, **p < 0.01, ***p < 0.001 as compared to the control. | |
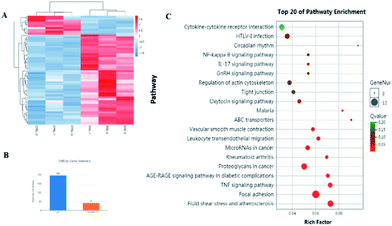 |
| Fig. 6 Results of the RNA sequencing analysis of saikosaponin-d in SK-BR-3 cells. (A) Differential heatmap of the genes cluster in SK-BR-3 cells after saikosaponin-d treatment. (B) Histogram of differential genes statistics (control vs. saikosaponin-d). (C) Pathway enrichment analysis of RNA sequencing in SK-BR-3 cells after saikosaponin-d treatment. | |
4. Conclusions
In conclusion, we firstly established the “multi-component – multi-target – multi-pathway” network by combining molecular docking, GO enrichment analysis, and pathway analysis to determine the potential targets and related pathways of B. chinense against breast cancer. The obtained results showed that saikosaponin-f (39), saikosaponin-d (14), saikosaponin-c (26), saikosaponin-h (54), saikosaponin-g (41), 3′′,6′′-O-diacetylsaikosaponin-d (20), 11α-methoxy-saikosaponin-f (40), and 6′′-O-acetylsaikosaponin-b1 (48) exhibited promising potential toward the treatment of breast cancer. These saikosaponin compounds were also predicted to interact with the apoptosis regulator Bcl-2, C-X-C chemokine receptor type 4, probable ATP-dependent RNA helicase DDX5, protein kinase C alpha, and proto-oncogene tyrosine-protein kinase Src related to breast cancer, which yielded a worthwhile explanation toward the synergistic mechanisms of TCM. In addition, six major signaling pathways were predicted to mediate the effects of B. chinense against breast cancer. Furthermore, experimental verification, including cytotoxicity assay, apoptosis analysis, and RNA sequencing, confirmed the effect of saikosaponin-d against breast cancer in addition to validating the network pharmacology prediction. Therefore, the combined methods of network pharmacology prediction and experimental verification may provide a reference as well as basis for studying the molecular mechanism of B. chinense. However, additional experiments are needed to prove our findings in understanding the further underlying pharmacological and molecular paradigms. Our research would be useful for facilitating studies of other Chinese herbs or formulas against cancers by using the network pharmacology method.
Conflicts of interest
The authors declare that they have no conflict of interest.
Acknowledgements
This research work was financially supported by the National Natural Science Foundation of China (No. 81703378), Foundation (No. 20170520250) from the Project of Science and Technology Department of Liaoning Province of China, and Foundation (No. LQ2017016) from the Project of Education Department of Liaoning Province of China. Authors sincerely acknowledge Prof. Jian Wang of Shenyang Pharmaceutical University for docking studies and TRIPOS, Inc.
Notes and references
- L. A. Torre, R. L. Siegel, E. M. Ward and A. Jemal, Global Cancer Incidence and Mortality Rates and Trends-An Update, Cancer Epidemiol., Biomarkers Prev., 2016, 25, 16–27 CrossRef PubMed.
- R. L. Siegel, K. D. Miller and A. Jemal, Cancer statistics, 2018, Ca-Cancer J. Clin., 2018, 68, 7–30 CrossRef PubMed.
- F. Bray, J. Ferlay, I. Soerjomataram, R. L. Siegel, L. A. Torre and A. Jemal, Global cancer statistics 2018: GLOBOCAN estimates of incidence and mortality worldwide for 36 cancers in 185 countries, Ca-Cancer J. Clin., 2018, 68, 394–424 CrossRef PubMed.
- D. Munoz, A. M. Near, N. T. van Ravesteyn, S. J. Lee, C. B. Schechter, O. Alagoz, D. A. Berry, E. S. Burnside, Y. Chang, G. Chisholm, H. J. de Koning, M. Ali Ergun, E. A. Heijnsdijk, H. Huang, N. K. Stout, B. L. Sprague, A. Trentham-Dietz, J. S. Mandelblatt and S. K. Plevritis, Effects of screening and systemic adjuvant therapy on ER-specific US breast cancer mortality, J. Natl. Cancer Inst., 2014, 106, 289 CrossRef PubMed.
- C. DeSantis, J. Ma, L. Bryan and A. Jemal, Breast cancer statistics, 2013, Ca-Cancer J. Clin., 2014, 64, 52–62 CrossRef PubMed.
- C. Sotiriou, S. Y. Neo, L. M. McShane, E. L. Korn, P. M. Long, A. Jazaeri, P. Martiat, S. B. Fox, A. L. Harris and E. T. Liu, Breast cancer classification and prognosis based on gene expression profiles from a population-based study, Proc. Natl. Acad. Sci. U. S. A., 2003, 100, 10393–10398 CrossRef CAS PubMed.
- L. S. You, R. An, K. Liang and X. Wang, Anti-Breast Cancer Agents from Chinese Herbal Medicines, Mini-Rev. Med. Chem., 2013, 13, 101–105 CrossRef CAS PubMed.
- L. Zeng and K. Yang, Exploring the pharmacological mechanism of Yanghe Decoction on HER2-positive breast cancer by a network pharmacology approach, J. Ethnopharmacol., 2017, 199, 68–85 CrossRef CAS PubMed.
- X. F. Song, T. Ren, Z. Zheng, T. Lu, Z. Wang, F. Du and H. Tong, Anti-tumor and immunomodulatory activities induced by an alkali-extracted polysaccharide BCAP-1 from Bupleurum chinense via NF-κB signaling pathway, Int. J. Biol. Macromol., 2017, 95, 357–362 CrossRef CAS PubMed.
- X. Q. Li, Y. N. Song, S. J. Wang, K. Rahman, J. Y. Zhu and H. Zhang, Saikosaponins: a review of pharmacological effects, J. Asian Nat. Prod. Res., 2018, 20, 399–411 CrossRef CAS PubMed.
- R. Y. Yao, Y. F. Zou and X. F. Chen, Traditional Use, Pharmacology, Toxicology, and Quality Control of Species in Genus Bupleurum L., Chin. Herb. Med., 2013, 5, 245–255 CrossRef.
- B. C. Yuan, R. Yang, Y. S. Ma, S. Zhou, X. Zhang and Y. Liu, A systematic review of the active saikosaponins and extracts isolated from Radix Bupleuri and their applications, Pharm. Biol., 2017, 55, 620–635 CrossRef CAS PubMed.
- M. L. Ashour and M. Wink, Genus Bupleurum: a review of its phytochemistry, pharmacology and modes of action, J. Pharm. Pharmacol., 2011, 63, 305–321 CrossRef CAS PubMed.
- J. C. Chen, N. W. Chang, J. G. Chung and K. C. Chen, Saikosaponin-A Induces Apoptotic Mechanism in Human Breast MDA-MB-231 and MCF-7 Cancer Cells, Am. J. Chin. Med., 2003, 31, 363–377 CrossRef CAS PubMed.
- A. L. Hopkins, Network pharmacology: the next paradigm in drug discovery, Nat. Chem. Biol., 2008, 4, 682–690 CrossRef CAS PubMed.
- J. S. Fang, L. Wang, T. Wu, C. Yang, L. Gao, H. Cai, J. Liu, S. Fang, Y. Chen, W. Tan and Q. Wang, Network pharmacology-based study on the mechanism of action for herbal medicines in Alzheimer treatment, J. Ethnopharmacol., 2017, 196, 281–292 CrossRef CAS PubMed.
- J. T. Xue, Y. L. Shi, C. Y. Li and H. J. Song, Network pharmacology-based prediction of the active ingredients, potential targets, and signaling pathways in compound Lian-Ge granules for treatment of diabetes, J. Cell. Biochem., 2019, 120, 6431–6440 CrossRef CAS PubMed.
- B. C. Zhu, W. T. Zhang, Y. Lu, S. N. Hu, R. Gao, Z. X. Sun, X. N. Chen, J. M. Ma, S. Guo, S. Y. Du and P. Y. Li, Network pharmacology-based identification of protective mechanism of Panax Notoginseng Saponins on aspirin induced gastrointestinal injury, Biomed. Pharmacother., 2018, 105, 159–166 CrossRef CAS PubMed.
- X. G. Liu, M. C. Lv, M. Y. Huang, Y. Q. Sun, P. Y. Gao and D. Q. Li, A network pharmacology study on the triterpene saponins from Medicago sativa L. for the treatment of Neurodegenerative diseases, J. Food Biochem., 2019, 43, e12955 Search PubMed.
- X. K. Liu, J. R. Wu, D. Zhang, K. Wang, X. Duan and X. Zhang, A Network Pharmacology Approach to Uncover the Multiple Mechanisms of Hedyotis diffusa Willd. on Colorectal Cancer, Evid.-Based Complementary Altern. Med., 2018, 2018, 6517034 Search PubMed.
- N. L. Zhu, C. P. Tang, C. H. Xu, C. Q. Ke, G. Lin, J. Jenis, S. Yao, H. C. Liu and Y. Ye, Cytotoxic germacrane-type sesquiterpene lactones from the whole plant of Inula cappa, J. Nat. Prod., 2019, 8, 919–927 CrossRef PubMed.
- B. W. Yang, N. Wang, S. Q. Wang, X. Li, Y. F. Zheng, M. Li, J. X. Song, F. X. Zhang, W. J. Mei, Y. Lin and Z. Y. Wang, Network-pharmacology-based identifification of caveolin-1 as a key target of Oldenlandia diffusa to suppress breast cancer metastasis, Biomed. Pharmacother., 2019, 112, 108607 CrossRef CAS PubMed.
- Q. Wang, X. L. Zheng, L. Yang, F. Shi, L. B. Gao, Y. J. Zhong, H. Sun, F. He, Y. Lin and X. Wang, Reactive oxygen species-mediated apoptosis contributes to chemosensitization effect of saikosaponins on cisplatin-induced cytotoxicity in cancer cells, J. Exp. Clin. Cancer Res., 2010, 29, 159 CrossRef CAS PubMed.
- B. Yuan, R. Yang, Y. Ma, S. Zhou, X. Zhang and Y. Liu, A systematic review of the active saikosaponins and extracts isolated from Radix Bupleuri and their applications, Pharm. Biol., 2016, 55, 620–635 CrossRef PubMed.
- R. Y. Liu and J. P. Li, Saikosaponin-d inhibits proliferation of human undifferentiated thyroid carcinoma cells through induction of apoptosis and cell cycle arrest, Eur. Rev. Med. Pharmacol. Sci., 2014, 18, 2435–2443 Search PubMed.
- B. Nathan, B. Gusterson, D. Jadayel, M. O'Hare, R. Anbazhagan, H. Jayatilake, S. Ebbs, K. Micklem, K. Price, R. Gelber, R. Reed, H. J. Senn, A. Goldhirsch and M. J. S. Dyer, Expression of BCL-2 in primary breast cancer and its correlation with tumour phenotype, Ann. Oncol., 1994, 5, 409–414 CrossRef CAS PubMed.
- K. Schorr, M. Li, S. Krajewski, J. C. Reed and P. A. Furth, Bcl-2 Gene Family and Related Proteins in Mammary Gland Involution and Breast Cancer, J. Mammary Gland Biol. Neoplasia, 1999, 4, 153–164 CrossRef CAS PubMed.
- X. Q. Li, Y. N. Song, S. J. Wang, K. Rahman, J. Y. Zhu and H. Zhang, Saikosaponins: a review of pharmacological effects, J. Asian Nat. Prod. Res., 2018, 20, 399–411 CrossRef CAS PubMed.
- D. Mukherjee and J. Zhao, The Role of chemokine receptor CXCR4 in breast cancer metastasis, Am. J. Cancer Res., 2013, 3, 46–57 CAS.
- D. Wang, J. Huang and Z. Hu, RNA Helicase DDX5 Regulates MicroRNA Expression and Contributes to Cytoskeletal Reorganization in Basal Breast Cancer Cells, Mol. Cell. Proteomics, 2012, 11, M111.011932 CrossRef PubMed.
- V. Hashemi, A. Masjedi, B. Hazhir-karzar, A. Tanomand, S. S. Shotorbani, M. Hojjat-Farsangi, G. Ghalamfarsa, G. Azizi, E. Anvari, B. Baradaran and F. Jadidi-Niaragh, The role of DEAD-box RNA helicase p68 (DDX5) in the development and treatment of breast cancer, J. Cell. Physiol., 2019, 234, 5478–5487 CrossRef CAS PubMed.
- E. L. Mayer and I. E. Krop, Advances in targeting SRC in the treatment of breast cancer and other solid malignancies, Clin. Cancer Res., 2010, 16, 3526–3532 CrossRef CAS PubMed.
- L. C. Cantley, The phosphoinositide 3-kinase pathway, Science, 2002, 296, 1655–1657 CrossRef CAS PubMed.
- L. F. Hernandez-Ayaa and A. M. Gonzalez-Angulo, Targeting the Phosphatidylinositol 3-Kinase Signaling Pathway in Breast Cancer, Oncologist, 2011, 16, 404–414 CrossRef PubMed.
- E. Lopez-Knowles, S. A. O'Toole, C. M. McNeil, E. K. A. Millar, M. R. Qiu, P. Crea, R. J. Daly, E. A. Musgrove and R. L. Sutherland, PI3K pathway activation in breast cancer is associated with the basal-like phenotype and cancer-specific mortality, Int. J. Cancer, 2010, 126, 1121–1131 CrossRef CAS PubMed.
- E. Tokunaga, Y. Kimura, K. Mashino, E. Oki, A. Kataoka, S. Ohno, M. Morita, Y. Kakeji, H. Baba and Y. Maehara, Activation of PI3K/Akt signaling and hormone resistance in breast cancer, Breast Cancer, 2006, 13, 137–144 CrossRef PubMed.
- F. Ciardiello and G. Tortora, EGFR antagonists in cancer treatment, N. Engl. J. Med., 2008, 358, 1160–1174 CrossRef CAS PubMed.
- G. Bethune, D. Bethune, N. Ridgway and Z. Xu, Epidermal growth factor receptor (EGFR) in lung cancer: an overview and update, J. Thorac. Dis., 2010, 2, 48–51 CAS.
- L. H. Huang and L. W. Fu, Mechanisms of resistance to EGFR tyrosine kinase inhibitors, Acta Pharm. Sin. B, 2015, 5, 390–401 CrossRef PubMed.
- R. Garzon, G. A. Calin and C. M. Croce, MicroRNAs in Cancer, Annu. Rev. Med., 2009, 60, 167–179 CrossRef CAS PubMed.
- V. Ambros, B. Bartel, D. P. Bartel, C. B. Burge, J. C. Carrington, X. Chen, G. Dreyfuss, S. R. Eddy, S. Griffiths-Jones, M. Marshall, M. Matzke, G. Ruvkun and T. Tuschl, A uniform system for microRNA annotation, RNA, 2003, 9, 277–279 CrossRef CAS PubMed.
- Y. Y. Chang, W. H. Kuo, J. H. Hung, C. Y. Lee, Y. H. Lee, Y. C. Chang, W. C. Lin, C. Y. Shen, C. S. Huang, F. J. Hsieh, L. C. Lai, M. H. Tsai, K. J. Chang and E. Y. Chuang, Deregulated microRNAs in triple-negative breast cancer revealed by deep sequencing, Mol. Cancer, 2015, 14, 36 CrossRef PubMed.
- C. Corcoran, A. M. Friel, M. J. Duffy, J. Crown and L. O'Driscoll, Intracellular and Extracellular MicroRNAs in Breast Cancer, Clin. Chem., 2011, 57, 18–32 CrossRef CAS PubMed.
- A. D. Theocharis, S. S. Skandalis, T. Neill, H. A. Multhaupt, M. Hubo, H. Frey, S. Gopal, A. Gomes, N. Afratis, H. C. Lim, J. R. Couchman, J. Filmus, R. D. Sanderson, L. Schaefer, R. V. Iozzo and N. K. Karamanos, Insights into the key roles of proteoglycans in breast cancer biology and translational medicine, Biochim. Biophys.
Acta, 2015, 1855, 276–300 CAS.
- M. S. Shawky, C. Ricciardelli, M. Lord, J. Whitelock, V. Ferro, K. Britt and E. W. Thompson, Proteoglycans: Potential Agents in Mammographic Density and the Associated Breast CancerRisk, J. Mammary Gland Biol. Neoplasia, 2015, 20, 121–131 CrossRef PubMed.
- M. Hattori and N. Minato, Rap1 GTPase: functions, regulation, and malignancy, J. Biochem., 2003, 134, 479–484 CrossRef CAS PubMed.
- L. Zhang, L. Chenwei, R. Mahmood, K. van Golen, J. Greenson, G. Li, N. J. D'Silva, X. Li, C. F. Burant, C. D. Logsdon and D. M. Simeone, Identification of a putative tumor suppressor gene Rap1GAP in pancreatic cancer, Cancer Res., 2006, 66, 898–906 CrossRef CAS PubMed.
- R. S. Mitra, M. Goto, J. S. Lee, D. Maldonado, J. M. Taylor, Q. Pan, T. E. Carey, C. R. Bradford, M. E. Prince, K. G. Cordell, K. L. Kirkwood and N. J. D'Silva, Rap1GAP promotes invasion via induction of matrix metalloproteinase 9 secretion, which is associated with poor survival in low N-stage squamous cell carcinoma, Cancer Res., 2008, 68, 3959–3969 CrossRef CAS PubMed.
- Y. H. Fan, H. W. Ding, D. D. Liu, H. R. Song, Y. N. Xu and J. Wang, Novel 4-amino quinazoline derivatives induce growth inhibition, cell cycle arrest and apoptosis via PI3Kα inhibition, Bioorg. Med. Chem., 2018, 26, 1675–1685 CrossRef CAS PubMed.
- X. Zhou, C. Y. Cao, A. T. Wan, G. G. Yue, F. H. Kwok, K. P. Sun, H. Fung, C. B. Lau, P. T. Puno and S. K. Tsui, Functional roles of eriocalyxin B in zebrafish revealed by transcriptome analysis, Mol. Omics, 2018, 14, 156–169 RSC.
Footnote |
† Electronic supplementary information (ESI) available. See DOI: 10.1039/c9ra08970e |
|
This journal is © The Royal Society of Chemistry 2019 |
Click here to see how this site uses Cookies. View our privacy policy here.