DOI:
10.1039/C9FO01780A
(Paper)
Food Funct., 2020,
11, 378-391
Nicotinamide riboside alleviates alcohol-induced depression-like behaviours in C57BL/6J mice by altering the intestinal microbiota associated with microglial activation and BDNF expression†
Received
5th August 2019
, Accepted 28th November 2019
First published on 3rd December 2019
Abstract
The gut microbiota play an important role in many central nervous system diseases through the gut microbiota–brain axis. Recent studies suggest that nicotinamide riboside (NR) has neuroprotective properties. However, it is unknown whether NR can prevent or protect against alcohol-induced depression. Furthermore, it is unclear whether its therapeutic action involves changes in the composition of the gut microbiome. Here, we investigated the effects of NR in the mouse model of alcohol-induced depression. Treatment with NR improved the alcohol-induced depressive behaviour in mice. In addition, NR decreased the number of activated microglia in the hippocampus, and it reduced the levels of pro-inflammatory (IL-1β, IL-6, and TNF-α) and anti-inflammatory (IL-10 and TGF-β) cytokines in the brain of mice with alcohol-induced depression. Furthermore, NR significantly upregulated BDNF and diminished the inhibition of the AKT/GSK3β/β-catenin signalling pathway in the hippocampus of these mice. 16S rRNA sequencing revealed that, compared with control and NR-treated mice, the gut microbiome richness and composition were significantly altered in the depressed mice. Spearman's correlation analysis showed that differential gut bacterial genera correlated with the levels of inflammation-related cytokines and BDNF in the brain. After faecal microbiota transplantation, cognitive behaviours, microglial activity, levels of cytokines and BDNF, and activation state of the AKT/GSK3β/β-catenin signalling pathway (which is downstream of the BDNF receptor, TrkB) in recipient mice were similar to those in donor mice. Collectively, our findings show that NR dietary supplementation protects against alcohol-induced depression-like behaviours, possibly by altering the composition of the gut microbiota.
Introduction
It is estimated that worldwide in 2015, more than 300 million people had depressive disorders, and the number of people with depression is increasing every year.1 Depression has been ranked as the single largest contributor to global disability and non-fatal morbidity. Furthermore, depression-related suicide accounts for close to 800
000 deaths every year.1 Gene–environment interactions play a key pathogenetic role in depression, and there are numerous risk factors for the disease.2 Alcohol is a toxic and dependence-producing psychoactive substance.3 Several studies in rodents and humans demonstrate that alcohol abuse is a major risk factor for the development of mental health conditions such as anxiety and depressive disorders.4,5
Recent studies suggest that the gut microbiota–brain axis plays a critical role in central nervous system diseases.6 It is a system of bidirectional communication between the brain and the gut microbiota, with interactions mediated, at least in part, by the nervous, metabolic, hormonal, and immune systems.7 Gut microorganisms are closely associated with microglial activation and inflammatory factor release, the expression of brain-derived neurotrophic factor (BDNF), and the activation of its downstream AKT/GSK3β/β-catenin pathway, which are involved in the pathophysiology of many central nervous system diseases including depression.8–10 The gut microbiota–brain axis has recently been shown to play an important role in brain dysfunction and the pathogenesis of depressive disorders.11–13 Several studies in rodents and humans show that alcohol consumption results in quantitative and qualitative dysbiosis in the intestine.14 Thus, the modulation of intestinal microecology has emerged as a potential therapeutic strategy for alcohol-induced depression.
Functional foods and nutrients can improve brain functions post-injury.15,16 Nicotinamide riboside (NR), a form of vitamin B3, is a nicotinamide adenine dinucleotide (NAD+) precursor that is mainly obtained from milk and yeast.17,18 Recent studies show that NR has neuroprotective properties, and that dietary intake of the compound alleviates Alzheimer's disease-associated cognitive decline and deficits in synaptic plasticity.19 NR can also protect against excitotoxicity-induced axonal degeneration and sensory neuropathy in mice.20,21 However, the effects of NR on alcohol-induced depression remain unclear.
In this study, we investigate the effect of NR on alcohol-induced depression-like behaviour in mice, and we assess the role of the intestinal microbiota in the therapeutic action of the compound. Moreover, we examine the relationship between intestinal microbiota composition and changes in biological indicators of alcohol-induced depression, including microglial activation and inflammatory cytokine release, BDNF levels, and activity of the downstream AKT/GSK3β/β-catenin signalling pathway.
Materials and methods
Animals
Seven-week-old male C57BL/6J mice, weighing 20–22 g, were obtained from Beijing Vital River Laboratory Animal Technology Co., Ltd (License No.: SCXK [Jing], 2016-0006). The mice were housed in a clean air-conditioned room, at a temperature of 21–23 °C and ∼50–60% relative humidity, in the specific-pathogen-free animal centre of Qingdao University. During the study, animals were fed with standard rodent diet and maintained under standard lighting conditions (lights on from 8 a.m. to 4 p.m.).
The protocol for the study was approved by the Animal Care and Use Committee of the Medical College, Qingdao University, and followed the institution's guidelines for the care and use of laboratory animals.
Production of the mouse model of alcohol-induced depression and intervention strategy
Twenty-one mice were housed in the same cage (535 × 390 × 200 mm [L × W × H]) and adaptively fed for 3 weeks to make the intestinal microfloral composition of the mice more uniform.22,23 Thereafter, the mice were ear-marked, weighed, and randomly divided into the following three groups (seven mice per group): Control, Model, and NR groups. Each mouse was housed individually in a cage (320 × 210 × 160 mm [L × W × H]).
The mouse model of alcohol-induced depression was produced according to a previous report, with slight modification.24 Based on previous reports and our preliminary experiments, we used a duration of alcohol exposure of 10 weeks.24,25 Briefly, from Monday to Thursday, the mice in the Model and NR groups were provided with fresh tap water from 8
:
00 a.m. to 4
:
00 p.m., followed by 15% alcohol solution (v/v) until 8
:
00 a.m. the next day. The mice in the Control group drank tap water during the 4 days. On Friday and Saturday, the mice did not receive any liquid. On Sunday, the mice were given fresh tap water. During the experiment, all the mice were allowed ad libitum access to food. The 15% alcohol solution (v/v) was prepared immediately prior to use (ESI Fig. 1A†). Mice in the Control and Model groups received normal saline (0.2 mL d−1) via an intragastric tube at 12:00 p.m. each day. The NR group received 400 mg kg−1 of NR (Hubei Jusheng Technology Co., Ltd, Hubei, China) by gavage daily.
Faecal microbiota transplantation (FMT)
After 8 weeks of alcohol exposure, fresh faeces were collected daily from the Control, Model and NR groups for a period of 2 weeks. Eighteen C57BL/6J recipient mice were randomly divided into 3 groups (6 mice per group) for FMT as follows: FMT Control (FMT C), FMT Model (FMT M), and FMT NR groups. The mice were provided drinking water containing broad-spectrum antibiotics (ampicillin, 1 g L−1; neomycin sulfate, 1 g L−1; metronidazole, 1 g L−1; vancomycin, 500 mg L−1; Sigma-Aldrich, Shanghai, China) for 3 weeks before receiving FMT.26,27 FMT was conducted according to a previous study with minor modifications. Briefly, each day, after the donor mice received intragastric of normal saline or NR, fresh faecal pellets were collected and placed in the icebox in an anaerobic bag. All the samples were prepared and transplanted within 2 h after collection. Stool samples of mice in the same group were mixed, weighed, and diluted with 1 mL of sterile saline per 0.1 g of stool. The mixture was placed at 4 °C for 15 min, shaken, and centrifuged at 68g for 3 min. The supernatant was then collected and filtered. Supernatants from the Control, Model and NR groups were collected and administered by gavage to the FMT Control, FMT Model and FMT NR groups, respectively (0.2 mL per mouse per day). FMT mice received sterile food, water and bedding. Each group was allocated its own set of experimental instruments to prevent cross-contamination, and the experiments were performed under aseptic conditions.
Sucrose preference test
The sucrose preference test was performed according to a previous report with minor modifications.28 Before the test, the mice were acclimatized to sucrose solution. At first, two bottles of 1% sucrose solution (w/v) were placed in each cage for 24 h, and then one bottle of sucrose solution was replaced with water for another 24 h to avoid sucrose neophobia. After adaptation, mice were deprived of liquid and food for 24 h, and then given the sucrose preference test. The mice were housed individually throughout the test duration. During the test, mice were presented simultaneously with two bottles; one containing 1% sucrose solution (w/v) and the other containing tap water; the bottles were weighed beforehand. At intervals of 0.5 h and 6 h, the bottles were switched to avoid position preference, and at intervals of 1 h and 12 h, the bottles were re-weighed. Sucrose consumption and water consumption were measured by comparing the weights of the bottles before and after the test. Sucrose preference was calculated as g sucrose consumed/(g sucrose consumed + g water consumed) (ESI Fig. 1B†).
Forced swim test
Mice were placed individually in a Plexiglas cylindrical container (25 cm height × 10 cm diameter) with a water depth of 10 cm maintained at a temperature of 23 ± 1 °C. In a test session, each mouse was forced to swim for a period of 6 min, and the last 4 min of the swim was recorded.29 During the test, mice with depressive behaviours became immobile as they stopped struggling and remained afloat passively, making only those movements necessary to keep their head above the water. The behaviour of each mouse was recorded during the test using a video camera (Handycam, Sony, Tokyo, Japan), and the immobility time was measured using SMART V3.0 software (Panlab Harvard Apparatus, Barcelona, Spain). The mice were considered immobile if the area covered during the test was less than 0.6 cm2 s−1 from preliminary experiments and was judged every 0.5 s by the software. Fresh water was introduced prior to each test to remove olfactory cues from mice tested previously. After the test, the mice were placed on a heating pad to dry.
Elevated plus maze
The elevated plus maze is a plus-shaped apparatus elevated above the floor by 50 cm and painted matte white. It consists of four opposite arms, each measuring 50 cm long × 10 cm wide. Two opposite arms are open to the surroundings while the other two opposite arms are closed with 30 cm-high walls. All the arms are interconnected forming an open area (10 × 10 cm) in the centre. Mice in each group were alternately tested in the maze for 5 min periods. Animals were placed at the intersection of the four arms facing an open arm.30 A video camera (Handycam, Sony) and a video tracking system (SMART V3.0, Panlab Harvard Apparatus) were used to automatically collect the behavioural data. Anxiety parameters included the frequency of entries and the time spent in the open arms. The entire elevated plus maze apparatus was cleaned with 75% alcohol solution after each trial to eliminate olfactory cues from the previously tested mice.
Y-maze task
The Y-maze consists of three identical white acrylic arms (50 × 32 × 16 cm [L × H × W]) positioned 120° to each other. The Y-maze test was performed according to a previous report with minor modifications.31 The test consisted of two trials separated by a 2 min interval. In the first trial, the novel arm of the Y-maze was blocked. Each mouse was placed at the end of the start arm, with its head pointing away from the centre of the maze, and was allowed to explore only the start arm and the familiar arm for 5 min. In the second trial, the novel arm was opened; mice were placed in the start arm and were allowed to explore all the three arms of the maze again for 5 min. The maze was wiped with a 75% alcohol solution before each test to remove olfactory cues from the previously tested animal. The time spent in the three arms and in the centre area were recorded using SMART V3.0 (Panlab Harvard Apparatus) (ESI Fig. 1C†).
Preparation of specimens
After the behavioural tests, the mice were deprived of food for 12 h, but were free to drink water. The mice were then anesthetized by intraperitoneal injection of sodium pentobarbital (40 mg kg−1), and blood was drawn from the eyeball before cervical dislocation. The mice were rapidly decapitated, and the brain was quickly removed. Some of the brains were randomly selected from each group for histological examination, and were accordingly fixed in 10% paraformaldehyde solution. The remaining brains were quickly dissected to remove the hippocampus for western blotting and enzyme-linked immunosorbent assay (ELISA). Blood was centrifuged at 956g for 10 min to collect serum. For microbiome analysis, 15 mice were randomly selected from the Control, Model and NR groups (5 mice per group), and the colon contents were collected into sterile centrifuge tubes using sterile forceps. Brain tissue, serum and colon contents were immediately flash-frozen in liquid nitrogen and stored at −80 °C until assay.
Immunofluorescence
Immunofluorescence labelling was performed according to our previously published method.32 Brain tissues were fixed in 10% paraformaldehyde overnight and embedded in paraffin. Tissues were sectioned and blocked with 5% normal goat serum in PBS for 30 min, and incubated with polyclonal goat anti-TMEM119 and polyclonal goat anti-CD68 antibodies (Santa Cruz Biotechnology, Dallas, TX) overnight at 4 °C. Thereafter, the sections were washed three times with PBS and incubated with anti-rabbit IgG or anti-mouse IgG for 60 min. DAPI was used to stain nuclei. The morphological changes in the microglial cells in the hippocampus were observed by fluorescence microscopy.
ELISA
Brain tissue was homogenized in 10% (w/v) phosphate buffer and centrifuged at 10
621g at 4 °C for 15 min. The supernatant was collected and analysed by ELISA. The levels of TNF-α, IL-6, IL-1β, IL-10 and TGF-β in brain homogenates and the total levels of brain-derived neurotrophic factor (BDNF) in serum were measured using commercial ELISA kits (USCN KIT Inc., Wuhan, Hubei, China) according to the manufacturer's instructions.
Western blotting
Total protein was extracted from the hippocampus using the Cytoplasmic Protein Extraction kit (Beyotime, Zhenjiang, Jiangsu, China) following the manufacturer's instructions, and quantified using the BCA Protein Assay Kit (Beyotime). Equal amounts of protein were resolved on 5–10% polyacrylamide gels and transferred onto PVDF membranes (Millipore, Bedford, MA). The membranes were blocked with 10% non-fat milk in Tris-buffered saline/Tween (TBST) and incubated with primary antibodies against BDNF (1
:
1000), TrkB (1
:
1000) (Abcam, Cambridge, England), p-Akt (Ser473) (1
:
2000), p-Akt (Thr308) (1
:
1000), p-GSK3β (Ser9) (1
:
2, 000), GSK3β (1
:
800), β-catenin (1
:
1000) (CST, Boston, USA), TMEM119 (1
:
500), CD68 (1
:
500) (Santa Cruz Biotechnology) or β-actin (1
:
1000) (Zhongshan Goldenbridge, Beijing, China) at 4 °C overnight. Subsequently, the membranes were washed three times with TBST for 10 min each and incubated with the corresponding secondary antibodies (Zhongshan Goldenbridge) for 2 h at 37 °C. Finally, the protein bands were visualized with an enhanced chemiluminescence detection kit (Beyotime). β-Actin served as an internal control.33
DNA extraction and 16S rRNA gene sequencing for microbiome analysis
After 10 weeks of intervention, fresh colon contents were collected from the mice in the Control, Model and NR treatment groups (5 mice per group) and stored at −80 °C until use. Genomic DNA was extracted from the samples using the QIAamp Fast DNA Stool Mini Kit (QIAGEN, Hilden, Germany) following the manufacturer's instructions. The extracted genomic DNA was quantified using a NanoDrop 2000 spectrophotometer (Thermo Fisher Scientific), and integrity was examined by agarose gel electrophoresis.
16S rRNA gene sequencing was performed by Realbio Genomics Institute (Shanghai, China). DNA from all the samples was amplified by polymerase chain reaction (PCR) using universal primers (341F and 806R) directed at the V3-V4 regions of the 16S ribosomal RNA (rRNA) gene. The amplified PCR products were quantified with a Qubit Fluorometer (Invitrogen, Carlsbad, CA, USA), followed by multiplex sequencing on an Illumina HiSeq PE250 platform (Illumina, Inc., San Diego, CA, USA) to obtain the paired-end reads of 2 × 250 bp. The raw reads were assembled with PANDAseq (AP Masella) and filtered according to the following quality control criteria: raw read length of 220–500 bp; minimum average Phred score of 20 (Q20); and maximum number of ambiguous bases (Ns) = 3. A total of 532
049 clean reads were generated from all the DNA samples, resulting in an average yield of 35
470 ± 1912 clean tags per sample. Contigs with 97% nucleotide sequence identity were clustered into operational taxonomic units (OTUs) with USEARCH. A representative sequence of each OTU was assigned to a species taxonomic level via the Ribosomal Database Project (RDP) with a minimum confidence threshold cutoff of 0.8.34 The raw sequence reads of the gut microbial contents have been registered in the Sequence Read Archive (SRA) database of GenBank (NCBI), under accession number PRJNA557221.
Both alpha and beta diversity metrics of the microbiome were generated using the QIIME package with default parameters after all the DNA samples were randomly subsampled to an even depth (22
957 reads). We used observed species diversity and Chao1 diversity indices for comparison of bacterial OTU richness, and the Shannon diversity index for comparison of bacterial OTU diversity of the gut microbiota. Principal coordinate analysis (PCoA) was performed on unweighted UniFrac distances of the relative abundances (normalized for each sample) of OTUs to visualize whether there was segregation of gut microbiota structure between the three groups. To test the differences in the microbial communities of the gut, we performed Analyses of Similarity (ANOSIM) to calculate R and P values using the phylogeny-based unweighted UniFrac distance metric. The LEfSe algorithm was used to analyse the differential abundance of gut microbiota among the groups. The correlation network between alcohol-induced depression-like behaviour-related indices and the intestinal microbiota was visualized with R Cytoscape software.33
Statistical analysis
Statistical analysis was performed using SPSS 23.0 (SPSS Inc., Chicago, IL, USA) and GraphPad Prism 5.0 (GraphPad Software, La Jolla, CA, USA). Quantitative data are reported as the mean ± standard error. Statistical differences in quantitative data between the groups were tested with one-way analysis of variance (ANOVA) and multiple t-tests with Bonferroni correction for continuous variables. The significance of comparisons between groups for non-parametric and parametric data with abnormal distribution was determined using the Kruskal–Wallis test, followed by the Mann–Whitney test. Association among variables was assessed using Spearman's rank correlation and tested for significance. Spearman's rank correlation was used to test associations between the differential genera and biochemical indexes, and a correlation heatmap was made using the R software package. P < 0.05 was considered statistically significant.
Results
Body weight and daily food consumption
The growth rate, body weight, and daily food consumption in the Model group were generally lower than those in the Control and NR groups. Specifically, there were significant differences at weeks 3, 4, 5, 7 and 10 (P < 0.05) (Fig. 1).
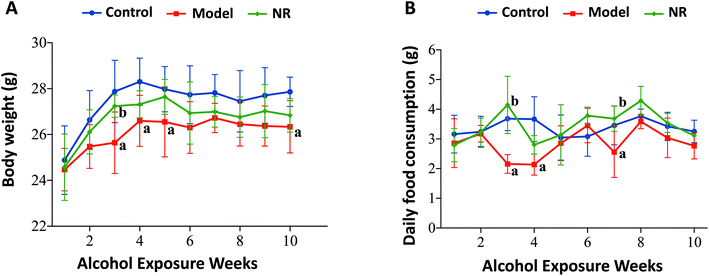 |
| Fig. 1 Body weight and daily food consumption. (A) Mean body weight of the mice. (B) Mean daily food consumption of the mice. The results are displayed as mean ± SD. aP < 0.05, Model group vs. Control group; bP < 0.05, NR group vs. Model group. | |
NR alleviates alcohol-induced cognitive and behavioural deficits
Before alcohol exposure, there were no significant differences in sucrose preference among the three groups (P < 0.05; Fig. 2A, left). After alcohol exposure, sucrose preference in the Model group was significantly decreased at 12 h compared with the Control and NR groups (P < 0.05; Fig. 2A, right). Similarly, alcohol exposure significantly increased the immobility time of mice in the forced swim test (P < 0.05; Fig. 2B). In the elevated plus maze test, the number of entries and the time spent in the open arms were significantly reduced in the Model group compared with the Control and NR groups (P < 0.05; Fig. 2C and D). In the Y-maze test, the time spent by the mice in the novel arm was decreased, while the time spent in the start arm was significantly increased, in the Model group compared with the Control and NR groups (P < 0.05; Fig. 2E and F). These results indicate that alcohol exposure can induce depression-like behaviour in mice, and that NR treatment effectively alleviates the symptoms.
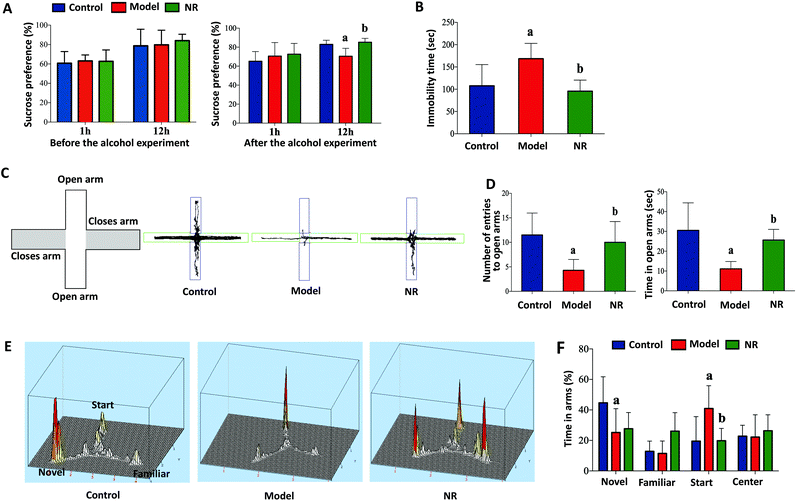 |
| Fig. 2 Cognitive and behavioural performance of mice. (A) Sucrose preference before alcohol exposure (left); sucrose preference after alcohol exposure (right). (B) Immobility time in the forced swim test. (C) A representative animal track in the elevated plus maze. (D) Number of entries into the open arms in the elevated plus maze (left); time spent in the open arms of the elevated plus maze (right). (E) Representative activity heat maps in the Y-maze task. (F) Time spent in the arms of the Y-maze task. Results are displayed as mean ± SD. aP < 0.05, Model group vs. Control group; bP < 0.05, NR group vs. Model group. | |
NR reduces alcohol-induced microglial activation in the hippocampus
Representative double immunofluorescence images of the hippocampus are shown in Fig. 3A. In the Model group, most of the TMEM119 staining colocalized with CD68 staining, suggesting that activated microglia are much more abundant in the hippocampus of mice with alcohol-induced depression. Western blot analysis was performed to assess expression levels of two microglial markers, TMEM119 and CD68. There were no significant differences in the levels of TMEM119; however, the levels of CD68 in the hippocampus of mice in the Model group were significantly increased compared with the Control and NR groups (P < 0.05; Fig. 3B). This result shows that while alcohol exposure did not change the number of microglia in the hippocampus, it increased their activation.
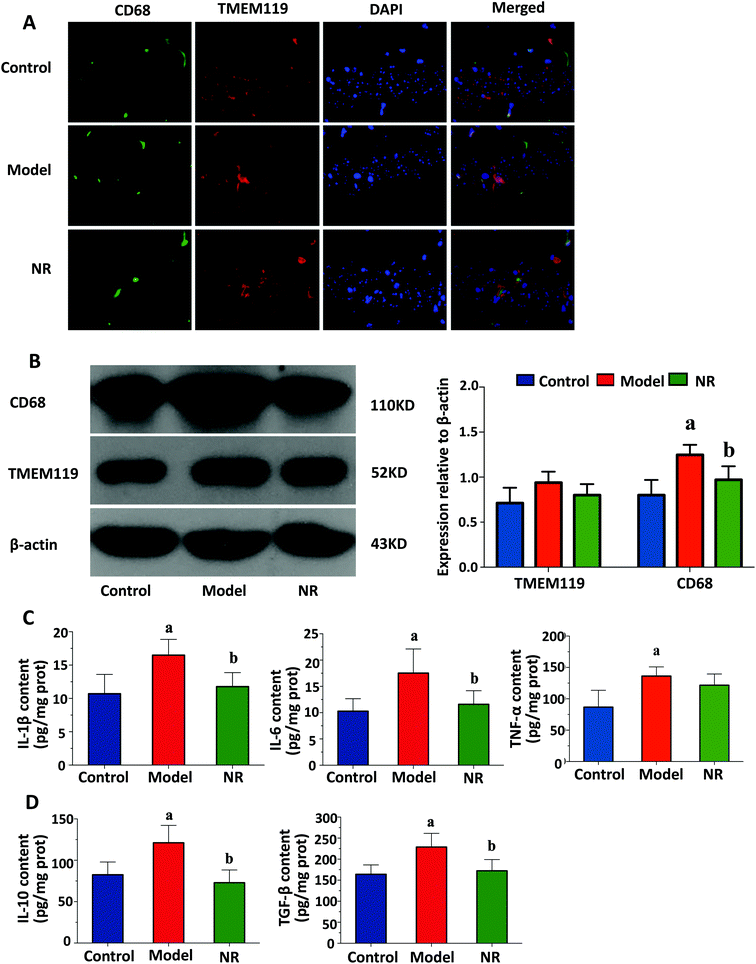 |
| Fig. 3 State of microglia in the hippocampus and the levels of inflammatory cytokines in the brain of mice. (A) Representative double immunofluorescence confocal microscopy images of CD68 (red) and TMEM119 (green) staining of microglia. Nuclei were counterstained with DAPI (blue). Scale bar = 20 μm. (B) Western blot analysis for CD68 and TMEM119 in the hippocampus (left); quantitative analysis of the western blot data (right); n = 5 mice per group; the experiment was performed three times. (C) The levels of pro-inflammatory cytokines in the brain. IL-1β (left), IL-6 (middle) and TNF-α (right). (D) The levels of anti-inflammatory cytokines in the brain. IL-10 (left) and TGF-β (right). Results are displayed as the mean ± SD. aP < 0.05, Model group vs. Control group; bP < 0.05, NR group vs. Model group. | |
NR attenuates alcohol-induced inflammatory cytokine release in the mouse brain
Alcohol exposure significantly increased the levels of pro-inflammatory (IL-1β, IL-6 and TNF-α) and anti-inflammatory cytokines (IL-10 and TGF-β) in the mouse brain. NR treatment significantly reduced the levels of these cytokines in mice exposed to alcohol (P < 0.05, Fig. 3C and D).
NR diminishes the alcohol-induced decrease in BDNF expression in the mouse hippocampus
ELISA revealed that the levels of serum BDNF were significantly decreased in the Model group compared with the Control and NR groups (P < 0.05; Fig. 4A). Furthermore, western blot analysis demonstrated that the expression levels of BDNF and TrkB in the hippocampus were significantly reduced in the Model group compared with the Control and NR groups (P < 0.05; Fig. 4B).
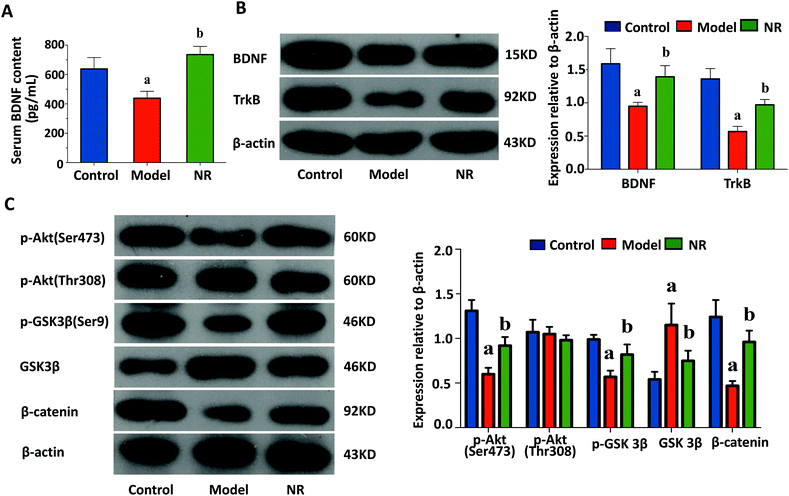 |
| Fig. 4 Levels of BDNF and TrkB and the activation status of the Akt/GSK3β/β-catenin signalling pathway. (A) Levels of serum BDNF assessed using ELISA. (B) Western blot analysis for BDNF and TrkB in the hippocampus (left); quantitative analysis of the western blot data (right); n = 5 mice per group; the experiment was performed three times. (C) Western blot analysis of proteins related to the Akt/GSK3β/β-catenin signalling pathway in the hippocampus (left); quantitative analysis of the western blot data (right); n = 5 mice per group; the experiment was performed three times. Data are presented as mean ± SEM. aP < 0.05, Model group vs. Control group; bP < 0.05, NR group vs. Model group. | |
NR modulates the alcohol-induced inhibition of the AKT/GSK3β/β-catenin signalling pathway in the hippocampus
The levels of p-AKT (Ser473), p-GSK3β and β-catenin were significantly decreased, while GSK3β was significantly upregulated, in the Model group compared with the Control and NR groups (P < 0.05; Fig. 4C). These results indicate that the Akt/GSK3β/β-catenin signalling pathway is significantly suppressed after alcohol intake, and activated following treatment with NR.
The effect of NR treatment on gut microbiota
Good's coverage (Fig. 5A, left), based on OTU richness, was >99.80%, suggesting that the vast majority of taxonomic units were detected in the samples. By comparing the bacterial alpha diversity between the Control, Model and NR groups, including community richness (Observed Species and Chao 1 index) and diversity (Shannon index), significant differences in community richness were observed between the Control and Model groups and between the NR and Model groups (P < 0.05; Fig. 5A, middle left and middle right). Although the diversity indices showed a decreasing trend (Control group > NR group > Model group), the inter-group differences were not statistically significant (Fig. 5A, right).
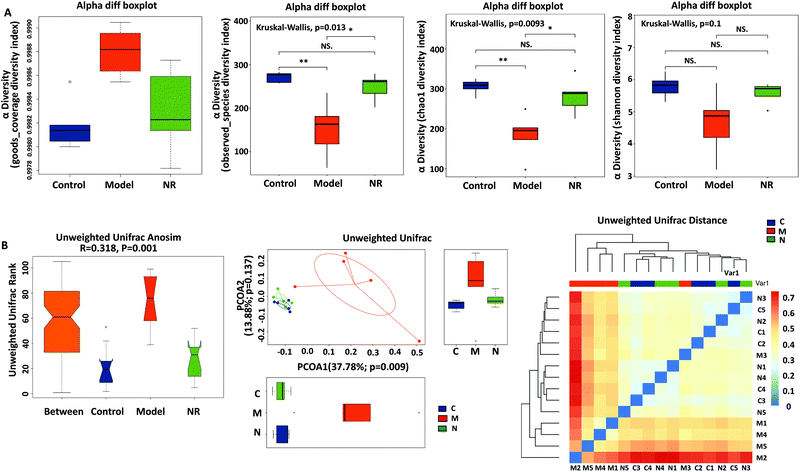 |
| Fig. 5 Diversity analysis of the gut microbiota. (A) Good's coverage was used to assess sequencing depth (left); observed species index (middle left) and Chao 1 index (middle right) were used to measure richness; Shannon index was used to estimate diversity (right); *P < 0.05; **P < 0.01; NS indicates that the inter-group differences were not statistically significant. (B) ANOSIM analysis of similarity (left). R-Values >0 indicate significant differences in the inter-group population compared with the intra-group population. P-Values <0.05 indicate a significantly different level between the groups. Principal coordinates analysis (PCoA) of the gut microbiota (middle). The percentage of variation explained by PCoA1 and PCoA2 are indicated on the axis. Distances between the samples are based on similarity in OTU composition (OTU similarity: 97%). A greater distance implies lower similarity, whereas similar OTUs will cluster together. A heatmap based on unweighted UniFrac distances of the 15 samples (right). Rows and columns represent the 15 samples. Dissimilarity between the samples is indicated by a colour gradient from blue (similar) to yellow to red (dissimilar). | |
Using beta-diversity analysis of ANOSIM based on unweighted UniFrac ranks, we found that vastly different bacterial community structures existed between the three groups (R = 0.318, P = 0.001; Fig. 5B, left). Moreover, the comparison of heatmaps based on unweighted UniFrac distances (Fig. 5B, right) showed that, compared with the Model group, lower distances were observed among the model and control samples. Principal Component Analysis (PCA) on unweighted UniFrac distances confirmed these results (Fig. 5B, middle). Based on PCA1 (37.78% of variance explained, P = 0.009) and PCA2 (13.88% of variance explained, P = 0.137) analyses, all the samples were grouped into three distinct clusters. The analyses revealed that the faecal microbial communities were different among the three groups, and that the pattern of microbiota in the NR group was relatively more similar to that in the Control group, while the Model group showed a greater difference with the other two groups.
The dominant genera (top 20) in the 16S rRNA sequence libraries were analysed in the three groups. From the pie charts of dominant genera, we found that there were different bacterial composition patterns in the three groups. The bacterial composition patterns of the Control and NR groups were similar, while that of the Model group was different from the other two groups (Fig. 6A). At the genus level, combined with the analysis of the dominant and the significantly differential species, we found that, compared with the Control and NR groups, the Model group microbiota was deficient in Prevotella, Barnesiella, Alloprevotella and Alistipes, while Akkermansia was significantly enriched. Furthermore, compared with the Control and NR groups, Bacterodies, Clostridium XIVa and Parabacteroides were reduced in the Model group; however, the differences were not significant (Fig. 6B and C).
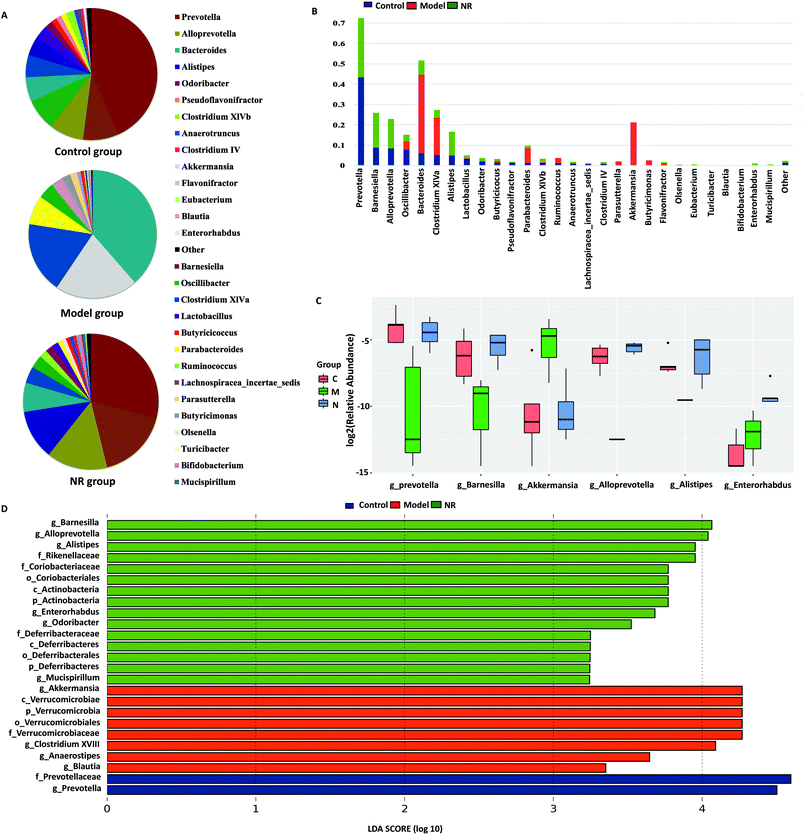 |
| Fig. 6 Analysis of the structure of the gut microbiota. (A) Dominant genera (top 20) assigned to Control, Model and NR groups. The area of different sectors in the pie chart represents the relative abundance of the various genera. (B) Top 20 genera from each group were consolidated into a pool of 28 dominant genera. The area of the histogram represents the relative abundance of the dominant genera. (C) Differential analysis of the relative abundance of bacteria at the genus level among the three groups. The relative abundance levels of six genera were significantly different among the three groups (P < 0.05). (D) The result of the LDA effect size (LEfSe) analysis. LEfSe was used to analyse total bacteria, which contribute to the differences at the phylum, class, order, family and genus levels (LDA score >2). | |
LEfSe analysis revealed that 25 OTUs at the phylum (3 OTUs), class (3 OTUs), order (3 OTUs), family (5 OTUs) and genus levels (11 OTUs) showed significant differences between the groups. Among the significantly differential OTUs, at the genus level, Akkermansia and Clostridium XVIII were the two most abundant bacteria in the Model group, while Barnesiella and Alloprevotella were the most abundant in the NR group (Fig. 6D).
To elucidate whether the changes in the gut microbiota of mice with alcohol-induced depression were correlated with brain inflammatory indices, the relationships between the 11 differential microflora genera and the five inflammation-related cytokines and BDNF were analysed using Spearman's correlation coefficient, based on hierarchical clustering and heatmaps. Brain BDNF level was significantly negatively correlated with the Model group-enriched bacteria, including Akkermansia, Clostridium XVIII, Anaerostipes and Blautia, and was significantly positively correlated with the Model group-deficient bacteria, including Prevotella, Alistipes, Mucispirillum, Alloprevotella, Barnesiella and Odoribacter. Conversely, inflammatory cytokines in the brain tended to be positively correlated with the Model group-enriched bacteria such as Akkermansia, Clostridium XVIII, Anaerostipes and Blautia, and tended to be negatively correlated with the Model group-deficient bacteria such as Prevotella, Alistipes, Mucispirillum, Alloprevotella, Barnesiella and Odoribacter. Specifically, IL-6 and TGF-β levels were significantly negatively correlated with Prevotella and Alistipes, but significantly positively correlated with Akkermansia. Il-1β levels were significantly positively correlated with Akkermansia, Anaerostipes and Blautia, and significantly negatively correlated with Prevotella, Alistipes, Mucispirillum, Barnesiella and Odoribacter. TNF-α levels were significantly positively correlated with Clostridium XVIII, but significantly negatively correlated with Barnesiella and Odoribacter. IL-10 levels were significantly positively correlated with Akkermansia, and significantly negatively correlated with Alistipes, Alloprevotella, Barnesiella and Odoribacter (Fig. 7).
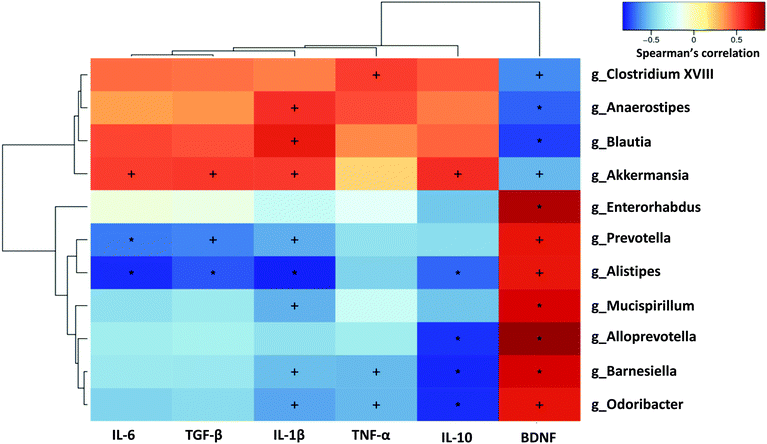 |
| Fig. 7 Correlation of inflammatory cytokines and BDNF with the differential genera of gut microbiota. Pearson product-moment correlation coefficients are represented by colour ranging from blue (negative correlation (−1)) to red (positive correlation (+1)). Significant correlations after P-value adjustment are indicated (+P < 0.05, *P < 0.01). | |
Changes in the recipient mice after FMT are similar to those in donor mice
To further clarify the role of gut microbiota in alcohol-induced depression in mice, we performed FMT experiments. After FMT, many of the changes in recipient mice, including changes in depression-like behaviour, the morphology of microglia, the levels of inflammatory cytokines and BDNF, and the status of the AKT/GSK3β/β-catenin signalling pathway, were similar to those in the donor mice. Specifically, mice in the FMT Model group showed depression-like behaviour, while mice in the FMT NR group showed no significant cognitive impairment (ESI Fig. 2†). Compared with the FMT Control group, the number of activated microglia in the hippocampus and the level of pro-inflammatory cytokines were significantly increased in the FMT Model group (P < 0.05, ESI Fig. 3A–C†). The anti-inflammatory cytokines also showed an increasing trend (ESI Fig. 3D†). In addition, BDNF was significantly downregulated in the FMT Model group (ESI Fig. 4A and B†), and the Akt/GSK3β/β-catenin signalling pathway was inhibited, compared with the FMT Control group (ESI Fig. 4C†). These changes were reduced in the FMT NR group, similar to the donor mice.
Discussion
Previous studies have shown neuroprotective and anti-depressive effects of NR. For example, Wang et al.35 found that NR effectively alleviates depression-like behaviour as well as spatial learning and memory deficits in adolescent rats after neonatal maternal deprivation. Brenner et al.36 found that NR supplementation increases BDNF content in the milk of mother mice, which is advantageous to the offspring as it increases adult hippocampal neurogenesis. The offspring of the mice are less anxious, with less depressive-like behaviour and improved spatial memory. Based on previous studies and our preliminary experiments, we identified 400 mg kg−1 NR as the optimal dose for the treatment of alcohol-induced depression-like behaviour in C57BL/6J mice.
Here, we found that the alcohol-induced depression-like behaviours were significantly ameliorated by oral administration of NR. We used the forced swim and sucrose preference tests to evaluate depressive like behaviours of mice treated with alcohol and NR. The forced swim test is one of the most commonly used tests for depressive-like behaviour in rodents.29 However, while the immobility time is associated with behavioural despair, it may also be affected by overall activity levels.37 Therefore, to substantiate the results, we used the sucrose preference test, which is not affected by overall activity levels, to co-evaluate the depression-like behaviour of mice. The sucrose preference test is used to assess anhedonia, which is a key symptom of human depression episodes.38 There was a significant decrease in the sucrose consumption rate and a significant increase in the duration of immobility in the forced swim test in the Model group compared with controls, which indicates that the mouse model of depression was successfully established in this study. NR significantly alleviated the depression-like behaviours in mice, suggesting that it has preventive and protective effects against alcohol-induced depression. Furthermore, NR administration significantly lessened the reduction in open-arm entries and residence time, which are related to anxiety, in the elevated plus maze.39 It also attenuated the impairment in spatial recognition memory in the Y-maze task.40
We also found that the body weight and daily food consumption were consistently lower in mice with alcohol-induced depression compared with mice in the Control and NR groups. Several studies on Korean subjects revealed that there is a U-shaped relationship between depression and body mass index.41,42 A study of a British birth cohort found that underweight subjects were associated with an increased risk of depression in both cross-sectional and longitudinal analyses.43 The abnormal emotions of depressive individuals are associated with decreased appetite. Appetite reduction, a depressive symptom, is listed in the Diagnostic and Statistical Manual of Mental Disorders, Fifth Edition.44
Microglial activation is closely related to depression.11,45 Previous studies in both rodent models of depression and in humans with major depressive disorder show that there is abnormal activation of microglia in the brain.46 The abnormal microglial activation can lead to the production of pro-inflammatory cytokines, neuronal apoptosis, inhibition of neurogenesis, reduced neurotransmitter synthesis and hippocampal volume reduction, all of which are related to depressive disorders.11 In this study, we found that the total number of microglia in the hippocampus of mice with alcohol-induced depression did not change significantly, but the number of activated microglia increased significantly. Thus, NR treatment effectively protects against microglial hyperactivation. Activated microglia can be categorized as M1-polarized or M2-polarized microglia. M1 (inflammatory) microglia are associated with the production of pro-inflammatory cytokines such as TNF-α, IL-1β and IL-6. In contrast, M2 microglia are pro-regenerative cells that are involved in tissue repair and the secretion of anti-inflammatory mediators, such as IL-4, IL-10 and TGF-β, and neurotrophic factors such as BDNF.47
Recent studies show that, in the central nervous system, overproduction of pro-inflammatory cytokines, particularly IL-1β, IL-6 and TNF-α, is likely to cause neuroinflammation and brain damage, leading to depressive mood, anxiety, and impaired memory and concentration, which subsequently transform into depression.48 The increase in the levels of pro-inflammatory cytokines in patients with depression can trigger the compensatory immune-regulatory system, which increases the levels of anti-inflammatory factors, such as IL-10, thereby neutralizing the excessive inflammatory response.49 However, studies on the levels of anti-inflammatory cytokines in patients and animals with depression have been inconsistent, which may be attributed to different triggering factors and different stages of depression.50,51 In our current study, compared with controls, the depressed animals had significantly increased levels of pro-inflammatory and anti-inflammatory cytokines, which are related to the activation status of microglia. After NR supplementation, the levels of pro-inflammatory and anti-inflammatory cytokines were both significantly lower compared with mice in the Model group, and were similar to those in control animals. Thus, NR effectively suppresses the inflammatory response in mice with alcohol-induced depressive-like behaviours.
Another factor closely related to depression is BDNF, which plays important roles in learning and memory, cognitive function, and the regulation of mood disorders.52 BDNF shows high binding affinity towards TrkB, and both are essential for synaptic plasticity, the formation of memories, and inflammation-related depression, all of which are thought to be involved in the pathophysiology of depression.52 BDNF/TrkB signalling is considered one of the central players in the pathogenesis, and a possible biomarker of, depression, and it has been associated with many antidepressants.53
Several studies show that BDNF levels in patients with major depressive disorder and in animal models of depression are significantly lower than in controls.54,55 Our present findings are in line with these observations. Several factors impact the expression of BDNF in the brain, such as microglia and inflammatory cytokines.56,57 Recent studies show that gut microbiota can also regulate BDNF expression and function in the hippocampus through the gut microbiota–brain axis.10
Previous studies demonstrate that the neuroprotective effects of BDNF may be mediated via the AKT/GSK3β/β-catenin signalling pathway,58 which mediates cell survival and regulates neuronal apoptosis.59 This pathway plays a critical role in neuroinflammation and neuroprotection.59,60 GSK3β, in particular, is a multifunctional serine-threonine kinase that plays an important role in the development of the central nervous system.61 GSK3β directly affects neurons via the regulation of neuronal cell death, migration, and differentiation.62 Furthermore, GSK3β may contribute to neuronal injury by modulating brain inflammation.63 Increased activation of GSK3β and inhibition of AKT/GSK3β/β-catenin signalling was detected in the brains of patients with major depression and rodents displaying depression-like behaviours.64 We observed similar changes in mice with alcohol-induced depression, which were ameliorated after NR administration.
Previous studies have shown that depression is related to changes in gut microbiota richness, diversity, and composition.65,66 Indeed, we found here that alcohol intake alters the composition of intestinal microbiota and reduces richness and diversity, and that dietary supplementation with NR can allay these changes.
The dominant bacterial genera in the gut of mice with alcohol-induced depression were significantly different from control and NR-treated mice. The prevalence of Prevotella, Barnesiella, Alloprevotella, Alistipes and Akkermansia were significantly different among the three groups. Furthermore, the three groups showed large differences in the levels of Bacterdies, Clostridium XIVa and Parabacteroides. Although several studies have reported changes in these genera in depressed individuals, the direction of these changes has been inconsistent.65,67 Here, we found that eight genera—Prevotella, Barnesiella, Alloprevotella, Alistipes, Akkermansia, Bacterdies, Clostridium XIVa and Parabacteroides—are more sensitive to alcohol-induced depression, although their role in the disease is unclear. Numerous factors may impact the intestinal microbiota, including diet, genetic factors, subject species, the specific model, environmental conditions, and drugs.68 Moreover, 16S rRNA surveys, which are often limited to the genus taxonomic level, fail to adequately resolve different species, particularly as different species from the same genus may contribute differentially to alcohol-induced depression.69,70 In addition, there are complex interactions among different genera of gut microbiota.68 Thus, these factors might account for the variability in observed genus-level bacterial changes among the different studies. Nonetheless, together, these previous reports and our current study show that gut microbiota play an important role in alcohol-induced depression.
Previous studies have shown that the intestinal microbiota and bacterial-derived short-chain fatty acids (SCFAs) strongly impact the abundance, gene expression profile, maturation and activation of microglia.8,71 In addition, many studies demonstrate that intestinal microbiota regulate the expression of BDNF in the hippocampus.72 In the present study, we found that the diversity and composition of intestinal microbiota in the mice with alcohol-induced depression were significantly different from mice in the Control and NR groups. In addition, microglial activation status and the levels of inflammation-related cytokines and BDNF in the mice with alcohol-induced depression were also significantly different from control and NR-treated animals. Furthermore, we found that the composition of intestinal microbiota was correlated with the levels of inflammation-related cytokines and BDNF in the brain tissue.
Based on our findings, we hypothesized that the changes in the intestinal microbiota play a critical role in the pathogenesis of alcohol-induced depression, and that NR alleviates the pathological changes. To test this hypothesis, we conducted FMT experiments. Notably, compared with donor mice, the mice in the FMT Model group not only exhibited depression-like behaviours, but also similarities in microglial activation status, cytokines and BDNF levels, and the activation state of the AKT/GSK3β/β-catenin signalling pathway. The characteristics of FMT NR mice were also similar to those of their donor mice. These results provide further evidence that NR ameliorates alcohol-induced depressive behaviours by affecting the intestinal microbiota.
Conclusions
In summary, our findings suggest that changes in the diversity and structure of the intestinal microbiota contribute to the development of alcohol-induced depression via several mechanisms, including activation of microglia and inflammatory cytokine release, inhibition of BDNF protein expression, and modulation of the AKT/GSK3β/β-catenin signal transduction pathway, which is downstream of the BDNF receptor, TrkB. Furthermore, we demonstrate that NR protects against alcohol-induced depression by improving the intestinal microbiota. To our knowledge, this is the first study showing that NR treatment effectively overcomes the bacterial dysbiosis caused by alcohol use. These novel findings provide further evidence that dietary supplements may positively impact human health. Previous studies have shown that NADH exhibits an antidepressant-like effect in Wistar rats in the forced swim test.73 Oral administration of NR, a precursor of NAD+, increases levels of the critical cofactor in the circulatory system.74 Further study is therefore needed to clarify whether the antidepressant-like effect of NR is mediated by NAD+/NADH. Nonetheless, our findings show that dietary supplementation with NR protects against alcohol-induced depression in mice, and they provide a foundation for the development of novel strategies for the prevention and treatment of this debilitating disorder.
Conflicts of interest
The authors declare no conflicts of interest.
Acknowledgements
This work was funded and supported by the National Natural Science Foundation of China (Grant No. 81573137 and 81872605), Key Research and Development Program in Shandong Province of China (Grant No. 2017GSF18167) and Medical Science and Technology Development Program in Shandong Province of China (Grant No. 2016WS0254). We thank Liyun Zeng and Feixiang Zhan from the Realbio Genomics Institute for their support of this study.
Notes and references
-
World Health Organization, Depression and other common mental disorders: global health estimates, World Health Organization, Geneva, 2017 Search PubMed
.
- C. Ménard, G. E. Hodes and S. J. Russo, Neuroscience, 2016, 321, 138–162 CrossRef PubMed
.
-
World Health Organization, Global status report on alcohol and health, World Health Organization, Geneva, 2018 Search PubMed
.
- J. M. Boden and D. M. Fergusson, Addiction, 2011, 106, 906–914 CrossRef PubMed
.
- D. M. Fergusson, J. M. Boden and L. J. Horwood, Arch. Gen. Psychiatry, 2009, 66, 260–266 CrossRef PubMed
.
- L. F. Iannone, A. Preda, H. M. Blottière, G. Clarke, D. Albani, V. Belcastro, M. Carotenuto, A. Cattaneo, R. Citraro, C. Ferraris, F. Ronchi, G. Luongo, E. Santocchi, L. Guiducci, P. Baldelli, P. Iannetti, S. Pedersen, A. Petretto, S. Provasi, K. Selmer, A. Spalice, A. Tagliabue, A. Verrotti, N. Segata, J. Zimmermann, C. Minetti, P. Mainardi, C. Giordano, S. Sisodiya, F. Zara, E. Russo and P. Striano, Expert Rev. Neurother., 2019, 19, 1037–1050 CrossRef CAS PubMed
.
- E. A. Mayer, K. Tillisch and A. Gupta, J. Clin. Invest., 2015, 125, 926–938 CrossRef PubMed
.
- K. I. Mosher and T. Wyss-Coray, Nat. Neurosci., 2015, 18, 930–931 CrossRef CAS PubMed
.
- J. F. Cryan and T. G. Dinan, Nat. Rev. Gastroenterol. Hepatol., 2015, 12, 494–496 CrossRef PubMed
.
- P. Bercik, E. Denou, J. Collins, W. Jackson, J. Lu, J. Jury, Y. Deng, P. Blennerhassett, J. Macri, K. D. McCoy, E. F. Verdu and S. M. Collins, Gastroenterology, 2011, 141, 599–609 CrossRef CAS PubMed
.
- R. Yirmiya, N. Rimmerman and R. Reshef, Trends Neurosci., 2015, 38, 637–658 CrossRef CAS PubMed
.
- F. Angelucci, S. Brenè and A. A. Mathé, Mol. Psychiatry, 2005, 10, 345–352 CrossRef CAS PubMed
.
- J. M. Beaulieu, R. R. Gainetdinov and M. G. Caron, Annu. Rev. Pharmacol. Toxicol., 2009, 49, 327–347 CrossRef CAS PubMed
.
- P. A. Engen, S. J. Green, R. M. Voigt, C. B. Forsyth and A. Keshavarzian, Alcohol Res., 2015, 37, 223–236 Search PubMed
.
- S. Akhondzadeh, P. L. Gerbarg and R. P. Brown, Psychiatr. Clin. North Am., 2013, 36, 25–36 CrossRef PubMed
.
- L. M. Manosso, M. Moretti and A. L. Rodrigues, Food Funct., 2013, 4, 1776–1793 RSC
.
- Y. Chi and A. A. Sauve, Curr. Opin. Clin. Nutr. Metab. Care, 2013, 16, 657–661 CrossRef CAS PubMed
.
- S. A. Trammell, L. Yu, P. Redpath, M. E. Migaud and C. Brenner, J. Nutr., 2016, 146, 957–963 CrossRef CAS PubMed
.
- Y. Hou, S. Lautrup, S. Cordonnier, Y. Wang, D. L. Croteau, E. Zavala, Y. Zhang, K. Moritoh, J. F. O'Connell, B. A. Baptiste, T. V. Stevnsner, M. P. Mattson and V. A. Bohr, Proc. Natl. Acad. Sci.U. S. A., 2018, 115, E1876–E1885 CrossRef CAS PubMed
.
- P. Vaur, B. Brugg, M. Mericskay, Z. Li, M. S. Schmidt, D. Vivien, C. Orset, E. Jacotot, C. Brenner and E. Duplus, FASEB J., 2017, 31, 5440–5452 CrossRef CAS PubMed
.
- M. V. Hamity, S. R. White, R. Y. Walder, M. S. Schmidt, C. Brenner and D. L. Hammond, Pain, 2017, 158, 962–972 CrossRef CAS PubMed
.
- M. S. Desai, A. M. Seekatz, N. M. Koropatkin, N. Kamada, C. A. Hickey, M. Wolter, N. A. Pudlo, S. Kitamoto, N. Terrapon, A. Muller, V. B. Young, B. Henrissat, P. Wilmes, T. S. Stappenbeck, G. Núñez and E. C. Martens, Cell, 2016, 167, 1339–1353 CrossRef CAS PubMed
.
- R. Liu, J. Hong, X. Xu, Q. Feng, D. Zhang, Y. Gu, J. Shi, S. Zhao, W. Liu, X. Wang, H. Xia, Z. Liu, B. Cui, P. Liang, L. Xi, J. Jin, X. Ying, X. Wang, X. Zhao, W. Li, H. Jia, Z. Lan, F. Li, R. Wang, Y. Sun, M. Yang, Y. Shen, Z. Jie, J. Li, X. Chen, H. Zhong, H. Xie, Y. Zhang, W. Gu, X. Deng, B. Shen, X. Xu, H. Yang, G. Xu, Y. Bi, S. Lai, J. Wang, L. Qi, L. Madsen, J. Wang, G. Ning, K. Kristiansen and W. Wang, Nat. Med., 2017, 23, 859–868 CrossRef CAS PubMed
.
- P. F. Carrara-Nascimento, M. F. Lopez, H. C. Becker, M. F. Olive and R. Camarini, Alcohol.: Clin. Exp. Res., 2013, 37, 961–968 CrossRef CAS PubMed
.
- B. S. Nelson, M. K. Sequeira and J. R. Schank, Addict. Biol., 2018, 23, 142–153 CrossRef CAS PubMed
.
- C. Chevalier, O. Stojanović, D. J. Colin, N. Suarez-Zamorano, V. Tarallo, C. Veyrat-Durebex, D. Rigo, S. Fabbiano, A. Stevanović, S. Hagemann, X. Montet, Y. Seimbille, N. Zamboni, S. Hapfelmeier and M. Trajkovski, Cell, 2015, 163, 1360–1374 CrossRef CAS PubMed
.
- Z. Ye, N. Zhang, C. Wu, X. Zhang, Q. Wang, X. Huang, L. Du, Q. Cao, J. Tang, C. Zhou, S. Hou, Y. He, Q. Xu, X. Xiong, A. Kijlstra, N. Qin and P. Yang, Microbiome, 2018, 6, 135–148 CrossRef PubMed
.
- M. Boyko, R. Kutz, J. Grinshpun, V. Zvenigorodsky, S. E. Gruenbaum, B. F. Gruenbaum, E. Brotfain, Y. Shapira and A. Zlotnik, Behav. Brain Res., 2015, 281, 358–363 CrossRef PubMed
.
- A. Can, D. T. Dao, M. Arad, C. E. Terrillion, S. C. Piantadosi and T. D. Gould, J. Visualized Exp., 2012, e3638 Search PubMed
.
- X. Hu, T. Wang, J. Luo, S. Liang, W. Li, X. Wu, F. Jin and L. Wang, Behav. Brain Funct., 2014, 10, 30–39 CrossRef PubMed
.
- T. Blackmore, S. Meftah, T. K. Murray, P. J. Craig, A. Blockeel, K. Phillips, B. Eastwood, M. J. O'Neill, H. Marston, Z. Ahmed, G. Gilmour and F. Gastambide, Alzheimers Res. Ther., 2017, 9, 77–72 CrossRef PubMed
.
- N. Ge, H. Liang, Y. Liu, A. G. Ma and L. Han, Food Chem. Toxicol., 2013, 62, 361–372 CrossRef CAS PubMed
.
- Y. Dong, H. Cheng, Y. Liu, M. Xue and H. Liang, Food Funct., 2019, 10, 3880–3889 RSC
.
- M. Xue, Y. Liu, R. Lyu, N. Ge, M. Liu, Y. Ma and H. Liang, PLoS One, 2017, 12, e0178684 CrossRef PubMed
.
- R. X. Wang, Z. K. Fang, S. Q. Yao, Y. Y. Xue, Y. Y. Zhao and Y. L. Ye, Chin. J. Clin. Pharmacol. Ther., 2018, 23, 1015–1021 Search PubMed
.
- C. Brenner, Curr. Dev. Nutr., 2019, 3, 961–962 CrossRef
.
- M. Hascoét and M. Bourin, Neuromethods, 2009, 42, 85–118 CrossRef
.
- R. Rygula, N. Abumaria, G. Flügge, E. Fuchs, E. Rüther and U. Havemann-Reinecke, Behav. Brain Res., 2005, 162, 127–134 CrossRef PubMed
.
- A. A. Walf and C. A. Frye, Nat. Protoc., 2007, 2, 322–328 CrossRef CAS PubMed
.
- M. X. Ma, Y. M. Chen, J. He, T. Zeng and J. H. Wang, Neuroscience, 2007, 147, 1059–1065 CrossRef CAS PubMed
.
- J. H. Lee, S. K. Park, J. H. Ryoo, C. M. Oh, J. M. Choi, R. S. McIntyre, R. B. Mansur, H. Kim, S. Hales and J. Y. Jung, Eur. Psychiatry, 2017, 45, 72–80 CrossRef PubMed
.
- J. Oh, J. H. Chae and T. S. Kim, Int. J. Obes., 2018, 42, 327–333 CrossRef CAS PubMed
.
- M. C. Geoffroy, L. Li and C. Power, Psychol. Med., 2014, 44, 2641–2652 CrossRef PubMed
.
-
American Psychiatric Association, Diagnostic and statistical manual of mental disorders (DSM-5®), American Psychiatric Pub, Washington, 2013 Search PubMed
.
- G. Singhal and B. T. Baune, Front. Cell. Neurosci., 2017, 11, 270–286 CrossRef PubMed
.
- L. R. Frick, K. Williams and C. Pittenger, Clin. Dev. Immunol., 2013 DOI:10.1155/2013/608654
.
- Y. Nakagawa and K. Chiba, Pharmaceuticals, 2014, 7, 1028–1048 CrossRef CAS PubMed
.
- C. L. Raison, L. Capuron and A. H. Miller, Trends Immunol., 2006, 27, 24–31 CrossRef CAS PubMed
.
- M. N. Noto, M. Maes, S. O. V. Nunes, V. K. Ota, A. C. Rossaneis, W. A. Verri Jr., Q. Cordeiro, S. I. Belangero, A. Gadelha, R. A. Bressan and C. Noto, Eur. Neuropsychopharmacol., 2019, 29, 416–431 CrossRef CAS PubMed
.
- K. Krieglstein, F. Zheng, K. Unsicker and C. Alzheimer, Trends Neurosci., 2011, 34, 421–429 CrossRef CAS PubMed
.
- D. R. Goldsmith, M. H. Rapaport and B. J. Miller, Mol. Psychiatry, 2016, 21, 1696–1709 CrossRef CAS PubMed
.
- B. Lu, G. Nagappan and Y. Lu, Exp. Pharmacol., 2014, 220, 223–250 CAS
.
- J. Zhang, W. Yao and K. Hashimoto, Curr. Neuropharmacol., 2016, 14, 721–731 CrossRef CAS PubMed
.
- M. L. Molendijk, P. Spinhoven, M. Polak, B. A. Bus, B. W. Penninx and B. M. Elzinga, Mol. Psychiatry, 2014, 19, 791–800 CrossRef CAS PubMed
.
- A. Berry, V. Bellisario, S. Capoccia, P. Tirassa, A. Calza, E. Alleva and F. Cirulli, Psychoneuroendocrinology, 2012, 37, 762–772 CrossRef CAS PubMed
.
- C. N. Parkhurst, G. Yang, I. Ninan, J. N. Savas, J. R. Yates 3rd, J. J. Lafaille, B. L. Hempstead, D. R. Littman and W. B. Gan, Cell, 2013, 155, 1596–1609 CrossRef CAS PubMed
.
- F. Calabrese, A. C. Rossetti, G. Racagni, P. Gass, M. A. Riva and R. Molteni, Front. Cell. Neurosci., 2014, 8, 430–437 Search PubMed
.
- X. T. Li, Z. Liang, T. T. Wang, J. W. Yang, W. Ma, S. K. Deng, X. B. Wang, Y. F. Dai, J. H. Guo and L. Y. Li, CNS Neurol. Disord.: Drug Targets, 2017, 16, 828–836 CrossRef CAS PubMed
.
- S. Zhao, J. Fu, X. Liu, T. Wang, J. Zhang and Y. Zhao, Neurol. Res., 2012, 34, 400–407 CrossRef CAS PubMed
.
- Y. Kitagishi, M. Kobayashi, K. Kikuta and S. Matsuda, Depress. Res. Treat., 2012, 2012, 752563 Search PubMed
.
- W. Y. Kim, E. M. Hur, W. D. Snider and F. Q. Zhou, Front. Mol. Neurosci., 2011, 4, 48 Search PubMed
.
- E. Beurel, S. F. Grieco and R. S. Jope, Pharmacol. Ther., 2015, 148, 114–131 CrossRef CAS PubMed
.
- R. S. Jope, Y. Cheng, J. A. Lowell, R. J. Worthen, Y. H. Sitbon and E. Beurel, Trends Biochem. Sci., 2017, 42, 180–192 CrossRef CAS PubMed
.
- J. Pláteník, Z. Fišar, R. Buchal, R. Jirák, E. Kitzlerová, M. Zvěřová and J. Raboch, Prog. Neuropsychopharmacol. Biol. Psychiatry, 2014, 50, 83–93 CrossRef PubMed
.
- H. Jiang, Z. Ling, Y. Zhang, H. Mao, Z. Ma, Y. Yin, W. Wang, W. Tang, Z. Tan, J. Shi, L. Li and B. Ruan, Brain, Behav., Immun., 2015, 48, 186–194 CrossRef PubMed
.
- M. C. Cenit, Y. Sanz and P. Codoñer-Franch, World J. Gastroenterol., 2017, 23, 5486–5498 CrossRef CAS PubMed
.
- S. G. Cheung, A. R. Goldenthal, A. C. Uhlemann, J. J. Mann, J. M. Miller and M. E. Sublette, Front. Psychiatry, 2019, 10, 34–51 CrossRef PubMed
.
- L. Dethlefsen, P. B. Eckburg, E. M. Bik and D. A. Relman, Trends Ecol. Evol., 2006, 21, 517–523 CrossRef PubMed
.
- M. V. Larsen, S. Cosentino, O. Lukjancenko, D. Saputra, S. Rasmussen, H. Hasman, T. Sicheritz-Pontén, F. M. Aarestrup, D. W. Ussery and O. Lund, J. Clin. Microbiol., 2014, 52, 1529–1539 CrossRef PubMed
.
- C. Noecker, C. P. McNally, A. Eng and E. Borenstein, Transl. Res., 2017, 179, 7–23 CrossRef CAS PubMed
.
- C. N. Heiss and L. E. Olofsson, J. Neuroendocrinol., 2019, 31, e12684 CrossRef PubMed
.
- R. Maqsood and T. W. Stone, Neurochem. Res., 2016, 41, 2819–2835 CrossRef CAS PubMed
.
- A. Rex, R. Schickert and H. Fink, Pharmacol., Biochem. Behav., 2004, 77, 303–307 CrossRef CAS PubMed
.
- S. E. Airhart, L. M. Shireman, L. J. Risler, G. D. Anderson, G. A. N. Gowda, D. Raftery, R. Tian, D. D. Shen and K. D. O'Brien, PLoS One, 2017, 12, e0186459 CrossRef PubMed
.
Footnotes |
† Electronic supplementary information (ESI) available. See DOI: 10.1039/C9FO01780A |
‡ The authors contributed equally to this work. |
|
This journal is © The Royal Society of Chemistry 2020 |
Click here to see how this site uses Cookies. View our privacy policy here.