DOI:
10.1039/D0RA09010G
(Paper)
RSC Adv., 2020,
10, 40867-40875
Accelerating the repurposing of FDA-approved drugs against coronavirus disease-19 (COVID-19)†
Received
22nd October 2020
, Accepted 2nd November 2020
First published on 10th November 2020
Abstract
The recent release of the main protein structures belonging to SARS CoV-2, responsible for the coronavirus disease-19 (COVID-19), strongly pushed for identifying valuable drug treatments. With this aim, we show a repurposing study on FDA-approved drugs applying a new computational protocol and introducing a novel parameter called IVSratio. Starting with a virtual screening against three SARS CoV-2 targets (main protease, papain-like protease, spike protein), the top-ranked molecules were reassessed combining the Inverse Virtual Screening novel approach and MM-GBSA calculations. Applying this protocol, a list of drugs was identified against the three investigated targets. Also, the top-ranked selected compounds on each target (rutin vs. main protease, velpatasvir vs. papain-like protease, lomitapide vs. spike protein) were further tested with molecular dynamics simulations to confirm the promising binding modes, obtaining encouraging results such as high stability of the complex during the simulation and a good protein–ligand interaction network involving some important residues of each target. Moreover, the recent outcomes highlighting the inhibitory activity of quercetin, a natural compound strictly related to rutin, on the SARS-CoV-2 main protease, strengthened the applicability of the proposed workflow.
Introduction
At the end of 2019, several cases of pneumonia caused by a novel virus were reported in Wuhan, China. This pathogen is a member of the Coronaviridae virus family, which is characterized by a positive-sense single-stranded RNA genome,1 and shares 79.5% sequence identity with another well-known coronavirus: the SARS-CoV;2–5 for this reason, the virus was called SARS-CoV-2.6–9 The failure of the containment measures and the high infectivity of the virus, transformed a local problem into a pandemic disease (The World Health Organization, March 11th, 2020). This tragic outbreak counts currently over 33 million cases and 1 million deaths worldwide, due mainly to severe respiratory syndrome and its complications. Scientists all over the world are joining the forces to come up with a cure for this disease, whether it is a small molecule or an antibody, and ameliorate the clinical picture of the patients. This resulted in around 3400 ongoing clinical trials, according to the National Institute of Health (data from https://clinicaltrials.gov/ct2/results?cond=COVID-19 accessed on September 30th). The current therapeutic protocols include known antiviral drugs10–12 as a specific molecule has not yet been found, though many studies are focusing on the repurposing of existing drugs to be used against either the infection itself or the symptoms and complications related to it.13 Several ongoing trials involving anti-interleukin-6-receptor (IL6R) drug tocilizumab are of great importance.14,15 In this framework, the structural elucidation of viral proteins, mainly spike glycoprotein (S), small envelope protein (E), matrix glycoprotein (M), and nucleocapsid protein (N),16 results extremely useful to design and create specific ligands for these targets. At the moment, due to the massive effort of the scientific community, over 400 protein structures of SARS-CoV-2, including the S protein, are available in the Protein Data Bank (https://www.rcsb.org/).17,18
Computational techniques are essential in these early stages of research as they can provide useful data in a short period, boosting the subsequent phases of research.19–25 For instance, the accurate prediction of the binding affinity represents one of the most interesting field of investigation.26–28 Moreover, to date there is no vaccine available, and the computational repurposing campaigns are privileged approaches as they deal with already approved drugs that will not need to pass the entire pre-clinical phase.29,30 In this framework, several new studies were published in the last few months concerning the use of existing drugs (alone or in combination) in fighting COVID-19.31–33 Obviously, some of the papers focused their attention on existing antiviral drugs (lopinavir, saquinavir, etc.) or molecules that showed a good activity on the SARS-CoV virus in the past years.34–36 The main strategy remains to prevent the virus from entering human cells by inhibiting one of the many components responsible for the viral uptake.34
Therefore, starting from the three main protein categories available in the database (the main protease, the spike protein, and the papain-like protein), we decided to carry out a drug repurposing study using the FDA-approved drugs to highlight new possible drug candidates. We used a new approach that foresaw the application of direct virtual screening to select the most valuable candidates for each target. Such molecules were then tested using an Inverse Virtual Screening (IVS) approach on a panel of viral proteins, including the three initial ones, to confirm the robustness of the obtained results. Moreover, we introduced a new reliability parameter, called IVSratio, to study the binding affinity of the compounds selected on the specific SARS-CoV-2 target compared to other “decoy” receptors. This parameter can give an indication whether the calculated binding affinity is within the top-ranked if compared with other targets.
Materials and methods
Input file preparation
The three-dimensional structure of the SARS-CoV-2 main protease, bound to an inhibitor (PDB: 6LU7),37 the spike protein receptor-binding domain (PDB: 6M0J),38 and the papain-like protease (PDB: 6W9C)39 were downloaded from the Protein Data Bank. The targets were cleaned and prepared following the automated protocol previously reported by us (available at http://computorgchem.unisa.it/cloe/).40 In detail the solvent molecules, ions, and unnecessary protein chains were removed; then, the structural features like bond order and protonation state were adjusted using the Protein Preparation Wizard.41,42 Due to the presence of an inhibitor in the crystal structure of the main protease, the molecular docking grid was built taking the centroid of the ligand as the center of the box (x: −10.83, y:12.57, z: 68.68) and extending the latter of 25 Å, 27 Å, and 25 Å on the x, y, and z-axis respectively. For the other two proteins, lacking an indication about the pharmacological site of interest, the putative binding pockets were detected using SiteMap43–45 and the centroid of the top-ranked one was used as the center of the docking grid (x: −32.31, y:16.72, z: 33.31 for papain-like protease and x: −32.31, y:12.72, z: 30.31 for the spike protein). The dimensions of the two boxes are 21 × 21 × 25 Å and 22 × 21 × 28 Å for the papain-like protease and the spike protein, respectively. The viral proteins composing the target panel for the Inverse Virtual Screening were prepared likewise. Concerning the ligands, the chemical structures of FDA-approved drugs were downloaded from the Selleckchem website (https://www.selleckchem.com/screening/fda-approved-drug-library.html) in the SDF format and prepared with LigPrep46 to assign the correct protonation state and regularize the geometries. The configurations were not altered in the final structure. Both ligands and targets were then converted into the PDBQT format using OpenBabel.47
Direct and Inverse Virtual Screening
The library of approved drugs was screened against the three viral targets using the software AutoDock Vina48 at the exhaustiveness of 64. From the results obtained, the best molecules were selected by setting an energetic cut-off of 0.5 kcal mol−1 from the best value of each protein. Such molecules were, then, tested against a panel composed of viral proteins already reported elsewhere40 using the IVS approach.49,50 We calculated the IVSratio with the following equation:
where BAligand is the calculated binding affinity of the ligand against the selected target and BAmax is the best binding affinity obtained against the whole panel.
MM-GBSA
The efficacy of the binding was further evaluated with MM-GBSA. The protein–ligand complexes with an IVSratio within 0.1 from the best result were tested using the software Prime with the VSGB solvent model and OPLS-2005 force field. The residues surrounding the ligand (6.0 Å) were allowed to move during the minimization to reduce steric clashes.
Molecular dynamics
The protein–ligand complexes selected for each target were prepared for the molecular dynamics simulations. Each complex was inserted in an orthorhombic box with a buffer distance of 10 Å in each space direction and solvated with TIP3P water molecules. The system charge was neutralized by adding Na+ or Cl− ions and then the physiological cell environment was mimicked by adding 0.15 M of NaCl. Once ready, the systems were simulated for 100 ns in NPT ensemble using the software Desmond36,37 after a relation phase carried out with an internal 5-step protocol. At first, the system was adjusted in NVT ensemble at 10 K with Brownian Dynamics for 100 ns, followed by two 12 ns steps at 10 K, first in NVT, and then in NPT ensemble, with restrains on solute heavy atoms. In the end, two steps (12 ns and 24 ns, respectively) were carried out at 300 K and in NPT ensemble with and without restrains.
MD analysis
Each trajectory was analyzed to extract qualitative information. In particular, the backbone RMSD, the RMSF and the radius of gyration (Rg) were calculated for each frame, taking the initial structure as reference.
Results and discussion
In order to identify repurposed FDA-approved drugs for treating COVID-19, we introduced a new computational protocol basing on the application of virtual screening, our recently introduced and implemented Inverse Virtual Screening approach, MM-GBSA calculations, and molecular dynamics simulations (Fig. 1). In detail, the three-dimensional structure of each ligand belonging to the FDA-approved drugs library was adjusted using LigPrep,46 which regularizes the geometries, and determines the correct protonation state at physiological pH. Three of the most important SARS-CoV-2 proteins were selected as targets: the virus main protease (PDB: 6LU7),37 the spike protein receptor-binding domain (PDB: 6M0J),38 and the papain-like protease (PDB: 6W9C).39 To narrow down the putative ligands for each protein, a virtual screening campaign was carried out using the software AutoDock Vina.48 We then selected the molecules within a binding affinity range of 0.5 kcal mol−1 from the best result for each protein target. This first group of 27 molecules (see ESI†) was used for an Inverse Virtual Screening campaign against a panel of viral proteins previously prepared40 in which we added the three SARS-CoV-2 proteins (1027 total proteins). In this way, we wanted to relate the predicted binding affinities on the investigated SARS CoV-2 targets with those obtained testing the same selected molecules on a large panel of other viral proteins, with the final aim of identifying the most robust results and to exclude putative false positives.
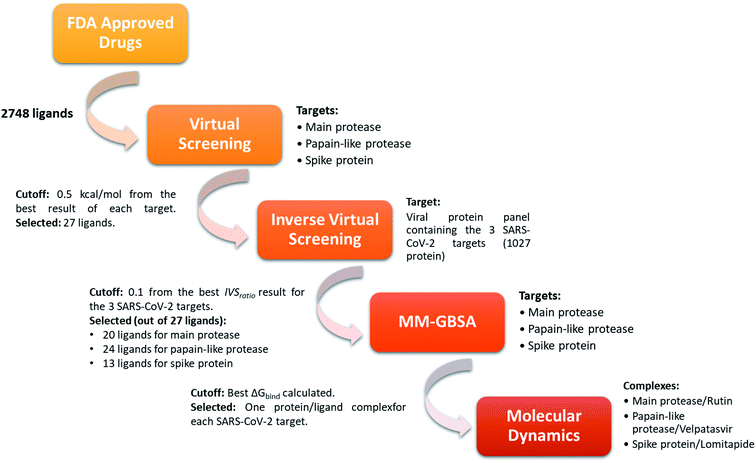 |
| Fig. 1 Schematic view of the computational approach used. In each step, the targets and the cutoff parameters are listed. | |
Indeed, for each molecule, we calculated the IVSratio by dividing the calculated binding affinity of the molecule against each of the SARS-CoV-2 protein by the best binding affinity calculated on the whole panel (see Materials and methods). In this way, we were able to estimate the discrepancy between the considered binding affinity and the best one, evaluating whether it falls above the average value. Using this parameter, we were confident that the protein–ligand complex was among the top-ranked ones and was not false positive result. The final additional refinement was carried out, at this point, with the calculation of the ΔGbind energy through MM-GBSA for the molecules that had an IVSratio within 0.1 from the best result. Out of the 27 ligands tested on each protein target, 20, 24, and 13 ligands were initially found within the selected range for the main protease, the papain-like protease, and the spike protein, respectively (Fig. 1). Some of them were redundant results and they were deleted from the final ranking (Table 1).
Table 1 Molecules selected from the IVS experiments. In the case of duplicates (tautomers), only the best binding affinity value was kept
Name |
CAS no |
Target |
Max affinity on the panela |
Binding affinitya |
MM-GBSAa |
IVSratio |
Expressed in kcal mol−1. |
Main protease |
Rutin |
207671-50-9 |
Cell proliferation |
−12.10 |
−9.50 |
−99.80 |
0.8 |
Entrectinib |
1108743-60-7 |
Trk receptor, ROS1 and ALK |
−13.00 |
−9.80 |
−86.56 |
0.8 |
Bromocriptine mesylate |
22260-51-1 |
D2 receptors |
−12.80 |
−9.70 |
−82.65 |
0.8 |
Peficitinib |
944118-01-8 |
JAK |
−12.70 |
−9.80 |
−81.46 |
0.8 |
Dihydroergotamine mesylate |
6190-39-2 |
Adrenergic receptor |
−13.00 |
−9.00 |
−74.39 |
0.7 |
Dolutegravir |
1051375-19-9 |
HIV integrase |
−11.30 |
−9.40 |
−70.62 |
0.8 |
![[thin space (1/6-em)]](https://www.rsc.org/images/entities/char_2009.gif) |
Papain-like protease |
Velpatasvir |
1377049-84-7 |
Hepatitis C NS5A |
−13.00 |
−7.50 |
−109.02 |
0.6 |
Dutasteride |
164656-23-9 |
5-Alpha reductase |
−12.80 |
−8.10 |
−86.40 |
0.6 |
Conivaptan |
168626-94-6 |
Vasopressin receptor |
−13.90 |
−8.30 |
−73.18 |
0.6 |
![[thin space (1/6-em)]](https://www.rsc.org/images/entities/char_2009.gif) |
Spike protien |
Lomitapide |
182431-12-5 |
MTP |
−12.70 |
−9.30 |
−98.46 |
0.7 |
Dihydroergotamine mesylate |
6190-39-2 |
Adrenergic receptor |
−13.00 |
−9.40 |
−84.67 |
0.7 |
Olaparib |
763113-22-0 |
PARP |
−11.80 |
−9.50 |
−80.19 |
0.8 |
From the analysis of this amount of data (see ESI Tables S1–S12†), some interesting results emerged (Table 1). Among them, two antiviral agents (dolutegravir acting as anti-HIV integrase and velpatasvir acting against Hepatitis C NS5A) were identified and represented interesting outcomes.
Specifically, the best ligand for the main protease, the papain-like protease, and the spike protein, according to the MM-GBSA calculations, were rutin, velpatasvir, and lomitapide, respectively (Fig. 2).
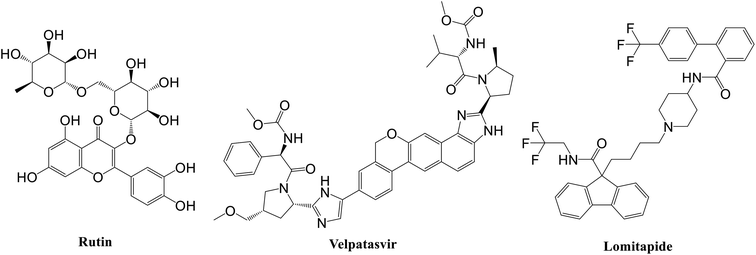 |
| Fig. 2 Chemical structure of the best ligand for each SARS-CoV-2 protein according to MM-GBSA experiments. | |
Rutin is a derivative of quercetin containing a glucose/rhamnose glycosyl group (rhamnose) at C-3. It is a flavonoid with antioxidant, antiproliferative, and anti-inflammatory properties that can be found in different plant species and is very common in Chinese traditional medicine. Recently, this natural compound was predicted active on the SARS-CoV-2 main protease by Abd El-Mordy et al.,51 Al-Zahrani,52 and in other studies,53–55 giving a further indication about the robustness of the presented method. Very interestingly, an important milestone was recently reached by Abian et al.56 who identified and tested in vitro quercetin, the precursor of rutin, against SARS-CoV-2, finding a very promising inhibitory activity on the main protease (Ki ∼ 7 μM). This experimental result indirectly corroborated the applicability of the proposed workflow for accelerating the repurposing of FDA-approved drugs for COVID-19 treatment, thus pushing for the future experimental evaluation of the other drugs here identified.
In this context, velpatasvir is interestingly used in the treatment of Hepatitis C,57 another single-stranded, positive-sense RNA virus, and its use in the treatment of SARS-CoV-2 was already suggested,58–60 though focusing the attention on the main protease. This represents a further indication of the robustness of the methodology. Lomitapide, on the other hand, is a drug used in the homozygous familial hypercholesterolemia,61,62 and the outcomes of the proposed methodology suggest an off-label application of this molecule. The three-dimensional representations of the docking poses are shown in Fig. 3, and they represent the starting point for the subsequent experiments and evaluations. It can be noticed from Fig. 3, especially for the main protease, that molecular docking results are in line with what reported,52,63 showing interactions with Glu166, Gly143, and Thr45. Eventually, the ligand–protein complexes with the best ΔGbind were simulated to test their stability throughout time. To assess the stability of the complexes, three different parameters were computed: RMSD, RMSF, and the radius of gyration. These parameters reflect the overall mobility of the structures and can be used to confirm the initial hypothesis of a privileged binding between the three targets and the selected ligands. On a general note, for each complex, the displacement of the backbone atoms, side chains, and ligand structure from the initial position fell in the range of the ordinary biomolecules RMSD (Fig. 4). Interestingly, the papain-like protease/velpatasvir showed the lowest RMSD values both for the protein and the ligand, indicating a highly stable complex, likely due to the strong protein–ligand contacts and interactions, despite its complex and bulky structure.
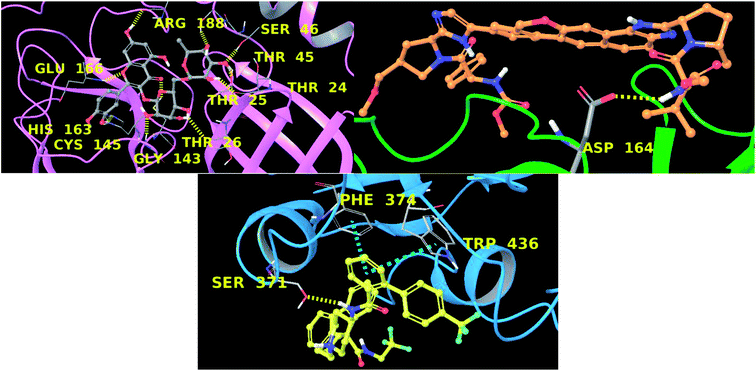 |
| Fig. 3 Three-dimensional representation main protease–rutin (up, left), papain-like protease–velpatasvir (up, right), and spike protein–lomitapide (down). Each protein is represented with ribbons (pink, green and cyan respectively) and ligands are in ball-and-stick. Hydrogen bonds are depicted as yellow dotted lines and π–π stacking as cyan dotted lines. Interacting residues are labelled in yellow. | |
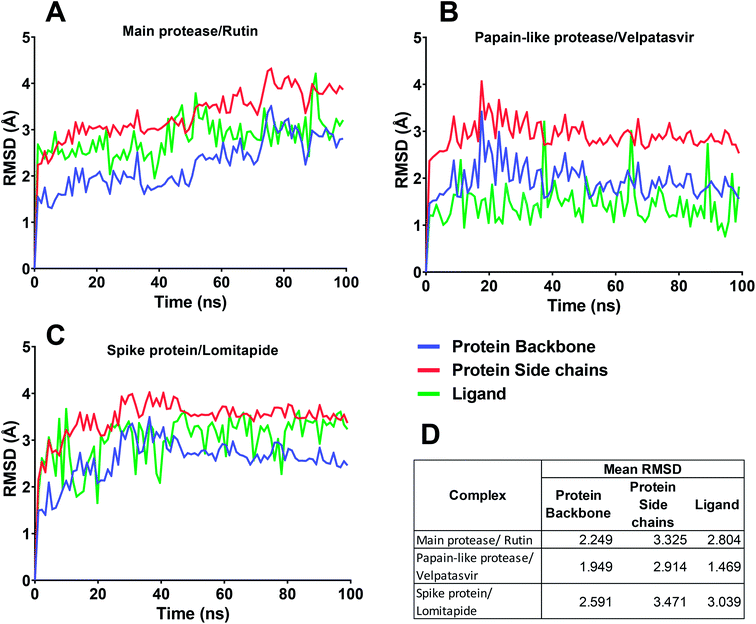 |
| Fig. 4 RMSD for the three protein–ligand complexes during the molecular dynamics simulations. (A–C) In each chart, the RMSD trend for protein backbone, protein side chains, and ligand are reported. (D) Mean RMSD value of the protein backbone, protein side chains, and ligand for the three complexes. | |
If we consider the RMSF trend, calculated averaging the backbone and the side chain values for each residue, it appeared small and within the normal range (Fig. 5). In detail, the main protease had no residue with mobility above 4 Å, indicating a high degree of stability. Concerning the papain-like protease, some of the residues (Cys192, Thr225, Cys226, and Gly227) featured a high degree of mobility, but none of them was involved in the ligand binding. The remaining amino acids showed very low RMSF values, corroborating the results obtained with the MM-GBSA and the RMSD analysis. Eventually, the spike protein showed a highly unstable fragment, from Ser477 to Glu484, which was not part of the binding site. We moved on considering the radius of gyration (Rg) distribution for each protein, which represents the change in protein ductility during the simulation (Fig. 6). The spike protein showed a peculiar trend with a curve characterized by two peaks, probably caused by the reduced dimensions of this system when compared to the other two. On the other hand, the distribution curve for the two proteases chains was narrow, indicating a uniform distribution of the measures around the mean value. The standard deviation reflected the behavior previously highlighted, with the main protease and the papain-like protease showing the most compact results. These data, thus, are in line with what previously stated on the relative stability of the complexes.
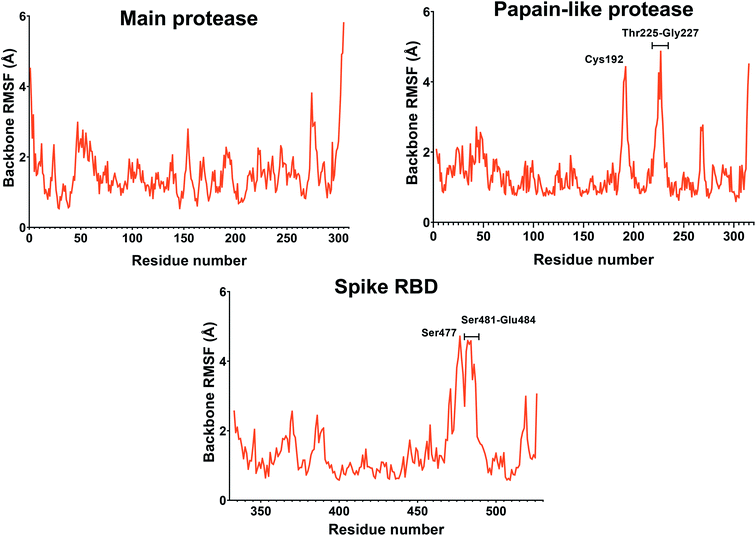 |
| Fig. 5 RMSF of the proteins during the simulation. The values reported representing the mean of the backbone and side chains RMSF values. Residues with values above 4 Å are labeled. | |
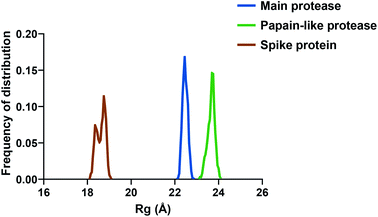 |
| Fig. 6 The radius of gyration (Rg) of the three proteins. | |
Concerning the protein–ligand interactions, they were evaluated throughout time (Fig. 7 and 8). The strength and the number of protein–ligand interactions followed the trend showed by the RMSD, RMSD, and Rg of the complexes. In detail, the main protease/rutin complex showed a high percentage of hydrogen bonds and water bridges due to its polar nature (Fig. 2 and 7) for almost the entire simulation; some residues, thus, were involved in interactions with the ligand for almost the 80% of the time (interactions fraction of ∼0.8). Velpatasvir established powerful interactions with Asp164 and Tyr264 that was kept stable during the simulation with an interactions fraction ≥ 1.0, due to the cumulative effect of each interaction type and the presence of two hydrogen bond acceptor on Asp164. These two residues were found to be relevant in stabilizing inhibitors in the SARS-CoV papain-like protease64 (Asp165 and Tyr265 in that paper), corroborating the idea of an interaction between the ligand and the target. The spike protein/lomitapide complex showed fewer and/or weaker interactions if compared to the other two systems, but, despite that, the residues involved in the binding are considered crucial for the interaction with the known human counterpart (ACE2 receptor),38,65 supporting the hypothesis of a good protein–ligand binding formulated based on the RMSD values. Overall, the MD simulations helped us confirming the data obtained with molecular docking, IVS experiments, and MM-GBSA, highlighting positive interactions between the selected molecules and the protein counterpart.
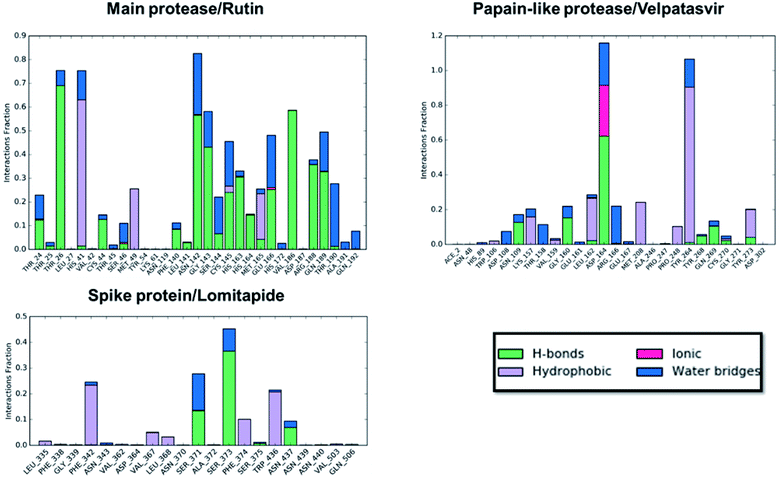 |
| Fig. 7 Protein–ligand interaction panels for the three ligand–protein selected complexes specifying the type of contacts detected. | |
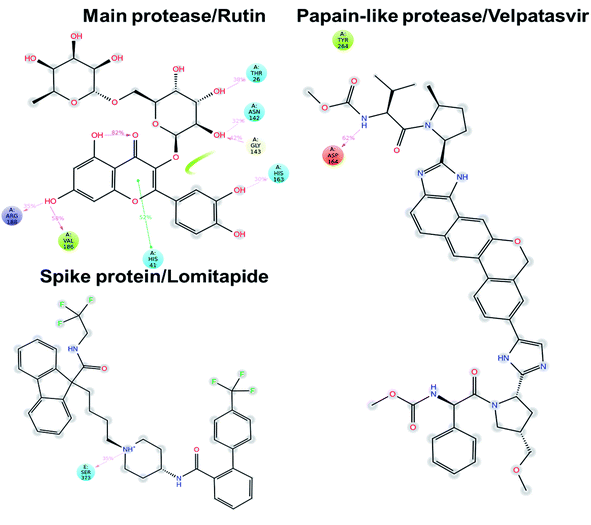 |
| Fig. 8 Protein–ligand interaction diagram detected during the molecular dynamics simulation. Interacting residues are labeled and the percentage of time is displayed for each interaction. Pink arrows represent hydrogen bond and the green lines the π–π stacking. | |
Conclusion
Summarizing, in this work a new computational protocol was proposed, combining classic Virtual Screening, Inverse Virtual Screening, MM-GBSA predictions, and molecular dynamics simulation, to carry out a repurposing campaign using FDA-approved drugs against COVID-19. We introduced a new parameter called IVSratio that can help to discriminate false-positive results and highlight new interacting compounds. The novelty is represented by the insertion of the Inverse Virtual Screening, a new and powerful method, to cross-check the results obtained from virtual screening. This step helps to limit the number of false-positive results and enhances the most promising bindings. In this way, we verified, using the IVSratio parameter, that the protein–ligand binding was still among the favorite ones even when the ligand was tested on multiple proteins. In this case, we focused our attention on three of the most important SARS-CoV-2 proteins: the main protease, the papain-like protease, and the spike protein, but this approach can be quickly re-iterated on further targets of interest. For each protein–ligand complex selected downstream, some results (e.g., rutin vs. main protease) are in line with recent outcomes, making the proposed screening scheme encouraging and giving a precious suggestion for further evaluations. Moreover, we would like to highlight the crucial role played by computational techniques in this emergency, which can provide valuable information in such a short time, helping the scientific community in the fight against this pandemic.
Conflicts of interest
There are no conflicts to declare.
Acknowledgements
The research leading to these results has received funding from AIRC under IG 2018-ID. 21397 project – P.I. Bifulco Giuseppe and MFAG 2017-ID. 20160 project – P.I. Lauro Gianluigi.
Notes and references
- A. R. Fehr and S. Perlman, in Coronaviruses: Methods and Protocols, ed. H. J. Maier, E. Bickerton and P. Britton, Springer New York, New York, NY, 2015, pp. 1–23 Search PubMed.
- W. Dai, B. Zhang, H. Su, J. Li, Y. Zhao, X. Xie, Z. Jin, F. Liu, C. Li, Y. Li, F. Bai, H. Wang, X. Cheng, X. Cen, S. Hu, X. Yang, J. Wang, X. Liu, G. Xiao, H. Jiang, Z. Rao, L.-K. Zhang, Y. Xu, H. Yang and H. Liu, Science, 2020, 368, 1331–1335 CrossRef CAS.
- P. Zhou, X.-L. Yang, X.-G. Wang, B. Hu, L. Zhang, W. Zhang, H.-R. Si, Y. Zhu, B. Li and C.-L. Huang, Nature, 2020, 579, 270–273 CrossRef CAS.
- F. Wu, S. Zhao, B. Yu, Y.-M. Chen, W. Wang, Z.-G. Song, Y. Hu, Z.-W. Tao, J.-H. Tian and Y.-Y. Pei, Nature, 2020, 579, 265–269 CrossRef CAS.
- R. Lu, X. Zhao, J. Li, P. Niu, B. Yang, H. Wu, W. Wang, H. Song, B. Huang and N. Zhu, Lancet, 2020, 395, 565–574 CrossRef CAS.
- N. Zhu, D. Zhang, W. Wang, X. Li, B. Yang, J. Song, X. Zhao, B. Huang, W. Shi and R. Lu, N. Engl. J. Med., 2020, 382, 727–733 CrossRef CAS.
- D. S. Hui, E. I. Azhar, T. A. Madani, F. Ntoumi, R. Kock, O. Dar, G. Ippolito, T. D. McHugh, Z. A. Memish and C. Drosten, Int. J. Infect. Dis., 2020, 91, 264–266 CrossRef CAS.
- Q. Li, X. Guan, P. Wu, X. Wang, L. Zhou, Y. Tong, R. Ren, K. S. M. Leung, E. H. Y. Lau and J. Y. Wong, N. Engl. J. Med., 2020, 382, 1199–1207 CrossRef CAS.
- J. F.-W. Chan, S. Yuan, K.-H. Kok, K. K.-W. To, H. Chu, J. Yang, F. Xing, J. Liu, C. C.-Y. Yip and R. W.-S. Poon, Lancet, 2020, 395, 514–523 CrossRef CAS.
- L. Nováková, J. Pavlík, L. Chrenková, O. Martinec and L. Červený, J. Pharm. Biomed. Anal., 2018, 147, 400–416 CrossRef.
- L. Nováková, J. Pavlík, L. Chrenková, O. Martinec and L. Červený, J. Pharm. Biomed. Anal., 2018, 147, 378–399 CrossRef.
- J. M. Sanders, M. L. Monogue, T. Z. Jodlowski and J. B. Cutrell, JAMA, J. Am. Med. Assoc., 2020, 323, 1824–1836 CrossRef CAS.
- S. Pushpakom, F. Iorio, P. A. Eyers, K. J. Escott, S. Hopper, A. Wells, A. Doig, T. Guilliams, J. Latimer, C. McNamee, A. Norris, P. Sanseau, D. Cavalla and M. Pirmohamed, Nat. Rev. Drug
Discovery, 2019, 18, 41–58 CrossRef CAS.
- V. Oldfield, S. Dhillon and G. L. Plosker, Drugs, 2009, 69, 609–632 CrossRef CAS.
- F. M. Buonaguro, I. Puzanov and P. A. Ascierto, J. Transl. Med., 2020, 18, 165 CrossRef CAS.
- P. Sang, S.-H. Tian, Z.-H. Meng and L.-Q. Yang, RSC Adv., 2020, 10, 15775–15783 RSC.
- H. M. Berman, J. Westbrook, Z. Feng, G. Gilliland, T. N. Bhat, H. Weissig, I. N. Shindyalov and P. E. Bourne, Nucleic Acids Res., 2000, 28, 235–242 CrossRef CAS.
- S. K. Burley, H. M. Berman, C. Bhikadiya, C. Bi, L. Chen, L. Di Costanzo, C. Christie, K. Dalenberg, J. M. Duarte, S. Dutta, Z. Feng, S. Ghosh, D. S. Goodsell, R. K. Green, V. Guranović, D. Guzenko, B. P. Hudson, T. Kalro, Y. Liang, R. Lowe, H. Namkoong, E. Peisach, I. Periskova, A. Prlić, C. Randle, A. Rose, P. Rose, R. Sala, M. Sekharan, C. Shao, L. Tan, Y.-P. Tao, Y. Valasatava, M. Voigt, J. Westbrook, J. Woo, H. Yang, J. Young, M. Zhuravleva and C. Zardecki, Nucleic Acids Res., 2018, 47, D464–D474 CrossRef.
- D. Sood, N. Kumar, A. Singh, V. Tomar, S. K. Dass and R. Chandra, ACS Omega, 2019, 4, 16233–16241 CrossRef CAS.
- N. Kumar, D. Sood, R. Tomar and R. Chandra, ACS Omega, 2019, 4, 21370–21380 CrossRef CAS.
- M. Yadav, S. Dhagat and J. S. Eswari, Eur. J. Pharm. Sci., 2020, 155, 105522 CrossRef CAS.
- Y. h. Taguchi and T. Turki, PLoS One, 2020, 15, e0238907 CrossRef CAS.
- N. Kumar, D. Sood, N. Sharma and R. Chandra, J. Chem. Inf. Model., 2020, 60, 421–433 CrossRef CAS.
- W. R. Ferraz, R. A. Gomes, A. L. S Novaes and G. H. Goulart Trossini, Future Med. Chem., 2020, 1815–1828 CrossRef CAS.
- L. Zhang, D. Lin, X. Sun, U. Curth, C. Drosten, L. Sauerhering, S. Becker, K. Rox and R. Hilgenfeld, Science, 2020, 368, 409–412 CrossRef CAS.
- T. Siebenmorgen and M. Zacharias, WIREs Computational Molecular Science, 2020, 10, e1448 CAS.
- J. Kim, J. Zhang, Y. Cha, S. Kolitz, J. Funt, R. Escalante Chong, S. Barrett, R. Kusko, B. Zeskind and H. Kaufman, J. Transl. Med., 2020, 18, 257 CrossRef CAS.
- V. Kairys, L. Baranauskiene, M. Kazlauskiene, D. Matulis and E. Kazlauskas, Expert Opin. Drug Discovery, 2019, 14, 755–768 CrossRef CAS.
- D. Gentile, V. Patamia, A. Scala, M. T. Sciortino, A. Piperno and A. Rescifina, Mar. Drugs, 2020, 18, 225 CrossRef CAS.
- O. O. Olubiyi, M. Olagunju, M. Keutmann, J. Loschwitz and B. Strodel, Molecules, 2020, 25, 3193 CrossRef CAS.
- N. Kumar, A. Awasthi, A. Kumari, D. Sood, P. Jain, T. Singh, N. Sharma, A. Grover and R. Chandra, J. Biomol. Struct. Dyn., 2020, 1–16 Search PubMed.
- N. Kumar, D. Sood, P. J. van der Spek, H. S. Sharma and R. Chandra, J. Proteome Res., 2020 DOI:10.1021/acs.jproteome.0c00367.
- S. Shahinshavali, K. A. Hossain, A. V. D. N. Kumar, A. G. Reddy, D. Kolli, A. Nakhi, M. V. B. Rao and M. Pal, Tetrahedron Lett., 2020, 61, 152336 CrossRef.
- A. K. Ghosh, M. Brindisi, D. Shahabi, M. E. Chapman and A. D. Mesecar, ChemMedChem, 2020, 15, 907–932 CrossRef CAS.
- R. Alexpandi, J. F. De Mesquita, S. K. Pandian and A. V. Ravi, Front. Microbiol., 2020, 11, 1796 CrossRef.
- M. Hagar, H. A. Ahmed, G. Aljohani and O. A. Alhaddad, Int. J. Mol. Sci., 2020, 21 Search PubMed.
- Z. Jin, X. Du, Y. Xu, Y. Deng, M. Liu, Y. Zhao, B. Zhang, X. Li, L. Zhang, C. Peng, Y. Duan, J. Yu, L. Wang, K. Yang, F. Liu, R. Jiang, X. Yang, T. You, X. Liu, X. Yang, F. Bai, H. Liu, X. Liu, L. W. Guddat, W. Xu, G. Xiao, C. Qin, Z. Shi, H. Jiang, Z. Rao and H. Yang, Nature, 2020, 582, 289–293 CrossRef CAS.
- J. Lan, J. Ge, J. Yu, S. Shan, H. Zhou, S. Fan, Q. Zhang, X. Shi, Q. Wang and L. Zhang, Nature, 2020, 581, 215–220 CrossRef CAS.
- J. Osipiuk, R. Jedrzejczak, C. Tesar, M. Endres, L. Stols, G. Babnigg, Y. Kim, K. Michalska and A. Joachimiak, (to be published), 2020, http://www.rcsb.org/structure/6W9C.
- S. De Vita, G. Lauro, D. Ruggiero, S. Terracciano, R. Riccio and G. Bifulco, J. Chem. Inf. Model., 2019, 59, 4678–4690 CrossRef CAS.
- G. Madhavi Sastry, M. Adzhigirey, T. Day, R. Annabhimoju and W. Sherman, J. Comput.-Aided Mol. Des., 2013, 27, 221–234 CrossRef CAS.
- Schrödinger Release 2020-1: Protein Preparation Wizard, Epik, Schrödinger, LLC, New York, NY, 2016, Impact, Schrödinger, LLC, New York, NY, 2016; Prime, Schrödinger, LLC 2020, New York, NY Search PubMed.
- T. A. Halgren, J. Chem. Inf. Model., 2009, 49, 377–389 CrossRef CAS.
- T. A. Halgren, Chem. Biol. Drug Des., 2007, 69, 146–148 CrossRef CAS.
- Schrödinger Release 2020-1, SiteMap, Schrödinger, LLC 2020, New York, NY Search PubMed.
- Schrödinger Release 2020-1, LigPrep, Schrödinger, LLC 2020, New York, NY Search PubMed.
- N. M. O'Boyle, M. Banck, C. A. James, C. Morley, T. Vandermeersch and G. R. Hutchison, J. Cheminf., 2011, 3, 33 Search PubMed.
- O. Trott and A. J. Olson, J. Comput. Chem., 2010, 31, 455–461 CAS.
- G. Lauro, M. Masullo, S. Piacente, R. Riccio and G. Bifulco, Bioorg. Med. Chem., 2012, 20, 3596–3602 CrossRef CAS.
- G. Lauro, A. Romano, R. Riccio and G. Bifulco, J. Nat. Prod., 2011, 74, 1401–1407 CrossRef CAS.
- F. M. Abd El-Mordy, M. M. El-Hamouly, M. T. Ibrahim, G. A. El-Rheem, O. M. Aly, A. M. Abd El-kader, K. A. Youssif and U. R. Abdelmohsen, RSC Adv., 2020, 10, 32148–32155 RSC.
- A. A. Al-Zahrani, Nat. Prod. Commun., 2020, 15, 1–4 CrossRef.
- M. T. Rehman, M. F. AlAjmi and A. Hussain, ChemRxiv, 2020 DOI:10.26434/chemrxiv.12362333.v2.
- A. Elmi, S. Al Jawad Sayem, M. Ahmed and F. Mohamed, ChemRxiv, 2020 DOI:10.26434/chemrxiv.12325844.v1.
- T. Huynh, H. Wang, W. Cornell and B. Luan, ChemRxiv, 2020 DOI:10.26434/chemrxiv.12281078.v1.
- O. Abian, D. Ortega-Alarcon, A. Jimenez-Alesanco, L. Ceballos-Laita, S. Vega, H. T. Reyburn, B. Rizzuti and A. Velazquez-Campoy, Int. J. Biol. Macromol., 2020, 164, 1693–1703 CrossRef CAS.
- S. L. Greig, Drugs, 2016, 76, 1567–1578 CrossRef CAS.
- Y. W. Chen, C.-P. B. Yiu and K.-Y. Wong, F1000Research, 2020, 9, 129 CAS.
- J. Ju, S. Kumar, X. Li, S. Jockusch and J. J. Russo, bioRxiv, 2020 DOI:10.1101/2020.01.30.927574.
- V. Mevada, P. Dudhagara, H. Gandhi, N. Vaghamshi, U. Beladiya and R. Patel, ChemRxiv, 2020 DOI:10.26434/chemrxiv.12115251.v2.
- D. Neef, H. K. Berthold and I. Gouni-Berthold, Expert Rev. Clin. Pharmacol., 2016, 9, 655–663 CrossRef CAS.
- X. Liu, P. Men, Y. Wang, S. Zhai, Z. Zhao and G. Liu, Am. J. Cardiovasc. Drugs, 2017, 17, 299–309 CrossRef CAS.
- S. T. Ngo, N. Quynh Anh Pham, L. Thi Le, D.-H. Pham and V. V. Vu, J. Chem. Inf. Model., 2020 DOI:10.1021/acs.jcim.0c00491.
- R. Chaudhuri, S. Tang, G. Zhao, H. Lu, D. A. Case and M. E. Johnson, J. Mol. Biol., 2011, 414, 272–288 CrossRef CAS.
- I. M. Ibrahim, D. H. Abdelmalek, M. E. Elshahat and A. A. Elfiky, J. Infect., 2020, 80, 554–562 CrossRef CAS.
Footnote |
† Electronic supplementary information (ESI) available: Document containing SMILES structure of the selected compounds and their docking score against the considered panel. See DOI: 10.1039/d0ra09010g |
|
This journal is © The Royal Society of Chemistry 2020 |
Click here to see how this site uses Cookies. View our privacy policy here.