DOI:
10.1039/D0SC02792H
(Edge Article)
Chem. Sci., 2020,
11, 8231-8239
High-content and high-throughput identification of macrophage polarization phenotypes†
Received
15th May 2020
, Accepted 17th July 2020
First published on 22nd July 2020
Abstract
Macrophages are plastic cells of the innate immune system that perform a wide range of immune- and homeostasis-related functions. Due to their plasticity, macrophages can polarize into a spectrum of activated phenotypes. Rapid identification of macrophage polarization states provides valuable information for drug discovery, toxicological screening, and immunotherapy evaluation. The complexity associated with macrophage activation limits the ability of current biomarker-based methods to rapidly identify unique activation states. In this study, we demonstrate the ability of a 2-element sensor array that provides an information-rich 5-channel output to successfully determine macrophage polarization phenotypes in a matter of minutes. The simple and robust sensor generates a high dimensional data array which enables accurate macrophage evaluations in standard cell lines and primary cells after cytokine treatment, as well as following exposure to a model disease environment.
Introduction
Macrophages are plastic leukocytes that perform a vast range of immune- and homeostasis-related functions, with their function and behavior dictated by environmental stimuli. Macrophages can be characterized as being activated into two major phenotypes, M1 and M2.1 M1 macrophages are associated with inflammation, including secretion of pro-inflammatory cytokines, engulfment of foreign entities, generation of reactive oxygen and nitrogen species, and assistance in T-helper type1 (Th1) cell responses to fight infection. Conversely, M2 macrophages perform anti-inflammatory and wound repair functions.2,3 Disturbance of the mechanisms that govern the balance of M1 and M2 states can result in a number of health problems, including infections, cancer, pregnancy complications, and inflammatory and autoimmune diseases.4,5 Given the significance and complexity of the roles macrophages play in biology and disease, knowledge of their activation and polarization state can provide critical information regarding the disease microenvironment, and be useful in selecting therapeutic approaches. For example, manipulation of tumor-associated macrophages (TAMs) provides a potential means to combat cancer. The tumor microenvironment releases factors that drive macrophages toward an M2-like phenotype,6 resulting in secretion of anti-inflammatory cytokines, promotion of tumor growth and invasion, and facilitation of metastases. Therapies are being developed to “re-educate” these TAMs from this immune-suppressing state to an antitumor M1 phenotype as a more effective, less toxic cancer treatment.7,8 The development of such entities would be facilitated by a means to evaluate macrophage characteristics in a straightforward and high-throughput manner.
Efforts to generate therapies based on macrophage phenotypic conversion (to stimulate immune activation or suppression) and evaluate macrophage immunes responses to other agents in drug discovery and toxicology are challenging due to the complexity of the polarization process. An increasing body of research reveals that macrophage polarization is more intricate than a two-state, M1/M2 conversion; rather, a spectrum of states exists.9–11 M2 macrophages can be further subclassified into M2a, M2b, M2c, among others, depending on the activating stimulus and resulting surface markers displayed.12 In addition, the macrophage polarization/sub-polarization process is dynamic and can evolve based on changes in the microenvironment.13–15 Complicating the matter further, macrophages can have mixed or overlapping M1 and M2-associated indicators. For instance, macrophages isolated from patients with advanced gastric and pancreatic cancers show high levels of both pro-inflammatory and anti-inflammatory cytokines. Both sets expressed IL-10 (M2-associated), while the former also had high levels of IL-12, and the latter IL-1β and TNF-α (M1 associated).16,17 These factors make it challenging to identify macrophage polarization states for diagnostic applications and fundamentally, to understand or identify phenotypes that are relevant to disease states.
Currently, the presence or levels of cellular and/or secreted biomarkers is most commonly used to detect and characterize macrophage polarization.12,18,19 While providing useful information, this approach is reliant on the specificity of the markers and requires multiple assays to obtain sufficient information for cellular evaluation. Additional limitations include: expression overlap between different polarization states (as mentioned above), poor phenotypic resolution of similar stimuli, non-translatable markers between mice and humans,12 and the fact that mRNA levels do not necessarily signal a robust difference in protein expression/at the functional level.19 In addition, the techniques used to identify the presence of biomarkers, such as RT-PCR, western blot, and flow cytometry, are expensive and not amenable to multiplexing or high-throughput applications. Thus, there is a strong need for a general high-throughput method that can be used to evaluate these cells and their characteristics to facilitate therapeutic design and understand phenotypic responses of macrophages to stimuli.
As an alternative to marker-specific approaches, chemical nose or array-based sensing employs and discerns selective interactions between analytes and sensor elements to generate unique patterns for each analyte. The resulting pattern can be further analyzed for quantitative classification. Once trained, the sensor can rapidly identify analytes based on pattern recognition. This approach has been successfully used in a wide range of systems including mammalian cells,20–22 bacteria,23–25 and proteins in biofluids.26–28 The strategy is ideal for cell phenotyping because changes in cellular responses yield variations in surface composition (e.g., protein, lipids, glycans, etc.) that result in different fingerprints, providing high-content information for each cellular state.29–31 Because macrophage polarization is accompanied by changes in cellular metabolism and surface protein expression,1,12,32 we hypothesized that an array-based sensing strategy would provide a general platform for discriminating macrophage phenotypic and sub-phenotypic states. Incorporation of this strategy into a multi-channel format would enable multidimensional, high-content output from a single microwell, rendering this method readily applicable to high-throughput screening.33
In this paper, we describe the development and application of a polymer–protein supramolecular assembly as a sensor array to gather high-throughput, high-content information on macrophage polarization state. The sensor is composed of only two elements: a guanidine-functionalized cationic poly(oxanorborneneimide) (PONI) polymer, and an anionic green fluorescent protein (GFP). The two entities form a complex through electrostatic interactions, resulting in a Förster resonance energy transfer (FRET) pair. When this sensor is applied to macrophages in different polarization/sub-polarization states, it yields fluorescent signals in five channels. The multidimensional output is then quantitatively analyzed using linear discriminant analysis (LDA) to reproducibly classify different macrophage activation states (Fig. 1). To the best of our knowledge, this combination of sensor elements resulting in a 5-channel output has not been reported previously. We validated the sensor with model macrophage RAW 264.7 cells and primary bone marrow-derived macrophages (BMDMs) stimulated with known M1 and M2 polarizing cytokines. The successful discrimination of M1 and M2 macrophages among the five subtypes demonstrates the ability of the sensor to accurately differentiate subtle phenotypic changes. We further evaluated the efficacy of the sensor system in a model disease environment, where macrophages were cultured in cancer cell-conditioned media, generating distinct patterns for macrophages exposed to different cancer types. Taken together, the sensor platform can classify macrophage phenotypes in a matter of minutes. Furthermore, this platform can read out the effects of subtle environmental changes on macrophages, providing a new tool for diagnostics and for fundamental studies of macrophage behavior. The information generated can provide valuable insights on macrophages in diseases, potentially improving efficiency of existing therapies and facilitating the development of new treatments.
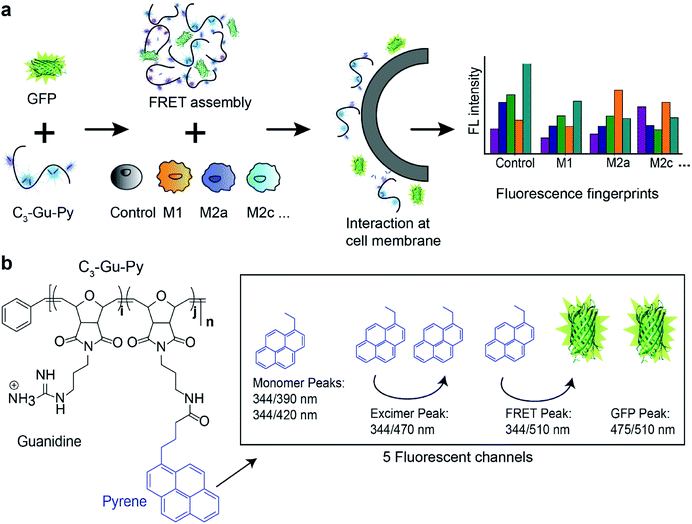 |
| Fig. 1 Schematic illustration of phenotyping macrophage activation states using array-based sensor. (a) FRET-based sensor assembly was formed between PONI–C3-guanidine-pyrene and GFP. Selective interactions of sensor elements at cell surface membrane resulted in fluorescence changes in all five channels, generating a distinct fingerprint for each cell activation state. (b) Chemical structure of PONI–C3-guanidine-pyrene and the resulting five fluorescence channels in the FRET complex. | |
Results
Supramolecular assembly of sensor
The sensor is designed to provide an information-rich, five-channel output with only two sensor elements. The first element of the sensor is a cationic poly(oxanorbornene) (PONI) random copolymer scaffold that incorporates a guanidine group and a pyrene dye molecule (C3-Gu-Py). The positively charged guanidine group ensures that selective interactions occur only when the complex is close to negatively charged cell surface functionalities. The solvatochromic pyrene molecule will alter its spectral properties when local environmental factors, such as polarity and hydrophobicity, change.34 In this way, both selectivity and sensitivity of the sensor are ensured. Through electrostatic interactions, cationic C3-Gu-Py forms a polymeric complex with an anionic GFP. In practice, the pyrene unit provides three signals, two corresponding to the free pyrene and one to the excimer form. The GFP then adds two channels: free GFP fluorescence and FRET with the excimer pyrene channel (Fig. 1b).
Initial studies focused on the optical characterization of the C3-Gu-Py/GFP supramolecular assembly. Polymer C3-Gu-Py was titrated with increasing concentrations of GFP. After 30 min of incubation, a simultaneous decrease in pyrene emission at 470 nm and increase of GFP emission at 510 nm was observed upon irradiation with 344 nm light (Fig. S1 and S2a†). Efficient fluorescence quenching of C3-Gu-Py was observed at higher concentrations of assembly (Fig. S2b†). The association constant Ka of 7.17 × 105 M−1 was derived by fitting the fluorescent titration curve.
The overall spectrum featured five distinguished output peaks that can be recorded from the sensor: pyrene monomers at 344/390 and 344/420, pyrene excimer at 344/470, GFP at 475/510, and FRET signal at 344/510. Based on the spectral flexibility, a concentration of 0.5 μM C3-Gu-Py and 50 nM GFP was selected for the following experiments. Dynamic light scattering data revealed the polymer assembly was ∼230 nm in diameter and the size slightly increased to ∼237 nm when GFP was added (Fig. S3†). Transmission electron microscopy images confirmed these results (Fig. S4†), indicating that a supramolecular assembly was formed between C3-Gu-Py and GFP.
Discrimination of M1 and M2 subtypes using RAW 264.7 cells
We first tested the ability of the sensor system to distinguish among macrophage phenotypes using the RAW 264.7 macrophage cell line. Established cytokines were used to stimulate the cells, with each activating macrophage through a different mechanism (Table 1), generating a distinct phenotypic state. RT-PCR results assessing standard M1 and M2 markers confirmed that cells were polarized into corresponding states after 48 h activation (Fig. 2). LPS and IFN-γ treated cells (M1 stimulation) showed significant increases in TNF-α and iNOS mRNA expression whereas the IL-4 (M2a stimulation) group had an increase in EGR2 and mannose receptor (MR) expression. Similar TNF-α levels observed between the combination treatment and the control group could be explained by the prolonged 48 h activation time, negative regulators such as NFκB and nuclear factor activated T cells,35,36 greater production of nitric oxide, and the fast intracellular turnover rate of TNF-α.37 Although the IL-10 group (M2c stimulation) was tested against multiple M2 markers, including EGR2, MR, and TGF-β, as well as the reduction of M1 marker iNOS, no significant changes in the levels of expression of any associated genes were observed (Fig. S7†).
Table 1 Mechanisms and effects of in vitro macrophage polarization of macrophages via different cytokines
Polarization stimulus |
Mechanism |
Surface marker change |
Resulting phenotype |
Lipopolysaccharide (LPS) |
Binds TLR 4, induces secretion of pro-inflammatory cytokines38 |
Increased expression levels of MHC-II, CD80, CD86; decreased levels of MRC1 or Fc-γ RII39 |
M1 |
Interferon-γ (IFN-γ) |
Binds IFN-γ receptor40 |
Combo (LPS + IFN-γ) |
Synergizes LPS and IFN-γ |
Interleukin 4 (IL-4) |
Binds IL-4Rα and IL-2R, down-regulates proinflammatory mediators1,32 |
Decreased expression of CD14 and CCR5;41 regulation of MHC-II, β2 integrins, chemokine CCL22/MDC42 |
M2a |
Interleukin 10 (IL-10) |
Deactivates macrophages by inhibiting production of pro-inflammatory cytokines1 |
Down-regulation of MHC II and co-stimulatory molecules1 |
M2c |
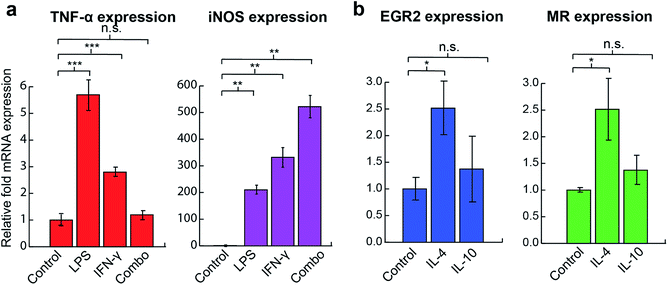 |
| Fig. 2 Macrophage activation confirmed by RT-PCR. (a) mRNA quantification of M1-associated genes, TNF-α and iNOS. (b) mRNA quantification of M2-associated genes, EGR2 and MR, according to treatment group. Control = non-treated cells, combo = LPS + IFN-γ treated. Fold changes in mRNA level were normalized to β-actin. Statistical significance was determined by two-tailed student t-test. * = p < 0.1, ** = p < 0.05, *** = p < 0.005, n = 3 biological replicates. n.s. = not significant. | |
Having confirmed that polarization had occurred, cells from each treatment group were plated on a 96-well microplate for overnight attachment. Equivalent cell numbers (10
000 per sample) were used to ensure that changes in sensor response were due to alterations in cell surface functionalities, not density. For the sensing process, C3-Gu-Py and GFP were premixed for 30 min to allow formation of stable FRET complexes. Subsequently, cells were washed once with phosphate buffered saline (PBS) and incubated with the sensor complex in the dark. Fluorescence signals were recorded every 15 min until equilibrium was reached. The 5-channel readout generated a distinct fluorescence pattern for each treatment group (Fig. 3a). We further utilized linear discriminate analysis (LDA) to test whether the five cell phenotypes could be robustly discriminated based upon their fluorescent signatures. As shown in Fig. 3b, the LDA plot revealed five distinct clusters for M1 and M2 subtypes with a correct classification of 100% (Tables S1 and S2†), demonstrating that each activation pathway resulted in a distinct cellular response. We further validated the reliability of the sensor by performing unknown sample identification and comparing the results against the training set. Among the 45 tested unknowns, 41 samples were predicted correctly into their corresponding group, giving a high percentage of correct unknown identification of 91% (Table S3†). The accuracy of unknown identification could be further improved by increasing the size and complexity of the training set if needed. Next, we investigated the necessity of having 5 channels of information from the sensor by comparing the performance of classification and unknown identification using either an individual sensor element or different combinations. The highest percentage of accuracy was achieved when all 5 channels were used, demonstrating the importance of multidimensional data in discriminating complex cell phenotypes (Fig. 3c).
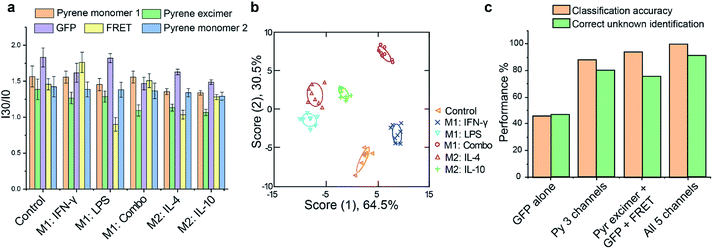 |
| Fig. 3 Discrimination of RAW 264.7 macrophages activated by M1 or M2 subtype stimuli using sensor complexes. (a) Fluorescence intensities of each treatment group were obtained at 30 min and normalized against sensor only. n = 8 biological replicates. (b) The fluorescence patterns were analyzed through linear discriminant analysis (LDA) and the first two canonical scores were plot-ted with 95% confidence ellipse (n = 8). (c) Correct classification percentage and unknown identification of M1 and M2 sub-types using different combinations of sensor channels. | |
Discrimination of M1 and M2 subtypes with primary macrophages
Immortalized macrophage cell lines provide a useful tool for assessing sensor response, however, these models differ in multiple aspects from their primary cell analogs. We next tested the sensor using physiologically relevant primary bone marrow-derived macrophages (BMDM). Progenitor cells were isolated from C57/B6 mice and induced to differentiate into macrophages using previously reported procedures.43 Once macrophage cells were obtained, we exposed them to M1 and M2 subtype polarization stimuli for 48 h as used above for RAW 264.7 cells. RT-PCR results confirmed appropriate activations in each case, with increases in TNF-α and iNOS mRNA expression for M1 related stimuli (LPS and/or IFN-γ) and EGR2 and MR mRNA levels for IL-4 stimulated M2 cells. Although IL-10 activation did not show substantial enhancement in EGR2 level, a nearly 6-fold increase in MR expression was observed (Fig. S8 and S9†). Following macrophage polarization, similar sensor procedure was performed. The fluorescence patterns observed were distinct from those of the RAW cells, consistent with differences that exist between the two cell models. Complete discrimination among the five assessed groups of M1 and M2 phenotypes was achieved with 96% correct classification (Fig. 4, Tables S4 and S5†). 92% of correct unknown identification confirmed the high reliability of our sensor (Table S6†).
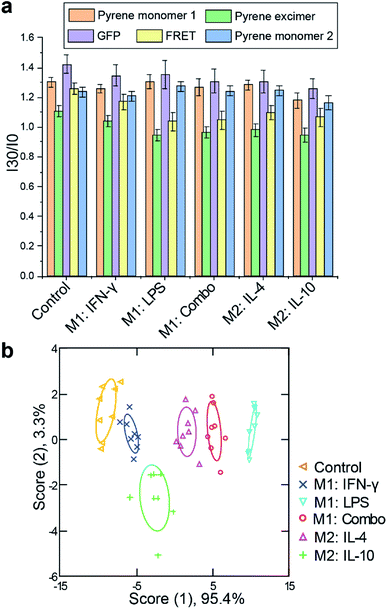 |
| Fig. 4 Discrimination of M1 and M2 subtypes of bone marrow-derived macrophages. (a) Fluorescence signals of the five sensor channels were obtained and normalized to sensor only. n = 8 biological replicates. (b) LDA plot of the first two canonical scores was plotted with 95% confidence ellipse (n = 8). | |
When the sensor complex interacts with cells, in most cases, all monitored fluorescence channels showed an increase in signal intensity. This suggests that upon interacting with macrophages, the sensor complex disaggregates, exposing its individual components to interact with the cell surface. Depending upon the local environment, the fluorescence intensities for individual molecules (pyrene, GFP, and FRET) also change. Since distinct fluorescence patterns were consistently observed for each stimulus, we believe this disruption process is modulated by cell surface functionalities and composition. Our previous studies have indicated that the sensor complex is highly sensitive to glycosylation patterns on cell surfaces.30 However, more mechanistic studies are needed in order to elucidate which other cell components are also interacting with the sensor elements.
Discrimination of macrophages exposed to conditioned media from different cancer cells
The above studies demonstrate that our sensor array was able to discriminate macrophages polarized with specific cytokines. However, biological microenvironments are often far more complex and have multiple stimuli. Hence, we assessed whether the sensor could discern macrophage phenotype in a model disease environment to address this issue. First, conditioned media was generated by culturing different types of cancer cells (HeLa, cervical carcinoma, and MCF7, mammary carcinoma) until ∼80% confluency was reached. Then, the culture media was extracted and used to stimulate macrophages for 48 h. RT-PCR results revealed different activation patterns for macrophages activated with media conditioned from different cell lines (Fig. S10†). C3-Gu-Py and GFP complexes were added to cells and the 5-channel fluorescence readouts were collected.
Distinct fluorescence signals were obtained for macrophages subjected to each of the conditioned media types. An LDA plot showed three well-separated clusters with 100% classification accuracy (Fig. 5, Tables S7 and S8†). When macrophages were exposed to cultured media conditioned by cervical cancer versus breast cancer cells, the sensing readout was dramatically different, indicating that a unique state of activation was present following each type of stimulation. A high percentage (96%) of correct unknown identification was also achieved (Table S9†). These results are exciting because it demonstrates that this method not only functions following single cytokine stimulation, but also in more complex environments. This is promising evidence that with careful evaluation, this sensing method could be applied to profile macrophages from individual patients, offering insights for precision medicine.
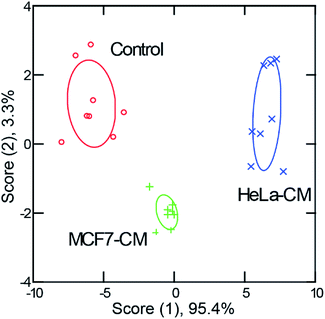 |
| Fig. 5 Discrimination of macrophage cells cultured under exposure to conditioned media from different cancer cell types for 48 h. The LDA plot of the first two canonical scores was obtained and plotted with 95% confidence ellipses (n = 8). CM is cancer cell conditioned medium, with the cell line type preceding it, control represent macrophages cultured using standard growth media. | |
Discussion
Macrophage polarization is a complex and dynamic process. With its roles in homeostasis and disease, it is important to be able to discern macrophages characteristics in a rapid and straight-forward manner. Compared with current methods of characterizing macrophage polarization, the sensor reported in this study has advantages of generating a multidimensional and high-content chemical readout regarding the cell surface in a high-throughput matter. Standard methods, such as RT-PCR and ELISA, can only capture a limited number of well-established markers for each cell activation state, and are independent (not multiplexed) assays, requiring a separate analysis for each. Considering the heterogeneity of macrophage polarization and the overlapping nature of M1 and M2 markers,11,12 it is also difficult to elucidate and differentiate activation states with standard methods. For instance, the multiple IL-10 markers used in our RT-PCR studies did not reveal significant changes. The ambiguity of a less-well characterized sub-phenotype could be because the end-point evaluation missed the dynamic changes on the macrophage marker expression during the 48 h activation.
In contrast, the array-based sensor utilizes selective interactions between sensor elements and the entire analyte surface to generate high-content fingerprints for each activation state. Once trained, the sensor can rapidly identify target analytes through pattern recognition. Although the C3-Gu-Py moiety has been utilized for bacterial sensing,44 its capability in mammalian cell sensing has not been investigated. By coupling the polymer with simple GFP through supramolecular interactions, sensor can discern less-characterized sub-phenotypes, such as IL-10 stimulated macrophages, which are challenging to identify using traditional methods like RT-PCR (Fig. 3 and 4). The 5-channel, high-content information gathered from the sensor is crucial in achieving a high level of classification accuracy and it allows us to address challenging biological questions from a chemical perspective. In addition, running assays like RT-PCR and ELISA can be time-consuming and error prone, with relatively high costs for thorough characterizations consisting of multiple markers. In contrast, the sensor material used here is synthetically easy to generate, and all components can be mixed in one microplate well, which not only reduces sensor material but is also compatible for high-throughput screening applications. What is more, accurate phenotyping can be obtained in less than an hour, making this method simple, robust, and rapid.
Due to the robust and facile nature of the system, there are many potential applications for the array-based sensing strategy. Altered immune states are a major factor in diseases including cancer, atherosclerosis, and auto-immune disorders.45–47 Macrophage polarization states are key in driving forward disease progression. Rapid assessment of their activation states can provide valuable information in selecting appropriate therapeutic strategies.48,49 Notably, the high-throughput nature of the method would facilitate the rapid screening of immune states for individual patients, enabling personalized medicinal approaches in tackling these immune-driven diseases. Furthermore, this strategy could be applied to other plastic immune cells, such as dendritic cells and T cells.50,51 By extending this sensor to other cell types, the status of major components of the immune system could be rapidly determined. This strategy can also greatly improve the drug discovery process, by allowing for rapid identification of altered cell states, and/or evaluation of immunogenicity following agent treatment.52 Potential immune adjuvants or anti-inflammatory entities could be screened together by using the sensor on immune cells in a multi-well plate format. With these capabilities, the sensor system not only has utility as a fundamental research tool, but as a high-throughput, high-content means for therapeutic screening against general plastic cell types.
Conclusions
In summary, we demonstrate the use of a simple and robust chemical system that can quickly capture the overall responses of activated macrophages in a high-throughput format, which is challenging with biomolecular tools. Through the supramolecular assembly of only two elements, a 5-channel output is achieved. The high level of information density enables us to accurately profile a spectrum of activation state of macrophages. The ability to use chemical entities to answer biological questions opens the doors for sensing and beyond.
Experimental
Materials
All reagents were purchased from Thermo-Fisher Scientific except where otherwise noted. All DMSO utilized was cell culture grade (Sigma). RT-PCR data was generated using a CFX Connect Real-Time PCR Detection System (Biorad, Hercules, CA). For assays requiring absorbance and fluorescence measurements, a SpectraMax M2 plate reader was used (Molecular Devices, San Jose, CA).
PONI–C3-guanidine polymer synthesis
Monomers and polymers were synthesized according to previous reports.53 Detailed synthetic scheme can be found in the ESI.†
Green fluorescent protein expression
GFP was constructed and characterized according to reported protocols.54 In short, Escherichia coli strain BL21 was transformed with plasmids containing GFP recombinant protein. After transformation and induction with IPTG, cells were lysed and purified by Co2+ nitrilotriacetate columns. Fluorescent proteins were further characterized by SDS-PAGE gel, scanning absorbance and emission spectrum. The results are consistent with previously reported work.30
Transmission electron microscopy (TEM)
TEM samples were prepared by either 0.5 μM of C3-Gu-Py only or mixing 0.5 μM of C3-Gu-Py with 50 nM of GFP in 10 mM HEPES buffer for 30 min in dark at room temperature. 5 μL of the solutions were then placed on 300 mesh copper grids (with formvar films) obtained from Electron Microscopy Sciences (EMS FF300-Cu) and allowed to dry overnight. The samples were analyzed using a TEM JOEL 2000FX at an acceleration voltage of 200 kV.
Fluorescent titration
0.5 μM C3-Gu-Py polymer was titrated with GFP at a concentration range from 0 to 200 μM in a black 96 well-microplate. The solution was mixed in 10 mM HEPEs buffer. After 30 min incubation at room temperature in dark, the fluorescence spectrum was measured at an excitation wavelength of 344 nm.
Binding affinity calculation
Fluorescence titration was utilized to calculate the binding affinity of the C3-Gu-Py polymer with GFP. The fluorescence decay of the C3-Gu-Py excimer as a function of GFP concentration was fitted to a one-site binding equation,55 which is:
where I is the fluorescence intensity of C3-Gu-Py excimer at a given concentration of GFP, I0 is the fluorescence intensity of C3-Gu-Py in the absence of GFP, Ilim is the fluorescence intensity when the quenching reaches a plateau, C0 refers to the concentration of C3-Gu-Py, and C is the concentration of GFP. Based on the equation, microscopic binding constant Ka was determined by using the non-linear least-squares curve fitting analysis in OriginPro (OriginLab Co., Northampton, USA).
Linear discriminant analysis (LDA)
LDA was applied on normalized fluorescence data to statistically classify each group, using SYSTAT software (version 11.0, SystatSoftware, Richmond, CA, U.S.A.). All variables were used in the complete mode and the tolerance was set as 0.001. Input data was transformed to canonical scores to best separate each group where the between-class variance was maximized while the within-class variance was minimized. After transformation, LDA reduces the dimensionality of the. The 2D plot pictorially shows where each data point lies in the new dimensional space. Therefore, the positive and negative values on the axis do not have any physiologically meaning.
Unknown identification
The identity of unknown samples was predicted by computing the Mahalanobis distance of the unknown data to the training groups using LDA.56 First, the normalized fluorescence responses of the unknown samples were converted to canonical scores in LDA, using the discriminant functions established from the reference set. Next, Mahalanobis distance of that case to the centroid of each training cluster in the LDA space was computed.56,57 The unknown sample was predicted to belong to the closest group, defined by the shortest Mahalanobis distance.
Cell culture
RAW 264.7 cells, HeLa and MCF7 cell lines were purchased from American Type Culture Collection (ATCC, Manassas, VA). Primary bone marrow derived macrophages (BMDMs) were isolated from freshly euthanized C57/B6 mice, donated generously by Dr Jessie Mager, Department of Veterinary and Animal Science, University of Massachusetts Amherst. The BMDMs were isolated, differentiated and cultured according to previously reported methods.43 All cells were cultured at 37 °C under a humidified atmosphere containing 5% CO2 using standard growth media consisted of high glucose Dulbecco's Modified Eagle Medium (DMEM) supplemented with 10% fetal bovine serum (FBS) and 1% antibiotics (100 μg mL−1 penicillin and 100 μg mL−1 streptomycin). Under the above culture conditions, the cells were sub-cultured approximately once every two to five days.
Macrophage polarization via activation agents
Both RAW 264.7 cells and BMDMs were treated with the following polarization stimuli for 48 h to induce the desired polarization state. LPS group: 50 ng mL−1, IFN-γ group: 50 ng mL−1, combo group: 50 ng mL−1 LPS and IFN-γ, IL-4 group: 30 ng mL−1, and IL-10 group: 30 ng mL−1. After 2 day polarization, cells were washed with PBS, trypsinized, and plated as 10
000 cells per well on a 96-well plate overnight before proceeding to sensing studies.
Macrophage polarization via cancer cell conditioned media
HeLa and MCF7 cell lines were cultured under DMEM medium supplemented with 10% FBS and 1% antibiotics for 2 days to reach above 80% confluency. The supernatant from each cell line was then collected and centrifuged for 5 minutes. Subsequently, 5 mL of the supernatant was transferred into a T25 culture flask containing RAW cells. After 48 h of culture, RAW 264.7 cells were washed with PBS, trypsinized and plated as 10
000 cells per well on a 96-well plate for overnight attachment.
Sensing studies
The sensor was prepared by mixing 0.5 μM of C3-Gu-Py with 50 nM of GFP in 10 mM HEPES buffer for 30 minutes in dark at room temperature. Subsequently, 150 μL of sensor solution was incubated with and without the cell populations (washed once with PBS) in 96-well microplates. The change in fluorescence intensity for each channel was recorded every 15 minutes at its respective wavelength (pyrene monomer: 344/390 nm and 344/420 nm, pyrene excimer: 344/470 nm, GFP: 475/510 nm, FRET: 344/510 nm) on a Molecular Devices SpectraMax M2 microplate reader using appropriate filters.
RT-PCR preparation
Cells were plated in 24-well plates at a density of 50
000 cells/well. Cells were treated with the appropriate polarization stimulus for 48 h. Following treatments, RNA was extracted following the procedure below.
RNA extraction and cDNA conversion
Approximately 1.5 μg RNA was harvested using the PureLink RNA Mini Kit (Ambion) following the manufacturer's instructions. SuperScript IV Reverse Transcriptase was used for the conversion of approximately 150 ng of RNA to cDNA, along with RNaseOut, 10 mM dNTPs, and 50 μM Random Hexamers (ThermoFisher, Pittsburgh, PA), also following the manufacturer's instructions.
RT-PCR preparation
Cells were plated in 24-well plates at a density of 50
000 cells per well. Cells were treated with the appropriate polarization stimulus for 48 h. Following treatments, RNA was extracted following the procedure below.
Quantitative RT-PCR
RT-PCR was performed on cDNA as prepared above using a CFX connect real-time system with iTaq Universal SYBR Green Supermix (Biorad, Hercules, CA). All DNA primers were purchased from Integrated DNA Technologies (Caralville, Iowa). The following primer sequences were used:
β-Actin (forward) GATCAGCAAGCAGGAGTACGA,
β-Actin (reverse) AAAACGCAGCGCAGTAACAGT;
iNOS (forward) GTTCTCAGCCCAACAATACAAGA,
iNOS (reverse) GTGGACGGGTCGATGTCAC;
TNF-α (forward) CCTGTAGCCCACGTCGTAG,
TNF-α (reverse) GGGAGTCAAGGTACAACCC;
EGR2 (forward) TGAGAGAGCAGCGATTGATT,
EGR2 (reverse) ATAACAGTCAGTGTGTCCCC;
Mannose receptor (forward) GGATGTTGATGGCTACTGGA,
Mannose receptor (reverse) AGTAGCAGGGATTTCGTCTG;
TGF-β (forward) GCGGACTACTATGCTAAAGA,
TGF-β (reverse) TTCTCATAGATGGCGTTGTT.
Analyses were performed as follows: the samples were first activated at 50 °C for 2 min, then 95 °C for 2 min. Then denaturing occurred at 95 °C for 30 s followed by annealing at 57 °C; the denature/anneal process was repeated over 40 cycles. Relative gene expression was determined by comparing the Ct value of the gene of interest to that of the β-actin housekeeping gene, by the 2ΔΔCt method.58 Three biological replicates were performed for each control group and three technical replicates were used for each biological replicate.
Conflicts of interest
There are no conflicts to declare.
Acknowledgements
V. M. R. acknowledges support from the NIH (DK121351 and EB022641) grant. Y. G. and J. A. M. were partially supported by a fellowship from the University of Massachusetts as part of the Chemistry-Biology Interface Training Program (National Research Service Award (T32 GM008515)) from the National Institutes of Health.
Notes and references
- F. O. Martinez, A. Sica, A. Mantovani and M. Locati, Front. Biosci., 2008, 13, 453–461 CrossRef CAS PubMed.
- P. J. Murray, J. E. Allen, S. K. Biswas, E. A. Fisher, D. W. Gilroy, S. Goerdt, S. Gordon, J. A. Hamilton, L. B. Ivashkiv, T. Lawrence, M. Locati, A. Mantovani, F. O. Martinez, J.-L. Mege, D. M. Mosser, G. Natoli, J. P. Saeij, J. L. Schultze, K. A. Shirey, A. Sica, J. Suttles, I. Udalova, J. A. van Ginderachter, S. N. Vogel and T. A. Wynn, Immunity, 2014, 41, 14–20 CrossRef CAS PubMed.
- F. O. Martinez and S. Gordon, F1000Prime Rep., 2014, 6, 13 Search PubMed.
- A. Shapouri-Moghaddam, S. Mohammadian, H. Vazini, M. Taghadosi, S.-A. Esmaeili, F. Mardani, B. Seifi, A. Mohammadi, J. T. Afshari and A. Sahebkar, J. Cell. Physiol., 2018, 233, 6425–6440 CrossRef CAS PubMed.
- Y.-C. Liu, X.-B. Zou, Y.-F. Chai and Y.-M. Yao, Int. J. Biol. Sci., 2014, 10, 520–529 CrossRef PubMed.
- R. Ostuni, F. Kratochvill, P. J. Murray and G. Natoli, Trends Immunol., 2015, 36, 229–239 CrossRef CAS PubMed.
- C. Belgiovine, M. D'Incalci, P. Allavena and R. Frapolli, Cell. Mol. Life Sci., 2016, 73, 2411–2424 CrossRef CAS PubMed.
- M. Wenes, M. Shang, M. Di Matteo, J. Goveia, R. Martín-Pérez, J. Serneels, H. Prenen, B. Ghesquière, P. Carmeliet and M. Mazzone, Cell Metab., 2016, 24, 701–715 CrossRef CAS PubMed.
- J. Xue, S. V. Schmidt, J. Sander, A. Draffehn, W. Krebs, I. Quester, D. De Nardo, T. D. Gohel, M. Emde, L. Schmidleithner, H. Ganesan, A. Nino-Castro, M. R. Mallmann, L. Labzin, H. Theis, M. Kraut, M. Beyer, E. Latz, T. C. Freeman, T. Ulas and J. L. Schultze, Immunity, 2014, 40, 274–288 CrossRef CAS PubMed.
- R. M. Ransohoff, Nat. Neurosci., 2016, 19, 987–991 CrossRef CAS PubMed.
- B.-Z. Qian and J. W. Pollard, Cell, 2010, 141, 39–51 CrossRef CAS PubMed.
- A. Mantovani, A. Sica, S. Sozzani, P. Allavena, A. Vecchi and M. Locati, Trends Immunol., 2004, 25, 677–686 CrossRef CAS PubMed.
- E. Mathew, A. L. Brannon, A. C. Del Vecchio, P. E. Garcia, M. K. Penny, K. T. Kane, A. Vinta, R. J. Buckanovich and M. P. di Magliano, Neoplasia, 2016, 18, 142–151 CrossRef CAS PubMed.
- C. Auffray, D. Fogg, M. Garfa, G. Elain, O. Join-Lambert, S. Kayal, S. Sarnacki, A. Cumano, G. Lauvau and F. Geissmann, Science, 2007, 317, 666–670 CrossRef CAS PubMed.
- A. Fernandez, M. Vermeren, D. Humphries, R. Subiros-Funosas, N. Barth, L. Campana, A. MacKinnon, Y. Feng and M. Vendrell, ACS Cent. Sci., 2017, 3, 995–1005 CrossRef CAS PubMed.
- H. Sugai, K. Kono, A. Takahashi, F. Ichihara, H. Kawaida, H. Fujii and Y. Matsumoto, J. Surg. Res., 2004, 116, 277–287 CrossRef CAS PubMed.
- O. Helm, J. Held-Feindt, E. Grage-Griebenow, N. Reiling, H. Ungefroren, I. Vogel, U. Krüger, T. Becker, M. Ebsen, C. Röcken, D. Kabelitz, H. Schäfer and S. Sebens, Int. J. Cancer, 2014, 135, 843–861 CrossRef CAS PubMed.
- G. A. Duque and A. Descoteaux, Front. Immunol., 2014, 5, 491 Search PubMed.
- C. A. Ambarus, S. Krausz, M. van Eijk, J. Hamann, T. R. D. J. Radstake, K. A. Reedquist, P. P. Tak and D. L. P. Baeten, J. Immunol. Methods, 2012, 375, 196–206 CrossRef CAS PubMed.
- Y. Geng, H. L. Goel, N. B. Le, T. Yoshii, R. Mout, G. Y. Tonga, J. J. Amante, A. M. Mercurio and V. M. Rotello, Nanomed. Nanotechnol. Biol. Med., 2018, 14, 1931–1939 CrossRef CAS PubMed.
- L. Wu, H. Ji, Y. Guan, X. Ran, J. Ren and X. Qu, NPG Asia Mater., 2017, 9, e356 CrossRef CAS.
- X. Yang, J. Li, H. Pei, Y. Zhao, X. Zuo, C. Fan and Q. Huang, Anal. Chem., 2014, 86, 3227–3231 CrossRef CAS PubMed.
- X. Li, H. Kong, R. Mout, K. Saha, D. F. Moyano, S. M. Robinson, S. Rana, X. Zhang, M. A. Riley and V. M. Rotello, ACS Nano, 2014, 8, 12014–12019 CrossRef CAS PubMed.
- J. R. Carey, K. S. Suslick, K. I. Hulkower, J. A. Imlay, K. R. C. Imlay, C. K. Ingison, J. B. Ponder, A. Sen and A. E. Wittrig, J. Am. Chem. Soc., 2011, 133, 7571–7576 CrossRef CAS PubMed.
- Y. Tao, X. Ran, J. Ren and X. Qu, Small, 2014, 10, 3667–3671 CrossRef CAS PubMed.
- Z. Pode, R. Peri-Naor, J. M. Georgeson, T. Ilani, V. Kiss, T. Unger, B. Markus, H. M. Barr, L. Motiei and D. Margulies, Nat. Nanotechnol., 2017, 12, 1161–1168 CrossRef CAS PubMed.
- D. Zamora-Olivares, T. S. Kaoud, K. N. Dalby and E. V. Anslyn, J. Am. Chem. Soc., 2013, 135, 14814–14820 CrossRef CAS PubMed.
- L. Motiei, Z. Pode, A. Koganitsky and D. Margulies, Angew. Chem. Int. Ed., 2014, 53, 9289–9293 CrossRef CAS PubMed.
- Y. Tao, M. Li and D. T. Auguste, Biomaterials, 2017, 116, 21–33 CrossRef CAS PubMed.
- S. Rana, N. D. B. Le, R. Mout, B. Duncan, S. G. Elci, K. Saha and V. M. Rotello, ACS Cent. Sci., 2015, 1, 191–197 CrossRef CAS PubMed.
- Y. Geng, W. J. Peveler and V. M. Rotello, Angew. Chem. Int. Ed., 2019, 58, 5190–5200 CrossRef CAS PubMed.
- F. O. Martinez, L. Helming and S. Gordon, Annu. Rev. Immunol., 2009, 27, 451–483 CrossRef CAS PubMed.
- S. Rana, N. D. B. Le, R. Mout, K. Saha, G. Y. Tonga, R. E. S. Bain, O. R. Miranda, C. M. Rotello and V. M. Rotello, Nat. Nanotechnol., 2015, 10, 65–69 CrossRef CAS PubMed.
- A. S. Klymchenko, Acc. Chem. Res., 2017, 50, 366–375 CrossRef CAS PubMed.
- H. T. Idriss and J. H. Naismith, Microsc. Res. Tech., 2000, 50, 184–195 CrossRef CAS PubMed.
- N. Parameswaran and S. Patial, Crit. Rev. Eukaryot. Gene Expr., 2010, 20, 87–103 CrossRef CAS PubMed.
- F. Held, E. Hoppe, M. Cvijovic, M. Jirstrand and J. Gabrielsson, J. Pharmacokinet. Pharmacodyn., 2019, 46, 223–240 CrossRef CAS PubMed.
- J. Wan, Y. Shan, Y. Fan, C. Fan, S. Chen, J. Sun, L. Zhu, L. Qin, M. Yu and Z. Lin, Mol. Med. Rep., 2016, 14, 4505–4510 CrossRef CAS PubMed.
- U. Boehm, T. Klamp, M. Groot and J. C. Howard, Annu. Rev. Immunol., 1997, 15, 749–795 CrossRef CAS PubMed.
- K. Schroder, P. J. Hertzog, T. Ravasi and D. A. Hume, J. Leukoc. Biol., 2004, 75, 163–189 CrossRef CAS PubMed.
- J. Wang, G. Roderiquez, T. Oravecz and M. A. Norcross, J. Virol., 1998, 72, 7642–7647 CrossRef CAS PubMed.
- A. Mantovani, S. Sozzani, M. Locati, P. Allavena and A. Sica, Trends Immunol., 2002, 23, 549–555 CrossRef CAS PubMed.
- J. Weischenfeldt and B. Porse, Cold Spring Harb. Protoc., 2008, 3, pdb.prot5080 CrossRef PubMed.
- S. Ngernpimai, Y. Geng, J. M. Makabenta, R. F. Landis, P. Keshri, A. Gupta, C. H. Li, A. Chompoosor and V. M. Rotello, ACS Appl. Mater. Interfaces, 2019, 11, 11202–11208 CrossRef CAS PubMed.
- E. Elinav, R. Nowarski, C. A. Thaiss, B. Hu, C. Jin and R. A. Flavell, Nat. Rev. Cancer, 2013, 13, 759–771 CrossRef CAS PubMed.
- A. Gisterå and G. K. Hansson, Nat. Rev. Nephrol., 2017, 13, 368–380 CrossRef PubMed.
- I. B. McInnes, C. D. Buckley and J. D. Isaacs, Nat. Rev. Rheumatol., 2016, 12, 63–68 CrossRef CAS PubMed.
- M. Benoit, B. Desnues and J.-L. Mege, J. Immunol., 2008, 181, 3733–3739 CrossRef CAS PubMed.
- C. N. Lumeng, J. L. Bodzin, A. R. Saltiel, C. N. Lumeng, J. L. Bodzin and A. R. Saltiel, J. Clin. Invest., 2007, 117, 175–184 CrossRef CAS PubMed.
- L. Zhou, M. M. W. Chong and D. R. Littman, Immunity, 2009, 30, 646–655 CrossRef CAS PubMed.
- S. J. Galli, N. Borregaard and T. A. Wynn, Nat. Immunol., 2011, 12, 1035–1044 CrossRef CAS PubMed.
- Q. Xu, J. T. Norman, S. Shrivastav, J. Lucio-Cazana and J. B. Kopp, Am. J. Physiol. Physiol., 2007, 293, F631–F640 CrossRef CAS PubMed.
- R. F. Landis, A. Gupta, Y. W. Lee, L. S. Wang, B. Golba, B. Couillaud, R. Ridolfo, R. Das and V. M. Rotello, ACS Nano, 2017, 11, 946–952 CrossRef CAS PubMed.
- M. De, S. Rana and V. M. Rotello, Macromol. Biosci., 2009, 9, 174–178 CrossRef CAS PubMed.
- B. Valeur, J. Pouget, J. Bourson, M. Kaschke and N. P. Ernsting, J. Phys. Chem., 1992, 96, 6545–6549 CrossRef CAS.
- P. C. Mahalanobis, Proc. Natl. Inst. Sci. India, 1936, 2, 49–55 Search PubMed.
- R. Gnanadesikan and J. R. Kettenring, Biometrics, 2006, 28, 81 CrossRef.
- T. D. Schmittgen and K. J. Livak, Nat. Protoc., 2008, 3, 1101–1108 CrossRef CAS PubMed.
Footnotes |
† Electronic supplementary information (ESI) available. See DOI: 10.1039/d0sc02792h |
‡ Y. G. and J. H. contributed equally to this work. |
§ Present address: State Key Laboratory of Food Science and Technology, School of Food Science of Jiangnan University, Jiangsu, China. |
|
This journal is © The Royal Society of Chemistry 2020 |
Click here to see how this site uses Cookies. View our privacy policy here.