Molecular insights into the Patched1 drug efflux inhibitory activity of panicein A hydroquinone: a computational study†
Received
2nd November 2020
, Accepted 4th January 2021
First published on 1st February 2021
Abstract
Human Hedgehog receptor Patched1 (PTCH1) is able to efflux chemotherapeutics of different chemical structure out of cancer cells thus contributing to multidrug resistance phenomena in tumor treatment. A screening of natural compounds purified from marine sponges led to the identification of the first PTCH1 efflux inhibitor, panicein A hydroquinone (PAH), demonstrated to increase doxorubicin toxicity in vitro and vemurafenib toxicity in vitro and in vivo. In this work we combined different computational techniques to gain molecular insights of the inhibitory activity of PAH and some of its active and inactive analogues. We first performed a thorough characterization and druggability analysis of the main putative substrate binding pockets known from available cryo-electron microscopy structures. Further, dynamical descriptors of the active and inactive PAH analogues were extracted from microsecond-long all-atom molecular dynamics simulations in water solution. Finally, a blind ensemble docking methodology coupled with the conformational analysis of compounds enabled rationalization of the interaction between PTCH1 and PAH and derivatives in terms of their intrinsic physico-chemical properties. Our results suggest that the Neck pocket is the preferential binding site for PAH analogues on PTCH1, and that compounds assuming an open cylindric-like shape in solution are most likely to be good binders for PTCH1.
Introduction
In the context of Multi Drug Resistance (MDR), the overexpression of membrane multidrug transporters, namely ATP Binding Cassette (ABC) transporters, is one of the main reasons of failure in clinical cancer treatment.1 Despite the efforts to develop small molecule inhibitors of these efflux systems, to date none of the proposed compounds has been approved by the Food and Drug Administration, due to severe side effects mainly related to the ubiquitous localization of these transporters.1
The Hedgehog receptor Patched1 (PTCH1) regulates the Hedgehog signaling pathway involved in tissue development and differentiation during embryogenesis, and tissue regeneration in adults.2 In this complex regulation system, PTCH1 prevents the activation of Smoothened (SMO). PTCH1 was shown to efflux cholesterol thus inducing a decrease of its concentration necessary for SMO stabilization at the plasma membrane.3 However, the details of the process are yet to be elucidated. To support this hypothesis, PTCH1 shares homology with a number of membrane-embedded proteins involved in cholesterol homeostasis, such as HMG-CoA reductase (HMGR), the rate-controlling enzyme in cholesterol biosynthesis, SCAP, an escort protein for sterol regulatory element binding proteins (SREBPs) and Niemann–Pick disease type C1 (NPC1).4
Several cryo-EM structures of PTCH1, alone and in complex with Sonic Hedgehog (SHH), are available, classifying5–11 PTCH1 as a transmembrane protein containing a 12 helices transmembrane domain (TM) and two extra-cellular domains, namely ECD1 and ECD2 (Fig. 1). Putative sterol-binding sites were identified in all reported structures of PTCH1, located in ECD1, between ECD1 and ECD2, and at two locations in the TMD (in the SSD and in the symmetry-related segment composed of TM helices 8–12). Unfortunately, the resolution of these PTCH1 cryo-EM structures (at best 3.5 Å) does not allow for the conclusive identification of the ligands, nor for visualization of their precise mode of binding to PTCH1. In favor of the transport activity of the protein, a hydrophobic channel has been proposed to run from the ECD to two openings: one above the SSD and one above the symmetry-related TM8–TM12 segment. In addition to its sterol transport role, PTCH1 has recently been shown to transport structurally different chemotherapeutics (doxorubicin, vemurafenib, cisplatin) out of cancer cells.12–15 Furthermore, PTCH1 belongs to the prokaryotic resistance-nodulation-division (RND) family of multidrug transporters, and like the other members of this family, its efflux activity is driven by the proton-motive force.3,12 Due to high glucose catabolism,16 the extracellular media is extremely acidic in cancer tissue making the drug efflux activity of PTCH1 specific for cancer cells expressing this protein.12 Moreover, PTCH1 is overexpressed in many cancer types, including adrenocortical carcinoma, breast, ovary, prostatic, melanoma, colon, brain and myeloid leukemia,17–21 particularly in cancer stem cells resistant to chemotherapeutic treatment.22
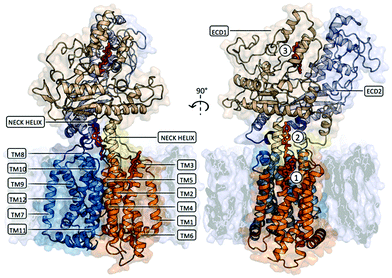 |
| Fig. 1 Membrane embedded PTCH1 (PDB ID 6n7h, chain A or globular monomer). The transmembrane domain is composed of 12 TM helices forming 2 pseudo-symmetrical RND domains (TM1–TM6 represented in orange cartoon and TM7–TM12 represented in blue cartoon). TM2–TM6 constitute a Sterol Sensing Domain (SSD) and a similar symmetry-related segment can be identified on TM8–TM12. Two extra-cellular domains, namely ECD1 and ECD2, are linked to the TM part of the protein: the N-termini and C-termini of ECD1 are linked to TM1 and TM2 by a long flexible linker and a Neck helix respectively (represented as yellow cartoon), while the N-termini and C-termini of ECD2 are linked to TM7 and TM8 by the same structural motifs (represented as purple cartoon). Two distinct domains can be identified in each ECD: an α + β sandwich fold proximal to the TM domain and a distal ‘helical domain’ composed of several α-helices and lengthy loops. Putative sterol-binding sites were identified in all reported structures of PTCH1, located in ECD1 (indicated as site 3 in the figure), between ECD1 and ECD2 (indicated as site 2), and one located in the SSD (indicated as site 1). | |
The first inhibitor of PTCH1-mediated drug efflux, a natural panicein A hydroquonone (PAH, with relative configuration E) extracted from marine sponge, has been reported in 2015.13 Further studies with synthetic PAH (a mixture of E and Z stereoisomers in ratio 3
:
2) showed that when administered in combination with standard therapeutics, such as doxorubicin or vemurafenib, the compound is able to increase the drug cytotoxicity in melanoma cells in vitro and in vivo without undesirable side effects, thus representing a promising novel therapeutic strategy.15 While PAH is able to successfully inhibit PTCH1-related drug efflux, its quinone-oxidized analogue, panicein A (PA), has no inhibitory activity. A first structure–activity-relationship analysis of the hydroquinone-like aromatic ring revealed the importance of the hydroxyl moiety in meta position since the meta-methoxylated analogue is inactive. With a slight decrease of activity, the elimination of the double bond still gives a compound able to interfere with the drug efflux.15
Molecular insights into the mechanism by which PAH and analogues bind to PTCH1 are addressed in this study by means of a combination of different computational techniques. The compounds included in this work are shown in Fig. 2. Based on target druggability analysis and ensemble docking results, the binding site for PAH analogues on PTCH1 has been hypothesized to be the Neck pocket. All-atom μs-long molecular dynamics (MD) simulations of the compounds in water solution show markedly different behaviour between the sets of active and inactive compounds, suggesting that compounds assuming a cylindrical (open) conformation in water solution are most-likely to bind to PTCH1 and favourably inhibit its drug efflux activity.
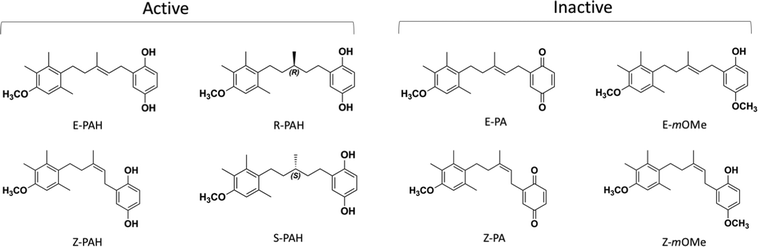 |
| Fig. 2 Chemical structures of the compounds included in the study: the stereoisomers and enantiomers of each structure (active: E-PAH and Z-PAH, R-PAH and S-PAH; inactive: E-PA and Z-PA, E-mOMe and Z-mOMe) were considered as they were synthesised and tested as a mixture of 2 forms.15 | |
Computational details
Pocket identification and analysis
Protein cryo-EM structure refinement.
The dimer structure of human PTCH1 (PDB ID 6n7h)9 was downloaded from the RCSB PDB and everything except the protein chain A (or globular chain) was removed from the starting structure. The choice of chain A for our study is supported by the following facts: (i) the transport of cholesterol by PTCH1 occurs in the absence of the SHH ligand, the receptor being in its monomeric form; (ii) chain B establishes extensive contacts with the SHH palmitoylated N-terminal, which inserts in between ECD1 and ECD2 (a region involved in the transport activity by PTCH1) leading to conformational rearrangements in the receptor. The missing side chains, mainly being located in N-terminal, C-terminal and intra-cellular loop regions, were modelled using MODELLER23 (http://salilab.org/modeller/) with backbone and resolved side chains restrained using the select_atoms method (i.e., only missing atoms could rearrange during the modelling process).
The TM6–TM7 missing loop going from R606 to K729 (excluded) is too long to be reliably modelled by homology, so TM6 and TM7 were connected following the engineered PTCH1 strategy24 (which was demonstrated to give a functional protein) as follows: TM6-RLDIFCCFTSPCVLHCLEPPC-TM7. The final structure (UniProt enumeration 73-619//720-1185) contains 1013 residues. The 5 top models (featuring the lowest DOPE scores) were visually inspected and considered in the following analysis. Hydrogens were added using pdb2pqr tools,25 which also minimizes steric clashes during the addition, and the protonation state of charged residues was assessed and assigned with PROPKA26 considering a physiological pH of 7.4. The pKa values and protonation states of the charged triad D513D514E1095 in this model were assigned as follows: D513 7.20 (deprotonated), D514 6.84 (deprotonated) E1095 11.17 (protonated). We checked the overall quality of the model using the web server MolProbity.27 MolProbity score combines the clash-score, rotamer and Ramachandran evaluations into a single score, normalized to be on the same scale as X-ray resolution. The final human PTCH1 model featured a good MolProbity Score of 1.24. Seven residues were Ramachandran outliers (0.71%) also present in the starting cryo-EM structure (out of the modelled regions).
Pocket detection.
The modelled protein was submitted to pocket detection analysis with CAVER Analyst 2.0.28 In this method, pockets are defined as the empty regions enclosing a large and a small spherical probe. We set the values of the radii to 1.7 Å and 3 Å (the latter being the default value) for the small and the large probe respectively. An estimation of the volume is finally calculated. The volume of each item (cavity or pocket) is computed by random sampling the bounding box of filling balls. Multiplying a rough evaluation of the hit probability by the bounding box volume gives an estimation of the real volume.
Fragment-based binding site identification.
FTMap is a webserver29 that identifies binding hot spots of macromolecules. These hot spots are defined as regions of the molecular surface involved in the binding of a variety of small organic probe molecules. While individual probes may bind at multiple sites, their simultaneous binding defines a hot spot. From a structural point of view, hot spots are characterized by a concave topology coupled to a mosaic-like pattern of polar and hydrophobic chemical groups. To identify hot spots, 16 small organic probe molecules of varying size, shape, and polarity are rigidly docked onto the surface of a macromolecule. Next, for each probe type, the docked probes are grouped into clusters and finally the different probe clusters are grouped in consensus clusters. The latter are ranked by the number of probe clusters they contain. An analysis on the residues in contact with the probe was also performed, using an atom-to-atom distance cutoff of 3.5 Å. The frequency of each contact residue is defined as follows:
Ligand characterization, molecular dynamics simulations and conformational analysis
Ligand physico-chemical properties.
Several molecular descriptors of PAH and derivatives were calculated with ChemAxon's suite of programs (Marvin 17.21.0, ChemAxon, https://www.chemaxon.com). In particular, we considered molecular weight, number of rotatable bonds, number of hydrogen bond donors/acceptors, physiological charge, log
P, log
S, molecular surface area, polar surface area and van der Waals volume. The molecular descriptors calculated for PAH and derivatives are shown in Table S1 (ESI†).
MD simulations.
The structures of the 8 above indicated ligands (Fig. 2) were sketched and converted into 3D xyz files with ChemAxon's Marvin suite. All compounds do not bear a net charge at physiological pH of 7.4. The structures were subsequently used as an input to Density Functional Theory geometry optimizations (B3LYP/6-31G** level) with the Gaussian package.30 Single-point energy calculations in vacuum were performed on the optimized geometry in order to generate the atomic partial charges fitting the molecular electrostatic potential. Atomic partial charges were generated through the restrained electrostatic potential (RESP) method31 implemented in the Antechamber package.32 All-atom MD simulations were performed in the presence of explicit water solution (0.1 M KCl) using the Amber18 package.33 Model systems were prepared with the program tleap of AmberTools18 adopting the TIP3P water model and the monovalent ion parameters. We used the General AMBER Force Field (GAFF)34 parameters and adopted the protocol described by Malloci et al.35
Post processing.
From all-atom 1 μs-long MD simulations we obtained structural and dynamical features of the compounds with the PTRAJ and CPPTRAJ programs.36 The first and second water shells were extracted using a lower and upper cutoff of 3.4 and 5.0 Å, respectively. For the analysis of intra- and inter-molecular H-bonds an angle and distance cutoffs of 135° (donor–hydrogen–acceptor angle) and 3.5 Å (donor–acceptor) were adopted, respectively. The MD trajectories were grouped in 10 structural clusters determined using the hierarchical agglomerative algorithm implemented in CPPTRAJ. The utility gmx rmsf of GROMACS37 was used to evaluate atomic root mean square fluctuations. Three morphology descriptors related to the gyration tensor, i.e., asphericity, acylindricity, and kappa2 were evaluated, as implemented in the PLUMED38 plugin. Asphericity and acylindricity give a measure of the deviation of the mass distribution from spherical and cylindrical symmetry, respectively while the relative shape anisotropy kappa2 reflects both symmetry and dimensionality. The dynamical evolution of the minimal projection area has been determined with the combined use of Open Babel39 and ChemAxon's Calculator Plugin. The distance between the geometrical centers of the two aromatic portions of the inhibitors was tracked using GROMACS utility gmx distance. The figures and graphs were generated with PyMOL40 and MATLAB (https://matlab.mathworks.com/), respectively.
Ensemble docking protocol
An extensive blind docking campaign was performed using Autodock Vina41 that implements a stochastic global optimization approach. We adopted a rectangular search space of size 80 Å × 80 Å × 130 Å enclosing the whole portion of the protein potentially exposed to the ligands. The exhaustiveness of the local search was set to 2048 (default 8). A cryo-EM structure of PTCH1 (PDB ID 6n7h), refined as previously described, was used as a representative conformation of the protein (other cryo-EM structures of the apo protein were not considered given the collapsed structure of the middle cavity, hereafter referred as Neck pocket). The flexibility of each compound was considered implicitly by employing the 10 representative conformations obtained from the MD simulation. Twenty docking poses were generated for each ligand conformation resulting in 200 binding modes per compound. A statistical contact analysis on the binding poses was performed in order to identify the protein residues involved in most frequent interactions with compounds (cutoff distance set to 3.5 Å). This analysis was restricted to two prominent binding pockets: Neck pocket and ECD pocket 1, as detected through CAVER Analyst (see below).
To ensure that only poses actually inside the selected cavities are taken into account, for each pocket we considered only docking poses in contact with at least 40% of the total number of residues lining the pocket (listed in Table 1). The number of contacts for each docking pose was then weighted by the corresponding cluster population fraction in order to prioritize high affinity docking poses whose ligand conformation is frequently found during the corresponding MD simulation. The number of poses considered for each ligand and the cluster populations coming from the MD trajectory are reported in Table S3 (ESI†).
Table 1 List of protein residues lining the three main putative binding pockets identified using CAVER Analyst. Residues underlined in bold are found with a frequency higher than 5% in the FTMap contact analysis
Neck pocket |
V125, L128, ![[W with combining low line]](https://www.rsc.org/images/entities/b_char_0057_0332.gif) ![[1 with combining low line]](https://www.rsc.org/images/entities/b_char_0031_0332.gif) ![[2 with combining low line]](https://www.rsc.org/images/entities/b_char_0032_0332.gif) , P155, L157, M363, F422, T424, L427, L431, F434, N496, A498, ![[L with combining low line]](https://www.rsc.org/images/entities/b_char_004c_0332.gif) ![[7 with combining low line]](https://www.rsc.org/images/entities/b_char_0037_0332.gif) ![[7 with combining low line]](https://www.rsc.org/images/entities/b_char_0037_0332.gif) , ![[D with combining low line]](https://www.rsc.org/images/entities/b_char_0044_0332.gif) ![[7 with combining low line]](https://www.rsc.org/images/entities/b_char_0037_0332.gif) ![[7 with combining low line]](https://www.rsc.org/images/entities/b_char_0037_0332.gif) , L777, I780, I791, Q794, F795, F800, Y801, N802, ![[Y with combining low line]](https://www.rsc.org/images/entities/b_char_0059_0332.gif) ![[1 with combining low line]](https://www.rsc.org/images/entities/b_char_0031_0332.gif) ![[0 with combining low line]](https://www.rsc.org/images/entities/b_char_0030_0332.gif) ![[1 with combining low line]](https://www.rsc.org/images/entities/b_char_0031_0332.gif) , ![[F with combining low line]](https://www.rsc.org/images/entities/b_char_0046_0332.gif) ![[1 with combining low line]](https://www.rsc.org/images/entities/b_char_0031_0332.gif) ![[0 with combining low line]](https://www.rsc.org/images/entities/b_char_0030_0332.gif) ![[1 with combining low line]](https://www.rsc.org/images/entities/b_char_0031_0332.gif) , ![[W with combining low line]](https://www.rsc.org/images/entities/b_char_0057_0332.gif) ![[1 with combining low line]](https://www.rsc.org/images/entities/b_char_0031_0332.gif) ![[0 with combining low line]](https://www.rsc.org/images/entities/b_char_0030_0332.gif) ![[1 with combining low line]](https://www.rsc.org/images/entities/b_char_0031_0332.gif) , F1147; |
ECD pocket 1 |
S206, L209, M216, I219, I220, L223, ![[Y with combining low line]](https://www.rsc.org/images/entities/b_char_0059_0332.gif) ![[2 with combining low line]](https://www.rsc.org/images/entities/b_char_0032_0332.gif) ![[2 with combining low line]](https://www.rsc.org/images/entities/b_char_0032_0332.gif) , P225, L227, W256, P261, F264, L265, W278, M281, L282, A285, V287, L330, S331, Y334, M335, W337, V932, A935, A936, Q938; |
ECD pocket 2 |
I210, T211, E212, T213, S277, W278, E280, M281, K284, W851, D878, D879, L882, S928, N929, D930, P931, V949, H950, D951, K952. |
Results and discussion
Protein characterization
CAVER analyst.
The cavity detection analysis led to the identification of 71 pockets and cavities on the protein surface. However, only 3 of them have an estimated volume large enough to be possibly occupied by the ligands and are represented in Fig. 3A (we set the threshold to volumes >300 Å3, that is approximately the van der Waals volume of PAH analogues, see Table S1, ESI†). The residues lining these specific regions are listed in Table 1. Notably, using a small probe radius lower than 1.60 Å led to the merging of the Neck and ECD pockets into a larger cavity, which is in agreement with the proposed tunnel for sterols transport.
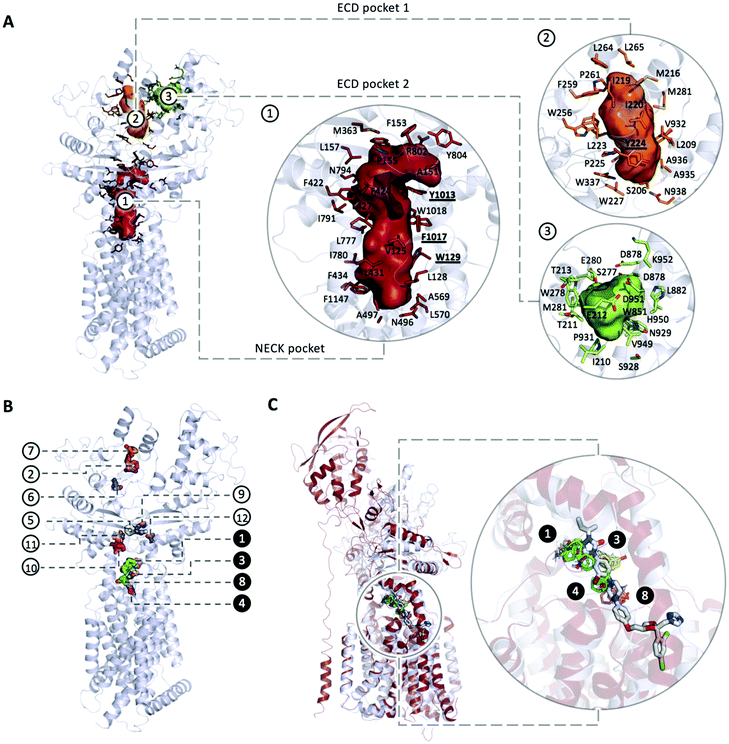 |
| Fig. 3 (A) Main pockets and pocket residues identified with CaverAnalyst; (B) mapping the structure of PTCH1 yields to 12 consensus clusters (CCs): CC1 (cyan, 24 probe clusters), CC2 (magenta, 12 probe clusters), CC3 (yellow, 10 probe clusters), CC4 (salmon, 9 probe clusters), CC5 (white, 8 probe clusters), CC6 (blue, 6 probe clusters), CC7 (orange, 4 probe clusters), CC8 (green, 4 probe clusters), CC9 (teal, 4 probe clusters), CC10 (pink, 4 probe clusters), CC11 (sand, 4 probe clusters), CC12 (purple, 5 probe clusters). All the identified CCs are located in a putative tunnel connecting the ECD pocket 1 and the Neck pocket; (B) the 1st and the 2nd top ranked CCs are represented in cyan and magenta respectively and are located within the cholesterol binding sites while major CCs density is located in the Neck (three more CCs namely CC3, CC4 and CC8, highlighted in black circles along with CC1). (C) Superimposition of PTCH1 (PDB ID 6n7h, represented in grey cartoon) and NPC1 (PDB ID 6UOX, represented in firebrick cartoon) in complex with its inhibitor itraconazole. Consensus Clusters CC1, CC3, CC4 and CC8 represented in cyan, yellow, green and salmon stick representation respectively are found to bind to PTCH1 in the same region as itraconazole binds to NPC1. | |
FTMap.
FTMap was run on the 5 top scored homology models of PTCH1 and all the runs gave identical results. The mapping the structure of PTCH1 yields to 12 consensus clusters (CCs, Fig. 3B). All 12 CCs are located in a putative tunnel connecting ECD binding site and Neck region with the first and second top ranked being in agreement with cholesterol experimental binding sites. The top ranked CC1 (cyan) binds 24 probe clusters in the Neck pocket, with all the different probe types being present, thus representing a relatively strong hot spot. Notably, 3 additional CCs, namely CC3, CC4 and CC8, were identified within 8 Å of the main CC further underlying the importance of this region of the protein in terms of druggability.
Interestingly, these 4 CCs are found in the same region of binding of itraconazole to NPC1, a PTCH1 homologous protein with cholesterol transport activity (Fig. 3C). NPC1 is located on the lysosomal membrane of eukaryotic cells where, along with the Niemann–Pick type C2 protein (NPC2), is responsible for cholesterol transport from the lysosomal lumen into the membrane. The NPC1 cholesterol transport was found to be inhibited by small molecules among which itraconazole. The structure of NPC1 in complex with itraconazole has recently been solved (PDB ID 6UOX) and it revealed the binding site being located along the putative sterol channel more specifically going from the proximity of the SSD to the central core of the receptor,42 analogous of the Neck pocket in PTCH1.
The PTCH1 residues involved in non-bonded and hydrogen-bonded interactions are represented in Fig. S1 (ESI†): while 124 residues give non-bonded interactions and 58 are involved in hydrogen bonded contacts, only a few residues were found with a frequency higher than 5%. Importantly, all of them are located in the Neck pocket and ECD pocket 1: W129, Y224, L775, D776, Y1013, F1017 and W1018.
Ligand dynamics in water solution
MD simulations revealed a markedly different behavior between the hydroquinone-like (active) and quinone-like (inactive) compounds: the former (E-PAH, Z-PAH, S-PAH, R-PAH) assume mainly a cylindrical (open) conformation in which the aromatic rings are far away from each other, while the latter (E-PA, Z-PA, E-mOMe, Z-mOMe) establish intramolecular π–π interactions and assume preferably a more spheric (closed) conformation (as can be appreciated in Fig. 4, columns 1, 2 and 3). Accordingly, all molecular descriptors displayed significantly marked differences between the two set of compounds (a full representation of all the descriptors calculated is shown Fig. S2 and S3, ESI†). To identify the predominant effect determining the different dynamical behavior of the two set of compounds we considered in detail the hydration, in terms of 1st and 2nd water shells population along with the occurrence of solute–solvent hydrogen bonds. While the number of water molecules in the 1st solvation shell are comparable for the different cases considered (Table 2), we found that the 2nd solvation shell of E-PAH, Z-PAH, S-PAH, R-PAH and E-PA, Z-PA, E-mOMe, Z-mOMe show a mono- and bi-modal distribution respectively, which is in line with the different conformation assumed during the simulation (Fig. 4, column 4).
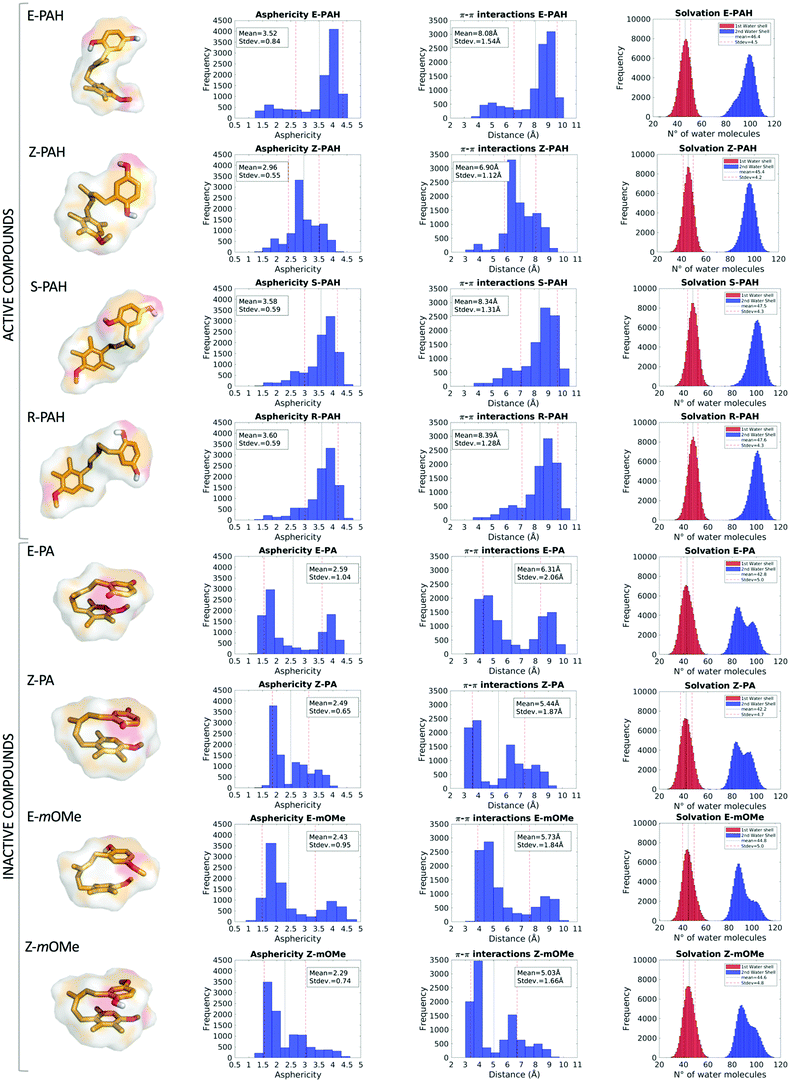 |
| Fig. 4
E-PAH, Z-PAH, S-PAH, R-PAH, E-PA, Z-PA, E-mOMe and Z-mOMe average structures extracted from μs MD simulation in the first column; asphericity, π–π interactions and solvation shells graphs are represented in the 2nd, 3rd and 4th column respectively. | |
Table 2 Comparison between the mean values of water molecules within the 1st solvation shell as well as the occurrence of solute–solvent hydrogen bonds during MD simulations
|
Water molecules within the 1st solvation shell |
Hydrogen-bond engagement with the solvent during MD (ortho/meta) |
E-PAH |
46.4 ± 4.5 |
90%/70% |
Z-PAH |
45.4 ± 4.2 |
90%/83% |
E-mOMe |
44.8 ± 5.0 |
94%/0.8% |
Z-mOMe |
44.6 ± 4.8 |
95%/0.8% |
E-PA |
42.8 ± 5.0 |
0.5%/0.4% |
Z-PA |
42.2 ± 4.8 |
0.6%/0.3% |
To investigate further the role that interactions established with the solvent (water solution) have in the determination of the open or closed conformation, the occurrence of solute–solvent hydrogen bonds was calculated for E-PAH, E-PA and E-mOMe (Table 2). The results show that for E-PAH, the hydroxyl groups are engaged in solute–solvent H-bonds as H-donors for almost 90% and 70% of the simulation time for the ortho and meta position, respectively, presumably representing an important variable for “stabilizing” the open conformation. Much lower occurrence of solute–solvent H-bond has been calculated for E-PA (0.5% and 0.4% of the simulation time for the ortho and meta position, respectively) indicating that solvation perhaps does not have a crucial impact in this case. Furthermore, the “bath” of surrounding water molecules establishing H-bonds with the solute could exert a frictional damping on the overall motions of the ligand as can be appreciated from the RMSD graph (Fig. S2, ESI†).
Blind ensemble docking
First, a validation of the method was performed by applying it to the native ligand found in the cryo-EM structure (PDB-ID 6n7h), cholesterol. The results show the Neck pocket being the prioritized site during the docking campaign (Fig. S3A, ESI†). Among the high affinity binding modes, several poses reproducing the cryo-EM data were found with the best ones given by conformation that is the second most frequently found during the MD simulation in water solution. Fig. S3B (ESI†) shows a superimposition of the native pose and the docking pose. Next to validation, docking and contact analysis were systematically performed for the 8 compounds under investigation that were found to differently inhibit the efflux activity of PTCH1.
The overall results from docking are collectively shown in Fig. 5A as spheres representing the centers of mass of each docking pose. The radii of the spheres are proportional to the cluster population derived from MD simulations, while the different colors highlight different binding affinities. E-PA, Z-PA, E-mOMe and Z-mOMe feature a large number of relatively low-affinity binding modes (>−9.0 kcal mol−1, blue spheres) mainly located in the peripheral regions of the protein. On the other hand, the hydroquinone-like compounds (E-PAH, Z-PAH, S-PAH, R-PAH) show the opposite behavior: the docking poses are mainly concentrated in the 2 binding regions, among which, the Neck pocket is the most populated one.
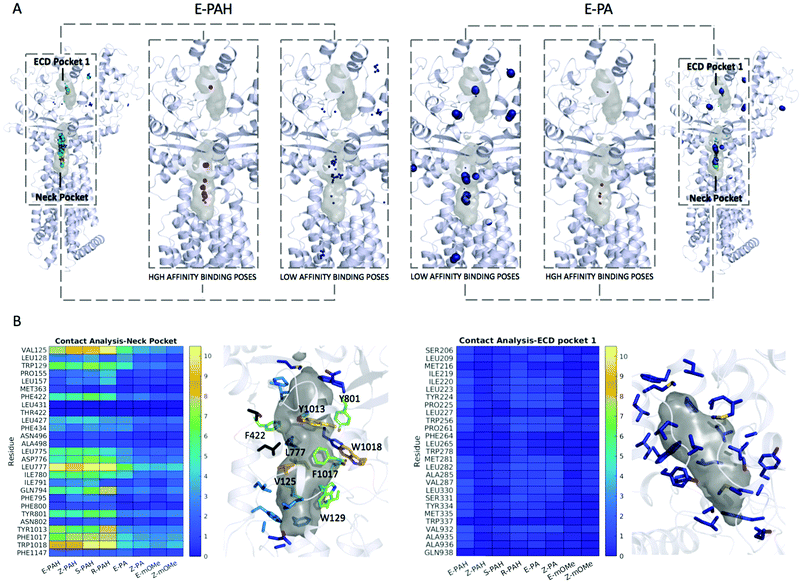 |
| Fig. 5 (A) Docking poses distribution for E-PAH and E-PA. The surface of the two pockets is represented in grey. The centers of mass of the docked poses are represented as spheres with radius increasing with the cluster population and colored according to the binding affinity with the following color-code: >−9.0 kcal mol−1, blue; [9.0, −9.5] kcal mol−1, cyan; [−9.5, −10.0] kcal mol−1, green; [−10.0, −10.5] kcal mol−1, white; ≤−10.5 kcal mol−1, red; (B) statistical contact analysis for the 8 PAH derivatives: residues are colored according to the color-code shown in the heat-map. | |
More in detail, a large number of high-affinity docking poses were identified among the first 4 clusters (including >70% of the conformations sampled along MD trajectories) for E-PAH, Z-PAH, S-PAH, R-PAH. In contrast, for E-PA, Z-PA, E-mOMe and Z-mOMe similar binding poses were found in less populated clusters. Note that, if considering the data without taking into account the MD-derived conformational clusters populations and thus considering all the conformations as equally likely, no relevant distinction was observed in terms of docking score and binding mode, when comparing the docking poses of the hydroquinone-like (active) and quinone-like (inactive) compounds (E-PAH vs. E-PA and Z-PAH vs. Z-PA).
A statistical analysis of the contacts established with the Neck and ECD pocket 1 is reported in Fig. 5B. Like for cholesterol (Fig. S4A, ESI†), and consistently with the hot spot identification results, the Neck pocket appears to be the preferred binding site for all compounds. However, there clearly appear to be differences in the occurrence of specific interactions, with residues VAL125, TRP129, ASP776, LEU777, GLY794, TYR801, TYR1013, PHE1017, and TRP1018 being specifically relevant for the interaction with the active ligands. Most of these residues bear aromatic groups and are scattered across the binding site, thus favoring relatively stronger interactions with more extended conformations of ligands that also have aromatic groups separated by flexible linkers.
Conclusions
Molecular aspects that guide the interference of E-PAH and related compounds with the drug efflux activity of PTCH1 are analyzed in this study. We first performed a thorough characterization and druggability analysis of the main putative substrate binding pockets of PTCH1, known from available cryo-EM structures. Further, 8 compounds, which showed different in vitro activity (as mixtures of E-PAH and Z-PAH, R-PAH and S-PAH, E-PA and Z-PA, E-mOMe and Z-mOMe), were submitted to μs-long all-atom MD simulations in water solution.
Analysis of the conformational landscape of compounds and evaluation of different dynamical descriptors were performed. The MD simulations in water solution revealed a markedly different behavior between the hydroquinone-like (active) and quinone-like (inactive) compounds: the former (E-PAH, Z-PAH, S-PAH, R-PAH) assume mainly a cylindrical (open) conformation in which the aromatic rings are far away from each other, while the latter (E-PA, Z-PA, E-mOMe, Z-mOMe) establish intramolecular π–π interactions and assume preferably a closed conformation.
Finally, the outcome of MD simulations was coupled to a blind ensemble docking methodology followed by a statistical contact analysis that enabled to rationalize the interaction between PTCH1 and PAH and derivatives in terms of their intrinsic physico-chemical properties. In particular, druggability analysis and blind ensemble docking suggest the Neck pocket as the preferential binding site for PAH analogues on PTCH1. Note that structural evidences of inhibitors of other PTCH1 homologous proteins, found to bind in the same region, support this hypothesis.42 In addition, our results suggest that compounds assuming a cylindric-like shape are able to better fit into the Neck pocket. Importantly, such an open conformation is preferred in solution by the active compounds, which bear two H-bond donor groups on the hydroquinone-like ring. From the present results, it remains unclear if the difference between active and inactive compounds in solution has key consequences for their interaction with PTCH1. While it is clear that even poorly sampled conformations in solvent could be “selected” by the protein giving high affinity complexes, the closed self-interacting geometry preferentially assumed by the inactive compounds could interfere with their ability to interact with PTCH1. Future investigations in our group will focus on characterizing the dynamical interaction of the compounds with the Neck region, e.g. by subjecting the top docking poses to all-atom MD simulations in the presence of physiological solvents. The results reported here will inform future drug design in order to synthesize compounds that will more potently inhibit PTCH1 drug efflux activity and, in combination with standard treatment, increase cancer cell death.
Conflicts of interest
There are no conflicts to declare.
Acknowledgements
S. K., G. M., A. V. V. and P. R. thank Andrea Bosin and Giovanni Serra (Cagliari) for technical assistance. The Authors acknowledge Méliné Simsir (Nice) for sharing her knowledge and experience about PTCH1 efflux. This research has received funding from the French government through the UCAJEDI Investments in the Future project with the reference number ANR-15-IDEX-01, Région PACA and BoostUrCareer project European Union's Horizon 2020 research and innovation program under grant agreement no. 847581. This paper is dedicated to Prof. Jan Peter Toennies (Göttingen). P. R. particularly thanks Prof. Toennies for bringing him close to the world of molecules: P.R.'s transition from surface physics to biophysics has greatly benefitted from the Göttingen period in the Max-Planck Institute directed by Prof. Toennies.
Notes and references
- R. J. Kathawala, P. Gupta, C. R. Ashby and Z. S. Chen, Drug Resist. Updates, 2015, 18, 1–17 CrossRef.
- M. Varjosalo and J. Taipale, Genes Dev., 2008, 22, 2454–2472 CrossRef CAS.
- M. Bidet, O. Joubert, B. Lacombe, M. Ciantar, R. Nehmé, P. Mollat, L. Brétillon, H. Faure, R. Bittman, M. Ruat and I. Mus-Veteau, PLoS One, 2011, 6, e23834 CrossRef CAS.
- X. Gong, H. Qian, X. Zhou, J. Wu, T. Wan, P. Cao, W. Huang, X. Zhao, X. Wang, P. Wang, Y. Shi, G. F. Gao, Q. Zhou and N. Yan, Cell, 2016, 165, 1467–1478 CrossRef CAS.
- X. Gong, H. Qian, P. Cao, X. Zhao, Q. Zhou, J. Lei and N. Yan, Science, 2018, 361, eaas8935 CrossRef.
- X. Qi, P. Schmiege, E. Coutavas, J. Wang and X. Li, Nature, 2018, 560, 128–132 CrossRef CAS.
- X. Qi, P. Schmiege, E. Coutavas and X. Li, Science, 2018, 362, eaas8843 CrossRef.
- Y. Zhang, D. P. Bulkley, Y. Xin, K. J. Roberts, D. E. Asarnow, A. Sharma, B. R. Myers, W. Cho, Y. Cheng and P. A. Beachy, Cell, 2018, 175, 1352–1364 CrossRef CAS , e14.
- H. Qian, P. Cao, M. Hu, S. Gao, N. Yan and X. Gong, Nat. Commun., 2019, 10, 2320 CrossRef.
- C. Qi, G. Di Minin, I. Vercellino, A. Wutz and V. M. Korkhov, Sci. Adv., 2019, 5, eaaw6490 CrossRef CAS.
- A. F. Rudolf, M. Kinnebrew, C. Kowatsch, T. B. Ansell, K. El Omari, B. Bishop, E. Pardon, R. A. Schwab, T. Malinauskas, M. Qian, R. Duman, D. F. Covey, J. Steyaert, A. Wagner, M. S. P. Sansom, R. Rohatgi and C. Siebold, Nat. Chem. Biol., 2019, 15, 975–982 CrossRef CAS.
- M. Bidet, A. Tomico, P. Martin, H. Guizouarn, P. Mollat and I. Mus-Veteau, Mol. Cancer Res., 2012, 10, 1496–1508 CrossRef CAS.
- L. Fiorini, M. A. Tribalat, L. Sauvard, J. Cazareth, E. Lalli, I. Broutin, O. P. Thomas and I. Mus-Veteau, Oncotarget, 2015, 6, 22282–22297 CrossRef.
- A. Hasanovic and I. Mus-Veteau, Cells, 2018, 7, 107 CrossRef.
- L. Signetti, N. Elizarov, M. Simsir, A. Paquet, D. Douguet, F. Labbal, D. Debayle, A. Di Giorgio, V. Biou, C. Girard, M. Duca, L. Bretillon, C. Bertolotto, B. Verrier, S. Azoulay and I. Mus-Veteau, Cancers, 2020, 12, 1500 CrossRef CAS.
- M. Damaghi, J. W. Wojtkowiak and R. J. Gillies, Front. Physiol., 2013, 4, 370 Search PubMed.
- A. Gonnissen, S. Isebaert, C. Perneel, C. M. McKee, F. Van Utterbeeck, E. Lerut, C. Verrill, R. J. Bryant, S. Joniau, R. J. Muschel and K. Haustermans, Am. J. Pathol., 2018, 188, 795–804 CrossRef CAS.
- S. Blotta, J. Jakubikova, T. Calimeri, A. M. Roccaro, N. Amodio, A. K. Azab, U. Foresta, C. S. Mitsiades, M. Rossi, K. Todoerti, S. Molica, F. Morabito, A. Neri, P. Tagliaferri, P. Tassone, K. C. Anderson and N. C. Munshi, Blood, 2012, 120, 5002–5013 CrossRef CAS.
- K. S. Jeng, I. S. Sheen, W. J. Jeng, M. C. Yu, H. I. Hsiau and F. Y. Chang, OncoTargets Ther., 2013, 7, 79–86 CrossRef.
- C. Zhao, A. Chen, C. H. Jamieson, M. Fereshteh, A. Abrahamsson, J. Blum, H. Y. Kwon, J. Kim, J. P. Chute, D. Rizzieri, M. Munchhof, T. VanArsdale, P. A. Beachy and T. Reya, Nature, 2009, 458, 776–779 CrossRef CAS.
- K. C. S. Queiroz, R. R. Ruela-De-Sousa, G. M. Fuhler, H. L. Aberson, C. V. Ferreira, M. P. Peppelenbosch and C. A. Spek, Oncogene, 2010, 29, 6314–6322 CrossRef CAS.
- C. R. Cochrane, A. Szczepny, D. N. Watkins and J. E. Cain, Cancers, 2015, 7, 1554–1585 CrossRef CAS.
- B. Webb and A. Sali, Curr. Protoc. Bioinf., 2016, 54, 5.6.1–5.6.37 Search PubMed.
- A. Fleet, J. P. Y. Lee, A. Tamachi, I. Javeed and P. A. Hamel, J. Biol. Chem., 2016, 291, 17557–17568 CrossRef CAS.
- T. J. Dolinsky, P. Czodrowski, H. Li, J. E. Nielsen, J. H. Jensen, G. Klebe and N. A. Baker, Nucleic Acids Res., 2007, 35, W522–W525 CrossRef.
- C. R. Søndergaard, M. H. M. Olsson, M. Rostkowski and J. H. Jensen, J. Chem. Theory Comput., 2011, 7, 2284–2295 CrossRef.
- V. B. Chen, W. B. Arendall, J. J. Headd, D. A. Keedy, R. M. Immormino, G. J. Kapral, L. W. Murray, J. S. Richardson and D. C. Richardson, Acta Crystallogr., Sect. D: Biol. Crystallogr., 2010, 66, 12–21 CrossRef CAS.
- A. Jurcik, D. Bednar, J. Byska, S. M. Marques, K. Furmanova, L. Daniel, P. Kokkonen, J. Brezovsky, O. Strnad, J. Stourac, A. Pavelka, M. Manak, J. Damborsky and B. Kozlikova, Bioinformatics, 2018, 34, 3586–3588 CrossRef CAS.
- D. Kozakov, L. E. Grove, D. R. Hall, T. Bohnuud, S. E. Mottarella, L. Luo, B. Xia, D. Beglov and S. Vajda, Nat. Protoc., 2015, 10, 733–755 CrossRef CAS.
-
M. J. Frisch, G. W. Trucks, H. B. Schlegel, G. E. Scuseria, M. A. Robb, J. R. Cheeseman, G. Scalmani, V. Barone, G. A. Petersson, H. Nakatsuji, X. Li, M. Caricato, A. Marenich, J. Bloino, B. G. Janesko, R. Gomperts, B. Mennucci, H. P. Hratchian, J. V. Ortiz, A. F. Izmaylov, J. L. Sonnenberg, D. Williams-Young, F. Ding, F. Lipparini, F. Egidi, J. Goings, B. Peng, A. Petrone, T. Henderson, D. Ranasinghe, V. G. Zakrzewski, J. Gao, N. Rega, G. Zheng, W. Liang, M. Hada, M. Ehara, K. Toyota, R. Fukuda, J. Hasegawa, M. Ishida, T. Nakajima, Y. Honda, O. Kitao, H. Nakai, T. Vreven, K. Throssell, J. A. Montgomery, Jr., J. E. Peralta, F. Ogliaro, M. Bearpark, J. J. Heyd, E. Brothers, K. N. Kudin, V. N. Staroverov, T. Keith, R. Kobayashi, J. Normand, K. Raghavachari, A. Rendell, J. C. Burant, S. S. Iyengar, J. Tomasi, M. Cossi, J. M. Millam, M. Klene, C. Adamo, R. Cammi, J. W. Ochterski, R. L. Martin, K. Morokuma, O. Farkas, J. B. Foresman and D. J. Fox, Gaussian16 (Revision A.03), 2016 Search PubMed.
- C. I. Bayly, P. Cieplak, W. D. Cornell and P. A. Kollman, J. Phys. Chem., 1993, 97(40), 10269–10280 CrossRef CAS.
- J. Wang, W. Wang, P. A. Kollman and D. A. Case, J. Mol. Graphics Modell., 2006, 25, 247–260 CrossRef CAS.
-
D. A. Case, I. Y. Ben-Shalom, S. R. Brozell, D. S. Cerutti, T. E. Cheatham, III, V. W. D. Cruzeiro, T. A. Darden, R. E. Duke, D. Ghoreishi, M. K. Gilson, H. Gohlke, A. W. Goetz, D. Greene, R. Harris, N. Homeyer, Y. Huang, S. Izadi, A. Kovalenko, T. Kurtzman, T. S. Lee, S. LeGrand, P. Li, C. Lin, J. Liu, T. Luchko, R. Luo, D. J. Mermelstein, K. M. Merz, Y. Miao, G. Monard, C. Nguyen, H. Nguyen, I. Omelyan, A. Onufriev, F. Pan, R. Qi, D. R. Roe, A. Roitberg, C. Sagui, S. Schott-Verdugo, J. Shen, C. L. Simmerling, J. Smith, R. S. Ferrer, J. Swails, R. C. Walker, J. Wang, H. Wei, R. M. Wolf, X. Wu, L. Xiao, D. M. York and P. A. Kollman, Amber 2018, 2018 Search PubMed.
- D. A. Case, J. M. Wang, R. M. Wolf, J. W. Caldwell and P. A. Kollman, J. Comput. Chem., 2004, 25, 1157–1174 CrossRef.
- G. Malloci, A. V. Vargiu, G. Serra, A. Bosin, P. Ruggerone and M. Ceccarelli, Molecules, 2015, 20, 13997–14021 CrossRef CAS.
- D. R. Roe and T. E. Cheatham, J. Chem. Theory Comput., 2013, 9, 3084–3095 CrossRef CAS.
-
M. J. Abraham, D. van der Spoel, E. Lindahl, B. Hess and the GROMACS team, GROMACS User Manual version, 2019 Search PubMed.
- M. Bonomi, D. Branduardi, G. Bussi, C. Camilloni, D. Provasi, P. Raiteri, D. Donadio, F. Marinelli, F. Pietrucci, R. A. Broglia and M. Parrinello, Comput. Phys. Commun., 2009, 180, 1961–1972 CrossRef CAS.
- N. M. O’Boyle, M. Banck, C. A. James, C. Morley, T. Vandermeersch and G. R. Hutchison, J. Cheminf., 2011, 3, 33 Search PubMed.
-
W. L. DeLano, Schrödinger LLC, 2020.
- O. Trott and A. J. Olson, J. Comput. Chem., 2010, 31, 455–461 CAS.
- T. Long, X. Qi, A. Hassan, Q. Liang, J. K. De Brabander and X. Li, Nat. Commun., 2020, 11, 152 CrossRef CAS.
Footnote |
† Electronic supplementary information (ESI) available. See DOI: 10.1039/d0cp05719c |
|
This journal is © the Owner Societies 2021 |
Click here to see how this site uses Cookies. View our privacy policy here.