Multiple site ground-based evaluation of carbonaceous aerosol mass concentrations retrieved from CAMS and MERRA-2 over the Indo-Gangetic Plain†
Received
22nd August 2021
, Accepted 27th October 2021
First published on 29th October 2021
Abstract
Recent progress in the availability of reanalysis data of Earth system variables with high spatial–temporal resolution provides valuable information for estimating the impacts of atmospheric aerosols. However, the aerosol module of reanalysis data has high uncertainty and must be validated with ground-based measurements for better accuracy and precision. In the present study, carbonaceous aerosol (black carbon and organic carbon) mass concentrations simulated by CAMS (Copernicus Atmosphere Monitoring Service) and MERRA-2 (Modern-Era Retrospective analysis for Research and Applications, version 2) were evaluated with ground-based measurements over different sites in the Indo-Gangetic Plain (IGP). The discrepancies in the reanalysis data for Black Carbon (BC) and Organic Carbon (OC) showed the influence of fresh and aged aerosols. Both CAMSRA and MERRA-2 reanalysis data underestimated the BC mass concentrations over the NW-IGP while overestimated over the central-IGP. These discrepancies were mainly due to inadequate knowledge about emissions and aging processes, especially over the central-IGP. Although the error in BC simulations is less than that in OC simulations, the inclusion/revision of local emissions will be helpful for accurate and precise simulations of carbonaceous aerosols over the IGP. For OC, observed deviations were complex mainly because of biases associated with complex atmospheric processes. At all four IGP study sites, CAMSRA overestimated OC, while MERRA-2 underestimated OC. The results clearly showed that the overestimation of CAMSRA simulated OC mass concentrations was due to the hygroscopic growth scheme, besides the poorly constrained escaped semi-volatile species from the primary organic aerosols. In contrast, the underestimation of MERRA-2 simulated OC was mainly due to existing ambiguities related to emissions at the NW-IGP while it was due to the aging scheme at the central-IGP. In addition, underestimation of organics could also be due to the formation of secondary organic aerosols (SOA) in the atmosphere from the regionally transported gas-phase “pool” of efficient precursors.
Environmental significance
The high-resolution measurement of carbonaceous aerosols is essential for the reduction of their uncertainty. The reanalysis of carbonaceous aerosol data has gathered significant attention because of its fine spatial–temporal resolution. These reanalysis data must be evaluated with ground-based measurements, as the aerosol module has high uncertainty. In this study, Black Carbon (BC) and Organic Carbon (OC) concentrations retrieved from reanalysis data were evaluated with ground-based observations over the Indo-Gangetic Plain. The results suggested that discrepancies in BC were mainly due to poorly constrained emissions and aging. Besides emissions, discrepancies in OC were also associated with secondary organics, which are discussed in the manuscript. It is expected that the results can be helpful for future parameterization for carbonaceous aerosol simulations over South Asia.
|
1. Introduction
Atmospheric pollutants negatively impact our environment in several different ways by deteriorating human health, climate, and agricultural yield.1–4 Amongst atmospheric pollutants, carbonaceous aerosols such as Black Carbon (BC) and Organic Carbon (OC) have attracted attention from the scientific community due to significant uncertainty associated with their impacts.5 Atmospheric BC particles are emitted from primary combustion processes, while OC particles originate from primary and secondary processes. Furthermore, primary and secondary organic aerosols undergo complex multistage heterogeneous oxidation and evaporation–condensation processes as they age.6–8 The relative contribution of primary vs. secondary organic aerosols to the total organic aerosol budget is uncertain.7 Carbonaceous aerosol concentrations and characteristics vary significantly with space and time due to their short atmospheric lifetime compared with gases, source variability, local meteorology, and characteristic transformation processes in the atmosphere.9,10 Thus, for a better understanding of the characteristics of carbonaceous aerosols, high-resolution measurement is essential.
Across the globe, the Indo Gangetic Plain (IGP) is one of the largest emitters of carbonaceous aerosols.5 Over the past few decades, several researchers have studied carbonaceous aerosol variability and its implications on climate and human health over different IGP locations.11–17 However, these studies are restricted to only a few urban and rural sites of the IGP. In terms of economic feasibility, a limited approach can assess carbonaceous aerosols (or any other earth system variables) at the ground level over the small grid across India or anywhere in the world. In this regard, data assimilation has been a very active field of research over the past decade, and it utilizes both ground-based in situ data and satellite data in atmospheric chemistry modeling.18 The most crucial advantage of data assimilation is its ability to generate complete time-window data, even though real-time data are not available. If a data assimilation approach is performed for past long-term or retrospective data (a decade or more), it is also called “reanalysis data”.19,20
In recent times, satellite/model reanalysis data of CAMS (Copernicus Atmosphere Monitoring Service) and MERRA-2 (Modern-Era Retrospective Analysis for Research and Applications, version 2) have gathered much scientific attention due to a wide variety of atmospheric variables and finer resolution.21,22 However, the uncertainty associated with aerosol products of these reanalysis data is generally high because of poorly constrained emissions and parameterization of the physical process, such as hygroscopic growth, mixing, and aerosol–cloud interactions.23–27 Moreover, the association of aerosol products of reanalysis data varies with geographical regions mainly due to the difference in constrained emissions and modeling approaches of aerosol chemistry and transport.28 Therefore, aerosol products should be validated locally or regionally with ground-based observations before drawing any further insights from them.
Earlier, several authors have reported the assessment of the CAMSRA and/or MERRA-2 simulated aerosol optical depth with AERONET observations.28–31 At the same time, limited studies have stated the association of reanalysis data of aerosol mass concentrations with ground-based observations.32 Previously, Buchard et al.33 reported the comparison of ground-based aerosol mass concentrations measured from approximately 300 sites across the United States with MERRA (version 1) reanalysis data. They have mentioned that MERRA underestimated BC and OC mass concentrations during the winter months mainly due to variability in emission sources and their strengths. Similar results were also reported for BC and OC mass concentrations measured over 55 sites across Europe.34 They have also observed that inadequate estimation of Secondary Organic Aerosols (SOAs) could also be an important reason for underestimation of OC over Europe. In China, Ma et al.35 reported the comparison of ground-based aerosol mass concentrations measured from two different sites with MERRA-2 reanalysis data. They have also stated that MERRA-2 overestimated BC mass concentrations, while OC was underestimated significantly during the winter months. It is noteworthy to mention that evaluation of these reanalysis data in the context of secondary aerosols is rather noticed or studied, although it contributes a dominant fraction of atmospheric organic carbon. The recent validation report of CAMSRA products showed clear evidence that the aerosol module overestimated organic aerosols, which further underestimated the dust fraction.36
Only a few studies have reported the association of reanalysis data of carbonaceous aerosol mass concentrations with ground-based observations over South Asia. For example, Saikia et al.37 and Pathak et al.38 reported the association of ground-based measured BC with CAMS and MERRA-2 at Dibrugarh, located in India's northeast region. Prabhu et al.21 reported the association of ground-based measured BC with CAMS and MERRA-2 at Dehradun, located in the foothills of western Himalayas. To the best of our knowledge, no prior study has attempted to validate OC reanalysis data with ground-based observations over South Asia. In addition, evaluation methods of carbonaceous aerosols (either BC or OC) derived from the reanalysis data are scarce over the IGP regions.
Measurement of organic and elemental carbon (carbonaceous aerosols) in the past several years over different locations of the IGP has been conducted in our laboratory. The main objectives of this study utilizing the past data sets are: (1) to compare CAMSRA and MERRA-2 simulated carbonaceous BC and OC mass concentrations with the ground-based observations, and (2) to identify the possible reasons for error propagations in the reanalysis data of BC and OC with particular emphasis on fresh vs. aged aerosols. It is expected that the output might be helpful to the scientific community for better evaluation of reanalysis data over the polluted IGP region of South Asia.
2. Methodology
2.1 Details of study sites
In the present study, in situ aerosol samples collected from four distinct locations of the Indo-Gangetic Plain were studied (Fig. 1). A brief description of the study sites is given below:
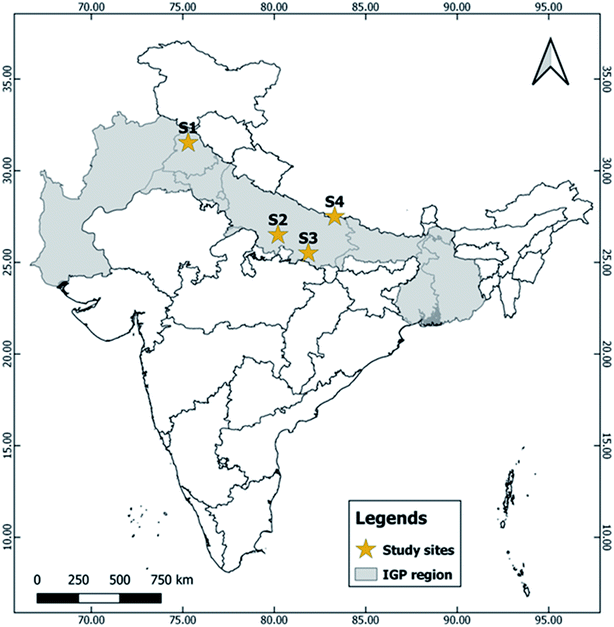 |
| Fig. 1 A map of India showing the study sites along with Indo-Gangetic Plain (IGP) region in grey shade. | |
(1) S1-Beas (31.52° N, 75.29° E, and 238 m AMSL).
Beas is situated in the North-Western Indo Gangetic Plain (NW-IGP). Agricultural residue burning is a common practice in the surrounding regions of Beas. During the pre-monsoon month of May, significant emissions are from wheat-residue burning, while paddy-residue burning emissions dominate during the post-monsoon months of October and November.39 Moreover, a detailed description of the study site, local meteorology, and other emission sources during the sampling campaigns is presented elsewhere.13,17,40
(2) S2-Kanpur (26.50° N, 80.20° E, and 142 m AMSL).
Kanpur is situated in the central-IGP region. Measurement campaigns were performed at the Indian Institute of Technology Kanpur campus situated at about 17 km upwind of Kanpur city. The dominant sources of carbonaceous aerosols in Kanpur city are vehicular exhausts, biomass/biofuel burning, industrial emissions, coal combustion, solid waste burning, heavy oil combustion, leather waste burning, and secondary formation.41,42 A detailed description of the study site, local meteorology, and other emission sources during the sampling campaigns is presented in our previous studies.43,44
(3) S3-Allahabad (25.50° N, 81.86° E, and 98 m AMSL).
Allahabad is situated in the central-IGP region located at approximately 200 km (aerial distance) downwind of Kanpur. The major sources of carbonaceous aerosols in Allahabad are automobile exhausts, biomass burning, small industrial activities, and railway traffic.45 A detailed description of the study site, local meteorology, and other emission sources during the sampling campaigns can be further explored in a previous study.46
(4) S4-Lumbini (27.49° N, 83.30° E, and 150 m AMSL).
Lumbini is also situated on the northern edge of the central-IGP region located at approximately 300 km (aerial distance) upwind of Kanpur and 250 km (aerial distance) upwind of Allahabad. The major sources of carbonaceous aerosols in Lumbini are agricultural residue burning, unpaved road dust, vehicular emissions, open burning (biomass and garbage), house heating and cooking using the traditional method, and industrial emissions.47,48 A detailed description of the study site, local meteorology, and other emission sources during the sampling campaigns has been demonstrated in our previous studies.16,49
2.2 Ground-based measurement of carbonaceous aerosols
Atmospheric particulate matter (PM2.5 or PM1) was collected onto pre-baked (400–600 °C for 6–8 hours) quartz fiber filters (Whatman, USA) using different high- to low-volume air samplers for the carbonaceous aerosol measurements.50,51 Details about sampling campaigns and instruments utilized are presented in Table 1. A small punch of quartz-fiber filters (0.53–1.5 cm2) was used to analyze Elemental Carbon (EC) and Organic Carbon (OC) mass concentrations using the thermal optical method. In the present study, quartz-fiber filters were analyzed using the Desert Research Institute (DRI) 2015 model and a Sunset Lab EC/OC thermo-optical carbon analyzer. On the DRI 2015 analyzer, samples were analyzed using the IMPROVE (Interagency Monitoring of Protected Visual Environment) thermal/optical transmittance protocol, while on the Sunset Lab analyzer, samples were analyzed using the NIOSH (National Institute for Occupational Safety and Health) transmittance protocol. Some of the ground-based data at Kanpur (data of the year 2014) were measured from the PM1 samples. We have extrapolated these carbonaceous aerosol data of PM1 into PM2.5 to make the ground-based data homogeneous; details are mentioned in Section S1 in the ESI.†
Table 1 Detail about the sampling campaigns over the IGP
Study sites |
No. of samples |
Sampling month and year |
Sampling duration (times in IST) |
PM size |
Instrument used for EC and OC |
S1-Beas |
33 |
October-2016; May-2018; August-2018 |
9 hour (9:00 to 18:00 and 21:00 to 6:00); 7 hours (21:00 to 4:00 and 9:30 to 16:30) |
PM2.5 |
DRI |
S2-Kanpur |
168 |
September-2014; October-2014; December-2015; January-2016 |
1 hour (from 8:30 to 18:30); 4 hours (21:30 to 1:30 and 2:00 to 6:00) |
PM1 and PM2.5 |
DRI and Sunset |
S3-Allahabad |
42 |
January-2016, February-2016; November-2016; December-2016 |
10 hours (21:00 to 7:00) |
PM2.5 |
DRI |
S4-Lumbini |
45 |
December-2017; January-2018 |
10 hours (10:00 to 20:00) |
PM2.5 |
DRI |
Further details about the filter handling, sampling procedure, quality control, and quality assurance during the sampling campaigns are reported elsewhere.13,16,43,44,46 In addition, information on the instrument's measurement principles is reported in previous studies.52–54 For simplicity, Elemental Carbon (EC) is written as Black Carbon (BC) throughout the manuscript.
2.3 Reanalysis data of carbonaceous aerosols
The CAMS reanalysis data are produced by the European Centre for Medium-Range Weather Forecasts (ECMWF) with the updated Integrated Forecast System (IFS), which uses the new version of the carbon bond mechanism developed in 2005 (CB05).55 In the CAMSRA aerosol module, anthropogenic emissions of carbonaceous aerosols were utilized from the MACCity inventory.56 However the fraction of anthropogenic secondary organic aerosols is calculated using a proxy by applying a scaling factor of 0.2 to the MACCity (Monitoring Atmospheric Composition and Climate) carbon monoxide emissions.57 The open biomass burning emissions were constrained by using a Global Fire Assimilation System, version 1.2 (GFASv1.2), dependent on the satellite-based fire radiative power.58 Moreover, employed emissions and detailed technical specifications of CAMSRA are reported elsewhere in Inness et al.55 The integrated forecast system simulates five aerosol types with a total of 12 prognostic tracers such as three size fractions of sea salts (0.030–0.55, 0.55–0.9, and 0.9–20 μm), three size fractions of dust (0.030–0.55, 0.55–0.9, and 0.9–20 μm), hydrophilic and hydrophobic components of organic matter and black carbon (four tracers), sulfate aerosol (SO42−), and the gas-phase sulfur dioxide (SO2) precursor. In the aerosol module of CAMSRA, all prognostic tracers are treated as externally mixed in the atmosphere. The meteorological model component of the IFS was used further to constrain the transportation of aerosols through advection, convection, and diffusion.
The MERRA-2 reanalysis data are produced by the NASA's Global Modeling and Assimilation Office.59 MERRA-2 uses the updated version of the Goddard Earth Observing System (GEOS-5) modeling system. Aerosols in MERRA-2 are simulated with a radiatively coupled version of the Goddard Chemistry, Aerosol, Radiation, and Transport (GOCART) model.60,61 In the GOCART aerosol module, the carbonaceous aerosol emissions are derived from natural, anthropogenic, and biomass burning sources. Natural sources include the formation of organics by the oxidation of biogenic emissions of the terpenes reported by Guenther et al.62 The anthropogenic emissions were utilized from the AeroCom Phase II dataset (HCA0_v1), which is reported in Diehl et al.63 The open biomass burning emissions were constrained by using the Quick Fire Emission Dataset (QFED) version 2.4-r6.64 Moreover, detailed discussion on constrained emissions in the MERRA-2 simulations of carbonaceous aerosols is mentioned in Randles et al.65 The GOCART model simulates five aerosol types with a total of 15 prognostic tracers such as sea salts (five size fractions of 0.03–10 μm), dust (five size fractions of 0.1–10 μm), hydrophilic and hydrophobic components of organic carbon and black carbon (four tracers), and sulfate aerosol (one tracer). In the GOCART model, all prognostic tracers are treated as externally mixed in the atmosphere. Some basic details of the used reanalysis products of CAMSRA and MERRA-2 are shown in Table 2.
Table 2 Some basic details of used reanalysis products of Copernicus Atmosphere Monitoring Service (CAMSRA) and Modern-Era Retrospective Analysis for Research and Applications, version 2 (MERRA-2)
|
CAMSRA |
MERRA-2 |
ERA5 |
Data type |
Grided |
Grided |
Grided |
Spatial resolution |
0.75° × 0.75° |
0.5° × 0.625° |
0.25° × 0.25° |
Temporal resolution |
3 hours |
1 hour |
1 hour |
Vertical resolution |
1000 hPa |
Surface |
Surface |
Short name |
EAC4 |
M2T1NXAER |
ERA5 |
Version |
n.a. |
5.12.4 |
n.a. |
2.4 Meteorological data
In the present study, basic meteorological data were retrieved from ERA5. ERA5 is the latest fifth-generation reanalysis dataset produced by ECMWF, which provides a wide range of commonly used land-surface and atmospheric variables with temporal coverage from 1950 to the present.66 The ERA5 version provides improved reanalysis data compared to its earlier ERA-interim version, which was used in several studies.66–69 ERA5 has a spatial resolution of 0.25° × 0.25° and a temporal resolution of 1 hour. Due to the unavailability of ground-based observations of meteorological data except for Lumbini, ERA5 reanalysis data such as u-wind, v-wind, temperature, relative humidity, and pressure were utilized. Some basic details of ERA5 reanalysis data used are also shown in Table 2.
2.5 Data analysis
All the reanalysis data were carefully downloaded from their respective platforms. For the retrieval of reanalysis data, we have chosen an approximately 25 km2 grid by keeping station coordinates in the center. There is a possibility that the selected grid (25 km2) falls under two different grids of reanalysis data. In that case, the average of those two grids was taken into consideration. The CAMSRA reanalysis data provide carbonaceous aerosols as mass mixing ratios (kg per kg of air) of hydrophilic and hydrophobic components of black carbon and Organic Matter (OM). These mass mixing ratios were converted into mass concentrations by multiplying the mass mixing ratios with air density at the ground (ρ = p/R × T). A ground-based study by Chakraborty et al.43 and Izhar et al.70 reported that the OM/OC ratio is equal to 2.2 at Kanpur and Lumbini situated in the central-IGP. This conversion factor was assumed for all the study sites to convert CAMSRA simulated OM to OC mass concentrations. Moreover, all the hydrophilic and hydrophobic components of BC or OC mass concentrations were compared with those of the carbonaceous aerosol mass concentrations measured from the atmospheric PM2.5 samples.
The CAMSRA (temporal resolution 3 hours) and MERRA-2 (temporal resolution 1 hour) simulated carbonaceous aerosol mass concentrations were averaged for a respective period of the measurement campaigns. Some of the available ground-based and reanalysis data have a difference between starting and ending times. In such a case, the nearest time for ground-based campaigns was chosen to average BC or OC mass concentrations, as it is difficult to interpolate BC and OC aerosol mass concentrations on a temporal scale. The maximum time difference between ground-based and reanalysis data is less than or equal to ±30 minutes. The outliers were eliminated very carefully to make sure that the removed data points are less than 2% of the entire data set. A more detailed discussion on performance statistics calculations is reported in Section S2 in the ESI.†
3. Results and discussion
3.1 Comparison between CAMSRA and MERRA-2 data over the IGP regions
The measurement sites were broadly divided into two regions based on their geographical location in the IGP: (1) north-western IGP and (2) central-IGP (Fig. 1). The different errors and biases associated with CAMSRA and MERRA-2 simulated BC and OC mass concentrations are mentioned in Table 3. Broadly, the ground-based measured BC and OC mass concentrations were moderately to strongly correlated with the simulated BC and OC mass concentrations (R < 0.05). Despite good correlations, the errors associated with the aerosol module are relatively high and can be attributed mainly to the spatial–temporal variation in emission sources and atmospheric processing.23–27 Overall, the estimated Mean Percentage Error (MPE) was significantly highest for CAMSRA data compared with the MERRA-2 data.
Table 3 Errors and biases with CAMSRA and MERRA-2 simulated BC and OC mass concentrations along with OC/BC ratio over the North-Western IGP (NW-IGP), and central-IGP regiona
Study regions |
|
FAC2 |
MB |
MPE |
MGE |
NMB |
NMGE |
RMSE |
R |
FAC2: fraction of predictions within a factor of two; MB: mean bias; MPE: mean percentage error; MGE: mean gross error; NMB: normalized mean bias; NMGE: normalized mean gross error; RMSE: root mean squared error; R: Pearson correlation coefficient.
|
NW-IGP (CAMSRA) (n = 33) |
BC |
0.76 |
−3.00 |
−19 |
3.84 |
−0.38 |
0.49 |
6.39 |
0.70 |
OC |
0.55 |
27.97 |
114 |
33.03 |
0.85 |
1.01 |
47.51 |
0.70 |
OC/BC |
0.18 |
8.47 |
189 |
8.47 |
1.53 |
1.53 |
8.74 |
0.52 |
NW-IGP (MERRA-2) (n = 33) |
BC |
0.30 |
−5.65 |
−53 |
5.74 |
−0.72 |
0.73 |
8.56 |
0.75 |
OC |
0.21 |
−21.89 |
−60 |
21.94 |
−0.67 |
0.67 |
29.78 |
0.81 |
OC/BC |
0.88 |
−0.41 |
8 |
2.11 |
−0.07 |
0.38 |
2.65 |
−0.03 |
Central-IGP (CAMSRA) (n = 206) |
BC |
0.66 |
0.89 |
62 |
2.58 |
0.20 |
0.59 |
3.66 |
0.51 |
OC |
0.17 |
64.51 |
250 |
64.77 |
2.08 |
2.09 |
79.31 |
0.72 |
OC/BC |
0.36 |
9.88 |
155 |
9.89 |
1.24 |
1.24 |
10.45 |
−0.16 |
Central-IGP (MERRA-2) (n = 253) |
BC |
0.76 |
0.21 |
18 |
1.94 |
0.06 |
0.52 |
3.04 |
0.66 |
OC |
0.58 |
−9.58 |
−34 |
11.40 |
−0.36 |
0.43 |
17.15 |
0.77 |
OC/BC |
0.70 |
−3.58 |
−39 |
3.65 |
−0.45 |
0.46 |
4.42 |
0.08 |
The concluding model/reanalysis data performance based on the correlation coefficient (R) and MPE could be misleading. In this regard, the Taylor diagram is one of the most critical methods for evaluating the model performance or relative merits concerning the true/ground-based values.71 Taylor diagrams for the present study are shown in Fig. 2. The graph shows the three complementary model performance statistics (correlation coefficient, the standard deviation, and the centered RMSE) that vary simultaneously in a single diagram. Earlier, several authors have utilized similar approaches to evaluate the performance/relative merits of different models or satellite data with measured or observed data of earth system variables.72–75
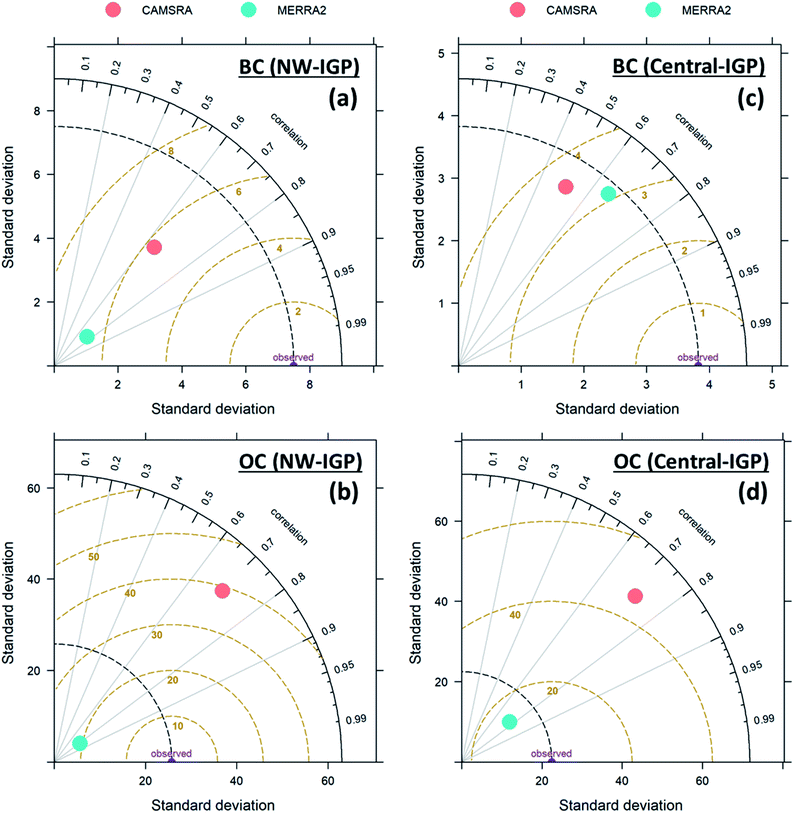 |
| Fig. 2 Taylor diagram with performance statistics of reanalysis data (correlation coefficient, the standard deviation, and the centered-RMSE). The observed standard deviation in ground-based BC and OC is shown with a purple color dot on the x-axis. The correlation is the cosine of the angle from the horizontal x-axis, the centered-RMSE is the distance from the purple point and the standard deviation is the distance from the origin of the plot. (a) and (b) represent the Taylor diagram for BC and OC at NW-IGP. (c) and (d) represents the Taylor diagram for BC and OC at central-IGP. | |
In the NW-IGP, carbonaceous aerosol measurements were performed at Beas village (S1), situated in India's Punjab state. MERRA-2 derived BC and OC mass concentrations were strongly correlated with the ground-based values; however, standard deviations were significantly less than those of the ground-based data (Fig. 2a and b). The standard deviations give information on the amplitude of variations, and it is proportional to the radial distance from the origin point of the graph.71 The standard deviation of CAMSRA simulated BC data was close to the standard deviation of ground-based data, as CAMSRA data were close to the black-colored dashed arc (Fig. 2a). The above observations suggest that the amplitude of variations in CAMSRA was matching with the ground-based BC. In the case of OC, MERRA-2 simulated OC data performed well (Fig. 2b).
In the central-IGP, carbonaceous aerosol measurements were conducted at three different sites, including Kanpur (S2), Allahabad (S3), and Lumbini (S4). At the central-IGP, all three statistics observed for MERRA-2 simulated BC and OC data have good agreement with the ground-based mass concentration data, for instance, better correlation coefficients, less centered RMSE, and identical standard deviations (Fig. 2c and d).
3.2 Overview of error propagation in the reanalysis data
The above results suggested that CAMSRA simulated better BC mass concentrations over the NW-IGP, while MERRA-2 simulated better mass concentrations over the central-IGP. In the case of OC, MERRA-2 simulated better OC mass concentrations over both the IGP regions. As both the IGP regions have different dominant sources of carbonaceous aerosols, IGP region-wise discussions are mentioned below:
(a) North-western IGP (NW-IGP).
It is well documented that the dominant emission sources of carbonaceous aerosols are primary sources such as agricultural residue burning over the NW-IGP.17,21,76,77 At the NW-IGP, both CAMSRA and MERRA-2 simulated BC mass concentrations were positively correlated with the measured BC mass concentrations with a correlation coefficient (R) of 0.70 (p < 0.05) and 0.75 (p < 0.05), respectively. Overall, both the reanalysis products underestimated the mean BC mass concentrations. The Mean Percentage Error (MPE) was −19% and −53% for CAMSRA and MERRA-2, respectively. Previously, several authors have reported the underestimation of BC simulated by CAMSRA and MERRA/MERRA-2 over several locations in India, Europe, and the United States.21,33,34 The major reason reported for the underestimation was poorly constrained emissions. In chemical transport models, local emission inventories are generally interpolated from the regional emissions,78 which is expected to increase biases in the emission parameterization. In addition, biases in meteorology simulation are also one of the critical reasons.35 For instance, overestimating surface wind and the boundary layer height can cause underestimation of aerosol mass concentrations because of more robust diffusion in the atmosphere.79
Like BC, reanalysis data of OC mass concentrations were positively correlated with the measured OC mass concentrations with correlation coefficients (R) of 0.70 (p < 0.05) and 0.81 (p < 0.05) for CAMSRA and MERRA-2, respectively. The CAMSRA overestimated the OC mass concentration with a MPE of +114%. An earlier study by Robinson et al.7 gave clear evidence that the semi-volatile species can be repartitioned and can escape from the primary organic aerosols into the gas phase. However, the CAMSRA simulates organic aerosols by assuming that (1) they are non-volatile and (2) condense irreversibly onto the existing aerosols.57 The observed results suggest that the overestimation of OC could be due to poorly constrained escaped semi-volatile and intermediate-volatile species from the organic aerosols. Earlier, Christophe et al.36 also observed overestimation of organic aerosols and the attributed major reason was overestimation of emissions and secondary organic aerosols. Further, the overestimation of OC can also impact the optical properties of the bulk aerosols, which may lead to biases in the radiative forcing estimation.36
At the same time, MERRA-2 underestimated the OC mass concentrations with a MPE of −60%, which is identical to that observed for BC (−53%). The time trend of the relative error of BC and OC was statistically significant, pointing towards errors mainly due to poorly constrained emissions. The results also showed that the escaped semi-volatile and intermediate-volatile species from the organic aerosols could be well constrained in MERRA-2 simulations. Earlier several studies have reported underestimation of MERRA/MERRA-2 simulated OC over the United States, Europe, and China mainly due to the inability to capture accurate emissions.33–35
(b) Central-IGP.
Similar to the NW-IGP, both the reanalysis data of BC mass concentrations were positively correlated with the measured value with a correlation coefficient (R) of 0.51 (p < 0.05) and 0.66 (p < 0.05) for CAMSRA and MERRA-2, respectively. However, the reanalysis products of CAMSRA and MERRA-2 overestimated the BC mass concentrations with an MPE of +62 and +18% for CAMSRA and MERRA-2. The overestimation of BC could be due to parameterization of emissions and meteorology. Recently, Ma et al.35 also reported overestimation of MERRA-2 BC mass concentrations over central and eastern China, mainly due to highly uncertain emission type and strength.
In the central-IGP, organic aerosols confine a significant fraction of secondary organic aerosols.82,83 The simulated OC mass concentrations were positively correlated with the measured OC mass concentrations with correlation coefficients (R) of 0.72 (p < 0.05) and 0.77 (p < 0.05) for CAMSRA and MERRA-2, respectively. Like the NW-IGP, CAMSRA overestimated and MERRA-2 underestimated the OC mass concentrations over the central-IGP. The MPE was observed to be +250 and −34% for CAMSRA and MERRA-2, respectively. In CAMSRA, the MPE for OC was approximately four times higher than that observed for BC. As discussed earlier, the overestimation of CAMSRA derived OC could be due to poorly constrained escaped semi-volatile species from the organic aerosols.
In the case of MERRA-2, the negative MPE value suggested that the aerosol module of MERRA-2 simulations constrained escaped semi-volatile species. Further, these repartitioned semi-volatile species form a “pool” of SOA precursors in the atmosphere, which may oxidize and form secondary organic aerosols (SOAs).7 The negative MPE value could be due to the inability to capture SOA formations from the “pool” of SOA precursors. As mentioned earlier, several studies have reported the underestimation of MERRA/MERRA-2 simulated OC mass concentrations.33–35 However, detailed discussion in the context of secondary OC is limited.
Overall, the error associated with the OC mass concentrations was higher than that of BC over the IGP regions. Besides the influence of escaped semi-volatile and intermediate-volatile species, the discrepancies in OC simulations could also be due to poorly constrained (a) nocturnal NOx radical initiated oxidation,84 (b) formation of secondary OC from the biogenic volatile organic compounds,85,86 and (c) aqueous-phase processing.87 A more detailed discussion on the influence of atmospheric processes is mentioned in the next section.
3.3 Influence of atmospheric processing on error propagation in the reanalysis data
The estimated errors in reanalysis data were regressed with the measured chemical markers of primary and secondary aerosols (e.g., primary organic carbon, secondary organic carbon, SO42−, NO3−, NH4+, Cl−, water-soluble organic carbon, and aerosol liquid water content) to get more insights into the influence of different atmospheric processes. The Aerosol Liquid Water Content (ALWC) measures the uptake of water by aerosol constituents at the deliquescence relative humidity and increases the size of aerosols,91 and can also be used as a proxy for the hygroscopicity of aerosols.92 The ALWC was calculated by using the thermodynamic ISORROPIA-II model in reverse mode.88 The ALWC was calculated as a function of the secondary inorganic species mass concentration, ambient temperature, and relative humidity. The mass concentrations of NO3−, SO42−, and NH4+ were taken as input inorganic aerosol species.
The observed relative error was in both positive and negative directions (Fig. S1 to S4 in the ESI†); therefore, the absolute values of the relative error were regressed with the parameters mentioned above. The correlation statistics for all four study sites are shown in Table S1† and discussed below:
(a) North-western-IGP (NW-IGP).
As discussed earlier, both the reanalysis products underestimated the BC mass concentrations, while OC mass concentrations were overestimated by CAMSRA and underestimated by MERRA-2. The FAC2 is a rigorous index widely used to evaluate model-simulated data, which falls within a factor of 2 for observed data.89 Such a rigorous index can exclude the influence of extreme values and errors. The simulation performance is considered reasonably good if the FAC2 value is greater than or equal to 50%.89 Overall, the estimated error for CAMSRA was small compared with that for MERRA-2 with a high FAC2 value above 55% (Table 3). The influence of different atmospheric processes on reanalysis data is discussed below:
CAMSRA.
It is a well-known fact that the BC particles undergo an aging process and get coated with non-absorbing inorganic and organic chemical constituents via coagulation with other particles and condensation of vapor.90 For CAMSRA derived BC data, the relationship of the absolute error with the chemicals mentioned above (SO42−, NO3−, NH4+, and Cl− normalized with BC) was insignificant. The insignificant association indicated that the error propagation in BC reanalysis data is irrespective of the aging or internal mixing of BC particles. The errors could be due to poorly constrained emissions. During the sampling campaigns in May and October, the dominant emission source of BC is open agricultural residue burning.39 However, it is expected that open burning activities could be well noticed because CAMSRA simulation utilizes satellite-based fire radiative power to constrain open emissions.55,58 Thus, rather than open burning, some other active emission sources were expected to be poorly constrained. Based on the carbon isotope study, Singh et al.17 reported that household biofuel burning was a vital emission source over the NW-IGP. Thus, emissions from household biomass/biofuel burning were anticipated to be poorly constrained in the CAMSRA simulations.
In the case of OC, the absolute relative error was statistically significant for the ALWC (R = 0.80, p < 0.05). As mentioned earlier, the ALWC can be used as a proxy for the hygroscopicity of aerosols. The results suggested that the errors were due to the hygroscopic growth scheme, besides the poorly constrained escaped semi-volatile species from the primary organic aerosols. In the CAMSRA aerosol module, the optical properties and mass of hydrophilic organics were assumed to change with relative humidity changes.80 In CAMSRA, optical properties of organic aerosols with respect to humidity are calculated using the OPAC (Optical Properties of Aerosols and Clouds) dataset.80 The used basic assumptions for the simulations of organics are based on the continental mixture described by Hess et al.,81 which is composed of 13% in-soluble species, 84% soluble species, and 3% soot particles.80
MERRA-2.
The absolute relative error in MERRA-2 derived BC mass concentrations was negatively correlated with SO42− normalized with the BC mass concentrations (R = −0.57, p < 0.05). The observed negative correlation indicated that MERRA-2 simulate well aging of BC particles with the secondary sulfate ion. The SO42−/BC ratio also increases with the dominance of fossil fuel combustion.93 The negative correlation also indicated that the absolute relative error increases with an increase in BC emissions from biomass/biofuel burning. Although agricultural residue burning is the dominant emission source, open burning is expected to be well constrained in MERRA-2 simulations similar to CAMSRA because it also considers the fire radiative power approach.27,58 Thus, it is likely that the propagated errors were mainly due to the household biomass/biofuel emissions, as mentioned earlier.
In the case of OC, the absolute relative errors were not significantly correlated with these hydrophilic chemical ions (SO42−, NO3−, NH4+, and Cl−). Interestingly, absolute relative errors were negatively correlated with WSOC/OC. These results showed that the underestimation of OC was mainly due to hydrophobic constituents, which are mainly emitted from primary combustion activities.61,94
(b) Central-IGP.
As discussed earlier, both the reanalysis products overestimated the BC mass concentrations, while OC mass concentrations were overestimated by CAMSRA and underestimated by MERRA-2. Overall, the estimated error for MERRA-2 was small compared with that of CAMSRA with a high FAC2 value above 60%, suggesting a better simulation of carbonaceous aerosols. The influence of different atmospheric processes on reanalysis data is discussed below:
CAMSRA.
At Kanpur (S2) and Allahabad (S3), the absolute relative error of BC was moderately to strongly correlated with SO42−, NO3−, NH4+, and/or Cl− normalized with BC, respectively (R > 0.3, p < 0.05). The positive correlation depicted that the error could be due to the aging of BC with secondary inorganic aerosol species. Moreover, the concentrations of SO42−, NO3−, NH4+, and Cl− increase with combustion activities. The poorly constrained emissions could also be one of the reasons for the overestimation of BC. The Cl−/OC and Cl−/BC ratios were studied to identify the most appropriate combustion sources and compared with the values given by Andreae.95 The HCl/OC and HCl/BC ratios for different emission sources are shown in Table S2 in the ESI.† During the sampling campaigns at Kanpur (Allahabad), the ratio of Cl−/OC and Cl−/BC was observed to be in the range of 0.001–0.40 (0.03–0.15) and 0.01–4.79 (0.16–0.76), respectively. The ratios showed a complex mixture of combustion sources over Kanpur. A detailed discussion on all possible emission sources over Kanpur is reported elsewhere.41,42 The dominant sources are biofuel, open agricultural residue, and garbage burning over Allahabad. In contrast, the association between absolute relative errors of BC and the above-mentioned chemicals was insignificant over Lumbini. These insignificant associations could be due to prevalent foggy conditions, as measurement campaigns focused on foggy days of cold winter months. The foggy conditions can impair visibility and local meteorology,96 negatively impacting satellite retrievals of aerosol products. We have collected the MODIS (Moderate Resolution Imaging Spectroradiometer) Terra and Aqua aerosol optical depth data (collection 6.1 having 3 km spatial resolution) during the sampling campaigns at Lumbini to check this assumption. The MODIS aerosol optical depth information was missing for approximately 80% of sampling days. This gave strong evidence that the prevalent foggy conditions negatively impacted the satellite retrievals. Thus, it is inferred that satellite retrievals play an essential role in the reanalysis data.
Similar to the NW-IGP, the absolute relative errors of OC were significantly correlated with those of the ALWC (R = 0.62, p < 0.05) over Kanpur. As mentioned earlier, the positive association depicted that the discrepancies were mainly due to the particle growth scheme regarding increasing relative humidity.80 In contrast, an insignificant relation was observed between absolute relative errors of OC and the ALWC over Allahabad and Lumbini. The insignificant association gave evidence that the particle growth scheme could not be an important reason for the overestimation over Allahabad and Lumbini. However, positive association with WSOC normalized with OC (R > 0.40, p < 0.05) showed that the propagated errors were due to hydrophilic fractions of organic aerosols. These results suggested that the aging scheme of hydrophobic-to-hydrophilic organics could also be one of the important reasons for the biases in CAMSRA data. It is worth mentioning that the discrepancies in the aging scheme of OC could not directly impact the total mass concentrations; however, indirectly, it can have an impact through a wet deposition scheme.
MERRA-2.
At Kanpur, the absolute relative errors of BC were positively correlated with NO3−, NH4+, and Cl− normalized with BC (R > 0.67, p < 0.05). The positive correlation depicted that the overestimation of BC was due to uncertain quantification of the aging of BC with secondary inorganic aerosol species. In addition, overestimated emissions could also be one of the important reasons. The results were identical to those observed for CAMSRA. In contrast, the correlation was insignificant over Allahabad and Lumbini, either due to prevalent foggy conditions that can impair the visibility, local meteorology, or satellite retrievals.96 A similar trend was also observed for the OC mass concentration data over Allahabad and Lumbini.
In Kanpur, the absolute relative error of OC was significantly correlated with WSOC/OC (R = 0.33, p < 0.05), which depicted that the induced error could be mainly due to hydrophilic fractions of organic aerosols. The global models generally parameterize the hydrophobic-to-hydrophilic carbonaceous aerosols as an exponential turnover with an approximate e-folding time of few days.97 For instance, the aerosol module of MERRA-2 (or GOCART) simulations considers 50% of emitted OC as hydrophobic, which undergo an aging process to become hydrophilic in 1.2 days.27,61,94 This hydrophilic fraction of OC is further assumed to be removed from the atmosphere through dry and wet scavenging with an overall lifetime of five days.61 The observed results suggested that the increase in hydrophilic fractions of OC leads to an increase in the underestimation of OC. Therefore, the most plausible reasons for the uncertainty could be: (1) emitted OC could have a higher (>50%) fraction of hydrophobic constituents, (2) e-fold time for the conversion of hydrophobic-to-hydrophilic fraction could be lower, and (3) the lifetime of OC could be higher over the central-IGP.
Moreover, earlier studies on organic aerosols over Kanpur gave clear evidence of enhancement of SOA formation because of (1) aqueous-phase processing, (2) nocturnal NOx radical initiated oxidation, and (3) the acid-catalyzed reaction during winter.82,83,98 It is expected that these processes could also lead to uncertainty in the MERRA-2 reanalysis. The negative correlation of the absolute relative error of OC with the ALWC and acidic anions (SO42− and NO3−) depicted that the processes mentioned above did not significantly affect the MERRA-2 simulations of OC.
The availability of hourly (from 8:30 to 18:30 IST) measured BC and OC mass concentrations allowed us to study the diurnal variation of measured and simulated carbonaceous aerosol mass concentrations. The diurnal variations of MERRA-2 and CAMSRA derived carbonaceous aerosols are shown in Fig. 3 and S5,† respectively. Interestingly, the average MERRA-2 simulated BC mass concentrations during different times showed good agreement with the average BC mass concentrations compared with the OC mass concentrations. MERRA-2 underestimates the BC mass concentrations before noon while overestimates in the afternoon. However, a direct comparison of the average measured and simulated carbonaceous aerosol mass concentrations and articulating a strong association could be misleading since the biases are positive and negative in both directions. Therefore, the relative error was calculated for each data point and is shown in Fig. S6 in the ESI.† The amplitude of the relative error was observed to be the highest during the afternoon when average BC and OC mass concentrations were in good agreement with MERRA-2.
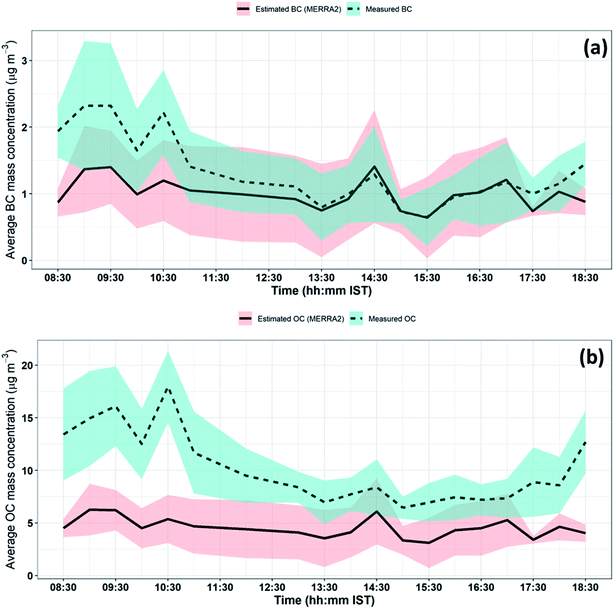 |
| Fig. 3 Diurnal variation of ground-based measured BC and OC mass concentrations along with MERRA-2 simulated mass concentrations of BC and OC over the Kanpur. The shaded area represents the standard deviations. (a) represents diurnal variations of BC, and (b) represents diurnal variations of OC. | |
4. Conclusion
In the present study, two widely used CAMSRA and MERRA-2 reanalysis data sets of carbonaceous aerosol mass concentrations were validated against ground-based measured data over different IGP locations. Moreover, efforts were made to identify possible reasons for error propagations in the reanalysis data of BC and OC mass concentrations, emphasizing fresh vs. aged aerosols.
Both CAMSRA and MERRA-2 underestimated the BC mass concentrations over the NW-IGP while overestimated over the central-IGP. The results suggested that primary emissions, especially household biofuel/biomass burning, were underestimated over the NW-IGP. In contrast, primary emissions were overestimated over the central-IGP. Emission sources over the central-IGP are complex; therefore, it is difficult to quantify the emission source/sources, which is/are responsible for the error propagation in the reanalysis data. Besides poorly constrained complex emissions, the aging of BC also led to the overestimation of BC sources over the central-IGP.
For OC, observed errors were complex mainly because of biases associated with the local emissions and atmospheric processes. Overall, CAMSRA overestimated the OC mass concentrations over both the IGP regions with a mean bias ranging from 28 to 84 μg m−3. The observed plausible reason was the poorly constrained hygroscopic growth scheme in the aerosol module, besides poorly constrained repartitioned semi-volatile and intermediate-volatile species from the primary organic aerosols. MERRA-2 underestimated the OC mass concentrations throughout the sampling campaigns with a mean bias range of −6 to −22 μg m−3. Over the NW-IGP, underestimation was mainly due to hydrophobic constituents of primary emissions, which are primarily due to household biofuel/biomass burning.
In contrast, the underestimation of OC over the central-IGP was due to the aging scheme in the aerosol module. Moreover, underestimation of organics could also be due to the formation of SOAs in the atmosphere from the regionally transported gas-phase “pool” of efficient precursors. It is worth mentioning that there is a lack of simultaneous and real-time ambient Volatile Organic Compounds (VOC) and OC measurements in the IGP. Besides the above mentioned possible reasons influencing reanalysis data of carbonaceous aerosols, satellite retrieval of aerosol products also plays a crucial role in accurate simulations of carbonaceous aerosols.
Although the above mentioned atmospheric processes cannot directly be utilized for the current parameterization of carbonaceous aerosols, it is expected that these mechanisms might be helpful for the future parameterization of carbonaceous aerosols over South Asia. Further, there is also a need to have better alternative ways to estimate secondary organic aerosols in the absence of highly time-resolved mass spectrometric measurements and the need to employ such advanced tools to unravel novel aerosol processing mechanisms is also suggested.
Author Contributions
AS and TG conceptualized the paper, AS wrote the manuscript with input and data collected by all the co-authors, and all co-authors reviewed the results and analysis and provided significant comments and edits to improve the manuscript.
Conflicts of interest
There are no conflicts to declare.
Acknowledgements
The authors are grateful to the ECMWF for providing CAMS data; and GMAO for providing MERRA-2 datasets. Saifi Izhar acknowledges ICIMOD for providing the research grant to work in Lumbini, Nepal. Tarun Gupta acknowledges funds provided by Indian National Science Academy (SP/YSP/113/2015(1091)). The authors express sincere thanks to three anonymous reviewers for critical evaluation and constructive suggestions to improve the manuscript. We appreciate Prof. Lin Wang and Prof. Nønne Prisle for their kind editorial handling.
References
- A. Soni, U. Kumar, V. Prabhu and V. Shridhar, Characterization, Source Apportionment and Carcinogenic Risk Assessment of Atmospheric Particulate Matter at Dehradun, situated in the Foothills of Himalayas, J. Atmos. Sol.-Terr. Phys, 2020, 199, 105205 CrossRef CAS.
- P. Rajeev, P. Rajput, D. K. Singh, A. K. Singh and T. Gupta, Risk assessment of submicron PM-bound hexavalent chromium during wintertime, Hum. Ecol. Risk Assess., 2018, 24(6), 1453–1463 CrossRef CAS.
- Z. Li, Y. Wang, J. Guo, C. Zhao, M. C. Cribb and X. Dong,
et al., East Asian Study of Tropospheric Aerosols and their Impact on Regional Clouds, Precipitation, and Climate (EAST-AIRCPC), J. Geophys. Res.: Atmos., 2019, 124(23), 13026–13054 CAS.
- X. Wang, C. Wang, J. Wu, G. Miao, M. Chen and S. Chen,
et al., Intermediate Aerosol Loading Enhances Photosynthetic Activity of Croplands, Geophys. Res. Lett., 2021, 48(7), 1–12 Search PubMed.
-
IPCC. Climate Change 2013: The Physical Science Basis. Contribution of Working Group I to the Fifth Assessment Report of the Intergovernmental Panel on Climate Change, Summary for Policymakers, ed. Stocker, T. F., Qin, D., Plattner, G.-K., Tignor, M., Allen, S. K., Boschung, J., Nauels, A., Xia, Y., Bex, V. and Midgley, P. M., Cambridge University Press, Cambridge, United Kingdom and New York, NY, USA, 2013 Search PubMed.
- N. M. Donahue, A. L. Robinson and S. N. Pandis, Atmospheric organic particulate matter: From smoke to secondary organic aerosol, Atmos. Environ., 2009, 43(1), 94–106 CrossRef CAS.
- A. L. Robinson, N. M. Donahue, M. K. Shrivastava, E. A. Weitkamp, A. M. Sage and A. P. Grieshop,
et al., Rethinking organic aerosols: Semivolatile emissions and photochemical aging, Science, 2007, 315(5816), 1259–1262 CrossRef CAS.
- R. K. Pathak, T. Wang, K. F. Ho and S. C. Lee, Characteristics of summertime PM2.5 organic and elemental carbon in four major Chinese cities: Implications of high acidity for water-soluble organic carbon (WSOC), Atmos. Environ., 2011, 45(2), 318–325 CrossRef CAS.
- V. Ramanathan and G. Carmichael, Global and regional climate changes due to black carbon, Nat. Geosci., 2008, 1, 221–227 CrossRef CAS.
- A. Soni, S. Decesari, V. Shridhar, V. Prabhu, P. Panwar and A. Marinoni, Investigation of potential source regions of atmospheric Black Carbon in the data deficit region of the western Himalayas and its foothills, Atmos. Pollut. Res., 2019 Aug 1, 10(6), 1832–1842 Search PubMed.
- S. Tiwari, U. C. Dumka, D. G. Kaskaoutis, K. Ram, A. S. Panicker and M. K. Srivastava,
et al., Aerosol chemical characterization and role of carbonaceous aerosol on radiative effect over Varanasi in central Indo-Gangetic Plain, Atmos. Environ., 2016, 125, 437–449 CrossRef CAS.
- M. Arif and S. Parveen, Carcinogenic effects of indoor black carbon and particulate matters (PM2.5 and PM10) in rural households of India, Environ. Sci. Pollut. Res., 2021, 28(2), 2082–2096 CrossRef CAS PubMed.
- V. Choudhary, G. K. Singh, T. Gupta and D. Paul, Absorption and radiative characteristics of brown carbon aerosols during crop residue burning in the source region of Indo-Gangetic Plain, Atmos. Res., 2021, 249, 105285 CrossRef CAS.
- V. Choudhary, P. Rajput, D. K. Singh, A. K. Singh and T. Gupta, Light absorption characteristics of brown carbon during foggy and non-foggy episodes over the Indo-Gangetic Plain, Atmos. Pollut. Res., 2018, 9(3), 494–501 CrossRef CAS.
- N. Singh, A. Mhawish, T. Banerjee, S. Ghosh, R. S. Singh and R. K. Mall, Association of aerosols, trace gases and black carbon with mortality in an urban pollution hotspot over central Indo-Gangetic Plain, Atmos. Environ., 2020, 246, 118088 CrossRef.
- S. Izhar, T. Gupta and A. K. Panday, Improved method to apportion optical absorption by black and brown carbon under the influence of haze and fog at Lumbini, Nepal, on the Indo-Gangetic Plains, Environ. Pollut., 2020, 263, 114640 CrossRef CAS.
- G. K. Singh, V. Choudhary, P. Rajeev, D. Paul and T. Gupta, Understanding the origin of carbonaceous aerosols during periods of extensive biomass burning in northern India, Environ. Pollut., 2020, 116082 Search PubMed.
- W. A. Lahoz and P. Schneider, Data assimilation: Making sense of Earth Observation, Front. Environ. Sci., 2014, 2, 1–28 Search PubMed.
- K. E. Trenberth and J. G. Olson, An Evaluation and Intercomparison of Global Analyses from the National Meteorological Center and the European Centre for Medium Range Weather Forecasts, Bull. Am. Meteorol. Soc., 1988, 69(9), 1047–1057 CrossRef.
- L. Bengtsson and J. Shukla, Integration of space and in situ observations to study global climate change, Bull. Am. Meteorol. Soc., 1988, 69(10), 1130–1143 CrossRef.
- V. Prabhu, A. Soni, S. Madhwal, A. Gupta, S. Sundriyal and V. Shridhar,
et al., Black carbon and biomass burning associated high pollution episodes observed at Doon valley in the foothills of the Himalayas, Atmos. Res., 2020, 243, 105001 CrossRef CAS.
- C. Zhao, Y. Lin, F. Wu, Y. Wang, Z. Li and D. Rosenfeld,
et al., Enlarging Rainfall Area of Tropical Cyclones by Atmospheric Aerosols, Geophys. Res. Lett., 2018, 45(16), 8604–8611 CrossRef.
- C. Textor, M. Schulz, S. Guibert, S. Kinne, Y. Balkanski and S. Bauer,
et al., Analysis and quantification of the diversities of aerosol life cycles within AeroCom, Atmos. Chem. Phys., 2006, 6(7), 1777–1813 CrossRef CAS.
- S. Kinne, M. Schulz, C. Textor, S. Guibert, Y. Balkanski and S. E. Bauer,
et al., An AeroCom initial assessment - Optical properties in aerosol component modules of global models, Atmos. Chem. Phys., 2006, 6(7), 1815–1834 CrossRef CAS.
- A. Benedetti, J. J. Morcrette, O. Boucher, A. Dethof, R. J. Engelen and M. Fisher,
et al., Aerosol analysis and forecast in the European Centre for Medium-Range Weather Forecasts integrated forecast system: 2. data assimilation, J. Geophys. Res.: Atmos., 2009, 114(13), 1–18 Search PubMed.
- N. A. J. Schutgens, T. Miyoshi, T. Takemura and T. Nakajima, Applying an ensemble Kalman filter to the assimilation of AERONET observations in a global aerosol transport model, Atmos. Chem. Phys., 2010, 10(5), 2561–2576 CrossRef CAS.
- C. A. Randles, A. M. da Silva, V. Buchard, P. R. Colarco, A. Darmenov and R. Govindaraju,
et al., The MERRA-2 aerosol reanalysis, 1980 onward. Part I: System description and data assimilation evaluation, J. Clim., 2017, 30(17), 6823–6850 CrossRef CAS.
- C. A. Gueymard and D. Yang, Worldwide validation of CAMS and MERRA-2 reanalysis aerosol optical depth products using 15 years of AERONET observations, Atmos. Environ., 2020, 225, 117216 CrossRef CAS.
- M. Aldabash, F. B. Balcik and P. Glantz, Validation of MODIS C6.1 and MERRA-2 AOD using AERONET observations: A comparative study over Turkey, Atmosphere, 2020, 11(9), 905 CrossRef.
- A. Misra, S. Tripathi, H. Sembhi and H. Boesch, Validation of CAMS AOD using AERONET Data and Trend Analysis at Four Locations in the Indo-Gangetic Basin, Ann. Geophys., 2020, 1–25 Search PubMed.
- T. Zhang, L. Zang, F. Mao, Y. Wan and Y. Zhu, Evaluation of Himawari-8/AHI, MERRA-2, and CAMS aerosol products over China, Remote Sens., 2020, 12(10), 1–16 Search PubMed.
- M. Chin, T. Diehl, Q. Tan, J. M. Prospero, R. A. Kahn and L. A. Remer,
et al., Multi-decadal aerosol variations from 1980 to 2009: A perspective from observations and a global model, Atmos. Chem. Phys., 2014, 14(7), 3657–3690 CrossRef.
- V. Buchard, A. M. da Silva, C. A. Randles, P. Colarco, R. Ferrare and J. Hair,
et al., Evaluation of the surface PM2.5 in Version 1 of the NASA MERRA Aerosol Reanalysis over the United States, Atmos. Environ., 2016, 125, 100–111 CrossRef CAS.
- S. Provençal, V. Buchard, A. M. da Silva, R. Leduc and N. Barrette, Evaluation of PM surface concentrations simulated by Version 1 of NASA's MERRA Aerosol Reanalysis over Europe, Atmos. Pollut. Res., 2017, 8(2), 374–382 CrossRef PubMed.
- X. Ma, P. Yan, T. Zhao, X. Jia, J. Jiao and Q. Ma,
et al., Evaluations of Surface PM 10 Concentration and Chemical Compositions in MERRA-2 Aerosol Reanalysis over Central and Eastern China, Remote Sens., 2021, 13(7), 1317 CrossRef.
-
Y. Christophe, M. Schulz, Y. Bennouna, H. J. Eskes, S. Basart, A. Benedictow, A.-M. Blechschmidt, S. Chabrillat, H. Clark, E. Cuevas, H. Flentje, K. M. Hansen, U. Im, J. Kapsomenakis, B. Langerock, K. Petersen, A. Richter, N. Sudarchikova, V. Thouret, A. Wagner, Y. Wang, T. Warneke and C. Zerefos, Validation Report for the CAMS Global Reanalyses of Aerosols and Reactive Trace Gases, years 2003–2018, Copernicus Atmosphere Monitoring Service (CAMS) report, May 2019, available from: https://atmosphere.copernicus.eu/sites/default/files/2019-06/CAMS84_2018SC1_D5.1.1-<?pdb_no 2018?>2018<?pdb END?>_v1.pdf Search PubMed.
- A. Saikia, B. Pathak, P. Singh, P. K. Bhuyan and B. Adhikary, Multi-model evaluation of meteorological drivers, air pollutants and quantification of emission sources over the upper Brahmaputra basin, Atmosphere, 2019, 10(11), 703 CrossRef CAS.
- B. Pathak, P. K. Bhuyan, A. Saikia, K. Bhuyan, P. Ajay and S. J. Nath,
et al., Impact of lockdown due to COVID-19 outbreak on O3 and its precursor gases, PM and BC over northeast India, Curr. Sci., 2021, 120(2), 322–331 CrossRef CAS.
- P. Rajput, M. Sarin, D. Sharma and D. Singh, Characteristics and emission budget of carbonaceous species from post-harvest agricultural-waste burning in source region of the Indo-Gangetic plain, Tellus, Ser. B: Chem. Phys. Meteorol., 2014, 66(1), 21026 CrossRef.
- G. K. Singh, V. Choudhary, T. Gupta and D. Paul, Investigation of size distribution and mass characteristics of ambient aerosols and their combustion sources during post-monsoon in northern India, Atmos. Pollut. Res., 2020, 11(1), 170–178 CrossRef CAS.
- P. Rai, A. Chakraborty, A. K. Mandariya and T. Gupta, Composition and source apportionment of PM1 at urban site Kanpur in India using PMF coupled with CBPF, Atmos. Res., 2016, 178–179, 506–520 CrossRef CAS.
-
N. Rastogi, Using Chemical Characterization Data to Apportion and Locate Sources of Air Pollutants and to Study Mixing State of Atmospheric Particles during Dust Storms, M.Tech. thesis, Indian Institute of Technology Kanpur, 2013 Search PubMed.
- A. Chakraborty, A. K. Mandariya, R. Chakraborti, T. Gupta and S. N. Tripathi, Realtime chemical characterization of post monsoon organic aerosols in a polluted urban city: Sources, composition, and comparison with other seasons, Environ. Pollut., 2018, 232, 310–321 CrossRef CAS PubMed.
- G. K. Singh, P. Rajeev, D. Paul and T. Gupta, Chemical characterization and stable nitrogen isotope composition of nitrogenous component of ambient aerosols from Kanpur in the Indo-Gangetic Plains, Sci. Total Environ., 2020, 763, 143032 CrossRef.
- S. K. Guttikunda, R. Goel and P. Pant, Nature of air pollution, emission sources, and management in the Indian cities, Atmos. Environ., 2014, 95, 501–510 CrossRef CAS.
- P. Rajeev, A. Kumar, G. Kumar, R. Chandra and T. Gupta, Chemical characterization , source identification and health risk assessment of polycyclic aromatic hydrocarbons in ambient particulate matter over central Indo-Gangetic Plain, Urban Clim., 2021, 35, 100755 CrossRef.
- P. Chen, C. Li, S. Kang, F. Yan, Q. Zhang and Z. Ji,
et al., Source apportionment of particle-bound polycyclic aromatic hydrocarbons in Lumbini, Nepal by using the positive matrix factorization receptor model, Atmos. Res., 2016, 182, 46–53 CrossRef CAS.
- L. Tripathee, S. Kang, D. Rupakheti, Z. Cong, Q. Zhang and J. Huang, Chemical characteristics of soluble aerosols over the central Himalayas: insights into spatiotemporal variations and sources, Environ. Sci. Pollut. Res., 2017, 24(31), 24454–24472 CrossRef CAS.
- S. Izhar, T. Gupta and A. K. Panday, Scavenging efficiency of water soluble inorganic and organic aerosols by fog droplets in the Indo Gangetic Plain, Atmos. Res., 2020, 235, 104767 CrossRef CAS.
- A. Kumar and T. Gupta, Development and field evaluation of a multiple slit nozzle-based high volume PM2.5 inertial impactor assembly (HVIA), Aerosol Air Qual. Res., 2015, 15(4), 1188–1200 CrossRef.
- A. Kumar and T. Gupta, Development and laboratory performance evaluation of a variable configuration PM1/PM2.5 impaction-based sampler, Aerosol Air Qual. Res., 2015, 15(3), 768–775 CrossRef.
- J. C. Chow, J. G. Watson, D. Crow, D. H. Lowenthal and T. Merrifield, Comparison of IMPROVE and NIOSH Carbon Measurements, Aerosol Sci. Technol., 2001, 34(1), 23–34 CrossRef CAS.
- J. C. Chow, J. G. Watson, L. W. A. Chen, M. C. O. Chang, N. F. Robinson and D. Trimble,
et al., The IMPROVE_A temperature protocol for thermal/optical carbon analysis: Maintaining consistency with a long-term database, J. Air Waste Manage. Assoc., 2007, 57(9), 1014–1023 CrossRef CAS.
- J. C. Chow, X. Wang, B. J. Sumlin, S. B. Gronstal, L. W. Antony Chen and D. L. Trimble,
et al., Optical calibration and equivalence of a multiwavelength thermal/optical carbon analyzer, Aerosol Air Qual. Res., 2015, 15(4), 1145–1159 CrossRef.
- A. Inness, M. Ades, A. Agusti-Panareda, J. Barré, A. Benedictow and A.-M. Blechschmidt,
et al., The CAMS reanalysis of atmospheric composition, Atmos. Chem. Phys. Discuss., 2019, 19, 3515–3556 CrossRef CAS.
- C. Granier, B. Bessagnet, T. Bond, A. D'Angiola, H. D. van der Gon and G. J. Frost,
et al., Evolution of anthropogenic and biomass burning emissions of air pollutants at global and regional scales during the 1980-2010 period, Clim. Change, 2011, 109(1), 163–190 CrossRef CAS.
- D. V. Spracklen, J. L. Jimenez, K. S. Carslaw, D. R. Worsnop, M. J. Evans and G. W. Mann,
et al., Aerosol mass spectrometer constraint on the global secondary organic aerosol budget, Atmos. Chem. Phys., 2011, 11(23), 12109–12136 CrossRef CAS.
- J. W. Kaiser, A. Heil, M. O. Andreae, A. Benedetti, N. Chubarova and L. Jones,
et al., Biomass burning emissions estimated with a global fire assimilation system based on observed fire radiative power, Biogeosciences, 2012, 9(1), 527–554 CrossRef CAS.
- R. Gelaro, W. McCarty, M. J. Suárez, R. Todling, A. Molod and L. Takacs,
et al., The modern-era retrospective analysis for research and applications, version 2 (MERRA-2), J. Clim., 2017, 30(14), 5419–5454 CrossRef PubMed.
- P. Colarco, A. Da Silva, M. Chin and T. Diehl, Online simulations of global aerosol distributions in the NASA GEOS-4 model and comparisons to satellite and ground-based aerosol optical depth, J. Geophys. Res.: Atmos., 2010, 115(14), D14207 CrossRef.
- M. Chin, P. Ginoux, S. Kinne, O. Torres, B. N. Holben and B. N. Duncan,
et al., Tropospheric aerosol optical thickness from the GOCART model and comparisons with satellite and sun photometer measurements, J. Atmos. Sci., 2002, 59, 461–483 CrossRef.
- A. Guenther, C. Nicholas, R. Fall, L. Klinger, W. A. Mckay and B. Scholes, A global model of natural volatile organic compound emissions, J. Geophys. Res., 1995, 100(94), 8873–8892 CrossRef CAS.
- T. Diehl, A. Heil, M. Chin, X. Pan, D. Streets, M. Schultz and S. K. Anthropogenic, biomass burning, and volcanic emissions of black carbon, organic carbon, and SO2 from 1980 to 2010 for hindcast model experiments, Atmos. Chem. Phys. Discuss., 2012, 12(9), 24895–24954 Search PubMed.
-
A. Darmenov and A. M. da Silva, Quick Fire Emissions Dataset (QFED): Documentation of Versions 2.1, 2.2 and 2.4, 2015, available from: https://gmao.gsfc.nasa.gov/pubs/docs/Darmenov796.pdf Search PubMed.
-
C. A. Randles, A. M. da Silva, V. Buchard, A. Darmenov, P. R. Colarco, V. Aquila, H. Bian, E. P. Nowottnick, X. Pan, A. Smirnov, H. Yu and R. Govindaraju, The MERRA-2 Aerosol Assimilation, 2016, available from: https://gmao.gsfc.nasa.gov/pubs/docs/Randles887.pdf Search PubMed.
- H. Hersbach, B. Bell, P. Berrisford, S. Hirahara, A. Horányi and J. Muñoz-Sabater,
et al., The ERA5 global reanalysis, Q. J. R. Meteorol. Soc., 2020, 146(730), 1999–2049 CrossRef.
- L. Hoffmann, G. Günther, D. Li, O. Stein, X. Wu and S. Griessbach,
et al., From ERA-Interim to ERA5: The considerable impact of ECMWF's next-generation reanalysis on Lagrangian transport simulations, Atmos. Chem. Phys., 2019, 19(5), 3097–3214 CrossRef CAS.
- Y. Xia, Y. Hu, Y. Huang, C. Zhao, F. Xie and Y. Yang, Significant Contribution of Severe Ozone Loss to the Siberian-Arctic Surface Warming in Spring 2020, Geophys. Res. Lett., 2021, 48(8), 1–9 CrossRef.
- Y. Sun and C. Zhao, Influence of Saharan Dust on the Large-Scale Meteorological Environment for Development of Tropical Cyclone Over North Atlantic Ocean Basin, J. Geophys. Res.: Atmos., 2020, 125(23), 1–14 Search PubMed.
- S. Izhar, T. Gupta, A. M. Qadri and A. K. Panday, Wintertime chemical characteristics of aerosol and their role in light extinction during clear and polluted days in rural Indo Gangetic plain, Environ. Pollut., 2021, 282, 117034 CrossRef CAS.
- K. E. Taylor, Summarizing multiple aspects of model performance in a single diagram, J. Geophys. Res., 2001, 106(D7), 7183–7192 CrossRef.
- A. Pozzer, A. De Meij, K. J. Pringle, H. Tost, U. M. Doering and J. Van Aardenne,
et al., Distributions and regional budgets of aerosols and their precursors simulated with the EMAC chemistry-climate model, Atmos. Chem. Phys., 2012, 12(2), 961–987 CrossRef CAS.
- Q. You, Y. Jiao, H. Lin, J. Min, S. Kang and G. Ren,
et al., Comparison of NCEP/NCAR and ERA-40 total cloud cover with surface observations over the Tibetan Plateau, Int. J. Climatol., 2014, 34(8), 2529–2537 CrossRef.
- J. L. F. Li, D. E. Waliser, G. Stephens, S. Lee, T. L'Ecuyer and S. Kato,
et al., Characterizing and understanding radiation budget biases in CMIP3/CMIP5 GCMs, contemporary GCM, and reanalysis, J. Geophys. Res.: Atmos., 2013, 118(15), 8166–8184 Search PubMed.
- S. D. Sanap, G. Pandithurai and M. G. Manoj, On the response of Indian summer monsoon to aerosol forcing in CMIP5 model simulations, Clim. Dynam., 2015, 45(9–10), 2949–2961, DOI:10.1007/s00382-015-2516-2.
- J. Prakash, A. Singhai, G. Habib, R. S. Raman and T. Gupta, Chemical characterization of PM1.0 aerosol in Delhi and source apportionment using positive matrix factorization, Environ. Sci. Pollut. Res., 2017, 24(1), 445–462 CrossRef.
- N. Rastogi, A. Singh, M. M. Sarin and D. Singh, Temporal variability of primary and secondary aerosols over northern India: Impact of biomass burning emissions, Atmos. Environ., 2016, 125, 396–403 CrossRef CAS.
- S. E. Puliafito, D. G. Allende, P. S. Castesana and M. F. Ruggeri, High-resolution atmospheric emission inventory of the argentine energy sector. Comparison with edgar global emission database, Heliyon, 2017, 3(12), e00489 CrossRef PubMed.
- D. T. Kleist, D. F. Parrish, J. C. Derber, R. Treadon, W. S. Wu and S. Lord, Introduction of the GSI into the NCEP global data assimilation system, Weather Forecast., 2009, 24(6), 1691–1705 CrossRef.
-
A. Bozzo, S. Remy, A. Benedetti, J. Flemming, P. Bechtold and M. J. Rodwell, et al., Implementation of a CAMS-Based Aerosol Climatology in the IFS, 2017, available from: http://www.ecmwf.int/en/research/publications Search PubMed.
- M. Hess, P. Koepke and I. Schult, Optical Properties of Aerosols and Clouds: The Software Package OPAC, Bull. Am. Meteorol. Soc., 1998, 79(5), 831–844 CrossRef.
- A. Chakraborty, P. Rajeev, P. Rajput and T. Gupta, Water soluble organic aerosols in indo gangetic plain (IGP): Insights from aerosol mass spectrometry, Sci. Total Environ., 2017, 599–600, 1573–1582 CrossRef CAS PubMed.
- A. K. Mandariya, T. Gupta and S. N. Tripathi, Effect of aqueous-phase processing on the formation and evolution of organic aerosol (OA) under different stages of fog life cycles, Atmos. Environ., 2019, 206, 60–71 CrossRef CAS.
- N. L. Ng, J. H. Kroll, A. W. H. Chan, P. S. Chhabra, R. Flagan and J. H. Seinfeld, Secondary organic aerosol formation from m-xylene, toluene, and benzene, Atmos. Chem. Phys., 2007, 7(3), 3909–3922 CrossRef CAS.
- R. J. Weber, A. P. Sullivan, R. E. Peltier, A. Russell, B. Yan and M. Zheng,
et al., A study of secondary organic aerosol formation in the anthropogenic-influenced southeastern United States, J. Geophys. Res.: Atmos., 2007, 112(13), 1–13 Search PubMed.
- J. A. de Gouw, A. M. Middlebrook, C. Warneke, P. D. Goldan, W. C. Kuster and J. M. Roberts,
et al., Budget of organic carbon in a polluted atmosphere: Results from the New England Air Quality Study in 2002, J. Geophys. Res. Atmos., 2005, 110(16), 1–22 Search PubMed.
- B. Ervens, A. G. Carlton, B. J. Turpin, K. E. Altieri, S. M. Kreidenweis and G. Feingold, Secondary organic aerosol yields from cloud-processing of isoprene oxidation products, Geophys. Res. Lett., 2008, 35(2), 4–8 CrossRef.
- C. Fountoukis and A. Nenes, ISORROPIAII: A computationally efficient thermodynamic equilibrium model for K+-Ca2+-Mg2+-NH4+-Na+-SO42−-NO3−-Cl−-H2O aerosols, Atmos. Chem. Phys., 2007, 7(17), 4639–4659 CrossRef CAS.
- J. C. Chang and S. R. Hanna, Air quality model performance evaluation, Meteorol. Atmos. Phys., 2004, 87(1–3), 167–196 Search PubMed.
- R. C. Moffet and K. A. Prather,
In situ measurements of the mixing state and optical properties of soot with implications for radiative forcing estimates, Proc. Natl. Acad. Sci. U. S. A., 2009, 106(29), 11872–11877 CrossRef CAS.
-
J. H. Seinfeld and S. N. Pandis, Atmospheric Chemistry and Physics from Air Pollution to Climate Change, Wiley, Chichester, 3rd edn, 2016 Search PubMed.
- K. Deetz, H. Vogel, S. Haslett, P. Knippertz, H. Coe and B. Vogel, Aerosol liquid water content in the moist southern West African monsoon layer and its radiative impact, Atmos. Chem. Phys., 2018, 18(19), 14271–14295 CrossRef CAS.
- T. Novakov, M. O. Andreae, R. Gabriel, T. W. Kirchstetter, O. L. Mayol-Bracero and V. Ramanathan, Origin of carbonaceous aerosols over the tropical Indian Ocean: Biomass burning or fossil fuels?, Geophys. Res. Lett., 2000, 27(24), 4061–4064 CrossRef CAS.
- W. F. Cooke, C. Liousse, H. Cachier and F. Radioactivit, Construction of a 1° × 1° fossil fuel emission data set for carbonaceous aerosol and implementation and radiative impact in the ECHAM4 model, J. Geophys. Res.: Atmos., 1999, 104(D18), 137–162 CrossRef.
- M. O. Andreae, Emission of trace gases and aerosols from biomass burning - An updated assessment, Atmos. Chem. Phys., 2019, 19(13), 8523–8546 CrossRef CAS.
- P. Rajput, A. Mandaria, L. Kachawa, D. K. Singh, A. K. Singh and T. Gupta, Chemical characterisation and source apportionment of PM1 during massive loading at an urban location in Indo-Gangetic Plain: Impact of local sources and long-range transport, Tellus, Ser. B: Chem. Phys. Meteorol., 2016, 68(1), 30659 CrossRef.
- M. Kanakidou, J. H. Seinfeld, S. N. Pandis, I. Barnes, F. J. Dentener and M. C. Facchini,
et al., Organic aerosol and global climate modelling: a review, Atmos. Chem. Phys. Discuss., 2004, 5, 1053–1123 CrossRef.
- D. S. Kaul, T. Gupta, S. N. Tripathi, V. Tare and J. L. Collett, Secondary organic aerosol: A comparison between foggy and nonfoggy days, Environ. Sci. Technol., 2011, 45(17), 7307–7313 CrossRef CAS PubMed.
Footnote |
† Electronic supplementary information (ESI) available. See DOI: 10.1039/d1ea00067e |
|
This journal is © The Royal Society of Chemistry 2021 |
Click here to see how this site uses Cookies. View our privacy policy here.