DOI:
10.1039/D1LC00533B
(Critical Review)
Lab Chip, 2021,
21, 3655-3666
Review: tomographic imaging flow cytometry
Received
16th June 2021
, Accepted 18th August 2021
First published on 13th September 2021
Abstract
Within the last decades, conventional flow cytometry (FC) has evolved as a powerful measurement method in clinical diagnostics, biology, life sciences and healthcare. Imaging flow cytometry (IFC) extends the power of traditional FC by adding high resolution optical and spectroscopic information. However, the conventional IFC only provides a 2D projection of a 3D object. To overcome this limitation, tomographic imaging flow cytometry (tIFC) was developed to access 3D information about the target particles. The goal of tIFC is to visualize surfaces and internal structures in a holistic way. This review article gives an overview of the past and current developments in tIFC.
1 Introduction
Flow cytometry is a long-term established method to perform population analysis of cells and particles based on their optical and spectroscopic properties.1 Imaging flow cytometry (IFC) extends this approach for the acquisition of particle images providing detailed insights into their spatial representation of cellular features and the colocalization of fluorescence signals. Depending on the application, different methods for particle focusing2 and imaging technologies3 can be used. In the 1970s, the research group of Leon Wheeless presented the first imaging flow cytometer.4 Due to technical limitations, it took another three decades until commercial imaging flow cytometers became available. The breakthrough for the commercial use of the IFC was achieved with the introduction of the ImageStream system in 2005.5 Further systems were the Imaging FlowCytobot (IFCB)6 and the FlowCam7 system. To obtain the morphological and spectral data of particles, there is a plethora of different approaches available.8–12 In addition, numerous scientific articles in recent years reflect a continuous progress in the IFC.13–16
However, these systems are limited to a 2D projection of a 3D object. Thus, the relative orientation of the examined particle to the detector limits the information obtained. To overcome this limitation, tIFC was introduced. The goal is the holistic three-dimensional imaging of surfaces and internal structures of particles. In order to obtain spatial information about the objects, they must be captured either as a z-stack, a holographic dataset or as a tilt series of 2D images of a rotating particle. First concepts initially dealt with the capture and induced rotation of single particles. In 1999, Reichle et al.17 captured particles in a 3D micro-electro-octopole. The particle rotation was induced by a rotating electric field in the MHz range. Later in 2013, Chau et al.,18 Benhal et al. (2014)19 and Yang et al. (2018)20 also used alternating electric fields for cell rotation. Huang et al. (2019)21 used a dielectrophoresis-based chip for spatial self-alignment and controlled rotation of cells. With this method, the cells can be rotated in all spatial orientations in a precisely controlled manner. Shelby and Chiu (2004),22 on the other hand, used cavities placed in channels to trap particles and cause them to rotate based on turbulence within the cavities. The introduction and removal of particles into and from the cavities is done by optical traps. Furthermore, rotation of the particles can be achieved by shear flow.23 In shear flow – such as the Poiseuille flow24,25 or Couette flow26,27 – the particles rotate due to the effect of the velocity gradient acting on the particles. Kolb et al.28 used in 2014 a so-called optical flow rotator for particle rotation. Here, the particles are captured by an optical two-beam trap. Since the focus of the optical trap is not located in the center of the channel, particle rotation occurs due to the parabolic velocity profile of the passing medium, which acts unevenly on the particle. The rotation velocity of the particles is precisely controlled by the flow velocity in the microfluidic chip.
The systems described above are capable of capturing particles and inducing particle rotation. However, only one single particle can be captured and measured at a time. Besides the resulting limitation in throughput, particles passing through the flow cell during the measurement are excluded from observation.
Merola et al. (2013)29 also used optical traps to capture sperm cells and rotate them hydrodynamically. By adding a spatial light modulator (SLM) to the system, they were able to trap several spermatozoa simultaneously and image them using digital holography. Bernard et al. (2017)30 utilized surface acoustic waves for the parallel capture and rotation of particles in several pressure nodes. By selective phase shifting between the piezoelectric substrates, a translational particle movement into the pressure nodes (focusing) and a so-called swirling movement (rotation) were generated.
Due to the multitude of publications in the field of tomographic imaging microscopy, the authors have decided to focus this review exclusively on methods in which the spatial information of the particles are obtained by IFC. In the following sections, different methods are briefly described and classified according to their practical application.
2 Particle arrangement
In standard IFC, particles are diluted in an aqueous solution for investigations. Lateral fluid focusing conveys the particles in a single file transportation arrangement at the center of the microfluidic channel. In previous concepts, the particles were made to rotate during image acquisition by a combination of active elements and fluid dynamic effects. Also, the particles have been either captured at once or in arranged cavities.22,31,32 The introduction of external forces into the channel always requires an adjustment of the operating parameters to the properties of the particle suspension. However, high throughput applications require continuous imaging of all particles passing through the flow cell. One option to achieve tomographic imaging of continuously flowing particles is to force their continuous self-rotation in shear flow during image acquisition. This requires the particle transport at a distinct off-center position of the microchannel, which can be realized by a preceding flow focusing step e.g. hydrodynamic33 or acoustophoretic.34 Also, self-alignment of particles in flow can be applied.35
2.1 Rotating particles
The rotation of flowing particles forms one fundamental basis for tIFC. First investigations and mathematical description of particle rotation in shear flow were done by Albert Einstein in 1906.36 This work was extended by Jeffery in 1923.37 He discovered that tumbling objects in the flow move on periodic orbits: the so-called “Jeffery orbits”. A continuous particle rotation can be achieved by focusing a sample stream into the shear field of a parabolic flow profile. The parabolic flow profile in a capillary is characterized by the Hagen–Poiseuille law. The maximum flow velocity is in the center of the channel, while no flow velocity is observed near the channel walls. (Fig. 1). The velocity gradient between these two locations can be used to induce a shear force to a flowing particle focused at an off-center position. The side of the particle closer to the wall experiences a lower flow velocity than the side closer to the center and the resulting shear rate creates a momentum on the particle. A spherical particle rotates at a constant angular velocity of: |  | (1) |
The shear rate
for a spherical particle is calculated by: | 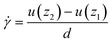 | (2) |
where, d is the particle diameter and u(z2) − u(z1) is the fluid velocity difference between the upper and the lower particle positions. The angular velocity (eqn (1)) of spherical particles is determined by their shear gradient (eqn (2)).
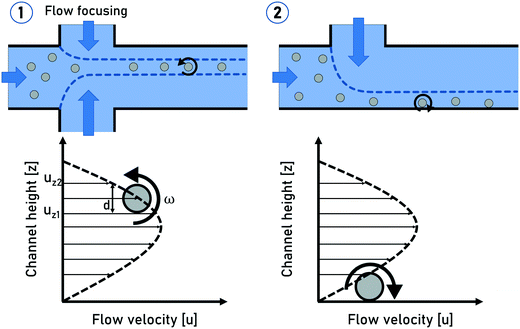 |
| Fig. 1 Schematic sketches of the spherical particle rotation in shear flow (1) and a particle rolling on the channel side wall (2). | |
The generation of particle rotation in microchannels can be realized in a statistic way by guiding all the particles through a capillary without any focusing. The particles will be statistically distributed within the parabolic flow profile and undergo self-rotation with different rotation frequencies depending on their location within the flow profile. With this approach, only particles located at a certain region within the channel will provide a sufficient angular velocity for tomographic imaging.
Another precise rotation control can be achieved by guiding all the particles directly to the channel side walls by hydrodynamic focusing, as published by Torino et al.38 in 2016. The no-slip condition creates a steep velocity gradient near the channel wall locations, causing the particles to roll on the channel wall with a high rotational frequency (Fig. 1). Even though all the particles will undergo self-rotation, the particle rotation is still not controlled due to the direct particle–wall contact. Furthermore, this method does not provide a uniform focal plane for non-uniformly sized particles, which can be challenging for imaging methods with a fixed focal plane. The advantages of this method lie in the robust particle focusing and a very high particle rotation frequency which can be a critical point to ensure a desired rotation angle within the field of view (FOV).
Particle focusing into a single file arrangement with a unique focal plane can also be achieved by inertial focusing in a high aspect ratio channel (width > height).39 Inertial particle migration in straight channels is achieved by the ratio of the opposing shear gradient lift force and wall-lift forces. Tan et al.40 performed multi angle imaging with a throughput of up to 2000 cells per second. The advantages of the inertial focusing are its simple and easy to manufacture chips with no need of sheet fluids. But the inertial effect only appears at high flow velocities in the cm s−1 to low m s−1 range. These high flow velocities require a correspondingly short exposure time in a range of only a few μs and a high-speed camera providing tens of thousands of FPS for multi angle imaging. Due to the short exposure times, fluorescence imaging can be challenging.
In recently published33,41 studies, we reported a microfluidic chip device for precise control of a narrow particle lamella within the focal plane of the imaging system. The innovative core of this design is a flow-rotation unit (FRU) where a vertical sample lamella is created via hydrodynamic flow focusing and subsequently hydrodynamically rotated incrementally at exactly 90 degrees, while its planarity was kept. During the transition of the FRU, the x-position of the sample sheet was transformed into a z-position. This means that a change of the x-position via an adjustment of the flow rate ratio at the flow focusing unit leads to a direct change of the z-position in the detection channel. This can be used to adjust the particles at defined positions within the parabolic z-velocity profile for the induction of shear-flow induced particle rotation.
2.2 Non-rotating particles
In the previous methods, the particles were actively or passively rotated while passing through the flow cell. The tomographic information resulted from the relative change of the particle orientation to a static detector over time. As previously described, particles located at the channel center position are not undergoing self-rotation. Particle focusing on the center of a flow cell is a standard method in flow cytometry for decades. This gave the advantage of using evaluated methods for particle focusing.
In general, the imaging detector beam path is oriented perpendicular to the flow direction. In order to achieve tomographic imaging of non-rotating particles, the tomographic information has to be generated by adapting imaging methods such as light-sheet fluorescence microscopy (LSFM).42,43 Another way is to apply an additional tilt angle between the flow direction and detector beam path. This can be achieved either by aligning the microfluidic chip with a given tilt angle to the detector system44 or by manufacturing a slanted channel45 in the microfluidic chip (Fig. 2). In both variations, the stack collection for 3D imaging is generated by the particles moving into and out of the focus as they flow through the tilt channel passing through the microscope imaging field of view. The tilt angle has to be determined in a way that the vertical motion of the particle covers its diameter.
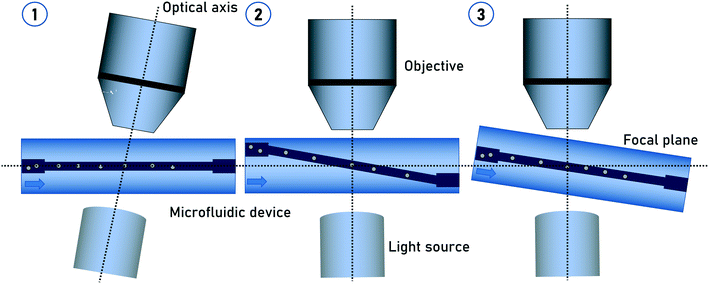 |
| Fig. 2 Arrangement variants to achieve image stacks of non-rotating particles. 1) The optical system is arranged with a tilted angle to a planar microfluidic channel. 2) A chip with a slanted microfluidic channel. The optical system is arranged orthogonally to the chip. 3) A chip with a tilted angle and the optical system is arranged planar. | |
3 tIFC methods
tIFC methods often represent a combination of imaging methods and particle arrangement (Fig. 3). The basic imaging technologies for tIFC are essentially similar to those for common IFC. Recently, Han et al. (2016)3 gave a profound overview about imaging technologies for imaging flow cytometry. The tIFC methods described here have basic similarities in terms of sample preparation and microfluidic focusing but differ in how and what information on the particles are recorded and evaluated. For example, the particles can be rotated or the microfluidic channel or the optical system can be tilted to record the spatial information. The basic requirement for high sample throughput is a high acquisition rate to record the data. The limiting factor is usually the acquisition hardware and not the microfluidics or the sample used. Table 1 shows the key parameters and the required hardware for the different methods. In the following section, key tIFC methods are described in terms of their imaging method.
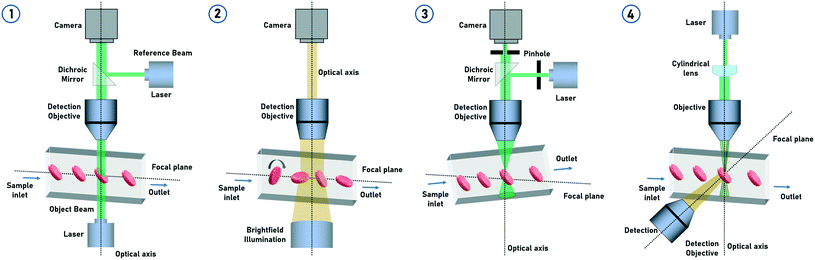 |
| Fig. 3 Overview of the different tIFC methods: 1) digital holography – a holographic z-stack of the particles is recorded as they pass through the FOV. 2) Widefield imaging – cells are put into controlled rotation via hydrodynamic focusing and imaged multiple times as they pass through the FOV. 3) Confocal microscopy – non-rotating particles passing through the FOV in a tilt-angled channel thereby capturing a z-stack of the particles. Out of focus light is blocked by the pinhole disk. Only light from the focal plane can pass through the pinhole. 4) Light-sheet microscopy – non-rotating particles pass through the narrow light-sheet formed by the cylindrical lens and are scanned multiple times. | |
Table 1 Summary of the tomographic imaging flow cytometry (tIFC) methods
Imaging method |
Particle rotation |
Flow cell arrangement |
Illumination |
Detector |
Spatial resolution |
Throughput |
Modality |
Ref |
Digital holography |
Rotating |
Planar |
Laser |
CCD |
0.5 μm |
∼150 particles per s |
Complex field |
44, 46 |
Non-rotating |
Tilt angle |
LED |
CMOS |
Confocal |
Non-rotating |
Tilt angle |
Laser |
CMOS |
μm range |
∼1.5 particles per s |
Fluorescence |
47
|
Widefield |
Rotating |
Planar |
Laser |
CMOS |
1.5 μm |
∼2000 particles per s |
Bright-field fluorescence |
33, 40, 45 |
Non-rotating |
Tilt angle |
LED |
sCMOS |
Light-sheet |
Non-rotating |
Planar |
Laser |
CMOS |
<1 μm |
∼500 particles per s |
Fluorescence |
32, 48 |
Tilt angle |
PMT |
Side-scattering |
3.1 Digital holography
Digital holography microscopy (DHM) is a powerful and often used label-free method to measure particles 3-dimensionally based on quantitative phase information. Particles injected into a flow cell undergo self-rotation (tumbling) induced by hydrodynamic effects under small confinement and flow strength. 3D refractive index maps of tumbling cells in continuous flow can thus be measured.49 Also randomly rolling particles in a microchannel for tomographic quantitative phase microscopy (TPM) are possible.46 The holographic image sequence is acquired with a Mach–Zehnder interferometer. The advantage of applying DHM over the rotating cells is that the tomographic information in terms of tilt series can be obtained by a static optical setup without changing the illumination angle. Therefore, the knowledge of the particle rotation angle in the partial images of the tilt series is necessary for the 3D reconstruction.50 The 3D information of the particles can also be obtained by a tilt angle arrangement of the setup.44 The flow cell is oriented at an angle of 4° with respect to the x-axis. Individual particle layers are recorded while translating the focal plane of the imaging system in an inclined orientation. This method uses in-focus and defocused bright-field images of the particles to reconstruct the corresponding quantitative phase images (Fig. 4).
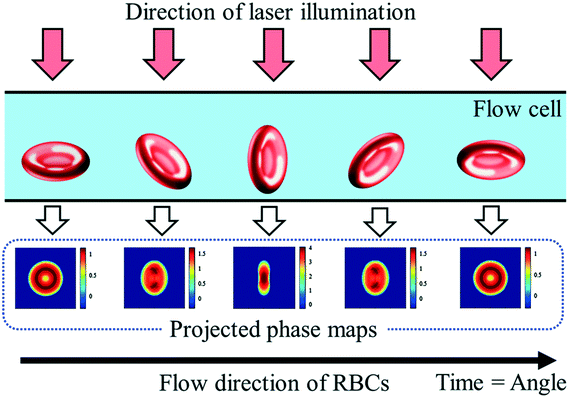 |
| Fig. 4 Schematic representation of erythrocytes from various orientations and their resulting projected phase maps. Reproduced from ref. 53 with permission from SPIE. | |
Two inline methods to determine the rotation angles directly from the holographic image sequence are used.29,46,51 The calculation is based on the refractive index (RI) distribution of the particles. For particles with a rather homogeneous RI (e.g. RBCs), the rotation angle is numerically estimated by aberration analysis using the Zernike fitting. For cells with an inhomogeneous RI (e.g. diatoms) with a detectable RI inner structure, the rotation angle is estimated by searching for twin-mirror phase-contrast maps (QPMs) due to a spatial symmetry in the reconstructed QPMs as a function of rotation angles. With this approach, a morphological classification of self-rotating cells has been demonstrated with a throughput of more than 150 cells per minute. The tomographic reconstruction is done by optical projection tomography,52,53 where the inputs are the aligned-oriented corresponding quantitative QPMs and the rotation angles. Typical numbers of QPMs in the field of view are ∼200 holograms per cell. DHM is a non-invasive imaging method which is also capable of live cell imaging. This provides cell morphology and internal structures based on their RI contrast and is a powerful tool for 3D surface reconstruction, such as red blood cell morphology. DHM is also suitable for mapping phase objects. Since the image plane can be chosen during DHM reconstruction, no 3D particle focusing is required during acquisition. The method is limited to the RI distribution within cells, and density adjustment of the buffer medium may also result in a lower contrast in the reconstructed images. It can also fail to reconstruct non-sparse complicated 3D arrangement of refractive indices.
3.2 Confocal imaging
Confocal microscopy is a technology for highlighting special structures within a particle.54–56 For this purpose, certain areas are selectively illuminated and thus made visible. Only when the particle is completely scanned, a 3D image of the particle emerges. The z-resolution of the particle depends on the number of images taken during the scan.57 The combination of confocal microscopy of cells in microfluidic flow with subsequent 3D image analysis was introduced by Quint et al. (2017).47 For this purpose, microfluidic channels were used with a tilt angle of approximately 3° with respect to the focal plane of the microscope (Fig. 5). A spinning microlens enhanced Nipkow disk is used for continuously capturing confocal cross-sectional images as the particles pass through the focal plane of the confocal microscope. With this approach, several hundred cells can be acquired and processed per minute. The system limitations in the throughput are the scanning performance of the mechanical actuators of the microscope and the frame rate of the used camera. This method is particularly suitable for applications where shape-related anomalies are important. For such use-cases, this method could replace the classic IFC. Confocal imaging provides high contrast fluorescence and reflected light images. Since the microlenses of the Nipkow disk block most of the excitation light, the signal strength must be considered. The lateral flow of the cells must be synchronized with the scan time of the optical system. For 3D imaging, particle rotation must be avoided.
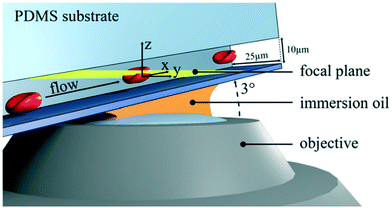 |
| Fig. 5 Sketch of a microfluidic confocal imaging approach. The setup consists of a microchannel with a tilt angle of approximately 3° with respect to the focal plane (yellow) and a spinning microlens enhanced Nipkow disk for confocal cross-sectional imaging. Reproduced from ref. 47 with permission from Appl. Phys. Lett. | |
3.3 Widefield microscopy
Widefield microscopy is useful for imaging multiple particles simultaneously. By imaging the particles in parallel, the throughput can be increased. There are tIFC widefield approaches that either use slanted channels or rotate the particles as they pass through the FOV. Kleiber et al. (2020)33 combined the approach, described in chapter 2.1, with widefield microscopy for the multidirectional imaging of rotating particles (Fig. 6). This 3D particle focusing allows all particles to pass through the flow cell in a single horizontal plane. The z-position in the microfluidic channel is also additionally adjustable. The particle rotation can be controlled by adjusting the sample lamella at an appropriate position of the parabolic velocity profile, which is realized by changing the flow rate ratios of the sheet flow inlets. This additionally allows the control of the width of the sample lamella in a range from covering the complete channel width to a single file arrangement. This provides a high flexibility for particle-arrangement, angular velocity and flow velocity adapted to the needs of the tomographic imaging method. The limitation is the maximum rotation velocity and the frame rate of the camera.
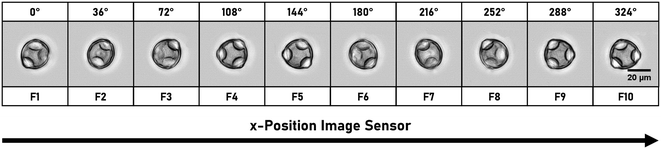 |
| Fig. 6 Multidirectional views of a C. avellana pollen image series (F1–F10) obtained with widefield microscopy. The particle is recorded several times at different x-positions on the image sensor as the particle passes through the FOV while rotating at a continuous velocity. | |
In addition, slanted channels can be used to create a tilt angle for widefield tomographic fluorescence imaging in flow.45 The stack collection is generated by the particles coming into and out of the focus as they flow through the slanted channel passing through the FOV of the microscope. The particles must pass individually through the microfluidic channel and are scanned as a z-stack. Low flow rates and narrow channels ensure that laminar flow conditions prevail. Rotation of the particles causes reconstruction problems with this approach. Each particle can be recorded with up to 200 slices, which results in a high spatial resolution. Due to the relatively simple measurement setup, this method can also be used by non-experts. Therefore, the method is suitable for cellular and point-of-care diagnostics. The main advantage of widefield techniques is their simple and well-known setup. At a larger FOV (e.g., when imaging multiple cells in parallel), fluorescence intensity can be critical at exposure times in the lower μs range. Also, lower magnification is usually accompanied by lower NA.
3.4 Light-sheet fluorescence microscopy
One method to obtain tomographic information from non-rotating particles is the light-sheet fluorescence microscopy (LSFM).58 By LSFM, only a narrow lateral light-sheet is focused in the microfluidic channel perpendicular to the detector system. Particles are excited layer by layer while passing the light beam, generating a stack of fluorescence images59 (Fig. 7). However, focusing of the particles in the center of the channel is required to ensure that all the particles are in the focus of the narrow excitation beam.60 The light-sheet can be formed with a collimator lens and a cylindrical lens in combination with an objective or the light-sheet forming lens that can be directly integrated in the microfluidic device.61 Also, two lasers can be operated alternately to obtain two fluorescence channels simultaneously. The depth of field and the FOV are depending on the beam thickness and the Rayleigh length. When the particles pass through the light-sheet, an intensity profile of each particle per slice is recorded and subsequently reconstructed into a 3D image. A change of the focus position would therefore have a direct influence on the fluorescence intensity. A challenge for LSFM is the long acquisition time for fluorescence imaging which has a direct influence on the particle throughput. Also, particle rotation should be avoided while passing through the light-sheet for tomographic imaging and reconstruction. With this tIFC method, up to 500 cells per s can be captured and analyzed.48 However, this method is also suitable if the particles are to be scanned spatially very accurately by adjusting the flow rate accordingly. In addition, convolutional neural networks (CNNs) are used to derive the 3D information from the measured light-sheet data of the particles.62 It has been shown that the side-scattering signals not only contain information about e.g. the cell granularity, but also information about the properties and functions of cells. Compared to confocal microscopy, LSFM also offers low background noise and high contrast in its fluorescence images but illuminates the entire image plane at once and offers higher excitation intensities because no light is blocked by pinholes. But again, LSFM is limited to fluorescence imaging, and self-rotation of the particles must be avoided as they pass through the light-sheet.
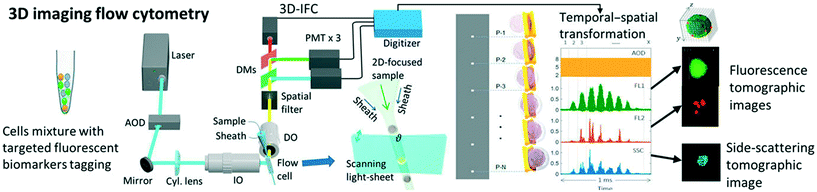 |
| Fig. 7 Schematic diagram of the light-sheet based tIFC cell type analysis workflow. Reproduced from ref. 62 with permission from AIP Publishing. | |
4 tIFC applications
In conventional FC, the particle type is derived from the scatter signal of the individual cells but offers no spatial information. With the introduction of IFC and later tIFC, both surface and internal properties are measured and visualized. The use of tIFC is therefore particularly suitable for applications where three-dimensional information of the studied particles is important. Additional DHM, bright-field and scatter-signal based methods can provide label-free tomographic imaging. The major information gains of tIFC are its 3D morphological representation and the visualization of substructures within a volumetric body. Fig. 8 shows the application areas of tIFC to date.
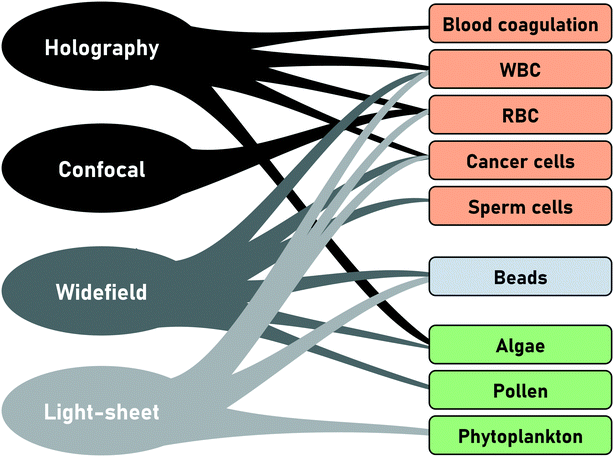 |
| Fig. 8 Application areas of the different tIFC methods. | |
Standard flow cytometry is the gold standard in routine diagnostics such as hematology and immunology. Morphological changes and 3D shape variations of RBCs can be diagnostic indicators of disease entities33,44,46,63,64 (Fig. 9). Aggregation structures of RBCs can indicate the degree of blood coagulation which plays an important role in the hemostasis process.53
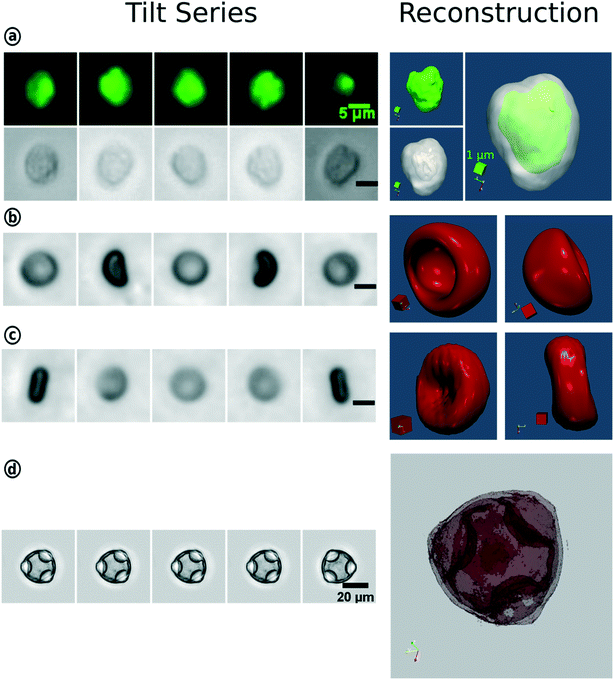 |
| Fig. 9 3D-reconstruction of WBC, RBC and hazel pollen based on the tIFC tilt series. (a) Fluorescence and bright-field imaging of a white blood cell and their merged reconstruction results. Bright-field tilt series and reconstruction results of the red blood cell with one-side concavity (b) and biconcave RBC (c). (d) Reconstruction for the hazel pollen. Reproduced from ref. 33 with permission from Royal Society of Chemistry. | |
Other fields of interest are the investigation of abnormal sperm morphology as a factor of male infertility.29 For marine application, phytoplankton taxonomy50,59 or live circle stage investigation41 can be enriched by the enhanced 3D information content. 3D side scatter patterns can be used to distinguish between different human cancer cell lines and between different subtypes of white blood cells using a CNN for image classification.62 Also, multi-directional imaging of single pollen grains by using CNNs can enhance the prediction accuracy for population analysis and also helps to prevent false-positive classification by improving the identification of unknown particle classes.33 Furthermore, a study of pollen morphology, quantity and viability can provide valuable information during the long-lasting plant-breeding processes.65
5 tIFC challenges and outlook
In IFC, and specifically in tIFC, there are numerous challenges that need to be considered and solved during the design and realization of microfluidic implementation. As in any automated optical system, the quality, stability and reproducibility of the process parameters including the adjustment of the optical system are important for the generation of long-term reproducible data. The following section will address some of the key challenges such as stable flow and focusing conditions, sample sedimentation and image data transfer.
In praxis, we observe effects which may disturb the stable operating conditions such as the flow rate, pulsation, particle sedimentation, particle clustering and blocking of the flow capillary. Pulsation is often caused either by air bubbles at arbitrary positions of the fluidic systems, clustering/aggregation of particles or vibrations acting on the device from the outside. Although air bubbles can be avoided by careful sample and buffer loading, clustering is more challenging. Clustering often comes along with two major problems. First, the image data processing in terms of segmentation of particle clusters can be very challenging. Second, these clusters can block the microfluidic channel which can lead to permanent blockage of the microfluidic channel or to pulsation of the fluids due to pressure build up (blockage) and pressure release (removal of the blockage). Some samples tend to build up clusters more than others, and some of the reasons are the particle morphology (e.g. spikes) or extracellular structures such as the flagella of microalgae which can cause an increase in interparticle adhesion. An example is the RBC aggregation of blood cells, which tend to form clusters.66,67 Additionally, the contamination of the sample buffer with debris can cause clustering. These effects must be minimized by optimized sample preparation such as filtering, adjusting the sample concentration or by addition of surfactants (e.g. Tween20). Also, the choice of the pump system can be critical since pulsations caused by the pump can disturb the particle focusing and the uniformity of the particle transport velocity which have a negative impact on the imaging (blurry images) and 3D reconstruction (angular velocity). Both modern pressure and syringe pumps can provide pulsation free flow with velocities down to nl s−1. Often, density gradients between the buffer and particle cause particle sedimentation or flotation.68 Both effects can lead to particles no longer being transported along the flow direction. Moreover, the formation of clusters is amplified since the fluid velocity at the channel walls is not sufficient to avoid the attachment of the particles at the channel walls. A density adjustment of the buffer can be done with a hydrophilic polysaccharide (e.g. Ficoll® 400) but it is not applicable to suspensions with heterogeneous densities and for applications where the buffer composition is critical for the viability of the cells.69 Both effects can lead to particles no longer being transported along the flow direction. Moreover, the formation of clusters is amplified since the particle velocity at the channel walls is not strong enough to dissolve the particles from these compounds. A density adjustment of the buffer can be done with a hydrophilic polysaccharide (e.g. Ficoll® 400) but it is not a sufficient solution for density inhomogeneous suspensions and for applications where the buffer composition is critical for the viability of the cells.68 Furthermore, the flow velocity can be increased to reduce the dwell time of the particles within the microfluidic system to prevent sedimentation or flotation. It has to be considered that a higher flow velocity comes along with shorter acquisition times (image blur) and higher frame rates (observations per particle). This requires a high-performance optical and image data recording system. The acquisition, processing and storage of the resulting data volumes is another limiting factor.70,71 Depending on the throughput, several 100 MB per second of data will be generated.14 The amount of data to be processed has been increasing continuously in recent years. Artificial intelligence and machine vision algorithms are now increasingly being used to process the IFC data. This is also illustrated by the continuously increasing number of publications in this research area. In IFC, these methods have already been used for several years to classify the measured particles based on their morphological properties. Convolutional neural networks (CNNs),12,72,73 deep learning14,74–77 or other machine learning methods78–82 are well suited for the analysis of IFC data. However, these methods are only partially used in tIFC so far but they could provide a significant added value. To further increase the data processing speed, graphics processing unit (GPU)-based computing systems are increasingly being used for image processing. With this approach, the processing of data is massively parallelized and thus accelerated.83–85 Another approach is the use of field-programmable gate arrays (FPGAs)86–88 or vision system on chip (VSoC) sensors.89,90 Here, a large part of the pre-processing of the image data is done close to the sensor and only the important data is transmitted to the controlling computer.
In recent years, also numerous new approaches and systems have been published that combine IFC with image-based high throughput cell sorting.91–93 For this purpose, the particles are focused on the center of the channel, imaged individually, and subsequently separated based on previously defined properties. By using very small ROIs (a few hundred pixels) in combination with high-performance computers, neural networks, deep learning and intelligent sensors, e.g. FGPA sensors for image acquisition, high cell throughputs (>1 million cells per s) are possible.94
With tIFC methods, the particles always have to be recorded several times in order to be able to create a 3D image or reconstruction afterwards. This step is computationally intensive and requires a certain amount of time. Therefore, most reported tIFC methods are not real-time capable. However, this is a basic requirement for image-based microfluidic sorting. In the future, by combining the high throughput systems described above with tIFC, much more information about the particles could be obtained than was previously possible using 2D images. This could address new application areas such as screening methods, drug testing, population analysis and fundamental research.
To the best of our knowledge, no commercial tIFC systems are available. As already indicated in the introduction, various IFC devices are available on the market. Although the ImageStream, the FlowCam and the CytPix flow cytometer cover a broad field of application, the Imaging FlowCytobot is mainly focusing on marine research application. The IFC and the tIFC are relatively young technologies, which still need to find its place in research and commercial applications. In particular, in clinical applications, methods for cell diagnostics have been established for decades. Therefore, the authors see research in this area rather that the field of application. Image-based sorting under high throughput, on the other hand, could give the technology an additional boost.
6 Conclusion
Tomographic imaging flow cytometry (tIFC) is a continuously growing subdiscipline of classical IFC, as indicated by the dynamically increasing number of publications and research projects. Two main approaches to obtain the tomographic information of the sample are utilized: the particles can either be moved actively by rotation through the microfluidic channel, or passively by tilting the optical system. Due to the steady increase in the performance of the hardware and software, larger amounts of data can be recorded, processed and analyzed in a shorter time. This will enable tIFC to significantly benefit in the future and address new application fields where 3D information is important. In the future, tIFC is likely to become increasingly important in clinical diagnostics, life sciences and environmental research.
All the described tIFC methods have been developed and published within the last few years. The systems were mostly demonstrated on a specific application. The commercialization of the systems will depend on whether the limitation especially in the complexity of the setup, throughput, spatial resolution, reproducibility, usability and stability can be overcome. It will be determined over the next few years whether these systems can be improved to the point where they can be commercialized.
Conflicts of interest
The authors have no conflict of interest to declare.
Acknowledgements
This article has received funding from the German Federal Ministry for Economic Affairs and Energy (BMWi) on the basis of a resolution of the German Bundestag for the project ‘Pollen3D’, funding signature ‘ZF 400 6811 CR7’ and Thüringer Aufbaubank (2018 SD 0066).
References
- A. Adan,
et al., Flow cytometry: basic principles and applications, Crit. Rev. Biotechnol., 2017, 37(2), 163–176 CrossRef CAS PubMed.
- R.-J. Yang, L.-M. Fu and H.-H. Hou, Review and perspectives on microfluidic flow cytometers, Sens. Actuators, B, 2018, 266, 26–45 CrossRef CAS.
- Y. Han,
et al., Review: imaging technologies for flow cytometry, Lab Chip, 2016, 16(24), 4639–4647 RSC.
- D. B. Kay, J. L. Cambier and L. Wheeless Jr., Imaging in flow, J. Histochem. Cytochem., 1979, 27(1), 329–334 CrossRef CAS PubMed.
- E. K. Zuba-Surma,
et al., The ImageStream System: a key step to a new era in imaging, Folia Histochem. Cytobiol., 2007, 45(4), 279–290 Search PubMed.
- R. J. Olson and H. M. Sosik, A submersible imaging-in-flow instrument to analyze nano-and microplankton: Imaging FlowCytobot, Limnol. Oceanogr.: Methods, 2007, 5(6), 195–203 CrossRef.
- C. K. Sieracki, M. E. Sieracki and C. S. Yentsch, An imaging-in-flow system for automated analysis of marine microplankton, Mar. Ecol.: Prog. Ser., 1998, 168, 285–296 CrossRef.
- D. A. Basiji,
et al., Cellular Image Analysis and Imaging by Flow Cytometry, Clin. Lab. Med., 2007, 27(3), 653–670 CrossRef PubMed.
- D. A. Basiji, Principles of Amnis Imaging Flow Cytometry, Methods Mol. Biol., 2016, 1389, 13–21 CrossRef PubMed.
- V. Haridas,
et al., Imaging flow cytometry analysis of intracellular pathogens, Methods, 2017, 112, 91–104 CrossRef CAS PubMed.
- B. S. Lambert, R. J. Olson and H. M. Sosik, A fluorescence-activated cell sorting subsystem for the Imaging FlowCytobot, Limnol. Oceanogr.: Methods, 2017, 15(1), 94–102 CrossRef.
- S. Dunker,
et al., Pollen analysis using multispectral imaging flow cytometry and deep learning, New Phytol., 2021, 229(1), 593–606 CrossRef PubMed.
- G. Gopakumar,
et al., Framework for morphometric classification of cells in imaging flow cytometry, J. Microsc., 2016, 261(3), 307–319 CrossRef CAS PubMed.
- H. Mikami,
et al., Virtual-freezing fluorescence imaging flow cytometry, Nat. Commun., 2020, 11(1), 1–11 CrossRef PubMed.
- R. Regmi, K. Mohan and P. P. Mondal, Light sheet based imaging flow cytometry on a microfluidic platform, Microsc. Res. Tech., 2013, 76(11), 1101–1107 CrossRef PubMed.
- M. Doan,
et al., Diagnostic Potential of Imaging Flow Cytometry, Trends Biotechnol., 2018, 36(7), 649–652 CrossRef CAS PubMed.
- C. Reichle,
et al., Electro-rotation in octopole micro cages, J. Phys. D: Appl. Phys., 1999, 32(16), 2128–2135 CrossRef CAS.
- L.-H. Chau,
et al., Self-rotation of cells in an irrotational AC E-field in an opto-electrokinetics chip, PLoS One, 2013, 8(1), e51577 CrossRef CAS PubMed.
- P. Benhal,
et al., AC electric field induced dipole-based on-chip 3D cell rotation, Lab Chip, 2014, 14(15), 2717–2727 RSC.
- X. Yang,
et al., Accurate extraction of the self-rotational speed for cells in an electrokinetics force field by an image matching algorithm, Micromachines, 2017, 8(9), 282 CrossRef PubMed.
- L. Huang, F. Liang and Y. Feng, A microfluidic chip for single-cell 3D rotation enabling self-adaptive spatial localization, J. Appl. Phys., 2019, 126(23), 234702 CrossRef.
- J. P. Shelby and D. T. Chiu, Controlled rotation of biological micro- and nano-particles in microvortices, Lab Chip, 2004, 4(3), 168–170 RSC.
- F. P. Bretherton, The motion of rigid particles in a shear flow at low Reynolds number, J. Fluid Mech., 1962, 14(2), 284–304 CrossRef.
-
J. L. Poiseuille, Experimental research on the movement of liquids in tubes of very small diameters, Mémoires presentés par divers savants a l'Académie Royale des Sciences de l'Institut de France, IX, 1846, pp. 433–544 Search PubMed.
- D. Oliver, Influence of particle rotation on radial migration in the Poiseuille flow of suspensions, Nature, 1962, 194(4835), 1269–1271 CrossRef.
-
M. F. A. Couette, Etudes sur le frottement des liquides, Gauthier-Villars, 1890 Search PubMed.
- C. Lun,
et al., Kinetic theories for granular flow: inelastic particles in Couette flow and slightly inelastic particles in a general flowfield, J. Fluid Mech., 1984, 140, 223–256 CrossRef.
- T. Kolb,
et al., Optofluidic rotation of living cells for single-cell tomography, J. Biophotonics, 2015, 8(3), 239–246 CrossRef CAS PubMed.
- F. Merola,
et al., Digital holography as a method for 3D imaging and estimating the biovolume of motile cells, Lab Chip, 2013, 13(23), 4512–4516 RSC.
- I. Bernard,
et al., Controlled rotation and translation of spherical particles or living cells by surface acoustic waves, Lab Chip, 2017, 17(14), 2470–2480 RSC.
- S. Torino,
et al., Microfluidic technology for cell hydrodynamic manipulation, AIMS Biophys., 2017, 4(2), 178–191 CAS.
- Q. Tang,
et al., On-chip simultaneous rotation of large-scale cells by acoustically oscillating bubble array, Biomed. Microdevices, 2020, 22(1), 13 CrossRef CAS PubMed.
- A. Kleiber,
et al., 3-Step flow focusing enables multidirectional imaging of bioparticles for imaging flow cytometry, Lab Chip, 2020, 20(9), 1676–1686 RSC.
- M. Antfolk,
et al., Focusing of sub-micrometer particles and bacteria enabled by two-dimensional acoustophoresis, Lab Chip, 2014, 14(15), 2791–2799 RSC.
- W. Lee,
et al., Dynamic self-assembly and control of microfluidic particle crystals, Proc. Natl. Acad. Sci. U. S. A., 2010, 107(52), 22413–22418 CrossRef CAS PubMed.
-
A. Einstein, Eine neue bestimmung der moleküldimensionen, ETH Zurich, 1905 Search PubMed.
- G. B. Jeffery, The motion of ellipsoidal particles immersed in a viscous fluid, Proc. R. Soc. London, Ser. A, 1922, 102(715), 161–179 Search PubMed.
- S. Torino,
et al., A Microfluidic Approach for Inducing Cell Rotation by Means of Hydrodynamic Forces, Sensors, 2016, 16(8), 1326 CrossRef PubMed.
- J. M. Martel and M. Toner, Inertial focusing in microfluidics, Annu. Rev. Biomed. Eng., 2014, 16, 371–396 CrossRef CAS PubMed.
- A. P. Tan,
et al., Continuous-flow cytomorphological staining and analysis, Lab Chip, 2014, 14(3), 522–531 RSC.
- D. Kraus,
et al., Three step flow focusing enables image-based discrimination and sorting of late stage 1 Haematococcus pluvialis cells, PLoS One, 2021, 16(3), e0249192 CrossRef CAS PubMed.
- R. M. Power and J. Huisken, A guide to light-sheet fluorescence microscopy for multiscale imaging, Nat. Methods, 2017, 14(4), 360–373 CrossRef CAS PubMed.
- P. A. Santi, Light sheet fluorescence microscopy: a review, J. Histochem. Cytochem., 2011, 59(2), 129–138 CrossRef CAS PubMed.
- S. S. Gorthi and E. Schonbrun, Phase imaging flow cytometry using a focus-stack collecting microscope, Opt. Lett., 2012, 37(4), 707–709 CrossRef PubMed.
- V. K. Jagannadh,
et al., Slanted channel microfluidic chip for 3D fluorescence imaging of cells in flow, Opt. Express, 2016, 24(19), 22144–22158 CrossRef CAS PubMed.
- F. Merola,
et al., Tomographic flow cytometry by digital holography, Light: Sci. Appl., 2017, 6(4), e16241 CrossRef CAS PubMed.
- S. Quint,
et al., 3D tomography of cells in micro-channels, Appl. Phys. Lett., 2017, 111(10) Search PubMed.
- Y. Han,
et al., Cameraless high-throughput three-dimensional imaging flow cytometry, Optica, 2019, 6(10), 1297–1304 CrossRef CAS.
- Y. Sung,
et al., Three-Dimensional Holographic Refractive-Index Measurement of Continuously Flowing Cells in a Microfluidic Channel, Phys. Rev. Appl., 2014, 1, 014002 CrossRef CAS PubMed.
- F. Merola,
et al., Phase contrast tomography at lab on chip scale by digital holography, Methods, 2018, 136, 108–115 CrossRef CAS PubMed.
- M. M. Villone,
et al., Full-angle tomographic phase microscopy of flowing quasi-spherical cells, Lab Chip, 2017, 18(1), 126–131 RSC.
- J. Sharpe,
et al., Optical projection tomography as a tool for 3D microscopy and gene expression studies, Science, 2002, 296(5567), 541–545 CrossRef CAS PubMed.
- H. Funamizu and Y. Aizu, Three-dimensional quantitative phase imaging of blood coagulation structures by optical projection tomography in flow cytometry using digital holographic microscopy, J. Biomed. Opt., 2018, 24(3), 1–6 Search PubMed.
- G. Rothmund,
et al., Confocal laser scanning microscopy as a new valuable tool in the diagnosis of onychomycosis–comparison of six diagnostic methods, Mycoses, 2013, 56(1), 47–55 CrossRef CAS PubMed.
- D. Shotton and N. White, Confocal scanning microscopy: three-dimensional biological imaging, Trends Biochem. Sci., 1989, 14(11), 435–439 CrossRef CAS PubMed.
- C. L. Smith, Basic confocal microscopy, Curr. Protoc. Neurosci., 2011, 56(1), 2.2.1–2.2.18 Search PubMed.
- K. Khairy, J. Foo and J. Howard, Shapes of red blood cells: comparison of 3D confocal images with the bilayer-couple model, Cell. Mol. Bioeng., 2008, 1(2), 173–181 CrossRef PubMed.
- E. J. Gualda,
et al., Three-dimensional imaging flow cytometry through light-sheet fluorescence microscopy, Cytometry, Part A, 2017, 91(2), 144–151 CrossRef PubMed.
- J. Wu, J. Li and R. K. Chan, A light sheet based high throughput 3D-imaging flow cytometer for phytoplankton analysis, Opt. Express, 2013, 21(12), 14474–14480 CrossRef CAS PubMed.
-
C. Rasmi, et al., Three Dimensional Imaging of HeLa cells using light sheet based imaging flow cytometry. in CLEO: Applications and Technology, Optical Society of America, 2017, p. JW2A.55 Search PubMed.
- F. Sala,
et al., High-throughput 3D imaging of single cells with light-sheet fluorescence microscopy on chip, Biomed. Opt. Express, 2020, 11(8), 4397–4407 CrossRef CAS PubMed.
- R. Tang,
et al., 3D side-scattering imaging flow cytometer and convolutional neural network for label-free cell analysis, APL Photonics, 2020, 5(12), 126105 CrossRef CAS.
-
A. S. Adewoyin, et al., Erythrocyte Morphology and Its Disorders, in Erythrocyte, IntechOpen, 2019 Search PubMed.
- L. Lanotte,
et al., Red cells' dynamic morphologies govern blood shear thinning under microcirculatory flow conditions, Proc. Natl. Acad. Sci. U. S. A., 2016, 113(47), 13289–13294 CrossRef CAS PubMed.
- E. H. Souza,
et al., Pollen morphology and viability in Bromeliaceae, An. Acad. Bras. Cienc., 2017, 89, 3067–3082 CrossRef CAS PubMed.
- J. L. McWhirter, H. Noguchi and G. Gompper, Deformation and clustering of red blood cells in microcapillary flows, Soft Matter, 2011, 7(22), 10967–10977 RSC.
-
O. Baskurt, B. Neu and H. J. Meiselman, Red blood cell aggregation, 2011 Search PubMed.
- J. J. Sherba,
et al., The effects of electroporation buffer composition on cell viability and electro-transfection efficiency, Sci. Rep., 2020, 10(1), 1–9 CrossRef PubMed.
- S. M. Kim, S. H. Lee and K. Y. Suh, Cell research with physically modified microfluidic channels: a review, Lab Chip, 2008, 8(7), 1015–1023 RSC.
- A. C. Zhang,
et al., Computational cell analysis for label-free detection of cell properties in a microfluidic laminar flow, Analyst, 2016, 141(13), 4142–4150 RSC.
- C. L. Chen,
et al., Deep learning in label-free cell classification, Sci. Rep., 2016, 6(1), 1–16 CrossRef PubMed.
- Y. Matsuoka, R. Nakatsuka and T. Fujioka, Automatic discrimination of human hematopoietic tumor cell lines using a combination of imaging flow cytometry and convolutional neural network, Hum. Cell, 2021, 34(3), 1021–1024 CrossRef PubMed.
- E. N. Mochalova,
et al., Precise quantitative analysis of cell targeting by particle-based agents using imaging flow cytometry and convolutional neural network, Cytometry, Part A, 2020, 97(3), 279–287 CrossRef PubMed.
- A. Allemang,
et al., The 3D reconstructed skin micronucleus assay using imaging flow cytometry and deep learning: A proof-of-principle investigation, Mutat. Res., Genet. Toxicol. Environ. Mutagen., 2021, 865, 503314 CrossRef CAS PubMed.
- S. Dunker,
et al., Combining high-throughput imaging flow cytometry and deep learning for efficient species and life-cycle stage identification of phytoplankton, BMC Ecology, 2018, 18(1), 1–15 CrossRef PubMed.
- S. Luo,
et al., Deep learning-enabled imaging flow cytometry for high-speed Cryptosporidium and Giardia detection, Cytometry, Part A, 2021 DOI:10.1002/cyto.a.24321.
- D. M. Siu,
et al., Deep-learning-assisted biophysical imaging cytometry at massive throughput delineates cell population heterogeneity, Lab Chip, 2020, 20(20), 3696–3708 RSC.
- H. Hennig,
et al., An open-source solution for advanced imaging flow cytometry data analysis using machine learning, Methods, 2017, 112, 201–210 CrossRef CAS PubMed.
- C. Lei,
et al., High-throughput imaging flow cytometry by optofluidic time-stretch microscopy, Nat. Protoc., 2018, 13(7), 1603–1631 CrossRef CAS PubMed.
- M. Lippeveld,
et al., Classification of human white blood cells using machine learning for stain-free imaging flow cytometry, Cytometry, Part A, 2020, 97(3), 308–319 CrossRef PubMed.
- S. Ota, I. Sato and R. Horisaki, Implementing machine learning methods for imaging flow cytometry, Microscopy, 2020, 69(2), 61–68 CrossRef CAS PubMed.
- S. Ota,
et al., Ghost cytometry, Science, 2018, 360(6394), 1246–1251 CrossRef CAS PubMed.
- Y. J. Heo,
et al., Real-time image processing for microscopy-based label-free imaging flow cytometry in a microfluidic chip, Sci. Rep., 2017, 7(1), 1–9 CrossRef PubMed.
- C. Probst,
et al., Advanced characterization of silicone oil droplets in protein therapeutics using artificial intelligence analysis of imaging flow cytometry data, J. Pharm. Sci., 2020, 109(10), 2996–3005 CrossRef CAS PubMed.
-
A. Vedhanayagam and A. S. Basu, Imaging Flow Cytometry at >13K events/s Using GPU-Accelerated Computer Vision, in 2019 IEEE SEeNSORS, IEEE, 2019 Search PubMed.
- A. K. Lau,
et al., Optofluidic time-stretch imaging–an emerging tool for high-throughput imaging flow cytometry, Lab Chip, 2016, 16(10), 1743–1756 RSC.
-
D. Lee, et al., A hardware accelerated approach for imaging flow cytometry. in 2013 23rd International Conference on Field programmable Logic and Applications, IEEE, 2013 Search PubMed.
- K. Goda,
et al., High-throughput single-microparticle imaging flow analyzer, Proc. Natl. Acad. Sci. U. S. A., 2012, 109(29), 11630–11635 CrossRef CAS PubMed.
-
J. Döge, et al., Low-Latency Image Acquisition and Processing with a Programmable Vision-System-on-Chip. in 2018 IEEE International Symposium on Circuits and Systems (ISCAS), IEEE, 2018 Search PubMed.
-
P. Reichel, et al., Simulation environment for a vision-system-on-chip with integrated processing. in Proceedings of the 9th International Conference on Distributed Smart Cameras, 2015 Search PubMed.
- Y. Gu,
et al., Machine learning based real-time image-guided cell sorting and classification, Cytometry, Part A, 2019, 95(5), 499–509 CrossRef PubMed.
- A. Isozaki,
et al., Intelligent image-activated cell sorting 2.0, Lab Chip, 2020, 20(13), 2263–2273 RSC.
- N. Nitta,
et al., Intelligent image-activated cell sorting, Cell, 2018, 175(1), 266–276. e13 CrossRef CAS PubMed.
- H. Kobayashi,
et al., Intelligent whole-blood imaging flow cytometry for simple, rapid, and cost-effective drug-susceptibility testing of leukemia, Lab Chip, 2019, 19(16), 2688–2698 RSC.
Footnote |
† Andreas Kleiber & Daniel Kraus are contributed equally to this work. |
|
This journal is © The Royal Society of Chemistry 2021 |
Click here to see how this site uses Cookies. View our privacy policy here.