DOI:
10.1039/D0NA00796J
(Review Article)
Nanoscale Adv., 2021,
3, 1331-1341
Biomarker sensing platforms based on fluorescent metal nanoclusters
Received
25th September 2020
, Accepted 10th January 2021
First published on 13th January 2021
Abstract
Metal nanoclusters (NCs) and their unique properties are increasing in importance and their applications are covering a wide range of areas. Their remarkable fluorescence properties and easy synthesis procedure and the possibility of functionalizing them for the detection of specific targets, such as biomarkers, make them a very interesting biosensing tool. Nowadays the detection of biomarkers related to different diseases is critical. In this context, NCs scaffolded within an appropriate molecule can be used to detect and quantify biomarkers through specific interactions and fluorescence properties of the NCs. These methods include analytical detection and biolocalization using imaging techniques. This review covers a selection of recent strategies to detect biomarkers related to diverse diseases (from infectious, inflammatory, or tumour origin) using fluorescent nanoclusters.
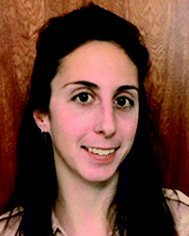 María V. Romeo | María V. Romeo developed the experimental part of her PhD in Biomedicine and Molecular Biology predoctoral program at the Basque University working with Dr Felipe Goñi de Cerio and Jesús Berganza at the Gaiker Technology Centre in Zamudio and with Dr Aitziber L. Cortajarena at CIC biomaGUNE in San Sebastian. She previously worked on the development of several biosensors at the Zaragoza University. Her research focuses on the evaluation of metal nanoclusters' potential as a biosensing tool for strategy development. She worked on nanocluster synthesis strategies, the evaluation of protein candidates to stabilize them, and the study of several physical supports to develop the biosensor and provide an easy to handle and sensitive biosensor. |
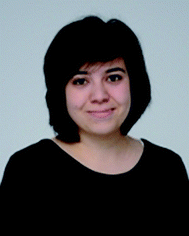 Elena López-Martínez | Elena López-Martínez is a PhD student currently enrolled in the Biomedicine and Molecular Biology doctoral program at the University of the Basque Country. She is developing her thesis in the Biomolecular Nanotechnology Lab, CIC biomaGUNE, under the supervision of Dr Carlos Sanchez-Cano and Dr Aitziber L. Cortajarena. She was previously working on her master's thesis in Biophysics at Dr Cortajarena's Lab at IMDEA Nanociencia in Madrid working with engineered protein modules to stabilize metal nanoclusters. Her research focuses on the use of engineered proteins for the development of different bionanomaterials, with a focus on metal-based nanostructures such as metal nanoclusters and nanoparticles. In particular, she has been studying the role of proteins in the stabilization and optical properties of these nanomaterials, as well as developing new tools for sensing and diagnosis based on protein–metal hybrids. She is also very passionate about science communication and is a tireless participant in and organizer of different outreach activities. |
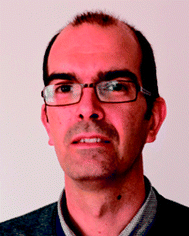 Jesús Berganza | Jesús Berganza received his Bachelor's degree in Biology in 1990 and Master's degree in Biomedical Engineering in 2017 from the University of the Basque Country. He has been working at the GAIKER Technology Centre since 1995 on research projects for the development of biosensors for detection of biomarkers and pathogenic microorganisms in the health, food and environmental fields. His research focuses on immunochemistry, immobilization of biological elements on sensor surfaces, biofunctionalization of micro/nanoparticles and development of immunochromatographic detection systems (lateral flow strips). |
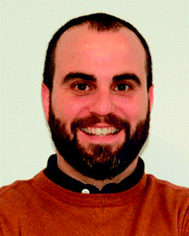 Felipe Goñi-de-Cerio Cerio | Dr Felipe Goñi-de-Cerio (PhD in Cell Biology) obtained his degree from the University of the Basque Country in Cellular Biology and Biochemistry. He completed his PhD studying hypoxia–ischemia brain damage and its treatment in 2007. His scientific career included a stay at the CIC bioGUNE research centre (Bizkaia, Spain). He has more than 10 years of experience in Gaiker as a researcher working on in vitro models and toxicological and functional studies. His research interests include polymer and metallic nanocomposites, in vitro model cultures, and toxicological and functional studies in pharmacology and nanomedicine. Currently he is the Biotechnology Area leader performing active research on product safety and efficacy for the chemistry, pharma and cosmetic industries using in vitro and ex vivo toxicology/efficacy protocols, as well as developing novel biosensors for applications in health, food and the environment. |
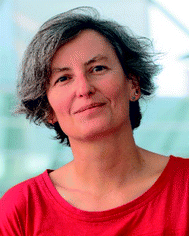 Aitziber L. Cortajarena | Prof. Aitziber L. Cortajarena received her PhD in Biochemistry from the University of the Basque Country, Spain in 2002. She then joined the group of Prof. Regan at Yale University, USA, as a Postdoctoral Fellow and later as an Associate Research Scientist working on protein design, structure, and function. In 2010, she started her independent research focused on bionanotechnology at IMDEA Nanociencia, Spain. In 2016, she moved to CIC biomaGUNE (San Sebastian, Spain) as Ikerbasque Research Professor to lead the Biomolecular Nanotechnology Lab. Her research focuses on protein engineering toward the generation of functional nanostructures and bioinspired materials for applications in nanobiotechnology and nanomedicine. |
Introduction
Colloidal metals are some of the first nanomaterials ever developed, primarily as a decorative staining method.1 Since the mid-XIX century2 and mainly during the last few decades, this field has been enriched with new synthesis pathways and applications. Especially in biomedicine and biosensing, the use of colloidal noble metals experienced an expansion that is still ongoing,3,4 in which the engineering of nanoparticles and nanoparticle-coordinated molecules holds the key to developing new functionalities and applications.
Metal nanoclusters
Metal nanoclusters (NCs) are ultrasmall nanoparticles whose diameter ranges from the sub-nanometre scale to ∼2 nm,5 and they can be synthesized with atomic precision. Their properties include photoluminescence, good photostability, and ligand-dependent and size-dependent tuneable fluorescence. Taking advantage of these properties, nanoclusters can be applied in a wide range of fields such as biodetection,6 theragnostics,3 biological labelling7 or bioimaging.8,96 These characteristics make NCs suitable tools for the detection and quantification of biomarkers.
Synthesis pathways to generate metal NCs
Stable and highly luminescent NCs can be obtained by several synthesis strategies that can be classified into two groups:9 (1) top-down synthesis approaches, consisting in the use of strong ligands, such as thiols,10 to produce a core reduction from larger precursors, and (2) bottom-up synthesis approaches in which NCs are grown from single atoms or ions. In general, synthesis protocols proceed with the reduction of a metal salt11 and the addition of a stabilizing agent that prevents the aggregation of the NCs (Fig. 1). The stabilizing agent plays a key role allowing the nucleation of metal atoms and their uniform growth, leading to monodisperse and size-tuneable NC samples. The number of constituent atoms must be controlled in order to tune the fluorescence properties.5,12 Template-mediated synthesis is a bottom-up strategy widely used to achieve monodisperse NCs with a defined number of atoms. There is a broad range of potential NC-templating molecules (Fig. 1), including small molecules containing thiols,13 such as glutathione (GSH)14 and 2-mercaptosuccinic acid (MSA),15 that work as both reducing and stabilizing agents and are characterized by the strong interaction of the sulfur atoms with gold. Polymers, like polyethyleneimine (PEI), are also good templating agents but they should contain hydroxyl or amino groups. Proteins are very interesting templating agents because of their stability and the possibility of encoding additional functionalities, for example specific target recognition capabilities.16 Likewise, DNA sequences17 have also been used to stabilize NCs and can be specifically designed for selective detection. Although the different templating agents present advantages and disadvantages, biomolecular templates play a prominent role in preventing aggregation18 and can additionally encode specific recognition capability for certain biomarkers, generating versatile multifunctional hybrid structures. DNA-templated NCs are widely used for the detection and quantification of biomarkers together with GSH-stabilized NCs. DNA is an interesting templating molecule because it presents several advantages:17 designable sequence and structure, tuneable size, and nitrogen-based composition which provides multiple coordination sites for the metal ions where NCs can grow. NC synthesis reactions are commonly carried out at neutral pH, thus preserving the physiological conditions.19 Also, thiol-containing molecules like GSH interact with gold atoms through S–Au bonds, which are close in strength to Au–Au bonds,20 leading to the formation of a protective surface layer around gold atoms and, therefore, highly stable NCs.
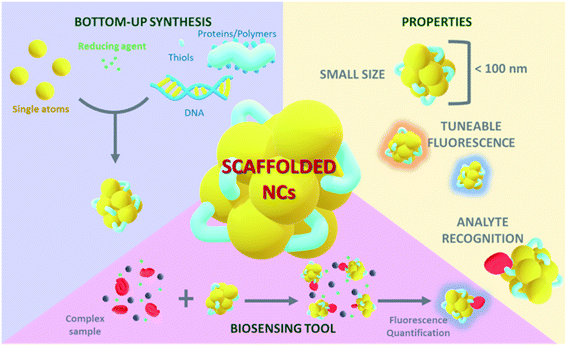 |
| Fig. 1 Overview of the NC synthesis, properties, and applications in biosensing. | |
Biomarkers
The initial application of biomarkers dates to Second World War, when culturing of infectious agents started as a practice to test antibiotics effectiveness.21 Since then, their characterization and applications have been increasingly prominent. The National Institute of Health (NIH, USA) defines a biomarker as ‘a characteristic that is objectively measured and evaluated as an indicator of normal biological processes, pathogenic processes, or pharmacological responses to a therapeutic intervention’.22 Biomarkers are typically used for diagnosing a disease, assessing its prognosis, and/or predicting the efficacy and safety of a drug. Biomarkers used in diagnostics have to be easy to obtain from the patient (blood, urine, or saliva), as well as providing fast results for early diagnosis and treatment.23 It is necessary to differentiate between disease-related or drug-related biomarkers. The first ones give insight into the effect of a treatment on a patient or the progression of the disease,24 whereas the second ones predict drug efficacy in patients and their response during the treatment.
Types of biomarkers.
There are numerous biomarkers available for assessing a wide range of diseases. Some common biomarkers are cholesterol for atherosclerosis,25 prostate-specific antigen (PSA) for the detection of prostate cancer,26,27 and human epidermal growth factor receptor 2 (HER2) for breast cancer.28 In general, biomarkers can have different origins: biomolecules such as proteins or peptides; cells or histological data; genetic markers including single genes, small gene clusters or large collections of genes, and microRNAs (miRNAs).29 Nowadays there are many new types of biomarkers being explored, for instance circulating biomarkers: nucleic acids such as miRNAs;30,31 exosomes, which can be found in almost all biological fluids and carry complex molecular information in the form of biomolecules;32 or even cancerous cells (circulating tumour cells – CTCs)33 released from solid tumours, although they have not been validated yet for clinical use.34
Detection of biomarkers.
There is a wide range of techniques that can be used for assessing the levels of biomarkers, from direct biological observations by functional imaging to quantitative assays, depending on the aim of the study. The measurement of specific molecular targets has been greatly facilitated by advances in molecular probes. One possibility is to generate specific molecules containing a fluorescent tag that can be used for both imaging and quantification assays. These molecules could be hybrid nanomaterials, such as functionalized metal nanoclusters.
This review compiles the recent studies on metal nanoclusters as tools for biosensing assays exploiting their tuneable luminescence properties and focusing on the detection of disease biomarkers in human samples.
Fluorescent nanoclusters for biosensing
The tuneable emission of luminescent NCs has been linked to several effects. The emission energy of a few-atom Au cluster shows a dependence on the free electron density and on the size of the metal cluster, i.e. the number of atoms.35 In these reports, the larger the size of the cluster, the more shifted it is towards red emission. In addition, changes in the chemical environment such as different solvents35 or coordinating molecules36 have been shown to affect the emission colour of NCs. Their remarkable fluorescence properties together with their tunability make biomolecular-stabilized metal NCs versatile tools for biosensing applications (Fig. 2).
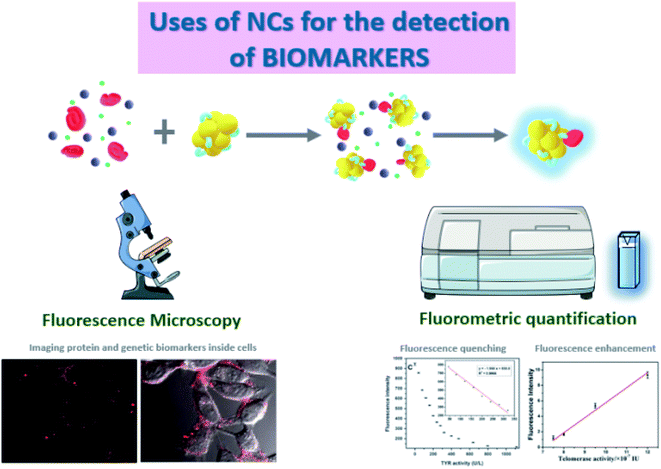 |
| Fig. 2 Main biomarker detection techniques discussed in this review. The fluorescence properties of metal NCs can be used (1) as labels for biomarkers in cell imaging using fluorescence microscopy; (2) as analytical tools for the quantification of the levels of the biomarkers in complex samples, in which the fluorescence can be enhanced or quenched depending on the biosensing mechanism engineered on the NCs. | |
However, the use of photoluminescence as the reporter of the presence of biomarkers has some limitations. First, the possible interference of the autofluorescence of different molecules present in raw biological fluid. Moreover, there may appear quenching effects caused by such molecules that interact non-specifically with the NCs. This phenomenon may open the possibility of targeting a biomarker that acts as a quencher, leading to an inversely proportional relation between the fluorescence intensity of the NCs and the concentration of the target biomarker. It is also important to address the potential drawbacks of using metal NCs in living cells when the biomarker detection is based on imaging techniques, such as cellular internalization and cellular viability.
Fluorescence spectroscopy is the most suitable tool to quantify the fluorescence emitted by the NCs when excited at a certain wavelength.37 Although these measurements are usually very robust, some interferences can appear, mostly Rayleigh and Raman scattering.38 On the one hand, Rayleigh scattering occurs when there is an elastic scattering of light by particles smaller than the wavelength of the incoming light,39 so it is often observed when working with small nanoparticles. This phenomenon is even larger when exciting at shorter wavelengths.40 On the other hand, Raman scattering is an inelastic scattering that depends on the resolution of the monochromators, and can be identified because it always appears at the same wavelength difference from the excitation light due to the molecular fingerprint of the vibrational states of the sample.41 In addition, the inner filter effect (IFE), i.e. the absorption of the excitation and/or emission light by the sample is a common problem in concentrated solutions of nanomaterials.42 The aforementioned spectral distortions can be critical and need to be considered, minimized, and corrected appropriately.43 Besides, the photoluminescence of NCs can also be used in fluorescence imaging,44,45 similarly to the staining tools and biomolecular tags based on fluorescent proteins. For example, NCs have been applied to reveal the distribution pattern of the selected target molecule inside living organisms and cells.
There are many possibilities when fluorescence detection is applied as a biosensing tool due to its high sensitivity, which can even be improved by the use of fluorescent nanoclusters. Several nanocluster properties can be exploited for sensing, including (1) Fluorescence Resonance Energy Transfer (FRET), which involves energy transfer between donor and acceptor elements that can be followed by a decrease of fluorescence at a certain wavelength linked to an increase at the acceptor emission wavelength and (2) Aggregation Induced Emission (AIE),46 a phenomenon that increases sensitivity when working with fluorophores. An example of AIE and 3D imaging described by Shamsipur et al.47 shows the enhancement of DNA-AgNC fluorescence due to its hybridization with graphene oxide (GO) and its use as a biosensing tool. In this case, the nanocluster fluorescence is quenched upon interaction with the target, cytochrome C (Cyt C).
Fluorescence microscopy is a suitable technique for imaging and quantification by different assays since it presents high sensitivity and the possibility of optical sectioning that provides very clear images of sub-cellular structures and, depending on the equipment, 3D reconstructions can be performed. An example of the use of a confocal microscope for imaging showed the detection of proteins in the cell surface,48 which are key regulatory elements in signal transduction and therefore potential biomarkers for physiological and pathological processes inside the cell.49 Folate Receptor (FR) is a cell surface protein overexpressed in specific malignant epithelial tumours.50 The detection assay relies on a reprogrammable DNA sequence that is specifically recognized by FRs, so FR can be imaged when the DNA sequence is used for the templating of fluorescent AgNCs. This method was applied for in vivo targeting and imaging of FRs on HeLa cells using Laser Confocal Scanning Microscopy (LCSM). Other studies combined both techniques, using LCSM to verify the accumulation of NCs in cells and fluorescence spectroscopy, which has higher sensitivity, to accurately quantify the fluorescence signal of the NCs.
Although fluorescence microscopy is a widely established technique, recent research efforts have been focused on improved imaging methodologies, for example, recent reports on in situ imaging with very high sensitivity applied to in vivo systems.51,52 The main advantage of the in situ set-up is the fact that the accumulation of certain molecules can be observed by the formation of fluorescent nanoclusters53 or the in situ monitoring of a biological reaction that leads to the formation or consumption of the target.54In vivo studies provide the possibility of following the progression over days in real biological systems.52 Increasing the resolution of biosensor imaging is also a challenge, and some pioneering techniques are reaching this goal with multicolour images of cells at 5–10 nm single-molecule localization precision in 3D.
Fluorescent nanocluster-based biomarker detection systems: examples and mechanisms
Biomarkers in cancer
One of the main biosensing applications focuses on the detection of cancer-related biomarkers. Cancer is the sixth most prevalent cause of death in the world as of 2016.55 Because of this cancer biomarkers are required tools to detect and assess the stage of the disease. The mechanisms of a non-tumour cell are determined by the cell cycle, an ordered set of events and checkpoints in the cell lifetime that controls its growth and division. Tumour phenomena break the control of the cell cycle over the proliferation potential of the cell,56 which leads to unrestricted cell division as well as to the up- and down-regulation of different molecules that can be considered tumour biomarkers. Due to the high percentage of the population suffering from this disease, there are many molecules being evaluated as biomarkers for diagnosis, determination of aggressiveness, treatment selection and prognosis. Tumour markers are defined as anything present in or produced by cancerous cells or other cells in response to cancer processes, which can be found in the blood, urine, and other tissues or body fluids. These markers have traditionally been mostly proteins, but in the last few decades genetic markers have allowed the detection of tumour gene expression. Although a unique tumour marker is not present in all types of cancer, some markers can be found in several different cancers. Currently, some of the most widely used and approved biomarkers for cancer diagnosis are the following: carcinoembryonic antigen,57 which is characteristic of colorectal cancer; HER2, a cell receptor that can be found in tissue samples from breast, ovarian, bladder, pancreatic and stomach cancer;58,59 lactate dehydrogenase, an enzyme found in the blood from lymphoma, leukaemia, melanoma and neuroblastoma patients;60,61 and prostate specific antigen or PSA, which allows the detection of prostate cancer.62 Over the past few years, the need for more molecules on which clinics can rely to diagnose cancer has led to a wide number of studies on molecules not yet recognized or approved for clinical trials, but which are under scrutiny for their feasibility as cancer biomarkers. These biomarkers are classified depending on their nature (Table 1).
Table 1 Summary of tumour biomarkers explained in this review including information of interest about the NC-based methods applied to detect them
Biomarker |
Type |
NCs |
λ
em (nm) |
Selectivity |
Assay |
Assay media |
LOD |
Linear range |
Applied in real biological samples.
Applied in clinical trials.
|
Cyt C47a |
Genetic |
DNA-AgNCs-GO |
610 |
High |
3D imaging |
Breast cancer cells |
— |
— |
miRNA-155 (ref. 66)b |
Genetic |
ssDNA-CuNCs |
580 |
High |
FL spectroscopy |
Saliva and human plasma |
11 pM |
50 pM to 10 nM |
miRNA-21 (ref. 65)b |
Genetic |
DNA-AuNCs |
450 |
Very high |
FL spectroscopy |
Human plasma |
0.7 pM |
1 pM to 10 nM |
Tyrosinase68 |
Protein |
Dopamine-Au/AgNCs |
426 |
Medium-high |
FL spectroscopy |
Phosphate buffer pH = 7.0 |
13.5 mU mL−1 |
45–319 mU mL−1 |
Telomerase69a |
Protein |
DNA-AgNCs |
635 |
Medium-high |
FL spectroscopy/imaging |
PBS pH = 7.0 |
50 cells |
— |
Terminal deoxynucleotidyl transferase (TdTase)72a |
Protein |
DNA-AgNCs |
585 |
High |
FL spectroscopy |
PBS pH = 8.0 |
0.8 mU mL−1 |
1–35 mU mL−1 |
Anterior Gradient Protein (AGR)73a |
Protein |
Aptamer-AgNCs |
488 |
Medium |
Imaging |
Breast cancer cells |
— |
— |
Mucins75a |
Protein |
PB-AuNCs |
580 |
Medium-high |
Flow cytometry |
Human plasma |
25 μg mL−1 |
0–1000 μg mL−1 |
Mucins76a |
Protein |
CuNCs |
317 |
High |
Imaging |
Breast cancer cells |
— |
— |
Heat Shock Protein 90 (Hsp90)78 |
Protein |
AuNCs |
440 |
Medium-high |
FL spectroscopy |
PBS pH = 7.4 |
20 μM (46 μg mL−1) |
5–150 μM |
Protein Tyrosine Kinase-7 (PTK-7)80a |
Protein |
ssDNA-AgNCs |
650 |
Medium-low |
FL spectroscopy |
PBS pH = 7.4 |
12 pM |
30 pM to 2 nM |
Cytochrome C (Cyt C)81a |
Protein |
DNA-AgNCs/haemoglobin-AuNCs |
440/610 |
Medium-high |
FL spectroscopy |
PBS pH = 7.4 |
186 ng mL−1 |
0–12 400 ng mL−1 |
Cytochrome C (Cyt C)82a |
Protein |
GSH-AuNCs |
625 |
Medium-high |
FL spectroscopy |
Human serum |
80 ng mL−1 |
20–100 000 mg mL−1 |
Human urine |
Genetic biomarkers.
Nucleic acids are promising molecules63 to detect changes in gene expression in cancerous cells. Their sequence-dependent specificity and their favourable chemical nature for the templating of metal NCs make nucleic acids valid candidates for quantifying and imaging gene-related events in cells. The most commonly used mechanism is the stabilization of NCs on a small nucleic acid fragment (such as RNA) that acts as a molecular beacon changing its fluorescence when it hybridizes with the target sequence.64 For instance, miRNA-155 has been used in several studies as the target molecule,65 due to its overexpression in tumour tissues, where it suppresses the expression of antioncogenes in apoptosis, metastasis, tissue invasion and cell proliferation. Borghei et al.66 described a method to quantify miRNA-155 using a system based on the hybridization of a custom single strand DNA molecule (ssDNA) which was also designed to template CuNCs. This system relies on the luminescence enhancement and on the Stokes shift of the CuNC emission that occurs as a result of the specific hybridization, which results in an excellent selectivity, a detection range from 50 pM to 10 nM, and a Limit of Detection (LOD) of 11 pM. Similarly, the same group also reported a sensor based on DNA-stabilized AuNCs for the detection of miRNA-21,65 a marker present in most solid tumours derived from epithelial cells, as well as in myeloma, glioblastoma, or leukaemia. This system provides a LOD of 0.7 pM and detection within the range of 1 pM to 10 nM. These sensors have been applied in clinical trials. Since both miRNA-155 and miRNA-21 are overexpressed in some cancer types, the latter system provides better sensitivity (Table 1) leading to detection at earlier stages.
Protein biomarkers.
Proteins are commonly used as markers for cancer detection due to their role in regulatory pathways as well as the importance of enzymes in maintaining the cell homeostasis. The general mechanisms involving the design of NC-based biosensors are based mainly on the use of the substrate of the target enzyme as a template for NCs. For instance, tyrosinase is a Cu-containing enzyme involved in melanin synthesis, and therefore its activity is implicated in melanoma development.67 Dopamine stabilized AuNCs and AgNCs68 allow the fluorescence quantification of tyrosinase activity, dopamine being its substrate. The quenching effect of the NC emission when tyrosinase recognizes the dopamine-NC system can be explained by the partial oxidation of dopamine, which hinders the photoinduced electron transfer (PET) between the NCs and the dopamine. This translates to a decrease of fluorescence emission when tyrosinase is present in a linear range between 45 and 319.5 mU mL−1 and at a LOD of 13.5 mU mL−1. Unfortunately, this method has only been tested with mushroom tyrosinase, and not human samples. An interesting combined approach of LCSM imaging and fluorescence spectroscopy was used for the detection and quantification of telomerase activity,69 which is considered a tumour biomarker due to its activity prolonging the telomeres, thus facilitating out of control cellular proliferation.70 This work evaluated the intracellular telomerase activity in different cell types using AgNCs stabilized by the telomerase-substrate DNA sequence. When telomerases extend the DNA substrate sequence adding a guanine-rich region, AgNCs increase their fluorescence due to the guanine proximity. Since the fluorescence is directly related to the telomerase activity, the increase of fluorescence means an increase on telomerase presence. In addition, a calibration curve was achieved to quantify the fluorescence, and thus the telomerase concentration, spectroscopically. The same mechanism was used for the detection of terminal deoxynucleotidyl transferase (TdTase), a tissue-specific enzyme involved in the rearrangement of lymphocyte antigen receptor genes on immature lymphocytes and acute lymphoblastic leukaemia cells.71 Chi et al.72 developed AgNCs templated by a guanine-rich DNA that bind specifically to TdTase, thus being able to quantify the enzymatic activity in a linear range between 1 and 35 mU mL−1 with a LOD of 0.8 mU mL−1. Another similar approach is to use aptamers as scaffolds for NCs. Lan et al.73 described a method to detect anterior gradient protein 2 homolog (AGR), a protein that hinders the cancer-protective role of p53 on glandular cells, so this protein is overexpressed in breast, pancreas or prostate cancer. Using AgNCs templated by an aptamer that allows the recognition of AGR, this group detected the protein in MCF-7 cells (breast cancer), while they did not detect it in non-glandular cancer cells (HeLa cells) using LCSM.
Not only enzymes, but other proteins have also been demonstrated to be useful as biomarkers, specially membrane or secreted proteins that have roles in cell signalling. Mucins, for example, are highly glycosylated secreted proteins that have an essential role in mucosa tissues, maintaining the polarization of epithelial cells,74 and thus its de-regulation implies cell proliferation and invasion. Dutta et al.75 reported a method to detect them using AuNCs stabilized with phenylboronic acid (PB), which can perform as a theragnostic molecule, being able to both label and treat cancer cells. PB binds mucins strongly and has a role as anticancer drug. The analytical parameters for this sensor are a LOD of 25 μg mL−1 and a calibration curve that follows a 2nd order polynomial equation from 0 to 10 mg mL−1. Huang et al.76 developed other sensor for mucins based on the interaction between cyclodextrin-functionalized CuNCs and di(adamantan-1-yl)phosphine used as a connector for the aggregation of the CuNCs, which improves their fluorescence and easy localization. The aggregated NCs are bound to mucin molecules using an aptamer. Heat Shock Proteins (Hsp) also have potential clinical uses as biomarkers for cancer diagnosis and prognosis.77 In this sense, Couleaud et al.78 designed a protein-NC sensor based on a previously engineered Hsp90-binding domain79 that enabled the stabilization of AuNCs. The fluorescence of the nanocluster specifically responds to the interaction with Hsp90, which allows the detection of target protein with a LOD of 46 μg of Hsp90 per mL. Other studies focused on membrane proteins that are also implied in signalling pathways and used the strategy of fluorescent AgNCs templated by ssDNA as previously described, which are designed to bind selectively protein tyrosine kinase-7.80
As a model biomarker, cytochrome C (Cyt C) deserves a special mention. It is a well-known molecule implicated in the early stages of cell apoptosis, and its malfunction is related to cell proliferation. Several strategies have been designed to detect Cyt C, such as DNA templated AgNCs,81 haemoglobin-AuNCs,81 and glutathione-capped NCs82 (Table 1), all of which rely on the quenching effect of the NC emission promoted by the presence of Cyt C.
Biomarkers in non-tumour diseases
Although the use of biomarkers in cancer is very extensive due to the need for diagnosis in the early stages of the disease, there are many other health conditions for which patients can take advantage of an earlier diagnosis (Table 2). Furthermore, the strategies to follow are the same, for example the use of DNA83–85 or aptamers86 for the scaffolding of AgNCs that label a target miRNA when it is hybridized or the use of substrate molecules as stabilizing agents for the detection of enzyme activity. Interestingly, the use of fluorescent NCs combined with an elegant mechanism of molecular recognition allows detecting not only internal factors such as genetic expression or enzymatic activity, but also a parasitic infection, such as malaria. Wang et al.84 used AgNCs scaffolded on dsDNA combined with a designed aptamer that recognizes Plasmodium falciparum lactate dehydrogenase (PfLDH), an enzyme increased in the blood serum of malaria patients that transforms pyruvate to lactate. This method has a LOD of 7.4 pg L−1. As infected patient samples are typically in a concentration range of 3–15 pg μL−1, this strategy can be used to detect the infection, but not in the early stages. However, when the potential of the system was tested for clinical diagnosis in serum samples resulted in a LOD of 37 pg μL−1, revealing that further optimization is needed for its clinical use. Patients with vascular diseases also could be potentially benefited of the detection of miRNA-21, a nucleotide sequence related to cardiac hypertrophy and heart failure. Lu et al.85 reported a detection system of such a genetic marker using AgNCs templated by triplex molecular beacons (tMBs). tMBs are composed of ssDNA forming an oligomer that includes a quencher that hinders the NC emission in the absence of the target molecule. When miRNA-21 reacts with the molecular beacon it results in a conformational change that separates the quencher and therefore the fluorescence emission from the NCs increases. Another study described by Ardekani et al.86 consisted in a method to quantify the detection of lysozyme, an enzyme present in several body fluids and used as a biomarker in diseases such as nephropathy, kidney injury, or rheumatoid arthritis,87 using DNA-templated AgNCs. The mechanism involved takes advantage of the strong binding interaction between lysozyme and a designed aptamer (bound to the DNA-AgNCs). This interaction removes the aptamer from the AgNC complex, enhancing its fluorescence.
Table 2 Summary of non-tumour markers described in this review including information of interest about NC-based methods applied to detect them
Biomarker |
Disease |
NCs |
λ (nm) |
Selectivity |
Assay |
Assay media |
LOD |
Linear range |
Applied in real biological samples.
Applied in clinical trials.
|
P. falciparum lactate dehydrogenase (PfLDH)84b |
Malaria |
DNA-AgNCs |
555–535 |
High |
FL spectroscopy |
Calf serum |
7.4 μg mL−1 |
1–35 mU mL−1 |
PBS pH = 7.4 |
miRNA-21 (ref. 85) |
Vascular diseases cardiac hypertrophy heart failure |
ssDNA-AgNCs |
520 |
High |
FL spectroscopy |
Human serum |
0.18 nM |
1.5–250 nM |
PBS pH = 6.4 |
Lysozyme86a |
Nephropathy, kidney injury, or rheumatoid arthritis |
DNA-AgNCs |
610 |
High |
FL spectroscopy |
Tris acetate buffer pH = 6.5 |
5.6 nM |
2–25 nM |
Creatine89 |
Kidney functional activity |
GSH-CuNCs |
585 |
Medium |
FL spectroscopy |
Human serum |
0.63 μg L−1 |
2.5–34 μg L−1 |
Tris HCl buffer pH = 7.0 |
Bilirubin90 |
Liver diseases |
BSA-AuNCs |
638 |
Medium-low |
FL spectroscopy |
PBS pH = 7.4 |
0.61 μM |
0.8–50 μM |
Dipicolinic acid91 |
Anthrax |
GSH-AuNCs |
370 |
High |
FL spectroscopy |
HEPES pH = 7.0 |
0.1 μM |
1–120 μM |
Zinc92a |
Alzheimer's disease |
ZnNCs |
670 |
Medium-high |
Imaging |
In vivo (mice) |
— |
— |
Scavengers receptors AI (SR-AI)94a |
Atherosclerosis |
GSH-Au/GdNCs |
600 |
Medium-high |
Imaging |
In vivo (mice) |
— |
— |
One of the main mechanisms of detection is based on the NC emission quenching effect when the biosensor is linked to the target biomarker. Some examples include the detection of creatinine in kidney functional activity disorders,88 using GSH-CuNCs that are destabilized in the presence of creatinine;89 the detection of bilirubin in the diagnosis of liver diseases using BSA stabilized AuNCs that disassemble when the complex BSA-bilirubin is formed;90 or the detection of miRNA 145 using a AgNC-DNA biosensor in the diagnosis of multiple sclerosis (MS) based on the hybridizing strategy already discussed. Halawa et al.91 used an eco-friendly approach to develop GSH-capped AuNCs initiated using samarium (Sm3+) lanthanides for the first time. This system allows the detection of dipicolinic acid, a robust biomarker for the identification of anthrax spores. The Sm3+ molecules interact strongly with dipicolinic acid, resulting in the fluorescence quenching of the AuNCs. Many other biosensing systems are focused on bioimaging the distribution pattern of the targeted biomarker in the body or tissue or inside the cells. This method is especially interesting in the diagnosis of diseases that hold a striking histological feature, such as neurological diseases based on the deposition of amyloids. Lai et al.92 reported a biosensor to diagnose Alzheimer's disease (AD). Aβ42 is a peptide derivative of the amyloid precursor protein (APP), which is a large transmembrane protein that forms aggregates implicated in the initiation of the pathogenic cascade. The amyloid cascade also modifies TAU, a microtubule-associated protein, leading to neurofibrillary tangle (NFT) formation. When this protein aggregates, during the formation of NFTs, it turns into insoluble disrupting the structure and functionality of the neuron. Taking this information into account, there are two possible biomarkers for AD, Aβ42 and the formation of neurofibrillary tangles. Many researches have demonstrated that copper and zinc are involved in the aggregation of Aβ42, so Lai et al.93 proposed a strategy based on in situ ZnONC synthesis to diagnose Alzheimer's disease. Taking advantage of the fact that zinc ions are able to pass through the blood–brain barrier, in vivo fluorescence imaging revealed the accumulation of ZnONCs on the AD affected area in the brain, which can be related to the stage of the disease. This study reveals a new strategy for effective diagnosis of AD.
Cardiovascular diseases also can be tracked using LCSM bioimaging, for example, by imaging scavenger receptors AI (SR-AI), which are phagocytic recognition receptors of endogenous and exogenous materials and play an important role as biomarkers. Wang et al.94 developed a method to detect plaques by fluorescence imaging of Au-GdNCs stabilized by glutathione,95 which were functionalized with a novel SR-AI specific peptide, PP1, in order to recognize the biomarker. The biodistribution of the biomarker was analyzed in in vivo studies (mice) by confocal microscopy allowing a semi-quantitative analysis by comparison of fluorescence intensities obtained for different individuals.
Conclusions and future perspectives
The use of nanoclusters as fluorescent reporters for the detection of biomarkers is increasing due to their stability and tuneable optical properties, and the huge variety of biomolecules with recognition capabilities used for their conjugation. For this purpose, NCs are commonly synthesized using a templating strategy that avoids NC aggregation, leading to stable nanomaterials of a specific size. Since the NC radius (i.e. the number of atoms comprising the clusters) and ligands define their fluorescence properties, using templating agents translates to defined size and optical properties. In addition, the templating molecule can also provide recognition capability for molecular targets. Therefore, nanoclusters synthesized following this strategy have both selectivity for the analyte and tuneable fluorescence properties that allow the specific recognition, detection, and quantification of the target molecule.
Currently, a large number of research lines in the detection of biomarkers are related to the use of nanoparticles, quantum dots, and/or nanodots. There is also an increasing number of publications related to NCs, since they have great potential for biomarker detection due to their tuneable properties. Considering the publications to date in this area, DNA is the most widely used templating agent. This can be attributed to the high tunability of this type of molecule and the highly specific and designable recognition against the selected biomarkers. Likewise, glutathione is also extensively used, because it provides very stable NCs with defined optical properties. Other biomolecules used as templates for NCs and featured in this review, such as proteins, are currently less widespread in clinical applications.
Depending on the purpose of the assay, two different strategies are the most commonly used to measure the fluorescence intensity of NCs. Imaging by fluorescence confocal microscopy is the one selected if the distribution of the target molecule within a cell or a tissue needs to be known. Meanwhile, fluorescence spectroscopy is the appropriate technique if more accurate quantitative results are required. Both techniques can be used on their own but together they allow an exhaustive study of the behaviour and concentration of the biomarkers.
The key to using NCs as fluorescent reporters in biosensing strategies is the fact that their optical properties can be tuned, leading to their specific detection and quantification. An additional asset is the fact that fluorescence techniques are more sensitive than absorption spectrometry techniques that are used for the detection of nanoparticles, and therefore lower biomarker concentrations can be detected leading to an early stage diagnosis of diseases.
These advantages make NCs an increasingly interesting detection and biosensing tool because depending on their nature and synthetic strategy they can be used in both in vitro and in vivo assays due to their biocompatibility. NC-based sensors have been shown to be useful in the detection of specific biomarkers and their tuneable fluorescence properties allow their quantification, which can be detected by several easy to handle techniques.
Conflicts of interest
There are no conflicts to declare.
Acknowledgements
The authors would like to acknowledge the Basque Country Government for funding (Elkartek KK-2017/00008, and COVID-2020/00010), the Center for Cooperative Research in Biomaterials (CIC biomaGUNE) and the GAIKER Technology Centre, Basque Research and Technology Alliance (BRTA). This work was partially supported by the European Research Council (ERC-CoG-648071-ProNANO and ERC-PoC-841063-NIMM) and the Agencia Estatal de Investigación, Spain (BIO2016-77367-C2-1-R and PID2019-111649RB-I00). This work was performed under the Maria de Maeztu Units of Excellence Program from the Spanish State Research Agency – Grant No. MDM-2017-0720 (CIC biomaGUNE). E. L.-M. thanks the Spanish Ministry of Science and Innovation for the FPI grant (BES-2017-079646).
Notes and references
- I. Freestone, N. Meeks, M. Sax and C. Higgitt, Gold Bull., 2007, 40, 270–277 CrossRef CAS.
- M. Faraday, Philos. Trans. R. Soc. London, 1857, 147, 145–181 CrossRef.
- Q. Zhang, M. Yang, Y. Zhu and C. Mao, Curr. Med. Chem., 2018, 25, 1379–1396 CrossRef CAS.
- J. Yang, F. Wang, H. Yuan, L. Zhang, Y. Jiang, X. Zhang, C. Liu, L. Chai, H. Li and M. Stenzel, Nanoscale, 2019, 11, 17967–17980 RSC.
- I. Chakraborty and T. Pradeep, Chem. Rev., 2017, 117, 8208–8271 CrossRef CAS.
- A. Aires, E. Lopez-Martinez and A. L. Cortajarena, Biosensors, 2018, 8, 110 CrossRef CAS.
- N. L. Rosi and C. A. Mirkin, Chem. Rev., 2005, 105, 1547–1562 CrossRef CAS.
- A. Aires, I. Llarena, M. Moller, J. Castro-Smirnov, J. Cabanillas-Gonzalez and A. L. Cortajarena, Angew. Chem., Int. Ed., 2019, 58, 6214–6219 CrossRef CAS.
-
Functional Nanometer-Sized Clusters of Transition Metals: Synthesis, Properties and Applications, The Royal Society of Chemistry, ed. W. Chen and S. Chen, 2014 Search PubMed.
- M. A. Habeeb Muhammed, S. Ramesh, S. S. Sinha, S. K. Pal and T. Pradeep, Nano Res., 2008, 1, 333–340 CrossRef.
-
N. Goswami, J. Li and J. Xie, in Frontiers of Nanoscience, ed. T. Tsukuda and H. Häkkinen, Elsevier, 2015, vol. 9, pp. 297–345 Search PubMed.
- Y. Du, H. Sheng, D. Astruc and M. Zhu, Chem. Rev., 2020, 120, 526–622 CrossRef CAS.
- L. Shang, S. Dong and G. U. Nienhaus, Nano Today, 2011, 6, 401–418 CrossRef CAS.
- M. M. F. Baig and Y.-C. Chen, Analyst, 2019, 144, 3289–3296 RSC.
- B. S. Ramesh, E. Giorgakis, V. Lopez-Davila, A. K. Dashtarzheneha and M. Loizidou, Nanotechnology, 2016, 27, 285101 CrossRef.
- J. Xie, Y. Zheng and J. Y. Ying, J. Am. Chem. Soc., 2009, 131, 888–889 CrossRef CAS.
- O. I. Wilner and I. Willner, Chem. Rev., 2012, 112, 2528–2556 CrossRef CAS.
- Z. Wu and R. Jin, Nano Lett., 2010, 10, 2568–2573 CrossRef CAS.
- C. Song, J. Xu, Y. Chen, L. Zhang, Y. Lu and Z. Qing, Molecules, 2019, 24, 4189 CrossRef CAS.
- H. Häkkinen, Nat. Chem., 2012, 4, 443–455 CrossRef.
- W. A. Colburn, J. Clin. Pharmacol., 2003, 43, 329–341 CrossRef.
- K. Strimbu and J. A. Tavel, Curr. Opin. HIV AIDS, 2010, 5, 463–466 CrossRef.
-
M. L. Lim, P. Jungebluth and P. Macchiarini, in Translational Regenerative Medicine, ed. A. Atala and J. G. Allickson, Academic Press, Boston, 2015, pp. 449–456 Search PubMed.
- R. Dienstmann, J. Rodon and J. Tabernero, Curr. Opin. Oncol., 2013, 25, 305–312 CrossRef CAS.
-
T. Brody, Nutritional Biochemistry, Academic Press, 1999 Search PubMed.
- O. Bratt, H. Garmo, J. Adolfsson, A. Bill-Axelson, L. Holmberg, M. Lambe and P. Stattin, J. Natl. Cancer Inst., 2010, 102, 1336–1343 CrossRef CAS.
- S. L. Chang, L. C. Harshman and J. C. Presti, J. Clin. Oncol., 2010, 28, 3951–3957 CrossRef.
- C. A. Purdie, L. Baker, A. Ashfield, S. Chatterjee, L. B. Jordan, P. Quinlan, D. J. A. Adamson, J. A. Dewar and A. M. Thompson, Br. J. Cancer, 2010, 103, 475–481 CrossRef CAS.
- Z. Qing, A. Bai, S. Xing, Z. Zou, X. He, K. Wang and R. Yang, Biosens. Bioelectron., 2019, 137, 96–109 CrossRef CAS.
- X. Li, L. Han, Y. Guo, Y. Chang, J. Yan, Y. Wang, N. Li, Y. Ding and J. Cai, New J. Chem., 2019, 43, 7997–8004 RSC.
- F. Berger and M. F. Reiser, Theranostics, 2013, 3, 943–952 CrossRef CAS.
- J. Carvalho and C. Oliveira, Front. Immunol., 2015, 5, 685 Search PubMed.
- Y. Yu, Y. Yang, J. Ding, S. Meng, C. Li and X. Yin, Anal. Chem., 2018, 90, 13290–13298 CrossRef CAS.
- N. Hayashi and H. Yamauchi, Breast Cancer, 2012, 19, 110–117 CrossRef.
- J. Zheng, C. Zhang and R. M. Dickson, Phys. Rev. Lett., 2004, 93, 077402 CrossRef.
- P. Londoño-Larrea, J. P. Vanegas, D. Cuaran-Acosta, E. Zaballos-García and J. Pérez-Prieto, Chem.–Eur. J., 2017, 23, 8137–8141 CrossRef.
-
D. S. Smith, M. Hassan and R. D. Nargessi, in Modern Fluorescence Spectroscopy, ed. E. L. Wehry, Springer US, Boston, MA, 1981, pp. 143–191 Search PubMed.
- L. D. Barron and A. D. Buckingham, Mol. Phys., 1971, 20, 1111–1119 CrossRef CAS.
-
Y. Band and Y. Avishai, Quantum Mechanics with Applications to Nanotechnology and Information Science, ScienceDirect, 2013 Search PubMed.
-
Nanoparticle Technology Handbook, Elsevier, 2018 Search PubMed.
-
Y. Dwivedi, in Molecular and Laser Spectroscopy, ed. V. P. Gupta and Y. Ozaki, Elsevier, 2020, pp. 483–520 Search PubMed.
- S. Kumar Panigrahi and A. Kumar Mishra, J. Photochem. Photobiol., C, 2019, 41, 100318 CrossRef CAS.
- T. Wang, L.-H. Zeng and D.-L. Li, Appl. Spectrosc. Rev., 2017, 52, 883–908 CrossRef CAS.
- L.-Y. Chen, C.-W. Wang, Z. Yuan and H.-T. Chang, Anal. Chem., 2015, 87, 216–229 CrossRef CAS.
- X. Wu, X. He, K. Wang, C. Xie, B. Zhou and Z. Qing, Nanoscale, 2010, 2, 2244–2249 RSC.
- W.-X. Wang, Y. Wu and H.-W. Li, J. Colloid Interface Sci., 2017, 505, 577–584 CrossRef CAS.
- M. Shamsipur, K. Molaei, F. Molaabasi, S. Hosseinkhani, A. Taherpour, M. Sarparast, S. E. Moosavifard and A. Barati, ACS Appl. Mater. Interfaces, 2019, 11, 46077–46089 CrossRef CAS.
- D.-J. Huang, Z. Wu, R.-Q. Yu and J.-H. Jiang, Talanta, 2018, 190, 429–435 CrossRef CAS.
- R. Lund, R. Leth-Larsen, O. N. Jensen and H. J. Ditzel, J. Proteome Res., 2009, 8, 3078–3090 CrossRef CAS.
- C.-Y. Ke, C. J. Mathias and M. A. Green, Adv. Drug Delivery Rev., 2004, 56, 1143–1160 CrossRef CAS.
- Z. Qing, J. Hu, J. Xu, Z. Zou, Y. Lei, T. Qing and R. Yang, Chem. Sci., 2020, 11, 1985–1990 RSC.
- Z. Qing, J. Xu, J. Hu, J. Zheng, L. He, Z. Zou, S. Yang, W. Tan and R. Yang, Angew. Chem., Int. Ed., 2019, 58, 11574–11585 CrossRef CAS.
- J. Li, X. Zhong, F. Cheng, J.-R. Zhang, L.-P. Jiang and J.-J. Zhu, Anal. Chem., 2012, 84, 4140–4146 CrossRef CAS.
- D. Li, Z. Qiao, Y. Yu, J. Tang, X. He, H. Shi, X. Ye, Y. Lei and K. Wang, Chem. Commun., 2018, 54, 1089–1092 RSC.
-
WHO, Top 10 causes of death, http://www.who.int/gho/mortality_burden_disease/causes_death/top_10/en/, accessed September 14, 2020 Search PubMed.
- D. Hanahan and R. A. Weinberg, Cell, 2011, 144, 646–674 CrossRef CAS.
- J. Liu, H.-W. Li and Y. Wu, RSC Adv., 2017, 7, 13438–13443 RSC.
- J. T. Jørgensen and M. Hersom, J. Cancer, 2012, 3, 137–144 CrossRef.
- T. Cooke, J. Reeves, A. Lanigan and P. Stanton, Ann. Oncol., 2001, 12, S23–S28 CrossRef.
- A. B. Philipp, D. Nagel, P. Stieber, R. Lamerz, I. Thalhammer, A. Herbst and F. T. Kolligs, BMC Cancer, 2014, 14, 245 CrossRef.
- R. J. Schneider, K. Seibert, S. Passe, C. Little, T. Gee, B. J. Lee, V. Miké and C. W. Young, Cancer, 1980, 46, 139–143 CrossRef CAS.
- I. M. Thompson, J. Nutr., 2006, 136, 2704S CrossRef CAS.
- F. Xu, T. Qing and Z. Qing, Nano Today, 2021, 36, 101021 CrossRef.
- J. M. Obliosca, M. C. Babin, C. Liu, Y.-L. Liu, Y.-A. Chen, R. A. Batson, M. Ganguly, J. T. Petty and H.-C. Yeh, ACS Nano, 2014, 8, 10150–10160 CrossRef CAS.
- M. Hosseini, E. Ahmadi, Y.-S. Borghei and M. Reza Ganjali, Methods Appl. Fluoresc., 2017, 5, 015005 CrossRef.
- Y.-S. Borghei, M. Hosseini and M. R. Ganjali, Microchim. Acta, 2017, 184, 2671–2677 CrossRef CAS.
- R. Sharman, K. Sullivan, R. M. Young and J. McGill, Gene, 2012, 504, 288–291 CrossRef CAS.
- H. Ao, Z. Qian, Y. Zhu, M. Zhao, C. Tang, Y. Huang, H. Feng and A. Wang, Biosens. Bioelectron., 2016, 86, 542–547 CrossRef CAS.
- S. Huang, H. Yao, W. Wang, J.-R. Zhang and J.-J. Zhu, J. Mater. Chem. B, 2018, 6, 4583–4591 RSC.
- K. Collins and J. R. Mitchell, Oncogene, 2002, 21, 564–579 CrossRef CAS.
- O. Peralta-Zaragoza, F. Recillas-Targa and V. Madrid-Marina, Immunology, 2004, 111, 195–203 CrossRef CAS.
- B.-Z. Chi, C.-L. Wang, Z.-Q. Wang, T. Pi, X.-L. Zhong, C.-Q. Deng, Y.-C. Feng and Z.-M. Li, Mikrochim. Acta, 2019, 186, 241 CrossRef.
- J. Lan, X. Wu, L. Luo, J. Liu, L. Yang and F. Wang, Talanta, 2019, 197, 86–91 CrossRef CAS.
- D. W. Kufe, Nat. Rev. Cancer, 2009, 9, 874–885 CrossRef CAS.
- D. Dutta, S. K. Sailapu, A. Chattopadhyay and S. S. Ghosh, ACS Appl. Mater. Interfaces, 2018, 10, 3210–3218 CrossRef CAS.
- Y. Huang, J. Ji, J. Zhang, F. Wang and J. Lei, Chem. Commun., 2019, 56, 313–316 RSC.
- J. Wu, T. Liu, Z. Rios, Q. Mei, X. Lin and S. Cao, Trends Pharmacol. Sci., 2017, 38, 226–256 CrossRef CAS.
- P. Couleaud, S. Adan-Bermudez, A. Aires, S. H. Mejías, B. Sot, A. Somoza and A. L. Cortajarena, Biomacromolecules, 2015, 16, 3836–3844 CrossRef CAS.
- A. L. Cortajarena, F. Yi and L. Regan, ACS Chem. Biol., 2008, 3, 161–166 CrossRef CAS.
- Z. Liu, W. Chen, Y. Han, J. Ouyang, M. Chen, S. Hu, L. Deng and Y.-N. Liu, Talanta, 2017, 175, 470–476 CrossRef CAS.
- M. Shamsipur, F. Molaabasi, S. Hosseinkhani and F. Rahmati, Anal. Chem., 2016, 88, 2188–2197 CrossRef CAS.
- H. Li, M. Yang, D. Kong, R. Jin, X. Zhao, F. Liu, X. Yan, Y. Lin and G. Lu, Sens. Actuators, B, 2019, 282, 366–372 CrossRef CAS.
- N. Mansourian, M. Rahaie and M. Hosseini, J. Fluoresc., 2017, 27, 1679–1685 CrossRef CAS.
- W.-X. Wang, Y.-W. Cheung, R. M. Dirkzwager, W.-C. Wong, J. A. Tanner, H.-W. Li and Y. Wu, Analyst, 2017, 142, 800–807 RSC.
- S. Lu, S. Wang, J. Zhao, J. Sun and X. Yang, ACS Sens., 2018, 3, 2438–2445 CrossRef CAS.
- L. S. Ardekani, T. T. Moghadam, P. W. Thulstrup and B. Ranjbar, Plasmonics, 2019, 14, 1765–1774 CrossRef CAS.
- I. Torsteinsdóttir, L. Håkansson, R. Hällgren, B. Gudbjörnsson, N.-G. Arvidson and P. Venge, Rheumatology, 1999, 38, 1249–1254 CrossRef.
- E. P. Randviir and C. E. Banks, Sens. Actuators, B, 2013, 183, 239–252 CrossRef CAS.
- R. Jalili and A. Khataee, Microchim. Acta, 2018, 186, 29 CrossRef.
- Z. Li, W. Xiao, R. Huang, Y. Shi, C. Fang and Z. Chen, Sensors, 2019, 19, 7 Search PubMed.
- M. I. Halawa, B. S. Li and G. Xu, ACS Appl. Mater. Interfaces, 2020, 12, 32888–32897 CrossRef CAS.
- L. Lai, C. Zhao, M. Su, J. Ye, H. Jiang and X. Wang, Sci. Bull., 2015, 60, 1465–1467 CrossRef CAS.
- L. Lai, C. Zhao, M. Su, X. Li, X. Liu, H. Jiang, C. Amatore and X. Wang, Biomater. Sci., 2016, 4, 1085–1091 RSC.
- J. Wang, M. Wu, J. Chang, L. Li, Q. Guo, J. Hao, Q. Peng, B. Zhang, X. Zhang and X. Li, Nanomedicine, 2019, 19, 81–94 CrossRef CAS.
- J. Wang, M. Wu, J. Chang, L. Li, Q. Guo, J. Hao, Q. Peng, B. Zhang, X. Zhang and X. Li, Nanomedicine, 2019, 19, 81–94 CrossRef CAS.
- A. Aires, D. Maestro, J. Ruiz del Rio, A. R. Palanca, E. Lopez-Martinez, I. Llarena, K. Geraki, C. Sanchez-Cano, A. V. Villar and A. L. Cortajarena, Chem. Sci., 2021 10.1039/D0SC05215A.
Footnote |
† These authors contributed equally to this work. |
|
This journal is © The Royal Society of Chemistry 2021 |
Click here to see how this site uses Cookies. View our privacy policy here.