DOI:
10.1039/D0RA06677J
(Paper)
RSC Adv., 2021,
11, 2185-2193
Quantitative proteomics approach to investigate the antibacterial response of Helicobacter pylori to daphnetin, a traditional Chinese medicine monomer†
Received
2nd August 2020
, Accepted 11th December 2020
First published on 7th January 2021
Abstract
Helicobacter pylori is a Gram-negative bacterium related to the development of peptic ulcers and stomach cancer. An increasing number of infected individuals are found to harbor antibiotic-resistant H. pylori, which results in treatment failure. Daphnetin, a traditional Chinese medicine, has a broad spectrum of antibacterial activity without the development of bacterial resistance. However, the antibacterial mechanisms of daphnetin have not been elucidated entirely. To better understand the mechanisms of daphnetin's effect on H. pylori, a label-free quantitative proteomics approach based on an EASY-nLC 1200 system coupled with an Orbitrap Fusion Lumos mass spectrometer was established to investigate the key protein differences between daphnetin- and non-daphnetin-treated H. pylori. Using the criteria of greater than 1.5-fold changes and adjusted p value <0.05, proteins related to metabolism, membrane structure, nucleic acid and protein synthesis, ion binding, H. pylori colonization and infection, stress reaction, flagellar assembly and so on were found to be changed under daphnetin pressure. And the changes of selected proteins in expression level were confirmed by targeted proteomics. These new data provide us a more comprehensive horizon of the proteome changes in H. pylori that occur in response to daphnetin.
1. Introduction
Helicobacter pylori (H. pylori) is a microaerophilic Gram-negative bacterium with extremely demanding growth conditions which is linked to the development of duodenal ulcers and stomach cancer.1,2 Increasing evidence suggests that H. pylori may be associated with multiple chronic disorders, such as neurological disorders, cerebrovascular and cardiovascular disorders, etc.3,4 In 2017, the WHO published its first ever list of antibiotic-resistant “priority pathogens”, and H. pylori was regarded as one of the 12 high priorities.5 Standard triple therapy has been the recommended treatment for H. pylori eradication for the past two decades,6 leading to the rising of antibiotic-resistant rate. Due to the decreased efficacy of treatment and increased antibiotic resistance, a large amount of research has focused on the discovery of new antimicrobials.
Daphnetin (7,8-dihydroxycoumarin) is a natural coumarin derivative with anti-inflammation, anti-microorganism and antioxidative properties.7,8 To date, neither toxic effects nor genetic toxicity have been observed in the treatment of daphnetin.9,10 Therefore, inclusive research interests were attached to study the activity and mechanism of daphnetin against multidrug resistant (MDR) H. pylori. In our previous research, daphnetin showed good activities against MDR H. pylori ATCC 43504, with minimal inhibit concentration (MIC) values as 25 μg mL−1. And preliminary exploration of the antibacterial mechanisms of daphnetin is presumed to be the increased translocation of phosphatidylserine, nucleic acid damage, up-regulated RecA expression, and decreased H. pylori adhesion,11 but a lot of work need to do to get a more comprehensive explanation of the mechanism.
Quantitative proteomics based on mass spectrometry (MS) has emerged as a preferred strategy to discover condition-related proteins, and few comparative analysis studies of H. pylori by 2-DE/MS or immunoproteomics have been performed to look for differences in protein expression.12,13 Moreover, quantitative proteomics has been employed to study antibiotic reactions in various bacteria,14 which helped us explain the mechanisms of antibacterial drug actions by differences in protein expression. As the data-dependent acquisition (DDA)-based label-free method has the properties of simplified sample preparation and low costs due to no isotope labeling. And parallel reaction monitoring (PRM) is widely used in targeted proteomics, with a tolerance of approximately 10–20 ppm.15 In this study, label-free quantitative proteomics and PRM methods were used to discover the differentially expressed proteins under daphnetin pressure and dig the mechanisms of daphnetin's action on H. pylori. Based on previous research methods,14,16 we applied subinhibitory doses of daphnetin (1/2 MIC) to give the bacteria an opportunity to respond to daphnetin and to continue to grow. The plate culture method was chosen to culture the bacteria, as culturing with H. pylori is very demanding.
2. Materials and methods
2.1 Cell culture and antibiotic treatment
Frozen H. pylori ATCC 43504 was thawed and recovered on Columbia blood agar plates. H. pylori cells for proteomics analysis were incubated with or without 12.5 μg mL−1 daphnetin (Meilun Biotech Co., Ltd., Dalian, China) for 3 days at 37 °C under microaerobic conditions as previously described,17 then collected, adjusted to the same amount and washed in PBS twice by centrifugation, stored at −80 °C.
2.2 Sample and peptide preparation
H. pylori cells were resuspended in the lysis buffer (50 mM NH4HCO3, 150 mM NaCl, 2% sodium deoxycholate, pH 8.5) and sonicated at 4 °C to increase the extraction efficiency.18 For label-free proteomics, proteins were reduced with 10 mM dithiothreitol (DTT) at 95 °C for 5 min. Protein samples were digested by trypsin (1
:
50 w/w, Promega, Madison, WI) overnight at 37 °C. Then, formic acid was added to a final concentration of 1% to remove sodium deoxycholate. Last, the sample was desalted by C18 reverse-phase tips (Reprosil-Pur Basic C18, 5 μm, Dr Maisch GmbH). All samples were dried with a CHRIST Freeze Dryer ALPHA 2-4D (CHRIST, Vaihingen, Germany) and stored at −80 °C before use.
2.3 Label-free quantification analysis
Standard bottom-up proteomics technique was used to analyze the samples on a Thermo Scientific Orbitrap Fusion Lumos platform coupled with an EASY-nLC 1200.19 Peptides were enriched on a trap column (Reprosil-Pur Basic C18 (3 μm, Dr Maisch GmbH, Germany), 20 × 0.15 mm) and separated on a C18 column (Reprosil-Pur Basic C18 (1.9 μm, Dr Maisch GmbH, Germany) 150 × 0.15 mm) at a flow rate of 600 μL min−1. The solvent buffer A consists of water with 0.1% formic acid, and that of solvent B was 80% acetonitrile with 0.1% formic acid. After sample loading, the gradient started from 9% of buffer B and then from 9 to 12% of buffer B for 3 min. Then, the gradient increased from 12 to 28% of buffer B for 39 min and from 28 to 36% for 11 min. Finally, the gradient ascended to 95% of buffer B and was maintained for 7 min. Three biological replicates were analyzed and the scan sequence consist of an MS1 spectrum (Orbitrap analysis; resolution, 120
000; mass range, 350–1550 m/z; RF lens, 50%; AGC target, 4× 105; maximum injection time, 50 ms) and an MS2 analysis with HCD accompanied by the following conditions: collision energy, 32%; AGC, 5 × 104; maximum injection time, 22 ms; Orbitrap solution, 15
000; and isolation window, 1.6 Da. For database analyses, these initial data were searched against H. pylori ATCC 43504 and ATCC 26695 combined databases from UniProt by the Thermo Scientific™ Proteome Discoverer™ version 2.2 (PD2.2) software. Trypsin was specified for protein digestion with two missed cleavages allowed for each peptide. The search parameters were set as previously described.20
2.4 Bioinformatics analysis
The results were exported from PD 2.2 and analyzed by “Wu Kong” platform powered by R language (https://www.omicsolution.org/wkomics/main/). Both experiments were repeated in triplicate to generate three biological replicates and three technical duplications. Groups with values greater than or equal to 2/3 were included. Missing values left were imputed by the KNN method. For relative quantification, the label-free quantification intensities were transformed with log2 function. Proteins with a CV (coefficient of variance) value below 20% in three biological replicates were subjected to quantitative analysis and one-way ANOVA, and p value was adjusted by q value method. To identify significant differences, proteins with adjusted p < 0.05 and fold change >1.5 was considered to be up- or down-regulated. Functional annotation and clustering enrichment of differentially expressed proteins were conducted by using the Gene Ontology (GO) enrichment tool. Due to the lack of H. pylori ATCC 43504-related database in the KEGG pathway database, we replaced the gene name with the corresponding gene name of H. pylori ATCC 26695 by the Blast method to perform KEGG pathway analysis. For the analysis of interactions between proteins, differentially expressed proteins were searched against the STRING database (http://string-db.org) by the same ID-changed method used in the KEGG pathway analysis.
2.5 Mass spectrometry for label-free PRM analysis
PRM was used to confirm the differentially expressed proteins. The sample processing method was the same as described above. Both experiments were repeated in triplicate. The precursor m/z, charge state and retention time of peptides were referred to in the prior label-free experiments. The same Orbitrap Fusion Lumos platform and nanoLC parameters were applied for the PRM study. The targeted MS1 parameters were as follows: resolution, 60
000; AGC target, 2.0 × 105; and maximum injection time, 50 ms. PRM scanning was performed at 30
000 resolution, 1 × 105 AGC target, 54 ms maximum injection time and 0.7 m/z isolation window with peptides in ±5 min retention time windows. Fragmentation was performed with a normalized collision energy of 32.21
2.6 Target peptides quantification
Data from PRM analysis were analyzed by Skyline,22 with spectral libraries built by DDA results. Briefly, the FASTA sequences were downloaded from the UniProtKB database, and imported into Skyline while fragmentation and selection are performed if they can match peptides in the spectral libraries. 2 or 3 peptides per protein were selected for quantification. Experiments were repeated in triplicate to generate three biological replicates. The data were normalized by total ionic abundance and processed using a log2 scale. Peptides were selected by one-way ANOVA with p-value <0.05 and fold change >1.5.
3. Results
3.1 The overall proteomic response of H. pylori
Our previous study found that daphnetin inhibited H. pylori ATCC 43504 with MIC of 25 μg mL−1.11 Because full growth inhibition would be impractical for proteomic research, we applied a sublethal concentration of daphnetin and monitored changes. In order to avoid the gap in the amount of bacteria introducing by daphnetin, before the pretreatment, we adjusted the amount of bacteria in different groups to be consistent. As shown in Fig. 1, label-free and PRM quantitative proteomics were applied to analyze the protein expression level of daphnetin-treated H. pylori ATCC 43504. In total, we identified 1282 bacterial proteins in three biological replicates, covering approximately 78% of the H. pylori proteome. Proteins with a large proportion of missing values and a CV value greater than 20% were excluded, 1207 proteins were used to perform correlation analysis. Replicates in the same group presented a linear correlation with R2 > 0.9 (see Fig. S1 in the ESI†), indicating good repeatability. Based on adjusted p value (p < 0.05) and max fold change >1.5,14 the expression of 152 proteins were finally identified to be significantly changed by treatment with daphnetin (Fig. 2A). Among which, there were 55 proteins exhibited decreased accumulation and 97 proteins exhibited increased accumulation (Table S1 in the ESI†). The hierarchical clustering of values is shown in Fig. 2B and demonstrates that the response to daphnetin was reproducible.
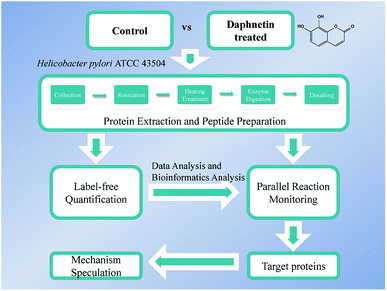 |
| Fig. 1 Quantitative proteomics workflow applied to daphnetin-treated and untreated H. pylori ATCC 43504. | |
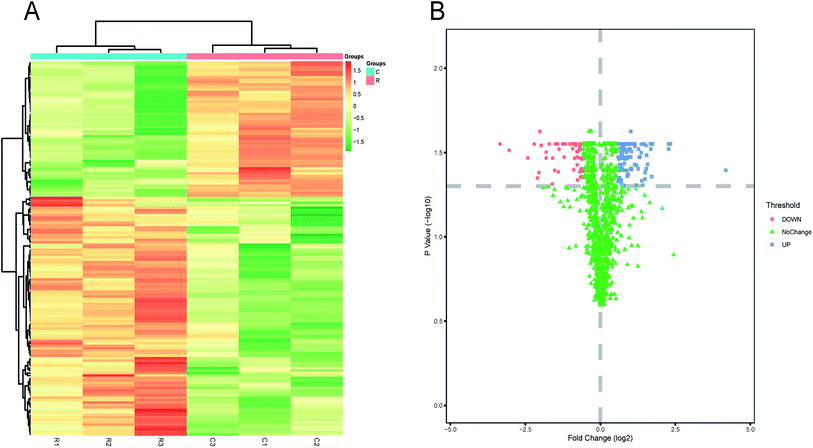 |
| Fig. 2 Protein profiles of H. pylori without and with daphnetin treatment. (A) Hierarchical clustering of the proteins' test after hypothetical test from three biological replicates. Red represents the highest relative expression, and green represents the lowest. C1, C2, and C3 (H. pylori controls); R1, R2, and R3 (daphnetin treated H. pylori). (B) Volcano distribution of log2 average normalized and scaled values for proteins from H. pylori with daphnetin treatment divided by p < 0.05 and fold change >1.5, red represents down-regulated and blue represents up-regulated. | |
3.2 Differentially expressed proteins in the daphnetin-treated H. pylori compared to control groups
By using GO annotation enrichment analysis, KEGG pathway analysis and STRING analysis as mentioned above, we further divided these differentially expressed proteins into different functional categories. As shown in Fig. S2 in the ESI,† the most enriched proteins in the GO annotation enrichment analysis were related to translation, purine nucleobase metabolic process, glycine metabolic process, ribosome biogenesis and various metabolic processes in the biological process category; cytoplasm, integral component of membrane, plasma membrane, ribosome, cell outer membrane and other membrane gradients in the cellular component category; and ATP binding, protein binding, metal ion binding, DNA binding, magnesium ion binding, RNA binding, etc. in the molecular function category. According to the KEGG pathway analysis results (see Fig. S3 in the ESI†), most of the proteins were involved in metabolic, secondary metabolite biosynthesis, microbial metabolism in diverse environments, carbon metabolism, biosynthesis of amino acids, epithelial cell signaling in H. pylori infection oxidative phosphorylation pathway, and etc., which means that daphnetin have extensive effects on the basic physiological activities of H. pylori. The results of STRING analysis (Fig. 3) showed that, there were clusters of genes which have strong interactions with each other, and genes such as tuf, tsf, rpsR, pheT, etc. constituted the hub of the interaction network, indicating the vital roles played by these genes. Based on these analysis results, proteins were chosen to perform targeted proteomics.
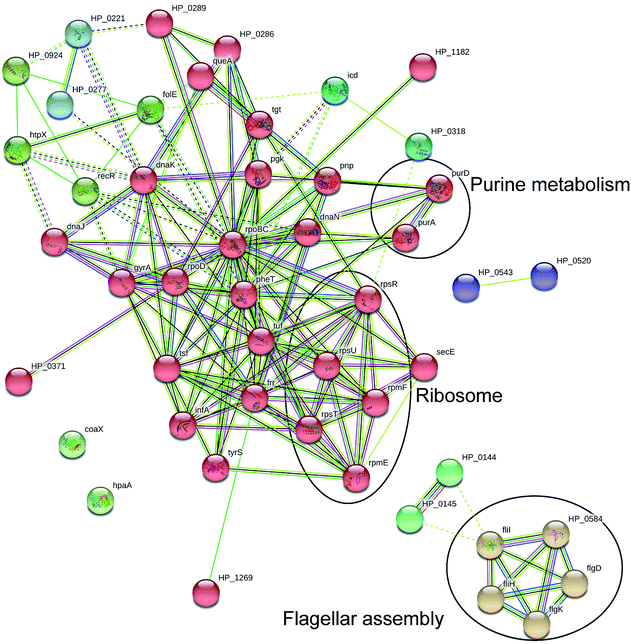 |
| Fig. 3 Protein–protein interaction networks of partial differentially expressed proteins under daphnetin stress. Interacting proteins were identified by using STRING with a high confidence score and clustered by MCL clustering, and homologous gene names in H. pylori ATCC 26695 were used due to the lack of an H. pylori ATCC 43504 database. Nodes represent proteins, while edges represent protein–protein interactions. Colored nodes represent clusters. Colored edges: known interactions from: teal, curated databases; magenta, experimentally determined; predicted interactions: green, gene neighborhood; red, gene fusions; blue, gene co-occurrence; other: yellow, text mining; black, co-expression; purple, protein homology. Partial KEGG pathways were marked by circle. | |
3.3 Targeted proteomics validation by PRM
In order to ensure reliable and repeatable measurements of protein expression in all the samples and to improve the sensitivity of the measurements, we applied a targeted MS-based method for defined protein quantification based on a PRM approach to validate protein expression differences. Peptides preparation process was the same as mentioned above. Three biological replicates were performed in all experiments. Protein candidates were selected from our label-free quantification measurements, and 2 or 3 unique peptides were chosen to quantify one protein. All raw data were analyzed and processed with Skyline software with spectral library built by DDA results. Peptides containing amino acids that are easily modified and missed cleavage were excluded from the analysis. A minimum dotp value of 0.7 was used to filter precursors to remove the noisy peaks and interfering fragment ions. The peptide peak areas were exported into Excel for data normalization by total ionic intensity and statistical analysis. In total, we reliably quantified 11 proteins with 25 peptides (see Table S2 in the ESI†) as significantly impacted proteins (adjusted p value <0.05 and fold change >1.5).
4. Discussion
In light to the latest severe drug resistance situation of H. pylori, discovering antibacterial drugs with new structures or new mechanisms is extremely imperative. Our previous studies have demonstrated that daphnetin has anti-H. pylori activity, regardless of the resistance patterns to other antibiotics. And the effect of daphnetin on H. pylori ATCC 43504 was initially explored. However, the antibacterial mechanisms of daphnetin have not been elucidated entirely. With the development of protein identification techniques, quantification proteomics by LC-MS has been gradually used in the discovery of drug-target and mechanism.14,23,24 In this study, the application of high-resolution mass spectrometry improved the accuracy and sensitivity of this approach. Three biological replicates are necessary because of the poor homogeneity of the microbial population. Normalization by total iron intensity is necessary in bacteria proteome analysis as the amount of bacteria is difficult to control accurately. PRM can solve problems when no antibody is available to help verify or when many protein needs to be quantified. By means of quantification proteomics, we found the mechanism of action of daphnetin was extensive and complex.
4.1 Extensive impacts of daphnetin on H. pylori ATCC 43504
As shown in Fig. 4, interestingly, among the differentially expressed proteins, membrane structure-related proteins accounted for the largest proportion, and approximately 1/3 of these proteins were down-regulated, while approximately 2/3 of those were up-regulated, which further verified the membrane structure changes induced by daphnetin found in the previous study.11 Apart from membrane structure-related proteins, the expression of proteins related to acid metabolic and nucleotide metabolic processes were stimulated, while many biosynthetic processes were suppressed by daphnetin, leading to the imbalance between synthesis and metabolism. What's more, up-regulated expression of ion binding related proteins and down-regulated expression of RNA binding, structural constituent of ribosome and structural molecule activity related proteins were also observed in daphnetin-treated groups, indicating the declining of the cell activities.
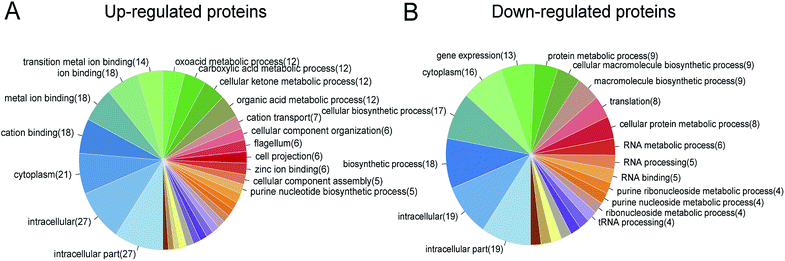 |
| Fig. 4 Pie charts of GO enrichment classification of significantly changed proteins (counts) under daphnetin stress. (A) GO enrichment classification of up-regulated proteins under daphnetin stress. (B) GO enrichment classification of down-regulated proteins under daphnetin stress. | |
Pathway enrichment analysis results showed that the expression levels of most proteins in the metabolic pathways, biosynthesis of secondary metabolites, microbial metabolism in diverse environments and carbon metabolism were up-regulated. And all the expression levels of the five proteins related to flagellar assembly pathway were up-regulated, while all the expression levels of proteins related to epithelial cell signaling in H. pylori infection pathway were down-regulated. Expression levels of four proteins related to ribosome were down-regulated, while those of four proteins related to purine metabolism pathway were up-regulated. Interestingly, five genes related to flagellar assembly pathway were proved to have strong interaction with each other by STRING analysis (Fig. 3), which was also reported in previous study.25 The expression levels of HP_0144 and HP_0145, genes related to cytochrome c oxidase, were down-regulated and have strong interaction with each other. As observed from Fig. 3 genes with important roles in the basic physical activities occupied the hub of interaction net constructed by String analysis. Among the hub genes, the expression levels of tgt, rpoBC, frr, rpsR, rpsT, rpmF, pheT, gyrA, queA, pnp, tuf and rpsU, related to gene function or protein expression, and purA, HP_0221, HP_1182, HP_1269, icd and pgk related to metabolism were all down-regulated. What's more, the expression levels of dnaJ and dnaK (heat shock proteins), combined with recR (recombination protein), and hugZ (heme oxygenase) were up-regulated under daphnetin pressure. As members of epithelial cell signaling in H. pylori infection pathway, interactions between HP_0520 (Cag1) and HP_0543 (Cag22) or hpaA were also observed in the interaction network as previous reported.26,27
4.2 Effects on epithelial cell signaling pathway during H. pylori infection
H. pylori secretes a number of proteinaceous factors which are essential for initial colonization and subsequent persistence in the host's stomach. I0ZHH0, a putative neuraminyllactose-binding hemagglutinin-like protein HpaA, can bind to the surface receptors of gastric epithelial cells and further damage the gastric mucosa.28 Vacuolating cytotoxin A (VacA), one of the major protein toxins, can bind to host cells and causes severe “vacuolation”.29,30 Therefore, the decreased putative vacuolating cytotoxin (VacA)-like protein (I0ZBK7) definitely affects the ability of the bacteria to colonize the stomach and the development of gastric disease. Furthermore, many Gram-negative pathogens have the type IV secretion systems (T4SS) which can translocate bacterial virulence factors into host cells to hijack cellular processes while delivering the CagA oncoprotein into gastric epithelial cells.31 As a member of Cag family, studies have found that CagF (I0ZJ86) might be a protein which is involved in the early steps of CagA recognition and delivery into the T4SS channel.32 In our study, the down-regulation of CagF and Cag1 (I0ZH94) may influence the translocation of virulence factor CagA into host cells. According to the KEGG pathway and string results, it is worth noting that HpaA, type-IV secretion system (T4SS), CagA, VacA together with BabA and UreI found in our previous study11 have vital roles in the epithelial cell signaling pathway during H. pylori infection (Fig. 5). Apart from the vacuolation effect mentioned above, it is reported that VacA can indirectly induce ulcerogenesis in mice. And CagA has been shown to interact with more and more host signaling molecules in phosphorylation-dependent and-independent ways such as MAPK signaling pathway. Our results proved that daphnetin reduced the expression levels of several important proteins in this pathway, suggesting the inhibition of H. pylori colonization and infection, which further explained our previous results that daphnetin exposure remarkably decreased the adherence of H. pylori to GES-1 cells compared with the control condition.11
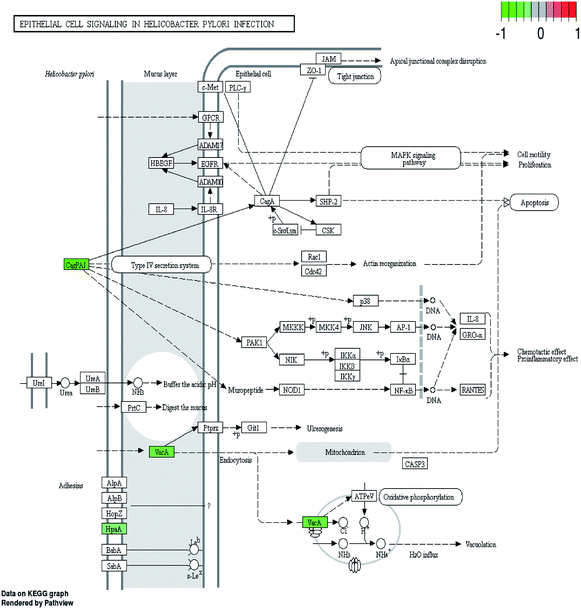 |
| Fig. 5 The epithelial cell signaling pathways in H. pylori infection. Proteins with decreased accumulation under daphnetin pressure are shown in green. | |
4.3 Effects on membrane structure, ion binding, nucleic acid synthesis and metabolism
Among the proteins with significantly different expression levels induced by daphnetin, the large amount of proteins related to membrane represents huge changes in the structure of the cell membrane. There are many proteins in membrane proteins that play important roles for the infection and colonization ability of H. pylori. The outer membrane protein OipA (I0ZBG0) is involved in the induction of IL-8 secretion and is related to the development of peptic ulcers and gastric cancer.33 Reduced OipA expression levels represent changes in the membrane structure and decreased infection ability. Studies have demonstrated a high popularity of strains with oipA, and significant associations have been found between functional oipA and cagA or vacA genotypes.34 The effects of daphnetin on these proteins reveal its potential capability to disrupt the H. pylori infection process. What's more, the expression of a considerable amount of proteins related to ion binding changed under daphnetin pressure. In H. pylori, the ability to acquire ion such as iron is essential for the successful colonization on the gastric niche and the subsequent infection in a mouse model.35 Thus the daphnetin-induced down-regulation of the iron(III) dicitrate transport protein FecA (I0ZG47) makes the colonization and infection process difficult.
Daphnetin influenced the expression level of a large amount of enzymes related to gene duplication, transcription and translation, fundamentally leading to internal synthesis and metabolism disorders. Apart from the down-regulated proteins related to ribosome, studies have demonstrated that the unique fused beta- and beta′-like subunits of DNA-dependent RNA polymerase (I0ZE29, ropBC) in H. pylori conduces to its capability to colonize the human gastric mucosa.36 Therefore, the suppression of rpoBC by daphnetin will affect the colonization of H. pylori. In addition, the decreased expression of DNA gyrase subunit A, gyrA (I0ZIK8), represents a decline in DNA synthesis ability or DNA damage. Translation elongation factor Tu (I0ZE21, EF-Tu) is an important component of the protein translation system.37 Recently, EF-Tu was reported to be a new secretory adherence factor in H. pylori and is related to H. pylori adaptation for host-pathogen interaction during pathogenesis.38 The down-regulated expression of EF-Tu not only affects protein translation but also the interaction with host cells.
The maximum amount of proteins, which changed significantly under daphnetin's pressure, belongs to the metabolism group, covering various metabolic and synthetic pathways. Among them, adenylosuccinate synthetase, AdSS (I0ZBQ4), is an enzyme that regulates purine metabolism of H. pylori. AdSS is a promising drug target in pathogenic organisms that utilize only the purine salvage pathway, the decreased expression level (shown in Fig. 6) under daphnetin pressure will influence the salvage of purine.39
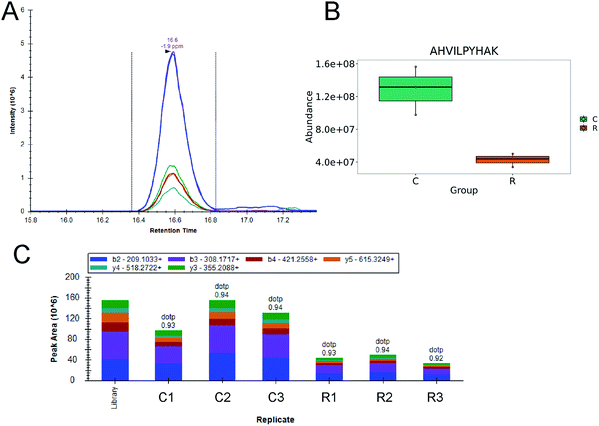 |
| Fig. 6 AHVILPYHAK peptide (I0ZBQ4) quantification results by PRM. One way ANOVA statistical test and q value method was used, and adjusted p < 0.05. (A) Extracted ion chromatogram of PRM fragment ions for AHVILPYHAK; (B) plot map of ion abundance comparison, C, control groups (marked green); R, daphnetin treated groups (marked orange); (C) the corresponding peak area replicates of daphnetin treated and untreated groups. Control groups: C1, C2, C3; daphnetin treated groups: R1, R2, R3. | |
4.4 Upregulated proteins
The ubiquitous molecular chaperones can control the dynamic balance of cell proteins, such as stressful growth conditions. In our study, the expression levels of highly conserved chaperone proteins DnaK (HSP70) and DnaJ (HSP40) were up-regulated under daphnetin pressure. DnaK is supposed to play an important role in promoting proliferation and antiapoptosis. Recent studies have shown that the expression level of HSP70 will affect the proliferation of gastric epithelial cells to a certain extent.40 Faced with the pressure of daphnetin, H. pylori increased expression levels of heat shock proteins to respond to stressors and slowed the proliferation of gastric epithelial cells. Apart from heat shock proteins, the expression levels of recombinational repair protein RecR were up-regulated for mediating recombinational repair of DNA damage caused by daphnetin.41 In addition to stress-responsive proteins, it is surprising that the expression of five proteins related to the flagellar assembly pathway were up-regulated. The phenomenon was also observed in acid treated H. pylori, as the flagellar protein and movement were activated.42
4.5 Speculation on the antibacterial mechanisms of daphnetin against H. pylori
As previous studies have elaborated, daphnetin possesses notable antioxidative, anti-inflammatory and anticoagulant activities in diabetic nephropathy, neurodegenerative disorders, and non-alcoholic fatty liver disease treatment through suppressing the LPS-induced activation of JAK/STAT cascades and ROS production, regulating p38 MAPK and JNK signaling pathway, enhancing the expression of HSP70 via ERK signaling pathway, etc.43–45 But little is known about the mechanisms of daphnetin's action on bacteria. In this study, quantitative proteomics was used to explore the possible mechanism of daphnetin on H. pylori ATCC 43504. And proteins related to metabolism, membrane structure, nucleic acid and protein synthesis, ion binding, H. pylori colonization and infection, stress reaction, flagellar assembly and so on were considered responsible for the drug action, indicating that daphnetin exerted its efficacy through multiple targets. Furthermore, among these proteins, VacA toxin, and AdSS were chosen as promising targets for inhibitor screening,39,46,47 and HpaA, VacA, Cag, gyrA and FecA were administered as vaccine antigens in animal models48–50 in other studies. All the results confirm that daphnetin is a promising antibacterial with specific and extensive antibacterial mechanisms. Although the in vitro antibacterial activity is not outstanding, we are full of hope for its in vivo efficacy, especially its inhibition of host-pathogen colonization and the infection process. With increasing multi-drug resistance rate of H. pylori, drugs with multiple targets can effectively slow the development of drug resistance. Additionally, to effectively inhibit the emergence of multidrug resistance, traditional Chinese medicine extracts with multiple targets, such as daphnetin, are worthy of further research and discovery.
5. Conclusions
The application of label-free quantitative proteomics and PRM provides an alternative method for seeking targets and mechanisms in antibiotic-treated bacteria. From the perspective of proteomics, the protein variation in bacteria becomes more intuitive and abundant. In general, the antibacterial mechanism of daphnetin to H. pylori are related to metabolism, membrane structure, nucleic acid and protein synthesis, ion binding, H. pylori colonization and infection, stress reaction, flagellar assembly and so on. These data suggest that daphnetin has great potential to be an effective anti-H. pylori clinical agent with potential in vivo activity and provide possible targets for anti-H. pylori compounds screening.
Conflicts of interest
The authors declare that they have no competing interests.
Acknowledgements
This study was supported by the CAMS Initiative for Innovative Medicine (grant number 2016-I2M-3-014, 2017-I2M-1-012), the National Mega-project for Innovative Drugs (grant number 2019ZX09721001), the National Natural Science Foundation of China (grant number 81803593, 81621064) and the Fundamental Research Funds for the Central Universities (grant number 3332018094). We are very grateful to Dr Wei Sun from Centralab Institute of Basic Medical Sciences Chinese Academy of Medical Sciences for his wise advice regarding bioinformatics analysis and paper writing. We also appreciate the manuscript editing services provided by American Journal Experts.
References
- J. R. Warren and B. Marshall, Lancet, 1983, 321, 1273–1275 CrossRef.
- B. E. Dunn, H. Cohen and M. J. Blaser, Clin. Microbiol. Rev., 1997, 10, 720–741 CrossRef CAS.
- J. D. de Korwin, G. Ianiro, G. Gibiino and A. Gasbarrini, Helicobacter, 2017, 22, e12411 CrossRef.
- G. J. Finn, B. S. Creaven and D. A. Egan, Biochem. Pharmacol., 2004, 67, 1779–1788 CrossRef CAS.
- WHO Publishes List of Bacteria for Which New antibiotics Are Urgently Needed, 2017, Available online: http://www.who.int/mediacentre/news/releases/2017/bacteria-antibiotics-needed/en/ Search PubMed.
- V. Papastergiou, S. D. Georgopoulos and S. Karatapanis, World J. Gastroenterol., 2014, 20, 9898 CrossRef CAS.
- H. M. Chawla, K. Chakrabarty, S. S. Chibber, A. N. Kalia and N. C. Chaudhury, Indian J. Pharm. Sci., 1980, 42, 138–139 CAS.
- H. Fukuda, S. Nakamura, Y. Chisaki, T. Takada, Y. Toda, H. Murata, K. Itoh, Y. Yano, K. Takata and E. Ashihara, Biochem. Biophys. Res. Commun., 2016, 471, 63–67 CrossRef CAS.
- Q. S. Wenwei Zou, Y. Fu and Z. Xia, Chin. Tradit. Pat. Med., 2013, 35, 39–45 Search PubMed.
- G. J. Finn, E. Kenealy, B. S. Creaven and D. A. Egan, Cancer Lett., 2002, 183, 61–68 CrossRef CAS.
- G. Wang, J. Pang, X. Hu, T. Nie, X. Lu, X. Li, X. Wang, Y. Lu, X. Yang, J. Jiang, C. Li, Y. Q. Xiong and X. You, Int. J. Mol. Sci., 2019, 20, 850 CrossRef CAS.
- P. R. Jungblut, F. Schiele, U. Zimny-Arndt, R. Ackermann, M. Schmid, S. Lange, R. Stein and K. P. Pleissner, Proteomics, 2010, 10, 182–193 CrossRef CAS.
- S. C. Baik, K. M. Kim, S. M. Song, D. S. Kim, J. S. Jun, S. G. Lee, J. Y. Song, J. U. Park, H. L. Kang and W. K. Lee, J. Bacteriol., 2004, 186, 949–955 CrossRef CAS.
- L. Xiaofen, H. Yingwei, P. Pei-Jing, C. Daijie and L. Henry, J. Proteome Res., 2013, 13, 1223–1233 Search PubMed.
- J. Woo, D. Han, J. I. Wang, J. Park, H. Kim and Y. Kim, J. Proteome Res., 2017, 16, 3419–3432 CrossRef CAS.
- Y. Sung-Ho, C. Chi-Won, K. Sang-Oh, P. Gun Wook, C. Kun, K. Kyung-Hoon, K. J. Young, Y. Jong Shin, L. Je Chul and C. Jong-Soon, J. Proteome Res., 2011, 10, 459–469 CrossRef.
- J. M. Whitmire and D. S. Merrell, Methods Mol. Biol., 2012, 921, 17–27 CrossRef CAS.
- B. Leng, J. Zeng, Z. Xiong, W. Lv and Y. Wan, J. Proteome Res., 2006, 5, 2547–2553 CrossRef.
- R. Karlsson, K. Thorell, S. Hosseini, D. Kenny, C. Sihlbom, Å. Sjöling, A. Karlsson and I. Nookaew, Front. Microbiol., 2016, 7, 1757 Search PubMed.
- G. Espadas, E. Borràs, C. Chiva and E. Sabidó, Proteomics, 2017, 17, 201600416 CrossRef.
- A. C. Peterson, J. D. Russell, D. J. Bailey, M. S. Westphall and J. J. Coon, Mol. Cell. Proteomics, 2012, 11, 1475 CrossRef.
- B. MacLean, D. M. Tomazela, N. Shulman, M. Chambers, G. L. Finney, B. Frewen, R. Kern, D. L. Tabb, D. C. Liebler and M. J. MacCoss, Bioinformatics, 2010, 26, 966–968 CrossRef CAS.
- I. Vranakis, I. Goniotakis, A. Psaroulaki, V. Sandalakis, Y. Tselentis, K. Gevaert and G. Tsiotis, J. Proteomics, 2014, 97, 88–99 CrossRef CAS.
- B. Cooper, N. Islam, Y. Xu, H. S. Beard, W. M. Garrett, G. Gu and X. Nou, Proteomics, 2018, 18, e1700461 CrossRef.
- M. C. Lane, P. W. O'Toole and S. A. Moore, J. Biol. Chem., 2006, 281, 508–517 CrossRef CAS.
- D. M. Pinto-Santini and N. R. Salama, J. Bacteriol., 2009, 191, 7343–7352 CrossRef CAS.
- R. Hauser, A. Ceol, S. V. Rajagopala, R. Mosca, G. Siszler, N. Wermke, P. Sikorski, F. Schwarz, M. Schick, S. Wuchty, P. Aloy and P. Uetz, Mol. Cell. Proteomics, 2014, 13, 1318–1329 CrossRef.
- C. Elisabet, N. M. Johanna, B. L. Ingrid, C. L. Nilsson and S. Ann-Mari, Infect. Immun., 2006, 74, 920–926 CrossRef.
- S. L. Palframan, T. Kwok and K. Gabriel, Front. Cell. Infect. Microbiol., 2012, 2, 92 Search PubMed.
- A. Link, C. Langner, W. Schirrmeister, W. Habendorf and P. Malfertheiner, World J. Gastroenterol., 2017, 23, 4712 CrossRef CAS.
- S. Backert, N. Tegtmeyer and W. Fischer, Future Microbiol., 2015, 10, 955–965 CrossRef CAS.
- C. Marc Roger, T. Elizabetta, M. Cesare and S. Markus, Infect. Immun., 2006, 74, 273 CrossRef.
- Y. Yamaoka, D. H. Kwon and D. Y. Graham, Proc. Natl. Acad. Sci. U. S. A., 2000, 97, 7533–7538 CrossRef CAS.
- T. Ando, R. M. Peek, D. Pride, S. M. Levine, T. Takata, Y. C. Lee, K. Kusugami, A. van der Ende, E. J. Kuipers, J. G. Kusters and M. J. Blaser, J. Clin. Microbiol., 2002, 40, 239–246 CrossRef CAS.
- A. Danielli, S. Romagnoli, D. Roncarati, L. Costantino, I. Delany and V. Scarlato, J. Bacteriol., 2009, 191, 3717–3725 CrossRef CAS.
- N. Zakharova, P. S. Hoffman, D. E. Berg and K. Severinov, J. Biol. Chem., 1998, 273, 19371–19374 CrossRef CAS.
- D. Wang, B. Luo, W. Shan, M. Hao, X. Sun and R. Ge, Metallomics, 2013, 5, 888–895 RSC.
- K. H. Chiu, L.-H. Wang, T.-T. Tsai, H.-Y. Lei and P.-C. Liao, J. Proteome Res., 2016, 16(1), 264–273 CrossRef.
- A. Bubic, N. Mrnjavac, I. Stuparevic, M. Lyczek, B. Wielgus-Kutrowska, A. Bzowska, M. Luic and I. Lescic Asler, J. Enzyme Inhib. Med. Chem., 2018, 33, 1405–1414 CrossRef CAS.
- L. Tao, H. Zou and Z. Huang, Gastroent. Res. Pract., 2014, 2014, 794342 Search PubMed.
- G. Wang, L. F. Lo and R. J. Maier, DNA Repair, 2011, 10, 373–379 CrossRef CAS.
- S. Ansari and Y. Yamaoka, Helicobacter, 2017, 22 DOI:10.1111/hel.12386.
- Z. Qi, S. Qi, L. Gui, L. Shen and Z. Feng, Oncol. Lett., 2016, 12, 1959–1964 CrossRef CAS.
- K. Xu, L. Guo, H. Bu and H. Wang, J. Pharmacol. Sci., 2019, 139, 91–97 CrossRef CAS.
- Y. Liu, L. Liao, Y. Chen and F. Han, Mol. Med. Rep., 2019, 19, 4673–4684 CAS.
- M. Junaid, A. K. Linn, M. B. Javadi, S. Al-Gubare, N. Ali and G. Katzenmeier, Toxicon, 2016, 118, 27–35 CrossRef CAS.
- A. Rehman, S. Akhtar, M. H. Siddiqui, U. Sayeed, S. S. Ahmad, J. M. Arif and M. K. Khan, Bioinformation, 2016, 12, 400–407 CrossRef.
- J. Nystrom and A. M. Svennerholm, Vaccine, 2007, 25, 2591–2598 CrossRef.
- F. Hongying, W. Xianbo, Y. Fang, B. Yang and L. Beiguo, Clin. Vaccine Immunol., 2014, 21, 126–132 CrossRef.
- M. Rizwan, A. Naz, J. Ahmad, K. Naz, A. Obaid, T. Parveen, M. Ahsan and A. Ali, BMC Bioinf., 2017, 18, 106 CrossRef.
Footnote |
† Electronic supplementary information (ESI) available. See DOI: 10.1039/d0ra06677j |
|
This journal is © The Royal Society of Chemistry 2021 |
Click here to see how this site uses Cookies. View our privacy policy here.