DOI:
10.1039/D0RA09320C
(Paper)
RSC Adv., 2021,
11, 2158-2166
Pharmacophore modeling and virtual screening studies for discovery of novel farnesoid X receptor (FXR) agonists
Received
2nd November 2020
, Accepted 27th December 2020
First published on 7th January 2021
Abstract
Farnesoid X receptor (FXR) agonists would be considered as an important therapeutic strategy for several chronic liver and metabolic diseases. Here we have employed an integrated virtual screening by combining ligand-based pharmacophore mapping and molecular docking to identify novel nonsteroidal FXR agonists. Eighteen compounds were selected for in vitro FXR agonistic activity assay, and results showed five compounds exhibiting promising FXR agonistic activity. Among these compounds, compounds F4 and F17 were the most remarkable in vitro activity by using homogeneous time resolved fluorescence (HTRF) assay and the full-length FXR reporter gene assay in HepG2 cells. Real-time PCR assay was performed to measure the expression of FXR target genes. Compounds F4 and F17 increased small heterodimer partner (SHP), in turn, suppress mRNA levels of cholesterol 7-alpha-hydroxylase (CYP7A1). The obtained compounds F4 and F17 from this study may be potential leads for developing novel FXR agonists in the treatment of metabolic diseases.
1. Introduction
Farnesoid X receptor (FXR, NR1H4), also known as bile acid receptor, is a human nuclear receptor that is expressed in a variety of tissues including liver, intestine, kidney and adrenal gland.1–3 FXR is the master controller for bile acids homeostasis through its regulation of bile acid synthesis, metabolism, secretion and absorption. Moreover, FXR also plays a role in glucose and lipid metabolism.4,5 Therefore, modulating FXR may be beneficial for treating of several chronic liver and metabolic diseases, such as dyslipidemia, hypertriglyceridemia, type 2 diabetes, obesity and NASH.6,7
Medicinal chemistry approaches have discovered a variety of FXR agonists.8–14 The FXR agonists can be divided into two different classes (steroidal and nonsteroidal FXR agonists) based on their structure (Fig. 1). As a endogenous ligand of FXR, chenodeoxycholic acid (CDCA, EC50 = 8 μM) is a hydrophobic primary bile acid that activates FXR involved in cholesterol metabolism.15 Highly potent steroidal FXR agonists obeticholic acid (OCA) has been derived from the CDCA.16 The most important and widely used nonsteroidal FXR agonist is GW4064, was developed by Glaxo-SmithKline (GSK), which served as a reference compound in many experiments.10,17 In addition, several other synthetic nonsteroidal FXR agonists fexaramine and turofexorate isopropyl (XL335 or WAY-362450) have been reported.18,19 However, many steroidal FXR agonists with poor selectivity and low affinity; the nonsteroidal FXR ligands have limitations owing to side effects and uncertain bioavailability.20,21 Therefore, there is still enormous need for developing novel classes of FXR agonists with potent activity and low side effects.
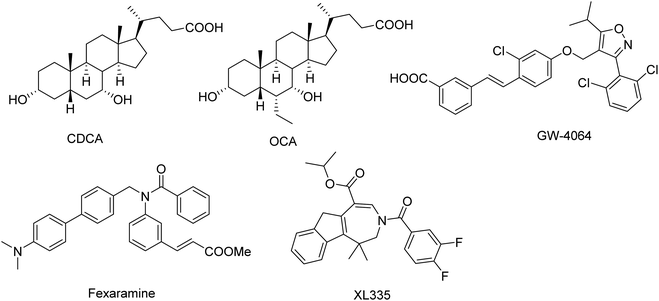 |
| Fig. 1 Chemical structures of important FXR agonists. | |
The aim of this study is to identify novel nonsteroidal FXR agonists through a computer-aided drug discovery approach. In this study, we constructed a ligand-based pharmacophore model based on common features of FXR agonists. Then the selected pharmacophore hypotheses were used to screen the FDA approved database, specs, targetMol and in our library databases. Further screening of the retrieved compounds was performed using molecular docking. Finally, 19 commercially available hit compounds were selected by our virtual screening approach, and evaluated for their in vitro FXR agonistic activity assay. This study reports novel and nonsteroidal starting points that can be further developed as more potent nonsteroidal FXR agonists.
2. Materials and methods
2.1. Reagents
18 commercially available hit compounds were purchased from Target Molecule Corp. for in vitro bioassay test. Biotinylated SRC1 peptide was synthesized by GL Biochem (Shanghai) Ltd. (China). Human FXR ligand binding domain (GST-FXRαLBD) was expressed and purified by Shanghai Shengong Biotechnology (Shanghai, China).
2.2. Ligand-based pharmacophore modeling
The ligand-based pharmacophore was generated using the ‘common feature pharmacophore generation’ protocol implemented in the Discovery Studio 3.0 program package. The structures in the training set were collected from published literature and the corresponding 3D structures were extracted from the X-ray protein structure to maintain the active conformation. The training set compounds with their active conformations were submitted to the common feature pharmacophore generation protocol HipHop module. Maximum pharmacophore hypotheses was set to 10. The minimum feature option was 4, and maximum feature option was 6.
2.3. Pharmacophore validation
The ligand-based pharmacophore modeling were validated using the ‘Ligand Profiler’ in Discovery Studio to mapping the selected molecules onto the best models. In order to perform the validation approach, a test set compounds consisting of five reported FXR agonists and four inactive compounds were mapped onto the pharmacophore model.
2.4. Molecular docking
Docking simulations of the ligands in the FXR active site were carried out using Glide docking in the Schrodinger suite. The grid file were generated to the receptor by the position of the co-crystallized ligand. The grid size were 10 Å × 10 Å × 10 Å of inner box and 20 Å × 20 Å × 20 Å of outer box. The OPLS_2005 force field was used for grid generation. To accomplish the molecular docking, the extra precision (Glide XP) protocol was selected with ligands docked flexibly.
2.5. Full-length FXR transactivation assay
HepG2 cells were cultured in DMEM high glucose, supplemented with 10% fetal calf serum (FBS), 1 mM sodium pyruvate (SP), penicillin (100 U mL−1), and streptomycin (100 μg mL−1) at 37 °C and 5% CO2. HepG2 cells were plated in a 12-well plate at 5 × 104 cells per well. HepG2 cells were transfected with BSEP-pGL3, pRL-SV40, and the expression plasmids pcDNA3-hFXR and pSG5-hRXR. Then 24 h after transfection, medium was changed to DMEM, now additionally containing 0.1% DMSO and the respective test compound or 0.1% DMSO alone as untreated control. Each concentration was tested in triplicate wells, and each experiment was repeated independently at least three times. After treatments, cells were assayed for luciferase activity using Dual-Glo luciferase assay system (Promega) according to the manufacturer's protocol.
2.6. FXR target gene quantification (qRT-PCR)
HepG2 cells were cultured in DMEM high glucose, supplemented with 10% fetal calf serum (FBS), 1 mM sodium pyruvate (SP), penicillin (100 U mL−1), and streptomycin (100 μg mL−1) at 37 °C and 5% CO2. Cells were primed 18 h with CDCA 10 μM, compounds F4 and F17 (2 μM). Total RNA was extracted from HepG2 cells by TRIzol Reagent (Invitrogen, Thermo Fisher Scientific, Carlsbad, CA), following the manufacturer's instruction. The amount of the purified RNAs and their quality were assessed by NanoDrop 2000 UV-Vis Spectrophotometer (Thermo Fisher Scientific, Wilmington, DE).
Total RNA (2 μg) was reverse-transcribed into cDNA using the High Capacity cDNA Reverse Transcription kit (Applied Biosystems, Foster City, CA) according to the manufacturer's protocol. FXR target gene expression was evaluated by quantitative real-time PCR analysis (RT-qPCR). Real-time PCR was performed by the 7500HT thermal cycler and SYBR Green master mix (Applied Biosystems). The primers pairs are listed in Table 1. All samples were analyzed in duplicate, measuring both the gene of interest and β-actin as an internal control. The relative mRNA expression was quantified by the 2−ΔΔCt method.
Table 1 The sequences of all the primers in quantitative RT-PCR analysis
Genes |
Forward primer |
Reverse primer |
SHP |
CCCCAAGGAATATGCCTGCC |
TAGGGCGAAAGAAGAGGTCCC |
CYP7A1 |
GAGAAGGCAAACGGGTGAAC |
GCACAACACCTTATGGTATGACA |
β-Actin |
TTGTTACAGGAAGTCCCTTGCC |
ATGCTATCACCTCCCCTGTGTG |
2.7. Time resolved fluorescence (HTRF) assay
Biotinylated SRC1 peptide (5′-biotin-CPSSHSSLTERHKILHRLLQEGSPS-CONH2) was synthesized by GL Biochem (Shanghai) Ltd. (China). Human FXR ligand binding domain (GST-FXRαLBD) was expressed and purified by Shanghai Shengong Biotechnology (Shanghai, China). The assay mixture contained 10 nM GST-FXRαLBD, 100 nM biotin-SRC1, 0.83 nM Eu-labeled anti-GST, and 41.75 nM streptavidin-XL665 (Cisbio, USA) in HTRF buffer. The HTRF buffer was composed of 50 mM Hepes pH 7.0, 125 mM KF, 0.125% CHAPS, and 0.05% dry milk. The white 384-well microplates were incubated at room temperature and then read with a CLARIOstar Microplate Reader (BMG LABTECH) and calculated using the equation (665 nm/620 nm) × 10
000.
3. Results and discussion
3.1. Ligand-based pharmacophore modeling
The structures of FXR agonists were collected from published literature.18–21 The agonists were carefully gathered in such a way to make sure that they were bioassayed under similar conditions. Six representative FXR agonists were chosen to form a training set (Fig. 2); these compounds were selected since they were extracted from the X-ray protein structure to maintain the active conformation and each one of them possesses a different scaffold. The training set was taken as the input ligands to create pharmacophore hypotheses by using the ‘Common Feature Pharmacophore Generation’ module in Discovery Studio program package.
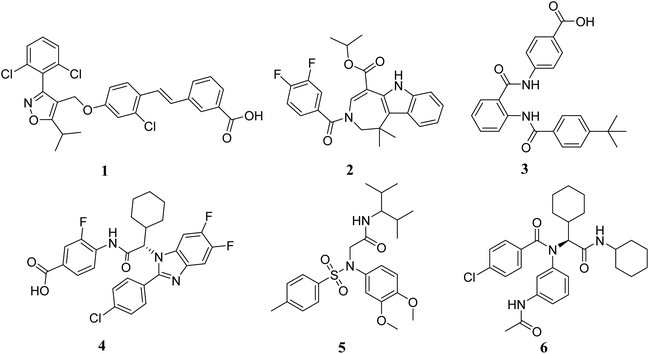 |
| Fig. 2 The training set compounds. | |
Qualitative HipHop models were built to identify the critical common essential chemical features. The most-active compounds (1 and 2) were considered with “principal” value of 2 and a “MaxOmitFeat” value of 0, the “Principal” and “MaxOmitFeat” values were set to 1 for the remaining four compounds (Table 2).
Table 2 In vitro FXR agonists activities of the selected compounds (EC50, nM)
The top-ten pharmacophore hypotheses were generated using this training set with scores ranging from 46.841 to 65.481 (Table 3). These hypotheses could be classified into three groups according to the pharmacophore features: RHHHA (01, 02), HHHA (05, 07) and RHHA (03, 04, 06, 08, 09, 10). Finally, hypothesis model 01 was selected due to its pharmacophore properties and structural requirements. As shown in Fig. 3, hypothesis model 01 contained five point pharmacophore containing one ring aromatic (R), three hydrophobic groups (H) and one H-bond acceptor (A), which were featured as the orange, cyan and green, respectively. The training set compounds can be appropriately matched to the pharmacophore model 01.
Table 3 Summary of the pharmacophore models for FXR agonists
Hypothesis |
Featuresa |
Rankb |
Direct hitc |
Partial hitd |
Max fit |
H, hydrophobic group; A, hydrogen bond acceptor. The ranking score of training set compounds fitting the hypothesis. Direct hit indicates whether (“1”) or not (“0”) a molecule in the training set mapped every feature in the hypothesis. Partial hit indicates whether (“1”) or not (“0”) a particular molecule in the training set mapped all but one feature in the hypothesis. Numeration of molecules is from right to left in both direct hit and partial hit. |
01 |
RHHHA |
65.481 |
111111 |
000000 |
5 |
02 |
RHHHA |
65.006 |
111111 |
000000 |
5 |
03 |
RHHA |
50.082 |
111111 |
000000 |
4 |
04 |
RHHA |
50.005 |
111111 |
000000 |
4 |
05 |
HHHA |
49.090 |
111111 |
000000 |
4 |
06 |
RHHA |
48.660 |
111111 |
000000 |
4 |
07 |
HHHA |
47.951 |
111111 |
000000 |
4 |
08 |
RHHA |
47.925 |
111111 |
000000 |
4 |
09 |
RHHA |
47.697 |
111111 |
000000 |
4 |
10 |
RHHA |
46.841 |
111111 |
000000 |
4 |
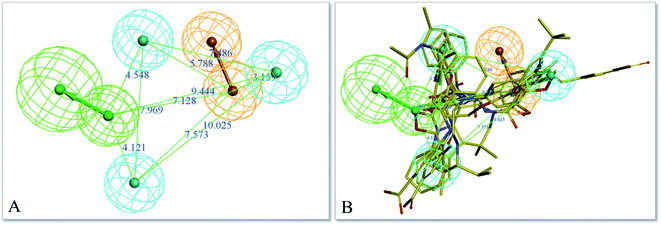 |
| Fig. 3 (A) Selected common-feature pharmacophore for FXR agonists consisting of one ring aromatic (R), three hydrophobic groups (H) and one H-bond acceptor (A), which were featured as the orange, cyan and green, respectively. (B) The training set compounds can be appropriately matched to the pharmacophore model 01. | |
3.2. Pharmacophore validation
Further validation of the final pharmacophore models were checked for their accuracy and relative reliability of performance by evaluating their abilities to selectively identify active and inactive compounds.18,26–28 For this purpose, a test set consisting of four reported FXR agonists and four inactive compounds were mapped onto the pharmacophore model 01. The results of the model mapping onto compounds and fit values are shown in Table 4. Analysis of these fit values showed that the hypothesis 01 can distinguish the active inhibitors from the inactive ones. Thus, it can be indicating that the hypothesis 01 is a reliable model which may be valuable in identifying diverse active FXR agonists from the database.
Table 4 Mapping of the test set compounds onto hypothesis 10
Test compd |
3D mapping onto hypo 1 |
Fit value |
IC50 |
T1 |
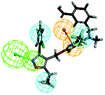 |
4.785 |
2 |
T2 |
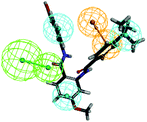 |
3.265 |
8 |
T3 |
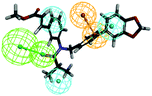 |
3.598 |
19 |
T4 |
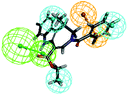 |
3.652 |
32 |
T5 |
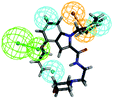 |
2.231 |
— |
T6 |
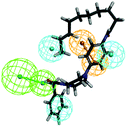 |
1.873 |
— |
T7 |
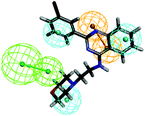 |
1.037 |
— |
T8 |
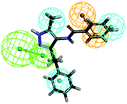 |
2.458 |
— |
3.3. Molecular docking
In order to evaluate the ligand interaction into FXR receptor binding pocket, the molecular docking was carried out using the Schrödinger suite. A published crystal structure of CDCA bound within the active site cavity of FXR receptor (PDB ID: 3DCT) with the resolution of 2.73 Å served as a useful template for the docking. The FXR receptor was prepared by the “protein preparation wizard” module in Maestro. The water molecules were removed and the hydrogens were added in the protein, energy minimization was done using OPLS force field. The grid file were generated to the receptor by the position of the co-crystallized ligand CDCA. The minimum energy conformation of the ligands was performed using the “LigPrep” module in maestro. Finally, the “ligand docking” was carried out using the maestro based on the grid using standard precision (SP) and extra precision (XP) docking algorithm.
3.4. Virtual screening
In order to identify novel FXR agonists, a multi-step virtual screening workflow including ligand-based pharmacophore screening, docking screening and finally a careful visual inspection of the molecules was performed. The workflow of the virtual screening was shown in Fig. 4. First, the validated ligand-based pharmacophore model Hypo01 was used to screen in the 3D multiple conformations FDA approved database, specs, targetMol and in our library databases for a total of 323
842 compounds. Based on the fit values (≥2) for a compound matching against pharmacophore, resulting in 500 screening hits kept in the chemical library. Second, the retrieved compounds from the pharmacophore search were saved as SD file and docked into the prepared receptor grid using standard precision (SP) and extra precision (XP) docking algorithm. The Glide XP scoring function was used to predict binding energy between compounds and FXR. According to the predicted binding energy (≤−6), top 50 compounds were picked out. After careful visual inspection, 18 commercially available hit compounds (Fig. 5) were selected and purchased from Target Molecule Corp. for in vitro bioassay test.
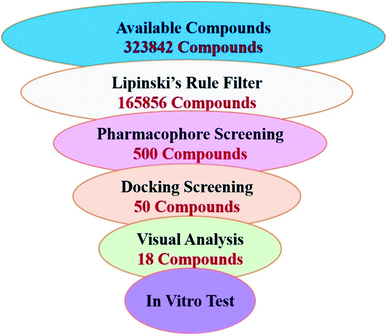 |
| Fig. 4 Workflow of the virtual screening protocol. | |
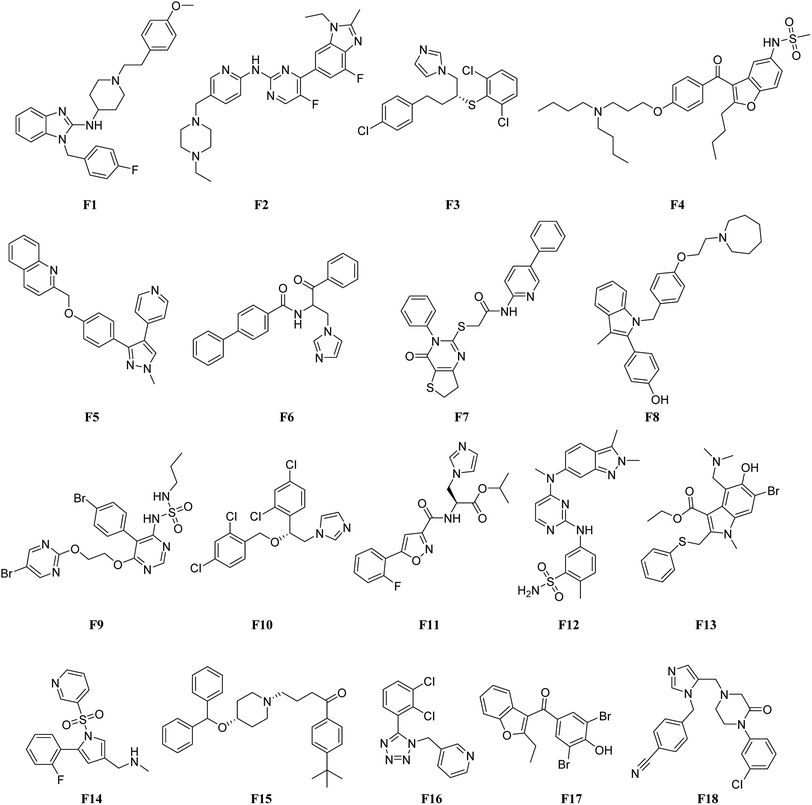 |
| Fig. 5 Structures of 18 hits selected by virtual screening. | |
3.5. In vitro biological evaluation
3.5.1. Time resolved fluorescence (HTRF) assay. The FXR activity of the selected compounds were evaluated by using homogeneous time resolved fluorescence (HTRF) assay. As reference compound, the response of CDCA at 50 μM was defined as 100% FXR activation. Vehicle control with 0.1% DMSO was set to 0% FXR activation. The in vitro HTRF assay activities are summarized in Fig. 6. The most selected compounds have no FXR agonistic activities (expressed as agonistic rate at 40 μM < 10%). Encouragingly, five compounds (F3, F4, F6, F9 and F17) displayed FXR agonistic activities. The most potent compounds F4 and F17 were further evaluated using a dose–response experiment. Compounds F4 and F17 displayed obvious FXR agonist activity, with the EC50 values of 26.4 μM and 15.6 μM with a relative maximum activation (rel. max. act.) of 50.76% and 53.38%, respectively. This results indicating that compounds F4 and F17 were defined as FXR agonists with moderate potency.
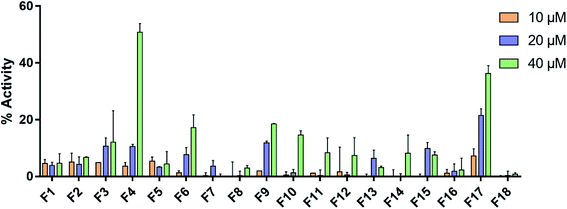 |
| Fig. 6 FXR agonistic activities of the selected compounds by HTRF assay. The response to 50 μM CDCA was set to 100%. | |
3.5.2. Full-length FXR transactivation assay. The most potent compounds F4 and F17 were further evaluated in a full-length FXR reporter gene assay in HepG2 cells. As shown in Figure7, compounds F4 and F17 exhibited significant FXR agonistic activity and could concentration-dependently modulate the activation.
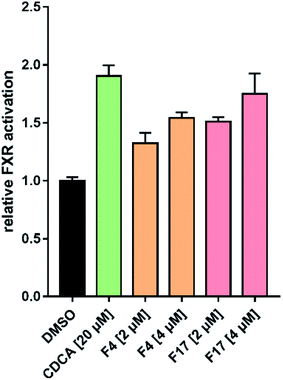 |
| Fig. 7 FXR transactivation assay in HepG2 cells. | |
3.5.3. FXR target gene quantification (qRT-PCR). To further investigate the FXR agonist activity of compounds F4 and F17, we determined theirs effect on FXR target genes expression on mRNA level in the HepG2 cells by quantitative PCR experiments. As shown in Fig. 8, Compounds F4 and F17 increased the expression of SHP and repressed mRNA levels of cholesterol 7-alpha-hydroxylase (CYP7A1). These results suggest that compounds F4 and F17 modulated FXR target genes similar to the endogenous agonist CDCA.
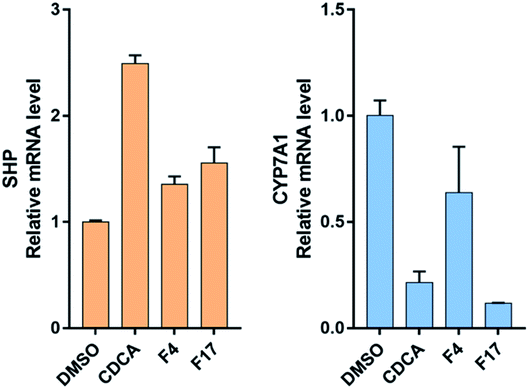 |
| Fig. 8 FXR target gene mRNA (SHP and CYP7A1) quantification in HepG2 cells with compounds F4 and F17 at 2 μM, CDCA at 50 μM. | |
3.6. In vitro cytotoxicity assay
The most potent compounds F4 and F17 were further evaluated for their in vitro cytotoxicity against A549 and HeLa cells. Cytotoxicity was measured using the CCK8 assay after incubation with the compounds for 24 h. As shown in Table 5, compound F4 showed moderate toxicity against HeLa cell with IC50 values of 25.24 μM. Compound F17 showed no activities toward tumor cell lines A549 and HeLa with IC50 > 50 μM.
Table 5 In vitro cytotoxicity of compounds on A549 and HeLa cells
Compd |
IC50a (μM) |
A549 |
HeLa |
The mean values of three independent experiments ± SE are reported. |
F4 |
>50 |
25.24 ± 0.23 |
F17 |
>50 |
>50 |
3.7. Molecular docking analysis
To investigate the binding mode of compounds F4 and F17, two highly active compounds, they were docked into the active site of FXR by using the CDOCKER program in the Discovery Studio 3.0 software. A published crystal structure of GW-4064 bound within the active site cavity of FXR receptor (PDB ID: 3DCT) with the resolution of 2.73 Å served as a useful template for generating binding modes. As shown in Fig. 9, the benzofuran ring of compounds F4 and F17 form hydrogen bonds with His447. Other atoms of compounds F4 and F17 contribute to interactions only by shape complementary and hydrophobic interactions with the surrounding residues Met328, Ile357, Ala291, Val295 and Leu287. In addition, hydrogen bonds formed between the tertiary amine of compound F4 and Arg331.
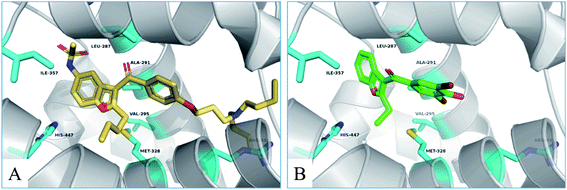 |
| Fig. 9 The binding mode of compounds F4 and F17 in the active site of FXR (PDB ID: 3DCT). | |
4. Conclusions
In this study, we described a rational ligand-based pharmacophore modeling, integrated with virtual screening and molecular docking, for the discovery of novel FXR agonists. Eighteen compounds were then selected for in vitro HTRF assay, and results showed five compounds exhibiting promising FXR agonistic activity. Among these compounds, compounds F4 and F17 were the most remarkable in vitro activity by using HTRF assay and the full-length FXR reporter gene assay in HepG2 cells. Real-time PCR assay was performed to measure the expressions of FXR target genes. Compounds F4 and F17 increased small heterodimer partner (SHP), in turn, suppress mRNA levels of cholesterol 7-alpha-hydroxylase (CYP7A1).
In addition, compounds F4 and F17, possessing a novel benzofuran and benzoyl scaffolds, were valuable for further optimization and provides a reference for the development of novel potent FXR agonists. Further structural optimization and structure–activity relationship studies (SARs) are still under investigation in our laboratory.
Conflicts of interest
There are no conflicts to declare.
Acknowledgements
This work is supported by the National Natural Science Foundation of China (Grant No. 81970726 and No. 81472232), Henan Provincial Natural Science Foundation (Grant No. 182300410323), First Class Discipline Cultivation Project of Henan University (Grant No. 2019YLZDYJ19), Plan for Scientific Innovation Talent of Henan Province (Grant No. 154100510004) and Key Program for Science & Technology from Henan Education Department (Grant No. 21A310001) to W.-D. C., the National Natural Science Foundation of China (Grant No. 81903444), China Postdoctoral Science Foundation (Grant No. 2018M640674), Key Science and Technology Program of Henan Province (Grant No. 192102310145) and Henan Postdoctoral Foundation (Grant No. 201902027) to S. Z., Major Scientific and Technological Innovation Project of Hebi to R. H., the National Natural Science Foundation of China (Grant No. 81970551 and No. 81672433) and the Fundamental Research Funds for the Central Universities and Research Projects on Biomedical Transformation of China-Japan Friendship Hospital (Grant No. PYBZ1803) to Y.-D. W.
References
- J. Y. Chiang, J. Lipid Res., 2009, 50, 1955 CrossRef CAS.
- H. Emina, C. Thierry and T. Michael, J. Hepatol., 2013, 58, 155–168 CrossRef.
- D. J. Parks, S. G. Blanchard, R. K. Bledsoe, G. Chandra, T. G. Consler, S. A. Kliewer, J. B. Stimmel, T. M. Willson, A. M. Zavacki and D. D. Moore, Science, 1999, 284, 1365–1368 CrossRef CAS.
- S. Modica, R. M. Gadaleta and A. Moschetta, Nucl. Recept. Signaling, 2010, 8, e005 CAS.
- Y. D. Wang, W. D. Chen, D. D. Moore and W. Huang, Cell Res., 2008, 18, 1087–1095 CrossRef CAS.
- G. Musso, M. Cassader and R. Gambino, Nat. Rev. Drug Discovery, 2016, 15, 249 CrossRef CAS.
- A. Roda, R. Pellicciari, A. Gioiello, F. Neri, C. Camborata, D. Passeri, F. F. De, S. Spinozzi, C. Colliva and L. Adorini, J. Pharmacol. Exp. Ther., 2014, 350, 56 CrossRef.
- D. Flesch, S. Y. Cheung, J. Schmidt, M. Gabler, P. Heitel, J. Kramer, A. Kaiser, M. Hartmann, M. Lindner, K. Lüddens-Dämgen, J. Heering, C. Lamers, H. Lüddens, M. Wurglics and E. Proschak, J. Med. Chem., 2017, 60, 7199–7205 CrossRef CAS.
- D. C. Tully, P. V. Rucker, D. Chianelli, J. Williams, A. Vidal and P. B. Alper, J. Med. Chem., 2017, 60, 9960–9973 CrossRef CAS.
- P. R. Maloney, D. J. Parks, C. D. Haffner, A. M. Fivush, G. Chandra, K. D. Plunket, K. L. Creech, L. B. Moore, J. G. Wilson, M. C. Lewis, S. A. Jones and T. M. Willson, J. Med. Chem., 2000, 43, 2971–2974 CrossRef CAS.
- S. M. W. van de Wiel, I. T. G. W. Bijsmans, S. W. C. van Mil and S. F. J. van de Graaf, Sci. Rep., 2019, 9, 2193 CrossRef.
- K. Fujimori, Y. Iguchi, Y. Yamashita, K. Gohda and N. Teno, Molecules, 2019, 24, 17 Search PubMed.
- V. Massafra, R. Pellicciari, A. Gioiello and S. W. C. van Mil, Pharmacol. Ther., 2018, 191, 162–177 CrossRef CAS.
- A. H. Ali, E. J. Carey and K. D. Lindor, Ann. Transl. Med., 2015, 3, 16 Search PubMed.
- R. Pellicciari, D. Passeri, F. De Franco, S. Mostarda, P. Filipponi, C. Colliva, R. M. Gadaleta, P. Franco, A. Carotti, A. Macchiarulo, A. Roda, A. Moschetta and A. Gioiello, J. Med. Chem., 2016, 59, 9201–9214 CrossRef CAS.
- R. Pellicciari, S. Fiorucci, E. Camaioni, C. Clerici, G. Costantino, P. R. Maloney, A. Morelli, D. J. Parks and T. M. Willson, J. Med. Chem., 2002, 45, 3569 CrossRef CAS.
- Y. Ma, Y. Huang, L. Yan, M. Gao and D. Liu, Pharm. Res., 2013, 30, 1447–1457 CrossRef CAS.
- J. Fu, P. Si, M. Zheng, L. Chen, X. Shen, Y. Tang and W. Li, Bioorg. Med. Chem. Lett., 2012, 22, 6848–6853 CrossRef CAS.
- S. Zhang, J. Wang, Q. Liu and D. C. Harnish, J. Hepatol., 2009, 51, 380–388 CrossRef CAS.
- D. L. Howarth, S. H. Law, J. M. Law, J. A. Mondon, S. W. Kullman and D. E. Hinton, Toxicol. Appl. Pharmacol., 2010, 243, 111–121 CrossRef CAS.
- C. Gege, E. Hambruch, N. Hambruch, O. Kinzel and C. Kremoser, Handb. Exp. Pharmacol., 2019, 256, 167–205 CAS.
- A. Akwabi-Ameyaw, J. Y. Bass, R. D. Caldwell, J. A. Caravella, L. Chen, K. L. Creech, D. N. Deaton, S. A. Jones, I. Kaldor, Y. Liu, K. P. Madauss, H. B. Marr, R. B. McFadyen, A. B. Miller, F. Navas III, D. J. Parks, P. K. Spearing, D. Todd, S. P. Williams and G. B. Wisely, Bioorg. Med. Chem. Lett., 2008, 18, 4339–4343 CrossRef CAS.
- F. Brenton, M. Richard, W. Tie-Lin, M. Paige, M. Brett, G. Xiao-Hui, F. Paul, L. Jiali, P. Parinaz and P. Mary, J. Med. Chem., 2009, 52, 904–907 CrossRef.
- H. G. F. Richter, G. M. Benson, K. H. Bleicher, D. Blum, E. Chaput, N. Clemann, S. Feng, C. Gardes, U. Grether, P. Hartman, B. Kuhn, R. E. Martin, J. M. Plancher, M. G. Rudolph, F. Schuler and S. Taylor, Bioorg. Med. Chem. Lett., 2011, 21, 1134–1140 CrossRef CAS.
- Z. Gaieb, S. Liu, S. Gathiaka, M. Chiu, H. W. Yang, C. H. Shao, V. A. Feher, W. P. Walters, B. Kuhn, M. G. Rudolph, S. K. Burley, M. K. Gilson and R. E. Amaro, J. Comput.-Aided Mol. Des., 2018, 32, 1–20 CrossRef CAS.
- U. Abel, T. Schlüter, A. Schulz, E. Hambruch, C. Steeneck, M. Hornberger, T. Hoffmann, S. Perović-Ottstadt, O. Kinzel, M. Burnet, U. Deuschle and C. Kremoser, Bioorg. Med. Chem. Lett., 2010, 20, 4911–4917 CrossRef CAS.
- D. Merk, C. Lamers, K. Ahmad, R. Carrasco Gomez, G. Schneider, D. Steinhilber and M. Schubert-Zsilavecz, J. Med. Chem., 2014, 57, 8035–8055 CrossRef CAS.
- K. C. Nicolaou, R. M. Evans, A. J. Roecker, R. Hughes, M. Downes and J. A. Pfefferkorn, Org. Biomol. Chem., 2003, 1, 908–920 RSC.
|
This journal is © The Royal Society of Chemistry 2021 |
Click here to see how this site uses Cookies. View our privacy policy here.