DOI:
10.1039/D0RA10459K
(Paper)
RSC Adv., 2021,
11, 8003-8018
Structural alterations in the catalytic core of hSIRT2 enzyme predict therapeutic benefits of Garcinia mangostana derivatives in Alzheimer's disease: molecular dynamics simulation study†
Received
12th December 2020
, Accepted 3rd February 2021
First published on 18th February 2021
Abstract
Recent studies have shown that inhibition of the hSIRT2 enzyme provides favorable effects in neurodegenerative diseases such as Alzheimer's disease. Prenylated xanthone phytochemicals including α-mangostin, β-mangostin and γ-mangostin obtained from Garcinia mangostana, a well-established tropical plant, have been shown experimentally to inhibit sirtuin enzymatic activity. However, the molecular mechanism of this sirtuin inhibition has not been reported. Using comprehensive integrated computational techniques, we provide molecular and timewise dynamical insights into the structural alterations capable of facilitating therapeutically beneficial effects of these phytochemicals at the catalytic core of the hSIRT2 enzyme. Findings revealed the enhanced conformational stability and compactness of the hSIRT2 catalytic core upon binding of γ-mangostin relative to the apoenzyme and better than α-mangostin and β-mangostin. Although thermodynamic calculations revealed favorable binding of all the phytochemicals to the hSIRT2 enzyme, the presence of only hydroxy functional groups on γ-mangostin facilitated the occurrence of additional hydrogen bonds involving Pro115, Phe119, Asn168 and His187 which are absent in α-mangostin- and β-mangostin-bound systems. Per-residue energy contributions showed that van der Waals and more importantly electrostatic interactions are involved in catalytic core stability with Phe96, Tyr104 and Phe235 notably contributing π–π stacking, π–π T shaped and π–sigma interactions. Cumulatively, our study revealed the structural alterations leading to inhibition of hSIRT2 catalysis and findings from this study could be significantly important for the future design and development of sirtuin inhibitors in the management of Alzheimer's disease.
1. Introduction
Alzheimer's disease (AD) remains one of the leading causes of dementia (accounting for 50–70% of cases) in the aged population as there is currently neither cure nor treatment to completely halt its progression.1–3 An estimated 47 million people were affected globally in 2015 and it has been predicted that approximately 131 million people will suffer from AD in the next 30 years.4–7 AD is a complex neurodegenerative disease, characterized by amyloid-β (Aβ42)-plaques and hyperphosphorylated-tau (p-tau) tangles deposition leading to significant modifications in the cortex and hippocampus.2,3 Despite the fact that the pathophysiology of AD remains poorly understood, several hypotheses has nonetheless been proposed including cholinergic hypothesis,8–11 amyloid hypothesis,12–14 tau hypothesis,15–17 neuroinflammation hypothesis,17,18 biometal dyshomeostasis hypothesis,19–21 oxidative stress,22–27 insulin-degrading enzyme theory,28–30 homocysteine,31,32 phosphodiesterase33,34 and monoamine oxidase hypothesis.35,36
However, only five medications have so far been approved for the management of AD by the Food and Drug Administration (FDA) including tacrine (which has been withdrawn due to hepatotoxicity), donepezil, galantamine, rivastigmine and memantine.3 The first four drugs listed are all acetylcholinesterase inhibitors (AChEI) while memantine is an N-methyl-D-aspartate (NMDA) receptor antagonist. These pharmacologic interventions as well as non-pharmacologic therapies are mainly employed to mitigate the disabling effects from cognitive and functional decline.2 Due to paucity of remedial efficacy of these medications together with AD pathophysiology complexity, the need for new therapeutic alternatives cannot be overemphasized. Emerging research into future AD treatments have reported disease-modifying therapies (DMTs), symptomatic cognitive enhancers and symptomatic agents with a myriad of mechanisms of action.1,2 Over the past few decades, scientists have increasingly explored the beneficial effects of plant extracts and isolated active constituents on the emergence and progression of AD.37–39 Plant parts including roots, rhizome, bark, leaves, fruits and seeds have been shown to possess the ability to inhibit the formation of toxic plagues, enhance cholinergic signaling, improve oxidative stress and inhibit neuroinflammation.40–42 Medicinal plants with associated active compounds found to be beneficial in AD treatment include but not limited to Curcuma longa (curcumin, demethoxycurcumin and bis-demethoxycurcumin),43–45 Bacopa monnieri (bacopasides III, bacopasides IV, bacosides A, bacosides B),46,47 Centella asiatica (asiatic acid, asiaticoside),48,49 Convolvulus pluricaulis,50 Ginkgo biloba (bilobalide),51 Zingiber officinaleis (gingerol, shagols, bisabolene, zingiberene),52,53 Allium sativum (garlic extract).54,55 Other important natural products reported to possess neuroprotective and anti-AD effects include quercetin,56–58 epigallocatechin-3-gallate,59 resveratrol,60,61 berberine,62,63 huperzine A,64,65 luteolin66 and rosmarinic acid.67
Protein post-translational modifications (PTMs) have been reported to play significant roles in neurodegenerative diseases including Alzheimer's and Parkinson's disease by potentiating protein aggregation.68 Tau and alpha-synuclein (ASYN) particularly go through several PTMs (especially acetylation) to potentiate their aggregation and neurotoxicity. Cacabelos et al.69 reported that sirtuins are components of the epigenetic machinery that contributes to AD pathogenesis. It has also been established that there is correlation between SIRT2-C/T genotype and AD susceptibility in specific human populations.69 Human sirtuin proteins (hSIRTs) are nicotinamide adenine dinucleotide-dependent (NAD+ dependent) histone deacetylase enzymes shown experimentally to be involved in various cell-related processes including metabolism, DNA regulation, stress responses and aging process.70,71
There exist seven isoforms of the human sirtuin enzyme, SIRT1-7, all displaying different substrate specificities and subcellular localizations.72,73 While SIRT1, 6, and 7 are nuclear enzymes72,74, SIRT3, 4, and 5 are found in the mitochondria.75,76 SIRT2, however is mainly cytosolic and has been reported to deacetylate tubulin and p300.77
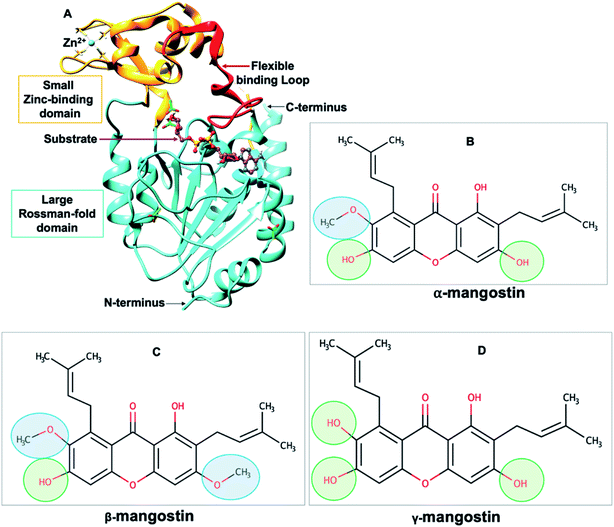 |
| Fig. 1 (A) Structure of human SIRT2 (hSIRT2) enzyme (PDB ID: 3ZGV)71 is shown as a cartoon model with the large Rossman-fold domain and zinc-binding domain in light blue and light brown, respectively. The flexible binding loop (red) is in a closed conformation and binds to a substrate (purple). (B–D) Chemical structures of Garcinia mangostana derivatives including α-mangostin, β-mangostin and γ-mangostin78 showing differences in attached functional groups. | |
All sirtuins possess a conserved catalytic core of approximately 275 amino acids with N- and C-terminal extensions.79,80 This catalytic core assumes an oval-shaped fold comprising of two globular subdomains linked by four loops. There is the large Rossman-fold domain which typically allows for NAD+ binding proteins and together with the smaller Zn2+-binding domain form the large groove for substrate binding.70 Binding of substrates to the active “large groove” site has been shown to induce a significant reorientation of the two domains relative to each other and also induce a closure of the cleft as well as the correct positioning of conserved residues for formation of the binding site tunnel.81 These rearrangements depict the dynamic structure of sirtuins. Although, a complex mechanism of physiological and pharmacological regulation of sirtuins has been reported, studies suggests that SIRT2 inhibition may have pharmacotherapeutic benefits on various age-related disorders including Alzheimer's disease.78,82
Recently, Garcinia mangostana (G. mangostana), a tropical plant commonly found in South East Asia with isolated active constituents including xanthones, anthocyanins, terpenes and tannins has been reported to possess anti-AD properties.83,84 Phytochemical analysis shows that G. mangostana contains α-mangostin, β-mangostin, γ-mangostin and 8-deoxygartanin which have all been shown to possess varying inhibitory capacities against hSIRT2 enzyme.78 Herein, we detailed the possible molecular mechanisms of hSIRT2 inhibition employed by G. mangostana derivatives for the treatment of AD. Using computational parameters, we explore the conformational dynamics of inhibitor binding to hSIRT2 enzyme as well determine the enzyme-inhibitor binding affinities.
2 Computational methodology
2.1 System preparation of SIRT2 enzyme and naturally occurring inhibitors (α-mangostin, β-mangostin and γ-mangostin)
SIRT2 protein with PDB ID: 3ZGV71 was downloaded from RSCB Protein Data Bank.85 We decided to use only chain A for this study since the two monomers of the hSIRT2 (PDB ID: 3ZGV) enzyme crystal structure are associated through a non-crystallographic symmetry depicting no significant difference (RMSD 0.38 Å over 293 atoms Cα atoms)71 in the two monomers. The 2D structures of G. mangostana derivatives (α-mangostin, β-mangostin and γ-mangostin) were prepared using Marvin Sketch software (http://www.chemaxon.com) and taken to 3D Avogadro molecular editor and visualizer software86 for auto-optimization, detachment of steric clashes and modification of existing interconnective bonds.
Multiple molecular docking of G. mangostana derivatives into the active site of the hSIRT2 enzyme was carried out using UCSF Chimera,87 followed by docking validation using Autodock Tools by redocking the phytochemicals in the protein and selecting docking scores and poses similar to those obtained with UCSF Chimera. In preparation for molecular dynamics (MD) simulations, enzyme-inhibitor complexes were standardized using Molegro Molecular Viewer (MMV).88 In all, four systems were prepared, consisting of unbound hSIRT2 (Apo), hSIRT2-α-mangostin, hSIRT2-β-mangostin and hSIRT2-γ-mangostin complex. These systems were then subjected to MD simulations of 330 ns using hitherto reported in-house protocols.89–92
2.2 Molecular dynamics simulations
This was done to investigate timescale motions of the component atoms of the enzyme-inhibitor systems against the unbound enzyme system to establish the net force and acceleration occurring in the different systems through the use of the PMEMD version of the graphical processor unit in AMBER 14.93 Parameterization for all inhibitors and the enzyme was carried out using FF14SB AMBER and GAFF force fields respectively while generation of partial charges for the inhibitors was carried out using Restrained Electrostatic Potential (RESP) and General Amber Force Field (GAFF) approach present in ANTECHAMBER.
Neutralization of the unbound and bound enzyme systems were done using sodium (Na+) and chloride (Cl−) counter ions, followed by the incorporation of hydrogen atoms in the AMBER 14 Leap module leading to generation of topology files. An orthorhombic box of TIP3P water molecules at a relative distance of 8 Å from all protein atoms was used to solvate all the systems.94 Energy minimization was carried out in two phases; partial minimization using 2500 steps with restraint potential of 500 kcal mol−1 Å−2 was done first followed by full minimization using 10
000 steps without conjugate restraints.
All systems were gradually heated from 0 to 300 K for 50 ps in a canonical ensemble (NVT) using a Langevin thermostat followed by equilibration for 5000 ps at a temperature of 300 K and a pressure of 1 bar maintained by a Berendsen barostat with a time-step of 2 fs in an NPT ensemble. Finally, MD simulations was conducted on all the systems and the corresponding trajectories were saved for every 1 ps run. Post-analysis follows MD simulations whereby, trajectories generated were used in AMBER PTRAJ module93,95 and CPPTRAJ module93,95 to analyze for root mean square deviations (RMSD), root mean square fluctuations (RMSF), radius of gyration (RoG), principal component analysis (PCA) and solvent accessible surface area (SASA).
2.3 Thermodynamic calculation
In a bid to establish the binding energies of the G. mangostana derivatives when bound to hSIRT2 enzyme, we employed the Molecular Mechanics Poisson-Boltzmann Surface Area (MM/PBSA) method,96,97 computationally designed for binding energy calculations. This method helps to predict interaction types present as well as the proportion of differential energy contributed by each amino acid residue towards inhibitor binding. 1000 complex frames extracted from the last 30 ns trajectories (which represents region of minimum deviations of less than 1.8 Å to reduce interference with ligand–protein activities due to entropy effects and taken as convergence) were used to perform calculations for the binding free energy and per-energy decomposition of the simulated systems. Total binding energy (ΔGbind) was then calculated from each snapshot using the equation below: |
ΔGbind = Gcomplex − (Genzyme − Ginhibitor)
| (1) |
|
ΔGbind = ΔGgas + Gsol − TΔS
| (2) |
ΔGbind is the summation of the gas phase energy and solvation energy minus entropy (TΔS) term.
|
ΔEgas = ΔEint + ΔEvdW + ΔEele
| (3) |
where Δ
Egas is regarded as the summation of AMBER force field internal energy, Δ
Eint (angle, bond and torsion), the van der Waals energy (Δ
EvdW) and the non-bonded electrostatic energy component (Δ
Eele). The solvation energy is calculated as follows:
Free energy of solvation and polar solvation contributions are denoted as Gsol and GPB respectively. GSA represents non-polar contributions calculated for solvent accessible surface area (SASA) where SASA is derived by employing 1.4 Å radius water probe, a surface tension constant, γ of 0.0072 kcal (mol Å2)−1 and constant b to 0 kcal mol−1. To estimate individual amino acid residue binding energy contributed towards enzyme structural stability and inhibitor affinity, we carried out analysis of per residue energy decomposition.
3 Results
3.1 Structural characterization of human SIRT2 (hSIRT2) apoenzyme upon the binding of G. mangostana derivatives
The catalytic core of hSIRT2 enzyme consisting primarily of the large Rossman-fold domain and small Zn2+-binding domain, exhibit complex structural perturbations depending on the bound ligand.98 In a bid to determine the changes in structural stability and compactness of the catalytic core of hSIRT2 when bound by the phytochemicals, we employed Cα Root Mean Square Deviations (RMSD) and Radius of Gyration (RoG) as investigational in silico parameters. Estimation of the Cα RMSD was also carried out to access the convergence of all the systems and convergence was generally observed after 300 ns of the simulation run whereby average RMSD of the bound systems relative to apo were lower than lower than 1.8 Å as illustrated in ESI Fig. 1.†
Previous studies89,92,99 showed that the structural stability of a protein or enzyme is inversely proportional to the observed RMSD value, that is, relatively higher RMSD value equates structural instability while lower RMSD value correlates to structural stability. As shown in Fig. 2A and ESI Table 1,† the Cα RMSD value (3.93 Å) was slightly higher in the apoenzyme as compared to the lowered RMSD values in the three mangostin-bound systems (RMSD values of 3.84 Å, 3.66 Å, and 3.34 Å for α-, β- and γ-mangostin respectively) suggesting that binding of α-, β- and γ-mangostin confers comparable atomistic stability at the catalytic core of hSIRT2 enzyme. This finding corroborates fewer atomistic deviations estimated for residues in the Rossman-fold domain and Zn2+-binding domain in the bound hSIRT2 systems especially in the γ-mangostin-bound system, an indication of conformational rigidity resulting from lowered atomistic motions (ESI Fig. 1†).
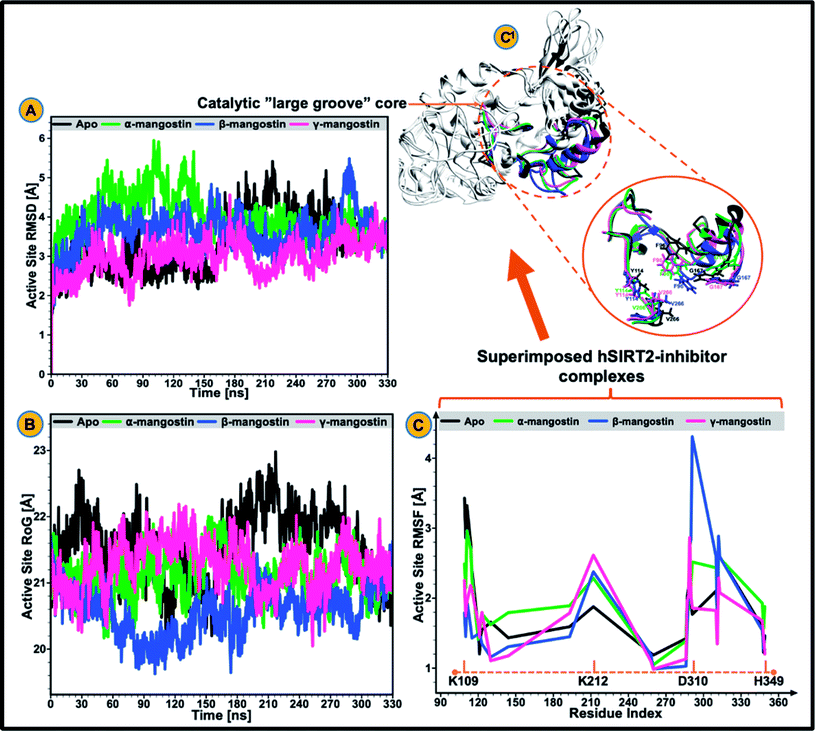 |
| Fig. 2 Structural analysis of the catalytic core of unbound (black), α-mangostin-bound (light green), β-mangostin-bound (blue) and γ-mangostin-bound hSIRT2 enzyme (strawberry). (A) Comparative active site Cα RMSD plots showing stability and atomistic deviations in the apo and three holo systems. (B) Comparative active site RoG plots among the apo and three holo systems. (C) hSIRT2 per-residue fluctuation plots showing the extent of atomistic fluctuations at the catalytic core from K109 to H349 with peak fluctuations at D310 in the β-mangostin ensemble. (C1) Comparative visualization of the catalytic core showing super-imposed form of the apo and the three holo structures. Inset: various motions of important catalytic core residues including F96, Y114, G167 and V266 during simulation (200 ns). | |
The average RMSDs estimated for all systems (both bound and unbound) as well as for the Rossman-fold domain and Zn2+-binding domain are given in ESI Table 2.† Further analysis of the compactness and atomistic mobility at the catalytic core was done by estimating the RoG of the systems. The correlation between RoG and structural compactness has also been previously reported97,99,100 and while a relatively high RoG could depict a structurally mobile enzyme, a low RoG would predict a structurally compact enzyme. As shown in Fig. 2B and ESI Table 1,† binding of G. mangostana derivatives increased the structural compactness of the catalytic core of hSIRT2 apoenzyme. The average RoG value of the unbound hSIRT2 catalytic core was 21.59 Å while for α-, β- and γ-mangostin-bound systems, it was estimated to be 21.02 Å, 20.49 Å and 21.08 Å respectively.
3.2 G. mangostana derivatives (only β and γ) lowers conformational flexibility in hSIRT2
We proceeded to further analyze possible perturbations at the hSIRT2 catalytic core using comparative conformational flexibility of the enzyme, reinforced by analysis plot of the catalytic core residues motions sustained across two principal components: PC1 and PC2. Using RMSF estimations to predict conformational flexibility of the enzyme, findings showed that residues in the unbound system maintained high fluctuations, while residues in the bound system exhibited slightly lower atomistic fluctuations at the enzyme catalytic core except for the α-mangostin-bound system which displayed a higher fluctuation that even exceeds that of the unbound system. This could be as a result of the binding orientation of the α-mangostin compound and the resultant interactions of the phytochemical with various hSIRT2 catalytic core residues. Although, an increase in conformational flexibility has historically been associated with enhanced enzyme activity particularly in mesophilic and psychrophilic enzymes, Kamal et al.101 argued that this is not usually the case. To this end, we believe that an aggregation of conformational behaviors by hSIRT2 enzyme will be more reliable in the prediction of possible hSIRT2 enzyme activity when bound to these phytochemicals. The estimated mean RMSF value of the hSIRT2 catalytic core for the unbound, α-mangostin-, β-mangostin- and γ-mangostin-bound systems were 1.97 Å, 2.11 Å, 1.93 Å and 1.66 Å respectively (ESI Table 1†). This could indicate that β-mangostin and γ-mangostin had superior activities to α-mangostin. Additionally, RMSF estimates for both the Rossman-fold binding domain and Zn2+-binding domain, constituting the catalytic core corroborate reduced atomistic motion of constituent amino acid residues when bound to G. mangostana derivatives as shown in ESI Table 2.† Furthermore, observation from PCA analysis of the catalytic core shows that relative atomistic motion in the unbound system is higher when compared to inhibitor-bound systems as depicted in Fig. 3E.
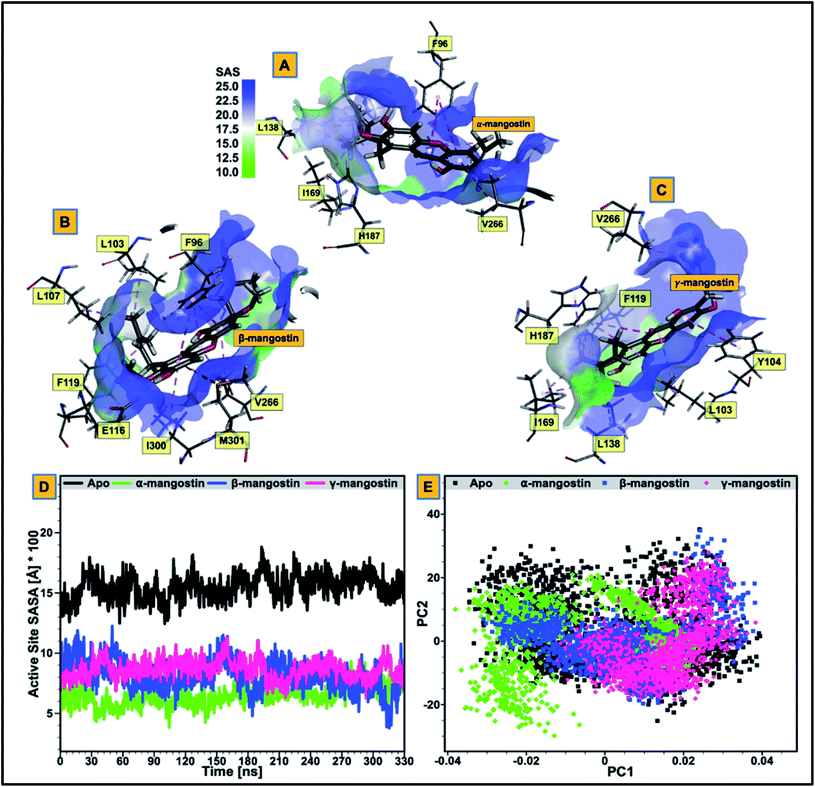 |
| Fig. 3 (A–C) Comparative visualization of solvent accessible surface area (SASA) of the catalytic core of hSIRT2 enzyme when bound to α-mangostin (A), β-mangostin (B) and γ-mangostin (C). The figure legend shows the progression from region of low solvent accessible surface area (light green) to region of high solvent accessible surface area (deep blue). (D) Comparative catalytic core SASA plots for the apo structure (black) and the three holo structures (α-mangostin (light green), β-mangostin (deep blue) and γ-mangostin (strawberry)). (E) Principal component analyses of the unbound hSIRT2 enzyme in comparison with the three inhibitor-bound forms. | |
3.3 Relative hydrophobicity of hSIRT2 catalytic core when substrate-bound predicts enhanced neuromodulatory action
We evaluated the effect of substrate binding on the change in solvent accessibility of amino acid residues constituting the catalytic core of the enzyme. This was achieved by estimating the surface area solvent accessibility (SASA) of the catalytic core of the apoenzyme (Fig. 3D), followed by SASA estimation of the Rossman-fold domain and Zn2+-binding domain that forms the active “large groove” site (ESI Fig. 2 and ESI Table 2†). As reported in previous studies, increased comparative SASA estimate predicts higher hydrophobicity of the catalytic core residues while reduced comparative SASA estimate correlates with higher hydrophilicity of the catalytic core residues.102 We discovered that change in accessibility (from unbound to bound) is significant for the residues that are involved in hSIRT2 catalytic core binding to G. mangostana derivatives.
The average catalytic core SASA estimate for the unbound system is 1454.30 Å while for α-, β- and γ-mangostin-bound systems, it is 646.09 Å, 830.13 Å and 603.11 Å respectively. This shows that the catalytic core residues of hSIRT2 enzyme when bound to G. mangostana derivatives possibly becomes buried in the large groove to mediate their neuromodulatory activities. The degree of change in SASA estimated for unbound system compared to the bound systems show the significant hydrophobic tendency of the residues as depicted in Fig. 3D and supported by independent SASA estimates for the Rossman-fold domain and Zn2+-binding domain of the bound systems, ESI Table 2.† In a bid to predict the inhibitor with the most tendency to bury catalytic core residues when bound, we estimated “ligand SASA” for the bound inhibitors with γ-mangostin showing the highest propensity to bury catalytic core residues (ESI Table 1†).
Comparative sequential visualization of the unbound and bound systems during timewise simulations show some changes in secondary structures of the hSIRT2 enzyme as a result of G. mangostana derivatives binding. We observed loop to α-helix transition for example at amino acid residues 107–110 (depicted in Fig. 4D–F), 115–116, 119, 125–126 and 136–137. Other transitions include loop to sheet and α-helix to loop transitions.
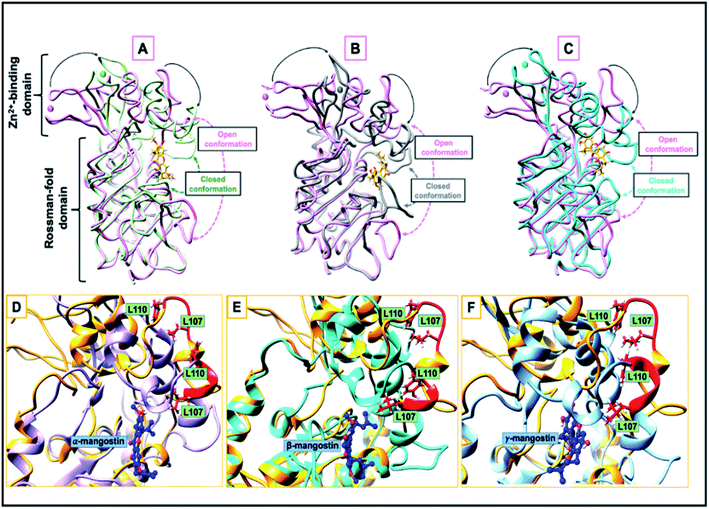 |
| Fig. 4 (A–C) Open and closed conformation comparison of the catalytic site between hSIRT2 structure (PDB ID: 3ZGV) in the unbound form (magenta) and in complex with (A) α-mangostin (light green), (B) β-mangostin (dark grey) and (C) γ-mangostin (cyan); liquorice view. The apo and halo structures are super-imposed while the inhibitors are shown in stick model. The Zn2+ ions are represented in spheres. (D–F) A loop-to-helix transition. Here, the loop of hSIRT2 (Leu107 to Leu110, colored in red) is unstructured in the unbound state (PDB ID: 3ZGV; light brown), adopts a helical conformation upon binding to (D) α-mangostin (light purple), (E) β-mangostin (cyan) and (F) γ-mangostin (light blue). | |
3.4 α, β, and γ-mangostins demonstrated systemic variations in their interaction mechanisms at the hSIRT2 catalytic core
Final decomposition energies contributed by each residue of the hSIRT2 catalytic core towards binding of α-mangostin, β-mangostin and γ-mangostin were calculated using the MM/PBSA method.103 This approach is essential since the stability and binding affinity of a substrate (therapeutic compound) to its target is primarily determined by the types of interaction and energy contributions of key binding site residues. Therefore, the energy contributions of individual catalytic core residues towards the binding affinity of Garcinia mangostana derivatives included electrostatic and van der Waals forces. Typically, residues with energies below −0.8 kcal mol−1 are considered important for enzyme-inhibitor molecular recognition, high affinity binding and relative stability of small molecular compounds.104
All investigated G. mangostana derivatives displayed considerable network of intermolecular interactions with binding site residues which could presume favorable binding affinities of all derivatives to hSIRT2 enzyme. α-Mangostin, β-mangostin and γ-mangostin were discovered to interact with hSIRT2 amino acid residues at the interface of the two globular domains through hydrogen bonding and hydrophobic interactions. All the three holo systems show peculiar intermolecular interactions but similar catalytic core residues (including both Rossman-fold and Zn2+-binding domain residues) were found to participate in intermolecular interactions across the three substrate–enzyme systems which include Ala85, Phe96, Arg97, Leu103, Tyr104, Pro115, Phe119, Leu134, Ile169, His187, Phe235 and Val266. We believe these are the major hSIRT2 catalytic core residues through which common G. mangostana-mediated inhibitory activities are propagated, although other important residues may contribute to selectivity of action of the G. mangostana derivatives when bound to hSIRT2 enzyme.
3.4.1 Enzyme-inhibitors hydrogen bond interaction network. Intermolecular hydrogen bond interactions between G. mangostana derivatives and various hSIRT2 enzyme catalytic core residues have been found to participate in stabilizing the isolates at the active site as depicted in Fig. 5A–C and ESI Fig. 4.† Hydrogen bond interactions in the α-mangostin-hSIRT2 system was mediated mainly by Ala85 (Ala85:H – α-mangostin:O4), Gln167 (α-mangostin:HO – Gln167:O), Ser263 (Ser263:H – α-mangostin:O5), Gln265 (α-mangostin:HO1 – Gln265:OE1) which were observed between 1 ns and 270 ns of the dynamic simulation run. The frequency of these hydrogen bond interactions was more (occurring in two's and three's) at the early stages of molecular dynamics simulations and appearing to reduce as the simulation progresses. Occurrence of hydrogen bond interactions in the β-mangostin-hSIRT2 system, on the other hand, occurred throughout the simulation process with this interaction type mediated mainly by Ala85 (Ala85:H – β-mangostin:O4), Glu116 (β-mangostin – Glu116:OE2), Gln167 (β-mangostin:H1 – Gln167:O and Gln167:HE22 – β-mangostin:O1), Ser263 (Ser263:HN – β-mangostin:O5) and Asn286 (Asn286:HD21 – β-mangostin:O5) as shown in ESI Table 3.† The presence of more hydroxy groups (4 –OH groups) on γ-mangostin and perhaps, better shape complimentarity at the catalytic core ensures that there are more participatory catalytic residues in the formation of hydrogen bond throughout the 330 ns simulation run than observed in the α-mangostin- and β-mangostin-bound systems. Hydrogen bond interactions in the γ-mangostin-hSIRT2 system was hence mediated by Ala85 (Ala85:H – γ-mangostin:O4), Pro115 (γ-mangostin:C21 – Pro115:H), Glu116 (γ-mangostin:H3 – Glu116:OE1), Phe119 (γ-mangostin:H – Phe119), Asn168 (γ-mangostin:H2 – Asn168:OD1), His187 (His187:HE2 – γ-mangostin:O3), Val233 (γ-mangostin:H1 – Val233:O) and Ser263 (Ser263:HG – γ-mangostin:O5). Percentage occupancy of these hydrogen bond interactions are cumulatively highest in the γ-mangostin-bound system than observed in the α-mangostin- and β-mangostin-bound systems as shown in ESI Table 3.†
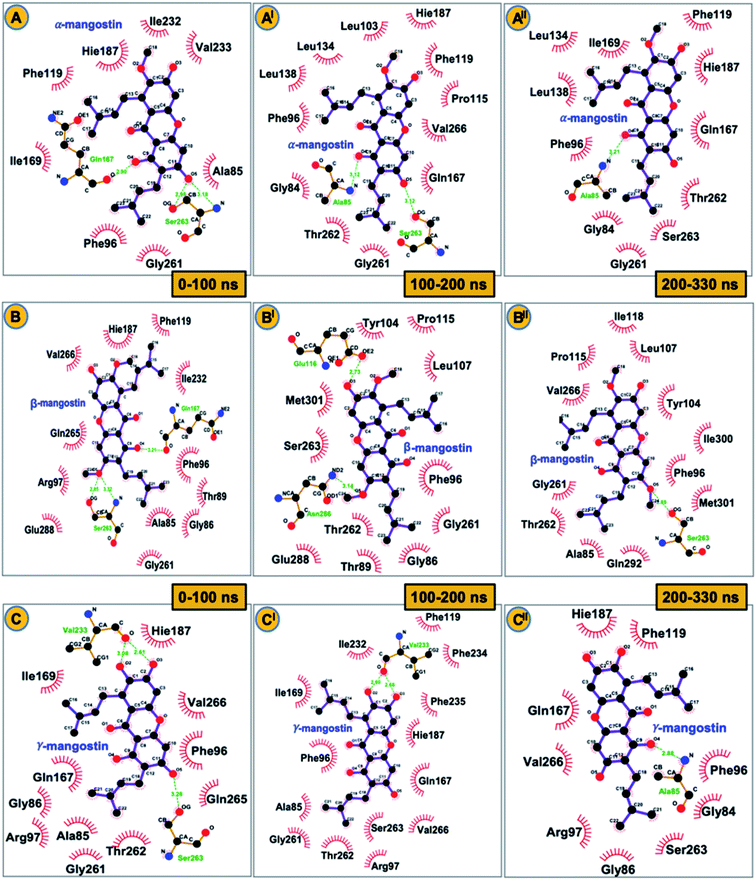 |
| Fig. 5 2D schematic representation of intermolecular hydrogen bond interactions contributing to stability of G. mangostana derivatives (α-mangostin (A), β-mangostin (B) and γ-mangostin (C)) at the catalytic core of hSIRT2 enzyme. Important participatory active site residues (including Ala85, Gln167 and Ser263 for α-mangostin-bound; Glu116, Ser263 and Asn286 for β-mangostin-bound; Ala85, Val233 and Ser263 for γ-mangostin-bound systems) between 0 – 330 ns simulation run (categorized into initial (0–100 ns), intermediate (100–200 ns) and final (200–330 ns) simulation timeframes) in the different enzyme-inhibitor complex ensembles are also shown. | |
3.4.2 Hydrophobic interaction contributions at the active site. Evidently, presence of two methoxy groups in β-mangostin increases the reactivity of the ligand and allows more hydrophobic interactions with catalytic core residues than seen in other phytochemicals. The dynamic interaction tendencies of the phytochemical (β-mangostin) ensure that there are more alkyl interactions with hSIRT2 catalytic core residue side chains like Phe96, Arg97, Leu107, Pro115, Leu118, Leu138, Ile169, Ile232 and Val266. Another important observation is the formation of π–alkyl, π–π stacked and π–π T shaped interactions with aromatic/hydrophobic residues (such as Phe96, Tyr104, Phe119 and His187) as well as π–sulfur interaction with Met301 of the hSIRT2 enzyme. While most of these hydrophobic interactions occur in the α-mangostin- and γ-mangostin-bound systems, none of them showed the π–sulphur interaction seen in the β-mangostin-bound system which may add to the reasons behind the anti-AD effect seen in these two phytochemicals. Also, all the three aromatic rings in β-mangostin were involved in hydrophobic interactions with the catalytic site residues which wasn't the case for both α-mangostin- and γ-mangostin-bound systems.It was observed that towards α-mangostin and β-mangostin binding, Leu103 contributed the highest total binding energy of −2.37 kcal mol−1 and −3.33 kcal mol−1 respectively while Phe119 with a total binding energy of −2.88 kcal mol−1 contributed most to γ-mangostin binding. This Phe119 interaction was achieved mainly through π–lone pair, π–π stacked and π-donor hydrogen bond interactions involving aromatic rings B and C of the γ-mangostin. Furthermore, we compared the contributions of the Rossman-fold domain residues and Zn2+-binding domain residues that constitute the large groove in the catalytic core in all simulated models since they are involved in catalysis.105
As shown in Fig. 6A–C, energy dynamics of all simulated models suggest all phytochemicals effectively modulate the hSIRT2 catalytic core though relatively higher favorable interactions (e.g. π–alkyl, π–π T shaped and π–π stacked) were found at the Zn2+-binding domain (such as Phe96 that showed total energy of −2.29 kcal mol−1, −3.33 kcal mol−1 and −1.25 kcal mol−1 in α, β, and γ-mangostin-bound systems respectively) than at the Rossman-fold domain such as Ile169 that showed total energy −1.03 kcal mol−1, −0.03 kcal mol−1 and −0.99 kcal mol−1 in α, β, and γ-mangostins respectively. Also, we unraveled through molecular visualization that binding of G. mangostana derivatives to the catalytic core of the hSIRT2 enzyme was characterized mainly by van der Waals interactions as depicted in Fig. 6A–C, though substantial electrostatic interactions were observed especially in the α-mangostin- and γ-mangostin-bound systems which could be responsible for the molecular recognition attributed to these two phytochemicals important for their beneficial neuromodulatory activity.
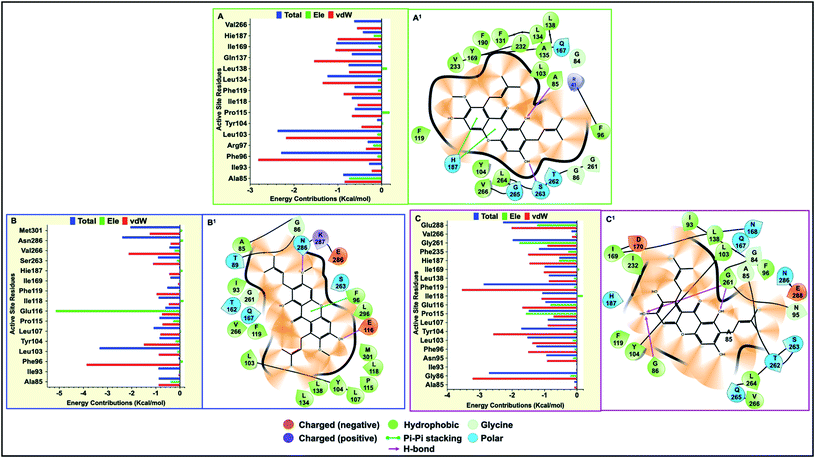 |
| Fig. 6 (A to C) Per-residue decomposition plots showing energy contributions among the hSIRT2 catalytic core residues when bound to α-mangostin (A), β-mangostin (B), and γ-mangostin (C). (A1 to C1) Shows the various important intermolecular interactions formed with hSIRT2 enzyme during the simulation period for α-mangostin (A1), β-mangostin (B1) and γ-mangostin (C1). | |
3.5 Differential binding energy profiles of α, β, and γ-mangostins
Estimations of energy contributions of the individual binding site residues and systemic binding modes/orientations of the compounds were further complemented with binding energy (ΔG) calculations for all inhibitor-enzyme systems. This approach was essential to determine the respective binding affinities of the compounds relative to their inhibitory potencies and mechanistic impacts when bound to the hSIRT2 catalytic core. As presented in Table 1, α-mangostin, β-mangostin and γ-mangostin were bound favorably to hSIRT2 with respective ΔG values of −34.56 kcal mol−1 and −47.87 kcal mol−1 and −35.73 kcal mol−1. Comparatively, β-mangostin recorded the highest van der Waals interaction (−55.15 kcal mol−1) followed by γ-mangostin with −46.74 kcal mol−1. Total electrostatic interactions in γ-mangostin-bound system of −9.91 kcal mol−1 shows that the γ-mangostin favors more electrostatic interactions relative to α-mangostin-bound system.
Table 1 Binding energy contributions of α-mangostin, β-mangostin and γ-mangostina
System |
Energy contributions (kcal mol−1) |
ΔEvdw |
ΔEele |
ΔEPB |
ΔEsurf |
ΔEgas |
ΔEsol |
ΔEbind |
ΔEele = electrostatic energy; ΔEvdw = van der Waals energy; ΔEgas = gas phase free energy; ΔEsol = solvation free energy; ΔEbind = total binding free energy. |
α-Mangostin |
−44.52 ± 0.12 |
−7.36 ± 0.24 |
23.36 ± 0.17 |
−6.03 ± 0.01 |
−51.88 ± 0.25 |
17.31 ± 0.17 |
−34.56 ± 0.15 |
β-Mangostin |
−55.15 ± 0.15 |
−15.87 ± 0.30 |
29.99 ± 0.18 |
−6.84 ± 0.01 |
−71.01 ± 0.31 |
23.15 ± 0.18 |
−47.87 ± 0.17 |
γ-Mangostin |
−46.74 ± 0.12 |
−9.91 ± 0.22 |
26.60 ± 0.16 |
−5.68 ± 0.01 |
−56.65 ± 0.21 |
20.92 ± 0.16 |
−35.73 ± 0.12 |
Also, a relatively high unfavorable polar solvation energies, ΔEgas and ΔEsol value in the β-mangostin- and γ-mangostin-bound systems shows that binding of these phytochemicals is more favorable at the hydrophobic hSIRT2 binding pocket compared to α-mangostin. These findings amplify experimental reports supporting the fact that α-mangostin, β-mangostin and γ-mangostin are phytochemical derivatives of G. mangostana with beneficial effects on many age-related diseases including Alzheimer's disease through their inhibitory actions on sirtuin enzymes.78
4 Discussion
We report here that the structural alterations characteristic of binding of G. mangostana prenylated xanthone isolates including α-mangostin, β-mangostin and γ-mangostin to hSIRT2 enzyme (Fig. 1–3) predicts their neuroprotective benefits in AD. Importantly, we show that these isolates favorably bind to hSIRT2 enzyme (an histone deacetylase enzyme with pathological roles in neurodegenerative diseases and cancer) as depicted in Table 1, leading to pharmacological inhibition of the enzyme. Furthermore, we identify the various hSIRT2 catalytic core residues involved in inhibitors binding (Fig. 5 and 6), contributing to stability of the enzyme active site and perpetuating the enzyme inhibition.
Scientists have over the years debated the link between protein dynamics and function using arguments related to the fact that enzyme structural flexibility affects its catalytic reactivity106,107 while some argued that catalysis is independent of collective dynamics.108,109 Newer studies however emphasized that the rate-limiting factor in catalysis is the preorganization of the active site and structural dynamics aid reorganization of conformational elements near the catalytic site.110 The hSIRT2 catalytic functional core stabilizes bound substrates and inhibitors using four associated loop regions, three of which are in the Rossman-fold domain while the fourth flexible loop is in the small Zn2+-binding domain.
A comparison between the hSIRT2 holo forms (inhibitor bound) and apo form (unbound) showed that the small Zn2+-binding domain moves closer to the large Rossman-fold domain upon G. mangostana derivatives binding as observed in other SIRTs71,81 (Fig. 4A–C). In their work on how catalytic loop motions expedite ligand recognition and binding in enzymes, Kurkcuoglu et al.111 posited that RMSD between the open and closed forms of the enzyme functional parts varies in the range of 0.9–3.9 Å after super-imposition of the open and closed structures. Comparison of average RMSD with respect to the γ-mangostin-bound system showed deviation in motion between the open and closed conformations to be 0.8 Å. Though, this is higher than deviations observed for the α-mangostin- and β-mangostin-bound systems, it sets a tone for other parameters used to investigate comparative conformational motions between the bound state (holo) and unbound state (apo).
It is therefore apparent that G. mangostana derivatives induce local structural changes, including rotation of the side chains of L107 and L110 to allow interactions with inhibitors. Also, conformational stability of the hSIRT2 enzyme is relatively higher in the Rossman-fold domain than in the Zn2+-binding domain which underlies the importance of Rossman-fold domain to inhibition of catalysis in the enzyme. Furthermore, reduced conformational flexibility of hSIRT2 catalytic loop in the three enzyme holo states relative to the apo state predicates the inhibition of enzyme catalysis of hSIRT2 when bound to G. mangostana derivatives. This observation agrees with experimental evidence which proposes that the loss of conformational motion affects enzymatic mechanism even if structure and electrostatics are preserved.107 B factor, which is also a measure of atomistic fluctuations is lowest in γ-mangostin-bound system when compared with other holo systems and the apo system (ESI Table 1†). hSIRT2 non-polar (hydrophobic) pocket is typically lined with solvent exposed residues in the apo state112 but binding of G. mangostana derivatives buries these functional residues deep in the catalytic core of the enzyme. This structural alteration is more pronounced in the γ-mangostin-bound system and it correlates with aforementioned structural dynamics lending credence to the potent hSIRT2 inhibitory capability of G. mangostana derivatives.
The calculated binding energy of the three mangostin-bound systems suggest comparatively favorable binding affinity of these prenylated xanthone isolates for hSIRT2 enzyme. Specific intermolecular interactions of hSIRT2 catalytic core residues and γ-mangostin involving hydrogen bond formation between Pro115, Phe119 and His187 of the Zn2+-binding domain and Asn 168 of the Rossman-fold domain with γ-mangostin are presumably very important to experimentally proven and superior neuromodulatory activity of γ-mangostin. These specific interactions are relatively non-existent in the α-mangostin- and β-mangostin-bound systems. Also, multiple hydrophobic interactions were formed across the three holo systems as illustrated in Fig. 6A1–C1 and 7A–C.
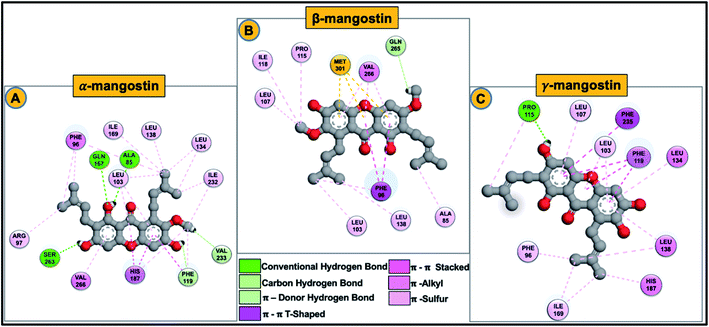 |
| Fig. 7 2D schematic diagram of G. mangostana derivatives (α-mangostin (A), β-mangostin (B) and γ-mangostin (C)) with important hSIRT2 enzyme participatory hydrophobic interactions. Chemical structure of phytochemicals are represented using ball and stick model. | |
The carbonyl-attached benzene ring of the derivatives was found to be very active allowing for varied types of interaction. The various favorable ligand orientations at the large groove of hSIRT2 allowed for stability of the catalytic core through hydrogen bond interactions of the two hydroxyl groups on C1 and C3 of the aromatic ring A in the α-mangostin. β-mangostin possess only two hydroxyl groups on C1 and C6 of the aromatic rings A and C respectively which evidently reduced the number of hydrogen bond interactions with the enzyme. The oxygen group that forms part of the –OCH3 functional group on C3, however engaged in hydrogen bond interactions with Ser263 at times during the course of simulations while the occurrence of bulky alkyl chains close to the hydroxy groups reduced hydrogen bond formation observed in other phytochemicals. In the γ-mangostin-bound system, –OH groups on C1 (interacting mainly with Ala85 and Asn168), C3 (interacting mainly with Pro115 and Glu116) and C6 (interacting mainly with His187) belonging to the aromatic rings A and C of the γ-mangostin allowed for more hydrogen bond interactions with catalytic residues of hSIRT2 enzyme.
Although, β-mangostin and α-mangostin systems show higher total binding energy than γ-mangostin which was presumably due to the presence of more reactive functional groups (three hydroxy and one methoxy group in α-mangostin and two hydroxy and two methoxy groups in β-mangostin) thereby elongating the side chains for interactions with catalytic core residues than in the γ-mangostin which possess 4 hydroxy groups, we believe that these hydroxyl groups allow for formation of more conventional and π-donor hydrogen bonds which are presumably important for neuroprotective activity and reduced toxicity. The favorable total binding energy of γ-mangostin-bound system as well as important hydrogen bond interactions with more participatory binding cavity residues together with the consistency of the structural alterations observed in the γ-mangostin-bound system, informed our hypothesis that γ-mangostin may be the most ideal of the three G. mangostana derivatives investigated as hSIRT2 inhibitory candidate for future drug development purposes. Although, this assertion, is supported by Yeong et al.78 in their recent experimental work on discovery of γ-mangostin and β-mangostin as potent and selective inhibitor of hSIRT2 enzyme for possible neuromodulatory and chemotherapeutic activities, we believe further investigations may be necessary to ascertain the most potent G. mangostana derivative.
5 Conclusion
G. mangostana-derived prenylated xanthone phytochemicals were docked to catalytic “large groove’ core of hSIRT2 enzyme and subjected to 330 ns molecular dynamics simulations with the resultant trajectories subsequently analyzed. Findings showed that γ-mangostin induced the most stable and compact structural conformation for hSIRT2 enzyme compared to β-mangostin and α-mangostin. These effects were particularly observed at the hydrophobic active site pocket of the enzyme and reinforced by similar findings when the zinc-binding and Rossman-fold domains were investigated. Relatively, presence of –OCH3 functional groups in α-mangostin and β-mangostin allows for more interactions with the hSIRT2 active site residues while the presence of only –OH groups in γ-mangostin ensures that favorable hydrogen bond interactions presumably important for neuroprotective activity are established with hSIRT2 active site non-polar residues.
Also, γ-mangostin was more deeply bound in the catalytic core of the enzyme allowing for favorable orientations and preventing possible unfavorable interactions that can cause toxicity. Moreover per-residue energy decomposition analysis showed favorable van der Waals forces, electrostatic and total energies were contributed towards binding of the phytochemicals. Although the relative binding free energies associated with ligand binding is highest in β-mangostin, all the phytochemicals including α-mangostin and γ-mangostin demonstrated favorable binding to the enzyme. When all investigations are put together, we discovered that the phytochemical derivatives of G. mangostana (α-mangostin, β-mangostin and γ-mangostin) examined are potent hSIRT2 inhibitors capable of exhibiting pharmacological activities (neuromodulatory activities) against Alzheimer's diseases. Further investigations may be necessary to prove which of the phytochemicals is the most potent hSIRT2 inhibitor.
Human and animal rights
No animals or humans were used for studies that are the basis of this research.
Funding
This research did not receive any specific grant from funding agencies in the public, commercial, or not-for-profit sectors.
Author contributions
Akawa OB: conceptualization, methodology, formal analysis, investigation, visualization, writing – original draft, writing – review & editing. Subair TI: analysis, writing – review & editing. Soremekun OS: writing – review & editing. Olotu FA: writing – review & editing, validation. Soliman MES: validation, supervision.
Conflicts of interest
The authors declare no conflict of interest, financial or otherwise.
References
- R. Loera-Valencia, et al., Current and emerging avenues for Alzheimer's disease drug targets, J. Intern. Med., 2019, 286(4), 398–437, DOI:10.1111/joim.12959.
- A. Atri, Current and Future Treatments in Alzheimer's Disease, Semin. Neurol., 2019, 39(2), 227–240, DOI:10.1055/s-0039-1678581.
- P. Patil, A. Thakur, A. Sharma and S. J. S. Flora, Natural products and their derivatives as multifunctional ligands against Alzheimer's disease, Drug Dev. Res., 2020, 81(2), 165–183, DOI:10.1002/ddr.21587.
- R. Brookmeyer, E. Johnson, K. Ziegler-Graham and H. M. Arrighi, Forecasting the global burden of Alzheimer's disease, Alzheimer's Dementia, 2007, 3(3), 186–191, DOI:10.1016/j.jalz.2007.04.381.
- R. Brookmeyer, et al., National estimates of the prevalence of Alzheimer's disease in the United States, Alzheimer's Dementia, 2011, 7(1), 61–73, DOI:10.1016/j.jalz.2010.11.007.
- C. P. Ferri, et al., Global prevalence of dementia: a Delphi consensus study Cleusa, Lancet, 2005, 366(9503), 2112–2117, DOI:10.7458/obs512011424.
- B. Winblad, et al., Defeating Alzheimer's disease and other dementias: a priority for European science and society, Lancet Neurol., 2016, 15(5), 455–532, DOI:10.1016/S1474-4422(16)00062-4.
- B. Js and H. Rj, Donepezil for dementia due to Alzheimer's disease (Review), 2018, vol. 6, DOI:10.1002/14651858.CD001190.pub3, http://www.cochranelibrary.com.
- P. T. Francis, A. M. Palmer, M. Snape and G. K. Wilcock, The cholinergic hypothesis of Alzheimer's disease: a review of progress, J. Neurol., Neurosurg. Psychiatry, 1999, 66(2), 137–147, DOI:10.1136/jnnp.66.2.137.
- N. Jiang, et al., Design, synthesis and biological evaluation of new coumarin-dithiocarbamate hybrids as multifunctional agents for the treatment of Alzheimer's disease, Eur. J. Med. Chem., 2018, 146, 287–298, DOI:10.1016/j.ejmech.2018.01.055.
- Y. Jiang, H. Gao and G. Turdu, Traditional Chinese medicinal herbs as potential AChE inhibitors for anti-Alzheimer's disease: a review, Bioorg. Chem., 2017, 75, 50–61, DOI:10.1016/j.bioorg.2017.09.004.
- O. B. Villaflores, Y. J. Chen, C. P. Chen, J. M. Yeh and T. Y. Wu, Curcuminoids and resveratrol as anti-Alzheimer agents, Taiwan, J. Obstet. Gynecol., 2012, 51(4), 515–525, DOI:10.1016/j.tjog.2012.09.005.
- A. J. Lin, et al., Spatial frequency domain imaging of intrinsic optical property contrast in a mouse model of alzheimer's disease, Ann. Biomed. Eng., 2011, 39(4), 1349–1357, DOI:10.1007/s10439-011-0269-6.
- R. E. Tanzi and L. Bertram, Twenty years of the Alzheimer's disease amyloid hypothesis: a genetic perspective, Cell, 2005, 120(4), 545–555, DOI:10.1016/j.cell.2005.02.008.
- J. Cummings, G. Lee, A. Ritter and K. Zhong, Alzheimer's disease drug development pipeline, Alzheimer's Dement. Transl. Res. Clin. Interv., 2018, 4, 195–214, DOI:10.1016/j.trci.2018.03.009.
- J. R. Roland and H. Jacobsen, Alzheimer's disease: from pathology to therapeutic approaches, Angew. Chem., Int. Ed., 2009, 48(17), 3030–3059, DOI:10.1002/anie.200802808.
- M. G. Savelieff, G. Nam, J. Kang, H. J. Lee, M. Lee and M. H. Lim, Development of multifunctional molecules as potential therapeutic candidates for Alzheimer's disease, Parkinson's disease, and amyotrophic lateral sclerosis in the last decade, Chem. Rev., 2018, 119(2), 1221–1322, DOI:10.1021/acs.chemrev.8b00138.
- Z. Qu, V. V. Mossine, J. Cui, G. Y. Sun and Z. Gu, Protective Effects of AGE and Its Components on Neuroinflammation and Neurodegeneration, NeuroMol. Med., 2016, 18(3), 474–482, DOI:10.1007/s12017-016-8410-1.
- K. P. Kepp, Bioinorganic Chemistry of Alzheimer’s Disease, Chem. Rev., 2012, 112(10), 5193–5239, DOI:10.1021/cr300009x.
- M. A. Deibel, W. D. Ehmann and W. R. Markesbery, Copper, iron, and zinc imbalances in severely degenerated brain regions in Alzheimer's disease: possible relation to oxidative stress, J. Neurol. Sci., 1996, 143(1–2), 137–142, DOI:10.1016/S0022-510X(96)00203-1.
- P. Faller, C. Hureau and O. Berthoumieu, Role of metal ions in the self-assembly of the Alzheimer's amyloid-β peptide, Inorg. Chem., 2013, 52(21), 12193–12206, DOI:10.1021/ic4003059.
- Z. Chen and C. Zhong, Oxidative stress in Alzheimer's disease, Neurosci. Bull., 2014, 30(2), 271–281, DOI:10.1007/s12264-013-1423-y.
- L. M. Sayre, G. Perry and M. A. Smith, Oxidative stress and neurotoxicity, Chem. Res. Toxicol., 2008, 21(1), 172–188, DOI:10.1021/tx700210j.
- D. J. Bonda, H. G. Lee, J. A. Blair, X. Zhu, G. Perry and M. A. Smith, Role of metal dyshomeostasis in Alzheimer's disease, Metallomics, 2011, 3(3), 267–270, 10.1039/c0mt00074d.
- M. A. Greenough, J. Camakaris and A. I. Bush, Metal dyshomeostasis and oxidative stress in Alzheimer's disease, Neurochem. Int., 2013, 62(5), 540–555, DOI:10.1016/j.neuint.2012.08.014.
- C. Cheignon, M. Tomas, D. Bonnefont-Rousselot, P. Faller, C. Hureau and F. Collin, Oxidative stress and the amyloid beta peptide in Alzheimer's disease, Redox Biol., 2018, 14, 450–464, DOI:10.1016/j.redox.2017.10.014.
- R. Sultana and D. A. Butterfield, Redox proteomics studies of in vivo amyloid beta-peptide animal models of Alzheimer's disease: insight into the role of oxidative stress, Proteomics: Clin. Appl., 2008, 2(5), 685–696, DOI:10.1002/prca.200780024.
- M. N. P. S. Matioli and R. Nitrini, Mecanismos que ligam a resistência insulínica cerebral à doença de alzheimer: uma breve revisão, Dement. Neuropsychol., 2015, 9(2), 96–102, DOI:10.1590/1980-57642015DN92000003.
- O. Pivovarova, A. Höhn, T. Grune, A. F. H. Pfeiffer and N. Rudovich, Insulin-degrading enzyme: new therapeutic target for diabetes and Alzheimer's disease?, Ann. Med., 2016, 48(8), 614–624, DOI:10.1080/07853890.2016.1197416.
- S. Rom, et al., Hyperglycemia-Driven Neuroinflammation Compromises BBB Leading to Memory Loss in Both Diabetes Mellitus (DM) Type 1 and Type 2 Mouse Models, Mol. Neurobiol., 2019, 56(3), 1883–1896, DOI:10.1007/s12035-018-1195-5.
- J. M. Zhuo, H. Wang and D. Praticò, Is hyperhomocysteinemia an Alzheimer's disease (AD) risk factor, an AD marker, or neither?, Trends Pharmacol. Sci., 2011, 32(9), 562–571, DOI:10.1016/j.tips.2011.05.003.
- J.-M. Zhuo, Diet-Induced Hyperhomocysteinemia Increases Amyloid-B. Formation and Deposition in a Mouse Model of Alzheimer's Disease, Curr. Alzheimer Res., 2009, 999(999), 1–10, DOI:10.2174/1567209199392222050.
- M. Cuadrado-Tejedor, et al., A First-in-Class Small-Molecule that Acts as a Dual Inhibitor of HDAC and PDE5 and that Rescues Hippocampal Synaptic Impairment in Alzheimer's Disease Mice, Neuropsychopharmacology, 2017, 42(2), 524–539, DOI:10.1038/npp.2016.163.
- J. Shi, et al., Cyclic AMP-dependent protein kinase regulates the alternative splicing of tau exon 10: a mechanism involved in tau pathology of Alzheimer disease, J. Biol. Chem., 2011, 286(16), 14639–14648, DOI:10.1074/jbc.M110.204453.
- M. Bortolato, K. Chen and J. C. Shih, Monoamine oxidase inactivation: from pathophysiology to therapeutics, Adv. Drug Delivery Rev., 2008, 60(13–14), 1527–1533, DOI:10.1016/j.addr.2008.06.002.
- S. Schedin-Weiss, et al., Monoamine oxidase B is elevated in Alzheimer disease neurons, is associated with γ-secretase and regulates neuronal amyloid β-peptide levels, Alzheimer's Res. Ther., 2017, 9(1), 1–19, DOI:10.1186/s13195-017-0279-1.
- N. S. Mohd Sairazi and K. N. S. Sirajudeen, Natural Products and Their Bioactive Compounds: Neuroprotective Potentials against Neurodegenerative Diseases, Evidence-based Complement, Altern. Med., 2020, 2020, 5–7, DOI:10.1155/2020/6565396.
- G. El-Saber Batiha, et al., The pharmacological activity, biochemical properties, and pharmacokinetics of the major natural polyphenolic flavonoid: quercetin, Foods, 2020, 9(3) DOI:10.3390/foods9030374.
- D. Wang, et al., Impact of natural products on the cholesterol transporter ABCA1, J. Ethnopharmacol., 2020, 249, 112444, DOI:10.1016/j.jep.2019.112444.
- O. A. Olajide and S. D. Sarker, Alzheimer’s disease: natural products as inhibitors of neuroinflammation, Inflammopharmacology, 2020, 28(6), 1439–1455, DOI:10.1007/s10787-020-00751-1.
- N. Ansari and F. Khodagholi, Natural Products as Promising Drug Candidates for the Treatment of Alzheimer's Disease: Molecular Mechanism Aspect, Curr. Neuropharmacol., 2013, 11(4), 414–429, DOI:10.2174/1570159x11311040005.
- T. T. Bui and T. H. Nguyen, Natural product for the treatment of Alzheimer's disease, J. Basic Clin. Physiol. Pharmacol., 2017, 28(5), 413–423, DOI:10.1515/jbcpp-2016-0147.
- B. B. Aggarwal, C. Sunfaram, N. MAlani and H. Ichikawa, The Molecular Targets and Therapeutic Uses of Curcumin in Health and Disease, 2007 Search PubMed.
- A. N. Begum, et al., Curcumin structure-function, bioavailability, and efficacy in models of neuroinflammation and Alzheimer's disease, J. Pharmacol. Exp. Ther., 2008, 326(1), 196–208, DOI:10.1124/jpet.108.137455.
- T. Hamaguchi, K. Ono and M. Yamada, Curcumin and Alzheimer's disease, CNS Neurosci. Ther., 2010, 16(5), 285–297, DOI:10.1111/j.1755-5949.2010.00147.x.
- A. Russo and F. Borrelli, Bacopa monniera, a reputed nootropic plant: an overview, Phytomedicine, 2005, 12(4), 305–317, DOI:10.1016/j.phymed.2003.12.008.
- M. Dhanasekaran, B. Tharakan, L. A. Holcomb, A. R. Hitt, K. A. Young and B. V. Manyam, Neuroprotective Mechanisms of Ayurvedic Antidementia Botanical Bacopa monniera, Phyther. Res., 2007, 21(10), 965–969, DOI:10.1002/ptr.2195.
- S. G. K. Muralidhara and M. M. S. Bharath, Exploring the Role of ‘Brahmi’ (Bacopa monnieri and Centella asiatica) in Brain Function and Therapy, Recent Pat. Endocr., Metab. Immune Drug Discovery, 2011, 5(1), 33–49, DOI:10.2174/187221411794351833.
- M. V. Kumar and Y. Gupta, Effect of Centella Asiatica on Cognition and Oxidative Stress in an Intracerebroventricular Streptozotocin Model of Alzheimer ’ S Disease in Rats, Clin. Exp. Pharmacol. Physiol., 2003, 30(5–6), 336–342 CrossRef CAS.
- J. Malik, M. Karan and K. Vasisht, Nootropic, anxiolytic and CNS-depressant studies on different plant sources of shankhpushpi, Pharm. Biol., 2011, 49(12), 1234–1242, DOI:10.3109/13880209.2011.584539.
- F. V. Defeudis, Bilobalide and neuroprotection, Pharmacol. Res., 2002, 46(6), 565–568, DOI:10.1016/S1043-6618(02)00233-5.
- B. H. Ali, G. Blunden, M. O. Tanira and A. Nemmar, Some phytochemical, pharmacological and toxicological properties of ginger (Zingiber officinale Roscoe): a review of recent research, Food Chem. Toxicol., 2008, 46(2), 409–420, DOI:10.1016/j.fct.2007.09.085.
- G. Oboh, A. O. Ademiluyi and A. J. Akinyemi, Inhibition of acetylcholinesterase activities and some pro-oxidant induced lipid peroxidation in rat brain by two varieties of ginger (Zingiber officinale), Exp. Toxicol. Pathol., 2012, 64(4), 315–319, DOI:10.1016/j.etp.2010.09.004.
- M. M. Essa, R. K. Vijayan, G. Castellano-Gonzalez, M. A. Memon, N. Braidy and G. J. Guillemin, Neuroprotective effect of natural products against Alzheimer's disease, Neurochem. Res., 2012, 37(9), 1829–1842, DOI:10.1007/s11064-012-0799-9.
- C. Borek, Recent Advances on the Nutritional Effects Associated with the Use of Garlic as a Supplement, 2001, vol. 6, pp. 972–976 Search PubMed.
- B. Ossola, T. M. Kääriäinen and P. T. Männistö, The multiple faces of quercetin in neuroprotection, Expert Opin. Drug Saf., 2009, 8(4), 397–409, DOI:10.1517/14740330903026944.
- M. Russo, C. Spagnuolo, I. Tedesco, S. Bilotto and G. L. Russo, The flavonoid quercetin in disease prevention and therapy: facts and fancies, Biochem. Pharmacol., 2012, 83(1), 6–15, DOI:10.1016/j.bcp.2011.08.010.
- S. C. Bischoff, Quercetin:
potentials in the prevention and therapy of disease, Curr. Opin. Clin. Nutr. Metab. Care, 2008, 11(6), 733–740, DOI:10.1097/MCO.0b013e32831394b8.
- N. Ahmad and D. K. Feyes, Green Tea Constituent Epigallocatechin-3-Gallate and Induction of Apoptosis and Cell Cycle Arrest in Human Carcinoma Cells Agarwal , Hasan Mukhtar * nolic compounds present in green tea Detection of Apoptosis by Quantification of Apoptosis by Flow Cytomet, J. Natl. Cancer Inst., 1881, 1881–1886 Search PubMed.
- F. Li, Q. Gong, H. Dong and J. Shi, Resveratrol, A Neuroprotective Supplement for Alzheimer's Disease, Curr. Pharm. Des., 2012, 18(1), 27–33, DOI:10.2174/138161212798919075.
- A. Kumar, P. S. Naidu, N. Seghal and S. S. V. Padi, Neuroprotective effects of resveratrol against intracerebroventricular colchicine-induced cognitive impairment and oxidative stress in rats, Pharmacology, 2007, 79(1), 17–26, DOI:10.1159/000097511.
- R. Salgia and P. Kulkarni, The Genetic/Non-genetic Duality of Drug ‘Resistance’ in Cancer, Trends Cancer, 2018, 4(2), 110–118, DOI:10.1016/j.trecan.2018.01.001.
- S. S. K. Durairajan, et al., Berberine ameliorates β-amyloid pathology, gliosis, and cognitive impairment in an Alzheimer's disease transgenic mouse model, Neurobiol. Aging, 2012, 33(12), 2903–2919, DOI:10.1016/j.neurobiolaging.2012.02.016.
- G. T. Ha, R. K. Wong and Y. Zhang, Huperzine a as potential treatment of Alzheimer's disease: an assessment on chemistry, pharmacology, and clinical studies, Chem. Biodiversity, 2011, 8(7), 1189–1204, DOI:10.1002/cbdv.201000269.
- Y. Wang, X. C. Tang and H. Y. Zhang, Huperzine a alleviates synaptic deficits and modulates amyloidogenic and nonamyloidogenic pathways in APPswe/PS1dE9 transgenic mice, J. Neurosci. Res., 2012, 90(2), 508–517, DOI:10.1002/jnr.22775.
- G. Seelinger, I. Merfort and C. M. Schempp, Anti-oxidant, anti-inflammatory and anti-allergic activities of luteolin, Planta Med., 2008, 74(14), 1667–1677, DOI:10.1055/s-0028-1088314.
- M. Petersen and M. S. J. Simmonds, Rosmarinic acid, Phytochemistry, 2003, 62(2), 121–125, DOI:10.1016/S0031-9422(02)00513-7.
- A. R. Esteves, A. M. Palma, R. Gomes, D. Santos, D. F. Silva and S. M. Cardoso, Acetylation as a major determinant to microtubule-dependent autophagy: Relevance to Alzheimer's and Parkinson disease pathology, Biochim. Biophys. Acta, Mol. Basis Dis., 2019, 1865(8), 2008–2023, DOI:10.1016/j.bbadis.2018.11.014.
- R. Cacabelos, et al., Sirtuins in alzheimer's disease: SIRT2-related genophenotypes and implications for pharmacoepigenetics, Int. J. Mol. Sci., 2019, 20(5) DOI:10.3390/ijms20051249.
- S. Moniot, M. Weyand and C. Steegborn, Structures, substrates, and regulators of mammalian Sirtuins – opportunities and challenges for drug development, Front. Pharmacol., 2012, 3, 1–5, DOI:10.3389/fphar.2012.00016.
- S. Moniot, M. Schutkowski and C. Steegborn, Crystal structure analysis of human Sirt2 and its ADP-ribose complex, J. Struct. Biol., May 2013, 182(2), 136–143, DOI:10.1016/j.jsb.2013.02.012.
- S. Michan and D. Sinclair, Sirtuins in mammals: insights into their biological function, Biochem. J., 2007, 404(1), 1–13, DOI:10.1042/BJ20070140.
- R. A. Frye, Phylogenetic classification of prokaryotic and eukaryotic Sir2-like proteins, Biochem. Biophys. Res. Commun., 2000, 273(2), 793–798, DOI:10.1006/bbrc.2000.3000.
- M. C. Haigis and D. A. Sinclair, Mammalian sirtuins: biological insights and disease relevance, Annu. Rev. Pathol.: Mech. Dis., 2010, 5, 253–295, DOI:10.1146/annurev.pathol.4.110807.092250.
- M. Gertz and C. Steegborn, Function and regulation of the mitochondrial Sirtuin isoform Sirt5 in Mammalia, Biochim. Biophys. Acta, Proteins Proteomics, 2010, 1804(8), 1658–1665, DOI:10.1016/j.bbapap.2009.09.011.
- E. L. Bell and L. Guarente, The SirT3 Divining Rod Points to Oxidative Stress, Mol. Cell, 2011, 42(5), 561–568, DOI:10.1016/j.molcel.2011.05.008.
- J. C. Black, A. Mosley, T. Kitada, M. Washburn and M. Carey, The SIRT2 Deacetylase Regulates Autoacetylation of p300, Mol. Cell, 2008, 32(3), 449–455, DOI:10.1016/j.molcel.2008.09.018.
- K. Y. Yeong, K. Y. Khaw, Y. Takahashi, Y. Itoh, V. Murugaiyah and T. Suzuki, Discovery of gamma-mangostin from Garcinia mangostana as a potent and selective natural SIRT2 inhibitor, Bioorg. Chem., 2020, 94, 103403, DOI:10.1016/j.bioorg.2019.103403.
- K. Zhao, X. Chai, A. Clements and R. Marmorstein, Structure and autoregulation of the yeast Hst2 homolog of Sir2, Nat. Struct. Biol., 2003, 10(10), 864–871, DOI:10.1038/nsb978.
- R. I. Tennen, E. Berber and K. F. Chua, Functional dissection of SIRT6: identification of domains that regulate histone deacetylase activity and chromatin localization, Mech. Ageing Dev., 2010, 131(3), 185–192, DOI:10.1016/j.mad.2010.01.006.
- M. S. Cosgrove, K. Bever, J. L. Avalos, S. Muhammad, X. Zhang and C. Wolberger, The structural basis of sirtuin substrate affinity, Biochemistry, 2006, 45(24), 7511–7521, DOI:10.1021/bi0526332.
- Y. Cen, Sirtuins inhibitors: the approach to affinity and selectivity, Biochim. Biophys. Acta, Proteins Proteomics, 2010, 1804(8), 1635–1644, DOI:10.1016/j.bbapap.2009.11.010.
- T. Karunakaran, G. C. L. Ee, I. S. Ismail, S. M. M. Nor and N. H. Zamakshshari, Acetyl- and O-alkyl-derivatives of β-mangostin from Garcinia mangostana and their anti-inflammatory activities, Nat. Prod. Res., 2018, 32(12), 1390–1394, DOI:10.1080/14786419.2017.1350666.
- J. Pedraza-Chaverri, N. Cárdenas-Rodríguez, M. Orozco-Ibarra and J. M. Pérez-Rojas, Medicinal properties of mangosteen (Garcinia mangostana), Food Chem. Toxicol., 2008, 46(10), 3227–3239, DOI:10.1016/j.fct.2008.07.024.
- H. M. Berman, et al., The protein data bank, Acta Crystallogr., Sect. D: Biol. Crystallogr., 2002, 58(6 I), 899–907, DOI:10.1107/S0907444902003451.
- M. D. Hanwell, D. E. Curtis, D. C. Lonie, T. Vandermeersch, E. Zurek and G. R. Hutchison, Avogadro: an advanced semantic chemical editor, visualization, and analysis platform, J. Cheminf., 2012, 4(17), 1–17, DOI:10.1016/j.aim.2014.05.019.
- E. F. Pettersen, et al., UCSF Chimera – a visualization system for exploratory research and analysis, J. Comput. Chem., 2004, 25(13), 1605–1612, DOI:10.1002/jcc.20084.
- M. M. V Windows and M. O. S. X, Molegro Molecular Viewer User Manual, 2011, p. 145 Search PubMed.
- M. Abdullahi, F. A. Olotu and M. E. Soliman, Allosteric inhibition abrogates dysregulated LFA-1 activation: structural insight into mechanisms of diminished immunologic disease, Comput. Biol. Chem., 2018, 73, 49–56, DOI:10.1016/j.compbiolchem.2018.02.002.
- M. Lawal, F. A. Olotu and M. E. S. Soliman, Across the blood-brain barrier: neurotherapeutic screening and characterization of naringenin as a novel CRMP-2 inhibitor in the treatment of Alzheimer's disease using bioinformatics and computational tools, Comput. Biol. Med., 2018, 98, 168–177, DOI:10.1016/j.compbiomed.2018.05.012.
- F. A. Olotu and M. E. S. Soliman, From mutational inactivation to aberrant gain-of-function: unraveling the structural basis of mutant p53 oncogenic transition, J. Cell. Biochem., 2018, 119(3), 2646–2652, DOI:10.1002/jcb.26430.
- O. S. Soremekun, F. A. Olotu, C. Agoni and M. E. S. Soliman, Drug promiscuity: exploring the polypharmacology potential of 1, 3, 6-trisubstituted 1, 4-diazepane-7-ones as an inhibitor of the ‘god father’ of immune checkpoint, Comput. Biol. Chem., 2019, 80, 433–440, DOI:10.1016/j.compbiolchem.2019.05.009.
- D. A. Case, et al., The Amber Molecular Dynamics Package, Amber, 2014, 14 Search PubMed , [online] available: http://ambermd.org/doc12/Amber14.pdf%0Ahttp://ambermd.org/.
- W. L. Jorgensen, J. Chandrasekhar, J. D. Madura, R. W. Impey and M. L. Klein, Comparison of simple potential functions for simulating liquid water, J. Chem. Phys., 1983, 79(2), 926–935, DOI:10.1063/1.445869.
- D. A. Case, et al., AmberTools12 Reference Manual, AMBER 12, Univ. California, San Fr., 2012, p. 535 Search PubMed.
- P. A. Kollman, et al., Calculating structures and free energies of complex molecules: combining molecular mechanics and continuum models, Acc. Chem. Res., 2000, 33(12), 889–897, DOI:10.1021/ar000033j.
- R. O. Kumi, O. S. Soremekun, A. R. Issahaku, C. Agoni, F. A. Olotu and M. E. S. Soliman, Exploring the ring potential of 2,4-diaminopyrimidine derivatives towards the identification of novel caspase-1 inhibitors in Alzheimer's disease therapy, J. Mol. Model, 2020, 26(4), 1–17, DOI:10.1007/s00894-020-4319-6.
- J. M. Villalba and F. J. Alcaín, Sirtuin activators and inhibitors, BioFactors, 2012, 38(5), 349–359, DOI:10.1002/biof.1032.
- C. Agoni, P. Ramharack and M. E. S. Soliman, Co-inhibition as a strategic therapeutic approach to overcome rifampin resistance in tuberculosis therapy: atomistic insights, Future Med. Chem., 2018, 10(14), 1665–1675, DOI:10.4155/fmc-2017-0197.
- O. S. Soremekun, F. A. Olotu, C. Agoni and M. E. S. Soliman, Drug promiscuity: exploring the polypharmacology potential of 1, 3, 6-trisubstituted 1, 4-diazepane-7-ones as an inhibitor of the ‘god father’ of immune checkpoint, Comput. Biol. Chem., 2019, 80, 433–440, DOI:10.1016/j.compbiolchem.2019.05.009.
- M. Z. Kamal, T. A. S. Mohammad, G. Krishnamoorthy and N. M. Rao, Role of active site rigidity in activity: Md simulation and fluorescence study on a lipase mutant, PLoS One, 2012, 7(4), 1–8, DOI:10.1371/journal.pone.0035188.
- S. Mukherjee and R. P. Bahadur, An account of solvent accessibility in protein-RNA recognition, Sci. Rep., 2018, 8(1) DOI:10.1038/s41598-018-28373-2.
- S. Genheden and U. Ryde, The MM/PBSA and MM/GBSA methods to estimate ligand-binding affinities, Expert Opin. Drug Discovery, 2015, 10(5), 449–461, DOI:10.1517/17460441.2015.1032936.
- J. Fang, et al., Inhibition of acetylcholinesterase by two genistein derivatives: kinetic analysis, molecular docking and molecular dynamics simulation, Acta Pharm. Sin. B, 2014, 4(6), 430–437, DOI:10.1016/j.apsb.2014.10.002.
- T. Z. B. Céline, M. Dubéa and A. L. Brewstera, Structural Basis for Sirtuin Function: What We Know and What We Don't, Biochim. Biophys. Acta, 2010, 1804(8), 1604–1616, DOI:10.1016/j.bbapap.2009.09.009.Structural.
- J. A. Baur, Z. Ungvari, R. K. Minor, D. G. Le Couteur and R. De Cabo, Are sirtuins viable targets for improving healthspan and lifespan?, Nat. Rev. Drug Discovery, 2012, 11(6), 443–461, DOI:10.1038/nrd3738.
- L. Chen, Medicinal Chemistry of Sirtuin Inhibitors, Curr. Med. Chem., 2011, 18(13), 1936–1946, DOI:10.2174/092986711795590057.
- V. Chopra, et al., The Sirtuin 2 Inhibitor AK-7 Is Neuroprotective in Huntington's Disease Mouse Models, Cell Rep., 2012, 2(6), 1492–1497, DOI:10.1016/j.celrep.2012.11.001.
- J. S. Disch, et al., Discovery of thieno[3,2-d]pyrimidine-6-carboxamides as potent inhibitors of SIRT1, SIRT2, and SIRT3, J. Med. Chem., 2013, 56(9), 3666–3679, DOI:10.1021/jm400204k.
- J. Du, et al., Sirt5 is a NAD-dependent protein lysine demalonylase and desuccinylase, Science, 2011, 334(6057), 806–809, DOI:10.1126/science.1207861.
- Z. Kurkcuoglu, A. Bakan, D. Kocaman, I. Bahar and P. Doruker, Coupling between Catalytic Loop Motions and Enzyme Global Dynamics, PLoS Comput. Biol., 2012, 8(9), 1–11, DOI:10.1371/journal.pcbi.1002705.
- M. S. Finnin, J. R. Donigian and N. P. Pavletich, Structure of the histone deacetylase SIRT2, Nat. Struct. Biol., 2001, 8(7), 621–625, DOI:10.1038/89668.
Footnote |
† Electronic supplementary information (ESI) available. See DOI: 10.1039/d0ra10459k |
|
This journal is © The Royal Society of Chemistry 2021 |
Click here to see how this site uses Cookies. View our privacy policy here.