DOI:
10.1039/D0RA10561A
(Paper)
RSC Adv., 2021,
11, 7750-7765
Demonstration of sweat-based circadian diagnostic capability of SLOCK using electrochemical detection modalities†
Received
15th December 2020
, Accepted 7th February 2021
First published on 17th February 2021
Abstract
SLOCK is a sweat-based circadian diagnostic platform used for mapping the user's chronobiology via cortisol and DHEA. In this work, we have demonstrated the detection capabilities of this sweat-based sensing platform using two electrochemical sensing modalities: Electrochemical Impedance Spectroscopy (EIS) and chronoamperometry. Wicking simulations for vertical versus horizontal flow patterns under potential bias were evaluated using COMSOL Multiphysics®. This work also highlights the biorecognition element characterization using Surface Plasmon Resonance (SPR) and FTIR. Sensor platform was evaluated for biomarker concentrations using doses spanning physiological ranges of 8–141 ng ml−1 and 2–131 ng ml−1 for cortisol and DHEA, respectively. Detailed analysis of impedance data is supported with electrochemical fitting of circuit components related to the biosensing process. Finally, human subject-based studies have been performed to understand the effect of sweating rate with respect to gland density on biosensing. Also, on-body mechanical resiliency studies have been performed to highlight the flexibility of this serpentine electrode-based sensing platform. The platform responds sensitively to the amount of circadian relevant biomarkers in the system with a limit of detection of 0.1 ng ml−1 for both cortisol and DHEA. Thus, the SLOCK platform offers to be an attractive vessel for facilitating the electrochemical detection of circadian relevant biomarkers and for self-monitoring of user's chronobiology.
1. Introduction
Sweat based biosensing offers a multifaceted approach towards revolutionizing healthcare. It provides improvements in diagnosis, self-management of chronic conditions, and early diagnosis or treatment of lifestyle disorders. Sweat hosts a panel of biomolecules like electrolytes, proteins, steroids, and nucleic acids, which holds powerful potential for detection of a diseased state in the body in a non-invasive manner. Blood offers to be the gold standard for quantification of biomarkers as it provides a direct assessment of the physiological concentrations at the source of production. However, it does have significant impediments when it comes to point of care monitoring, examples include being invasive or having a complex biochemical composition.1 Unlike blood, non-invasive biofluids are convenient samples for quantification of biomarkers. Biofluids like sweat, tears, saliva, and interstitial fluid (ISF) are some of these targets. Kim et al. report that these biofluids offer easy accessibility without affecting or damaging the epidermal integrity, reduce chances of infections, and offer to be user friendly.2 Out of the options for non-invasive biofluids, sweat is the front runner for offering diagnostic capabilities as point of care and point of need devices. Sweat based sensing offers to be an attractive solution for real time, minute by minute sensing for monitoring disease progression. Some of the features of this type of non-invasive sensing include ease of sample collection (especially for passive sampling) and having relatively simple biochemical composition, which makes it less susceptible for biomarkers to be degraded in sweat as compared to saliva and ISF. The biomarkers originate in either blood or ISF and are filtered through capillaries into the epidermal matrix. Biomarkers have two main entry points while filtering from blood/ISF to sweat, first the transcellular pathway i.e. through the cells via the lipid bilayer and second, the paracellular pathway i.e. in between the cells via the tight junctions. This is one of the reasons for the low biomarker concentration in sweat when compared to blood.3 Few biomarkers that are of prime importance to the work presented here are adrenal steroids like cortisol and DHEA. These circadian relevant biomarkers are lipid based and are transported transcellularly into the sweat gland coil. This transport makes sure that the concentrations are not further diluted and enables easy detection via secreted sweat.
Optimizing the sampling of sweat for detection has significant importance while designing sweat-based detection technologies. Sweat composition is highly correlated with the position and type of production (eccrine versus apocrine), this in turn affects the accuracy and reliability of detection. Traditional sampling methods involve sampling the sweat separately and then using it for detection, which cannot satisfy the needs of real time in situ monitoring.4 There are several issues such as sample evaporation, degradation, and contamination. The most common traditional method of sampling sweat are sweat patches. These patches are porous, hydrophilic and are attached to the skin surface using an adhesive layer. They are convenient, light weight, flexible, economical and do not require specialized lab equipment or skilled personnel while performing the collection. However, the surface of these patches are prone to contamination from outer skin surface, susceptible to high volume loss,5 and prone to hydromeiosis effect that might reduce the amount of sweat collected.6 Macroducts are another widely used sampling devices for commercial collection of sweat and performing analyte detection. Typically, macroducts require stimulation of sweat via a process known as iontophoresis. During iontophoresis, a sweat stimulating cholinergic drug i.e. pilocarpine is applied to the skin surface and a small current (1.5 mA) is passed through the epidermis for a period of 5–7 minutes. Following this, a microfluidic collector is applied and the sweat in the coiled tubes of the collector is extracted for analysis.7 This technique is FDA approved and is commercially used for the diagnosis of cystic fibrosis. When compared to the patch method of collection, they solve the problem by avoiding sample loss, evaporation and potential hydromeiosis. They do provide the ability to perform dynamic detection of biomarkers to an extent. However, due to the stimulation required to generate a sample, it might degrade the structure of target molecule during sample collection and affect the stratum corneum layer of epidermis. This might also affect subsequent sampling from that region and create local inflammation. Moreover, they create local irritation at the site of collection and might create false spikes in biomarker, especially for biomarkers concerned with stress and anxiety in the body. Also, the procedure is tedious, requires a trained professional, and gives insufficient volumes for multiple analyses. Generally, sampling sweat traditionally has a delay between sampling and detection which leads to loss of crucial real time data while performing time sensitive monitoring of sweat components.8 A solution to this is integrating sampling with detection which is addressed in this work by incorporating a passive sweat based detection platform. Passive sampling has significant advantages like the ability to work with low sample volumes, reduced susceptibility of sample degradation, and easy sample collection. This work presents an extensive analysis of sweat gland density-based rate of sweat secretion and optimal sensor placement to provide maximum passive sweat sample collection. This paper also evaluates the effect of flow direction on transport with respect to the pore distribution of the employed nanoporous membrane to optimize for wicking.
As discussed earlier, the process of filtration of biomarkers from blood to sweat is responsible for dilution of the biomarker amount secreted via sweat. This poses a challenge for biomarker quantification during passive sampling.1 This work addresses the challenge by incorporating electrochemical detection modalities to amplify the sensing response and provide enhanced sensitivity with lower detection limits. Electrochemical detection offers the advantage of performing rapid and label-free detection. This makes them ideal detection modalities for designing point-of-need self-monitoring platforms. Electrochemical Impedance Spectroscopy (EIS) is a powerful tool for developing label free assays for molecular diagnostics and disease monitoring applications. Furthermore, electrochemical sensors increase the robustness and facilitate integration with portable electronics.2 Also, transition to flexible porous substrates, like the one presented in this work, increases the mechanical resiliency for prolonged on-body sensing.9 The nanoporous membrane also performs effective entrapment of target biomarker and provides enhanced interaction with the biorecognition element. Thus, increasing overall sensitivity of the platform.10
The work presents a comprehensive characterization of the SLOCK platform and demonstrates the circadian diagnostic capabilities using EIS and chronoamperometry. This work builds on the previously published work on the SLOCK platform.11,12 In this work, we provide additional in-depth characterization of the electrochemical sensing capabilities of this biosensor and evaluate its feasibility as a wearable self-monitoring platform, which is distinct from the previously published work. This work offers a detailed combination of benchtop study and on-body study to understand the sensitivity of SLOCK for detecting cortisol and DHEA in human sweat. This work also uses Surface Plasmon Resonance (SPR) and Fourier Transform Infrared Spectroscopy (FTIR) to characterize the biorecognition element and the binding phenomena associated. Previously, the SLOCK platform was characterized using benchtop mechanical bending studies.12 In this work, we evaluate the effect of on-body, wearable form factor generated mechanical oscillations on the sensor response in order to test the mechanical resiliency of this serpentine-electrode based platform. Analysis using two different electrochemical techniques sets the stage for incorporating this platform with portable electronics towards wearable biosensing. Additionally, the sweat rate and gland density characterization explores the location-based sampling efficiency for this passive-sweating based sensor. Finally, a circuit model based electrochemical fitting analysis of the response is provided for an in-depth understanding of the biomarker concentration dependent sensitivity of this circadian monitoring platform.
2. Results and discussion
2.1. Sensor surface characterization
Scanning electron micrograph of the nanoporous membrane is depicted in Fig. 1a. This highlights the porous network of the polyamide fibers used to wick sweat on to the electrode surface. Fig. 1b is the processed binary image for understanding the effective pore-strand ratio for estimation of porosity and computing the wicking pattern. Based on the protocol described by Feng et al.13 the image processing protocol involves cropping the image, adjusting the pixel intensity, filtering using an averaging filter to smoothen out the image, followed by a binary conversion. This processing offers an enhanced view of the SEM micrograph and is able to extract the fiber versus pore density. This pore density characterization was performed with the aid of ImageJ software. This rough estimate of pore density, which was calculated to be 0.8 (80%), was used as an input for the COMSOL simulations that were carried out to further map the wicking profiles.
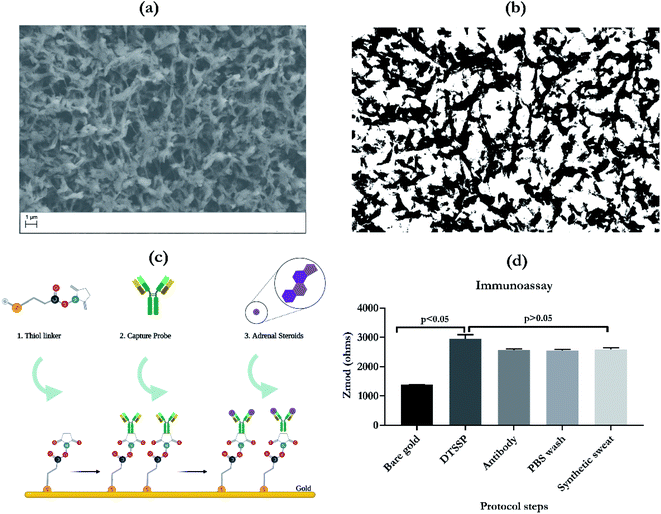 |
| Fig. 1 Sensor characterization: (a) scanning electron micrograph of nanoporous polyamide membrane; (b) processed binary image of nanoporous PA membrane, highlighting the porosity (ImageJ); (c) schematic for functionalization and immunoassay for sensing platform; (d) impedance based baseline characterization of functionalization of sensor surface (partially created with BioRender.com). | |
The process of functionalization of the bio-recognition element i.e. capture probe is highlighted in Fig. 1c. The principle of detection for this work is based on affinity between the target biomarker and the antibody (capture probe). In this process, gold–thiol chemical affinity is exploited to immobilize the capture probe on the electrode surface. This capture probe is an IgG antibody specific for the target biomarker of interest. Once the surface of the gold electrode has been functionalized, the platform is tested using the samples which are spiked with target biomarkers, cortisol and DHEA. In this work, the SLOCK platform functionalization was further characterized using EIS, as illustrated in Fig. 1d. The Zmod response over the different assay steps for functionalization was recorded. From the figure, it can be observed from the raw impedance values that there is a significant change in Zmod, going from bare gold to DTSSP immobilization with a p value < 0.05. DTSSP is the water-soluble form of the thiol linker used to immobilize the antibody. Following the immobilization of the thiol linker, the antibody was incubated on the surface. This is confirmed by the drop in impedance response going from DTSSP to antibody. Following this PBS wash and synthetic sweat wash was applied to the surface to remove the unbound or physisorbed molecules present on the surface. For the steps following the DTSSP step, no significant change was observed in the impedance, p > 0.05. This highlights the stability of the functionalized platform and that it can be further used for testing using target biomarkers.
2.2. Wicking simulations using COMSOL Multiphysics
Understanding the wicking patterns of the substrate used for sweat-based applications is important to the design process for wearable circadian diagnostics. Substrates with nanoporosity offer significant advantages for improving sensing performance. They offer signal enhancement by processes like size-based exclusion, nanoconfinement, and macromolecular crowding.14 This work uses finite element analysis for understanding the effect of flow directionality in the presence of an electrical field. The circadian sensing platform uses electrochemical detection modality to detect the target biomarker, cortisol or DHEA in a sweat sample. Being aqueous in nature, the sweat sample rapidly wicks through the sensing area of the electrode on the hydrophilic surface of the nanoporous membrane. The process of transport across the surface of this membrane is driven by capillary transport or capillary imbibition. The relationship of the flow in the nanopores is akin to the flow in hollow tubes.15 The Lucas–Washburn law best describes the relationship between the liquid front distance and diffusive coefficient for transport in porous media. The ‘transport of dilute species in porous media (tds)’ module in COMSOL was used to understand the transport by applying these laws. Transport of a concentrated species going from 0 to 1 mol m−3 was evaluated over the period of 0 to 100 s. This is presented in Fig. 2. Horizontal flow has been presented in Fig. 2a and b. The electrode geometry was imported via the CAD import module and the polyamide nanoporous properties and porosity calculated from Fig. 1b were used as inputs for the substrate. The schematic of the applied potential is presented in the ESI as Fig. S1.† The working electrode is set at 10 mV against the reference electrode. The black streamlined horizontal lines highlight the direction of flow of the concentrated species. The color-coded bar on the right side of the graphs indicates the concentration of the species. As observed from Fig. 2b, the surface concentration is highest near the electrode specific area of the sensor. Similarly, Fig. 2c and d demonstrate the vertical flow profile of the concentrated species under biased electrode conditions. For the time frame between 0 to 100 seconds, it can be observed that the concentration profile spreads linearly from top to the bottom of the graph, with maximum concentration close to the flow origin region of the sensing surface. From these simulations, it can be inferred that for the wicking transport, horizontal wicking is faster than vertical wicking transport, especially in the presence of electrochemically biased electrode conditions. For the process of detection, the immobilization process is specific to the electrode area, which is called the sensing region. The sweat sample is loaded on to the working area of the sensing surface and the change in response to the binding of target biomarker to the immobilized capture probe is recorded against the reference electrode. These simulations highlight the optimal patterns of fluid loading on the sensor surface to ensure maximum surface reactivity is captured. Rapid lateral wicking also ensures that the sensor can work with ultra-low volumes of fluid. The current system works with volumes of 10 μl, which can easily be collected by passive sampling. The directionality of wicking also gives an idea about the positionality of electrodes before fabrication to ensure rapid wicking of sample takes place. Passive sampling of sweat ensures that there is no artifact of stress due to sampling observed in the biomarker levels, especially for cortisol. These biomarkers are susceptible to undergo a ‘false’ rise as they are associated with the homeostasis pathway after the body is exposed to stress. With passive sampling strategy, the subject does not have to take additional efforts towards generating a sample that might be a stressful task in some cases. Also, this highlights the advantage of passive sweat based sensing over traditional gold standard methods like blood tests, as there is no need for pricking the skin surface or even the need of skilled professionals to perform the task of sample procurement.
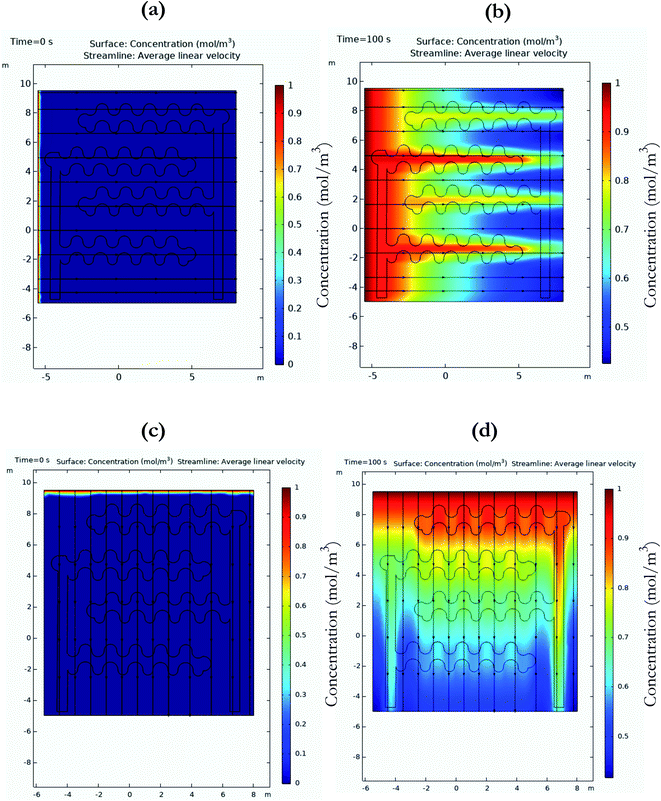 |
| Fig. 2 COMSOL Multiphysics simulations: horizontal wicking simulation for transport of species from 0 to 1 mol m−3 concentration (a) time period = 0 second, (b) time period: 100 seconds; vertical wicking simulations for transport of charged species for (c) time period = 0 second and (d) time period: 100 seconds. | |
2.3. Sensor binding chemistry characterization
2.3.1. Binding characterization using surface plasmon resonance (SPR). Surface plasmon resonance (SPR) is an optical technique used for the real time analysis of biochemical interactions with high sensitivity. The main sensing system of the instrument has a combination of a sensor, microfluidic channels, and a transducer. The transducer outputs the refractive index related signal based on the input received from the immobilized surface chemistry on the sensor surface. The biorecognition element in the SLOCK biosensing platform is a key component of the detection system. This element contributes to the specificity part of the biosensor. Generally, the biorecognition element can be either enzymes, antibodies, apatamers or proteins. The SLOCK platform employs an IgG antibody as the biorecognition element that is highly specific for the target circadian relevant biomarker. Previously the binding chemistry was characterized using Fourier transform infrared spectroscopy (FTIR) for immobilization on gold sensing surface using a thiol chemistry.16,17 The structure of a typical antibody is presented in the ESI Fig. S2.† The process of immobilization on the sensor surface is that the Fc region of the antibody anchors onto the gold surface, exposing the antigen binding site upwards for offering binding sites for biomarker detection. Thus, it is imperative to characterize the Fc region of the capture probe to make sure the detection is functioning with maximum sensitivity. A schematic of the process of detection has been presented in Fig. 3a. Detailed assay protocol has been presented in the materials Section 3.4. The process utilizes carboxyl sensors and the protein A immobilization chemistry for characterization of the antibody binding. Fig. 3b corresponds to the optical signal obtained from the assay for each of the steps displayed in the schematic above. The sensor surface was initially cleaned using a 10 mM HCl solution. The depression in the signal at the HCl step in Fig. 3b highlights the surface cleaning process. Following the surface cleaning, EDC–NHS chemistry was utilized to prepare the surface. This step was observed as a bump-plateau signal response. This bump-plateau signal indicates the process of association, dissociation, and stabilization. The stabilization was observed by the signal flat lining after immobilization. Amine coupling is a commonly used surface functionalization process. The reaction of introducing the EDC/NHS combination on the carboxyl sensor surface generates succinimide esters. EDC along with NHS are highly specific for the carboxyl surface and form an NHS ester that allows for conjugation of primary amines at physiological pH.18 For characterization of the biorecognition element, protein A coupling chemistry was utilized. Protein A is a cell wall component of Staphylococcus aureus and specifically binds to the Fc region of the antibody.19 This immobilization step can be observed in Fig. 3b as a peak in signal for both channels 1 and 2. This confirmed successful immobilization of protein A on the SPR sensor surface. Following this a blocking agent that consists of a bovine serum albumin based solution was used to block the non-specific binding regions. The IgG antibody, which is the biorecognition element of interest for the biosensing platform was immobilized only in channel 2. This is illustrated by the increase in signal to 6000 RU with the antibody binding to the protein A molecule. This assay confirms that the Fc region of the antibody is active, and it can be used as a biorecognition element to perform biomarker detection.
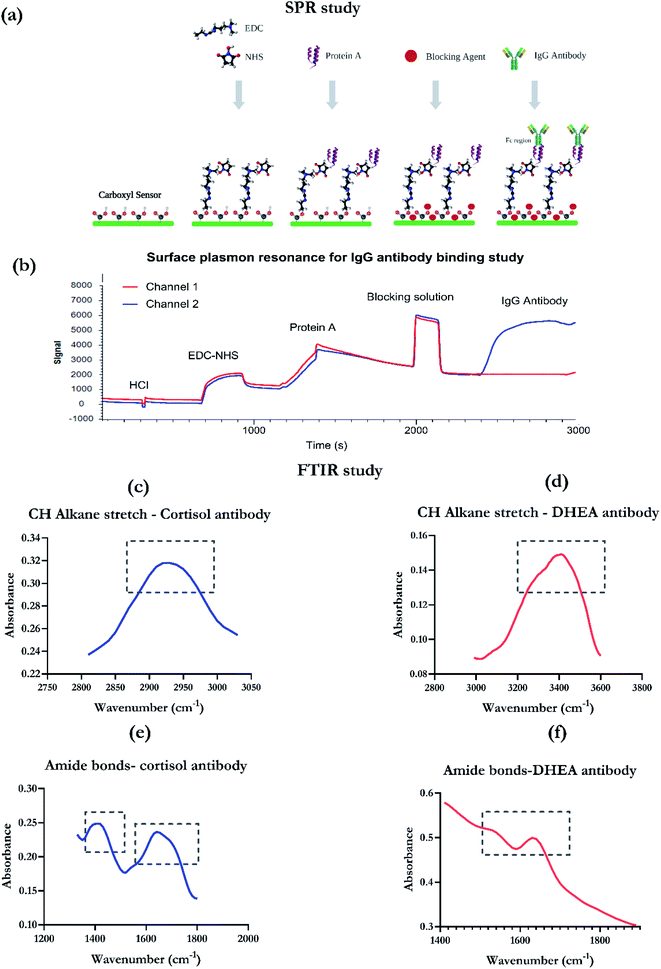 |
| Fig. 3 Surface plasmon resonance characterization: (a) schematic depicting the different steps for characterization of biorecognition element-IgG antibody, (b) SPR signal for sensor functionalization and characterization of IgG antibody binding; FTIR characterization: CH-alkane stretch for (c) cortisol antibody and (d) DHEA antibody, amide bond relevant peaks for (e) cortisol antibody and (f) DHEA antibody (partially created with BioRender.com). | |
2.3.2. Binding characterization using Fourier transform infrared spectroscopy (FTIR). Fourier transform infrared spectroscopy was used to characterize the binding chemistry between the gold functionalized substrate and the biorecognition probe. The probes used were cortisol specific antibody for the cortisol sensor and DHEA specific antibody for the DHEA sensor. Table 1 summarizes the different peaks associated with the FTIR spectrum of cortisol and DHEA. Thiol–gold affinity was exploited for anchoring the antibody on the gold microelectrode surface. Fig. 3c–f highlights the FTIR peaks relevant to the immobilized molecules. The peak illustrated in Fig. 3c and d between 3000–2600 cm−1 is representative of the CH alkane stretch for the thiol linker used to immobilize the antibody on the gold surface.20 Protein immobilization can be observed by the amide bond relevant peaks that appear in the 1650–1400 cm−1 range. Fig. 3e highlights the amide I (peak between 1600–1800 cm−1) and amide II (peak between 1500–1400 cm−1). The peaks are due to the secondary structure of the protein, which is the bio recognition probe for the cortisol sensor. Similarly, for DHEA the amide I and II bonds are highlighted by the peaks between the 1650 and 1400 cm−1 range. This characterization for interaction of the capture probe with the electrode platform confirms the successful functionalization and sensitivity of the capture probe for the sensing platform.
Table 1 FTIR peaks related to functionalization
Functional group |
Peak for cortisol antibody |
Peak for DHEA antibody |
CH alkane stretch of thiol linker |
3000–2900 cm−1 |
3200–2500 cm−1 |
Amide I bond (antibody specific) |
1600–1800 cm−1 |
1600–1700 cm−1 |
Amide II bond (antibody specific) |
1500–1400 cm−1 |
1500–1600 cm−1 |
2.4. Sensor response characterization using electrochemical detection modalities
2.4.1. Decoding the EIS and chronoamperometric response. EIS and chronoamperometry are the widely used electrochemical detection modalities for biosensing. Fig. 4a and b offer a comprehensive summary of the two techniques using an electrode–electrolyte sensing model. This section explores the nuances of the two electrochemical sensing modalities and decodes the sensitivity of each technique with respect to sweat-based circadian diagnostics. EIS has an input sinusoidal AC voltage and the output is a frequency dependent impedance response. The two main output response graphs are the Nyquist and Bode plots. Nyquist plot includes the imaginary versus real impedance component, whereas Bode plot includes the magnitude and phase change of Zmod (modulus of impedance) against frequency. Fig. 4a describes the electrode–electrolyte interface for non-faradaic EIS detection. With the application of potential bias to the sensing system, there is an electrical double layer (EDL) formation which is highlighted by the active stage of the Fig. 4a. The capacitive double layer can be mapped using Randle's equivalent circuit (depicted in Fig. S4†). The different components of the circuit are EDL capacitance (Cdl), resistance to charge transfer (Rct), and solution resistance (Rs). The first two components are direct responses of the capacitive modulations occurring at the EDL interface due to the binding between the capture probe and target biomarker. The latter maps to the bulk solution effects of the buffer that the target biomarkers are suspended in. For non-faradaic EIS response, the principle of detection involves the re-arrangement of ions with the binding between the target molecule and the immobilized capture probe.21–24 Table 2 offers the advantages and disadvantages of using EIS as a technique for biosensing.
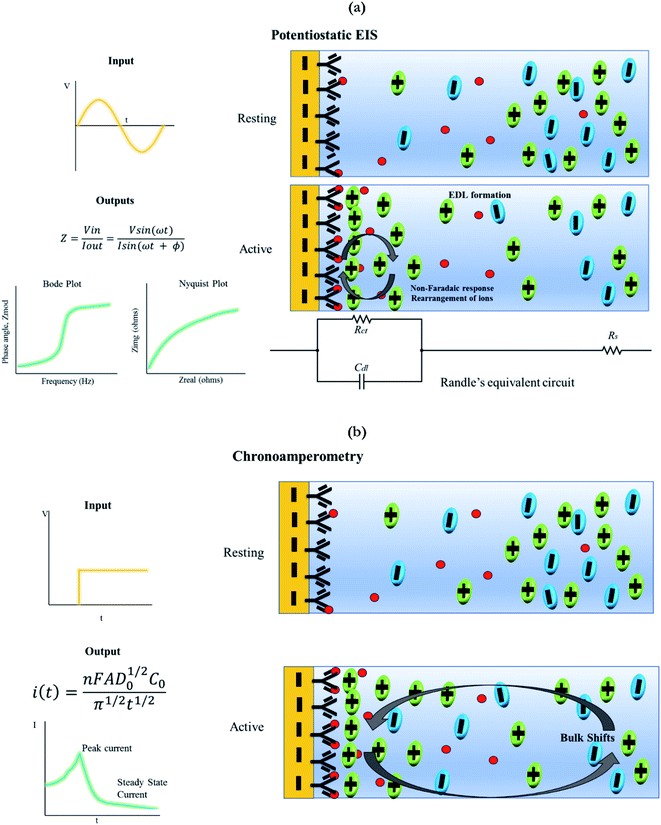 |
| Fig. 4 Electrochemical technique schematic: (a) schematic highlighting the input, output and process occurring at the electrode–electrolyte interface in an Electrochemical Impedance Spectroscopy (EIS) study, Randle's equivalent circuit components: Rs – solution resistance, Rct – charge transfer resistance, and Cdl (capacitance of electrical double layer); (b) schematic describing the input, output curves, explained with Cottrell equation, and process at the electrode–electrolyte interface for a typical chronoamperometry experiment. | |
Table 2 Features of EIS detection modality
Advantages |
Disadvantages |
Can isolate bulk solution effects from EDL related binding effects |
Technique is laborious |
Real time sensing, SF EIS provides time dependent changes in capacitive modulations of the EDL in response to target molecule binding to capture probe |
Post processing is needed |
Easy coupling to portable electronics |
Requires reaction to be at a steady state, which might take some time. Also, selecting optimal frequency is crucial before performing SF EIS, which requires additional experiments |
Chronoamperometry is a DC based technique which inputs a step DC potential and the output of current versus time is recorded. On excitation with the step potential, there is a spike in the current response, followed by an exponential decay. The peak current is extracted to characterize the dose dependent response of the system.22 This study involves mapping the rate of change in current with respect to an applied input step potential. The analysis of this data offers insights into many electrochemical processes, such as creation of new species in a solution, identification of adsorption or diffusion.25 Like non-faradaic EIS, this technique also does not require the use of a labelling probe. Fig. 4b describes the chronoamperometric response. As discussed earlier, the input for this technique includes a step potential and the output includes mapping the current response. The output current response has two components, the peak current in response to the applied step potential and the exponential decay to a steady state current value.21 This current response is governed by the equation highlighted in schematic, also known as the Cottrell equation. The principle of detection is that with the induction of a step potential bias and after optimal incubation time, the current associated with the electrode capacitance is proportional to the concentration of analyte present in the system.26 The schematic depicts two stages of the functionalized electrode system. Going from rest to active stage of measurement, there is charging and discharging of this capacitive layer which leads to bulk shifts in charges of the electrolyte from the bulk solution to the electrode–electrolyte interface, which are mainly diffusion driven.27 Some of the key advantages and disadvantages of using chronoamperometry are highlighted in Table 3.14,26
Table 3 Features of chronoamperometric detection modality
Advantages |
Disadvantages |
Simplicity in data procurement, good for biosensing |
Bulk solution effects are observed in sensing response and cannot be isolated |
Real time sensing |
Problems with selectivity of sensing response |
Easy coupling to wearable electronics |
Solutions that have a complex constitution are prone to issues with high background noise while detection of a target molecule |
Electrochemical detection modalities have significant advantages over commercially used optical diagnostic tests. Features like rapid response rates, easy coupling to microprocessors, lower detection limits, wider dynamic ranges, enhanced sensitivity, ability to miniaturize sensing platforms, easy fabrication, cost-effectiveness, and user-friendly nature, make electrochemical sensors highly desirable for building biosensing platforms.9,28,29 In the following section, investigation into mapping the dose dependent response of the SLOCK sensing platform using these two electrochemical detection techniques has been carried out.
2.4.2. Sensor response for cortisol. Single frequency EIS (SFEIS) is used for analyzing the antigen–antibody binding and the kinetics behind it. The frequency was fixed at 15 Hz, which was the point in the Bode plot,12 where maximum capacitive behavior of the sensor was observed. Fig. 5a illustrates the time dependent impedance response change represented as change in Zmod from blank dose and the respective phase angle changes. The dose range tested was between 0.1 ng ml−1 to 500 ng ml−1. From the figure, it can be observed that with the increase in dose concentrations, the impedance response (blue curve) increases proportionally. This is supported by the phase angle values (red curve) becoming increasingly capacitive with each dose concentration. This response highlights the capacitive modulations causing the change in response due to the binding between the target molecule i.e. cortisol and the capture probe i.e. immobilized antibody. The non-faradaic EIS response does not involve a redox labelling probe and is solely dependent on the changes in the interfacial charge modulations occurring when a target binds to the immobilized antibody.30 With single frequency EIS, real-time biomarker mapping via sensor response can be observed. This is of prime importance while designing circadian diagnostic devices that map the user's chronobiology over a specific time-period. For cortisol, the sensing response shows a step change in impedance with the increase in concentration from 0.1–500 ng ml−1. The values are represented as average of N = 3, with inter-assay variation of <20%. The single frequency EIS is reproducible from chip to chip with a dose response standard deviation < 10% and SEM < 5%. This sensitivity parameters are within the assay validation metrics set by the Clinical Lab Standards Institute (CLSI).31
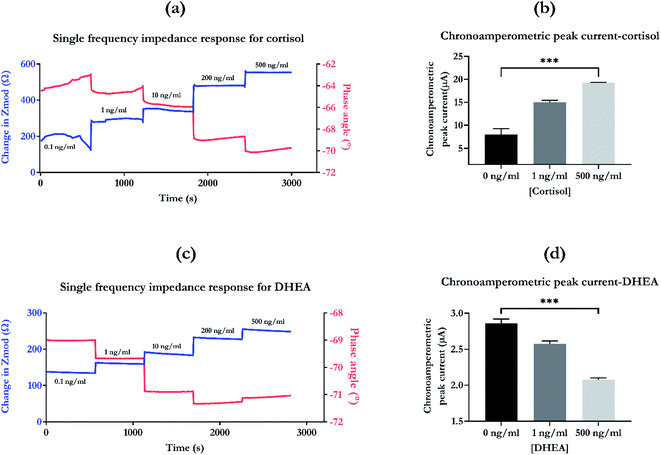 |
| Fig. 5 Sensor response for biomarkers: (a) single frequency EIS response for cortisol; (b) chronoamperometric peak current response for cortisol; (c) single frequency EIS response for DHEA; (d) chronoamperometric peak current response for DHEA. | |
Chronoamperometry was used to test the SLOCK platform's sensitivity to target biomarker. The peak current in response to the step potential have been extracted for baseline (0 ng ml−1), low dose (1 ng ml−1), and high dose (500 ng ml−1). The chronoamperometric dose dependent peak current response for cortisol is illustrated in Fig. 5b. There is an increase in current going from 0 to 1 ng ml−1, and from 1 ng ml−1 to 500 ng ml−1. There is a highly significant change between the baseline, the low and high levels of biomarkers with a p value of 0.001. Chronoamperometric response maps the bulk effects of charge modulations in the solution due to binding between the target and capture probe. For cortisol, it confirms the sensitivity of the SLOCK platform to the changes in cortisol concentration. The difference between the chronoamperometric response and the SFEIS based response is that diffusion effects are predominantly present in the former technique. However, using both SFEIS, an AC input-based technique and chronoamperometry, a DC based technique, provides a thorough analysis and validates the biomarker concentration dependent response of the sensing platform. It removes the bias that the detection modality might interfere and create a false shift in response, which might be inaccurately interpreted as a concentration dependent response.
In addition to this cross-reactivity studies capture the specificity of the sensing platform. Fig. S3a† highlights the sensor response for cortisol and other cross-reactive molecules. From this graph it can be inferred that the sensor response for the specific molecule (cortisol) is significantly higher than the signal for DHEA (non-specific) and prednisone (non-specific) with a p value < 0.05. This confirms the sensitivity and specificity of the sensing platform.
2.4.3. Sensor response for DHEA. DHEA sensitivity of the SLOCK platform was evaluated in a similar manner with SFEIS and chronoamperometry detection modalities. This is illustrated in Fig. 5c and d. It can be observed from the plots that with the addition of DHEA in the system, there is an increase in the impedance response from baseline. This testing is carried over the concentration range of 0.1 ng ml−1 to 500 ng ml−1, which encompasses the physiologically relevant range of DHEA i.e. 2–131 ng ml−1.11 Capacitive EDL modulations occurring due to binding are the key elements responsible for this characteristic dose-dependent response of the sensing platform. This is also confirmed by the shift in phase angle towards −90° with the addition of DHEA in the sensing system. This temporal variation helps identify the real-time, continuous sensing capabilities for the SLOCK platform. The change in impedance is presented as an average of N = 3 with <20% intra-assay variation.Chronoamperometric peak currents that were extracted for baseline (0 ng ml−1), low (1 ng ml−1) and high dose (500 ng ml−1) of DHEA are presented in Fig. 5d. The recorded response decreases with the increase in DHEA concentration. The sensing platform has the ability to significantly distinguish between baseline, low and high levels of DHEA with a p value of 0.001. When compared to cortisol, the response has an opposite trend for the chronoamperometric response. This phenomenon can be explained with the overall binding mechanism difference and hence, the charge distribution difference between the two molecules. Previously, we evaluated the zeta potentials for cortisol and DHEA assays for low and high concentrations of biomarkers.11 For cortisol, the zeta potential values going from 1 to 100 ng ml−1 changed from −7.32 ± 0.11 mV to −4.81 ± 0.11 mV. This is contributed by the overall surface charge of cortisol, which is neutral. Hence, the chronoamperometric bias draws out the response based on the input step potential applied and there is low contribution of charge from the actual molecule itself. However, for DHEA assay, the zeta potential going from 1 to 100 ng changes from −4.92 ± 0.28 mV to −6.07 ± 0.61 mV, increasing in magnitude. This highlights the difference in the molecular binding between the two molecules and their capture probe. DHEA molecules using detection probes are more susceptible to hyper-crowding, leading to the decrease in overall chronoamperometric peak current as observed in Fig. 5d. This also highlights the ability of EIS to capture the specific electrode–electrolyte interfacial changes and separate them from the bulk changes, making it a superior technique for performing biosensing.
In addition to this, the cross-reactivity of the DHEA platform was evaluated and is illustrated in Fig. S3b.† The results highlight the specificity of the platform for DHEA where the response is highly significant (p < 0.01) than cortisol (non-specific) and prednisone (non-specific). This confirms the sensitivity of the sensing platform for the target biomarker for the respective immobilized biorecognition probe.
2.5. Sweat rate characterization study
Humans have approximately 2–5 million eccrine sweat glands distributed all over the body with an average of 15–340 per cm2 of skin surface area. The primary function of these glands is to perform thermal regulation after exposure to hot temperatures or during physical exercise. Eccrine glands are classified as both secretory and excretory organ.32 The glands develop before birth and are functional throughout the lifetime of an individual. They are densely populated on the palms and soles with ranges of 200–600 glands per cm2 and 200–400 per cm2, respectively. A schematic of the various gland densities on the different anatomical regions is presented in Fig. 6a.32–34 Axillary area (underarm) has a combination of eccrine and apocrine glands at a ratio of 1
:
1.35 Forearms and back have densities of 40–150 per cm2 and 31–121 per cm2, respectively. Ankles have lower densities as compared to the other sites with ranges of 20–70 gland per cm2. Based on the pattern of secretion and the composition of the sweat secreted, sweat glands are categorized into three types namely, eccrine, apocrine and apoeccrine glands.32,36 The schematic, Fig. 6a also highlights the distribution of eccrine glands, depicted by blue and apocrine glands, depicted by the red circles. Circadian relevant biomarkers cortisol and DHEA are secreted in eccrine and apocrine sweat respectively, which is the reason for increased interest in capturing the sweating pattern from the chosen locations presented in Fig. 6a. While designing sweat based wearable, these sweat gland densities need to be accounted for to optimize and maximize the sampled volume. This is crucial for a passive sweat based platform as capturing higher gland density per area of the sensor is important. Wearable biosensing can fundamentally improve by understanding the physiology and functioning of the eccrine sweat gland. It is also crucial for characterizing the flow rate dependant response for biomarkers. Understanding the sweat secretion patterns also holds the key to designing platforms that are unique to the detection of a specific target biomarker and for understanding their correlations to blood and plasma biomarker levels.37
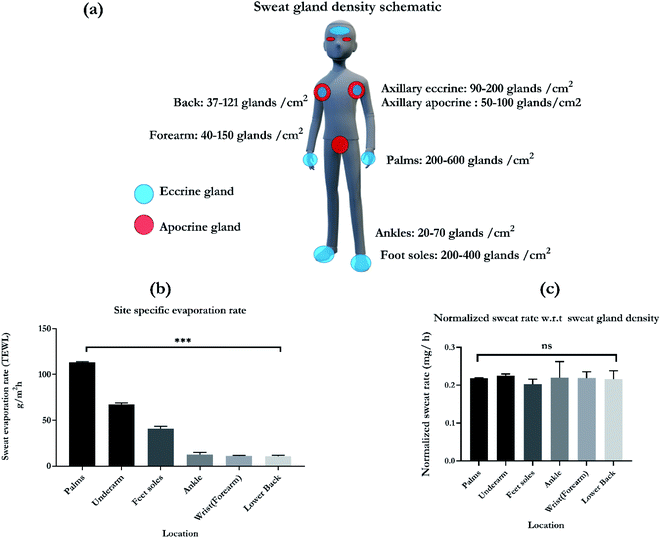 |
| Fig. 6 Sweat rate characterization: (a) sweat gland density schematic highlighting the location based gland density and the distribution of eccrine versus apocrine glands; (b) transepidermal water loss (TEWL) from skin surface to characterize sweat rate for different locations of the body; (c) normalized sweat rate with sweat gland density for optimal placement. | |
Gland counting methods are susceptible to large variations. This is because the anatomical number of sweat glands can exceed the number of functional or physiologically active glands.34 Surface evaporation rates from the sites of interest can help estimate the sample volumes that would be collected with passive sampling. This work presents the characterization of skin surface evaporation rates with the measurement of transepidermal water loss (TEWL; g m−2 h−1). This indirect albeit quantitative measure of sweating indicates the amount of water that evaporated from the skin surface during a fixed period of time.38 The readings are measured using a hand-held VapoMeter (Delfin Technologies Ltd, Finland) that assesses the surface evaporation rate within 10 seconds. Fig. 6b depicts the various sweating rate values for widely preferred locations for sweat based sensors. From the TEWL values, it can be observed that palms (113 ± 0.6 g m−2 h−1), underarms (axillary region, 67.5 ± 1.5 g m−2 h−1), and soles (40.66 ± 2.6 g m−2 h−1) have the highest rates of evaporation. This is in corroboration with the gland density values found at those locations. Followed by this the evaporation rates for ankles, wrist, and lower back are 12.5 ± 2.4 g m−2 h−1, 11 ± 0.8 g m−2 h−1, and 10.8 ± 1.1 g m−2 h−1, respectively. The sweating rates significantly change depending on the different locations of the skin surface, with a p value of 0.001. Upon normalization with the sweat gland density, the sweating rates show no significant difference, which is illustrated by Fig. 6c. This indicates that the wearable systems can be tuned to capture the gland density based sweating evaporation rate in order to compensate for a convenient sampling location on the body. For passive sampling, the sensor design can be optimized to maximize coverage of gland density to collect sufficient samples of sweat. At the passive state of the body with a normal sweating rate, the body produces 5–10 nL per gland per cm2.37 For sampling, this amounts to volumes of 5–10 μl as the working volume. The SLOCK sensing platform is designed using a combination of macroscale and nanoscale porosity and is calibrated to work with 10 μl of sample volume. Circadian relevant biomarkers like cortisol and DHEA are passively secreted and are speculated to be independent of sweating rate.37 Thus, understanding the rate and dynamics of sweating rate can be isolated from the biomarker concentration fluctuations and can be studied solely from a collection or sampling methodology perspective. Having low sampling volumes can also allow for sensing of biomarkers with good correlations to the blood/serum levels. Also, they can be conveniently and ergonomically adopted as self-monitoring tools. This is especially important for circadian tracking systems like SLOCK that is designed to capture the diurnal fluctuations of cortisol and DHEA for understanding the circadian phase of the individual.
2.6. Sensor response in human sweat
2.6.1. Impedance response for cortisol and DHEA: Nyquist and circuit fitting. Nyquist plots are a part of the primary output response of an EIS study. They are frequency dependent signatures of the impedance response. Typically, non-faradaic Nyquist plot looks like an incomplete semi-circle. This is due to the lack of a redox probe that creates the characteristic semicircle followed by 45° angled diffusion line.23,39 The Nyquist response of the sensor for cortisol and DHEA is illustrated in Fig. 7a and b. For both the Nyquist plots, there is an evidence of a dose-dependent response, as shown by the decrease in intensity of the blue curve with increasing concentration. For cortisol, the radius of the Rct curve decreases with the increase in concentration, going from 0.1 ng ml−1 to 200 ng ml−1. Similarly, for the Nyquist for DHEA dose-dependent response, a decreasing Rct is observed with the increase in dose concentration of DHEA from 0.1 ng ml−1 to 200 ng ml−1. However, there is a difference in the curvature of the response between the two molecules. This is an artifact of the difference in the chemical structure and thus, the slight difference in the binding response signature produced. This hypothesis has been validated in detail using zeta-potential study,11 and also the chronoamperometric response, which was presented in the previous section.
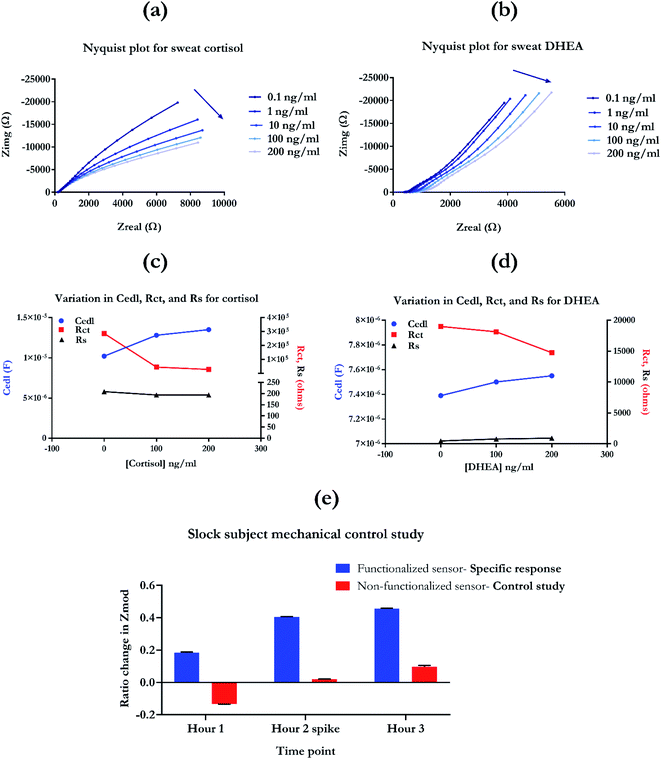 |
| Fig. 7 Electrochemical response in human sweat: (a) Nyquist plot for cortisol in human sweat; (b) Nyquist plot for DHEA in human sweat; (c) electrochemical fitting data for Cdl, Rct, and Rs trend for cortisol; (d) electrochemical fitting data for Cdl, Rct, and Rs trend for DHEA; (e) on-body mechanical control study for sensor impedance response. | |
The Nyquist plot can be used to electrochemically fit the sensor response using the Randle's equivalent circuit. Fig. S4† represented the modified Randle's equivalent circuit that was used to fit and extract the impedance response components of the sensing system. The three important parameters that define the impedance response captured using EIS are EDL capacitance (Cedl), resistance to charge transfer (Rct), and solution resistance (Rs). An ideal response would not have any significant contribution through the bulk solution effect. Having low Rs values also means that the sensor system is not perturbed by conductance or ionic pH dependent changes in the bulk solution.23,24 Mapping the electrochemical circuit components helps decode the interfacial phenomena and truly understand the EDL based response for detection. The electrochemical fitting response for cortisol and DHEA is presented in Fig. 7c and d. From Fig. 7c, it can be observed that with the increase in sensing response from 0–100 ng ml−1, there is an increase in the Cedl (blue curve), followed by simultaneous decrease in Rct (red curve). This can be attributed to the binding between cortisol molecule and the antibody present, which creates capacitive modulations appearing as increase in Cedl. Similarly, for the DHEA molecule, a similar increase in Cedl with the addition of 100 ng ml−1 can be observed. The binding between the target molecule and antibody causes a conformational change in the structure of the antibody. This causes a charge build-up in the spatial region around the capture probe, characterized by the increase in capacitance of the EDL. This facilitates the ‘transfer’ or rather, re-arrangement of these charged species, leading to decrease in the Rct of the sensing response, as observed in Fig. 7c and d. Solution resistance, illustrated by the black curve does not show any significant change going from 0–200 ng ml−1 of the biomarker concentration. The magnitude remains in the lower range of 200–220 Ω. This highlights that the sensing response is not influenced by the bulk solutions effect and is specifically responding to the binding phenomena in this affinity-based system. This is true for both cortisol and DHEA as observed from the Fig. 7c and d. For higher concentrations of cortisol and DHEA, i.e. 200 ng ml−1, the response further increases for Cedl and decreases for Rct. This confirms the sensitivity of the sensor to the concentration of target present in the system in real-time. With the increase in amount of biomarker, the functionalized antibody binding sites are bound with the target molecules, leading to an increase in capacitance of EDL, followed by a simultaneous decrease in Rct. Overall, mapping the circuit elements highlights the binding specific response of the EIS study. It offers an incredible insight into the phenomenon of dose dependent non-faradaic sensing aspect of the biosensing platform.17,40–42 Thus, the results from electrochemical fitting bolster the capabilities of this circadian diagnostic platform for mapping the changes in the chronobiology-relevant biomarker concentrations. It also confirms the high sensitivity of the platform for the presence of target biomarkers, cortisol and DHEA.
2.6.2. Capturing the on-body mechanical resiliency: motion-based artifacts. In the previous publication, we highlighted the ability of SLOCK to capture the spike and fall in levels of circadian-relevant biomarkers in human subjects.11 In order to fully understand the circadian diagnostic performance, this platform was evaluated for mechanical stability and motion contributed response change using on-body subject-based experiments. This result highlights the response generated by motion artifacts caused due to ‘human factors’ and is depicted in Fig. 7e. Based on the experimental design, non-functionalized sensor surface was used as the control study for capturing the motion-based impedance response. From Fig. 7e, it can be observed that for hour 1, the response has a negative impedance change of 1.3 ± 0.1%. The subject performs brief physical exercise before hour 2 to cause a spike in cortisol levels. By addition of this spike, which is the phase of maximum mechanical disturbance, it can be observed that the non-functionalized sensor responds with an impedance change of 2 ± 0.06%. Similarly, for hour 3, following the period of rest after exposure to spike, there is a slight increase in impedance. However, the non-specific responses are significantly lower than the functionalized sensor based specific responses, that have magnitudes 18 ± 0.5%, 40 ± 0.1%, and 45 ± 0.2% for hours 1, 2 and 3 respectively. This study indicates that the response for biomarker rise and fall is specific and is a direct result of the binding response between the biomarker and immobilized capture probe. As discussed earlier, the impedance response of the sensor is used to capture the non-faradaic, dose-dependent EDL capacitive modulations due to the re-arrangement of charged species at the electrode–electrolyte interface. For the non-functionalized sensor surface, the response is mainly driven by the bulk effects of the buffer present, i.e. sweat and the motion artifacts of the user, in this case. However due to serpentine design of the electrode, the effect of motion artifacts on the response of the sensor is minimized. Previously, we described the benchtop bending study along the x- and y-axis over the period of 100 bending cycles.12 With this study, a ‘true’ on-body experience for mechanical bending is captured. Despite the presence of high amount of on-body mechanical oscillations, the sensor system is able to respond specifically to the target concentration. It can be concluded that this system is stable for on-body use and motion artifacts do not significantly hamper the impedance response of the sensing platform.
3. Experimental
3.1. Reagents and materials
The thiol linker, 3,3′-dithiobis(sulfosuccinimidyl propionate) (DTSSP) and phosphate buffered saline (PBS) was procured from ThermoFisher Scientific Inc. (Waltham, MA, USA). Cortisol molecule (hydrocortisone), cortisol IgG antibody, and DHEA IgG antibody were purchased from Abcam (Cambridge, MA, USA). DHEA was procured from Avanti Polar lipids, Inc. (Alabaster, AL, USA). Synthetic sweat was prepared according to the protocol described in Mathew et al.43 Human sweat was obtained from Lee Biosolutions Inc. (St. Louis, MO, USA). Millipore DI water was used to perform all buffer preparation and dilutions.
3.2. Sensor fabrication and immunoassay
Sensor fabrication was performed according to previously described protocols using a nanoporous polyamide substrate (GE Healthcare Life Sciences (Piscataway, NJ, USA)).12,17 Sensor functionalization was performed using the protocol described in Upasham et al.12 Sensors were incubated with thiol linker DTSSP and antibody (10 μg ml−1) for a period of 24 hours at 4 °C. Functionalized sensors were then used for performing electrochemical studies. All electrochemical characterization was carried out using Gamry Reference 600 potentiostat (Gamry Instruments, PA, USA).
3.3. COMSOL Multiphysics® software simulations
Electrode wicking simulations were carried out using the licensed software version of COMSOL Multiphysics® v5.4. The modules used for simulations were transport of diluted species in porous media, electrostatics, and primary current distribution. AutoDesk™ AutoCAD software was used to map the electrode geometry and the CAD import module was used for input in COMSOL for performing simulations. Arrow guided streamline 2D plots were exported and used to represent the direction of flow. The mode of study used was dynamic with time ranging from 0 to 100 seconds.
3.4. Experimental details for SPR experiments
SPR studies were performed using the Open SPR instrument (Nicoya, Canada). Reagents and kits for characterization were procured from Nicoya (Canada). Carboxyl chips were used as the sensor for binding characterization. Protein A immobilization kit was employed for characterizing the biorecognition element, which is an IgG antibody. The protocol for immobilization is highlighted in the schematic presented as Fig. 3a and was according to the protocol provided with the protein A sensors kit tech guide. The concentrations used for the reagents were 10 Mm HCl (pH 2–3), 20 μg ml−1 protein A, and 50 μg ml−1 of IgG antibody (Abcam, USA).
3.5. Experimental setup for FTIR
Fourier transform infrared spectrum was captured using the Thermo scientific Nicole iS50 FTIR in Attenuated Total Reflectance (ATR) mode. The tool has a germanium ATR crystal, deuterated triglycine sulfate (DTGS) detector and a KBr window. The sample was prepared on a gold deposited polyamide nanoporous substrate. The spectrum was recorded between 675 cm−1 and 4000 cm−1 with a resolution of 0.5 cm−1 and 256 scans.
3.6. Experimental details for single frequency EIS
Single frequency experiments were carried out at the frequency of 15 Hz, where maximum capacitance behavior is observed for the SLOCK platform. Doses for biomarkers were created by spiking synthetic sweat pH 6 (SS pH 6) with concentrations ranging from 0.1 ng–500 ng ml−1. Change in Zmod from baseline i.e. blank SS pH 6 and phase shift was calculated and presented in the Fig. 4. The time period of experiment for both cortisol and DHEA was based on previously optimized protocol. The experiment length was 3000 s for cortisol and 2860 s for DHEA.
3.7. Experimental details for chronoamperometry
Chronoamperometry was performed by applying step input potential of 250 mV to the electrode and output current spectra was recorded for the period of 60 seconds. This was performed for baseline (0 ng ml−1), low dose (1 ng ml−1) and high dose (500 ng ml−1) of biomarkers, cortisol and DHEA in SS pH 6. The doses were prepared according to the protocol mentioned in the previous section and were drop casted on the sensor surface for testing. Extraction of the peak current and data analysis was performed using Gamry Echem Analyst™ software.
3.8. Experimental details for sweat evaporation study
Sweat evaporation was measured using VapoMeter (Delfin Technologies, Finland). The protocol for measurement using the device involved measuring the transepidermal water loss (TEWL) at the skin surface for period of 5 seconds with a total of 3 measurements at each location. The TEWL maps the sweat rate at the site of interest. The sites chosen were palms, underarms, feet soles, ankles, forearms, and lower back. The site-specific evaporation study had N = 3 measurements for one subject. The measurements were carried out under the IRB protocol 19–23, approved by the IRB board at University of Texas at Dallas.
3.9. Experimental details for sensor response in human sweat
Human sweat-based calibration dose response studies (Nyquist plots) were recorded using EIS performed over the frequency range of 1–10
000 Hz. The doses were spanned across the physiologically relevant range i.e. 0.1, 1, 10
100, and 200 ng ml−1, in human sweat. Electrochemical fitting was performed using the Nyquist and Bode plots obtained using these EIS experiments, with the aid of ZView® electrochemical fitting software. For the human subject based data, the on-body protocol was performed using the protocol described in Upasham et al.11 One subject with N = 3 sensors were used for performing data analysis. Functionalized versus non-functionalized sensor surface was used to capture the on-body mechanical displacement of the sensing response. The sock-based sensor was used to capture the change in response after exposure to maximum mechanical stimulations on the sensing surface. All trials were conducted according to the IRB protocol number: 18–116, approved by the Institute Review Board at University of Texas at Dallas.
3.10. Statistical analysis
Data is represented as mean ± SEM. The data is represented with N = 3. Inter-assay variation is <20%, intra-assay variation is <5%, which is CLSI guideline compliant.31,44 Significance test was carried out using ANOVA followed by post hoc Tukey test with α of 0.05. Non-linear regression and statistical analysis were performed using Graph Pad Prism version 8.01 (Graph Pad Software Inc., La Jolla, CA, USA).
4. Conclusions
SLOCK is an electrochemical circadian diagnostic platform for mapping the user's chronobiology using biomarkers, cortisol and DHEA. The work demonstrates the biomarker-based sensitivity using electrochemical detection modalities. The limit of detection is 0.1 ng ml−1 for cortisol and DHEA. The dynamic range of detection is 0.1 to 500 ng ml−1. Also, on-body sweat rate characterization highlights the effect of optimal location for passive sweat sampling on the biosensing performance. In addition, wearable form factor-based motion related artifacts are minimal, and the serpentine electrode-based sensor is mechanically resilient. Electrochemical fitting using Randle's equivalent circuit highlights the specificity of the sensing platform for binding of target biomarker. Thus, SLOCK has circadian diagnostic capabilities and is a robust, sensitive, and highly selective sweat-based chronobiology tracking platform.
Conflicts of interest
Dr Shalini Prasad has a significant interest in Enlisense LLC, a company that may have a commercial interest in the results of this research and technology. The potential individual conflict of interest has been reviewed and managed by The University of Texas at Dallas and played no role in the study design; in the collection, analysis, and interpretation of data; in the writing of the report, or in the decision to submit the report for publication.
Acknowledgements
The authors would like to thank Ambalika Tanak for helping with the scanning electron microscopy pictures. The authors would also like to thank Serena Bhadsavle for helping with the figure schematics for EIS and chronoamperometry.
References
- A. J. Jajack, Enabling sweat-based biosensors: Solving the problem of low biomarker concentration in sweat, University of Cincinnati, 2018 Search PubMed.
- J. Kim, A. S. Campbell, B. E.-F. de Ávila and J. Wang, Wearable biosensors for healthcare monitoring, Nat. Biotechnol., 2019, 37, 389–406 CrossRef CAS.
- J. Heikenfeld, Non-invasive analyte access and sensing through eccrine sweat: Challenges and outlook circa 2016, Electroanalysis, 2016, 28, 1242–1249 CrossRef CAS.
- C. Liu, T. Xu, D. Wang and X. Zhang, The role of sampling in wearable sweat sensors, Talanta, 2020, 212, 120801 CrossRef CAS.
- M. Constantinescu and B. C. Hilman, The Sweat Test for Quantitation of Electrolytes: A Challenge in Precision, Lab. Med., 1996, 27, 472–477 CrossRef.
- A. Mena-Bravo and M. D. Luque de Castro, Sweat: A sample with limited present applications and promising future in metabolomics, J. Pharm. Biomed. Anal., 2014, 90, 139–147 CrossRef CAS.
- K. B. Hammond, N. L. Turcios and L. E. Gibson, Clinical evaluation of the macroduct sweat collection system and conductivity analyzer in the diagnosis of cystic fibrosis, J. Pediatr., 1994, 124, 255–260 CrossRef CAS.
- O. Parlak, V. F. Curto, E. Ojeda, L. Basabe-Desmonts, F. Benito-Lopez and A. Salleo, in Wearable Bioelectronics, Elsevier, 2020, pp. 65–88 Search PubMed.
- J. R. Windmiller and J. Wang, Wearable Electrochemical Sensors and Biosensors: A Review, Electroanalysis, 2013, 25, 29–46 CrossRef CAS.
- S. P. Adiga, C. Jin, L. A. Curtiss, N. A. Monteiro-Riviere and R. J. Narayan, Nanoporous membranes for medical and biological applications, Wiley Interdiscip. Rev.: Nanomed. Nanobiotechnol., 2009, 1, 568–581 CAS.
- S. Upasham and S. Prasad, SLOCK (sensor for circadian clock): passive sweat-based chronobiology tracker, Lab Chip, 2020, 20, 1947–1960 RSC.
- S. Upasham, K. Thai, R. Muthyala and S. Prasad, Flexible, low volume detection of chronobiology biomarkers from human sweat, Analyst, 2020, 145, 784–796 RSC.
- F. H. She, K. L. Tung and L. X. Kong, Calculation of effective pore diameters in porous filtration membranes with image analysis, Robot. Comput. Integrated Manuf., 2008, 24, 427–434 CrossRef.
- N. K. Mintah Churcher, S. Upasham, P. Rice, S. Bhadsavle and S. Prasad, Development of a flexible, sweat-based neuropeptide Y detection platform, RSC Adv., 2020, 10, 23173–23186 RSC.
- M. Liu, J. Wu, Y. Gan, D. A. H. Hanaor and C. Q. Chen, Tuning capillary penetration in porous media: Combining geometrical and evaporation effects, Int. J. Heat Mass Transfer, 2018, 123, 239–250 CrossRef.
- S. Upasham, A. Tanak, B. Jagannath and S. Prasad, Development of ultra-low volume, multi-bio fluid, cortisol sensing platform, Sci. Rep., 2018, 8, 16745 CrossRef.
- S. Upasham, S. Bhadsavle and S. Prasad, Non-invasive monitoring of a circadian relevant biomarker from easily accessible body fluids using hybrid aqueous-ionic buffer interfaces on flexible substrates, Anal. Methods, 2019, 11, 1229–1236 RSC.
- M. J. E. Fischer, in Surface plasmon resonance, Springer, 2010, pp. 55–73 Search PubMed.
- M. G. Gore, A. G. Popplewell, W. F. Ferris, M. Scawen and T. Atkinson, Novel immunoglobulin binding proteins, Biochem. Soc. Trans., 1992, 20, 289S CrossRef CAS.
- R. D. Munje, S. Muthukumar, B. Jagannath and S. Prasad, A new paradigm in sweat based wearable diagnostics biosensors using Room Temperature Ionic Liquids (RTILs), Sci. Rep., 2017, 7, 1950 CrossRef.
- A. J. Bard, L. R. Faulkner, J. Leddy and C. G. Zoski, Electrochemical methods: fundamentals and applications, Wiley, New York, 1980, vol. 2 Search PubMed.
- A. J. Bard, Electrochemical Methods, Fundam. Appl., 1980 Search PubMed.
- E. P. Randviir and C. E. Banks, Electrochemical impedance spectroscopy: an overview of bioanalytical applications, Anal. Methods, 2013, 5, 1098 RSC.
- S.-M. Park and J.-S. Yoo, Peer Reviewed: Electrochemical Impedance Spectroscopy for Better Electrochemical Measurements, Anal. Chem., 2003, 75, 455A–461A CAS.
- A. A. P. Ferreira, C. V. Uliana, M. de Souza Castilho, N. C. Pesquero, M. V. Foguel, G. P. dos Santos, C. S. Fugivara, A. V. Benedetti and H. Yamanaka, Amperometric biosensor for diagnosis of disease, InTech, Rijeka, Croatia, 2013, pp. 253–289 Search PubMed.
- R. K. Franklin, S. M. Martin, T. D. Strong and R. B. Brown, Chemical and Biological Systems: Chemical Sensing Systems for Liquids, 2016 Search PubMed.
- B. Rezaei and N. Irannejad, Electrochemical detection techniques in biosensor applications, Elsevier Inc., 2019 Search PubMed.
- A. Chen and S. Chatterjee, Nanomaterials based electrochemical sensors for biomedical applications, Chem. Soc. Rev., 2013, 42, 5425–5438 RSC.
- W. Putzbach and N. J. Ronkainen, Immobilization techniques in the fabrication of nanomaterial-based electrochemical biosensors: A review, Sensors, 2013, 13, 4811–4840 CrossRef CAS.
- C. Gautier, C. Esnault, C. Cougnon, J.-F. Pilard, N. Casse and B. Chénais, Hybridization-induced interfacial changes detected by non-Faradaic impedimetric measurements compared to Faradaic approach, J. Electroanal. Chem., 2007, 610, 227–233 CrossRef CAS.
- M. Moretti, D. Sisti, M. B. Rocchi and E. Delprete, CLSI EP17-A protocol: A useful tool for better understanding the low end performance of total prostate-specific antigen assays, Clin. Chim. Acta, 2011, 412, 1143–1145 CrossRef CAS.
- K. Sato, W. H. Kang, K. Saga and K. T. Sato, Biology of sweat glands and their disorders. I. Normal sweat gland function, J. Am. Acad. Dermatol., 1989, 20, 537–563 CrossRef CAS.
- K. Wilke, A. Martin, L. Terstegen and S. S. Biel, A short history of sweat gland biology, Int. J. Cosmet. Sci., 2007, 29, 169–179 CrossRef CAS.
- N. A. Taylor and C. A. Machado-Moreira, Regional variations in transepidermal water loss, eccrine sweat gland density, sweat secretion rates and electrolyte composition in resting and exercising humans, Extreme Physiol. Med., 2013, 2, 4 CrossRef.
- S. Nawrocki and J. Cha, The etiology, diagnosis, and management of hyperhidrosis: A comprehensive review: Etiology and clinical work-up, J. Am. Acad. Dermatol., 2019, 81, 657–666 CrossRef.
- G. E. Folk and A. Semken, The evolution of sweat glands, Int. J. Biometeorol., 1991, 35, 180–186 CrossRef.
- Z. Sonner, E. Wilder, J. Heikenfeld, G. Kasting, F. Beyette, D. Swaile, F. Sherman, J. Joyce, J. Hagen, N. Kelley-Loughnane and R. Naik, The microfluidics of the eccrine sweat gland, including biomarker partitioning, transport, and biosensing implications, Biomicrofluidics, 2015, 9, 31301 CrossRef CAS.
- H. A. Tetteh, S. S. Groth, T. Kast, B. A. Whitson, D. M. Radosevich, A. C. Klopp, J. D'Cunha, M. A. Maddaus and R. S. Andrade, Primary Palmoplantar Hyperhidrosis and Thoracoscopic Sympathectomy: A New Objective Assessment Method, Ann. Thorac. Surg., 2009, 87, 267–275 CrossRef.
- H. Stevenson, N. R. Shanmugam, A. P. Selvam and S. Prasad, The Anatomy of a Nonfaradaic Electrochemical Biosensor, SLAS Technol. Transl. Life Sci. Innov., 2017, 23, 5–15 Search PubMed.
- N. R. Shanmugam, S. Muthukumar and S. Prasad, Ultrasensitive and low-volume point-of-care diagnostics on flexible strips – a study with cardiac troponin biomarkers, Sci. Rep., 2016, 6, 33423 CrossRef CAS.
- K.-C. Lin, B. Jagannath, S. Muthukumar and S. Prasad, Sub-picomolar label-free detection of thrombin using electrochemical impedance spectroscopy of aptamer-functionalized MoS2, Analyst, 2017, 142, 2770–2780 RSC.
- B. Jagannath, S. Muthukumar and S. Prasad, Electrical double layer modulation of hybrid room temperature ionic liquid/aqueous buffer interface for enhanced sweat based biosensing, Anal. Chim. Acta, 2018, 1016, 29–39 CrossRef CAS.
- M. T. Mathew, E. Ariza, L. A. Rocha, A. C. Fernandes and F. Vaz, TiCxOy thin films for decorative applications: Tribocorrosion mechanisms and synergism, Tribol. Int., 2008, 41, 603–615 CrossRef CAS.
- R. J. McEnroe, M. F. Burritt, D. M. Powers, D. W. Rheinheimer and B. H. Wallace, 2010.
Footnote |
† Electronic supplementary information (ESI) available. See DOI: 10.1039/d0ra10561a |
|
This journal is © The Royal Society of Chemistry 2021 |
Click here to see how this site uses Cookies. View our privacy policy here.