DOI:
10.1039/D0RA10840E
(Paper)
RSC Adv., 2021,
11, 14632-14653
In silico exploration of lignin peroxidase for unraveling the degradation mechanism employing lignin model compounds
Received
25th December 2020
, Accepted 10th March 2021
First published on 20th April 2021
Abstract
Lignin peroxidase is a heme-containing biocatalyst, well-known for its diverse applications in the fields from environmental chemistry to biotechnology. LiP-mediated oxidative catalysis is H2O2-dependent, and can oxidize phenolic, and non-phenolic substrates by oxidative cleavage of the C–C and C–O bonds of lignin. In contrast to fungi-derived LiP, the binding affinity of bacterial-derived LiP to lignin at the molecular level is poorly known to date. Tremendous wet-lab studies have been unveiled that provide degradation and biotransformation information on kraft lignin, whilst studies on the completely transformed compounds and the degradation of each transformed compounds simultaneously during degradation are scarce. To gain an understanding of the degradation process using docking, and MDS based studies, we assessed the binding affinity of selected lignin model compounds with bacterial origin LiP and validated such docked complexes exploiting 30 ns molecular dynamics simulations. We selected and picked a total of 12 lignin model compounds for molecular modeling analysis, namely two chlorinated lignin model compounds (monomer) (2-chlorosyringaldehyde and 5-chlorovanillin), eight standard lignin model compounds (veratryl alcohol, syringyl alcohol, sinapyl alcohol, methyl hydroquinone, guaiacol, coniferyl alcohol, catechol, and 4-methoxy phenol), while, two 4-O-5, and β-O-4 linkage-based multimeric model compounds (dimer: 2-methoxy-6-(2-methoxy-4-methylphenoxy)-4-methylphenol; trimer: syringyl β-O-4 syringyl β-O-4 sinapyl alcohol). Far more specific binding residues were observed from XP-Glide docking, as TYR, HIP (protonated histidine), PHE, VAL, ASP, THR, LYS and GLN. The binding affinity was confirmed by the Gibbs free energy or binding energy (ΔG) score; furthermore, it is found that the maximum binding energy seems to be observed for 4-methoxyphenol with a Glide score of −3.438 with Pi–Pi stacking and H-bond type bonding interactions, whilst the lowest XP Gscore as −8.136 with Pi–Pi stacking and H-bond (side chain) type bonding interactions were found for the trimer model compound. The docked complexes were further evaluated for deep rigorous structural and functional fluctuation analyses through high-performance molecular dynamics simulations-DESMOND, after a post simulation run of 30 ns. The RMSD trajectory analyses of the protein-ligands were found to be in the equilibrium state at the end of simulation run for multimeric lignin model compounds. In addition, ionic ligand–protein interaction occurs among chlorinated compounds, while hydrophobic and H-bond contacts have frequently been observed in all lignin-model compounds. The findings herein demonstrate that bacterial LiP can effectively catalyze multiple lignin model compounds, and it might further be used as an effective tool for sustainable mitigation of diverse environmental contaminants.
Introduction
Lignin is found as a primary organic chemical in plant cells, along with cellulose and hemicellulose.1 Three sorts of phenylpropanoids act as a polymer forming unit in the complex structure of lignin polymers, i.e. p-hydroxyphenyl (H), guaiacyl (G) and syringyl (S) (from p-coumaryl alcohol, coniferyl alcohol, and sinapyl alcohol, respectively).2,3 As a natural organic polymer, lignin exists near about 20% in the lignocellulosic component.4 Several cross-linked phenolic linkages provide strength and complex structural framework as key linkage contributors to the lignin polymer. The key component of soft and hardwood lignin contains a variety of common linkages, i.e. β-5/α-O-4, β-O-4, α-carbonyl, etc.5–7 The pulp & paper industries utilize a massive amount of plants as raw materials (lignocellulosic) for manufacturing of papers in different quality. During the paper processing steps, the lignin undergoes with several chemical reactions, which leads to depolymerization of lignin polymer and cleavage, consequently many lignin derivative as low molecular weight compounds generates, and expelled in wastewater (i.e.chlorinated, phenolics). The pulping, bleaching, and deinking processes are the key chemical process stages where specific chemical agents, i.e. Na2S, NaOH and ClO2, are utilized, which are often accountable for producing different lignin-derived pollutants.8 This contributes to the processing and drainage of chlorinated, sulfonated phenol, and several free phenolic monomer units through processing plants, and such may triggers significant environmental hazards in the form of different toxicological endpoints.9,10 Therefore, sufficient degradation of lignin-based compounds is needed to keep the environment clean and safe from diverse lignin-related contaminants. The high complexity of the lignin structure in a polymer raises the main challenge in the study of molecular routes for the biodegradation of lignin.11,12 The presence of phenolic compounds, including chlorine, sodium, and sulfite, makes it highly biologically recalcitrant toward degradation. Since the structure and chemical composition are dependent on lignin, whether it is softwood or hardwood, the full structural, functional attributes of lignin is still less known to date.13 Thus, the uncertainty in the structural chemistry ranges from plant to plant is the second biggest challenge. Biotransformation and biodegradation of kraft lignin have been evaluated tremendously with ligninolytic enzymes; however, clear information on completely bio-transformed compounds is very scarce. In silico studies could provide the screening of each transformed compound to understand the degradation mechanism at the molecular level.14–16 For this, various lignin model compounds have been selected to replace lignin for the degradation process. KL as a model lignin has been widely studied in conventional in situ bioremediation. Depolymerized lignin yields various lignin model compounds, which are poorly studied at the molecular level. In this context, numerous lignin model compounds have been used in a computational study, including veratryl alcohol, syringyl alcohol, sinapyl alcohol, methyl hydroquinone, guaiacol, coniferyl alcohol, catechol, and 4-methoxyphenol as standard lignin model compounds. Besides the standard monomeric lignin model compounds, numerous polymeric lignin model compounds (β-O-4, and 4-O-5 linkage-based), i.e. a dimer and trimer (dimer: 2-methoxy-6-(2-methoxy-4-methylphenoxy)-4-methylphenol; trimer: syringyl β-O-4 syringyl β-O-4 sinapyl alcohol) have also been exploited in computational studies to understand the lignin degradation mechanism using ligninolytic enzymes.17–21 To carry out such a computational study, numerous members of the ligninolytic enzyme family (Often oxidoreductases in catalytic nature) have been exploited in molecular dynamics simulations and molecular docking assays to determine their binding affinity and behavior in the catalysis of lignin model compounds.22–25
Lignin peroxidase (EC 1.11.1.14) is a member of the lignin-modifying enzymes (LME), often heme-containing H2O2-dependent oxidoreductases, which seems to act on a wide range of non-phenolic, phenolic, and lignin-derived compounds.26–28 In addition to the remediation of several phenolic and non-phenolic compounds, LiP has been used as a promising biocatalyst in industrial processing for diverse biotechnological applications.29–31 Numerous bacteria and fungi are known for the extracellular production of LiP.12,32 The LiP-mediated catalytic reaction is hydrogen peroxide (H2O2)-dependent and completes it catalytic cycle in four specific catalytic reaction cycles.33,34 Radical cations are responsible for the basic oxidative properties of LiP by one-electron oxidation, oxidation of an electron, leading to the cleavage of the side chain component, resulting in demethylation, intramolecular addition, and subsequently rearrangement.29,35 Specific catalytic oxidative processes related to LiP include hydroxylation of benzyl methylene groups and benzyl alcohol oxidation into aldehydes or ketones, along with the oxidation of phenol.36,37 A range of protein structures (LiP) at different resolutions have been reported since the 1990s, which are freely available in the Protein Databank (https://www.rcsb.org) and can be accessed worldwide for scientific/academic purposes.38–41 Structures of fungal-derived LiP at varying resolution are available in the PDB, and are quite a bit more prevalent than structures of bacterial-derived LiPs or dye-decolorizing peroxidases (DyP). From the structural perspective, the protein structure of LiP from Phanerochaete chrysosporium comprises 351 amino acid residues, and two chains (A and B) in its complex (PDB: 1B85).42,43 The structure and functional attributes of LiP are similar or identical to those of DyP.44 LiP has been shown to have similar ligninolytic activity to DyP-type peroxidase from Enterobacter lignolyticus. DyP-type peroxidase comprises 318 amino acid residues, and four chains (A, B, C and D) in its protein complex (PDB ID: 5VJ0).43,45 Similar to DypA, a DypB-based study has been reported for the oxidizing catalysis of polymeric lignin, and lignin model compounds by cleavage of C(α)–C(β) bonds, which concluded the significant potential for lignin oxidation.46,47 As stated above, fungi-derived LiPs have been studied tremendously in the last few decades for catalytic activity (oxidative cleavage) toward lignin, and other similar compounds.48,49 However, no bacterial-origin LiP structure is available for in silico study, whilst several bacterial-derived LiPs have already been reported for conventional lignin degradation and dye decolorisation studies.9,50–53 The active site with key binding residues of bacterial LiP is unknown to date. Furthermore, there is no clear understanding of the molecular basis for lignin biodegradation in association LiP with lignin. The scarce knowledge about the degraded compounds and their toxicity assessment is the key flaw of conventional bioremediation. To address this flaw, we conducted an in silico study to evaluate the degradation potential of LiP utilizing several lignin model compounds.14,15,54 To achieve this, comparative modeling of bacterial-derived LiP, and the use of build protein model for docking studies, and simulations of 30 ns, were performed with selected lignin model compounds, which could provide the binding and structural interactions. Furthermore, such molecular modeling findings might be used for protein–ligand binding/catalyzing interaction (LiP–lignin model compound) at the molecular and atomic levels. Therefore, in the present study, we performed the protein structural and functional analyses using five stage computational methodologies (Fig. 1). Furthermore, we evaluated the degradation efficiency of LiP through Glide-docking by contrasting with a known control (azure-b). A total of 12 standard lignin model compounds in three flavor were used and comparatively analyzed for binding energy, key interacting AA residues, and certain bondings type for all chlorinated, phenolic, and polymeric compounds. The comparative modeling, comparative binding energy, ligand interactions with possible bond interactions and three-dimensional binding pocket residue analysis, followed by rigorous structural and functional analysis through MDS of 30 ns, are the focus of this study. For further validation, the docked complexes were inspected through Desmond (High-performance molecular dynamics simulations) simulation of 30 ns. Post simulation analysis indicates that multimeric complexes were considered to be in an equilibrium state with hydrophobic and H-bond type contacts. In addition to the binding affinity with several lignin model compounds, binding and interactional attributes have indeed been highlighted in the structural and functional dynamics of the protein (LiP).
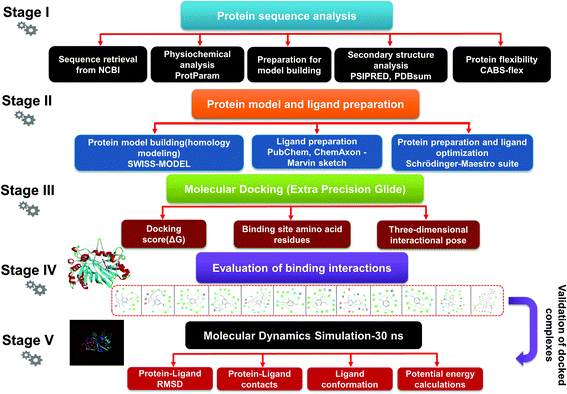 |
| Fig. 1 The detailed methodology used to carry out a comparative modeling, docking study, and MDS of selected lignin model compounds corresponding to bacterial-derived LiP. Five different stages depict the different workflows, from the first stage to final evaluation of the docked complex through 30 ns simulation, and post simulation analyses. | |
Materials and methods
Physicochemical properties analysis
The PPs help to determine the essential characteristics of a protein in different biological forms. The PPs of the selected protein sequence (LiP) were predicted using the ExPASy ProtParam tool (https://web.expasy.org/protparam/).55,56 The described server was utilized to predict, e.g. molecular weight (mol. wt), molecular calculations, the composition of amino acid residues, the molecular formula, the composition of atoms, the theoretical isoelectric point (pI), the total number of positive (Arg + Lys) and negative (Asp + Glu) residues (+R/−R), the extinction coefficient (EC), the instability index (AI), and the grand average of hydropathicity (GRAVY).
Secondary structure (SS) and sequence annotated by structure (SAS) analysis
Secondary Structure Prediction (SS) is an in silico technique, which is entirely based on hydrogen bonding patterns and certain geometric constraints; it assigns all residues from possible states.57 The annotated secondary structure elements (SSEs) could be predicted using the available PSSP tools.58 Three basic SSEs are; alpha-helix, beta-sheet, and turn, which could be predicted from the available SSP tool with query sequence of intrest. The secondary structure elements for the α-helix, β-sheet, and turn of the amino acid sequences of the modeled LiP protein were predicted using two SSP tools, namely; PSI-blast-based secondary structure prediction (PSIPRED) (http://bioinf.cs.ucl.ac.uk/psipred/) and the self-optimized prediction method with alignment (SOPMA) (https://npsa-prabi.ibcp.fr/cgi-bin/npsa_automat.pl?page=/NPSA/npsa_sopma.html).59–61 Secondary structure prediction of the query protein gives insight into the various elementary components of the protein. However, an overview of regions or sites of interest in a protein sequence including; post-translation modifications, binding sites, active sites present in the enzyme, etc., could be predicted and marked with SAS. We performed the “SAS – Sequence Annotated by Structure” prediction for the target modeled protein (LiP) to understand and predict annotation for various functional attributes throughout the protein sequence. The “SAS – Sequence Annotated by Structure” server from EMBL-EBI was used by accessing the following URL address: https://www.ebi.ac.uk/thornton-srv/databases/sas/.62,63 The listed web resource was exploited for SAS prediction of the query protein sequence, corresponding to each amino acid of bacterial LiP.
Protein 3D model prediction exploiting comparative modeling
We picked the translated protein sequence from the nucleotide sequence of the bacterial LiP gene from NCBI with GenBank: CP002272.1.64 The retrieved nucleotide sequence from the genome of Enterobacter lignolyticus SCF1 was further exploited for translation to obtain the protein sequence using the standard tool available on NCBI.65,66 The nucleotide sequence length was 900 bp in size, while the translated FASTA sequence comprised of 299 amino acid residues count (Table 1). The protein FASTA sequence was used to building a protein model and such model further proceeded into protein flexibility modeling and docking studies follwed by MDS. The obtained protein sequence (LiP) was used as a query sequence for constructing a protein model through comparative modeling. We used the SWISS-MODEL (http://swissmodel.expasy.org) server for 3D structure prediction from the query sequence in automated mode.67,68 Comparative modeling was carried out using the best matching template in automated mode.
Table 1 The query FASTA sequence (Bacterial origin-LiP) with a single letter identifier. Sequence length consisting of 299 amino acid residue count, used for protein modeling, and molecular modeling analysis
Query sequence |
MSQVQSGILPEHCRAAIWIEANVKGDVNALRECSKVFADKLAGFEAQFPDAHLGAVVAFGHDTWRALSGGVGAEELKDFTPYGKGLAPATQYDVLIHILSLRHDVNFSVAQAAMAAFGDAVEVKEEIHGFRWVEERDLSGFVDGTENPAGEETRREVAVIKDGVDAGGSYVFVQRWEHNLKQLNRMSVHDQEMMIGRTKVANEEIDGDERPETSHLTRVDLKENGKGLKIVRQSLPYGTASGTHGLYFCAYCARLYNIEQQLLSMFGDTDGKRDAMLRFTKPVTGGYYFAPSLDKLLAL |
Model evaluation/assessment
Built protein models often include numerous errors and quality issues, as a consequence of which such a model would not be useful in protein studies.69 In order to assess the reliability and accuracy of the stereochemical quality of the model, it is important to confirm and evaluate the quality of the model. A different methods have been used to evaluate the internal consistency and reliability of the modeled lignin peroxidase (LiP) structure. PROCHECK, MolProbity and a few other programs were used to assess the stereochemical quality of the model by quantifying residues in the permitted Ramachandran plot zones.70–72
Model flexibility prediction
The CABS-flex 2.0 (http://biocomp.chem.uw.edu.pl/CABSflex2) is a protein flexibility prediction server, with an efficient simulation engine that could be used for large-scale protein conformational transitions of protein systems.73 The CABS-flex model is a computationally efficient alternative to all-atomic molecular dynamics – a conventional protein simulation methodology. We used the query protein's (modeled-LiP) FASTA sequence for the prediction of protein flexibility, to access the protein fluctuations; the prediction methodologies of the server rely on molecular dynamics simulation-based data and methodology.
Ligand preparation and filtration for docking
We selected a total of 12 ligands to be used in this study along with one known control ligand (Table 2), which were prepared for molecular docking. Among them, two were chlorinated lignin compounds (chlorosyringaldehyde and 5-chlorovanillin).74,75 The other eight were standard reported lignin model compounds in monomer form (veratryl alcohol, syringyl alcohol, sinapyl alcohol, methyl hydroquinone, guaiacol, coniferyl alcohol, catechol and 4-methoxyphenol). Subsequently, two multimeric lignin model compounds in dimer and trimer form with 4-O-5 and β-O-4 type linkages (dimer: 2-methoxy-6-(2-methoxy-4-methylphenoxy)-4-methylphenol; trimer: syringyl β-O-4 syringyl β-O-4 sinapyl alcohol) were selected.76–78 We retrieved all of the ligands from the PubChem database (https://pubchem.ncbi.nlm.nih.gov).79 Two multimeric lignin model compounds as ligands were sketched and refined with ChemAxon-Marvin sketch (version 18.24) (https://chemaxon.com/products/marvin) used in SDF file format.80 In addition to the selected lignin model compounds as a ligand, we picked one compound as a control (azure-b), which is a known substrate in LiP assays.81 Before performing the protein–ligand docking using Schrödinger–Glide, the energy of all ligands was minimized. All described ligands were optimized with the LigPep 2.5 module (Schrödinger release 2020-3: LigPrep, Schrödinger, LLC, New York, NY, 2020).82 However, during the optimization process, the ionization states, tautomers, stereochemical errors, and ring conformations were corrected using Epik 2.3. (Schrödinger release 2020-3: Epik, Schrödinger, LLC, New York, NY, 2020) at the pH scale of 7 ± 2.83,84 Besides, compounds with reactive functional groups were snipped throughout optimization using OPLS-2005.85
Table 2 Chemical and molecular details of the selected lignin model compounds to be used for molecular docking, and MDS analysis. Two chlorinated lignin model compounds, eight standard monomeric lignin model compounds, and the remaining two multimeric lignin model compounds are listed consisting of structural and chemical attributes
S. no. |
Ligand |
Molecular structure |
IUPAC name |
Molecular formula |
Molecular weight (g mol−1) |
1 |
2-Chlorosyringaldehyde |
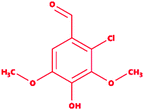 |
2-Chloro-4-hydroxy-3,5-dimethoxybenzaldehyde |
C9H9ClO4 |
216.62 |
2 |
5-Chlorovanillin |
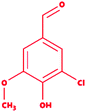 |
3-Chloro-4-hydroxy-5-methoxybenzaldehyde |
C8H7ClO3 |
186.59 |
3 |
Veratryl alcohol |
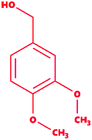 |
3,4-Dimethoxybenzyl alcohol |
C9H12O3 |
168.19 |
4 |
Syringyl alcohol |
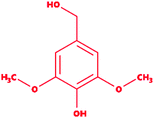 |
4-Hydroxy-3,5-dimethoxybenzyl alcohol |
C9H12O4 |
184.19 |
5 |
Sinapyl alcohol |
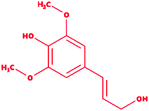 |
4-[(E)-3-Hydroxyprop-1-enyl]-2,6-dimethoxyphenol |
C11H14O4 |
210.23 |
6 |
Methyl hydroquinone |
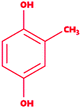 |
2-Methylbenzene-1,4-diol |
C7H8O2 |
124.14 |
7 |
Guaiacol |
 |
2-Methoxyphenol |
C7H8O2 |
124.14 |
8 |
Coniferyl alcohol |
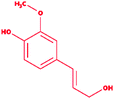 |
4-[(E)-3-Hydroxyprop-1-enyl]-2-methoxyphenol |
C10H12O3 |
180.2 |
9 |
Catechol |
 |
1,2-Dihydroxybenzene |
C6H6O2 |
110.11 |
10 |
Mequinol |
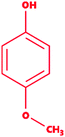 |
4-Methoxyphenol |
C7H8O2 |
124.14 |
11 |
Dimer (guaiacyl 4-O-5 guaiacyl) |
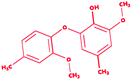 |
2-Methoxy-6-(2-methoxy-4-methylphenoxy)-4-methylphenol |
C16H18O4 |
274.31 |
12 |
Trimer (syringyl β-O-4 syringyl β-O-4 sinapyl alcohol) |
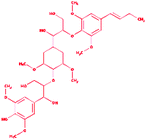 |
2-{4-[(1E)-But-1-en-1-yl]-2,6-dimethoxyphenoxy}-1-(4-{[1,3-dihydroxy-1-(4-hydroxy-3,5 dimethoxyphenyl)propan-2-yl]oxy}-3,5 dimethoxycyclohexyl)propane-1,3-diol |
C34H50O13 |
666.761 |
* |
Control (azure-b) |
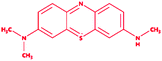 |
3-Dimethyl-[7-(methylamino)phenothiazin-3-ylidene]azanium; chloride |
C15H16ClN3S |
305.8 |
Protein model preparation
Protein preparation, protein structure refinement, and energy minimization. Before being used for molecular docking, the selected protein model, LiP, was applied for protein structure refinement and energy minimization using the protein preparation wizard of the Schrödinger suite. The modeled PDB structure file is not appropriately suitable for molecular docking with Glide.86,87 A standard PDB file often consists of heavy atoms and may contain a co-crystal ligand itself; other components, including water molecules, metal ions, and other cofactors, might be present. The selected protein (LiP) was prepared by exploiting the protein preparation wizard (pre-processed, optimized, and minimized), through the addition of hydrogen atoms, hydrogen bond optimization, and verifying the protonation states of HIS, GLN, and ASN amino acid residues (Schrödinger release 2020-3: Glide, Schrödinger, LLC, New York, NY, 2020).82,88 Energy minimization was achieved with the help of a default constraint of 0.3 Å RMSD and applying the OPLS 2005 force field.85
Extra precision Glide docking
Grid preparation. The LiP receptor grid was generated by specifying the (active) site residue, which was identified by the SiteMap tool. Once the receptor grid was generated around the binding site of LiP, the ligands were docked with the protein (LiP) using the Glide version 5.8 (Grid-based ligand docking with energetics) docking protocol.89
XP docking using Glide. Computational docking is a method often used to measure the binding behavior of one molecule (protein or receptor) to the other molecule (ligand) that bonds to form a stable complex. A docking study was carried out with all compounds (A total of 12 compounds) using the Glide, Schrödinger suite (Schrodinger v2019-1, Maestro v11.9). All prepared ligands (optimized and minimized energy) were docked with the modeled protein (LiP) using a Monte Carlo based simulation algorithm (MCSA). In Glide docking, there are two types of scoring function – Glide score 2.5 SP (Standard Precision Glide) and Glide score 2.5 XP (Extra Precision Glide). SP is a default-docking procedure; it will screen out a large number of ligands of unknown quality. Nevertheless, the XP docking and scoring function is a more influential and discerning process, where the running time is longer than SP docking. In XP docking, each ligand generates 10
000 poses. The generated structure is highly accurate and finally suggests the excellent docked complexes based on the energy term Emodel. The best orientation structures were further ranked based on XP Gscore.90 A lower XP Gscore for a structure indicates better interaction towards binding site residues. Glide evaluated the docked conformers (G) score. The G value was calculated accordingly as shown below:
Gscore = a × vdW + b × Coul + Lipo + Hbond + Metal + BuryP + RotB + site |
Molecular dynamics simulation analysis for validation of the docked complexes
The Desmond package (Schrodinger v2019-1) (Maestro v11.9 and Desmond v5.7) was utilized to run a MDS employing the docked protein complex (LiP + lignin model compounds) to find stability and catalytic interactions throughout a simulation run of 30 ns. The integrated force field OPLS3 was used (Optimized Potentials for Liquid Simulations).91,92 We built a systematic model using the model system builder wizard to carry out the MDS with a run duration of 30 ns for LiP, and a corresponding set of a total of 12 ligand (lignin model compounds) complexes.93 By extending the specifications, a startup process with the system builder as SPC (simple point charge) was built, and a water model as a solvent within the periodic boundary condition of the cubic box set at 10 Å × 10 Å × 10 Å for the complex of concern was also built. In addition, the energy of the system was minimized, and salt of a specific concentration was also added, namely Na+ with a concentration of 85.130 mM (total charge + 52) and Cl− with a concentration of 50.751 mM (total charge − 31). For a simulation run of 30 ns, the said system was built, and also initiated under the NPT ensemble, where a 300 K temperature was maintained by the Berendsen thermostat algorithm and 1 atm pressure by the Berendsen barostat algorithm throughout the simulation process.90 Coulombic interactions were analyzed using the smooth particle mesh Ewald simulation method with a cut-off of 9.0 Å distance by implementing the SHAKE algorithm.94 Output parameters as the default were set for output report generation with respect to Cα, and the ligand. A 30 ns trajectory of the protein (Cα) and ligand interaction was captured to evaluate protein and ligand behavior throughout the simulation run of about 30 ns.
Results
Physicochemical properties analysis
ProtParam is an online tool for determining the specific physical and chemical parameters of a query protein stored in the Protein Data Bank, SWISS-Prot or TrEMBL or even from a user-input based protein sequence. Computational physicochemical analysis of the modeled protein (modeled LiP) was obtained from ProtParam online tools. The computed parameters of the PP tool include the molecular weight, theoretical pI, amino acid composition, atomic composition, and extinction coefficient; the estimated half-life, instability index, aliphatic index, and grand average of hydropathicity (GRAVY) were set to obtain the expected results. The functional and structural features of a protein can be reflected in its PPs. We found results in different PPs, including molecular weight: 33
040.30 Da, theoretical pI: 5.41, molecular formula: C1465H2273N409O442S11, the total number of atoms: 4600, and so on. The detailed results of the predicted computational PPs has been revealed in Table 3, whilst the amino-acid residues in percentage with the atomic distribution has shown in Fig. 2(A and B) respectively.
Table 3 Computational physicochemical attributes of the modeled protein (LiP). Different parameters have been predicted exploiting the ExPASY/ProtParam web resource
Protein (enzyme) |
Amino acid count |
Molecular weight |
Molecular formula |
Theoretical pI |
Instability index (II) |
Aliphatic index |
Grand average of hydropathicity (GRAVY) |
Atomic composition |
C |
H |
N |
O |
S |
Modeled LiP protein |
299 |
33040.30 Da |
C1465H2273N409O442S11 |
5.41 |
40.09 |
81.57 |
−0.279 |
1465 |
2273 |
409 |
442 |
11 |
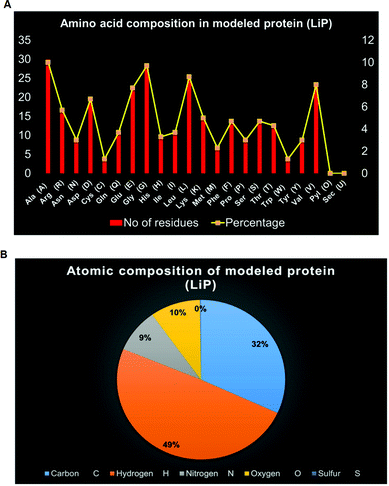 |
| Fig. 2 (A) Distribution of amino acid residues in bacterial-derived LiP. The statistical plot depict the number of amino-acid residues along with the percentage (secondary axis). (B) Distribution of atomic composition (i.e. C, H, N, S, and O) of modeled LiP (bacterial derived lignin peroxidase). | |
Secondary structure (SS) and sequence annotated by structure (SAS) analysis
Secondary structure elements (SSEs) of a protein, like alpha-helix and beta-strands, are the key constituent parts of a protein structure in its three-dimensional architecture. The SS was analyzed, and we found the result of the query protein sequence using the PSIPRED and SOPMA SSP tools. Furthermore, the sequence annotated by structure was also obtained from the EMBL-EBI server. The secondary structure prediction results of the modeled LiP as the query protein predicted using SOPMA were found to be 35.45% as alpha-helix, 18.06% as extended strand, 42.81% as random coil. Results from the PSIPRED server were also obtained in graphical cartoon form, with helices, strands, and coils in the color-coded legends (Fig. 3(A and B)). The annotation of various extended vital parts of SSEs, including sheets, beta hairpins, beta bulges, strands, helices, helix–helix interactions, beta turns, gamma turns, domains, ligand-binding sites and loops, was obtained from SAS – (Sequence Annotated by Structure) as shown in Fig. 4. The detailed results of SSP obtained from SOPMA has been explained in Table 4.
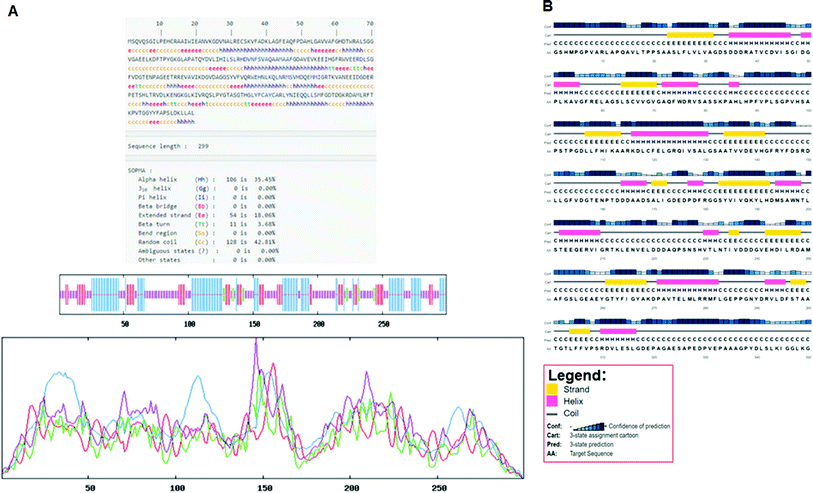 |
| Fig. 3 (A) Secondary structure prediction results from SOPMA (Self-Optimized Prediction Method with Alignment). The different secondary structure elements predicted after computing the query sequence (i.e. alpha-helix, beta-strand, turn, coils, and so on). Such can be observed through the figure. (B) Secondary structure prediction results from PSIPRED. A graphical legend based on the corresponding key AA residues (helix, strand, and coil) can be observed in the designated figure. | |
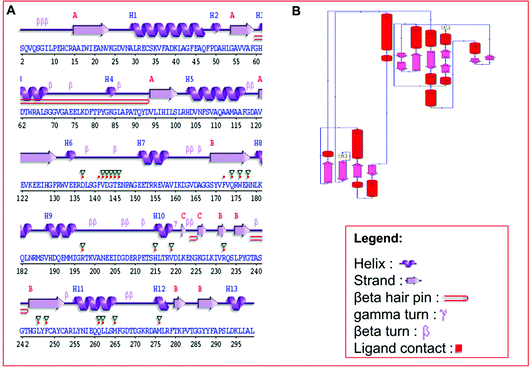 |
| Fig. 4 (A) Predicted results of the built protein model (LiP), the SAS – Sequence Annotated by Structure, prediction for determining, and revealing the annotation of a sequence as protein attributes of its functionalities. The different elements, including helix, beta-sheet, coil, hairpin loop, and beta-turn, along with ligand contacts present in the protein, has depicted. (B) Domain representation, predicted from build LiP model exploiting PDBSum – EMBL-EBI online resource. | |
Table 4 The secondary structure elements present in bacterial lignin peroxidase. SSP computed exploiting the SOPMA tool
S. no. |
Protein name |
Alpha helix (Hh) |
Extended strand (Ee) |
Beta turn (Tt) |
Random coil (Cc) |
1 |
Lignin peroxidase |
35.45% |
18.06% |
3.68% |
42.81% |
Protein 3D model prediction exploiting comparative modeling
We obtained a protein model of the query protein sequence (bacterial-LiP) using the SWISS-MODEL server in automated mode. For the query sequence, we found a total of 33 matching templates. We picked the 5vj0.1. A template with maximum identity (100%) for model building and further model assessment reports. We built a model by picking a maximum identity template chain as a 100% sequence identity, GMQE-0.97, QSQE-0.77, resolution-1.9 Å, and the oligo state as a homodimer with 2X heme ligands in a protein complex (Fig. 5). The built model based on the stated template was analyzed for quality parameters that were found to be QMEAN-0.32, cβ-0.31, solvation-0.87 with torsion-0.10. The built model was found to belong to the DyP type peroxidase family, which is a ligninolytic enzyme. A further modeled LiP model was subjected to structural assessment to evaluate the structure for various parameters, i.e. Ramachandran favored MolProbity, Rotamer Outliers, and so on. We found MolProbity score = 0.65, 98.82% for Ramachandran favoured, while 0.00% as Ramachandran outliers. For Rotamer outliers we found 0.42% (B75 GLU, B171 VAL). For C-beta deviation – 1 (B202 ASN), bad bonds value – 1/4836 (B103 HIS), and bad angles value – 38/6566 (A104 ASP, B206 ASP, (B9 LEU-B10 PRO), (A9 LEU-A10 PRO), B104 ASP, A22 ASN, A274 ASP, B274 ASP, B294 ASP, A50 ASP, A266 PHE, B22 ASN, B266 PHE, (A49 PRO-A50 ASP), B178 HIS, B61 HIS, A206 ASP, B244 HIS, B215 HIS, B103 HIS, A12 HIS, A103 HIS, A189 HIS, A178 HIS, (B147 ASN-B148 PRO), B189 HIS, B9 LEU, (A147 ASN-A148 PRO), A9 LEU, B12 HIS, B52 HIS, A128 HIS, A52 HIS, (A290 ALA-A291 PRO), B128 HIS). Furthermore, quality estimate and residue quality has shown in the respective figures. The specified protein model (Modeled-LiP) was further used for the docking, and MDS experimentations, after the optimization of this constructed model followed by model assessment/validation utilizing the available resources. Three-dimensional structural poses, rendered in different styles, have shown in Fig. 6.
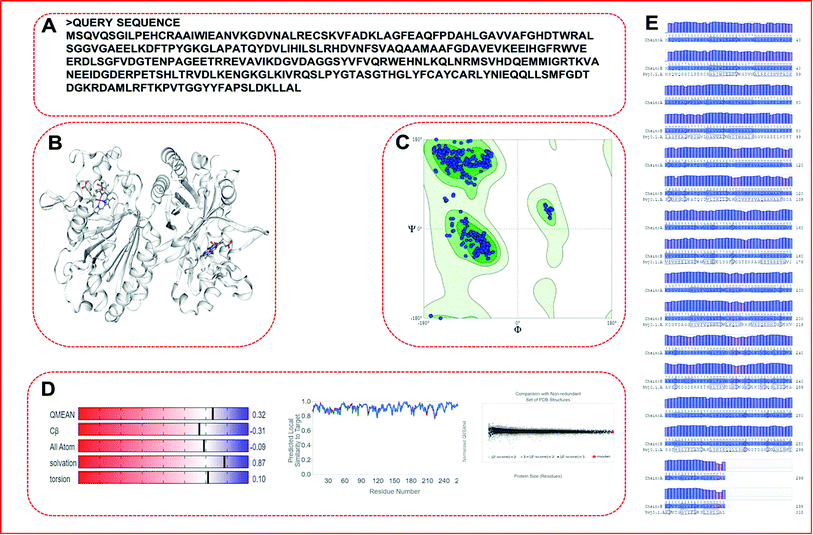 |
| Fig. 5 SWISS-MODEL-based homology modeling result. Query sequence used for building the protein model by matching the maximum score with the contrasting template. (A) Represent the query sequence in a single letter codon. (B) Represent the built protein model (dimer form, consisting of two identical chains). (C) Ramachandran plot for the coordinates quality and correctness for steriochemical aspects. (D) Structural assessment for different parameters. (E) Protein-blast of query residues corresponding to matching template along with residue quality. | |
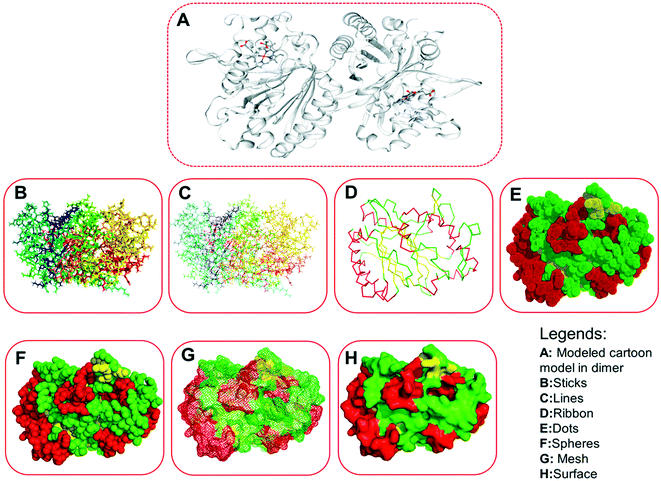 |
| Fig. 6 Three-dimensional structures of the modeled protein (LiP), rendered in a different form. (A) Represents the original complex in cartoon form, as derived from the homology modeling, consisting of several ligands. (B) Sticks form. (C) Lines form. (D) Ribbon form. (E) Dots form. (F) Spheres. (G) Mesh form. (H) Surface form. | |
Model evaluation/assessment
The structural validation of the built protein model (Modeled LiP) was carried out using PROCHECK, RAMPAGE, ERRAT, and Verify 3D online server. The model evaluation analysis was revealed and have described in subsequent sections. PROCHECK was used for prediction of the analysis of residues by residential geometry and the overall structural geometry governs the stereochemical quality of a protein structure. Verify 3D determines a structural class based on its location and environment (alpha, beta, loop, polar, non-polar, etc.) and compares results with suitable structures, to determine the compatibility of an atomic model (3D) with its amino acid sequence (1D). ERRAT is a crystallography-determined verification program for protein structures. The Ramachandran plot provides a convenient view of the torsion angle distribution in a protein structure. PROCHECK was used to measure the stereochemical quality residue-by-residue of protein structures with the Ramachandran plot (RP) analyses. The RP analysis found that 92.0% of the model and template amino acids were in the most favorable region and 7.2% were in the additional allowed region, 0.6% of amino acids generously are in allowed regions, and 0.1% are in disallowed regions. The above validation indicates that the backbone conformation of the constructed LiP models and their non-bonded interactions are both reasonable within a typical standard range. PROCHECK, RAMPAGE, ERRAT, and Verify 3D have checked and verified the modeled LiP protein model. A reasonable sequence to structure agreement was shown by the Verify 3D assessment, since no amino acid has a negative score, and 97.32% of the residues have averaged 3D-1D score ≧ 0.2, it should be noted that the above zero compatibility values match the acceptable side-chain environment. ERRAT overall quality factor was obtained as A: 97.3154. While the PROCHECK score Out of 9 evaluations was – errors: 0; warning: 5; pass: 4. The validation with different structural and stereochemical analysis results of the model LiP model has shown in Fig. 7.
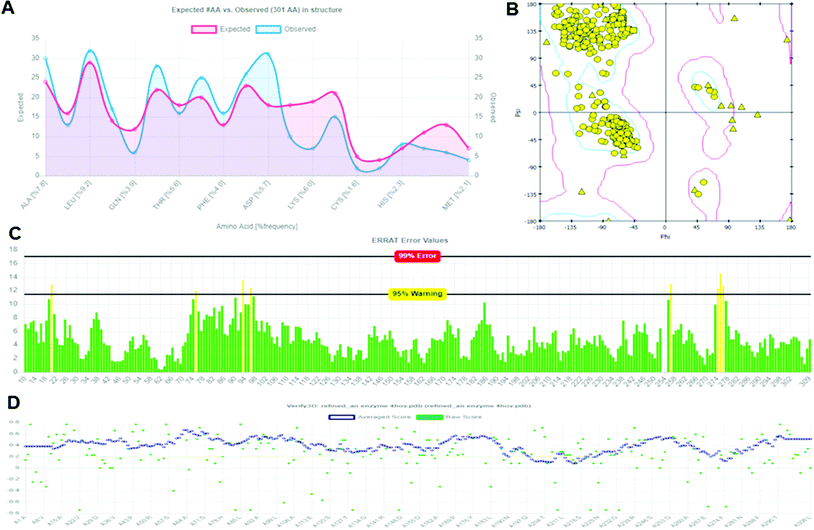 |
| Fig. 7 Protein model assessment report generated for assessing the quality, and structural parameters of the built LiP model. (A) Ramachandran interactive plot. (B) 2D Ramachandran plot. (C) ERRAT error values graph. (D) Verify 3D graph. | |
Model flexibility prediction
The CABS-flex is an effective modeling method for fast protein structure flexibility simulations. The flexibility of the modeled protein (LiP) was predicted using the CABS flex server, utilizing the built LiP model. It is focused on the CABS models, an established tool for the modeling of coarse-grained proteins. A Protein Structure in PDB (or Protein PDB Code) is the only data needed as an input. A significant feature of CABS protein models is that their spatial resolution (in C-alpha chain) allows the reconstruction of a whole-atomic depiction of physically realistic models. As a starting point for CABS simulation, the input structure has been used for predicting the flexibility of the LiP model. We found results in different parameters, including project information, model detail (a total of ten models were built), and contact maps with trajectories along with fluctuations plot. The flexibility prediction of the LiP model has summarized in Fig. 8A–C.
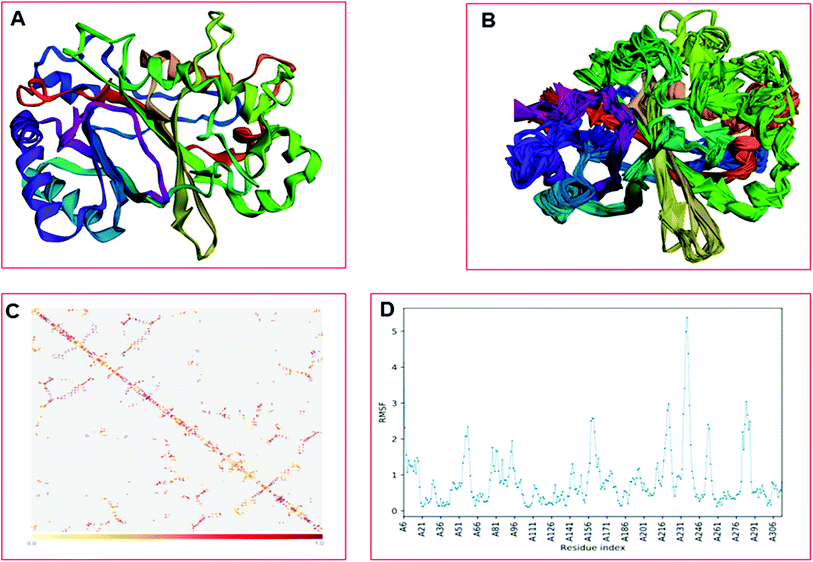 |
| Fig. 8 Built protein's model (LiP) flexibility prediction. (A) Cα trace representation of a single model in refine mode. (B) Superimposition of all ten models. (C) The contact map. (D) The fluctuation plot of the amino acid residue distribution. | |
Extra precision Glide docking
Docking results in different parameters were obtained from the docking program, in a single output file consisting of the energy score and several interactional parameters. Protein + ligand complex files were also obtained for each ligand, and each were further explored for three-dimensional ligand interactions, exploring active site amino acid residues with possible bond interactions. The Glide docking score for chlorinated ligand complexes was found as −4.763 kcal mol−1 (XP Gscore) for 2-chlorosyringaldehyde with XP H-bond score −0.517 kcal mol; this docked complex interacts with HIP 226 and PHE 261 amino acids with Pi–Pi stacking and H-bond (side-chain) interacting bonds. The Glide docking score was −4.723 kcal mol−1 (XP Gscore) for 5-chlorovanillin with a −0.607 XP H-bond score found; TYR 187, HIP 226, ARG 244, and PHE 261 residues recognized as active site residues were further found to have making contact with Pi–Pi stacking, H-bond (side-chain), salt bridge, and hydration site (displacement) type interactions. Moreover, the binding free energy for the docked chlorinated phenolic docked complex was predicted using prime/MM-GBSA, which gave a significant score of −55.388 kcal mol−1 for 2-chlorosyringaldehyde and −47.558 kcal mol−1 for 5-chlorovanillin.
Three amino acid residues, HIP 226, PHE 261, and THR 271, are involved in the interaction with LiP by forming PI–Pi stacking, H-bond, and hydration site (displaced) type interactions in veratryl alcohol with a Glide docking score value of −4.829 kcal mol−1 (XP Gscore). Two amino acid residues (HIP 226 and THR 271) were found to be involved in forming an interaction with syringyl alcohol with a Glide docking score value of −5.047 kcal mol−1 (XP Gscore). Sinapyl alcohol was found to have two crucial amino acid residues, HIP 226 and THR 271, for interacting by forming Pi–Pi stacking, H-bond, and hydration site (displaced) type bonding with a Glide docking score value of −5.212 kcal mol−1 (XP Gscore). Methyl hydroquinone and guaiacol reveal the same two interacting amino acid residues (HIP 226 and PHE 261), with H-bond, Pi–Pi stacking, and hydration site (displaced type) interactions, comprising a Glide docking score values of −3.735 kcal mol−1 (XP Gscore), and −4.007 kcal mol−1 (XP Gscore), respectively. Coniferyl alcohol reveals interactions with VAL 152, ASP 153, THR 209 and LYS 210 amino acid residues with H-bond (side chain) and H-bond (backbone) type interactions with a Glide docking score value of −5.312 kcal mol−1 (XP Gscore). Catechol was found to form interactions with VAL 152, THR 155 and ARG 244 residues by Pi–Pi stacking and H-bond type interactions with a Glide docking score value of −5.544 kcal mol−1 (XP Gscore). 4-Methoxy phenol was found to interact with three amino acid residues (GLN 185, HIP 226 and PHE 261) by forming Pi–Pi stacking and H-bond (side chain) type interactions with a Glide docking score value of −3.438 kcal mol−1 (XP Gscore). The polymeric lignin model compounds (dimer and trimer) were also analyzed for binding or interacting key residues with possible bond interactions. For the dimer compound, the Glide docking score value was obtained as −7.117 kcal mol−1 (XP Gscore), with two interacting amino acid residues (HIP 226 and PHE 261) by forming Pi–Pi stacking type bonding. For trimer compound docking, the Glide docking score value was obtained as −8.136 kcal mol−1 (XP Gscore), with three key interacting amino acid residues (HIP 226, VAL 227 and ARG 244), involved in Pi-cation and H-bond type bonding. We compared all docked compounds with a known control (azure-b) which seems to have a docking score value as −4.707 kcal mol−1 (XP Gscore). The trimer compound was found to have the lowest binding affinity, in contrast to the control, which indicates that the dimer and trimer compounds have significantly lower binding energy scores. The comparative results of the docked compounds have revealed in Table 5. The comparative statistical XP Gscore graph has shown in Fig. 9. However, the binding interactions of the stated ligands in 3-D view have shown in Fig. 10–12.
Table 5 Comparative docking analysis of all twelve docked lignin model compounds with a known control. The XP Gscore, XP HB score, and binding free energy value of the docked complex (LiP protein and a total of 12 lignin model compounds as ligands). The interaction profile of the ligand with its respective contact with amino-acids with possible bond interactions has also been listed. The three-dimensional structural analysis is shown in subsequent designated figures
S. no. |
Docked complex |
XP Gscore (kcal mol−1) |
XP-HB score (kcal mol−1) |
Prime/MM-GBSA (kcal mol−1) |
Interacting residues |
Interaction type |
1 |
2-Chlorosyringaldehyde |
−4.763 |
−0.517 |
−55.388 |
HIP 226, PHE 261 |
Pi–Pi stacking, H-bond (sidechain) |
2 |
5-Chlorovanillin |
−4.723 |
−0.607 |
−47.558 |
TYR 187, HIP 226, ARG 244, PHE 261 |
Pi–Pi stacking, H-bond (sidechain), salt bridge, hydration site (displacement) |
3 |
Veratryl alcohol |
−4.829 |
−0.968 |
−48.579 |
HIP 226, PHE 261, THR 271 |
Pi–Pi stacking, H-bond, hydration site (displaced) |
4 |
Syringyl alcohol |
−5.047 |
−1.16 |
−54.01 |
HIP 226, THR 271 |
Pi–Pi stacking, H-bond |
5 |
Sinapyl alcohol |
−5.212 |
−0.83 |
−44.839 |
HIP 226, THR 271 |
Pi–Pi stacking, H-bond, hydration site (displaced) |
6 |
Methyl hydroquinone |
−3.735 |
−0.35 |
−27.72 |
HIP 226, PHE 261 |
H-Bond, Pi–Pi stacking, hydration site (displaced) |
7 |
Guaiacol |
−4.007 |
−0.948 |
−33.473 |
HIP 226, PHE 261 |
Pi–Pi stacking |
8 |
Coniferyl alcohol |
−5.312 |
−0.83 |
−29.615 |
VAL 152, ASP 153, THR 209, LYS 210 |
H-Bond (sidechain), H-bond (backbone) |
9 |
Catechol |
−5.544 |
−2.88 |
−33.73 |
VAL 152, THR 155, ARG 244 |
Pi–Pi stacking, H-bond |
10 |
4-Methoxyphenol |
−3.438 |
−0.685 |
−30.035 |
GLN 185, HIP 226, PHE 261 |
Pi–Pi stacking, H-bond (side chain) |
11 |
Dimer |
−7.117 |
−0.96 |
−14.469 |
HIP 226, PHE 261 |
Pi–Pi stacking, H-bond |
12 |
Trimer |
−8.136 |
−2.499 |
−25.983 |
HIP 226, VAL 227, ARG 244 |
Pi-cation, H-bond |
* |
Control (azure-b) |
−4.707 |
−0.956 |
−62.335 |
GLU 239, ARG 244 |
Salt bridge, Pi–Pi stacking |
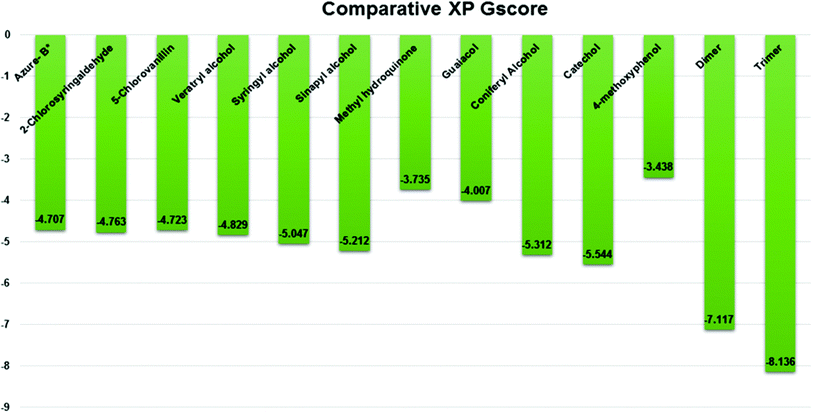 |
| Fig. 9 Comparative XP Gscore graph of the docked lignin model compounds (a total of 12). | |
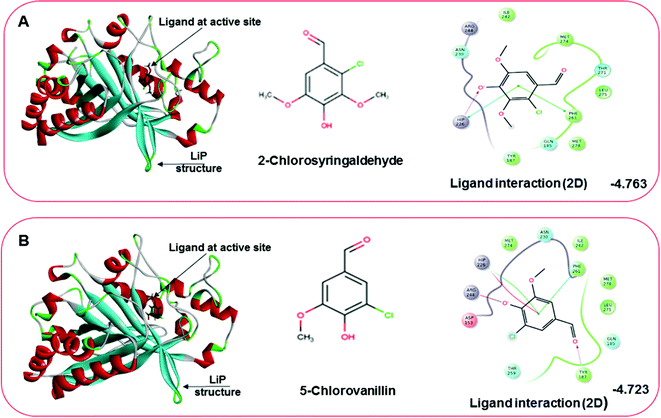 |
| Fig. 10 Docking pose of the docked chlorinated compound with binding energy. (A) Representation of docked compound as 2-chlorosyringaldehyde. The left image depicting the bound ligand at the active site, while the right-hand side depicts the 2D interactive plot ligand contacts. (B) Representation of the second chlorinated compound as 5-chlorovanillin, bound at the active site shown in the left-hand figure, while the interactive 2D ligand contacts are shown in the right-hand figure. | |
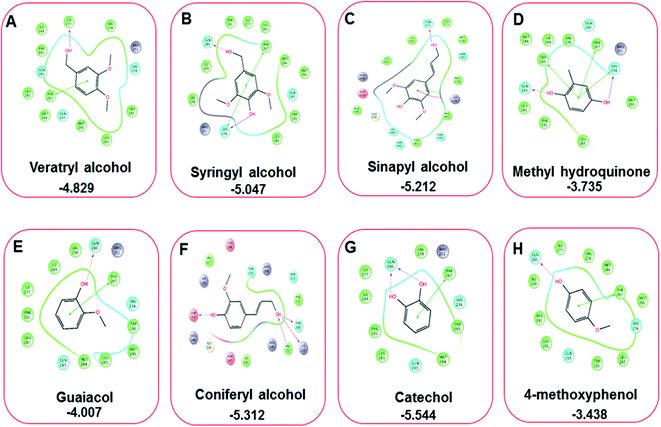 |
| Fig. 11 A comparative 2D interactive ligand contact plot of a total of eight monomeric lignin model compounds. (A–H) (Veratryl alcohol, syringyl alcohol, sinapyl alcohol, methyl hydroquinone, guaiacol, coniferyl alcohol, catechol, 4-methoxyphenol, respectively). Docked compounds have shown with the binding energy. | |
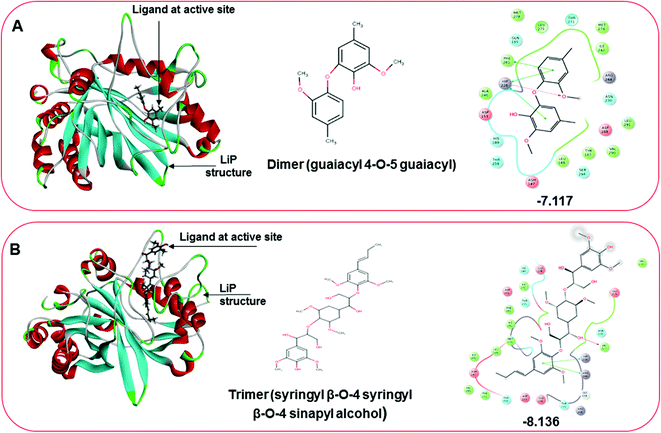 |
| Fig. 12 Docking pose of multimeric lignin model compounds. (A) The left-hand pose depicts the three-dimensional pose of the ligand (dimer-guaiacyl 4-O-5 guaiacyl) at the active site of LiP, while an interactive 2D figure of the ligand interaction is shown in the right-hand figure. (B) The left-hand pose depicts the three-dimensional pose of the ligand (trimer-syringyl β-O-4 syringyl β-O-4 sinapyl alcohol) at the active site of LiP, while an interactive 2D figure of the ligand interaction is shown in the right-hand figure. | |
Molecular dynamics simulation event analyses
The LiP and ligand (a set of a total of 12 lignin model compounds) were employed in molecular dynamics simulation to investigate the protein–ligand behaviors and interaction over the duration run of 30 ns. We analyzed all of the docked complexes rigorously for protein–ligand interactions, ligand conformation, protein ligand contacts, and also for the system potential energy score at the end of simulation run. The protein–ligand RMSD trajectory was analyzed for each complex, using simulation quality analysis (SQA), simulation event analysis (SEA) and the simulation interaction diagram (SID); such features are an in-built part of the Desmond module for calculating the energies, root-mean-square deviation (RMSD), and root-mean-square fluctuation (RMSF). SQA is a useful parameter to qualitatively validate the system stability throughout the simulated length of time for the given temperature, pressure, and volume of the total simulation. The Root Mean Square Deviation (RMSD) is used to measure the average change in displacement of a selection of atoms for a particular frame concerning a reference frame. The docked conformations of the lignin model compounds at the active sites of LiP enzymes exhibited similar molecular contacts, including hydrogen bonding (Hb) contact, hydrophobic (Ph) contact, aromatic–aromatic (Ar) contact, hydrophilic–hydrophobic (HH) contact, and acceptor–acceptor (AA) contact, which were consistently present in each docked complex. MD simulations were further assessed and evaluated for the robustness and stability of the predicted 3D structures of the LiP–lignin complexes. At the end of the 30 ns simulation, it was observed that such LiP–lignin complexes (all 12 model compounds) were stable. The RMSD for the LiP (Cα) was also found to be stable, corresponding to lignin as the ligands (Fig. 13A–L). The protein backbone became equilibrated after 30 ns with a mean RMSD value of 1.5 Å. The complexes C, E, G, I, J, K and L clearly showed the stability of the protein backbone and ligand compounds by attaining the system in an equilibrium state at the end of the simulation run. However, the lignin model compounds (A, B, D, F, and H) become stable initially, but relatively less stable at the end of the simulation run. Hydrophobic and H-bond both seemed essential points for binding of the lignin model compounds to LiP. All 12 lignin model compounds were found to have hydrophobic and H-bond type contacts during simulation, the detailed interactions of the ligand and LiP residues are depicted in Fig. 14. The chlorinated lignin model compounds (Fig. 14A and B) were found to form an ionic contact with residue HIS 226. (Fig. 16 depicts the protein–ligand contact histogram, and displays the type of protein–ligand interactions; a plot provides a timeline representation of the same contacts, which can help with visualizing the possible interactions found during the simulation run). In chlorinated complexes (2-chlorosyringaldehyde, 5-chlorovanillin), HIS 226 was found to have an ionic-type protein–ligand contact. TYR-187, HIS-226, ASN-230, ILE-242, and ARG-244 were found to form H-bond type contacts. However, hydrophobic type protein–ligand contacts were observed among TYR-187, HIS-226, PHE-261, MET-274 and LEU-275 residues.
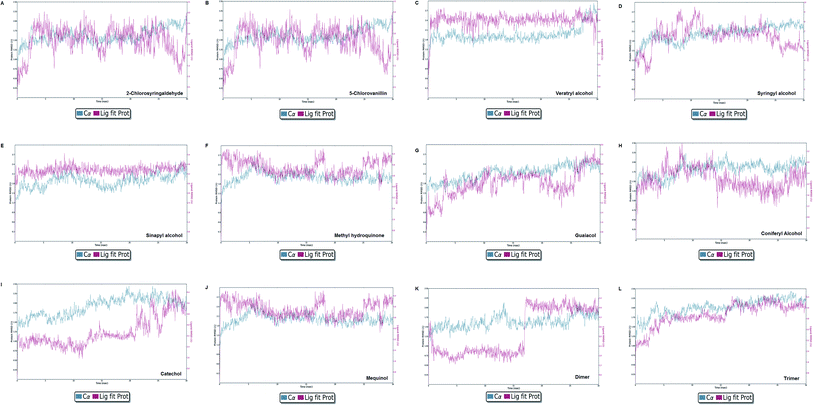 |
| Fig. 13 Protein–ligand RMSD plot of all docked complexes. The system found to be at an equilibrium state at the end of the simulation run can be seen clearly for complex C, E, G, I, J, K, and L. However, A, B, C, F, and H were found in equilibrium state initially. | |
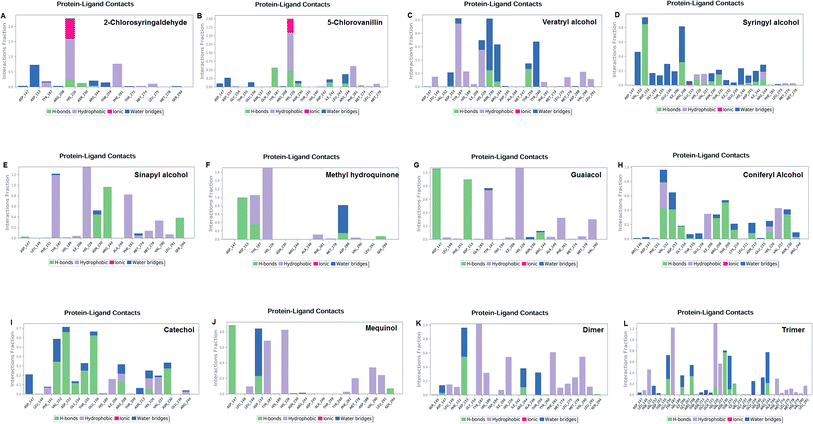 |
| Fig. 14 Protein–ligand contact plot. Protein interaction with the corresponding ligand is monitored throughout the simulation and depicts the possible four types of bond interactions. However, hydrophobic, and H-bond type contacts are crucial in all 12 lignin model compounds. | |
For all (Eight monomeric lignin model compounds), a total of 20 H-bond type interactions were found among the ASP-147, VAL-152, ASP-153, GLY-154, THR-155, GLU-156, ILE-206, ARG-208, THR-209, LYS-210, ASN-213, HIS-226, VAL-227, ASN-230, THR-231, ASP-241, ARG-244, THR-259, ASP-288 and SER-294 AA residues. Meanwhile, a total of 19 hydrophobic type contacts were found among the ASP-147, LEU-148, LEU-149, PHE-151, GLN-185, THR-187, TRP-194, HIS-189, ILE-206, HIS-226, VAL-227, ASP-245, ALA-246, PHE-261, MET-274, MET-278, LEU-275, VAL-290 and LEU-291 residues.
For multimeric lignin model compounds, a total of 11 H-bond type interactions were found involving ASP-147, ASP-153, GLU-156, ILE-206, ARG-208, HIS-226, ASN-230, THR-231, ILE-232, ARG-244, and SER-294 type residues. However, a total of 19 residues were observed to form hydrophobic interactions (SER-145, LEU-149, PHE-151, GLY-154, TYR-187, HIS-189, TRP-194, ILE-206, VAL-227, ILE-242, ALA-246, TYR-260, PHE-261, MET-274, LEU-275, MET-278, PHE-279, VAL-290 and LEU-291).
Eventually, the MD simulation results demonstrated that complexes during a simulation run were very stable. Protein–ligand binding is only feasible when the change in the system's Gibbs free energy (ΔG) is negative when the system achieves equilibrium at constant pressure and temperature. Since the extent of the ligand–protein interaction is defined by the magnitude of the negative ΔG, it could be considered to determine the stability or, alternatively, the ligand affinity to a provided acceptor. The average potential energy of all systems were also analyzed (Fig. 15). However, the maximum P_E was found for 2-chlorosyringaldehyde, which was observed as −132
506.645 kcal mol−1. Meanwhile, the minimum was observed for sinapyl alcohol as −115
583.316 kcal mol−1. The PE plots with detailed descriptions in tabular form has described in Fig. 15.
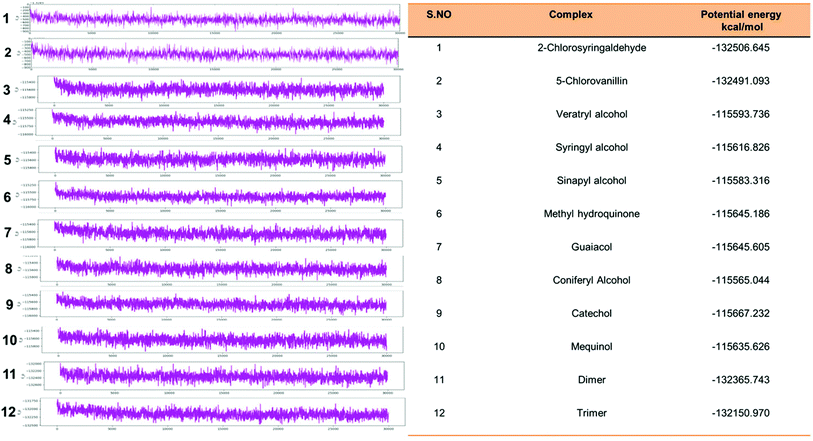 |
| Fig. 15 Potential energy plot of all docked complex systems. P_E plots with the specified calculated energy has shown with the designated figures along with the P_Energy attributes. | |
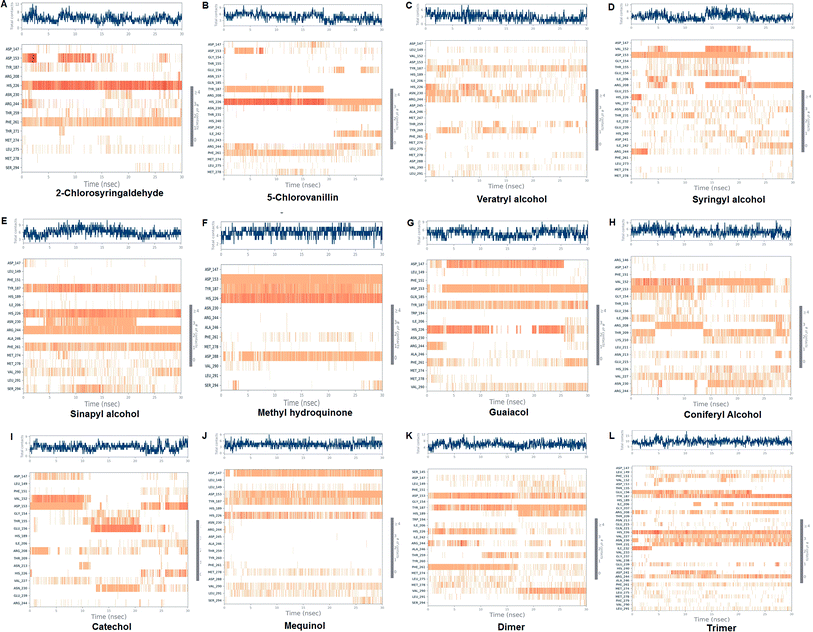 |
| Fig. 16 Protein–ligand contact histograms, displaying the type of protein–ligand interactions. Plots provide a timeline representation of the same contacts. | |
Discussion
The environmentally sustainable nature of biodegradation technology has made it even more advantageous for environmental remediation. Lignin and lignin-derivatives, phenolics, are among notable contaminants, often produced during the industrial manufacturing process, and thus as byproducts expelles from several industries. LiP-mediated biodegradation of lignin and its derivates is a most promising example. In our previous study on Bio-transformation of kraft lignin, we achieved a 65% bacterial-mediated degradation of kraft lignin with Serratia liquefaciens.9 Wet-lab studies are scarce in complete information on the interactions among AA and ligands, and even the clear-cut role of enzymes at the atomic level is still unclear. To address such flaws, to increase the catalytic/degradation efficiency of LiP, and determine the binding mechanism, virtual screening, predictive approaches, and structural analysis must be exploited in context to enhance the catalytic potential of the enzyme. Computational studies, including molecular docking and molecular dynamics simulation, are among the vital computational techniques that have been increasingly adopted in the field of predictive bioremediation.15,75 A similar ligninolytic enzyme (Laccase)-based study, exploiting several lignin model compounds, has been conducted in the last couple of years.23,77 However, bacterial-derived LiP-mediated computational studies are poorly known at present. Therefore, we conducted this study to fill the research gap with observing the binding affinity of lignin model compounds (2 chlorinated, 8 monomer, and 2 multimeric compounds) with bacterial derived LiP. To assess the protein structural and functional attributes of bacterial derived LiP, we predicted the secondary structure of bacterial LiP; protein flexibility and computational physicochemical properties were also analyzed from a catalytic point of view, we accessed the protein (LiP) interactions through docking to evaluate and understand the binding affinity with the corresponding key amino acid residues. We found the key binding interacting amino acid residues were PHE, VAL, ASP, THR, LYS, ARG and GLN as contributing residues of the active side with Pi–Pi stacking, H-bond (side-chain), salt bridge, and hydration site (displacement) type interactions. Molecular docking with Glide, the protein preparation often carried out using protein preparing wizard, that perform a notable change in specific amino acids, i.e. HIS, CYS by protonated them that lead to slightly labeling conversion in 2D results diagram, HIP for HIS, and CYX for CYS seemed to have rotamer property of amino acids. In our current study, the key findings included the protein modeling, namely comparative modeling, model flexibility predictions, comparative molecular docking, and MDS-30 ns for exploring the binding/catalysis behavior of bacterial LiP with a set of 12 lignin model compounds of 3 types (i.e., monomer, chlorinated, and multimeric). From the docking results, the binding energy has been evaluated comparatively to elucidate the binding behavior of bacterial LiP with different lignin compounds, which might be further interpreted for catalytic efficiency of the enzyme. However, to validate the docked complexes, MDS was performed, which further indicated that the complexes were in the equilibrium state at the end of the 30 ns simulation run. The ionic interactions (HIS-226) have been observed as protein–ligand contacts in the chlorinated compounds during the simulation. However, hydrophobic and H-bond type interactions were frequently observed in the remaining 10 lignin model compounds. The potential energy was also measured after the end of the simulation run. We observed maximum P_E in 2-chlorosyringaldehyde, which was observed as −132
506.645 kcal mol−1. Meanwhile, the minimum P_E was observed for sinapyl alcohol as −115
583.316 kcal mol−1.
In recognition and support of our present research, we picked a set of 12 lignin model compounds in 3 flavor; 2 chlorinated compounds (2-chlorosyringaldehyde, 5-chlorovanillin). A set of 8 standard monomeric lignin model compounds (veratryl alcohol, syringyl alcohol, sinapyl alcohol, methyl hydroquinone, guaiacol, coniferyl alcohol, catechol, 4-methoxyphenol), and 2 multimeric lignin model compounds in β-O-4, and 4-O-5 linkage form (dimer and trimer) were used to carry out the docking study, followed by validation of the docked complexes through DESMOND- assisted high performance molecular dynamics simulation (MDS). Awasthi et al., (2014) conducted a similar study, but laccase instead of LiP was used for the docking study, followed by MDS with GROMACS 4.5.5 package for evaluation of the degradation behavior of lignin with laccase.76 Awasthi et al. (2014) reported laccase and several lignin model compounds (four lignin model compounds; monomer, dimer, trimer, tetramer) for catalysis behavious, in a subsequent study, a total of 11 amino acid residues of fungal laccase were found to interact with the lignin model compounds (LEU185, ASP227, ASN229, PHE260, SER285, PHE286, GLY413, ALA414, PRO415, ILE476, and HIS479). Subsequently, laccases with higher redox potential phenylalanine were found at the active site in the laccase as the key binding residue to bind with lignin model compounds, which is related to the findings currently being made. From a contrasting perspective, our study revealed a total of 9 amino acid residues (VAL-152, ASP-153, GLN-185, TYR-187, LYS-210, HIS-226, ARG-244, PHE-261, and THR-271) interacting with a total of 12 lignin model compounds.
Another similar molecular modeling based study (molecular docking, and MDS) for understanding the binding/catalysis mechanism at the atomic level has been reported exploiting laccase and a set of 5 lignin model compounds (2,6-dimethoxyphenol, ferulic acid, guaiacol, sinapic acid, and vanillyl alcohol).78 In that subsequent study, Chen et al. (2015) performed laccase-mediated predictive biodegradation (ligninolytic enzyme) exploiting 5 lignin model compounds, which were docked, and simulations of 10 ns or 10
000 ps were carried out to understand the catalysis potential of laccase. Subsequently, 7 hydrophobic amino acid residues (PHE, PRO, ALA, GLY, ASP, and PHE) were found to interact during the docking with lignin model compound 2,6-dimethoxyphenol.77 LEU, SER, PRO, PRO, PHE, and ALA were reported as hydrophobic interactions with feluric acid. ASP, ILE, SER, ARG, GLN, and THR for guaiacol. PRO, GLY, ASP, ASN, ILE, PRO, PHE, ALA, and ASN for sinapic acid. However, such research lacked the ligand conformation, rigorous protein–ligand contacts analysis and potential energy of the system.
Conclusion
This present study explored the molecular basis of bacterial derived LiP through structural and molecular modeling analysis. A total of 12 different lignin model compounds have been used to evaluate the binding/catalytic affinity with LiP. We assessed the structure and PPs of bacterial-derived LiP exploiting secondary structure prediction for constituent elementary component analysis. Comparative modeling has shown that the query sequence coincides with the highest identity of DyP-type peroxidase, suggesting that the model enzyme involved is identical to the DyP-type peroxidase. The binding energy score was found to be the lowest in the trimer compound in comparison to the control compound. Furthermore, MDS indicated the equilibrium states of the docked complexes. Such binding affinity can be interpreted to understand the biodegradation mechanism of lignin model compounds at the atomic level, which further indicates that LiP has significant potential to catalyze several types of lignin and phenolic model compounds. In order to gain a deep understanding of the catalytic efficiency of bacterial-derived LiP, both molecular docking and subsequent wet-lab studies must be carried out. High potential LiP, with effective strategies, could play a crucial role in developing engineered microorganisms (GEMs) for mitigation of lignin, and lignin-derived pollutants from the environment. Such approaches would help significantly in the field of bioremediation and other miscellaneous applications at the industrial level.
Author contributions
All listed authors have made significantl contribution to this work, however, AKS contributed to designing, performing and analyzing the LiP mediated in silico study, the design and preparation of selected lignin model compounds, LiP optimization, model building, comparative modeling, structural & functional prediction, table preparation, and drew all of the figures used in this manuscript. Moreover, AKS developed this manuscript by using, and compiling the data derived from computational experimentations. The author KSK performed XP-Glide-Docking, DESMOND-assisted simulation study on their under licensed workstation (Schrodinger-Maestro Suite) and confirmed and validated the final docking, and simulations results. AU evaluated and analyzed the results for binding interactions, simulations interactions and finally approved the results to be used in this manuscript. AR edited the manuscript, proofread, and approved the contents before submission in RSC-Advances.
Conflicts of interest
All listed authors confirm that there is no conflict of interest for this work.
Acknowledgements
The author AKS thanks the University Grant Commission (UGC) New Delhi, for financial support. The Academy of Scientific & Innovative Research (AcSIR) (An Institute of National Importance) Ghaziabad-201002, India is thankfully acknowledged. We also thank the Director of the CSIR-Indian Institute of Toxicology Research (IITR), Lucknow, India, for his encouragement and support. Author AR acknowledges Department of Biotechnology (DBT), Government of India, New Delhi, for partially supporting this work (Grant No. BT/PR20460/BCE/8/1386/2016). The authors KSK and AU are also thankful to the Department of Biotechnology (DBT), Ministry of Science and Technology for providing the Bioinformatics facility through BTISNet programme (BT/BI/25/001/2006). Author KSK is highly thankful to DBT for sanctioning Junior Research Fellowship and Senior Research Fellowship by DBT (BT/BI/25/037/2012 [BIF-SVIMST]). This manuscript no. (CSIR-IITR, manuscript communication record) is 3672.
References
- R. Vanholme, B. Demedts, K. Morreel, J. Ralph and W. Boerjan, Plant Physiol., 2010, 153, 895–905 CrossRef CAS PubMed.
- M. S. Ganewatta, H. N. Lokupitiya and C. Tang, Polymers, 2019, 11, 1176 CrossRef PubMed.
- A. Lourenço, J. Rencoret, C. Chemetova, J. Gominho, A. Gutiérrez, J. C. del Río and H. Pereira, Frontiers in Plant Science, 2016, 7, 1612 CrossRef PubMed.
- D. Tarasov, M. Leitch and P. Fatehi, Biotechnol. Biofuels, 2018, 11, 269 CrossRef PubMed.
- M. Erfani Jazi, G. Narayanan, F. Aghabozorgi, B. Farajidizaji, A. Aghaei, M. A. Kamyabi, C. M. Navarathna and T. E. Mlsna, SN Appl. Sci., 2019, 1, 1094 CrossRef CAS.
- J. Deng, T. Xiong, H. Wang, A. Zheng and Y. Wang, ACS Sustainable Chem. Eng., 2016, 4, 3750–3756 CrossRef CAS.
- V. Kumar, I. S. Thakur and M. P. Shah, in Microbial Bioremediation & Biodegradation, ed. M. P. Shah, Springer Singapore, Singapore, 2020, pp. 1–48, DOI:10.1007/978-981-15-1812-6_1.
- N. P. Cheremisinoff and P. E. Rosenfeld, in Handbook of Pollution Prevention and Cleaner Production, ed. N. P. Cheremisinoff and P. E. Rosenfeld, William Andrew Publishing, Oxford, 2010, pp. 179–259, DOI:10.1016/B978-0-08-096446-1.10006-1.
- A. K. Singh, P. Yadav, R. N. Bharagava, G. D. Saratale and A. Raj, Front. Microb., 2019, 10, 2364 CrossRef PubMed.
- A. K. Singh, M. Bilal, H. M. N. Iqbal, A. S. Meyer and A. Raj, Sci. Total Environ., 2021, 145988, DOI:10.1016/j.scitotenv.2021.145988.
- F. J. Ruiz-Dueñas and A. T. Martínez, Microb. Biotechnol., 2009, 2, 164–177 CrossRef PubMed.
- G. Janusz, A. Pawlik, J. Sulej, U. Świderska-Burek, A. Jarosz-Wilkołazka and A. Paszczyński, FEMS Microbiol. Rev., 2017, 41, 941–962 CrossRef CAS PubMed.
- K. K. Pandey and A. J. Pitman, J. Polym. Sci., Part A: Polym. Chem., 2004, 42, 2340–2346 CrossRef CAS.
- A. Singh, P. Chowdhary and A. Raj, in Contaminants and Clean Technologies, ed. A. Raj and P. Chowdhary, CRC Press, 2020, p. 348, DOI:10.1201/9780429275852.
- A. K. Singh, P. Chowdhary and A. Raj, in Microorganisms for Sustainable Environment and Health, ed. P. Chowdhary, A. Raj, D. Verma and Y. Akhter, Elsevier, 2020, pp. 249–285, DOI:10.1016/B978-0-12-819001-2.00013-9.
- A. K. Singh and A. Raj, Environ. Sci. Eur., 2020, 32, 107 CrossRef.
- P. Ortiz-Bermudez, K. C. Hirth, E. Srebotnik and K. E. Hammel, Proc. Natl. Acad. Sci., 2007, 104, 3895–3900 CrossRef CAS PubMed.
- O. Rubilar, M. C. Diez and L. Gianfreda, Crit. Rev. Environ. Sci. Technol., 2008, 38, 227–268 CrossRef CAS.
- A. B. McKague, D. W. Reeve and F. Xi, Nord. Pulp Pap. Res. J., 1995, 10, 114 CrossRef CAS.
- W. Deng, H. Zhang, X. Wu, R. Li, Q. Zhang and Y. Wang, Green Chem., 2015, 17, 5009–5018 RSC.
- Y. Wang, Q. Wang, J. He and Y. Zhang, Green Chem., 2017, 19, 3135–3141 RSC.
- J. O. Romero, E. Fernández-Fueyo, F. Avila-Salas, R. Recabarren, J. Alzate-Morales and A. T. Martínez, Comput. Struct. Biotechnol. J., 2019, 17, 1066–1074 CrossRef CAS PubMed.
- M. Awasthi, N. Jaiswal, S. Singh, V. P. Pandey and U. N. Dwivedi, J. Biomol. Struct. Dyn., 2015, 33, 1835–1849 CrossRef CAS PubMed.
- M. Francesca Gerini, D. Roccatano, E. Baciocchi and A. D. Nola, Biophys. J., 2003, 84, 3883–3893 CrossRef CAS PubMed.
- S. Besombes and K. Mazeau, Biopolymers, 2004, 73, 301–315 CrossRef CAS PubMed.
- G. de Gonzalo, D. I. Colpa, M. H. Habib and M. Fraaije, J. Biotechnol., 2016, 236, 110–119 CrossRef CAS PubMed.
- P. Dvořák, P. I. Nikel, J. Damborský and V. de Lorenzo, Biotechnol. Adv., 2017, 35, 845–866 CrossRef PubMed.
- L. Pollegioni, F. Tonin and E. Rosini, FEBS J., 2015, 282, 1190–1213 CrossRef CAS PubMed.
- A. O. Falade, U. U. Nwodo, B. C. Iweriebor, E. Green, L. V. Mabinya and A. I. Okoh, MicrobiologyOpen, 2017, 6, e00394 CrossRef PubMed.
- K. Min, T. Yum, J. Kim, H. M. Woo, Y. Kim, B.-I. Sang, Y. J. Yoo, Y. H. Kim and Y. Um, Biotechnol. Biofuels, 2017, 10, 212 CrossRef PubMed.
- A. K. Singh, M. Bilal, H. M. N. Iqbal and A. Raj, Int. J. Biol. Macromol., 2021, 177, 58–82 CrossRef CAS PubMed.
- A. O. Falade, O. A. L. Eyisi, L. V. Mabinya, U. U. Nwodo and A. I. Okoh, Biotechnol. Rep., 2017, 16, 12–17 CrossRef PubMed.
- L. T. M. Pham, S. J. Kim and Y. H. Kim, Biotechnol. Biofuels, 2016, 9, 247 CrossRef PubMed.
- D. W. S. Wong, Appl. Biochem. Biotechnol., 2009, 157, 174–209 CrossRef CAS PubMed.
- D. W. Wong, Appl. Biochem. Biotechnol., 2009, 157, 174–209 CrossRef CAS PubMed.
- F. J. Ruiz-Dueñas and Á. T. Martínez, Microb. Biotechnol., 2009, 2, 164–177 CrossRef PubMed.
- T. Vangeel, W. Schutyser, T. Renders and B. F. Sels, Top. Curr. Chem., 2018, 376, 30 CrossRef PubMed.
- P. W. Rose, A. Prlić, A. Altunkaya, C. Bi, A. R. Bradley, C. H. Christie, L. D. Costanzo, J. M. Duarte, S. Dutta, Z. Feng, R. K. Green, D. S. Goodsell, B. Hudson, T. Kalro, R. Lowe, E. Peisach, C. Randle, A. S. Rose, C. Shao, Y.-P. Tao, Y. Valasatava, M. Voigt, J. D. Westbrook, J. Woo, H. Yang, J. Y. Young, C. Zardecki, H. M. Berman and S. K. Burley, Nucleic Acids Res., 2016, 45, D271–D281 Search PubMed.
- H. Berman, K. Henrick, H. Nakamura and J. L. Markley, Nucleic Acids Res., 2007, 35, D301–D303 CrossRef CAS PubMed.
- T. L. Poulos, S. L. Edwards, H. Wariishi and M. H. Gold, J. Biol. Chem., 1993, 268, 4429–4440 CrossRef CAS.
- N. Kunishima, K. Fukuyama, H. Matsubara, H. Hatanaka, Y. Shibano and T. Amachi, J. Mol. Biol., 1994, 235, 331–344 CrossRef CAS PubMed.
- W. Blodig, A. T. Smith, W. A. Doyle and K. Piontek, J. Mol. Biol., 2001, 305, 851–861 CrossRef CAS PubMed.
- H. Berman, K. Henrick and H. Nakamura, Nat. Struct. Mol. Biol., 2003, 10, 980 CrossRef CAS PubMed.
- R. Singh, J. C. Grigg, W. Qin, J. F. Kadla, M. E. Murphy and L. D. Eltis, ACS Chem. Biol., 2013, 8, 700–706 CrossRef CAS PubMed.
- R. Shrestha, G. Huang, D. A. Meekins, B. V. Geisbrecht and P. Li, ACS Catal., 2017, 7, 6352–6364 CrossRef CAS PubMed.
- M. Ahmad, J. N. Roberts, E. M. Hardiman, R. Singh, L. D. Eltis and T. D. Bugg, Biochemistry, 2011, 50, 5096–5107 CrossRef CAS PubMed.
- X. Wang, B. Yao and X. Su, Int. J. Mol. Sci., 2018, 19, 3373 CrossRef PubMed.
- M. Sugiura, H. Hirai and T. Nishida, FEMS Microbiol. Lett., 2003, 224, 285–290 CrossRef CAS.
- X. Qin, H. Luo, X. Zhang, B. Yao, F. Ma and X. Su, Biotechnol. Biofuels, 2018, 11, 302 CrossRef CAS PubMed.
- K. Min, G. Gong, H. M. Woo, Y. Kim and Y. Um, Sci. Rep., 2015, 5, 8245 CrossRef CAS PubMed.
- G. K. Parshetti, S. Parshetti, D. C. Kalyani, R.-a. Doong and S. P. Govindwar, Ann. Microbiol., 2012, 62, 217–223 CrossRef CAS.
- E. Vignali, F. Tonin, L. Pollegioni and E. Rosini, Appl. Microbiol. Biotechnol., 2018, 102, 10579–10588 CrossRef CAS PubMed.
- C. R. Taylor, E. M. Hardiman, M. Ahmad, P. D. Sainsbury, P. R. Norris and T. D. Bugg, J. Appl. Microbiol., 2012, 113, 521–530 CrossRef CAS PubMed.
- A. K. Singh, M. Bilal, H. M. N. Iqbal and A. Raj, Sci. Total Environ., 2021, 770, 144561 CrossRef CAS PubMed.
- ProtParam, https://web.expasy.org/protparam/, (accesed on March-10-2021) Search PubMed.
- E. Gasteiger, C. Hoogland, A. Gattiker, M. R. Wilkins, R. D. Appel and A. Bairoch, in The proteomics protocols handbook, Springer, 2005, pp. 571–607 Search PubMed.
- J. Reeb and B. Rost, in Encyclopedia of Bioinformatics and Computational Biology, ed. S. Ranganathan, M. Gribskov, K. Nakai and C. Schönbach, Academic Press, Oxford, 2019, pp. 488–496, DOI:10.1016/B978-0-12-809633-8.20267-7.
- R. Santhoshkumar and A. Yusuf, J. Genet. Eng. Biotechnol., 2020, 18, 24 CrossRef CAS PubMed.
- D. W. A. Buchan and D. T. Jones, Nucleic Acids Res., 2019, 47, W402–W407 CrossRef CAS PubMed.
- L. J. McGuffin, K. Bryson and D. T. Jones, Bioinformatics, 2000, 16, 404–405 CrossRef CAS PubMed.
- C. Geourjon and G. Deléage, Bioinformatics, 1995, 11, 681–684 CrossRef CAS PubMed.
- D. Milburn, R. A. Laskowski and J. M. Thornton, Protein Eng., 1998, 11, 855–859 CrossRef CAS PubMed.
- R. A. Laskowski, J. Jabłońska, L. Pravda, R. S. Vařeková and J. M. Thornton, Protein Sci., 2018, 27, 129–134 CrossRef CAS PubMed.
- N. R. Coordinators, Nucleic Acids Res., 2016, 44, D7–D19 CrossRef PubMed.
- NCBI, https://www.ncbi.nlm.nih.gov/nuccore/CP002272.1, (accesed on March-10-21) Search PubMed.
- K. M. Deangelis, P. D'Haeseleer, D. Chivian, J. L. Fortney, J. Khudyakov, B. Simmons, H. Woo, A. P. Arkin, K. W. Davenport, L. Goodwin, A. Chen, N. Ivanova, N. C. Kyrpides, K. Mavromatis, T. Woyke and T. C. Hazen, Stand. Genomic Sci., 2011, 5, 69–85 CrossRef CAS PubMed.
- T. Schwede, J. Kopp, N. Guex and M. C. Peitsch, Nucleic Acids Res., 2003, 31, 3381–3385 CrossRef CAS PubMed.
- A. Waterhouse, M. Bertoni, S. Bienert, G. Studer, G. Tauriello, R. Gumienny, F. T. Heer, T. A. P. de Beer, C. Rempfer, L. Bordoli, R. Lepore and T. Schwede, Nucleic Acids Res., 2018, 46, W296–W303 CrossRef CAS PubMed.
- R. W. Hooft, G. Vriend, C. Sander and E. E. Abola, Nature, 1996, 381, 272 CrossRef CAS PubMed.
- R. A. Laskowski, M. W. MacArthur, D. S. Moss and J. M. Thornton, J. Appl. Crystallogr., 1993, 26, 283–291 CrossRef CAS.
- I. W. Davis, A. Leaver-Fay, V. B. Chen, J. N. Block, G. J. Kapral, X. Wang, L. W. Murray, W. B. Arendall III, J. Snoeyink, J. S. Richardson and D. C. Richardson, Nucleic Acids Res., 2007, 35, W375–W383 CrossRef PubMed.
- C. J. Williams, J. J. Headd, N. W. Moriarty, M. G. Prisant, L. L. Videau, L. N. Deis, V. Verma, D. A. Keedy, B. J. Hintze, V. B. Chen, S. Jain, S. M. Lewis, W. B. Arendall III, J. Snoeyink, P. D. Adams, S. C. Lovell, J. S. Richardson and D. C. Richardson, Protein Sci., 2018, 27, 293–315 CrossRef CAS PubMed.
- M. Jamroz, A. Kolinski and S. Kmiecik, Nucleic Acids Res., 2013, 41, W427–W431 CrossRef PubMed.
- P. Ortiz-Bermúdez, K. C. Hirth, E. Srebotnik and K. E. Hammel, Proc. Natl. Acad. Sci., 2007, 104, 3895 CrossRef PubMed.
- A. K. Singh, P. Chowdhary and A. Raj, in Contaminants and Clean Technologies, CRC Press, 2020, pp. 65–91 Search PubMed.
- M. Awasthi, N. Jaiswal, S. Singh, V. P. Pandey and U. N. Dwivedi, J. Biomol. Struct. Dyn., 2015, 33, 1835–1849 CrossRef CAS PubMed.
- M. Chen, G. Zeng, Z. Tan, M. Jiang, H. Li, L. Liu, Y. Zhu, Z. Yu, Z. Wei, Y. Liu and G. Xie, PLoS One, 2011, 6, e25647 CrossRef CAS PubMed.
- M. Chen, G. Zeng, C. Lai, J. Li, P. Xu and H. Wu, RSC Adv., 2015, 5, 52307–52313 RSC.
- S. Kim, P. A. Thiessen, E. E. Bolton, J. Chen, G. Fu, A. Gindulyte, L. Han, J. He, S. He, B. A. Shoemaker, J. Wang, B. Yu, J. Zhang and S. H. Bryant, Nucleic Acids Res., 2016, 44, D1202–D1213 CrossRef CAS PubMed.
- Marvin, https://chemaxon.com/products/marvin Search PubMed.
- F. S. Archibald, Appl. Environ. Microbiol., 1992, 58, 3110–3116 CrossRef CAS PubMed.
- R. A. Friesner, R. B. Murphy, M. P. Repasky, L. L. Frye, J. R. Greenwood, T. A. Halgren, P. C. Sanschagrin and D. T. Mainz, J. Med. Chem., 2006, 49, 6177–6196 CrossRef CAS PubMed.
- J. R. Greenwood, D. Calkins, A. P. Sullivan and J. C. Shelley, J. Comput.-Aided Mol. Des., 2010, 24, 591–604 CrossRef CAS PubMed.
- J. C. Shelley, A. Cholleti, L. L. Frye, J. R. Greenwood, M. R. Timlin and M. Uchimaya, J. Comput.-Aided Mol. Des., 2007, 21, 681–691 CrossRef CAS PubMed.
- D. Shivakumar, J. Williams, Y. Wu, W. Damm, J. Shelley and W. Sherman, J. Chem. Theory Comput., 2010, 6, 1509–1519 CrossRef CAS PubMed.
- K. M. Elokely and R. J. Doerksen, J. Chem. Inf. Model., 2013, 53, 1934–1945 CrossRef CAS PubMed.
- R. A. Friesner, J. L. Banks, R. B. Murphy, T. A. Halgren, J. J. Klicic, D. T. Mainz, M. P. Repasky, E. H. Knoll, M. Shelley, J. K. Perry, D. E. Shaw, P. Francis and P. S. Shenkin, J. Med. Chem., 2004, 47, 1739–1749 CrossRef CAS PubMed.
- B. K. Kumar, Faheem, K. V. G. C. Sekhar, R. Ojha, V. K. Prajapati, A. Pai and S. Murugesan, J. Biomol. Struct. Dyn., 2020, 1–24, DOI:10.1080/07391102.2020.1824814.
- S. Tahlan, S. Kumar, K. Ramasamy, S. M. Lim, S. A. A. Shah, V. Mani and B. Narasimhan, BMC Chem., 2019, 13, 90 CrossRef PubMed.
- S. K. Katari, P. Natarajan, S. Swargam, H. Kanipakam, C. Pasala and A. Umamaheswari, Journal of receptor and signal transduction research, 2016, 36, 558–571 CrossRef CAS PubMed.
- D. Shivakumar, J. Williams, Y. Wu, W. Damm, J. Shelley and W. Sherman, J. Chem. Theory Comput., 2010, 6, 1509–1519 CrossRef CAS PubMed.
- E. Harder, W. Damm, J. Maple, C. Wu, M. Reboul, J. Y. Xiang, L. Wang, D. Lupyan, M. K. Dahlgren, J. L. Knight, J. W. Kaus, D. S. Cerutti, G. Krilov, W. L. Jorgensen, R. Abel and R. A. Friesner, J. Chem. Theory Comput., 2016, 12, 281–296 CrossRef CAS PubMed.
- A. K. J. Bowers, E. Chow, H. Xu, R. O. Dror, M. P. Eastwood, B. A. Gregersen, J. L. Klepeis, I. Kolossvary, M. A. Moraes and F. D. Sacerdoti, et al., Presented in part at the Proceedings of the 2006 ACM/IEEE conference on Supercomputing, Tampa, Florida, 2006 Search PubMed.
- H. M. Berman, J. Westbrook, Z. Feng, G. Gilliland, T. N. Bhat, H. Weissig, I. N. Shindyalov and P. E. Bourne, Nucleic Acids Res., 2000, 28, 235–242 CrossRef CAS PubMed.
|
This journal is © The Royal Society of Chemistry 2021 |
Click here to see how this site uses Cookies. View our privacy policy here.