DOI:
10.1039/D1RA04195A
(Paper)
RSC Adv., 2021,
11, 23607-23615
Experimental design approaches to optimize ultrasound-assisted simultaneous-silylation dispersive liquid–liquid microextraction for the rapid determination of parabens in water samples†
Received
30th May 2021
, Accepted 30th June 2021
First published on 6th July 2021
Abstract
This work describes a rapid solvent-minimized process to effectively determine four common paraben preservatives (methyl-, ethyl-, propyl- and butyl-paraben) in surface water samples. The method involved the use of a combination of a novel ultrasound-assisted simultaneous-silylation within dispersive liquid–liquid microextraction (UASS-DLLME) with detection by gas chromatography-tandem mass spectrometry (GC-MS/MS). To overcome the challenges related to the different experimental conditions, multivariate experimental design approaches conducted by means of a multilevel categorical design and a Box–Behnken design were utilized to screen and optimize parameters that have significant influences on the efficiency of silylation and extraction. The method was then validated and shown to provide low limits of quantitation (LOQs; 1–5 ng L−1), high precision (3–11%), and satisfactory mean spiked recoveries (accuracy; 79–101%). Upon analyzing samples of surface water obtained from the field, we found that, in total, there was a relatively high concentration of the target parabens ranging from 200 to 1389 ng L−1. The sources of the elevated levels of these parabens may be from the release of untreated municipal wastewater in this region, and also due to the widespread application of parabens in personal care and food products.
1. Introduction
Paraben derivatives are a group of alkyl esters of p-hydroxybenzoic acid, and are commonly used as antimicrobial agents in cosmetics, pharmaceutical and personal care products (PPCPs), and food products.1 Their wide application suggests that they may have long-lasting effects on the environment and that humans may be exposed to relatively elevated levels of these compounds. In the last two decades, there has been increasing awareness of the effects of paraben derivatives on mammalian endocrine processes, since exposure to these derivatives and their subsequent increased concentration in the body can result in reproductive problems and even cancer.2,3 Paraben residues had been identified in aquatic related samples all over the world, such as in surface water, wastewater, swimming pool water, sediments, and aquatic biota, all of which have been reviewed by Piao et al.4 and Ocaña-González et al.5 It is more worrisome that they have also been detected in breast milk, human blood and urine.6–9 Due to the deficiency in wastewater treatment, especially in rural provinces and towns, throughout the Asia-Pacific region, this is an urgent issue that needs to be addressed because wastewater that is directly discharged into the aquatic environment can cause an extensive contamination of surface water. The growing release of such residues into our environment and the potential long-term adverse effects required to develop a reliable, simple, and accurate analytical method to identify their occurrence and fate in aquatic environments.
Various extraction techniques have been adopted to determine parabens residues in aqueous samples, as extensively reviewed elsewhere.4,5,10 Solid-phase extraction (SPE) is the most commonly used method for extracting parabens from aqueous samples but, unfortunately, this procedure frequently involves long extraction times, and cartridge blocking problems often occur. Solvent-free extraction methods, such as solid-phase microextraction (SPME) and stir bar sorptive extraction (SBSE) have also been used, but these protocols can be costly and time-consuming, with additional issues regarding fragile coating layers and carryover problems. Dispersive liquid–liquid microextraction (DLLME), compared to SPE, SPME and SBSE, it has the benefits of being convenient, rapid, carryover-free, and cost-effective. DLLME has been used successfully to isolate and determine parabens residues in wastewater and tap water samples.10 Gas chromatography-mass spectrometry (GC-MS), GC-tandem mass spectrometry (GC-MS/MS), and liquid chromatography combined with tandem mass spectrometry (LC-MS/MS) are among the commonly used techniques for the detection of parabens residues in various matrices.4,5,10 Nowadays, applications of LC-MS/MS for the detection of PPCPs and various pesticides have been increasingly reported, but GC-MS or GC-MS/MS continues to be the technique of choice to detect these compounds due to its superior separation characteristics, low matrix effect, high distinguishing power and availability.11 A post-derivatization step is usually employed to improve GC separation and sensitivity by increasing the volatility of hydroxylated analytes, but with the drawbacks to this approach include the need for heating the reaction mixture (above 60 °C) and increasing the length of time needed (at least 30 min) to complete the analysis. To increase sample throughput, the in situ or simultaneous derivatization of compounds in aqueous samples combined with DLLME has been extensively reviewed by Sajid.12
An ultrasound-assisted DLLME has been developed to accelerate the formation of a finely dispersed mixture and to increase the rate of mass transfer of analytes from the aqueous phase to the extraction solvent, as described and reviewed by Albero et al.13 The aim of the present study was to develop a simple and straightforward ultrasound-assisted simultaneous-silylation within dispersive liquid–liquid microextraction (UASS-DLLME) for the rapid extraction of selected parabens in surface water samples before their analysis by GC-MS/MS. Compared to previous studies, the novelty and objective of this study was to optimize the UASS-DLLME parameters by using dual multivariate experimental design approaches instead of the commonly used one-factor-at-a-time protocol in an effort to improve the method optimization steps. These approaches can minimize the number of experiments needed, optimize factors together, and reduce overall cost.14 In this study, the chemicals for use as extractants, dispersants and silylation that could affect USAA-DLLME were first screened and selected by a Factorial Multilevel Categoric Design (FMLCD), and then Box–Behnken Design (BBD) was employed to determine and quantify the optimum points. The accuracy and precision of the procedure were then evaluated and the overall method validated. Finally, analysis of water samples collected from the environment by the developed method was carried out to prove its practicality and applicability for the determination of selected parabens at the trace-level.
2. Materials and methods
2.1. Reagents, chemicals and standards
All reagents, chemicals and standards are commercially available and were used without further purification. The purities of the target standards (i.e., methyl-, ethyl-, propyl- and butyl-paraben) were all greater than 98%, and their structures and properties are summarized in Table S1.† Detailed information regarding reagents, chemicals and target standards are provided in the ESI.†
2.2. Real water samples collection
Two sets of surface water samples were collected: (1) samples from campus ditches at National Central University (n = 3, pH 6.5–6.8; specific conductance: 627–641 µS cm−1), those received wastewater from dormitory laundries and showers, and (2) samples from the Lao-Jie River (n = 8, pH 6.3–7.2; specific conductance: 300–2290 µS cm−1). The Lao-Jie River, a typical river in northern Taiwan, is a 36.7 km long river that originates in the hillside terrace regions of Tao-Yuan County. It runs through two major industrial parks with a variety of textiles, dyeing and chemical industries. It also passes through Chung-Li city, which houses about half-a-million residents. The Lao-Jie River receives runoff from both industrial wastewater treatment plants and untreated municipal wastewater directly from houses in the city and surrounding suburbs. The sampling sites and locations on the Lao-Jie River are shown in Fig. S1.† Duplicate samples (250 mL for each) were collected and transported on ice to the laboratory, where they were filtered to remove the particulate matter, and then stored at 4 °C.
2.3. UASS-DLLME procedure
The UASS-DLLME procedure was accomplished under optimal conditions: a 10 mL aliquot of a water sample (containing 0.5 g of sodium chloride) was placed in a 15 mL screw capped glass tube with a conical bottom. Acetone (600 µL) as a dispersant, 20 µL of tetrachloroethylene (as an extractant), and 20 µL of MTBSTFA (as a silylating agent) were mixed and rapidly injected into the aqueous sample. A cloudy suspension was formed containing fine droplets of the extractant. The glass tube was immediately immersed in a preheated (60 °C) ultrasonic bath (Bransonic 5210, Branson, Brookfield, CT, USA) for 2 min to facilitate the simultaneous silylation of the target analytes in the fine droplets and to increase the extraction efficiency. Separation of the emulsion phases was done via centrifugation at 5000 rpm (maximum rate) for 10 min. The organic sedimented phase (10 µL) was withdrawn by a syringe, and the target TBDMS-derivatives were subsequently detected using GC-MS and GC-MS/MS techniques.
2.4. Instrument analysis
The determination and quantification of target analytes involved the use of a Varian 450 GC coupled with a Varian 220 ion-trap mass spectrometer system (Walnut Creek, CA, USA) set to electron ionization (EI) mode. The final extractant (10 µL) was directly introduced into the GC injection-port via a ChromatoProbe (Varian) device. The temperature of the injection-port was kept at 90 °C for 1.5 min to vaporize the solvent, and the temperature was then rapidly increased to 300 °C to introduce the TBDMS-derivatives into the analytical column. The target TBDMS-derivatives were separated on a DB-5MS capillary column (30 m × 0.25 mm i.d., 0.25 µm film thickness) from Agilent Technologies (Santa Clara, CA, USA). The GC oven temperature was programed as follows: 70 °C for 4 min; ramping the temperature 10 °C min−1 up to 270 °C, and holding at 270 °C for 2 min. Transfer line, manifold, ion source, and ion trap temperatures were maintained at 280, 40, 180 and 160 °C, respectively. The conditions of full-scan EI spectra were set to the following parameters: mass range 100–500 m/z, scan time 1 s, solvent delay 15 min, emission current 10 µA (at 70 eV electron energy), and an automatic gain control target set at 20
000. For tandem-in-time MS/MS analysis, the scan time was set at 1.0 s, the amplitude range for resonant collision-induced dissociation (CID) was from 1.0 to 1.5 V, and the excitation time set at 25 ms. A three-step method was used to evaluate and optimize the MS/MS operating conditions for each precursor ion at their corresponding resonant CID conditions.15 The target TBDMS-derivatives produced characteristic fragmentation patterns that represented mainly [M]+ and [M − 57]+ (i.e., [M − C(CH3)3]+) fragments, and [M − 57]+ ion is dominant on the mass spectrum. Therefore, the molecular ions of TBDMS-derivatives were selected as the precursor ions, and their intense characteristic transitions [M − 57]+ were applied as product ions for identification and quantification. Table 1 presents an overview of the retention times, the MS/MS detection characteristics of precursor ions and product ions for the target TBDMS-derivatives.
Table 1 MS/MS detection characteristics, retention time, calibration range, linearity, LOD and LOQ
Analytea |
RT (min) |
Precursor ion (m/z) [M]+ |
Product ion (m/z) [M − C(CH3)3]+ |
Calibration range (ng L−1) |
r2 |
Mandel test F-valueb |
LOD (ng L−1) |
LOQ (ng L−1) |
Analyte is as the TBDMS-derivative. Fcritical = 10.13 at the 95% confidence level.LOD: limits of detection; LOQ: limits of quantification. |
MeP |
17.05 |
266.1 |
209.3 |
5–1000 |
0.9968 |
0.53 |
2.0 |
5.0 |
EtP |
17.83 |
280.1 |
223.4 |
2–500 |
0.9986 |
2.98 |
0.3 |
1.0 |
PrP |
18.90 |
294.2 |
237.3 |
2–500 |
0.9990 |
2.96 |
0.3 |
1.0 |
BuP |
19.95 |
308.2 |
251.3 |
2–500 |
0.9989 |
0.89 |
0.3 |
1.0 |
2.5. Statistic experimental design
Statistic experimental designs were performed via Factorial Multilevel Categoric Design (FMLCD) and Box–Behnken Design (BBD) using the Design-Expert 10.0.3 software (Stat-Ease, Inc.; Minneapolis, MN, USA). FMLCD is a type of factorial design that is primarily used to screen for the most influential chemicals for the extractants, dispersants and silylation out of many possibilities that could have affected the efficiency of the UASS-DLLME. The Box–Behnken Design (BBD), one of the most commonly used response surface methodologies, was applied to perform method optimization.16 For FMLCD, the three major non-numeric categories were: the extractant (i.e., carbon tetrachloride, tetrachloroethylene and chlorobenzene); the dispersant (i.e., ethanol, propanol and acetone); the silylating agent (i.e., MTBSTFA and BSTFA). For BBD, the three major factors, considered as independent variables, were (A) volume of the extractant, (B) volume of the dispersant, and (C) percentage of sodium chloride added.
3. Results and discussion
3.1. Optimization of UASS-DLLME
3.1.1. Screening design: FMLCD. Several factors could potentially affect UASS-DLLME extraction efficiency, such as the type and volume of the extractant, the dispersant, and the silylating agent being used, as well as the ratio of extractant to dispersant. To enhance the extraction efficiency, the extractant should have a high extraction capability for the target analytes, a low solubility in water, and a density that is higher than that of water. Furthermore, to enhance the efficiency of the formation of an emulsion when the extractant is injected into the water sample, the dispersant should be miscible with both the organic extractant and water. Based on previous studies and availability involving water sample pretreatment using various DLLME techniques, carbon tetrachloride, tetrachloroethylene and chlorobenzene were found to be frequently used as extractants, and are excellent extractants for extracting polar and hydroxylated micropollutants from water samples.12,17–19 Regarding the dispersant, ethanol, propanol and acetone are commonly mixed with an extractant to increase extraction efficiency. MTBSTFA and BSTFA are two commonly used silylating agents for hydroxylated analytes that are applied to increase the detection sensitivity of GC-MS or GC-MS/MS.FMLCD with 2 or 3 non-numeric categories was first employed to select which type of chemicals to use, as mentioned above (also as listed in Table 2) and that would increase the efficiency of the USAA-DLLME. Table 2 displays all of the experiments of the design domain (18 runs, fully randomized) with their corresponding experimental results (illustrated as total peak area). Preliminary ANOVA results indicated that the model, the extractant, the dispersant, and the silylating agent were all statistically significant at the 95% confidence level for FMLCD (Table S2†). As shown in “Run 7” in Table 2, the highest total peak area (refer to “the best extraction efficiency”) was obtained when the extractant and the dispersant were tetrachloroethylene and acetone, respectively, and MTBSTFA was used as the silylating agent. These results are consistent with our previous findings, in which tetrachloroethylene and acetone were used to successfully extract various salicylate and benzophenone-type UV filters from aqueous samples by a UA-DLLME procedure.20 However, in the present work, MTBSTFA and not BSTFA provided the optima derivatization results, likely due to the fact that MTBSTFA is a highly reactive reagent and its TBDMS-derivatives are relative stable in the presence of water.21 Although MTBSTFA is unstable in aqueous solutions, it would be compatible with the DLLME procedure if the kinetics of the derivatization and extraction of the hydroxylated parabens were faster than the decomposition of MTBSTFA.20,22
Table 2 Experimental factors and categories for factorial multilevel categoric design matrix, and results as total peak area for water samples pretreated by UASS-DLLMEa
Factor |
Categorical-1 |
Categorical-2 |
Categorical-3 |
CCl4: carbon tetrachloride; C2Cl4: tetrachloroethylene; BzCl: chlorobenzene; BSTFA: N,O-bis(trimethylsilyl)trifluoroacetamide; MTBSTFA: N-methyl-(tert-butyldimethylsilyl)-trifluoroacetamide. |
Extractant (A) |
CCl4 |
C2Cl4 |
BzCl |
Dispersant (B) |
Ethanol |
Propanol |
Acetone |
Silylating agent (C) |
BSTFA |
MTBSTFA |
|
Run |
Categorical-1 extractant (A) |
Categorical-2 dispersant (B) |
Categorical-3 silylating agent (C) |
Total peak area (×106) |
1 |
BzCl |
Ethanol |
BSTFA |
4.98 |
2 |
CCl4 |
Propanol |
BSTFA |
5.32 |
3 |
CCl4 |
Propanol |
MTBSTFA |
9.05 |
4 |
BzCl |
Acetone |
BSTFA |
12.17 |
5 |
BzCl |
Ethanol |
MTBSTFA |
6.42 |
6 |
C2Cl4 |
Ethanol |
MTBSTFA |
14.58 |
7 |
C2Cl4 |
Acetone |
MTBSTFA |
28.05 |
8 |
C2Cl4 |
Propanol |
BSTFA |
18.57 |
9 |
CCl4 |
Acetone |
BSTFA |
13.64 |
10 |
CCl4 |
Acetone |
MTBSTFA |
17.28 |
11 |
CCl4 |
Ethanol |
BSTFA |
4.18 |
12 |
C2Cl4 |
Acetone |
BSTFA |
20.18 |
13 |
BzCl |
Propanol |
MTBSTFA |
7.18 |
14 |
C2Cl4 |
Propanol |
MTBSTFA |
14.57 |
15 |
BzCl |
Acetone |
MTBSTFA |
17.56 |
16 |
C2Cl4 |
Ethanol |
BSTFA |
8.42 |
17 |
CCl4 |
Ethanol |
MTBSTFA |
6.56 |
18 |
BzCl |
Propanol |
BSTFA |
5.41 |
3.1.2. Optimization design: BBD. The volumes of tetrachloroethylene and acetone were next further optimized by BBD coupled with the 3D response surface plots. It was previously reported that mixing the same volumes of the silylating agent and extractant with the dispersant provides the best derivatization and extraction results.20,22 Therefore, the third factor that was selected for BBD optimization was the percentage of salt added (for salting-out effect) instead of the volume of silylating agent used. Three levels of these factors were: the volume of tetrachloroethylene (15, 20 and 30 µL), the volume of acetone (400, 600 and 800 µL), and the percentage of sodium chloride added (1, 5 and 10%). Table 3 displays all of the experiments of the design domain (15 runs, fully randomized, containing three replicates of the central points) with their corresponding results represented as the total peak area. The results of ANOVA show that the model, the volume of tetrachloroethylene (A) and the volume of acetone (B) were statistically significant at the 95% confidence level (as summarized in Table S3†). The F-value for “Lack-of-Fit” was insignificant, which confirmed that the model fit the response variables with a near-perfect prediction at the 95% confidence level. Hereafter, the quadratic polynomial equation to predict the extraction efficiency in terms of actual factors is as follows:
Y = 2.69 × 107 − 2.52 × 106A + 1.42 × 106B − 1.06 × 106C + 1.22 × 106AB + 9.27 × 104AC + 1.64 × 106BC − 4.63 × 106A2 − 4.18 × 106B2 − 2.96 × 106C2 |
where Y is the total peak area of the four parabens, A is the volume of tetrachloroethylene, B is the volume of acetone, and C is the percentage of sodium chloride added. The recoveries (%) of individual spiked target analyte for each experimental condition of BBD are listed in Table S4.† According to the different experimental conditions, the recoveries ranged from 43% to 95%. Upon evaluation of the experimental results and the prediction of desirability function, the following experimental conditions would be expected to provide optimal results: tetrachloroethylene (as the extractant) 20 µL, acetone (as the dispersant) 600 µL, and salt (sodium chloride) addition 5%.
Table 3 Experimental results based on the Box–Behnken design for UASS-DLLME and their extraction efficiency presented as total peak area
Run |
A: C2Cl4 (µL) |
B: acetone (µL) |
C: salt added (%) |
Total peak area (×106) |
1 |
20 |
600 |
5 |
27.68 |
2 |
20 |
800 |
1 |
20.52 |
3 |
30 |
800 |
5 |
19.34 |
4 |
20 |
600 |
5 |
26.58 |
5 |
30 |
600 |
10 |
15.21 |
6 |
15 |
800 |
5 |
20.23 |
7 |
30 |
400 |
5 |
13.88 |
8 |
15 |
600 |
1 |
23.47 |
9 |
20 |
800 |
10 |
20.82 |
10 |
15 |
400 |
5 |
19.12 |
11 |
20 |
400 |
10 |
16.24 |
12 |
15 |
600 |
10 |
22.15 |
13 |
20 |
600 |
5 |
27.61 |
14 |
30 |
600 |
1 |
16.31 |
15 |
20 |
400 |
1 |
22.66 |
The trends for the most important parameters can be evaluated and depicted by the 3D response surface plots, which were estimated from the BBD for each pair of independent variables. Fig. 1(a) shows the response surface obtained when the volume of tetrachloroethylene is plotted against the volume of acetone with a fixed percentage (5%) of sodium chloride added; Fig. 1(b) illustrates the response surface developed for the volume of tetrachloroethylene and the percentage of sodium chloride added, with the volume of acetone fixed at 600 µL; and Fig. 1(c) displays the response surface obtained as a function of the volume of acetone and the percentage of sodium chloride added, for 20 µL of tetrachloroethylene.
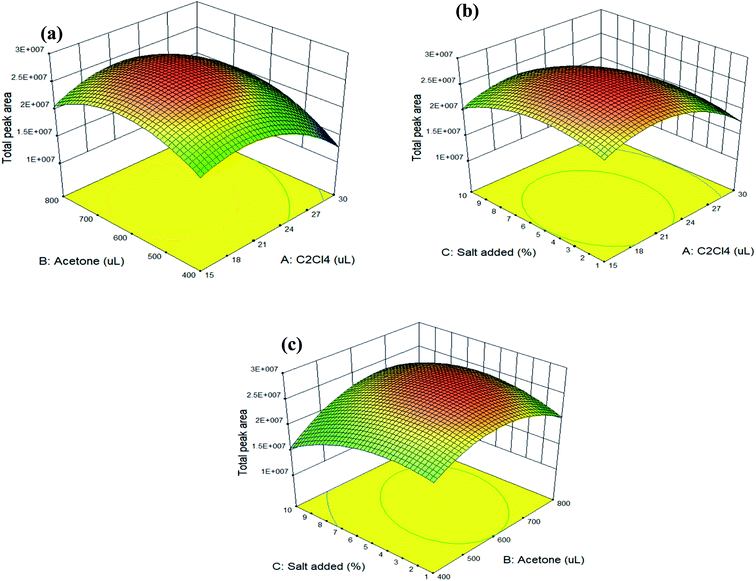 |
| Fig. 1 Response surface plots of the total peak area for target analytes estimated from the BBD for each pair of independent variables: (a) volume of tetrachloroethylene vs. volume of acetone; (b) volume of tetrachloroethylene vs. percentage of sodium chloride added; (c) volume of acetone vs. percentage of sodium chloride added. Experimental conditions: a 10 mL water sample (containing 0.5 g sodium chloride) was placed in a 15 mL glass tube, and a mixture of 600 µL of acetone, 20 µL of tetrachloroethylene, and 20 µL of MTBSTFA was then rapidly injected into the water sample. A cloudy suspension was formed, and the solution was sonicated for 2 min in a preheated (60 °C) ultrasonic bath. The phases were then separated by centrifugation at 5000 rpm for 10 min. | |
These collective results indicate that the optimum conditions for extracting these four parabens from aqueous samples by the UASS-DLLME technique were the rapid injection of a mixture, which was comprised of 20 µL of tetrachloroethylene (extractant), 600 µL of acetone (dispersant), and 20 µL of MTBSTFA (silylating agent), into 10 mL of water (containing 0.5 g of sodium chloride) in a glass tube with a conical bottom. After ultrasonication for 2.0 min (at 60 °C) and centrifugation at 5000 rpm for 10 min, the sedimented phase 10 µL was directly introduced into the GC-MS/MS. The spiked recovery, as calculated under the optimized conditions, ranged from 81 to 101% with an average of 92 ± 6% (as listed in Table 4: average spiked recovery of intra-day for two spiked concentrations).
Table 4 Accuracy and precision of the method
Analytes |
Intra-day |
Inter-day |
5 ng L−1 |
500 ng L−1 |
5 ng L−1 |
500 ng L−1 |
Average spiked recovery (accuracy, %, intra-day n = 5 and inter-day n = 20). Relative standard deviation (RSD) of spiked recovery is given in parentheses (precision, %, intra-day n = 5 and inter-day n = 20). |
MeP |
81a (11)b |
101a (5)b |
79a (9)b |
98a (4)b |
EtP |
91 (7) |
92 (3) |
88 (7) |
93 (3) |
PrP |
89 (6) |
101 (5) |
83 (9) |
96 (5) |
BuP |
92 (9) |
91 (4) |
90 (8) |
93 (5) |
3.2. Validation of the developed method
To evaluate the performance of the UASS-DLLME, the linearity, limits of detection (LODs), limits of quantification (LOQs), precision, accuracy, selectivity, carryover effect and matrix effect of the developed method were validated based on protocols that were previously reported elsewhere.23,24 All experimental data was obtained under the optimum conditions.
The quantification of the target analytes was calculated from the six-level calibration curves, as indicated by the response factors, covering the range from 5 to 1000 ng L−1 for MeP, and from 2 to 500 ng L−1 for the other three target parabens (Table 1), each divided by the fixed concentration of an internal standard (i.e., p-terphenyl-d14). The precision of the calibration curve, as indicated by the relative standard deviation (RSD) of response factors, was 5, 7, 6 and 7% for MeP, EtP, PrP and BuP, respectively. The coefficients of determination (r2) exceeded 0.9968 for all four target analytes (Table 1). The linearity was also confirmed by a Mandel's fitting test.25 As shown in Table 1, all of the calculated F-values were lower than the tabulated limit (Ftab = 10.13) at a 95% confidence level, thus confirming that the linear regression fits the experimental data better than quadratic regression.
The LODs and LOQs of the developed method were experimentally calculated as the concentration for each target analyte as its corresponding TBDMS-derivative on the basis of the signal-to-noise (S/N) ratio of 3 and 10, respectively. The values for the LODs were 0.3–2.0 ng L−1, and values of the LOQs were 1.0–5.0 ng L−1 (Table 1). The results imply that trace amounts (i.e., at parts per trillion level) of target parabens could be easily extracted and determined in environmental water samples by using UASS-DLLME coupled with GC-MS/MS.
Intra-day and inter-day analyses were used to evaluate the precision, accuracy and repeatability of the method. Intra-day precision was determined by analyzing five spiked “River-6” water samples at two concentration levels (20 ng L−1 and 500 ng L−1) on the same day (n = 5). Inter-day precision was evaluated by conducting replicates on five consecutive days (n = 20). Table 4 shows that the precisions (RSDs) of intra- and inter-day analysis were 11% or less, and the accuracy (as the average spiked recovery) ranged from 79 to 101%.
To assess selectivity, non-spiked and spiked real water samples were analyzed, and no interfering peaks were observed at or close to the retention times (RT) of the target parabens, as shown in Fig. S2.† These GC-MS/MS chromatograms indicate that excellent selectivity can be achieved and that the target analytes could be easily identified through their RTs. Furthermore, no carryover effect of the thermally degraded components from the sample matrix in the injection-port was detected. This can be attributed to the use of the ChromatoProbe device with a disposable micro-vial.
The matrix effect was evaluated by the “recovery function” procedure with spiking the standards to final extracts from the pool river water samples. The recovery function was calculated by plotting the “found concentration” versus result obtained from the standard calibration curves (six-level) for each target analyte, as described elsewhere.25 The calculated slope and intercept of the recovery function was then compared with the unity and zero by a t-test (with confidence intervals at 95%). For this study, the t-calculated values ranged from 0.67 to 1.23, which were all in the confidence interval of the t-tabulated value (±t(95%,df=6) = ± 2.447) (Table 5). This is an indication that compared to results obtained using standard calibration curves, the procedure of spiking the standards to the final extracts from the pool river water samples did not significantly alter the final results.25 Therefore, the accurate quantitative determination of the four target parabens in surface water samples can be achieved due to the absence of significant matrix effect.
Table 5 Concentrations (ng L−1) of selected target parabens detected in water samples by using UASS-DLLME coupled GC-MS/MS
Samples |
MeP |
EtP |
PrP |
BuP |
Detected average concentration (n = 3). Standard deviation of the detected concentration (n = 3). Pool river water samples were used to evaluated the matrix effect at 95% confidence interval, and ttab value is ±t(95%,df=6) = ±2.447.n.d.: not detected. |
Campus ditch-1 |
182a ± 7b |
136a ± 6b |
707a ± 14b |
272a ± 8b |
Campus ditch-2 |
95 ± 6 |
67 ± 5 |
97 ± 6 |
99 ± 8 |
Campus ditch-3 |
78 ± 6 |
n.d. |
432 ± 11 |
111 ± 8 |
River-1 |
176 ± 5 |
63 ± 5 |
n.d. |
56 ± 6 |
River-2 |
274 ± 11 |
n.d. |
266 ± 11 |
310 ± 9 |
River-3 |
n.d. |
71 ± 8 |
476 ± 11 |
147 ± 7 |
River-4 |
114 ± 5 |
93 ± 9 |
123 ± 7 |
467 ± 7 |
River-5 |
634 ± 6 |
n.d. |
620 ± 7 |
135 ± 12 |
River-6 |
98 ± 7 |
n.d. |
102 ± 13 |
n.d. |
River-7 |
211 ± 16 |
n.d. |
253 ± 10 |
86 ± 13 |
River-8 |
143 ± 11 |
n.d. |
66 ± 12 |
86 ± 10 |
tcal-value for matrix effectc |
1.23 |
0.67 |
1.02 |
0.91 |
Overall, the satisfactory precision and accuracy on the method demonstrate that the UASS-DLLME coupled with GC-MS/MS detection is capable of achieving excellent repeatability, reproducibility, sensitivity and selectivity for the identification and quantification of the four target parabens in surface water samples.
3.3. Parabens in water samples
The developed method was then used for the analysis of surface water samples collected from campus ditches and the Lao-Jie River (as described in Section 2.2). As shown in Table 5, the total concentrations of the four target parabens varied from 358 to 1297 ng L−1 in campus ditches water samples, and ranged from 200 to 1389 ng L−1 in river water samples. MeP, PrP and BuP were quantitatively determined in nearly all of the water samples, while EtP was detected at low concentrations with a relatively low detection frequency (45%) in this study. For the campus ditch samples, the highest total concentration (1297 ng L−1) was detected in “Campus ditch-1”, which was collected from the wastewater outlet of shower and laundry from the women's dormitory. For river water samples, the highest total concentration (1389 ng L−1) was found in “River-5”, which received untreated municipal wastewater directly from Chung-Li city. Fig. S2† demonstrates typical GC-MS/MS chromatograms for (a) non-spiked, and (b) spiked “River-5” water samples.
Table 6 compares results obtained in this study to those reported in previous studies. It should be noted that the concentrations of parabens measured in our study are higher than those found in surface water samples from some European countries (i.e., Switzerland, Portugal, United Kingdom) and United State.26–29 Nevertheless, our data is similar to the values for surface water samples collected in Poland, Japan, India, and China (i.e., from Beijing and Pearl River Delta).30–34 Moreover, the highest concentrations of these compounds were detected in the São Paulo state of Brazil, which have concentration ranges in the high µg L−1 levels.35 This disparity reveals that surface waters in developing countries may have comparable or levels of parabens residues higher than those detected in many more developed countries, where PPCP are more effectively removed in sewage or wastewater treatment plants since their plants are regulated, monitored and maintained routinely. We could speculate that, as such, higher levels could be expected in countries like Taiwan due to the local priorities disregarding wastewater processing, and the lack of advanced municipal or industrial wastewater treatment facilities.
Table 6 Comparison of our results (ng L−1) with previous reported of parabens in various surface water samples in the different countriesa
Sampling area, country |
MeP |
EtP |
PrP |
BuP |
References |
n.d.: not detected; n.q.: not quantified. |
Chung-Li, Taiwan |
n.d.–630 |
n.d.–130 |
n.d.–710 |
n.d.–460 |
This study |
Düebendorf, Switzerland |
3.1–17 |
<0.3–1.6 |
<0.5–5.8 |
<0.2–2.8 |
26 |
Ria de Aveiro estuary, Portugal |
<1.6–62 |
<0.3–6.7 |
<0.5–64 |
<0.2–42 |
27 |
South Wales, United Kingdom |
<0.3–400 |
<0.5–15 |
<0.2–24 |
<0.3–52 |
28 |
Greater Pittsburgh area, United States |
2.2–17.3 |
n.d. |
n.d.–12 |
n.d.–0.2 |
29 |
Tamilnadu, India |
n.d.–22.8 |
2.5–147 |
n.d.–57 |
n.q. |
32 |
Greater Poland Voivodeship, Poland |
1.7–1598 |
0.8–27.5 |
0.5–93.9 |
0.6–22.6 |
30 |
Tokushima and Osaka, Japan |
25–676 |
<1.3–64 |
<0.8–207 |
<0.6–163 |
31 |
Beijing, China |
0.8–920 |
n.d.–294 |
n.d.–595 |
n.d.–41.5 |
33 |
Pearl River Delta, China |
n.q.–1062 |
n.d. |
n.q.–3142 |
n.d. |
34 |
São Paulo state, Brazil |
n.d.–275 000 |
<800–30 500 |
<500–52 100 |
<800–19 900 |
35 |
3.4. Methods comparison
Table 7 lists a comparison between our UASS-DLLME method and previously reported methods for sample pretreatment and detection. Compared with the UASS-DLLME, the SPE procedures required more than 40 to 200 min (depending on the SPE flow rate, sample volume and SPE manifold setup), and more than 15 mL of organic solvents were used for cartridge elution.30,32 For solvent-free microextraction methods, more than 20 min was needed for the SPME,36 and 60 min for the SBSE.37 The LOQs of UASS-DLLME coupled with GC-MS/MS are similar to, or even lower than those reported by using other microextraction methods coupled with GC-MS(/MS), and even LC-MS/MS (Table 7), moreover, the accuracy and precision were comparable to those for previously reported methods. Furthermore, in situ or simultaneous derivatization was applied to improve the GC-MS or GC-MS/MS detection and to increase the throughput of sample analysis, SPE with post-silylation required heating at 70 °C and 30 min was needed to reach completion.32 Although, compared with LC-ESI-MS/MS and LC-ESI-TOF-MS,30,38 a derivatization step was required in our method, the outstanding features of our procedure was the use of trace amounts of extraction solvent (20 µL), less sample volume (10 mL), and the fact that the benefits of the DLLME technique (such as speed, simplicity, and efficiency) were fully exploited.
Table 7 Comparison of our developed method with previous studies of surface water samplesa
Extraction method |
Sample volume |
Time required |
Detection method |
Derivatization |
Spiked recovery (%) |
Precision (%RSD) |
LOQ (ng L−1) |
References |
UASS-DLLME: ultrasound-assisted simultaneous-silylation dispersive liquid–liquid microextraction; SPE: solid-phase extraction; HS-SPME: head-space solid-phase microextraction; SBSE: stir-bar sorptive extraction; RDSE: rotating disk sorptive extraction; TD: thermal desorption. LOQ: limits of quantification. Off-line post-MSTFA silylation at 70 °C for 30 min was applied for the study. |
UASS-DLLME |
10 mL |
<15 min |
GC-MS/MS |
Yes |
79–101% |
<11% |
1.0–5.0 |
This study |
SPE |
1000 mL |
200 min + elution time |
GC-MS |
Yesb |
81–98% |
<4% |
1.5–1.8 |
32 |
SPE |
100 mL |
40 min + elution time |
LC-ESI-MS/MS |
No |
98–107% |
<2.1% |
0.4–2.1 |
30 |
HS-SPME |
10 mL |
<20 min |
GC-MS/MS |
Yes |
85–102% |
<10% |
4–17 |
36 |
SBSE |
5 mL |
60 min |
TD-GC-MS |
Yes |
45–105% |
<9.4% |
3.6–13.7 |
37 |
RDSE |
20 mL |
70 min |
LC-ESI-TOF-MS |
No |
60–67% |
<9.7% |
27–55 |
38 |
4. Conclusions
In this study, we reported on a simple and straightforward technique in which UASS-DLLME coupled with GC-MS/MS was used to detect selected parabens residues in various surface water samples. We successfully applied dual multivariate experimental designs to optimize the different working conditions in order to improve the extraction performance. These designs proved to be an efficient way to identify and optimize a set of parameters to achieve a realistic result due to its benefits such as allowing multiple response optimizations, identifying the factor interactions, and requiring a minimum number of experimental runs. Ultrasonic technique was utilized in the acceleration of both the rate of mass transfer of DLLME and the silylation reactions, which allowed for the silylation to be fully integrated into the extraction of parabens from aqueous samples. The method was comprehensively validated by evaluating the linearity, LODs, LOQs, accuracy, precision and selectivity of the method, and was confirmed to be suitable for the rapid determination of target parabens at trace-levels (ng L−1) in surface water. Although the amount of samples analyzed was limited, our preliminary results indicate that parabens, in part, due to their extensive use, have widely contaminated our aquatic environments. The results further reveal that the sources of the elevated levels of parabens residues in our samples may be from the release of untreated municipal wastewater in this region due to the lack of local municipal wastewater treatment plants. From this study, UASS-DLLME is proved as a method that has many advantages, and promises to be a viable option for use in routine analysis. Currently, environmental monitoring programs have been implemented throughout Taiwan to collect further information on the occurrence and fate of parabens residues in our aquatic environment.
Author contributions
Chi-Zhong Hsieh: data curation, methodology, draft preparation. Wu-Hsun Chung: software, visualization, validation. Wang-Hsien Ding: conceptualization, supervision, writing – reviewing and editing.
Conflicts of interest
The authors declare that they have no conflict of interest.
Acknowledgements
This study was supported by a grant from the National Science Council of Taiwan under contract No. NSC MOST 108-2113-M-008-002. We thank the Instrumental Center of National Central University for instrumental support. We also thank Mr James Ding, who checked over the English and grammar usage within this manuscript.
References
- M. G. Soni, I. G. Carabin and G. A. Burdock, Food Chem. Toxicol., 2005, 43, 985–1015 CrossRef CAS.
- R. Golden, J. Gandy and G. Vollmer, Crit. Rev. Toxicol., 2005, 35, 435–458 CrossRef CAS PubMed.
- R. S. Tavares, F. C. Martins, P. J. Oliveira, J. Ramalho-Santos and F. P. Peixoto, Reprod. Toxicol., 2009, 27, 1–7 CrossRef CAS PubMed.
- C. Piao, L. Chen and Y. Wang, J. Chromatogr. B: Anal. Technol. Biomed. Life Sci., 2014, 569, 138–148 Search PubMed.
- J. A. Ocaña-González, M. Villar-Navarro, M. Ramos-Payán, R. Fernández-Torres and M. A. Bello-López, Anal. Chim. Acta, 2015, 858, 1–15 CrossRef.
- A. Azzouz, A. J. Rascón and E. Ballesteros, J. Pharm. Biomed. Anal., 2016, 119, 16–26 CrossRef CAS.
- E. P. Hines, P. Mendola, O. S. von Ehrenstein, X. Ye, A. M. Calafat and S. E. Fenton, Reprod. Toxicol., 2015, 54, 120–128 CrossRef CAS.
- N. Raza, K. H. Kim, M. Abdullah, W. Raza and R. J. C. Brown, Trends Anal. Chem., 2018, 98, 161–173 CrossRef CAS.
- H. Y. Zhou, H. C. Chen and W. H. Ding, J. Pharm. Biomed. Anal., 2018, 150, 469–473 CrossRef CAS PubMed.
- T. Grześkowiak, B. Czarczyńska-Goślińska and A. Zgoła-Grześkowiak, Trends Anal. Chem., 2016, 75, 209–226 CrossRef.
- Y. Pico, A. H. Alfarhan and D. Barcelo, Trends Anal. Chem., 2020, 122, 115720 CrossRef CAS.
- M. Sajid, Trends Anal. Chem., 2018, 106, 169–182 CrossRef CAS.
- B. Albero, J. L. Tadeo and R. A. Pérez, Trends Anal. Chem., 2019, 118, 739–750 CrossRef CAS.
- S. T. Narenderan, S. N. Meyyanathan and V. V. S. Reddy Karri, Food Chem., 2019, 289, 384–395 CrossRef CAS.
- D. Y. Tsai, C. L. Chen and W. H. Ding, Food Chem., 2014, 154, 211–216 CrossRef CAS.
- S. L. C. Ferreira, R. E. Bruns, H. S. Ferreira, G. D. Matos, J. M. David, G. C. Brandão, E. G. P. da Silva, L. A. Portugal, P. S. dos Reis, A. S. Souza and W. N. L. dos Santos, Anal. Chim. Acta, 2007, 597, 179–186 CrossRef CAS.
- M. Saraji and M. K. Boroujeni, Anal. Bioanal. Chem., 2014, 406, 2027–2066 CrossRef CAS.
- M. Sajid and K. Alhooshani, Trends Anal. Chem., 2018, 108, 167–182 CrossRef CAS.
- E. G. Primel, S. S. Caldas, L. C. Marube and A. L. V. Escarrone, Trends Anal. Chem., 2017, 14, 1–18 CrossRef CAS.
- J. W. Wu, H. C. Chen and W. H. Ding, J. Chromatogr. A, 2013, 1302, 20–27 CrossRef CAS PubMed.
- C. Schummer, O. Delhomme, B. M. R. Appenzeller, R. Wennig and M. Millet, Talanta, 2009, 77, 1473–1482 CrossRef CAS PubMed.
- R. Montes, I. Rodríguez, E. Rubí and R. Cela, J. Chromatogr. A, 2009, 1216, 205–210 CrossRef CAS.
- N. Pérez-Lemus, R. López-Serna, S. I. Pérez-Elvira and E. Barrado, Chemosphere, 2020, 258, 127273 CrossRef.
- Y. T. Ao, Y. C. Chen and W. H. Ding, J. Hazard. Mater., 2021, 401, 123383 CrossRef CAS PubMed.
- J. M. Chen, C. C. Yang, W. H. Chung and W. H. Ding, RSC Adv., 2016, 6, 96510–96517 RSC.
- N. Jonkers, H. P. E. Kohler, A. Dammshäuser and W. Giger, Environ. Pollut., 2009, 157, 714–723 CrossRef CAS.
- N. Jonkers, A. Sousa, S. Galante-Oliveira, C. M. Barroso, H. P. E. Kohler and W. Giger, Environ. Sci. Pollut. Res., 2010, 17, 834–843 CrossRef CAS.
- B. Kasprzyk-Hordern, R. E. Dinsdale and A. J. Guwy, Water Res., 2008, 42, 3498–3518 CrossRef CAS.
- L. Renz, C. Volz, D. Michanowicz, K. Ferrar, C. Christian, D. Lenzner and T. El-Hefnawy, Ecotoxicology, 2013, 22, 632–641 CrossRef CAS PubMed.
- B. Czarczyńska-Goślińska, A. Zgoła-Grześkowiak, M. Jeszka-Skowron, R. Frankowski and T. Grześkowiak, J. Environ. Manage., 2017, 204, 50–60 CrossRef.
- H. Yamamoto, I. Tamura, Y. Hirata, J. Kato, K. Kagota and S. Katsuki, Sci. Total Environ., 2011, 410–411, 102–111 CrossRef CAS.
- B. R. Ramaswamy, G. Shanmugam, G. Velu, B. Rengarajan and D. G. J. Larsson, J. Hazard. Mater., 2011, 186, 1586–1593 CrossRef CAS PubMed.
- W. Li, L. Gao, Y. Shi, Y. Wang, J. Liu and Y. Cai, Sci. Total Environ., 2016, 539, 262–270 CrossRef CAS.
- X. Peng, Y. Yu, C. Tang, J. Tan, Q. Huang and Z. Wang, Sci. Total Environ., 2008, 397, 158–166 CrossRef CAS PubMed.
- C. A. Galinaro, F. M. Pereira and E. M. Vieira, J. Braz. Chem. Soc., 2015, 26, 2205–2213 CAS.
- J. Regueiro, E. Becerril, C. Garcia-Jares and M. Llompart, J. Chromatogr. A, 2009, 1216, 4693–4702 CrossRef CAS PubMed.
- A. M. C. Ferreira, M. Möder and M. E. F. Laespada, Anal. Bioanal. Chem., 2011, 399, 945–953 CrossRef.
- M. Becerra-Herrera, V. Miranda and P. Richter, Anal. Sci., 2020, 36, 675–679 CrossRef CAS PubMed.
Footnote |
† Electronic supplementary information (ESI) available. See DOI: 10.1039/d1ra04195a |
|
This journal is © The Royal Society of Chemistry 2021 |
Click here to see how this site uses Cookies. View our privacy policy here.