DOI:
10.1039/D1TA02791C
(Paper)
J. Mater. Chem. A, 2021,
9, 19914-19921
A phase-change gel based pressure sensor with tunable sensitivity for artificial tactile feedback systems†
Received
2nd April 2021
, Accepted 3rd June 2021
First published on 3rd June 2021
Abstract
The somatosensory system in the skin plays an essential role for human hands to perform adaptive interactions with external environments, such as tactile sensing and handling objects. For artificial pressure sensors, the realization of high sensitivity and a broad sensing range to emulate biological tactile receptors is desirable but challenging. Herein, we report a novel strategy to design a phase-change-gel (PC-gel)-based pressure sensor with tunable sensitivity and sensing ranges. By tuning the Young's modulus of the PC-gel through a phase change, the pressure sensor can switch between a high sensitivity mode with a low limit of detection (2 Pa) and a low sensitivity mode with a broad sensing range (up to 350 kPa). By integrating a PC-gel-based sensor on a robotic gripper, we fabricated an artificial tactile feedback system to emulate object handling. Based on real-time monitoring of the tactile signal, the system autonomously adjusted the grasping force to adapt to various objects with different stiffness. This work provides a new strategy for designing functional pressure sensors, and paves the way for intelligent robotic technologies, including adaptive grasping, biomimetic robots, and human–machine interactions.
Introduction
Human hands exhibit skillful object handling with the help of sensory feedback systems, which rely on the cooperation of somatosensory organs and neural systems.1–9 During object handling, tactile information perceived by tactile receptors in the human skin is transmitted and analyzed by neural systems, which control finger muscles to adjust the grasping force for accommodating different objects sequentially (Fig. 1a). The high sensitivity and broad sensing range of the somatosensory organs play key roles in this sensory feedback system to achieve an adaptive grasp of objects with various stiffness, no matter if they are soft or hard.10–14 The emulation of such smart bio-functions in artificial pressure sensors will enrich various electronic applications, such as health monitoring, electronic skin, and robotic control.4,15–19
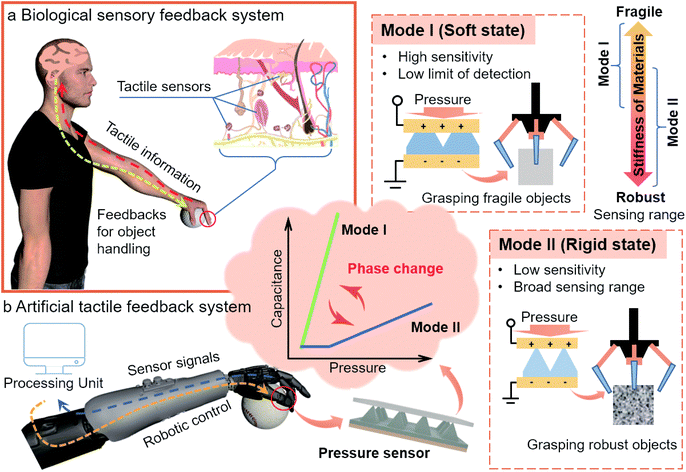 |
| Fig. 1 Schematic illustration of a biological sensory feedback system and an artificial tactile feedback system. (a) Schematic diagram of signal transmitting in the human body during object handling. (b) An artificial tactile feedback system consists of a pressure sensor, robotic hand, and controlling system. The PC-gel-based pressure sensor can switch between two operating modes owing to the phase change of the PC-gel. In Mode I, the pressure sensor with the PC-gel in the soft state exhibits high sensitivity, guiding the robotic gripper to adaptively grasp soft objects. In Mode II, the pressure sensor with the PC-gel in the rigid state presents relatively low sensitivity but a broad sensing range, endowing the robotic gripper with the capability of grasping hard objects. | |
A lot of artificial pressure sensors based on various principles have been well developed, including capacitive, magnetic, piezoresistive, and piezoelectric effects.12,17,20–26 Recently, owing to the simple device architecture and low energy cost, capacitive pressure sensors have drawn increasing attention for constructing tactile sensory systems and electronic skin.10–12 Elastic dielectric materials are widely used as sensing elements in capacitive pressure sensors due to their deformability under mechanical forces.27–29 Lowering the Young's modulus of dielectric materials and engineering a micro-to-milli structure on the dielectric layers have been proved as effective strategies to improve the sensitivity and the limit of detection (LOD). These modifications enhance the deformability of the dielectric layer; however, they lead to inevitable sacrifice of the detection range.9–11,30 Therefore, to emulate the tactile sensing ability of the human skin, balancing sensitivity and the sensing range in artificial pressure sensors is still a tough challenge to overcome.
By introducing different structural designs during manufacturing, the performance parameters of pressure sensors including sensitivity and the sensing range can be tuned to adapt to different working environments.13,18,31 However, once a pressure sensor is fabricated, it will usually exhibit unalterable sensing parameters due to the fixed structure and Young's modulus of the dielectric material.10,29 Therefore, we suppose that using a material with a tunable modulus as the dielectric layer would be able to modulate the sensitivity and sensing range of the pressure sensor.32,33 In this work, we design a phase-change-gel (PC-gel)-based pressure sensor with tunable operating modes that can realize high sensitivity and a broad sensing range in one pressure sensor (Fig. 1b). The PC-gel undergoes phase change between two solid states at room temperature, a soft state with a Young's modulus of 300 kPa and a rigid state with 342 MPa. With dramatic changes in deformability of the dielectric layer, the sensor can switch between two operating modes. In Mode I, the pressure sensor with the soft dielectric layer is able to sense force as low as 2 Pa and exhibits a high sensitivity of 21 kPa−1 with a high signal-to-noise ratio. In Mode II, low deformability of the rigid dielectric layer endows the device with relatively low sensitivity but a broad sensing range (up to 350 kPa). Moreover, we integrated the pressure sensor on a robotic gripper to form an artificial tactile feedback system. By real-time monitoring the tactile signals, the system can change operating modes and adjust the grasping forces to accommodate the stiffness of the target objects, no matter hard or soft.
Materials and methods
Chemicals and materials
Acrylamide (AAm, 99%) for use as the monomer and poly(ethylene glycol)diacrylate (PEGDA, Mw ≈ 1000) for use as the chemical crosslinker were purchased from Aladdin Chemistry Co., China. 1-Hydroxycyclohexyl phenyl ketone for use as the photo-initiator (photo-initiator, Irg.2959), calcium chloride hexahydrate (CaCl2·6H2O), and strontium chloride hexahydrate (SrCl2·6H2O) were purchased from Shanghai Macklin Biochemical Co., Ltd. A SYLGARD®184 silicone elastomer kit (PDMS) was purchased from Dow Corning (USA). The indium doped tin oxide coated PET film (ITO/PET film, 5–7 Ω sq−1) was purchased from Zhuhai Kaivo Optoelectronic Technology Co., Ltd. All the chemicals were used as received without further treatments.
Characterization
The X-ray diffraction (XRD) patterns of gels were monitored directly without any treatment by utilizing the Cu Kβ X-ray beam on a Smartlab 3kw (Rikagu, Japan). The scanning rate was fixed at 8° min−1 and the scanning scope of 2θ was from 10° to 80°. Mechanical testing was performed with a universal tester (INSTRON model 3344) at a cross-head speed of 10 mm min−1. The cyclic experiment was performed using a TMS-Pro texture analyzer (Food Technology Corporation). A scanning electron microscope (SEM, SU3500, Hitachi) was employed to observe the cross-sectional images. The scanning electron microscopy (SEM) image of the soft gel processed by solvent displacement was taken on a Carl Zeiss G300 FE-SEM System.
Preparation of the PDMS mold
PDMS solution was prepared by adequately stirring the PDMS base and curing agent (Sylgard 184, Dow Corning) in a mass ratio of 10
:
1. Then, the mixture was put in a vacuum chamber to degas air bubbles. Finally, the mixture was poured into the pyramidal mold, and cured at 80 °C for 2 hours (Fig. S1†).
Synthesis of the phase-change gel
Polymer precursors were prepared by mixing CaCl2·6H2O, the AAm monomer, PEGDA and the photo-initiator at 95 °C. Once the polymer precursors were homogeneous and clear, the above solution was quickly transferred to the PDMS mold and cured by irradiating with an ultraviolet light source (365 nm, LED floodlight) at a 10 cm distance for 90 s (440 mW cm−2) under room conditions. Samples were then cooled to room temperature before use (Fig. S1 and S2†).
Fabrication and testing of the pressure sensor
The ITO/PET film was used as the top and bottom electrodes, and the micropatterned PC-gel was used as the dielectric layer between ITO/PET films. The capacitance was monitored using a LCR meter (E4980, Keysight Technologies) at 10 kHz under 1 V.
Results and discussion
Phase-change gel based on calcium chloride
We employed polyacrylamide and calcium chloride hexahydrate (CaCl2·6H2O), one of the typical phase-change materials, to synthesize the PC-gel (Fig. S2†). The calcium chloride hexahydrate switches between solid and liquid states at the phase-change temperature (29 °C).34 Incorporating such a phase-change material into polyacrylamide endows the resulting gel with the ability to switch between rigid and soft states (Fig. 2a). Due to the supercooling effect, the PC-gel switches to a rigid state below 11 °C. Therefore, a small amount (1% w/v) of SrCl2·6H2O was employed as a nucleation agent to increase the phase-change temperature to the range of room temperature (above 17 °C) (Fig. 2b). The X-ray diffraction (XRD) spectrum of the PC-gel in the rigid state matches well with the characteristic peak of CaCl2·6H2O (No. 02-0298), proving that the formation of CaCl2·6H2O crystals results in the phase change of the PC-gel (Fig. 2c). Besides, the PC-gel in the rigid state was measured with SEM (Fig. S3†); the particle-like CaCl2·6H2O crystals aggregated in the polymer networks, wherein the flake size was roughly 1 μm. The characteristic peak disappeared after exposing it to a temperature exceeding 29 °C, indicating that the melt of CaCl2·6H2O crystals makes the PC-gel phase to change to the soft state.
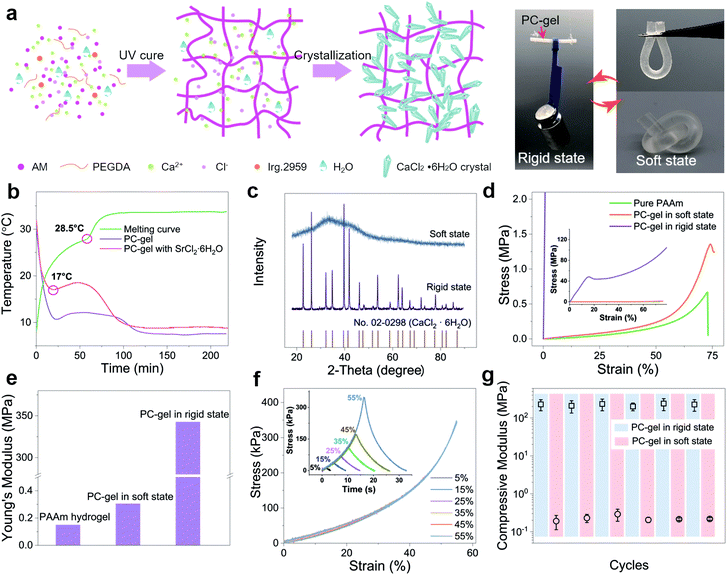 |
| Fig. 2 Characteristics and mechanical properties of the phase-change gel. (a) Schematic of components in the PC-gel and the phase change process. The PC-gel in the rigid state with a cross-sectional diameter of 4.6 mm can hold a weight of 500 g, and that in the soft state can tie knots or bend without breaking. (b) XRD spectrum of the PC-gel in soft and rigid states. (c) Phase-change temperatures of a PC-gel. (d) The elastic compression of a PAAm gel, and PC-gel in soft and rigid states. (e) Young's modulus of the PAAm gel, PC-gel in soft and rigid states. (f) The stress to strain figure of a PC-gel in the soft state with different reversible strain changes. (g) Switching properties of a PC-gel between soft and rigid states. | |
The prepared PC-gel in the rigid state exhibited high compressive strength and can sustain a load of 500 g without any deformation (Fig. 2a). In contrast, the PC-gel in the soft state undergoes various deformations like knotting and bending without any damage (Fig. 2a). Besides, the PC-gel in the rigid state became highly stiff (342 MPa), and exhibited extreme strength (110 MPa) at 90% strain (Fig. 2d and e). Gradual loading-unloading tests with various strains were performed to evaluate energy dissipation of a sample. For the PC-gel in the soft state, the negligible hysteresis loops (Fig. 2f), indiscernible residual deformation and the overlapping contour of the corresponding stress–time curves showed that there was almost no internal fracture of the network under deformation.
In contrast, the PC-gel in the rigid state exhibited pronounced mechanical hysteresis under large strains (Fig. S4a†), indicating that the filled CaCl2·6H2O crystal crushed. However, successive cyclic compression tests with a fixed strain (1% strain) showed a well consistency for stress (Fig. S4b†), indicating that the PC-gel in the rigid state has certain elasticity under small strains. All of these results prove that incorporating crystals into a polymer network is a facile process to prepare a tough and stiff gel. Moreover, the PC-gel can steadily switch between the rigid state and soft state by tuning the temperature between 10 °C and 30 °C (Fig. 2g).
PC-gel based pressure sensor
By using a template method, we engineered an array of pyramidal structures on the surface of a thin PC-gel film (Fig. S1†). Due to the polymerization of acrylamide when mixing it with CaCl2·6H2O at 95 °C during gel synthesis, the molding process in the template should be completed as fast as possible (less than 30 s), and the size of the pattern on the template should not to be smaller than 1 mm for reducing the defects. For assembling the PC-gel-based pressure sensor, the fabricated pyramidal PC-gel film was sandwiched between two layers of flexible ITO/PET electrodes (Fig. 3a).
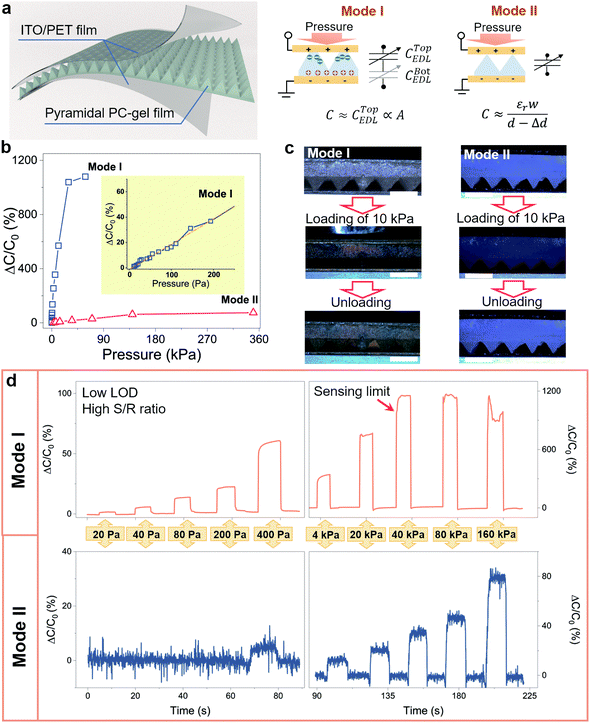 |
| Fig. 3 Sensing properties of the PC-gel-based pressure sensor. (a) The device architecture of the PC-gel-based pressure sensor and the sensing principles of Mode I and Mode II. (b) Figure of relative change of capacitance to pressure of the PC-gel-based pressure sensor in Mode I and Mode II. (c) Deformability of PC-gel layers in the pressure sensor under applied pressure (scale bar: 2 mm). (d) Real-time monitoring of sensing signals in a low and high pressure range. | |
The sensing characteristic of the PC-gel-based pressure sensor is closely related to the gel states. To achieve a fast switch between the gel states, we used a thermoelectric cooler (TEC) to adjust the temperature around the sample to test the PC-gel in the soft state (∼30 °C) and rigid state (∼10 °C). In Mode I, the capacitance change of the device (ΔC/C0) increased linearly with the applied pressure, presenting a high sensitivity up to 21 kPa−1 (Fig. 3b and S5†) and exceeding many capacitive pressure sensors based on hydrogels (Table S1†). The high sensitivity can be attributed to the excellent deformability of the PC-gel in the soft state, which showed an obvious change of the contacting area under loadings (Fig. 3c and S6†). Moreover, due to the formation of an electrical double layer (EDL), the initial capacitance (C0) and a capacitive sensitivity in Mode I were measured and calculated to be 5.8 nF and 1.16 nF kPa−1.12 These two values were thousands of times higher than those in Mode II (3 pF, 0.01 pF kPa−1), resulting in a high signal-to-noise ratio (Fig. 3a).11 When high pressure (above 40 kPa) was applied to the device in Mode I, the pyramidal gel with good deformability would be totally squashed and lose its sensing ability (Fig. 3c and S6†). In Mode II, the PC-gel in the rigid state with a high Young's modulus displayed tiny shape changes (<1.5%) under applied pressure in the sensing range, making the sensor to show a relatively low sensitivity of 0.42 kPa−1 (Fig. 3c and d). However, the sensing range in Mode II was much broader than that in Mode I, which reached 350 kPa (Fig. 3d). Higher pressure applied to the pressure sensor in Mode II would cause irreversible shape changes on the pyramidal structure and the loss of sensing performance, which can be recovered after phase change (Fig. 2g and S7†).
To compare the sensing properties of our sensors in Mode I and Mode II, we collected the real-time monitored signals (Fig. 3d). In the weak pressure range (0–400 Pa), the pressure sensor in Mode I shows a low detection limit and distinguishes different weak pressures with a high signal-to-noise ratio, which can be attributed to the electrical double layer. For Model II, the device generated obvious noise in a low pressure range, and cannot present valid sensing signals up to the loading pressure of 0.4 kPa. In a high pressure range (4–160 kPa), the pressure sensor in Mode I reached its limit at the pressure of 40 kPa, while the sensor in Mode II exhibited a reliable sensing ability.
The mechanical stability of the pressure sensor in two modes was investigated by repeatedly subjecting it to loadings of 5.5 kPa and 100 kPa for 500 cycles. The sensing performance of the device remained stable during the cycling test (Fig. S8†). The response time of the PC-gel-based pressure sensor was tested by loading and unloading a 50 g weight on its surface. The device showed a fast response time of hundreds of milliseconds in Mode I and Mode II (Fig. S9†), respectively. It is worth noting that the shape change of the rigid PC-gel in Mode II under applied loadings within the working range is quite small (<1.5%), which is not affected by the mechanical hysteresis and endows the device with good repetitive response capability (Fig. S4b and S8b†). Furthermore, the change of the water amount under ambient conditions is a serious problem for any electric devices based on hydrogels. In our case, an unsealed sensor shows unstable sensing properties and low operability because of absorbing water (Fig. S10†). To solve this problem, the PC-gel-based pressure sensor is encapsulated with a thin PE film and can maintain reliable sensing properties for at least one week (Fig. S10 and S11†).
Artificial tactile feedback system
Robotic hands that mimic human hands to grasp a hard or soft object have wide application in modern industry. However, they face a great challenge in modulating sensitivity or the sensing range for adaptively grasping. Here, by attaching the PC-gel-based pressure sensor on a robotic gripper, we realized an artificial tactile feedback system that can exhibit adaptive grasping strategies in response to the tactile feedback (Fig. 4a). During the process of grasping objects, the grasping force read by the pressure sensor was real-time monitored using an analyzer, which emulated the somatosensation of touch during object handling by human hands. When the signal reached the pre-set threshold, the robotic hand was controlled to lift and move the object by a robot controller (Fig. 4a). The analysis of the tactile signals and the commands generated for controlling the robotic hands were accomplished by Python programming.
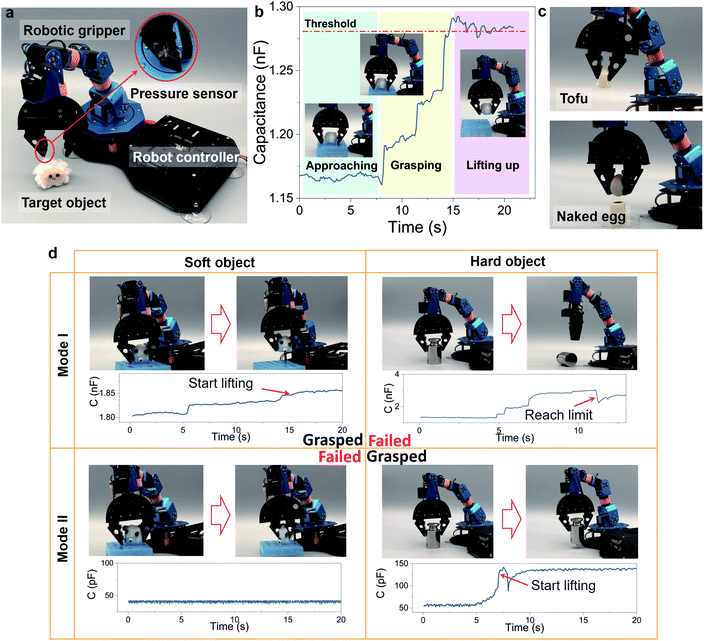 |
| Fig. 4 The artificial tactile feedback system for adaptive grasping. (a) Photographs of the fully assembled artificial tactile feedback system with a PC-gel based pressure sensor, a robotic hand and a control panel. (b) Capacitance response during grasping a cotton ball, and the photographs of grasping a piece of tofu and a naked egg by an artificial tactile feedback system (c). (d) The artificial tactile feedback system in Mode I successfully grasped a soft cotton toy, while it failed to grasp a robust steel weight that was beyond the sensing range of the pressure sensor in Mode I. The artificial tactile feedback system in Mode II can grasp a hard and heavy weight. However, it was not able to read the grasping force during grasping the soft cotton toy and squashed it. | |
Based on the artificial tactile feedback system, the robotic hand can autonomously adjust the grasping force for handling objects. With the help of the high sensitivity PC-gel-based pressure sensor in Mode I, the robotic hand presented the capability to grasp fragile and soft objects (Fig. 4 and S12†). Firstly, the values of the capacitance threshold corresponding to the texture of the object were pre-set in the program. Secondly, during the grasping process, the gripper kept closing and applied force on the target object, while the tactile signal was real-time monitored and compared with the threshold. Finally, when the signal was above the threshold or reached the sensing limit of the pressure sensor, the robotic gripper would stop closing and lift the object up.
For the operation of grasping a cotton ball (Fig. 4b) in Mode I, before the robotic gripper coming into contact with the surface of the cotton ball, the capacitance read by the PC-gel-based pressure sensor showed stable signals without obvious changes. After coming into contact with the surface, the signal gradually increased as the gripper kept closing. As it reached the pre-set threshold, the robotic gripper successfully lifted the ball up. Other fragile and light objects, such as a piece of Tofu and a naked egg, were also grasped by our artificial tactile feedback system without causing any damage (Fig. 4c and S12†).
Two operating modes with complementary parameters, including high sensitivity and a broad sensing range, endow the artificial tactile feedback system with the capability of adaptively grasping objects with various degree of softness. We used a panda toy made of cotton as the target object, which was only about 400 mg in weight. The tactile feedback system in Mode I was able to sense the subtle grasping force and pick the toy up without causing any damage, while the robotic hand in Mode II would squash the toy totally. In contrast, for a hard object, such as a steel weight of 500 g, the robotic hand in Mode II clearly sensed the grasping force and successfully lifted it up. Meanwhile, the artificial tactile feedback system in Mode I failed to grasp the steel weight due to the grasping force beyond its the sensing range (ESI Video,†Fig. 4d). Compared with a conventional pressure sensor with a fixed operating mode, the PC-gel-based pressure sensor presented reliable sensing properties of high sensitivity and a broad sensing range with remarkable adaptivity, which makes it suitable to serve as a versatile sensing device in different applications.
Conclusion
Herein, we designed a pressure sensor with adjustable switch between high sensitivity and a wide detection range. A pyramidal PC-gel film with phase transition ability acts as an active layer in this sensor. We investigated the mechanical performance of the PC-gel at different temperatures, which exhibits a compressive modulus of 300 kPa in the soft state and a more than 600 times higher modulus in the rigid state. As a result, the PC-gel based pressure sensor shifted from low sensitivity to high sensitivity due to the difference in compressive ability. By integrating the pressure sensor on a robotic gripper, we can modulate the grabbing motion by real-time reading the pressure feedback. According to the compliance and modulus of target objects, the robotic gripper can be adjusted to grab a soft and light object in high sensitivity mode, or a hard and heavy object in low sensitivity mode. This work will provide a potential and innovative strategy for the design of soft robotics and electronic skin with tunable ability.
Author contributions
H. J. fabricated the devices and performed the device measurements. H. J., Y. L., and X. W. designed and carried out the grasping demonstration of the robotic gripper. Y. L. and J. H. supervised the project. Y. L., L. X., and H. J. wrote the manuscript. All the authors contributed to the analysis of the results and commented on the manuscript.
Conflicts of interest
There are no conflicts to declare.
Acknowledgements
This work was supported by the Qilu Young Scholarship Funding of Shandong University. This work was also supported by the Natural Science Foundation of China (No. 22002073), the Natural Science Foundation of Shandong Province (ZR2020QB064), and the Natural Science Foundation of Jiangsu Province (BK20200231).
Notes and references
- B. W. Zhu, H. Wang, Y. Q. Liu, D. P. Qi, Z. Y. Liu, H. Wang, J. C. Yu, M. Sherburne, Z. H. Wang and X. D. Chen, Adv. Mater., 2016, 28, 1559–1566 CrossRef CAS PubMed.
- C. Byrne and C. L. Lim, Br. J. Sports Med., 2007, 41, 126–133 CrossRef PubMed.
- L. Alwis, T. Sun and K. Grattan, Measurement, 2013, 46, 4052–4074 CrossRef.
- A. Zimmerman, L. Bai and D. D. Ginty, Science, 2014, 346, 950–954 CrossRef CAS PubMed.
- K. Arshak, E. Moore, G. Lyons, J. Harris and S. Clifford, Sens. Rev., 2004, 24, 181–198 CrossRef.
- M. Wang, Y. F. Luo, T. Wang, C. J. Wan, L. Pan, S. W. Pan, K. He, A. Neo and X. D. Chen, Adv. Mater., 2021, 33, 2003014 CrossRef CAS PubMed.
- S. H. Wang, J. Y. Oh, J. Xu, H. Tran and Z. A. Bao, Acc. Chem. Res., 2018, 51, 1033–1045 CrossRef CAS PubMed.
- B. C. K. Tee, A. Chortos, A. Berndt, A. K. Nguyen, A. Tom, A. McGuire, Z. C. Lin, K. Tien, W. G. Bae, H. Wang, P. Mei, H. H. Chou, B. Cui, K. Deisseroth, T. N. Ng and Z. Bao, Science, 2015, 350, 313–316 CrossRef CAS PubMed.
- Y. Liu, K. He, G. Chen, W. R. Leow and X. Chen, Chem. Rev., 2017, 117, 12893–12941 CrossRef CAS PubMed.
- S. R. A. Ruth, V. R. Feig, H. Tran and Z. N. Bao, Adv. Funct. Mater., 2020, 30, 2003491 CrossRef CAS.
- Y. Chang, L. Wang, R. Li, Z. Zhang, Q. Wang, J. Yang, C. F. Guo and T. Pan, Adv. Mater., 2021, 33, e2003464 CrossRef PubMed.
- M.-J. Yin, Z. Yin, Y. Zhang, Q. Zheng and A. P. Zhang, Nano Energy, 2019, 58, 96–104 CrossRef CAS.
- N. N. Bai, L. Wang, Q. Wang, J. Deng, Y. Wang, P. Lu, J. Huang, G. Li, Y. Zhang, J. L. Yang, K. W. Xie, X. H. Zhao and C. F. Guo, Nat. Commun., 2020, 11, 209 CrossRef CAS PubMed.
- Y. Pang, K. Zhang, Z. Yang, S. Jiang, Z. Ju, Y. Li, X. Wang, D. Wang, M. Jian, Y. Zhang, R. Liang, H. Tian, Y. Yang and T. L. Ren, ACS Nano, 2018, 12, 2346–2354 CrossRef CAS.
- L. Q. Tao, K. N. Zhang, H. Tian, Y. Liu, D. Y. Wang, Y. Q. Chen, Y. Yang and T. L. Ren, ACS Nano, 2017, 11, 8790–8795 CrossRef CAS.
- Q. Wu, Y. C. Qiao, R. Guo, S. Naveed, T. Hirtz, X. S. Li, Y. X. Fu, Y. H. Wei, G. Deng, Y. Yang, X. M. Wu and T. L. Ren, ACS Nano, 2020, 14, 10104–10114 CrossRef CAS PubMed.
- J. Q. Zhang, L. J. Wan, Y. Gao, X. L. Fang, T. Lu, L. K. Pan and F. Z. Xuan, Adv. Electron. Mater., 2019, 5, 1900285 CrossRef.
- L. Beker, N. Matsuhisa, I. You, S. R. A. Ruth, S. Niu, A. Foudeh, J. B. Tok, X. Chen and Z. Bao, Proc. Natl. Acad. Sci. U. S. A., 2020, 117, 11314–11320 CrossRef CAS.
- H. L. Huang, L. Han, J. F. Li, X. B. Fu, Y. L. Wang, Z. L. Yang, X. T. Xu, L. K. Pan and M. Xu, J. Mater. Chem. A, 2020, 8, 10291–10300 RSC.
- S. M. Won, H. Wang, B. H. Kim, K. Lee, H. Jang, K. Kwon, M. Han, K. E. Crawford, H. Li, Y. Lee, X. Yuan, S. B. Kim, Y. S. Oh, W. J. Jang, J. Y. Lee, S. Han, J. Kim, X. Wang, Z. Xie, Y. Zhang, Y. Huang and J. A. Rogers, ACS Nano, 2019, 13, 10972–10979 CrossRef CAS.
- L. Qiu, M. Bulut Coskun, Y. Tang, J. Z. Liu, T. Alan, J. Ding, V. T. Truong and D. Li, Adv. Mater., 2016, 28, 194–200 CrossRef CAS PubMed.
- X. Yu, H. Wang, X. Ning, R. Sun, H. Albadawi, M. Salomao, A. C. Silva, Y. Yu, L. Tian, A. Koh, C. M. Lee, A. Chempakasseril, P. Tian, M. Pharr, J. Yuan, Y. Huang, R. Oklu and J. A. Rogers, Nat. Biomed. Eng., 2018, 2, 165–172 CrossRef CAS PubMed.
- R. Puers, Sens. Actuators, A, 1993, 37, 93–105 CrossRef.
- S. Li, H. Pan, Y. Wang and J. Sun, J. Mater. Chem. A, 2020, 8, 3667–3675 RSC.
- Q. Zhang, X. Liu, L. J. Duan and G. H. Gao, J. Mater. Chem. A, 2021, 9, 1835–1844 RSC.
- W. Lu, P. Yu, M. Jian, H. Wang, H. Wang, X. Liang and Y. Zhang, ACS Appl. Mater. Interfaces, 2020, 12, 11825–11832 CrossRef CAS PubMed.
- S. S. Kumar and B. Pant, Microsyst. Technol., 2014, 20, 1213–1247 CrossRef CAS.
- Z. Li, S. Zhang, Y. Chen, H. Ling, L. Zhao, G. Luo, X. Wang, M. C. Hartel, H. Liu, Y. Xue, R. Haghniaz, K. Lee, W. Sun, H. Kim, J. Lee, Y. Zhao, Y. Zhao, S. Emaminejad, S. Ahadian, N. Ashammakhi, M. R. Dokmeci, Z. Jiang and A. Khademhosseini, Adv. Funct. Mater., 2020, 30, 2003601 CrossRef CAS.
- S. C. Mannsfeld, B. C. Tee, R. M. Stoltenberg, C. V. Chen, S. Barman, B. V. Muir, A. N. Sokolov, C. Reese and Z. Bao, Nat. Mater., 2010, 9, 859–864 CrossRef CAS PubMed.
- S. Li, Y. Zhang, Y. Wang, K. Xia, Z. Yin, H. Wang, M. Zhang, X. Liang, H. Lu, M. Zhu, H. Wang, X. Shen and Y. Zhang, InfoMat, 2020, 2, 184–211 CrossRef CAS.
- J. D. Shi, L. Wang, Z. H. Dai, L. Y. Zhao, M. D. Du, H. B. Li and Y. Fang, Small, 2018, 14, 1800819 CrossRef PubMed.
- F. K. Yang, A. Cholewinski, L. Yu, G. Rivers and B. Zhao, Nat. Mater., 2019, 18, 874–882 CrossRef CAS PubMed.
- J. Wei, G. Wei, Y. Shang, J. Zhou, C. Wu and Q. Wang, Adv. Mater., 2019, 31, e1900248 CrossRef.
- K. E. N'Tsoukpoe, H. U. Rammelberg, A. F. Lele, K. Korhammer, B. A. Watts, T. Schmidt and W. K. L. Ruck, Appl. Therm. Eng., 2015, 75, 513–531 CrossRef.
Footnote |
† Electronic supplementary information (ESI) available. See DOI: 10.1039/d1ta02791c |
|
This journal is © The Royal Society of Chemistry 2021 |
Click here to see how this site uses Cookies. View our privacy policy here.