DOI:
10.1039/D1TB00674F
(Review Article)
J. Mater. Chem. B, 2021,
9, 8157-8173
SARS-CoV-2 and approaches for a testing and diagnostic strategy
Received
27th March 2021
, Accepted 15th July 2021
First published on 15th July 2021
Abstract
The COVID-19 pandemic has led to an unprecedented global health challenge, creating sudden, massive demands for diagnostic testing, treatment, therapies, and vaccines. In particular, the development of diagnostic assays for SARS-CoV-2 has been pursued as they are needed for quarantine, disease surveillance, and patient treatment. One of the major lessons the pandemic highlighted was the need for fast, cheap, scalable and reliable diagnostic methods, such as paper-based assays. Furthermore, it has previously been suggested that paper-based tests may be more suitable for settings with lower resource availability and may help alleviate some supply chain challenges which arose during the COVID-19 pandemic. Therefore, we explore how such devices may fit in a comprehensive diagnostic strategy and how some of the challenges to the technology, e.g. low sensitivity, may be addressed. We discuss the properties of the SARS-CoV-2 virus itself, the COVID-19 disease pathway, and the immune response. We then describe the different diagnostic strategies that have been pursued, focusing on molecular strategies for viral genetic material, antigen tests, and serological assays, and innovations for improving the diagnostic sensitivity and capabilities. Finally, we discuss pressing issues for the future, and what needs to be addressed for the ongoing pandemic and future outbreaks.
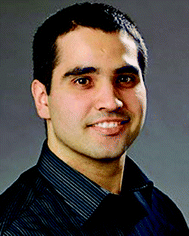
Delyan R. Hristov
| Delyan R. Hristov is a postdoc in the Engineering Department at University of Massachusetts Boston. He obtained his BSc from Sofia University in 2011 and PhD from University College Dublin (UCD) in 2015. After that, he served as a postdoc at the UCD School of Chemistry and School of Veterinary Science. He joined the group of Hamad-Schifferli in 2018. |
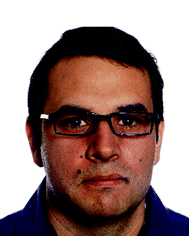
Jose Gomez-Marquez
| Jose Gomez-Marquez leads the Little Devices Lab at MIT. The group aims to lower barriers to medical prototyping by bringing the worlds of health and manufacturing together. He is a co-inventor of the Ampli and MEDIKit platform, a series of design building blocks that empower doctors and nurses around the world to invent their own medical technologies. Gomez-Marquez has served the European Union's Science Against Poverty Taskforce and has participated as an expert advisor in the President Barack Obama's Council for Advisors on Science & Technology. |
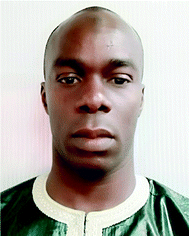
Djibril Wade
| Djibril Wade successfully completed his PhD in Biomedical Sciences from the University of Antwerp, Belgium, in 2015 on the implementation of a National CD4 quality control program and decentralization of CD4 monitoring for PLHIV in Senegal. He is a research scientist working at RARS-IRESSEF and in 2018 was a visiting researcher at Boston University. Since 2017, Djibril works as a project manager of iLEAD, collaborating with IVD companies and scientists on innovations with potential to improve laboratory value chains. He has authored 16 publications in international peer-reviewed journals, and was selected as an Africa Rising Star by the African Academy of Sciences and the BMGF in 2019. |
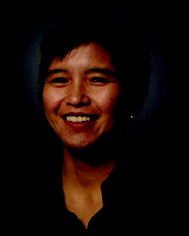
Kimberly Hamad-Schifferli
| Kimberly Hamad-Schifferli is an Associate Professor in the Engineering Department and the School for the Environment at the University of Massachusetts Boston. She obtained her SB from MIT in 1994 and PhD from the University of California at Berkeley in 2000, and was a postdoctoral associate at the MIT Media Lab. She was a faculty member in the Departments of Mechanical Engineering and Biological Engineering from 2002 to 2012. |
I. Background on SARS-CoV-2 and COVID-19
The COVID-19 pandemic, caused by the SARS-CoV-2 virus, originated in China's Wuhan province in December of 2019, has become an unprecedented worldwide emergency. Its prominence, rate of infection and potentially fatal outcomes have led to a number of measures including bans on large public gatherings and the close of many businesses. The personal and economic toll of the pandemic has led to an uncertain path forward. One of the major challenges of this crisis has been developing reliable and affordable testing at a scale available to the public.
Clinical diagnosis of COVID-19 is not straightforward as many of the clinical symptoms are relatively common, e.g., cough, fever, and sore throat, which are similar to other respiratory infections. This complicates the identification of COVID and also leads to misdiagnosis of other similar diseases.5–8 Beyond the relatively common and variable symptomology patients may also have a viral or bacterial co-infection which could result in both, abnormal disease patterns and worse outcomes.9–11 Therefore, diagnostics tools are often used as confirmation. Computerized tomography (CT) scans are a widely available and commonly implemented non-molecular diagnostics tool. About 85% of symptomatic and 50% of non-symptomatic COVID-19 patients exhibit “ground-glass” opacities apparent in a CT scan.12–14
Innovations in materials science has led to novel strategies for diagnostics, particularly for paper-based systems that utilize nanoparticles for the readout. In the first part of this review, we describe what is presently known about the SARS-CoV-2 virus, its life cycle and infection pathways, and identify the similarities and differences to other coronaviruses in terms of structure, symptomology and clinical diagnosis. In the second part, we discuss the rapid and lab-based detection methods for SARS-CoV-2. In the third part, we discuss the pressing future issues in the context of present day knowledge.
A. Details on coronaviruses
Virus classification.
Virus structure and classification are crucial for developing a mitigation strategy in the case of outbreaks. The classification of RNA viruses considers genetic variability, which often results in viruses with similar genome sequences being classified as variants or strains of the same virus. A metaphor to explain this is different people may be classified as members of an identifiable group. The distinction between members of the same viral species and similar species is the degree of RNA variability. Once the virus species is identified, it is named to identify its proximity to other prominent members of the same viral family.
The full taxonomy of SARS-CoV-2 is: Riboviria > Orthornavirae > Pisuviricota > Pisoniviricetes > Nidovirales > Cornidovirineae > Coronaviridae > Orthocoronavirinae > Betacoronavirus > Sarbecovirus > Severe acute respiratory syndrome-related coronavirus. SARS-CoV-2 belongs to the coronavirus family, is a beta coronavirus, and is classified as SARS-related coronavirus (NCBI taxonomy browser, ID 2697049).
Coronaviruses are enveloped with crown like particles (hence the name) with an average diameter of 80–120 nm. Spike proteins protrude from their surface (Fig. 1a). They have single-stranded, positive-sense RNA (+ssRNA) genomes of approximately 26–32 kb.1,16 There exists both a group and subgroup classification system, 1a and b, 2a–d, etc. Each group is designated using a Greek letter, i.e. alpha coronaviruses (αCoV), beta coronaviruses (βCoV), gamma coronaviruses (γCoV), and delta coronaviruses (δCoV).
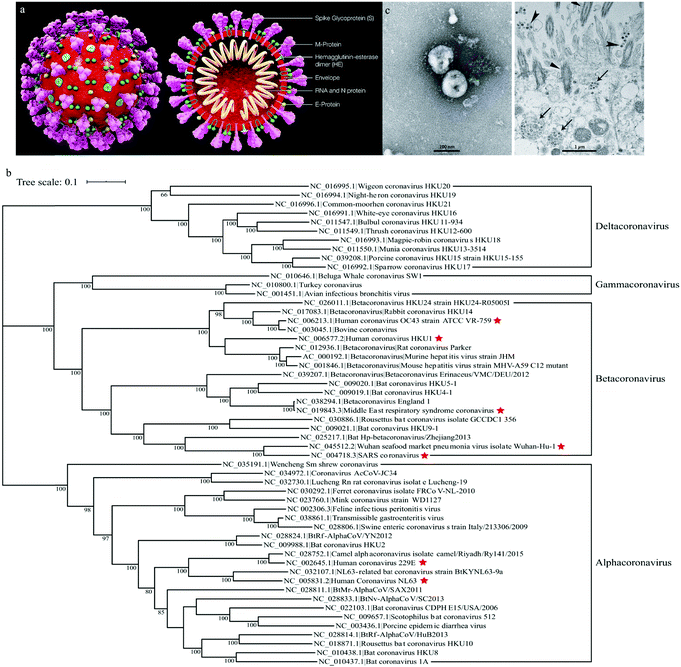 |
| Fig. 1 (a) Structure of coronaviruses (Wikipedia), (b) phylogenetic tree of coronaviruses1 and (c) TEM of SARS-CoV-2.2 | |
There are presently seven identified human coronaviruses (Fig. 1b). The first isolated member of the human coronavirus family is HCoV-OC43 (βCoV), which was isolated in 1967. Coronaviruses were largely considered non-lethal until the 2002/2003 SARS-CoV (βCoV) epidemic which resulted in 8273 confirmed cases and 776 deaths (9.6% fatality).17 Three more human coronaviruses, HCoV-NL63 (αCoV),18 CoV-HKU1 (βCoV),19 and MERS-CoV (βCoV) in 2012,20 were identified in the next decade. SARS-CoV-2 emerged in December of 2019 and resulted in the COVID-19 global pandemic.2 Presently, there are no identified human γCoV or δCoV.
Of the seven human coronaviruses presently known, HCoV-OC43, 229E, NL63 and HKU1 are nonlethal and mainly cause seasonal acute respiratory tract infections (ARIs).21–24 These viruses often circulate in the same population in colder months and account for ∼10% of hospitalizations for ARIs.25,26 On rare occasions (∼1%), co-infection with more than one coronavirus has been observed, but co-infection with other respiratory viruses is more common.21,26 These four coronaviruses are occasionally detected in patients with no symptoms used as a control group.18,21 Long term studies at various locations suggest there are no prevalent virus species. Instead, infections vary based on the location and by year. Reports suggest a biannual prevalence cycle which may be due to a level of transitory immunity developed in the population. Furthermore, studies have suggested that there may be some cross-reactive immunity which protects populations from getting infected with viruses from similar species, e.g. a HCoV-OC43 outbreak may prevent a CoV-HKU1 outbreak.22,23,26 However, other studies found no evidence of cross-immunity.27
MERS-HCoV, SARS-CoV, and SARS-CoV-2 are considered to be lethal coronaviruses all of which are notably βCoV. Of these, MERS-HCoV has the highest reported fatality rate and the lowest infectious rate. According to the WHO, there have been a total of 2519 cases of laboratory confirmed MERS-CoV infections, including 866 associated deaths, and a fatality rate of 34%,28 which have occurred as smaller outbreaks distributed around the world.29,30 SARS-CoV is more infectious, but less lethal. There were over 8000 cases of confirmed SARS infection during the 2002 and 2003 outbreak with a fatality rate of ∼9.6%.31,32 Comparatively, SARS-CoV-2 has the lowest fatality rate, but is much more contagious.33 Confirmed cumulative COVID-19 cases in the first trimesters of 2020 were ∼770
000, which had risen to ∼33
500
000 by the end of September and ∼83
500
000 by the end of December of the same year, according to WHO data. The deaths in the same period were ∼40
000, 1
000
000, and 1
819
000, respectively. The overall fatality rate of the COVID-19 pandemic as of December 2020 is ∼2.2%. According to data from the US CDC, the mortality of males over 18 years of age is twice that of females (Table 1).3,4 Similar patterns have been observed in other countries.34,35
Table 1 Breakdown of COVID-19 infection and death rate in the US as of 10.10.2020. All numbers are in thousands of cases. *C is the number of cases and D is the number of deaths as reported by the CDC COVID Data Tracker.3,4 Population data obtained from Statista.com15
Age group |
Population statistics |
COVID-19 case statistics |
COVID-19 death statistics |
Male (×103) |
Female (×103) |
Total (×103) |
Male (×103) |
Female (×103) |
Total (×103) |
Male (×103) |
Female (×103) |
Total (×103) |
C* |
% |
C* |
% |
C* |
% |
D* |
% |
D* |
% |
D* |
% |
0–4 |
10 010 |
9570 |
19 580 |
52 |
0.5 |
49 |
0.5 |
102 |
0.5 |
0.02 |
0.03 |
0.02 |
0.04 |
0.04 |
0.04 |
5–17 |
31 693 |
30 360 |
62 053 |
185 |
0.6 |
189 |
0.6 |
374 |
0.6 |
0.04 |
0.02 |
0.03 |
0.01 |
0.06 |
0.02 |
18–29 |
23 060 |
22 070 |
45 130 |
615 |
2.7 |
682 |
3.1 |
1297 |
2.9 |
0.53 |
0.09 |
0.28 |
0.04 |
0.81 |
0.06 |
30–39 |
22 230 |
21 930 |
44 160 |
450 |
2.0 |
459 |
2.1 |
909 |
2.1 |
1.40 |
0.31 |
0.63 |
0.14 |
2.03 |
0.22 |
40–49 |
20 000 |
20 320 |
40 320 |
406 |
2.0 |
427 |
2.1 |
833 |
2.1 |
3.39 |
0.84 |
1.51 |
0.35 |
4.89 |
0.59 |
50–64 |
30 590 |
32 330 |
62 920 |
554 |
1.8 |
567 |
1.8 |
1122 |
1.8 |
15.69 |
2.83 |
8.08 |
1.42 |
23.77 |
2.12 |
65–74 |
14 700 |
16 790 |
31 490 |
208 |
1.4 |
204 |
1.2 |
412 |
1.3 |
19.68 |
9.47 |
12.47 |
6.10 |
32.15 |
7.80 |
75–84 |
7000 |
8970 |
15 970 |
107 |
1.5 |
127 |
1.4 |
234 |
1.5 |
22.24 |
20.78 |
18.13 |
14.25 |
40.37 |
17.23 |
85+ |
2380 |
4230 |
6610 |
57 |
2.4 |
115 |
2.7 |
173 |
2.6 |
19.33 |
33.75 |
28.99 |
25.16 |
48.33 |
28.01 |
Coronavirus structure and infection pathway.
SARS-CoV-2 and SARS-CoV are the closest human coronaviruses with a ∼93% sequence similarity between their envelope (E), membrane (M), and nucleocapsid (N). Notably, their spike proteins, which mediate the cellular uptake, have a ∼82% structural similarity, which decreases to 73% for the receptor binding domain (RBD).36 Of these proteins, N and S are most commonly used for detection and treatment.
The N protein is located within the SARS-CoV-2 virion and is responsible for modulating its structure, replication, and transcription. The protein is mostly expressed during the acute phase of the disease and is abundant in the cell cytoplasm.37–39 N protein is of interest because it induces an immune response in the host faster than the S protein.39–41
S proteins are responsible for the cell recognition and entry. S consist of two subunits, where S1 is external to the envelope and contains the RBD, and S2 is in the envelope. The S protein of SARS-CoV-2 is one of the most prominent evolution sites.36,42 It has been shown that viral uptake into cells is strongly dependent on the spike structure.42,43 The number of reported naturally occurring S variants was 329 as of May 2020.42 A classification analysis shows the emergence of viral clusters (or lineages) which may have variant infectiousness and lead to different disease severity.44,45 The evolution of SARS-CoV-2 is partially understood. The D614G clade, which was dominant in the second half of 2020, has been shown to be more infectious.46–51 The defining mutation of D614G is a substitution at position 614 where an aspartate (D) is replaced by glycine (G) compared to the Wuhan reference strain, and this mutation is often accompanied by several others. Cross immunity between viral strains also is not well understood. There have been several reported cases of SARS-CoV-2 re-infection which can be attributed to different strains.52–57 A large-scale study (63
444 patients) of recurrent positive tests showed that ∼0.01% of patients had prolonged viral shedding periods (>42 days) which could be explained by reinfection.58 Since then more infectious clades have been isolated in various countries.59
There are three receptor-mediated cellular uptake mechanisms which have been proposed in the literature, through the angiotensin-converting enzyme 2 (ACE2), CD147, and CD26 (DPP4) receptors. The ACE2 mechanism, also exploited by SARS-CoV and HCoV-NL63,60–64 is most commonly cited and considered to be the most prevalent cellular uptake mechanism.65–69 The higher infectious rate of SARS-CoV-2 as compared to that of SARS-CoV has been attributed to the higher spike protein affinity for the ACE2 receptor, 4.7 nM for SARS-CoV-2 compared with 31 nM, for SARS-CoV from SPR. Other reports suggest a SARS-CoV-2 affinity of ∼15 nM.56,67,70,71 The viral cellular uptake is complex and involves several steps. After ACE2 binding the S1 protein has to be cleaved from the viral capsid by the TMPRSS2 protease, or potentially CatB/L to enable cell entry.65 SLC6A19 (B0AT1), a neutral amino acid transporter, has been reported to prevent viral entry by preventing S1 cleavage.66 Some have suggested that changes in the ACE2 structure could impact the virus binding and thus patient outcomes.72
The CD147 and CD26 receptor dependent uptake pathways have not been investigated to the same degree as that of ACE2.73,74 As of writing this article there is little data on the mechanism of interactions between SARS-CoV-2 S and either of the two receptors. Parallels are suggested between SARS-CoV-2, SARS-CoV, HIV, and other viruses binding to CD147 which involves peptidylprolyl isomerase A (PPIA).75,76 How SARS-CoV-2 interacts with the immune system, its manifestation as clinical symptoms such as inflammation,77 reported drug successes74,76,78 and the prevalence of some co-morbidities75,79 may be explained by the entry through the CD147 pathway. However, infection through the CD147 pathway is disputed.80 There is little mechanistic data on the CD26 receptor pathway, which is more commonly associated with MERS-CoV.81–83
B. Infection route and disease propagation
Virus incubation occurs between 1 and 14 days after infection, during which time the person is still able to infect others.84 At present, data suggest that the main infection route of SARS-CoV-2 is through inhalation of large, infected saliva droplets typically present within 2 m of the point of generation.84–86 Transmission through smaller droplets which can stay suspended in air is considered to be limited.85,87 This is largely based on the relatively low viral reproductive number (R0 < 18), effectiveness of non-medical masks, and transmission mechanism of SARS-CoV.85,86,88 Some reports have attempted to develop spread models through saliva droplet mechanics. Stadnytskyi et al. calculated the probability of a virus particle being present in droplets of various sizes as 37%, 0.37%, and 0.01% for a 50, 10, and 3 μm, respectively, and the effect of evaporation on transmission.89 Others have discussed the effect of droplet size, shape, and chemical makeup on infectious rates.87,90–92 Interestingly, it was observed that some people may act as super spreaders due to their droplet generation patterns.90,93
Though airborne transmission is considered rare, it has been reported that it is more likely in enclosed spaces with poor ventilation or in locations where a large number of sick individuals congregate.85,90,94–96 A high concentration or abnormal droplet properties can lead to virus accumulating in the environment over time.89,92 Inappropriate ventilation can lead to larger particles being spread beyond the 2 m diameter.87,90 These concerns may be especially relevant in hospital settings. Some reports suggest that asymptomatic patients are less contagious, possibly due to lower droplet generation.97,98 Conversely activities generating a lot of droplets, such as singing, may increase the infection rate.91 On a molecular level the inhalation pathway is interesting due to the relatively high expression of ACE2 and TMPSS2 in the oral and nasal cavity, and respiratory system together with low expression of SLC6A19.99,100
C. SARS-CoV-2 immune response and duration
Understanding the timing and duration of seroconversion is crucial for diagnostics as the target of interest changes with the immune response. The immune response is a complex process which involves activation of several systems. These include the synthesis of inflammatory cytokines, innate and at a later stage the adaptive immune system.42,101–103 Most viruses, including SARS-CoV-2 interact with the immune system to subvert or suppress it.104–107
Most studies report seroconversion rates within two weeks of infection (Fig. 2).108–113 Interestingly, in at least one study, IgM generation had a similar rate to that of IgG and not all patients developed an IgM response.108 Both antibody generation and prevalence time in serum were reported to scale with disease severity.42,110 Asymptomatic and mild cases had low antibody generation and prevalence similar to that of non-lethal coronaviruses,114,115 while more severe cases had a more pronounced immune response for longer times, similar to other lethal coronaviruses.115–117 Though cross-immunity with other viruses is not well understood, emerging studies suggest some individuals may have some degree of pre-existing immune response.118,119
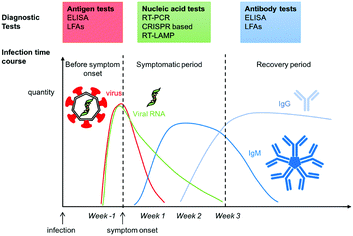 |
| Fig. 2 Infection time course for COVID-19 and symptom onset. Diagnostic test assay at each stage for different biomarkers from the virus. | |
There is little data on the persistence of generated antibodies. In a notable report, Prevost et al. observed a 26% decrease in antibody concentration in patient serum within the three month study period in a large cohort of 365
000.109 IgG prevalence changed from 6% in June/July to 4.4% in late September. Importantly, antibody prevalence was established via a self-administered lateral flow immunoassay (LFA),120 thus there could be some thresholding limitations. Iyer et al. reported a median seroreversion time, i.e. the time taken to lose antibody seroprevalence, of 49, 71, and 90 days for IgM, IgA, and IgG, respectively.121 Other studies report antibody decrease in a similar timeframe.122,123 In contrast, other reports find little change in the IgG seroprevalence over a three-month period.124–126 Such inconsistencies could be attributed to the differences in antibody measurement methods used, cohort size and demographics, and infection severity. More recently, longer-term studies have suggested that 95% of individuals have a sustained immune response for 5 to 8 months post-symptom onset.127
A short and/or low persistence, or poor cross-species immunity of the SARS-CoV-2 response could lead to re-infection and account for the 3 to 61% patient re-admission rates into hospitals following discharge.128–131 Though these mechanisms are poorly understood for COVID-19, they have been proposed for non-lethal coronaviruses.114,130,132–134 Hospital re-admission could also be due to false negatives, the use of different diagnostics tools, and the lack of guidelines standardization in the field.104,128,135
D. SARS-CoV-2 vaccine development and impact on antigen diagnostics
Vaccine development has been seen as a sustainable solution to the COVID-19 pandemic and started in the first trimester of 2020.136,137 The number of vaccine candidates in the production pipeline has increased from ∼78 before May 2020138 to 158–166 in September/October 2020.139–141 As of February 2021, there are at least seven different vaccines that have been administered to more than 175 million people worldwide.137,142 Two have been approved by select countries.137 Pfizer and Moderna candidates, both of which are mRNA vaccines, have reported a 90% and 95.4% efficiency, respectively, and were approved in December 2020 with others to follow.143–146
Widespread vaccination would impact the applicability of antibody and seroconversion studies for disease detection which are more likely to be used to monitor the vaccine efficiency and seroreversion rate.
II. Approaches for COVID-19 diagnostics
Molecular diagnostics developed for SARS-CoV-2 detection are RT-PCR, ELISA, and rapid paper-based among others. Industry has led the way in innovative approaches, accelerated by mechanisms for emergency approval and increased funding for commercial systems.147–149 Considerations for COVID-19 testing include technical factors, such as diagnostic sensitivity and specificity, and also availability, cost, turnaround time, and the end user who performs the assay.150,151 Because of the massive demand for tests, a range of different test types are necessary to be able to continually meet the demand. We compare diagnostic approaches and materials innovations that can potentially improve them. Because of the format, the majority of materials and chemistry advancements have been primarily focused in the lateral flow assay formats.
A. Nucleic acid diagnostic tests
The most common diagnostic approach is the reverse transcription polymerase chain reaction (RT-PCR), or quantitative RT-PCR (qRT-PCR), both of which detect the presence of the viral genetic material present upon infection, and which persists up to a couple of weeks post-infection (Fig. 2).
Viral RNA is detected by first reverse transcribing it into DNA, which is then enzymatically amplified until it reaches a detectable level. The presence of the DNA is optically read out using fluorophore–quencher probes specific to the DNA sequences of interest. PCR results are often described in terms of the cycle threshold (Ct), i.e. the number of replication cycles before a detectable fluorescence signal appears.152,153 Commercial diagnostics typically test for the presence of a specific SARS-CoV-2 gene set. These are usually the ORF1b and ORF8 genes, with sometimes including the S, N or E genes. The patient sample for PCR is usually a nasopharyngeal (NP) swab and thus requires an initial extraction step to isolate the nucleic acid from the sample matrix.
PCR has high sensitivity and can detect trace amounts of target, and so it has the potential to detect an infection at an early stage before the immune response and the patient exhibits symptoms. The lowest LODs observed have been 9 copies per mL from PerkinElmer with a low false positive rate.154 Furthermore, due to the specificity of the nucleic acids, RT-PCR can differentiate between species of coronaviruses as well as clades within species.21,26,52–57 Furthermore, the methodology can be used on a wide variety of samples and can yield information on patient viral shedding and infectiousness.155–157 Due to the rapid turnaround times on sequencing and the sharing of genetic codes, the scientific community was able to rapidly develop a RT-PCR diagnostic and several commercial tests were approved early in the pandemic. Many institutions have established guidelines for patient diagnosis by PCR as the gold standard.158
There are several drawbacks to RT-PCR, mostly in field detection. The procedure must be done in a centralized lab location, using various reagents (e.g. primers) and instruments operated by trained technicians. Because of this, it requires relatively high resources to run the tests, and beyond the abovementioned personnel and lab equipment, this includes sample transport and cold chains for reagents. A single test can require several hours, where the time from obtaining a sample to receiving a result can be days depending on the laboratory load. Though RT-PCR can be used for multiplexed testing, clade testing complex multiplexing procedures could cost more than $3000,159 which makes them impractical for widespread use. Laboratory setup required for a new facility can cost more than $15
000.160,161 Setting up and running diagnostic labs can be costly and time consuming, making scale-up difficult, especially if it is only needed temporarily.
Supply chain logistics can also be a limiting factor, and diagnostic testing was held up at stages of the pandemic due to shortages of necessary components, such as the DNA extraction kits and swabs for taking samples. Low resource areas or those with a modest population density may not have local testing facilities and thus samples would need to be shipped adding time to diagnosis.
Point-of-care tests: nucleic acid.
Because of the shortcomings of lab-based PCR, alternatives that are amenable to point-of-care use have been explored.
Isothermal amplification techniques.
Isothermal amplification lends itself well to a point-of-care format as it requires fewer reagents and simplifies the instrumentation requirements. Isothermal techniques can amplify the nucleic acids at a fixed temperature, and thus does not require thermal cycling. This removes the need for instrumentation that can dynamically control the temperature of the reaction. There are now several isothermal amplification techniques available. The most widely used technique is loop mediated isothermal amplification (LAMP), which relies on a strand displacement mechanism.162 Amplification and detection are done simultaneously in a single tube, and thus LAMP can achieve results more quickly than traditional PCR. Furthermore, the reaction is more robust with respect to pH and temperature. Consequently, LAMP can be adapted to novel formats, such as bead-based readouts, nanoparticle labels, nanopores, microfluidic devices, and others.163
RT-LAMP has been developed successfully for SARS-CoV-2 diagnostics. While not as sensitive as RT-qPCR, it is significantly simpler to carry out, and the RNA isolation step can even be removed.164 The first FDA approved at-home COVID test in the US was an RT-LAMP system from Lucira. The test is fully self-contained and simple to use, and the system includes an instrument for readout. The Lucira tests can be carried out with the result in 30 min, with an estimated cost of ∼$50.165
Nucleic acid tests on paper substrates.
Many point-of-care tests use paper as a substrate, which can serve as a robust medium for the reaction and also be used for sample separation and purification. Paper is of low-cost and can be manufactured on a large scale.166 Efforts to use paper substrates for nucleic acid tests have been accelerated by developments using CRISPR (clustered regularly interspaced short palindromic repeats).167 While the CRISPR RNA-guided endonuclease-based nucleic acid editing tool has been pursued for various applications, it has also been adapted for use as a molecular diagnostic.168 Specific high-sensitivity enzymatic reporter unlocking (SHERLOCK) has been commercialized (Mammoth) for SARS-CoV-2 detection. The nucleic acid in a sample is isothermally amplified and then incubated with Cas13-crRNA and reporter RNA that has a fluorophore–quencher pair. If the target is present, the reporter RNA is cleaved, unquenching the fluorophore. The format is on a paper immunochromatographic strip for fluorescence readout of the probe strand at the test line. It exhibits sensitivity and specificity comparable to that of traditional PCR, with detection limits of 1–10 copies per μL or 2 aM of DNA/RNA.169,170 SHERLOCK for CRISPR obtained an FDA emergency use authorization (EUA). Similar approaches using CRISPR have been explored such as DNA endonuclease-targeted CRISPR trans-reporter (DETECTR).
Direct detection of nucleic acids with novel biosensors.
In addition, routes to directly detect viral nucleic acids without amplification or reverse transcription have been pursued. Direct detection removes the need for steps associated with amplification, and have the potential to minimize contamination issues. For example, nucleic acids can be detected electrochemically with improved sensitivity through the use of developed labels and/or probes.171 Zhao et al. employed a “supersandwich” where the probe that can hybridize to the target is a gold and iron oxide nanoparticle complex, and the electrode with the capture probe strand is a p-sulfocalix[8]arene (SCX8) – functionalized graphene oxide. The calixarene serves to enhance the electrochemical signal. By doing so they were able to achieve ultrasensitive detection, with a limit of detection (LOD) of 200 copies per mL in clinical specimens. Furthermore, they were able to adapt the electrode into a portable system that could be readout with a smartphone.172
The plasmonic response of devices can be used to detect SARS-CoV-2.173 Qiu et al. explored a combination of plasmonic sensors with photothermal heating to directly detect SARS-CoV-2 nucleic acids.174 The authors conjugated thiolated DNA strands to the two-dimensional gold nanoislands to enable the detection of a target RNA. Successful binding was indicated by a change in the localized surface plasmon resonance response (LSPR). They were able to generate a secondary signal by strand dehybridization caused by heat generated by illuminating the LSPR. Non-matching pairs were displaced with a higher dissociation rate constant than complementary strands, hence improving the sensor discriminatory performance. Using a combined dual mode sensor can lead to achieving an LOD down to 0.22 pM.
B. Paper-based rapid immunoassays for antigens
Other diagnostics test for the presence of viral antigens, i.e. proteins that are produced by cells in response to the infection, or proteins composing the virus itself. SARS-CoV-2 infection does not result in the secretion of specific antigens at high concentrations, so the target antigens are the viral proteins.
Lateral flow immunoassays (LFA) have been used as the format for point-of-care diagnostics for a wide range of diseases.175 Assays consists of a sample pad, conjugate pad, test strip, and wick (Fig. 3a). Briefly, the biofluid is added to the strip and migrates towards the absorbent pad by capillary action. The biomarker binds to antibodies conjugated to gold nanoparticles that are dried down in the conjugate pad. The biomarker–nanoparticle–antibody conjugate then migrates through the strip and binds to immobilized capture antibodies at the test line (Fig. 3b). This double binding event, where the antigen binds to both the immobilized antibodies and the antibody on the nanoparticle, is called a “sandwich immunoassay” and results in a visible color at the test line due to the presence of the gold nanoparticles, which have a strong color due to their surface plasmon resonance (SPR) (Fig. 3).176–178
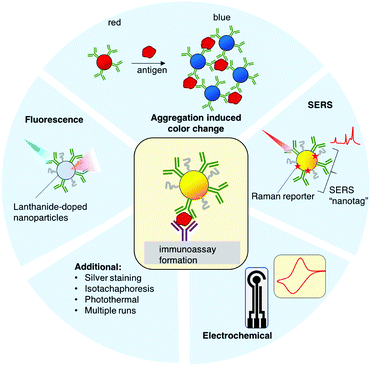 |
| Fig. 3 Lateral flow immunoassays (LFAs). The patient sample, containing the antigen, is added to the sample pad and wicks through the strip. Nanoparticle–antibody conjugates dried in the conjugate pad bind to the antigen, and the complex is captured at the test line to form a sandwich immunoassay. The control line has immobilized antibodies that bind to the nanoparticle-antibody conjugate itself. A positive test is indicated by color appearing at both the test (T) and control (C) lines. A negative test results in color only at the control line. | |
LFAs are attractive due to their ease of use, ease of operation by non-experts, and their short response times, which can be minutes instead of hours or days. The required sample volumes are relatively small (<100 μL). Typically, a biological fluid is added directly to the strip with little or no sample preparation. Manufacturing and scale-up of an off-the-shelf LFA test is relatively easy, resulting in tests that cost as little as a few $USD to produce.179 Many LFAs are stable for long periods of time and do not require special storage conditions,175 and the majority of them do not need electrical power or external devices for operation. Thus, LFAs are attractive for widespread application. They largely fulfill the WHO ASSURED criteria (affordable, sensitive, specific, user-friendly, rapid and robust, equipment-free and deliverable to end-user) for diagnostics to be used in low resource settings.180,181
There are several commercial SARS-CoV-2 antigen test kits on the market, including those by Quidel, Abbott, Becton Dickinson, and other companies.182,183 Many of these go from the sample to produce the result within minutes and are easy to use. However, a trade-off exists between the performance and rapid turnaround times, where antigen tests have lower specificity and sensitivity than nucleic acid based tests. In particular, LFAs for SARS-CoV-2 have met several challenges as viral infection does not result in secretion of a protein biomarker like nonstructural protein 1 (NS1) for the case of flaviviruses,184 such as dengue, or glycoprotein (GP) for filoviruses (Ebola).185 LFAs are relatively easy to multiplex so that they can detect multiple antigens.186
LFAs require antibodies that bind to the antigens in pairs, and their performance is highly dependent on optimizing the binding affinity of antibodies. Efforts to evaluate the performance of a large number of antibody pairs have helped shorten the test development time and aided in reagent discovery. Cate et al. screened 673 different antibodies for the SARS-CoV-2 N protein that can bind to antigens in pairs.187 They tested combinations of the different antibodies on a high throughput robotic screening platform and identified the pairs that could bind to the viral proteins. Antibodies were ranked quantitatively in signal intensity that they produced in pairs. These kinds of tools to share data helped accelerate the diagnostic discovery process.
LFAs and other paper-based immunoassays have low sensitivity and selectivity compared to PCR, typically in the 10–100 ng mL−1 range.178 In addition, LFAs can exhibit high variability which leads to low reliability, which was demonstrated during the first wave of the 2020 COVID-19 pandemic where lower regulations for diagnostics led to a number of low performance tests flooding the market.
Innovations in antigen tests.
Because of the limited sensitivity of SARS-CoV-2 LFAs, methods to increase their sensitivity have been pursued. Some studies estimate that antigen test sensitivity needs to increase by an order of magnitude.186,188 This can be achieved by utilizing a digital reader, which quantifies the test line intensity. Additional strategies can be to implement additional labels which can be read either colorimetrically (Abbott BinaxNOW with Ag CARD), by fluorescence (Quidel, Sofia), via a Raman spectrometer, or chemiluminescence through enzymatic amplification.189
State of the art advancements to antigen LFAs come in many forms. Surface enhanced Raman spectroscopy (SERS) has been applied to LFAs to increase the sensitivity of the signal readout. The Raman spectra of a molecular analyte are often used as a unique identifier, where it serve as a “molecular fingerprint.” Raman spectral intensity is greatly enhanced by several orders of magnitude (109 or greater) when the analyte is in proximity to a roughened or nanoscale noble metal surface. SERS is highly sensitive and has been used to detect analytes down to attomoles, and the dyes do not suffer from photobleaching like with fluorophores. The formats of SERS that have been useful for diagnostics are those that employ a Raman “nanotag,” which is a gold or silver nanoparticle decorated with a Raman reporter and conjugated to the antibodies specific for the target.190 The presence of the target is detected by recording the Raman spectra of the reporter molecule in the nanotag. This strategy has been used to enhance the sensitivity of paper immunoassays for other diseases in a multiplexed manner, where each nanotag is functionalized with a different Raman reporter, such as for dengue and zika nonstructural protein 1 (NS1).191,192
Another route to increase the sensitivity of LFAs is to use lanthanide-doped nanoparticles, such as Eu doped polystyrene. Lanthanides possess narrow fluorescence emission lines with long lifetimes and Stokes shifts larger than standard fluorophores. Because of their long fluorescence lifetimes, time-resolved fluorescence can be used to remove the background fluorescence. Readout is achieved with a fluorescent reader equipped for time resolved measurements. Time resolved fluorescence has been used successfully to increase the sensitivity of LFAs for HIV and for prostate specific antigens.193,194
Other efforts have been made using electrochemical sensing elements to increase sensitivity over the traditional paper immunoassays. Seo et al. have successfully utilized a graphene based field effect transistor (FET) to detect spike proteins.195 SARS-CoV-2 spike antibodies were chemically conjugated to a graphene sheet, which is incorporated into the FET. The device results in a change in the electrical response when a spike protein is present. The authors also demonstrated utility in clinical samples, with a LOD of 1 fg mL−1 for the spike protein, and 1.6 × 101 pfu mL−1 for the virus.
The phenomenon of gold NP aggregation is often used for biosensing, where the presence of the target is indicated by a color change. Ventura et al. used this colorimetric change for detecting SARS-CoV-2 proteins.196 Gold NPs were conjugated to antibodies for spike, envelope, and membrane proteins. In the presence of virions, the NPs aggregated due to the antibody–antigen binding, resulting in a shift of the SPRs, due to the proximity of the NPs to one another. This can also be detected with the naked eye, as the color of the sample is changed from red to blue. They determined a LOD of a virion concentration corresponding to a threshold count (Ct) = 36.5.
Additional strategies to improve the sensitivity of LFAs included isotachophoresis,197 photothermal heating,198 silver staining, and multicolored nanoparticles for multiplexing,184 all of which can be applied to SARS-CoV-2 LFAs.
C. Serological tests for SARS-CoV-2 antibodies
A viral infection can be detected through the host immune response, specifically through the presence of IgM and IgG antibodies. This strategy, commonly referred to as serological testing, is often used to detect for present or past exposure, seroconversion rate, and sometimes long-term immunity to an infectious disease. IgM is an indicator of an early stage infection, while IgG persists longer (Fig. 2).199 Whether the presence of these antibodies for SARS-CoV-2 indicates immunity to COVID-19 is still being determined.200 Because of the timing of the seroprevalence windows and the limited sensitivity of the IgG/IgM tests, they are not recommended for disease diagnosis. However, the low production cost, rapid turnaround and mass manufacturing capability make this strategy particularly lucrative to monitor the rate of seroconversion and seroreversion, i.e. the loss of antibodies over time, in the population.
ELISA.
IgG/IgM assays are traditionally performed using enzyme linked immunosorbent assay (ELISA). ELISA uses a plate reader format and thus is typically performed in a centralized lab location. Usually the antigen such as the SARS-CoV-2 spike protein is immobilized on the plate well, and then the patient sample is added. If antibodies for the target antigen are present in the sample, (e.g., anti-spike IgG and/or IgM) they will bind to the plate after washing steps. The presence of IgG/IgM is tested via a labeled secondary antibody which broadly binds to human IgG or IgM. An example of a typical label is horseradish peroxidase (HRP) which converts a substrate such as 3,3′,5,5′-tetramethylbenzidine (TMB) into a colorimetric species and thus produces an optical signal.201 Like PCR, ELISA has high sensitivity and selectivity, but these depend on the antibodies used and the protocol. The selectivity and specificity of serology tests are in the 80–100% range.202–204 ELISA shares some of the drawbacks of PCR regarding instrumentation and trained personnel which can result in testing bottlenecks.
Serological paper immunoassays.
LFAs have been adapted for SARS-CoV-2 IgG/IgM detection. These have been successfully developed for many other diseases, including visually read out tests that use gold nanoparticles.34,205 During the pandemic, several commercial COVID-19 antibody tests appeared on the market, but none were sufficient to gain FDA approval. While they were easy to use, many of them suffered from low sensitivity and highly variable results, yielding both false positives and false negatives.206,207 Some showed a sensitivity/selectivity as low as 30% in some clinical settings, which increased to >90% after the 14–20 day seroconversion threshold.203,206 Due to their lack of specificity and high variability, these were eventually were taken off the market.
Serological LFAs can be optimized using the same strategies to enhance antigen LFAs discussed earlier. Liu et al.208 used a sandwich immunoassay enhanced with a nanotag. The spike protein was conjugated to SiO2/Ag core–shell particles that contain the Raman dye 5,5′-dithiobis-(2-nitrobenzoic acid) (DTNB). DTNB is a well-characterized Raman-active species with narrow spectral lines. Anti-human IgG and IgM were immobilized on the test lines. This format could enhance the signal to detect IgG and IgM with 800X higher sensitivity compared to a visual readout of AuNPs. While the instrument used is a benchtop system, it could be extended for use with a commercially available handheld Raman spectrometer. By conjugating Eu-doped nanoparticles (LNPs) to anti-IgG for use as the label antibody, with immobilized nucleoprotein on the test line, Chen et al. were able to detect anti-nucleocapsid IgG antibodies developed in response to SARS-CoV-2 in confirmed patient samples.209
D. Important factors in LFAs
Recognizing the underlying phenomena occurring in LFAs is key to improving their design and performance. The impacting factors can be grouped into several general categories: (i) physical substrate, (ii) sample type, (iii) chemistry of the immunoprobe used, (iv) its interaction with the media, (v) running conditions and (vi) others. Other factors to consider are the label size vs substrate pore size, substrate cohesion and interaction with the solvent and sample flow rate.178,210–212
Immunoprobe design is multi-layered and can be broken down to (i) nanoparticle materials, (ii) conjugation chemistry, (iii) stabilization chemistry and (iv) targeting agent affinity. Nanoparticles are mostly used as labels, thus the signal type and strength is considered one of their prime attributes. Gold nanoparticles are commonly used due to their chemical stability, ease of synthesis and functionalization and controllable, visible color.213 However, many other materials have been successfully applied as discussed below. The material properties may often be altered or improved by the addition of tags or secondary labelling agents, for example, the use of SERS tags to lower detection limits.
Stabilization and conjugation chemistry are often related and affect the stability and functionality of the immunoprobes. We have previously discussed this factor to some length.177,178,214 Conjugation can be done physiochemically, i.e. by adsorption, or by covalent bonds. Polyethylene glycol (PEG) is typically used to stabilize the particles.177,215 Furthermore, both conjugation and stabilization chemistries can affect the interaction of the immunoprobe with molecules in the sample, such as other proteins. In turn this could impact the particle behavior in the test.177,216
Matrix effects are challenging for any biological application using nanoparticles, which is no exception for diagnostics.217 In paper-based immunoassays nanoparticle aggregation can lead to signal reduction and thus higher test-to-test signal variability. Another often overlooked factor is biomolecular corona formation. This is the adsorption of molecules native in the sample onto the immunoprobe.218–220 Coronas can be beneficial for assay performance as they can passivate the nanoparticle and reduce aggregation, but detrimental due to partial screening of immunoprobe functionality leading to a lower signal.
E. Impact of computational methods on paper-based assays
Beyond test development, another strategy to improve LFAs and diagnostic approaches in general is the application of computational and large data modelling methods. Such models have been used to improve the design of nanoparticles and binding strategies used in drug design and diagnostics, paper flow along other aspects of LFAs.221 Computational methods have also been used to improve the sensitivity and selectivity of existing tests by advanced image and data analysis. For example, Rodriguez-Quijada et al. were able to develop a relatively simple multiplex test to detect and differentiate Dengue and Zika viruses in samples by using differently colored gold immunoprobes where test results were analysed using machine learning.184 Finally, big data analysis for enhanced monitoring and containment of the disease has been proposed. The COVID-19 pandemic demonstrated the ability of such platforms to empower scientists, inform legislative decision making and affect public sentiment.222 An additional area of research which has been gaining interest of late is the internet of medical things (IoMT).223 In future this strategy could help improve LFAs and other point-of-care tests by integrating the various levels of the information chain, from clinical validation test to large-scale disease monitoring (Fig. 4).224
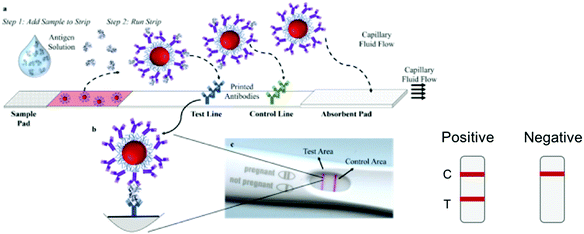 |
| Fig. 4 Innovations in antigen testing to improve the sensitivity of COVID-19 antigen tests. | |
III. Challenges for COVID-19 diagnostics and future directions
A. Challenges
Despite all of the progress in COVID-19 diagnostic development, many challenges remain (Fig. 5).225 Despite the fact that LFAs are one of the formats most amenable to POC use, they have not been as widespread in COVID-19 testing as PCR.
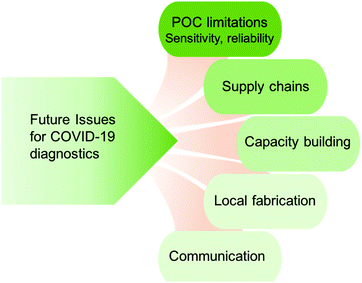 |
| Fig. 5 Continuing challenges and issues for COVID-19 diagnostic strategies. | |
First, the sensitivity of COVID-19 POC tests limits their ability as a diagnostic tool. Rapid paper tests have typical LODs of 10–100 ng mL−1, which is sufficient for many other diseases such as dengue and other flaviviruses, where the biomarker of nonstructural protein 1 (NS1) is present in the blood of infected patients at levels of a few orders of magnitude higher than these LODs, and is present within 1–2 days. However, for COVID-19, antigen levels are anticipated to be much lower. Furthermore, the time course of when antigens are present at detectable levels is not as immediate as for flaviviruses, where the long asymptomatic period hinders detection. While there is always a trade-off between sensitivity and portability/ease of use for LFAs, the nature of the viral infection impedes the ability to diagnose via antigen detection.
Another major challenge for COVID-19 POC antigen tests is their poor repeatability, which is somewhat linked to their sensitivity. Some reports have found that the repeatability of antigen tests is nonideal. Recommendations from regulatory agencies early in the pandemic suggested end users to take antigen tests multiple times, especially from different manufacturers. In previous outbreaks of emerging infectious diseases such as Zika and Ebola viruses, antigen test development took 1 year with the development efforts greatly expedited by health and funding agencies. As work continues in diagnostic development, these issues may be addressed with further refinement. This will undoubtedly be bolstered by other efforts in improving the capabilities of antigen tests through materials and sensing innovations.
B. Supply chain logistics
Furthermore, COVID-19 has underscored issues that have not been as prominent for other diseases with smaller disease burdens. For example, the massive demand for diagnostics has led to bottlenecks in the supply chain for the biological reagents and materials and testing personnel. While the innovation cycle was greatly accelerated for COVID-19, with genetic sequences, antibodies, and vaccines developed at a record pace, issues of scalability and global access have hindered efforts to surveil and contain the disease. The vulnerability of supply chains and stockpiling of materials for emergency response were also underlined. Areas with poor infrastructure, both road, and utility, present a further challenge.
C. Capacity building in low and middle income countries
Such issues are expedited in resource limited settings where access to testing facilities or even medical services may be limited.150,226,227 Sending self-contained systems are ultimately limited in their efficacy, as they are black boxes that provide only short term solutions. Locations may not have the trained personnel to use such systems or alter them to fit local needs. Stockpiling and supply chain vulnerability are exacerbated in such settings. One possible route is to invest in the development of local expertise and fabrication of reagents and increase engagement with such communities.228 Innovations for decentralized drug synthesis and diagnostics development can be applied. This further reinforces the need to invest and develop more tests which adhere to the ASSURED criteria181 to help alleviate scale-up and supply challenges.
Efforts to address these challenges have been in the form of capacity building, especially in low and middle income countries which have had comparatively lower testing rates. The African Union and African CDC formed the Partnership to Accelerate COVID-19 Testing (PACT)229 with the goal to expand testing throughput to contain transmission.230 Examples include DiaTropix in Senegal to fabricate low-cost tests, in collaboration with industrial partners and the non-profit Foundation for Innovative New Diagnostics (FIND). Because the diagnostic tools will be locally produced, it avoids issues with external supply chains, and thus can improve accessibility. Furthermore, it can help towards responding to future outbreaks, as the infrastructure for fabricating diagnostic tools will already be in place.231 Finally, capacity-building efforts must ensure that they result in sustainable solutions, accounting for use of local reagents, as well as service and maintenance for equipment. In these settings, big data analysis and IoMT could be especially effective in improving the resource deployment and combating future disease outbreaks.
D. Communication to the public
Another aspect of disease management which should not be overlooked is appropriate communication of information to public facing media and the public directly. While scientific understanding of containment aspects and strategies, such as mask wearing and social distancing, is important, suitable implementation and adherence need also to be considered. Miscommunication is detrimental, where false negative/positive result rates, diagnostic strategies, and the reasoning behind them need to be clearly communicated and explained. Fostering public understanding and trust through clear and transparent communication and policies can help improve the disease burden through higher compliance.
III Conclusions
Perhaps the lessons learned can serve as a silver lining to the COVID-19 pandemic. It has underlined faults and vulnerabilities within the existing healthcare systems, and has highlighted the specific emerging fields.232 The data and understanding accumulated during the COVID-19 pandemic, if applied well, can not only help reduce the prevalence and spread of other diseases such as influenza but also reduce the impact of future global pandemics.
Fears of zoonotic crossover into humans are not new,233 as all three of the lethal coronaviruses have made this transition in the last two decades. Thus, it is unlikely that this pandemic would be the last of its kind. Furthermore, the increase in international travel makes containment by a single country unlikely. The COVID-19 pandemic has taught us that we need to be better prepared for future outbreaks. Improving global and local testing capacity and improving the scientific, industrial, and manufacturing infrastructure is vital.
It is likely that SARS-CoV-2 will become a continual health concern, even with the deployment of several vaccines.234,235 Vaccine access is not universal, so diagnostics are still necessary for diagnosing patients and also disease surveillance. This leads to an unprecedented global need for diagnostics to both monitor the spread of the disease and the seroconversion/seroreversion rate in the population. A sound viral detection strategy is important for containing future outbreaks and managing societal well-being.
Clearly, understanding the viral infectious profile in the host is important for using this information to develop diagnostics and procedure standardization. It would help improve standard procedures and ensure that the appropriate sample is collected depending on patient disease history. Such a strategy could (i) reduce false positive/negative rates, thus improving confidence in results, (ii) ensure infected patients are identified promptly and released when appropriate, thus improving patient outcomes and containing the spread, and (iii) improve disease proliferation monitoring and understanding. Implementing a robust and actionable standardization strategy would help differentiate between an abnormal viral shedding profile and re-infection. In turn, this could help identify and contain new viral strains more rapidly. Ultimately, innovations in new materials for devices, probes, and sensors can aid in meeting the demands of higher sensitivity, lower cost, and robustness and repeatability.223,236
Author contributions
DRH, DW, JGM, and KHS contributed to the writing of this manuscript.
Conflicts of interest
There are no conflicts to declare.
Acknowledgements
The authors would like to thank for supporting funds from the University of Massachusetts Boston.
Notes and references
- B. Chen, E.-K. Tian, B. He, L. Tian, R. Han, S. Wang, Q. Xiang, S. Zhang, T. El Arnaout and W. Cheng, Signal Transduction Targeted Ther., 2020, 5, 1–16 CrossRef PubMed
.
- N. Zhu, D. Zhang, W. Wang, X. Li, B. Yang, J. Song, X. Zhao, B. Huang, W. Shi and R. Lu, N. Engl. J. Med., 2020, 727–733 CrossRef CAS PubMed
.
-
Demographic Trends of COVID-19 cases and deaths in the US reported to CDC, Centers for Disease Control, 2020.
- Weekly updates by select demographic and geographic characteristics: Provisional death counts for Coronavirus Disease 2019 (COVID-19), Centers for Disease Control, 2020.
- G. Yan, C. K. Lee, L. T. Lam, B. Yan, Y. X. Chua, A. Y. Lim, K. F. Phang, G. S. Kew, H. Teng and C. H. Ngai, Lancet Infect. Dis., 2020, 20, 536 CrossRef CAS PubMed
.
- A. Wilder-Smith, H. Tissera, E. E. Ooi, J. Coloma, T. W. Scott and D. J. Gubler, Am. J. Trop. Med. Hyg., 2020, 103, 570–571 CrossRef PubMed
.
- R. Yousefzai and A. Bhimaraj, JACC: Case Reports, 2020, 1614–1619 Search PubMed
.
- L. Epelboin, R. Blondé, M. Nacher, P. Combe and L. Collet, J. Trav. Med., 2020, 27, taaa114 CrossRef PubMed
.
- J. Stowe, E. Tessier, H. Zhao, R. Guy, B. Muller-Pebody, M. Zambon, N. Andrews, M. Ramsay and J. L. Bernal, medRxiv, 2020 DOI:10.1101/2020.09.18.20189647
.
- D. Kim, J. Quinn, B. Pinsky, N. H. Shah and I. Brown, JAMA, 2020, 2085–2086 CrossRef CAS PubMed
.
- Y. Kondo, S. Miyazaki, R. Yamashita and T. Ikeda, BMJ Case Reports CP, 2020, 13, e236812 CrossRef PubMed
.
- U. Teichgräber, A. Malouhi, M. Ingwersen, R. Neumann, M. Reljic, S. Deinhardt-Emmer, B. Löffler, W. Behringer, J.-C. Lewejohann, A. Stallmach and P. Reuken, Respir. Res., 2021, 22, 13 CrossRef PubMed
.
- T. C. Kwee and R. M. Kwee, RadioGraphics, 2020, 40, 1848–1865 CrossRef PubMed
.
- Y. Wang, C. Dong, Y. Hu, C. Li, Q. Ren, X. Zhang, H. Shi and M. Zhou, Radiology, 2020, 200843 Search PubMed
.
-
E. Duffin, Resident population of the United States by sex and age as of July 1, 2019, https://www.statista.com/statistics/241488/population-of-the-us-by-sex-and-age/.
- I. M. Artika, A. K. Dewantari and A. Wiyatno, Heliyon, 2020, e04743 CrossRef PubMed
.
- Summary of probable SARS cases with onset of illness from 1 November 2002 to 31 July 2003 World Health Organization, 2004.
- L. Van Der Hoek, K. Pyrc, M. F. Jebbink, W. Vermeulen-Oost, R. J. Berkhout, K. C. Wolthers, P. M. Wertheim-van Dillen, J. Kaandorp, J. Spaargaren and B. Berkhout, Nat. Med., 2004, 10, 368–373 CrossRef CAS PubMed
.
- P. C. Woo, S. K. Lau, C.-M. Chu, K.-H. Chan, H.-W. Tsoi, Y. Huang, B. H. Wong, R. W. Poon, J. J. Cai and W.-K. Luk, J. Virol., 2005, 79, 884–895 CrossRef CAS PubMed
.
- A. M. Zaki, S. Van Boheemen, T. M. Bestebroer, A. D. Osterhaus and R. A. Fouchier, N. Engl. J. Med., 2012, 367, 1814–1820 CrossRef CAS PubMed
.
- M. Owusu, A. Annan, V. M. Corman, R. Larbi, P. Anti, J. F. Drexler, O. Agbenyega, Y. Adu-Sarkodie and C. Drosten, PLoS One, 2014, 9, e99782 CrossRef PubMed
.
- R. Dijkman, M. F. Jebbink, E. Gaunt, J. W. Rossen, K. E. Templeton, T. W. Kuijpers and L. van der Hoek, J. Clin. Virol., 2012, 53, 135–139 CrossRef PubMed
.
- N. Friedman, H. Alter, M. Hindiyeh, E. Mendelson, Y. Shemer Avni and M. Mandelboim, Viruses, 2018, 10, 515 CrossRef PubMed
.
- A. Jean, C. Quach, A. Yung and M. Semret, Pediatr. Infect. Dis. J., 2013, 32, 325–329 CrossRef PubMed
.
- I. M. Mackay, K. E. Arden, D. J. Speicher, N. T. O’Neil, P. K. McErlean, R. M. Greer, M. D. Nissen and T. P. Sloots, Viruses, 2012, 4, 637–653 CrossRef CAS PubMed
.
- I. Heimdal, N. Moe, S. Krokstad, A. Christensen, L. H. Skanke, S. A. Nordbø and H. Døllner, J. Infect. Dis., 2019, 219, 1198–1206 CrossRef PubMed
.
- A. W. Edridge, J. Kaczorowska, A. C. Hoste, M. Bakker, M. Klein, K. Loens, M. F. Jebbink, A. Matser, C. M. Kinsella and P. Rueda, Nat. Med., 2020, 1–3 Search PubMed
.
- MERS situation update, World Health Organization, 2020.
- K. Kim, T. Tandi, J. W. Choi, J. Moon and M. Kim, J. Hosp. Infect., 2017, 95, 207–213 CrossRef CAS PubMed
.
- A. Assiri, A. McGeer, T. M. Perl, C. S. Price, A. A. Al Rabeeah, D. A. Cummings, Z. N. Alabdullatif, M. Assad, A. Almulhim and H. Makhdoom, N. Engl. J. Med., 2013, 369, 407–416 CrossRef CAS PubMed
.
- C.-M. Chu, V. C. Cheng, I. F. Hung, K.-S. Chan, B. S. Tang, T. H. Tsang, K.-H. Chan and K.-Y. Yuen, Emerging Infect. Dis., 2005, 11, 1882 CrossRef PubMed
.
- Y.-H. Hsieh, C.-C. King, C. W. Chen, M.-S. Ho, S.-B. Hsu and Y.-C. Wu, J. Theor. Biol., 2007, 244, 729–736 CrossRef PubMed
.
- S. M. Kissler, C. Tedijanto, E. Goldstein, Y. H. Grad and M. Lipsitch, Science, 2020, 368, 860–868 CrossRef CAS PubMed
.
- F. Mauvais-Jarvis, Diabetes, 2020, 69, 1857–1863 CrossRef CAS PubMed
.
- K. Kragholm, M. P. Andersen, T. A. Gerds, J. H. Butt, L. Østergaard, C. Polcwiartek, M. Phelps, C. Andersson, G. H. Gislason and C. Torp-Pedersen, Clin. Infect. Dis., 2020, ciaa924 CrossRef PubMed
.
- L. Shafique, A. Ihsan and Q. Liu, Pathogens, 2020, 9, 240 CrossRef
.
- W. Liu, L. Liu, G. Kou, Y. Zheng, Y. Ding, W. Ni, Q. Wang, L. Tan, W. Wu and S. Tang, J. Clin. Microbiol., 2020, 58, e00461-20 Search PubMed
.
- Q. Ye, A. M. West, S. Silletti and K. D. Corbett, bioRxiv, 2020 DOI:10.1101/2020.05.17.100685
.
- J. Van Elslande, B. Decru, S. Jonckheere, E. Van Wijngaerden, E. Houben, P. Vandecandelaere, C. Indevuyst, M. Depypere, S. Desmet and E. André, Clin. Microbiol. Infect., 2020, 26, 1557. e1551–1557. e1557 Search PubMed
.
- P. D. Burbelo, F. X. Riedo, C. Morishima, S. Rawlings, D. Smith, S. Das, J. R. Strich, D. S. Chertow, R. T. Davey Jr and J. I. Cohen, J. Infect. Dis., 2020, 222, 206–213 CrossRef CAS PubMed
.
- A. Hachim, N. Kavian, C. A. Cohen, A. W. Chin, D. K. Chu, C. K. Mok, O. T. Tsang, Y. C. Yeung, R. A. Perera and L. L. Poon, Nat. Immunol., 2020, 21, 1293–1301 CrossRef CAS
.
- A. K. Azkur, M. Akdis, D. Azkur, M. Sokolowska, W. van de Veen, M. C. Brüggen, L. O’Mahony, Y. Gao, K. Nadeau and C. A. Akdis, Allergy, 2020, 75, 1564–1581 CrossRef CAS PubMed
.
- C. Yi, X. Sun, J. Ye, L. Ding, M. Liu, Z. Yang, X. Lu, Y. Zhang, L. Ma and W. Gu, Cell. Mol. Immunol., 2020, 1–10 Search PubMed
.
- P. Forster, L. Forster, C. Renfrew and M. Forster, Proc. Natl. Acad. Sci. U. S. A., 2020, 117, 9241–9243 CrossRef CAS PubMed
.
- S. Ozono, Y. Zhang, H. Ode, T. S. Tan, K. Imai, K. Miyoshi, S. Kishigami, T. Ueno, Y. Iwatani, T. Suzuki and K. Tokunaga, Nat. Commun., 2021, 12, 848 CrossRef CAS PubMed
.
- T. Koyama, D. Platt and L. Parida, Bull. W. H. O., 2020, 98, 495 CrossRef PubMed
.
- S. Isabel, L. Graña-Miraglia and J. M. Gutierrez,
et al.
, Sci. Rep., 2020, 10, 14031 CrossRef CAS PubMed
.
- B. Korber, W. M. Fischer, S. Gnanakaran, H. Yoon, J. Theiler, W. Abfalterer, N. Hengartner, E. E. Giorgi, T. Bhattacharya and B. Foley, Cell, 2020, 182(812-827), e819 Search PubMed
.
- M. Becerra-Flores and T. Cardozo, Int. J. Clin. Pract., 2020, e13525 CAS
.
- D. Mercatelli and F. M. Giorgi, Front. Microbiol., 2020, 11, 1800 CrossRef PubMed
.
- Q. Guan, M. Sadykov, S. Mfarrej, S. Hala, R. Naeem, R. Nugmanova, A. Al-Omari, S. Salih, A. Al Mutair and M. J. Carr, Int. J. Infect. Dis., 2020, 100, 216–223 CrossRef CAS PubMed
.
- J. Van Elslande, P. Vermeersch, K. Vandervoort, T. Wawina-Bokalanga, B. Vanmechelen, E. Wollants, L. Laenen, E. André, M. Van Ranst, K. Lagrou and P. Maes, Clin. Infect. Dis., 2021, 73, 354–356 CrossRef PubMed
.
- K. K.-W. To, I. F.-N. Hung, J. D. Ip, A. W.-H. Chu, W.-M. Chan, A. R. Tam, C. H.-Y. Fong, S. Yuan, H.-W. Tsoi and A. C.-K. Ng, Clin. Infect. Dis., 2020, ciaa1275 CrossRef PubMed
.
- R. L. Tillett, J. R. Sevinsky, P. D. Hartley, H. Kerwin, N. Crawford, A. Gorzalski, C. Laverdure, S. C. Verma, C. C. Rossetto and D. Jackson, Lancet Infect. Dis., 2020, 21, 52–58 CrossRef PubMed
.
- V. Gupta, R. C. Bhoyar, A. Jain, S. Srivastava, R. Upadhayay, M. Imran, B. Jolly, D. Sharma, P. Sehgal and G. Ranjan, Clin. Infect. Dis., 2020, ciaa1451 CrossRef PubMed
.
- J. D. Goldman, K. Wang, K. Röltgen, S. C. A. Nielsen, J. C. Roach, S. N. Naccache, F. Yang, O. F. Wirz, K. E. Yost, J.-Y. Lee, K. Chun, T. Wrin, C. J. Petropoulos, I. Lee, S. Fallen, P. M. Manner, J. A. Wallick, H. A. Algren, K. M. Murray, Y. Su, J. Hadlock, J. Jeharajah, W. R. Berrington, G. P. Pappas, S. T. Nyatsatsang, A. L. Greninger, A. T. Satpathy, J. S. Pauk, S. D. Boyd and J. R. Heath, medRxiv, 2020 DOI:10.1101/2020.09.22.20192443
.
- B. Prado-Vivar, M. Becerra-Wong, J. J. Guadalupe, S. Marquez, B. Gutierrez, P. Rojas-Silva, M. Grunauer, G. Trueba, V. Barragan and P. Cardenas, SSRN, 2020 DOI:10.2139/ssrn.3686174
.
- E. Hamed, S. Sedeeq, A. S. Alnuaimi, M. Syed, M. A. ElHamid, B. Alemrayat, A. Mukhtar, A. AlFehaidi and H. A/Qotba, J. Infect., 2021, 82, 84–123 Search PubMed
.
- A. Gómez-Carballa, X. Bello, J. Pardo-Seco, F. Martinón-Torres and A. Salas, Genome Res., 2020, 30, 1434–1448 CrossRef PubMed
.
- K. Pyrc, R. Dijkman, L. Deng, M. F. Jebbink, H. A. Ross, B. Berkhout and L. Van der Hoek, J. Mol. Biol., 2006, 364, 964–973 CrossRef CAS PubMed
.
- H. Hofmann, K. Pyrc, L. Van Der Hoek, M. Geier, B. Berkhout and S. Pöhlmann, Proc. Natl. Acad. Sci. U. S. A., 2005, 102, 7988–7993 CrossRef CAS PubMed
.
- Q. Shen, X. Xiao, A. Aierken, W. Yue, X. Wu, M. Liao and J. Hua, J. Cell. Mol. Med., 2020, 24, 9472–9477 CrossRef CAS PubMed
.
- X. Zou, K. Chen, J. Zou, P. Han, J. Hao and Z. Han, Front. Med., 2020, 1–8 CAS
.
- D. S. Dimitrov, Cell, 2003, 115, 652–653 CrossRef CAS
.
- M. Hoffmann, H. Kleine-Weber, S. Schroeder, N. Krüger, T. Herrler, S. Erichsen, T. S. Schiergens, G. Herrler, N.-H. Wu and A. Nitsche, Cell, 2020, 181, 271–280 CrossRef CAS PubMed
.
- R. Yan, Y. Zhang, Y. Li, L. Xia, Y. Guo and Q. Zhou, Science, 2020, 367, 1444–1448 CrossRef CAS PubMed
.
- D. Wrapp, N. Wang, K. S. Corbett, J. A. Goldsmith, C.-L. Hsieh, O. Abiona, B. S. Graham and J. S. McLellan, Science, 2020, 367, 1260–1263 CrossRef CAS PubMed
.
- E. S. Winkler, A. L. Bailey, N. M. Kafai, S. Nair, B. T. McCune, J. Yu, J. M. Fox, R. E. Chen, J. T. Earnest and S. P. Keeler, Nat. Immunol., 2020, 1–9 Search PubMed
.
- P. Zhou, X.-L. Yang, X.-G. Wang, B. Hu, L. Zhang, W. Zhang, H.-R. Si, Y. Zhu, B. Li and C.-L. Huang, Nature, 2020, 579, 270–273 CrossRef CAS PubMed
.
- J. Lan, J. Ge, J. Yu, S. Shan, H. Zhou, S. Fan, Q. Zhang, X. Shi, Q. Wang and L. Zhang, Nature, 2020, 581, 215–220 CrossRef CAS PubMed
.
- A. C. Walls, Y.-J. Park, M. A. Tortorici, A. Wall, A. T. McGuire and D. Veesler, Cell, 2020, 181, 281–292 CrossRef CAS PubMed
.
- M. Hussain, N. Jabeen, F. Raza, S. Shabbir, A. A. Baig, A. Amanullah and B. Aziz, J. Med. Virol., 2020, 92, 1580–1586 CrossRef CAS PubMed
.
- K. Wang, W. Chen and Z. Zhang,
et al.
, Signal Transduction Targeted Ther., 2020, 5, 283 CrossRef CAS PubMed
.
- H. Ulrich and M. M. Pillat, Stem Cell Rev. Rep., 2020, 1–7 Search PubMed
.
- B. Ahmetaj-Shala, R. Vaja, S. S. Atanur, P. M. George, N. S. Kirkby and J. A. Mitchell, JACC: Basic to Translational Science, 2020, 5, 1111–1123 Search PubMed
.
- C. Liu and D. Zhu, Med. Drug Discovery, 2020, 100056 CrossRef PubMed
.
- A. O. Sehirli, S. Sayiner and N. Serakinci, Mol. Biol. Rep., 2020, 1–5 Search PubMed
.
- H. Bian, Z.-H. Zheng, D. Wei, Z. Zhang, W.-Z. Kang, C.-Q. Hao, K. Dong, W. Kang, J.-L. Xia, J.-L. Miao, R.-H. Xie, B. Wang, X.-X. Sun, X.-M. Yang, P. Lin, J.-J. Geng, K. Wang, H.-Y. Cui, K. Zhang, X.-C. Chen, H. Tang, H. Du, N. Yao, S.-S. Liu, L.-N. Liu, Z. Zhang, Z.-W. Gao, G. Nan, Q.-Y. Wang, J.-Q. Lian, Z.-N. Chen and P. Zhumed, Rxiv, 2020 DOI:10.1101/2020.03.21.20040691
.
- U. Radzikowska, M. Ding, G. Tan, D. Zhakparov, Y. Peng, P. Wawrzyniak, M. Wang, S. Li, H. Morita and C. Altunbulakli, Allergy, 2020, 75, 2828–2844 CrossRef PubMed
.
- J. Shilts, T. W. M. Crozier, E. J. D. Greenwood, P. J. Lehner and G. J. Wright, Sci. Rep., 2021, 11, 143 CrossRef PubMed
.
- G. Lu, Y. Hu, Q. Wang, J. Qi, F. Gao, Y. Li, Y. Zhang, W. Zhang, Y. Yuan and J. Bao, Nature, 2013, 500, 227–231 CrossRef CAS PubMed
.
- V. S. Raj, H. Mou, S. L. Smits, D. H. Dekkers, M. A. Müller, R. Dijkman, D. Muth, J. A. Demmers, A. Zaki and R. A. Fouchier, Nature, 2013, 495, 251–254 CrossRef CAS PubMed
.
- N. Vankadari and J. A. Wilce, Emerging Microbes Infect., 2020, 9, 601–604 CrossRef CAS PubMed
.
- H. Li, Y. Wang, M. Ji, F. Pei, Q. Zhao, Y. Zhou, Y. Hong, S. Han, J. Wang and Q. Wang, Front. Cell Dev. Biol., 2020, 8, 618 CrossRef PubMed
.
- M. Klompas, M. A. Baker and C. Rhee, JAMA, 2020, 324, 441–442 CrossRef CAS PubMed
.
- R. Sommerstein, C. A. Fux, D. Vuichard-Gysin, M. Abbas, J. Marschall, C. Balmelli, N. Troillet, S. Harbarth, M. Schlegel and A. Widmer, Antimicrob. Resist. Infect. Contr., 2020, 9, 1–8 CrossRef PubMed
.
- F. Yang, A. A. Pahlavan, S. Mendez, M. Abkarian and H. A. Stone, Physical Review Fluids, 2020, 5, 122501 CrossRef
.
- S. Flaxman, S. Mishra, A. Gandy, H. J. T. Unwin, T. A. Mellan, H. Coupland, C. Whittaker, H. Zhu, T. Berah and J. W. Eaton, Nature, 2020, 584, 257–261 CrossRef CAS PubMed
.
- V. Stadnytskyi, C. E. Bax, A. Bax and P. Anfinrud, Proc. Natl. Acad. Sci. U. S. A., 2020, 117, 11875–11877 CrossRef CAS PubMed
.
- S. Shao, D. Zhou, R. He, J. Li, S. Zou, K. Mallery, S. Kumar, S. Yang and J. Hong, J. Aerosol Sci., 2020, 151, 105661 CrossRef PubMed
.
- D. Mürbe, M. Fleischer, J. Lange, H. Rotheudt and M. Kriegel, OSF Preprints, 2020 DOI:10.31219/osf.io/znjeh
.
- M. Alsved, A. Matamis, R. Bohlin, M. Richter, P.-E. Bengtsson, C.-J. Fraenkel, P. Medstrand and J. Löndahl, Aerosol Sci. Technol., 2020, 54, 1245–1248 CrossRef CAS
.
- A. Endo, S. Abbott, A. J. Kucharski and S. Funk, Wellcome Open Res., 2020, 5, 67 Search PubMed
.
- A. Heinzerling, P. M. J. Stuckey, T. Scheuer, K. Xu, K. M. Perkins, H. Resseger, S. Magill, J. R. Verani, S. Jain and M. Acosta, Morb. Mortal. Wkly. Rep., 2020, 69, 472–476 CrossRef CAS PubMed
.
- L. Morawska and J. Cao, Environ. Int., 2020, 105730 CrossRef CAS PubMed
.
- L. Morawska and D. K. Milton, Clin. Infect. Dis., 2020, 71, 2311–2313 CAS
.
- A. Heinzerling, P. M. J. Stuckey, T. Scheuer, K. Xu, K. M. Perkins, H. Resseger, S. Magill, J. R. Verani, S. Jain and M. Acosta, Morb. Mortal. Wkly. Rep., 2020, 69, 472–476 CrossRef CAS PubMed
.
- D. Buitrago-Garcia, D. Egli-Gany, M. J. Counotte, S. Hossmann, H. Imeri, A. M. Ipekci, G. Salanti and N. Low, PLoS Med., 2020, 17, e1003346 CrossRef CAS PubMed
.
- W. Sungnak, N. Huang, C. Bécavin, M. Berg, R. Queen, M. Litvinukova, C. Talavera-López, H. Maatz, D. Reichart and F. Sampaziotis, Nat. Med., 2020, 26, 681–687 CrossRef CAS PubMed
.
- C. Wu and M. Zheng, medRxiv, 2020 DOI:10.1101/2020.02.11.20022228
.
- S. N. Mueller and B. T. Rouse, Clin. Immunol., 2008, 421 Search PubMed
.
-
S. L. Klein and S. Huber, Sex hormones and immunity to infection, Springer, 2010, pp. 93–122 Search PubMed
.
- S. L. Klein, BioEssays, 2012, 34, 1050–1059 CrossRef CAS PubMed
.
- Z. Gao, Y. Xu, Y. Guo, D. Xu, L. Zhang, X. Wang, C. Sun, S. Qiu and K. Ma, Infect., Genet. Evol., 2020, 104494 CrossRef CAS PubMed
.
- H.-w. Jiang, H.-n. Zhang, Q.-f. Meng, J. Xie, Y. Li, H. Chen, Y.-x. Zheng, X.-n. Wang, H. Qi and J. Zhang, Cell. Mol. Immunol., 2020, 17, 998–1000 CrossRef CAS PubMed
.
- R. Zhou, K. K.-W. To, Y.-C. Wong, L. Liu, B. Zhou, X. Li, H. Huang, Y. Mo, T.-Y. Luk and T. T.-K. Lau, Immunity, 2020, 53, 864–877 CrossRef CAS PubMed
.
- A. Bouayad, Rev. Med. Virol., 2020, e2135 Search PubMed
.
- Q.-X. Long, B.-Z. Liu, H.-J. Deng, G.-C. Wu, K. Deng, Y.-K. Chen, P. Liao, J.-F. Qiu, Y. Lin and X.-F. Cai, Nat. Med., 2020, 1–4 CAS
.
- J. Prévost, R. Gasser, G. Beaudoin-Bussières, J. Richard, R. Duerr, A. Laumaea, S. P. Anand, G. Goyette, M. Benlarbi and S. Ding, Cell Rep. Med., 2020, 1, 100126 CrossRef PubMed
.
- J. Seow, C. Graham and B. Merrick,
et al.
, Nat. Microbiol., 2020, 5, 1598–1607 CrossRef CAS PubMed
.
- B. Lou, T.-D. Li, S.-F. Zheng, Y.-Y. Su, Z.-Y. Li, W. Liu, F. Yu, S.-X. Ge, Q.-D. Zou and Q. Yuan, Eur. Respir. J., 2020, 2000763 CrossRef CAS PubMed
.
- N. M. A. Okba, M. A. Müller, W. Li, C. Wang, C. H. GeurtsvanKessel, V. M. Corman, M. M. Lamers, R. S. Sikkema, E. de Bruin, F. D. Chandler, Y. Yazdanpanah, Q. Le Hingrat, D. Descamps, N. Houhou-Fidouh, C. B. E. M. Reusken, B.-J. Bosch, C. Drosten, M. P. G. Koopmans and B. L. Haagmans, medRxiv, 2020 DOI:10.1101/2020.2003.2018.20038059
.
- J. Qu, C. Wu, X. Li, G. Zhang, Z. Jiang, X. Li, Q. Zhu and L. Liu, Clin. Infect. Dis., 2020, 71, 2255–2258 CrossRef CAS PubMed
.
- P. K. Kiyuka, C. N. Agoti, P. K. Munywoki, R. Njeru, A. Bett, J. R. Otieno, G. P. Otieno, E. Kamau, T. G. Clark and L. van der Hoek, J. Infect. Dis., 2018, 217, 1728–1739 CrossRef CAS PubMed
.
- P. Kellam and W. Barclay, J. Gen. Virol., 2020, 101, 791–797 CrossRef CAS PubMed
.
- J.-W. Song, C. Zhang, X. Fan, F.-P. Meng, Z. Xu, P. Xia, W.-J. Cao, T. Yang, X.-P. Dai and S.-Y. Wang, Nat. Commun., 2020, 11, 1–10 Search PubMed
.
- W. Liu, A. Fontanet, P.-H. Zhang, L. Zhan, Z.-T. Xin, L. Baril, F. Tang, H. Lv and W.-C. Cao, J. Infect. Dis., 2006, 193, 792–795 CrossRef PubMed
.
- A. Grifoni, D. Weiskopf, S. I. Ramirez, J. Mateus, J. M. Dan, C. R. Moderbacher, S. A. Rawlings, A. Sutherland, L. Premkumar and R. S. Jadi, Cell, 2020, 181, 1489–1501 CrossRef CAS PubMed
.
- J. Braun, L. Loyal, M. Frentsch, D. Wendisch, P. Georg, F. Kurth, S. Hippenstiel, M. Dingeldey, B. Kruse and F. Fauchere, Nature, 2020, 1–5 Search PubMed
.
-
H. Ward, G. Cooke, C. Atchison, M. Whitaker, J. Elliott, M. Moshe, J. C. Brown, B. Flower, A. Daunt, K. Ainslie, D. Ashby, C. Donnelly, S. Riley, A. Darzi, W. Barclay, P. Elliottand for the REACT study teammedRxiv, 2020 DOI:10.1101/2020.10.26.20219725.
- A. S. Iyer, F. K. Jones, A. Nodoushani, M. Kelly, M. Becker, D. Slater, R. Mills, E. Teng, M. Kamruzzaman and W. F. Garcia-Beltran, Sci. Immunol., 2020, 5, eabe0367 CrossRef PubMed
.
- M. M. Patel, N. J. Thornburg, W. B. Stubblefield, H. K. Talbot, M. M. Coughlin, L. R. Feldstein and W. H. Self, JAMA, 2020, 324, 1781–1782 CrossRef CAS PubMed
.
- W.’H. Self, M. W. Tenforde and W. B. Stubblefield,
et al.
, Morb. Mortal. Wkly. Rep., 2020, 69, 1762–1766 CrossRef CAS PubMed
.
- G. Moncunill, A. Mayor, R. Santano, A. Jiménez, M. Vidal, M. Tortajada, S. Sanz, S. Méndez, A. Llupià and R. Aguilar, J. Infect. Dis., 2021, 223, 62–71 CrossRef CAS PubMed
.
- B. Isho, K. T. Abe, M. Zuo, A. J. Jamal, B. Rathod, J. H. Wang, Z. Li, G. Chao, O. L. Rojas and Y. M. Bang, Sci. Immunol., 2020, 5, eabe5511 CrossRef PubMed
.
- L. B. Rodda, J. Netland, L. Shehata, K. B. Pruner, P. A. Morawski, C. D. Thouvenel, K. K. Takehara, J. Eggenberger, E. A. Hemann and H. R. Waterman, Cell, 2020, 184, 169–183 CrossRef PubMed
.
- J. M. Dan, J. Mateus and Y. Kato,
et al.
, Science, 2021, 371, eabf4063 CrossRef CAS PubMed
.
- T. L. Dao and P. Gautret, Eur. J. Clin. Microbiol. Infect. Dis., 2020, 1–13 Search PubMed
.
- Q. Mei, J. Li, R. Du, X. Yuan, M. Li and J. Li, Lancet Infect. Dis., 2020, 20, 1004–1005 CrossRef CAS PubMed
.
- N. Baumgarth, J. Nikolich-Žugich, F. E.-H. Lee and D. Bhattacharya, J. Immunol., 2020, 205, 2342–2350 CrossRef CAS PubMed
.
- P. Habibzadeh, M. M. Sajadi, A. Emami, M. H. Karimi, M. Yadollahie, M. Kucheki, S. Akbarpoor and F. Habibzadeh, Biochem. Med., 2020, 30, 355–356 CrossRef
.
- K. Callow, H. Parry, M. Sergeant and D. Tyrrell, Epidemiol. Infect., 1990, 105, 435–446 CrossRef CAS PubMed
.
- X. Chi, R. Yan, J. Zhang, G. Zhang, Y. Zhang, M. Hao, Z. Zhang, P. Fan, Y. Dong and Y. Yang, Science, 2020, 369, 650–655 CrossRef CAS PubMed
.
- Y. Weisblum, F. Schmidt, F. Zhang, J. DaSilva, D. Poston, J. C. Lorenzi, F. Muecksch, M. Rutkowska, H.-H. Hoffmann and E. Michailidis, eLife, 2020, 9, e61312 CrossRef CAS PubMed
.
- S. Tomassini, D. Kotecha, P. W. Bird, A. Folwell, S. Biju and J. W. Tang, J. Infect., 2020, 82, 282–327 CrossRef PubMed
.
- F. Amanat and F. Krammer, Immunity, 2020, 52, 583–589 CrossRef CAS PubMed
.
- F. Krammer, Nature, 2020, 586, 516–527 CrossRef CAS PubMed
.
- T. T. Le, Z. Andreadakis, A. Kumar, R. G. Roman, S. Tollefsen, M. Saville and S. Mayhew, Nat. Rev. Drug Discovery, 2020, 19, 305–306 CrossRef PubMed
.
- M. Jeyanathan, S. Afkhami, F. Smaill, M. S. Miller, B. D. Lichty and Z. Xing, Nat. Rev. Immunol., 2020, 1–18 Search PubMed
.
- S. P. Kaur and V. Gupta, Virus Res., 2020, 198114 CrossRef CAS PubMed
.
- M. D. Shin, S. Shukla, Y. H. Chung, V. Beiss, S. K. Chan, O. A. Ortega-Rivera, D. M. Wirth, A. Chen, M. Sack and J. K. Pokorski, Nat. Nanotechnol., 2020, 15, 646–655 CrossRef CAS PubMed
.
- J. Routh, National Institutes of Health press release, 2020 Search PubMed
, https://www.nih.gov/news-events/news-releases/fourth-large-scale-covid-19-vaccine-trial-begins-united-states.
- E. E. Walsh, R. Frenck, A. R. Falsey, N. Kitchin, J. Absalon, A. Gurtman, S. Lockhart, K. Neuzil, M. J. Mulligan and R. Bailey,
et al.
, medRxiv, 2020 DOI:10.1101/2020.08.17.20176651
.
- J. Bowden, HHS secretary: FDA will move ‘as quickly as possible’ on Moderna, Pfizer vaccines, The Hill, 2020 Search PubMed
, https://thehill.com/policy/healthcare/public-global-health/526113-hhs-secretary-fda-moderna-pfizer-vaccines-coronavirus.
- GVN Statement on Pfizer and BioNTech Data from COVID-19 Vaccine Study, Global Virus Network, 2020.
-
J. Palca, Moderna's COVID-19 Vaccine Shines In Clinical Trial, National Public Radio, NPR, 2020 Search PubMed
.
- X. Yuan, C. Yang, Q. He, J. Chen, D. Yu, J. Li, S. Zhai, Z. Qin, K. Du, Z. Chu and P. Qin, ACS Infect. Dis., 2020, 6, 1998–2016 CrossRef CAS PubMed
.
- E. Sheikhzadeh, S. Eissa, A. Ismail and M. Zourob, Talanta, 2020, 220, 121392 CrossRef CAS PubMed
.
- L. J. Carter, L. V. Garner, J. W. Smoot, Y. Li, Q. Zhou, C. J. Saveson, J. M. Sasso, A. C. Gregg, D. J. Soares, T. R. Beskid, S. R. Jervey and C. Liu, ACS Cent. Sci., 2020, 6, 591–605 CrossRef CAS PubMed
.
- O. Vandenberg, D. Martiny, O. Rochas, A. van Belkum and Z. Kozlakidis, Nat. Rev. Microbiol., 2020, 19, 171–183 CrossRef PubMed
.
- M. Venter and K. Richter, J. Clin. Pathol., 2020, 73, 370 CrossRef CAS PubMed
.
- M. L. Wong and J. F. Medrano, Biotechniques, 2005, 39, 75–85 CrossRef CAS PubMed
.
- I. M. Mackay, K. E. Arden and A. Nitsche, Nucleic Acids Res., 2002, 30, 1292–1305 CrossRef CAS PubMed
.
- Y. Yan, L. Chang, W. Luo, J. Liu, F. Guo and L. Wang, J. Mol. Diagn., 2021, 23, 300–309 CrossRef CAS PubMed
.
- X. He, E. H. Lau, P. Wu, X. Deng, J. Wang, X. Hao, Y. C. Lau, J. Y. Wong, Y. Guan and X. Tan, Nat. Med., 2020, 26, 672–675 CrossRef CAS PubMed
.
- Y.-H. Xing, W. Ni, Q. Wu, W.-J. Li, G.-J. Li, W.-D. Wang, J.-N. Tong, X.-F. Song, G. W.-K. Wong and Q.-S. Xing, J. Microbiol., Immunol. Infect., 2020, 53, 473–480 CrossRef CAS PubMed
.
- Y. Sohn, S. J. Jeong, W. S. Chung, J. H. Hyun, Y. J. Baek, Y. Cho, J. H. Kim, J. Y. Ahn, J. Y. Choi and J.-S. Yeom, J. Clin. Med., 2020, 9, 2924 CrossRef CAS PubMed
.
- A. Afzal, J. Adv. Res., 2020, 149–159 CrossRef CAS PubMed
.
- J. B. Mahony, G. Blackhouse, J. Babwah, M. Smieja, S. Buracond, S. Chong, W. Ciccotelli, T. O'Shea, D. Alnakhli and M. Griffiths-Turner, J. Clin. Microbiol., 2009, 47, 2812–2817 CrossRef PubMed
.
- K. Ramdas, A. Darzi and S. Jain, Nat. Med., 2020, 1–2 Search PubMed
.
- C. Sheridan, Nat. Biotechnol., 2020, 38, 515–518 CrossRef CAS PubMed
.
- D. Thompson and Y. Lei, Sens. Actuator Rep., 2020, 2, 100017 Search PubMed
.
- A. Gansen, A. M. Herrick, I. K. Dimov, L. P. Lee and D. T. Chiu, Lab Chip, 2012, 12, 2247–2254 RSC
.
- V. L. Dao Thi, K. Herbst, K. Boerner, M. Meurer, L. P. M. Kremer, D. Kirrmaier, A. Freistaedter, D. Papagiannidis, C. Galmozzi, M. L. Stanifer, S. Boulant, S. Klein, P. Chlanda, D. Khalid, I. Barreto Miranda, P. Schnitzler, H.-G. Kräusslich, M. Knop and S. Anders, Sci. Transl. Med., 2020, 12, eabc7075 CrossRef CAS PubMed
.
- J. J. Schellenberg, M. Ormond and Y. Keynan, J. Clin. Virol., 2021, 136, 104764 CrossRef CAS PubMed
.
- R. Pelton, TrAC, Trends Anal. Chem., 2009, 28, 925–942 CrossRef CAS PubMed
.
- P. Kumar, Y. S. Malik, B. Ganesh, S. Rahangdale, S. Saurabh, S. Natesan, A. Srivastava, K. Sharun, M. I. Yatoo, R. Tiwari, R. K. Singh and K. Dhama, Front. Cell. Infect. Microbiol., 2020, 10, 639 Search PubMed
.
- J. S. Gootenberg, O. O. Abudayyeh, M. J. Kellner, J. Joung, J. J. Collins and F. Zhang, Science, 2018, 360, 439 CrossRef CAS PubMed
.
- J. Joung, A. Ladha and M. Saito,
et al.
, N. Engl. J. Med., 2020, 383, 1492–1494 CrossRef CAS PubMed
.
- L. Guo, X. Sun, X. Wang, C. Liang, H. Jiang, Q. Gao, M. Dai, B. Qu, S. Fang, Y. Mao, Y. Chen, G. Feng, Q. Gu, R. R. Wang, Q. Zhou and W. Li, Cell Discovery, 2020, 6, 34 CrossRef CAS PubMed
.
- A. K. Kaushik, J. S. Dhau, H. Gohel, Y. K. Mishra, B. Kateb, N.-Y. Kim and D. Y. Goswami, ACS Appl. Bio Mater., 2020, 3, 7306–7325 CrossRef CAS
.
- H. Zhao, F. Liu, W. Xie, T.-C. Zhou, J. OuYang, L. Jin, H. Li, C.-Y. Zhao, L. Zhang, J. Wei, Y.-P. Zhang and C.-P. Li, Sens. Actuators, B, 2021, 327, 128899 CrossRef CAS PubMed
.
- A. Ahmadivand, B. Gerislioglu, Z. Ramezani, A. Kaushik, P. Manickam and S. A. Ghoreishi, Biosens. Bioelectron., 2021, 177, 112971 CrossRef CAS PubMed
.
- G. Qiu, Z. Gai, Y. Tao, J. Schmitt, G. A. Kullak-Ublick and J. Wang, ACS Nano, 2020, 14, 5268–5277 CrossRef CAS PubMed
.
-
B. O’Farrell, in Lateral Flow Immunoassay, ed. R. Wong and H. Tse, Humana Press, Totowa, NJ, 2009, pp. 1–33 DOI:10.1007/978-1-59745-240-3_1
.
- S. Eustis and M. A. El-Sayed, Chem. Soc. Rev., 2006, 35, 209–217 RSC
.
- D. Hristov, A. J. Pimentel, G. Ujialele and K. Hamad-Schifferli, ACS Appl. Mater. Interfaces, 2020, 12, 34620–34629 CrossRef CAS PubMed
.
- D. R. Hristov, C. Rodriguez-Quijada, J. Gomez-Marquez and K. Hamad-Schifferli, Sensors, 2019, 19, 554 CrossRef PubMed
.
-
S. Rosen, in Lateral Flow Immunoassay, ed. R. Wong and H. Tse, Humana Press, Totowa, NJ, 2009, pp. 1–15 DOI:10.1007/978-1-59745-240-3_2
.
- H. Kim, D.-R. Chung and M. Kang, Analyst, 2019, 144, 2460–2466 RSC
.
- N. P. Pai, C. Vadnais, C. Denkinger, N. Engel and M. Pai, PLoS Med., 2012, 9, e1001306 CrossRef PubMed
.
- F. M. Liotti, G. Menchinelli, E. Lalle, I. Palucci, S. Marchetti, F. Colavita, M. La Sorda, G. Sberna, L. Bordi, M. Sanguinetti, P. Cattani, M. R. Capobianchi and B. Posteraro, Clin. Microbiol. Infect., 2021, 27, 487–488 CrossRef CAS PubMed
.
- S. Lambert-Niclot, A. Cuffel, S. Le Pape, C. Vauloup-Fellous, L. Morand-Joubert, A.-M. Roque-Afonso, J. Le Goff and C. Delaugerre, J. Clin. Microbiol., 2020, 58, e00977–e00920 CrossRef CAS PubMed
.
- C. Rodríguez-Quijada, J. Gomez-Marquez and K. Hamad-Schifferli, ACS Nano, 2020, 14, 6626–6635 CrossRef PubMed
.
- C.-W. Yen, H. de Puig, J. Tam, J. Gómez-Márquez, I. Bosch, K. Hamad-Schifferli and L. Gehrke, Lab Chip, 2015, 15, 1638–1641 RSC
.
- D. Hristov, H. Rijal, G.-M. Jose and K. Hamad, ChemRxiv, 2020 DOI:10.26434/chemrxiv.13476738.v1
.
- D. Cate, H. Hsieh, V. Glukhova, J. D. Bishop, H. G. Hermansky, B. Barrios-Lopez, B. D. Grant, C. E. Anderson, E. Spencer, S. Kuhn, R. Gallagher, R. Rivera, C. Bennett, S. A. Byrnes, J. T. Connelly, P. K. Dewan, D. S. Boyle, B. H. Weigl and K. P. Nichols, ChemRxiv, 2020 DOI:10.26434/chemrxiv.12709538.v1
.
- D. Hristov, H. Rijal, J. Gomez-Marquez and K. Hamad-Schifferli, Anal. Chem., 2021, 93, 7825–7832 CrossRef CAS PubMed
.
- Y. Hirotsu, M. Maejima, M. Shibusawa, Y. Nagakubo, K. Hosaka, K. Amemiya, H. Sueki, M. Hayakawa, H. Mochizuki, T. Tsutsui, Y. Kakizaki, Y. Miyashita, S. Yagi, S. Kojima and M. Omata, Int. J. Infect. Dis., 2020, 99, 397–402 CrossRef CAS PubMed
.
- L. Russo, M. Sanchez-Purra, C. Rodríguez-Quijada, B. M. Leonardo, V. Puntes and K. Hamad-Schifferli, Nanoscale, 2019, 11, 10819–10827 RSC
.
- M. Sánchez-Purrà, M. Carré Camps, H. de Puig Guixé, I. Bosch, L. Gehrke and K. Hamad-Schifferli, ACS Infect. Dis., 2017, 3, 767–776 CrossRef PubMed
.
- M. Sanchez-Purra, B. Roig-Solvas, C. Rodríguez-Quijada, B. Leonardo and K. Hamad-Schifferli, ACS Omega, 2018, 3, 10733–10742 CrossRef CAS PubMed
.
- E. Juntunen, T. Myyryläinen, T. Salminen, T. Soukka and K. Pettersson, Anal. Biochem., 2012, 428, 31–38 CrossRef CAS PubMed
.
- J. Liu, B. Du, P. Zhang, M. Haleyurgirisetty, J. Zhao, V. Ragupathy, S. Lee, D. L. DeVoe and I. K. Hewlett, Biosens. Bioelectron., 2014, 61, 177–183 CrossRef CAS PubMed
.
- G. Seo, G. Lee, M. J. Kim, S.-H. Baek, M. Choi, K. B. Ku, C.-S. Lee, S. Jun, D. Park, H. G. Kim, S.-J. Kim, J.-O. Lee, B. T. Kim, E. C. Park and S. I. Kim, ACS Nano, 2020, 14, 5135–5142 CrossRef CAS PubMed
.
- B. D. Ventura, M. Cennamo, A. Minopoli, R. Campanile, S. B. Censi, D. Terracciano, G. Portella and R. Velotta, ACS Sens., 2020, 5, 3043–3048 CrossRef CAS PubMed
.
- K. T. C. Babak, Y. Moghadam and J. D. Posner, Anal. Chem., 2015, 87, 1009–1017 CrossRef PubMed
.
- Z. Qin, W. C. W. Chan, D. R. Boulware, T. Akkin, E. K. Butler and J. C. Bischof, Angew. Chem., Int. Ed., 2012, 51, 4358–4361 CrossRef CAS PubMed
.
- D. W. Denning, A. Kilcoyne and C. Ucer, Br. Dent. J., 2020, 229, 521–524 CrossRef PubMed
.
- A. La Marca, M. Capuzzo, T. Paglia, L. Roli, T. Trenti and S. M. Nelson, Reprod. Biomed. Online, 2020, 41, 483–499 CrossRef CAS PubMed
.
- S. Kasetsirikul, M. Umer, N. Soda, K. R. Sreejith, M. J. A. Shiddiky and N.-T. Nguyen, Analyst, 2020, 145, 7680–7686 RSC
.
- J. D. Whitman, J. Hiatt and C. T. Mowery,
et al.
, Nat. Biotechnol., 2020, 38, 1174–1183 CrossRef CAS PubMed
.
- J. Van Elslande, E. Houben, M. Depypere, A. Brackenier, S. Desmet, E. André, M. Van Ranst, K. Lagrou and P. Vermeersch, Clin. Microbiol. Infect., 2020, 26, 1082–1087 CrossRef CAS PubMed
.
- M. Tré-Hardy, A. Wilmet, I. Beukinga, J. Favresse, J.-M. Dogné, J. Douxfils and L. Blairon, J. Med. Virol., 2021, 93, 803–811 CrossRef PubMed
.
- M. Lisboa Bastos, G. Tavaziva, S. K. Abidi, J. R. Campbell, L.-P. Haraoui, J. C. Johnston, Z. Lan, S. Law, E. MacLean, A. Trajman, D. Menzies, A. Benedetti and F. Ahmad Khan, BMJ, 2020, 370, m2516 CrossRef PubMed
.
- E. R. Adams, M. Ainsworth and R. Anand,
et al.
, Wellcome Open Res., 2020, 5, 139 Search PubMed
.
- M. M. Serrano, D. N. Rodríguez, N. T. Palop, R. O. Arenas, M. M. Córdoba, M. D. O. Mochón and C. G. Cardona, J. Clin. Virol., 2020, 129, 104529 CrossRef CAS PubMed
.
- H. Liu, E. Dai, R. Xiao, Z. Zhou, M. Zhang, Z. Bai, Y. Shao, K. Qi, J. Tu, C. Wang and S. Wang, Sens. Actuators, B, 2020, 129196 Search PubMed
.
- Z. Chen, Z. Zhang, X. Zhai, Y. Li, L. Lin, H. Zhao, L. Bian, P. Li, L. Yu, Y. Wu and G. Lin, Anal. Chem., 2020, 92, 7226–7231 CrossRef CAS PubMed
.
- G. E. Fridley, C. A. Holstein, S. B. Oza and P. Yager, MRS Bull., 2013, 38, 326–330 CrossRef CAS
.
- E. Fu, B. Lutz, P. Kauffman and P. Yager, Lab Chip, 2010, 10, 918–920 RSC
.
- C. Parolo, M. Medina-Sánchez, A. de la Escosura-Muñiz and A. Merkoçi, Lab Chip, 2013, 13, 386–390 RSC
.
-
P. Chun, in Lateral Flow Immunoassay, ed. R. Wong and H. Tse, Humana Press, Totowa, NJ, 2009, pp. 1–19 DOI:10.1007/978-1-59745-240-3_5
.
- J. O. Tam, H. de Puig, C.-W. Yen, I. Bosch, J. Gómez-Márquez, C. Clavet, K. Hamad-Schifferli and L. Gehrke, J. Immunoassay Immunochem., 2017, 38, 355–377 CrossRef CAS PubMed
.
- D. R. Hristov, H. Lopez, Y. Ortin, K. O'Sullivan, K. A. Dawson and D. F. Brougham, Nanoscale, 2021, 13, 5344–5355 RSC
.
- H. de Puig, I. Bosch, M. Carre-Camps and K. Hamad-Schifferli, Bioconjugate Chem., 2017, 28, 230–238 CrossRef CAS PubMed
.
- L. Soddu, D. N. Trinh, E. Dunne, D. Kenny, G. Bernardini, I. Kokalari, A. Marucco, M.
P. Monopoli and I. Fenoglio, Beilstein J. Nanotechnol., 2020, 11, 550–567 CrossRef CAS PubMed
.
- A. Salvati, A. S. Pitek, M. P. Monopoli, K. Prapainop, F. B. Bombelli, D. R. Hristov, P. M. Kelly, C. Åberg, E. Mahon and K. A. Dawson, Nat. Nanotechnol., 2013, 8, 137–143 CrossRef CAS PubMed
.
- M. P. Monopoli, C. Åberg, A. Salvati and K. A. Dawson, Nat. Nanotechnol., 2012, 7, 779–786 CrossRef CAS PubMed
.
- R. Cai and C. Chen, Adv. Mater., 2019, 31, 1805740 CrossRef CAS PubMed
.
- F. Schaumburg, P. A. Kler and C. L. A. Berli, Sens. Actuators, B, 2018, 259, 1099–1107 CrossRef CAS
.
-
Z. Allam, in Surveying the Covid-19 Pandemic and its Implications, ed. Z. Allam, Elsevier, 2020, pp. 71–79, DOI: DOI:10.1016/B978-0-12-824313-8.00004-8
.
- M. A. Mujawar, H. Gohel, S. K. Bhardwaj, S. Srinivasan, N. Hickman and A. Kaushik, Mater. Today Chem., 2020, 17, 100306 CrossRef CAS PubMed
.
- S. Jain, M. Nehra, R. Kumar, N. Dilbaghi, T. Hu, S. Kumar, A. Kaushik and C.-Z. Li, Biosens. Bioelectron., 2021, 179, 113074 CrossRef CAS PubMed
.
- P. Paliwal, S. Sargolzaei, S. K. Bhardwaj, V. Bhardwaj, C. Dixit and A. Kaushik, Frontiers in Nanotechnology, 2020, 2, 5 CrossRef
.
- I. O. Ogunkola, Y. A. Adebisi, U. F. Imo, G. O. Odey, E. Esu and D. E. Lucero-Prisno Iii, Int. J. Health Plann. Manag., 2020, 35, 1302–1305 CrossRef PubMed
.
- A. K. Giri and D. R. Rana, Biosafety and Health, 2020, 2, 53–56 CrossRef
.
- J. Gomez-Marquez and K. Hamad-Schifferli, Adv. Healthcare Mater., 2019, 1900184, DOI:10.1002/adhm.201900184,
.
- P. Ondoa, Y. Kebede, M. M. Loembe, J. N. Bhiman, S. K. Tessema, A. Sow, A. A. Sall and J. Nkengasong, Lancet Microbe, 2020, 1, e103–e104 CrossRef CAS PubMed
.
- A. Olalekan, B. Iwalokun, O. M. Akinloye, O. Popoola, T. A. Samuel and O. Akinloye, Afr. J. Lab. Med., 2020, 9, a1255 CAS
.
- M. Peplow, Chem. Eng. News, 2020, 98, 27 Search PubMed
.
- J. Gomez-Marquez and K. Hamad-Schifferli, Nat. Nanotechnol., 2020, 16, 484–486 CrossRef PubMed
.
- C. Gortazar, L. A. Reperant and T. Kuiken,
et al.
, PLoS Pathog., 2014, 10, e1004129 CrossRef PubMed
.
- C. M. Saad-Roy, C. E. Wagner, R. E. Baker, S. E. Morris, J. Farrar, A. L. Graham, S. A. Levin, M. J. Mina, C. J. E. Metcalf and B. T. Grenfell, Science, 2020, 370, 811 CrossRef CAS PubMed
.
- F. G. Sandmann, N. G. Davies and A. Vassall,
et al.
, Lancet Infect. Dis., 2021, 21, 962–974 CrossRef CAS PubMed
.
- H. C. Yalcin and A. Kaushik, Emergent Mater., 2021, 4, 1–2 CrossRef CAS PubMed
.
|
This journal is © The Royal Society of Chemistry 2021 |
Click here to see how this site uses Cookies. View our privacy policy here.