Proteomic characterization of phagocytic primary human monocyte-derived macrophages†
Received
15th March 2022
, Accepted 12th May 2022
First published on 12th May 2022
Abstract
Macrophages play a vital role in the innate immune system, identifying and destroying unwanted cells. However, it has been difficult to attain a comprehensive understanding of macrophage protein abundance due to technical limitations. In addition, it remains unclear how changes in proteome composition are linked to phagocytic activity. In this study we developed methods to derive human macrophages and prepare them for mass spectrometry analysis in order to more-deeply understand the proteomic consequences of macrophage stimulation. Interferon gamma (IF-g), an immune stimulating cytokine, was used to induce macrophage activation, increasing phagocytosis of cancer cells by 2-fold. These conditions were used to perform comparative shotgun proteomics between resting macrophages and stimulated macrophages with increased phagocytic activity. Our analysis revealed that macrophages bias their protein production toward biological processes associated with phagocytosis and antigen processing in response to stimulation. We confirmed our findings by antibody-based western blotting experiments, validating both previously reported and novel proteins of interest. In addition to whole protein changes, we evaluated active protein synthesis by treating cells with the methionine surrogate probe homopropargylglycine (HPG). We saw increased rates of HPG incorporation during phagocytosis-inducing stimulation, suggesting protein synthesis rates are altered by stimulation. Together our findings provide the most comprehensive proteomic insight to date into primary human macrophages. We anticipate that this data can be used as a launchpoint to generate new hypotheses about innate immune function.
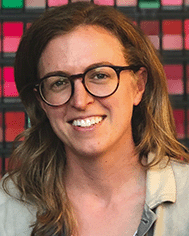
Balyn Zaro
| Balyn Zaro, PhD is an Assistant Professor at UC San Francisco. Her lab leverages chemical biology and proteomics to study the innate immune system. She is particularly interested in how macrophages recognize self and non-self. She completed her PhD studies in chemical biology in the laboratory of Matthew Pratt, PhD, where she developed chemical reporters of protein glycosylation. For her postdoctoral studies, she worked in the laboratory of Ben Cravatt, PhD, utilizing chemical proteomics to characterize protein reactivity of covalent drugs. Prior to the start of her independent career, she gained additional training in innate immunity with Irving Weissman, MD. |
Introduction
Macrophage surveillance is a critical component of the innate immune system. In healthy individuals, macrophages circulate and engage both cells and pathogens in order to identify foreign or apoptotic/exhausted cells for programmed cell removal (PrCR).1 This 3-step process involves recognition, phagocytosis (or engulfment) and lysosomal digestion. In response to external stimuli, macrophages can increase their ability to perform PrCR, and this has been demonstrated during infection and inflammation.2 However, the exact mechanisms driving PrCR regulation remain poorly understood. Challenges currently facing the field include the need for primary human terminally differentiated cells and complexity surrounding the study of cell–cell interactions.
The process of recognition between a macrophage and another cell is regulated by proteins referred to as ‘eat me’ or ‘don’t eat me’ signal ligands and receptors. Signal proteins on the surface of cells can engage receptors on the surface of macrophages and either promote or inhibit downstream signals of phagocytosis.3 To date, there are 4 known ‘don’t eat me’ ligand-receptor pairs, but evidence suggests there are others.4–7 Several ‘eat me’ signals are also known, but again the complete repertoire of ligand/receptor pairs is unknown.8–11
Diseased cells evade macrophage-mediated PrCR by upregulating expression of ‘don’t eat me’ ligands. This phenomenon has been demonstrated in cancer, infection, neurodegeneration and atherosclerosis.4,12–14 Therapeutic blockade of these proteins increases the ability of macrophages to clear diseased or infected cells.15 These reagents are currently being evaluated in the clinic for the treatment of pre-malignancies and malignancies.16,17 Despite the broad interest in developing therapeutics that increase phagocytosis of infected or diseased cells and pathogens, the mechanism by which this increase occurs is not entirely understood. In addition, the macrophage side of the interaction is even less well-characterized. The role of the macrophage in regulating recognition and phagocytosis has not been well-studied, again due to the technical challenges of studying terminally differentiated cells.
Given the ubiquity of macrophage-mediated PrCR in basic and disease biology, there is much interest in understanding the repertoire of proteins detectable in primary monocyte-derived human macrophages and characterizing how protein abundance is altered in response to stimuli that promote phagocytosis. Several groups have previously performed mass spectrometry (MS) analysis on human monocyte-derived macrophages, but these studies have been centered on highly specific perturbations, involved mixed cell populations, required extensive instrument time (up to 18 h per sample), used significant sample input, or applied differentiation conditions which could inadvertently alter macrophage protein expression.18–21 Other previous works have employed immortalized cell lines, which are macrophage-like and capable of phagocytosis, but lack many of the features of a terminally differentiated cell.22,23 Transcriptomic analyses have also been conducted, but reports by ourselves and others have shown that RNA and protein are not well correlated.24,25 Finally, CRISPR screens for genes critical for phagocytosis have been conducted in macrophage-like cell lines, but remain technically challenging in primary cells.26
Recent advances in MS instrumentation and analysis have allowed us to deeply profile cellular proteomes from much fewer cells with greater confidence than ever before.27 As a result, proteomic characterization of rare primary cell types is feasible and generates reliable, high-quality datasets. In addition, chemical tools such as Bio-Orthogonal Non-Canonical Amino acid Tagging (BONCAT) have been developed to capture protein synthesis within a constrained time window.28 This approach utilizes a methionine surrogate equipped with a biorthogonal chemical handle, for example homopropargylglycine (HPG). Methionine probes such as HPG are amenable to Cu(I)-catalyzed azide-alkyne cycloaddition (CuAAC) with a corresponding enrichment or fluorescent tag for subsequent identification or visualization.29 These unnatural amino acids can be supplemented in media to resolve active protein synthesis and monitor changes in response to stimuli.28 This strategy has been applied to macrophage models including THP1 and RAW264.7.22,23 The approach has also shown success measuring the response of resting primary T cells to activation.30 However, to date a BONCAT approach has not been applied to primary human innate immune cells.
Given our interest in applying these technologies to understand PrCR, we set out to characterize proteins detectable in primary monocyte-derived human macrophages under conditions that promote macrophage phagocytosis. Our goal was to establish a workflow for generating proteomic datasets from primary human cells in order to provide a foundational, hypothesis-generating understanding of protein abundance in phagocytic primary human monocyte-derived macrophages. Our approach was two-fold: (1) to characterize the whole proteome of macrophages when phagocytosis is increased. (2) To characterize proteins synthesized following stimulation to increase phagocytosis. We generated highly pure human monocyte derived macrophages with minimal perturbation beyond cytokine stimulation (at least 98% purity across 3 donors, Fig. S1, ESI†). Approximately 6000 proteins were detectable in both conditions, a 3-fold improvement in coverage when employing our statistical cut-offs. Importantly this dataset is collectively less resource intensive – requiring just 90 min of MS acquisition time (vs. 18 h), no fractionation, minimal sample input (200 ng), and no sample pooling (previously 20 donors), allowing for true biological replicates.
Results and discussion
To profile proteomic changes that occur during pro-phagocytic conditions, we first set out to identify a stimulating agent capable of increasing phagocytosis. We selected interferon-gamma (IF-g), a known activator of macrophages that induces expression of MHC complexes.24,31 To confirm that IF-g leads to increased macrophage phagocytosis, we employed an established flow cytometry based co-culture phagocytosis assay.5 Human monocyte-derived macrophages were exposed to IF-g (100 U mL−1, 5 ng mL−1, 72 h) or PBS in IMDM prior to co-incubation with fluorescently labeled colorectal cancer cells (2 h, SW620, Fig. 1(A)). Macrophage phagocytosis was measured via flow cytometry, yielding two populations of cells: macrophages which did not phagocytose cancer cells (CD11b+, GFP−, Non-Eaters) and macrophages which phagocytosed cancer cells (CD11b+, GFP+, Eaters) (Fig. 1(A) and Fig. S1 and S2, ESI†). We observed a 2.41 ± 0.62-fold increase in phagocytosis upon stimulation across replicates (Fig. 1(B) and Fig. S2, ESI†). This trend was consistent across two separate human donors, demonstrating IF-g treatment consistently increases phagocytosis (Fig. S2, ESI†).
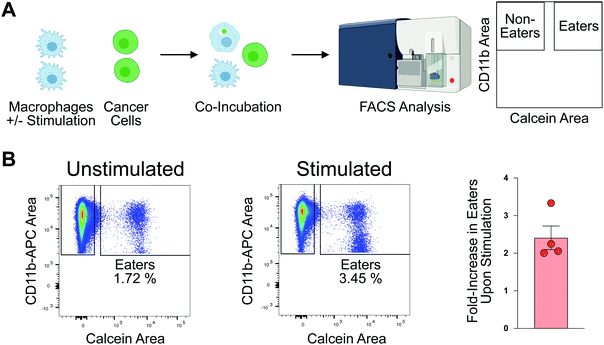 |
| Fig. 1 Macrophage phagocytosis increases with interferon-gamma (IF-g) treatment. (A) Donor-derived macrophages are stimulated with IF-g (72 h, R&D Systems) or PBS control prior to co-incubation with calcein stained SW620s (2 h). Cell populations are analyzed via flow cytometry for CD11b and calcein intensity. (B) Stimulated macrophages show a 2.41 ± 0.62-fold increase in Eaters upon IF-g treatment across 4 replicates. | |
Having confirmed that IF-g stimulation primes macrophages for phagocytosis, we focused our efforts on characterizing proteomic changes induced by cytokine treatment. Macrophages derived from three human donors were subjected to IF-g or PBS treatment (100 U mL−1, 5 ng mL−1, 72 h) prior to MS sample preparation. For each sample, 200 ng of purified peptide was subjected to MS analysis in technical duplicate (Fig. 2(A)). Data was filtered to include only proteins with a −10
log(p-value) of at least 20 and 1% peptide and protein FDRs (Fig. S3, ESI†). Individual donors showed comparable IDs, with an average ratio of 0.974 ± 0.025 between IF-g (+Stim) and PBS control conditions (−Stim, Fig. 2(A) and Table S1, ESI†). These findings indicate that any changes in the macrophage proteome during activation, and therefore increased phagocytic capacity, are not the result of significant changes to protein diversity. To further analyze these data, we summed spectral counts for each protein across the 3 biological replicates analyzed in technical duplicates (6 samples total) for each condition. We further ensured proteins were of high confidence by requiring at least 10 spectral counts be detected across the 6 replicates (Fig. S3A and Table S2, ESI†). This combined list yielded a total of 6098 proteins found in −Stim and 5922 in +Stim across the 3 donors (Table S2, ESI†). These results recapitulate our findings for each individual donor, where we found comparable protein IDs across +Stim and −Stim conditions (Fig. 2(B)). To identify proteins differentially expressed between conditions, we generated a ratio of +Stim/−Stim spectral counts and defined proteins which had a ratio of 3 or more as “Up in +Stim” and those with a ratio less than or equal to 0.33 to be “Up in −Stim” (Fig. S3A and Tables S3 and S4, ESI†). With these criteria, 276 proteins were found in “Up in −Stim” and 240 were found in “Up in +Stim” (Fig. 2(B) and Fig. S3B, Tables S3 and S4, ESI†). These findings align with an overall trend showing minimal diversity changes between treatment conditions, leading us to explore specific protein differences.
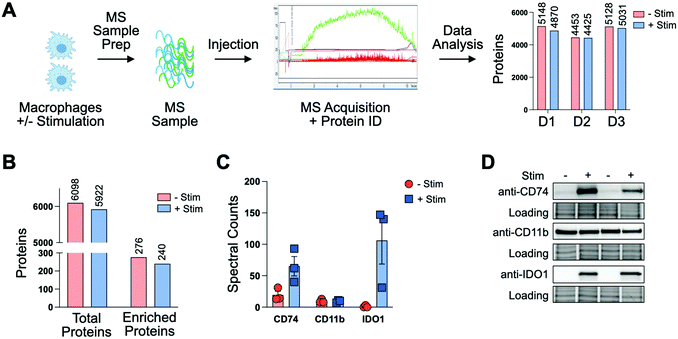 |
| Fig. 2 Pro-phagocytic stimulation does not influence protein diversity but alters abundance of specific proteins. (A) For 3 biological replicates, donor-derived macrophages are stimulated with IF-g (+Stim) or PBS (−Stim) prior to lysis, digestion, and MS acquisition. (B) Summed spectral counts across 3 donors show comparable spectral counts between −Stim and +Stim. Differentially detected protein IDs follow a similar trend. (C). Spectral counts for CD11b, CD74 and IDO1 are consistent across donors and are altered in response to pro-phagocytic stimulation. (D). Ratios observed by MS are recapitulated via western blot. | |
To further confirm the quality of our dataset, we referenced our data for proteins known to be associated with macrophages. The canonical macrophage marker CD11b was found to have a median ratio of 0.77 ± 0.23 between treatment conditions, suggesting IF-g treatment does not alter levels of the known surface marker (Fig. 2(C) and Table S1, ESI†). In our “Up in +Stim” list we identified CD74, also known as HLA class II histocompatibility antigen gamma chain (Fig. 2(C) and Table S3, ESI†). CD74 is important for antigen processing in MHC Class II and serves as a receptor for the macrophage migration inhibitory factor. The protein also prevents premature peptide binding and facilitates transport to endosomes for further MHC processing.32 IF-g is known to modify MHC complex expression. More specifically, it has been shown that IF-g treatment enhances CD74 expression, although its contribution to macrophage function is context specific.33,34 CD74 was detected across all 3 donors and shown to be present in both conditions, though it was more abundant with IF-g stimulation (Fig. 2(C) and Table S2, ESI†).
We next asked which proteins were most differentially expressed in our +Stim condition and identified Indoleamine 2,3-dioxygenase 1 (IDO1, Fig. 2(C) and Table S2, ESI†). IDO1 has been demonstrated to have immunosuppressive capabilities that can prevent continued inflammation response and can increase phagocytic capability in RAW264.7, a cell line model of mouse macrophages.35 However, its role in human macrophage activation has not been explored. In our dataset, not only was IDO1 the most differentially expressed, but it was also exclusively detected in +Stim samples (Fig. 2(C) and Fig. S3, ESI†).
To verify the relative values determined through our unbiased shotgun MS analysis, we went on to perform antibody-based experiments. Macrophages from two donors were subjected to IF-g or vehicle treatment prior to lysis, SDS-PAGE, and Western blotting. Importantly, CD11b showed comparable levels across both conditions for both donors (Fig. 2(D) and Fig. S4B, ESI†). Consistent with MS data, CD74 was detectable in lysate from both conditions, but much greater in +Stim (Fig. 2(D) and Fig. S4A, ESI†). Excitingly, blots for IDO1 also recapitulated our MS results with exclusive detection in +Stim macrophages (Fig. 2(D) and Fig. S4C, ESI†).
Moving forward with confidence in our strategy, we looked more-broadly at proteins found in “Up in +Stim” (240 proteins) and “Up in −Stim” (276 proteins) data sets (Tables S3 and S4, ESI†). To prevent biasing our analyses we employed a reference list of all proteins observed during whole protein MS analysis of our primary human monocyte-derived macrophages rather than the complete human proteome. Statistical overrepresentation analysis of biological processes in our “Up in +Stim” dataset revealed up to 33-fold enrichment in processes associated with phagocytosis, peptide antigen assembly, and multiple cell immunity pathways (Fig. 3 and Table S5, ESI†).36,37 Identical overrepresentation analysis of cell components revealed significant remodeling of the cell surface, with modifications occurring on IgG complexes, MHCs, and plasma membrane associated proteins (Table S5, ESI†). Surface remodeling of MHC expression reflects previous literature reporting on the effects of IF-g treatment.31 Enrichment in macrophage surface modifications coincided with a statistically significant underrepresentation of cytoplasm and intracellular organelles (0.79 and 0.77-fold enrichment, respectively), further suggesting a bias toward surface reengineering upon activation (Table S5, ESI†). We performed the same analyses on the 276 proteins found in the “Up in −Stim” dataset but found no statistically significant results across all annotation sets. Altogether, these high-level analyses revealed +Stim macrophages bias protein production toward a common defined set of pathways leading to a greater capacity for phagocytosis.
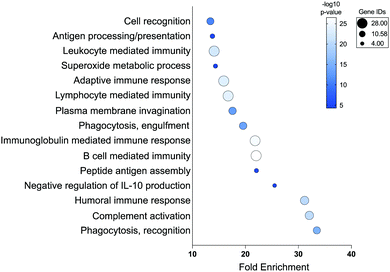 |
| Fig. 3 Statistical overrepresentation analysis of biological processes in “Up in +Stim” Dataset. Statistical analysis via Fisher's exact test and correction via False Discovery Rate. | |
In addition to understanding proteins detectable in human macrophages at steady-state and upon pro-phagocytic activation, we were interested in understanding differences in protein synthesis under conditions relevant to phagocytosis in a defined amount of time. To this end, we employed BONCAT with the methionine mimic HPG28 (Fig. 4(A)). To the best of our knowledge, this is the first report of HPG labeling in human terminally differentiated primary innate immune cells. To compare HPG incorporation in monocyte-derived primary human macrophages to an immortalized cancer cell line, macrophages or SW620 cells were treated with HPG or methionine control (4 mM, 2 h). Treated cells were washed, lysed, and subjected to CuAAC, or click-chemistry, with TAMRA-azide. In-gel fluorescence scanning revealed HPG incorporation that is nearly undetectable in macrophages compared to SW620 labeling (Fig. S5, ESI†). To investigate if HPG incorporation was occurring in macrophages, we cultured macrophages which had been treated with IF-g or PBS (100 U mL−1, 5 ng mL−1, 72 h) in methionine-free media supplemented with methionine or HPG (4 mM, 2 h). Following treatment, cells were lysed and subjected to treatment with TAMRA-azide under CuAAC conditions. In-gel fluorescence revealed incorporation of HPG in both +Stim and −Stim macrophages (Fig. 4(B)). Unique bands were readily detectable in +Stim macrophages. Representative examples are denoted by asterisks (*, Fig. 4(B)). Taken together, these results revealed that, while monocyte-derived macrophages are actively synthesizing proteins, they are doing so at a much slower rate than an immortalized cancer cell line.
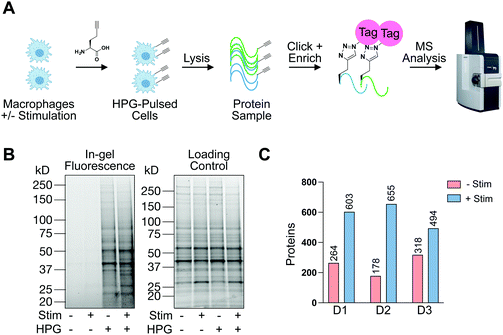 |
| Fig. 4 Identification of actively synthesized proteins in resting and stimulated macrophages. (A) Chemical proteomic approach to identify proteins synthesized upon IF-g stimulation. Newly synthesized proteins are tagged with homopropargylglycine (HPG) and biotinylated via CuAAC click reaction with biotin-azide. Biotinylated proteins are enriched, digested, and identified by MS. (B) HPG incorporation validated via click chemistry with rhodamine-azide and proteins resolved by SDS-PAGE. (C) Proteins which are “Made −Stim” and “Made in +Stim” macrophages across three biological replicates as identified by MS analysis. *− denotes representative bands differentially detected between −Stim and +Stim conditions. | |
Knowing that HPG incorporation was much lower than traditional cell culture models, we optimized our protocols to improve sample recovery and employed state-of-the-art MS instrumentation to maximize protein detection and coverage. +Stim or −Stim macrophages (IF-g, 100 U mL−1, 5 ng mL−1, 72 h) were treated with HPG or methionine control (4 mM, 2 h). Proteins synthesized during incubation were biotinylated under CuAAC conditions and purified by streptavidin enrichment prior to MS analysis (Fig. 4(A) and Table S6, ESI†).
MS experiments were performed on 3 donors in technical duplicate. Ratios were generated from spectral counts detected in HPG-enriched samples compared to those of methionine control samples. At least 5 spectral counts from the HPG-enriched sample and a ratio of at least 3 above methionine control was required for a protein to be considered as detectable (Fig. S6, ESI†). Across all 3 donors, +Stim macrophages had on average 2.5-fold more proteins detected than −Stim macrophages (Fig. 4(C) and Table S6, ESI†).
To further analyze this first-in-class dataset, we combined data from all 3 donors and their technical replicates, for a total of 6 replicates per condition (Fig. S6A and Table S7, ESI†). For each replicate we required our standard database search parameters and then summed the total number of spectral counts for each condition (Fig. S6A and Table S7, ESI†). Sum ratios were generated for HPG-treated vs. methionine-treated. For each protein we required at least 2 unique peptides and 10 spectral counts across all replicates (Fig. S6A, ESI†). Proteins that were at least 3-fold more detected in HPG-treated samples compared to methionine-treated samples were considered to be “Made in −Stim” and/or “Made in +Stim” (Fig. S6A and Tables S8, S9 ESI†). With these requirements we identified 242 proteins as “Made in −Stim” and 573 proteins as “Made in +Stim” (Fig. 5(A) and Fig. S6B and SC, Tables S8 and S9, ESI†). These results recapitulated our findings across individual replicates with a 2.4-fold increase in proteins detected in +Stim macrophages.
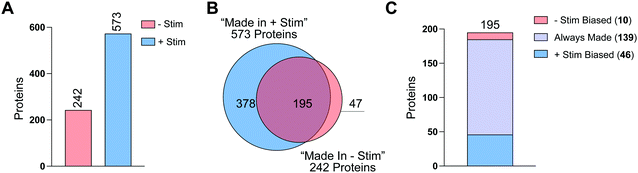 |
| Fig. 5 +Stim macrophages synthesize more proteins than −Stim macrophages. (A) Number of proteins detected in +Stim and −Stim HPG experiments across 3 biological replicates. Proteins were required to be at least 3-fold more enriched with HPG treatment compared to methionine treatment. (B) There are 378 unique proteins in “Made in +Stim”, while just 47 unique proteins are found in “Made in −Stim”. +Stim and −Stim macrophages synthesize 195 common proteins. (C) From the 195 shared proteins, 10 are more frequently in “Made in −Stim” (−Stim biased) and 46 are more frequently in “Made in +Stim” (+Stim biased). To be considered biased, protein had to be detected at least 3-fold more often in +Stim or −Stim. | |
A total of 195 proteins were found in both “Made in −Stim” and “Made in +Stim” macrophages (Fig. 5(B) and Table S10, ESI†). However, this analysis did not delineate whether these 195 targets were more frequently synthesized in one condition or another. Therefore, we further evaluated these targets to determine if they were more abundant in −Stim or +Stim macrophages. We required a 3-fold increase in detection between conditions for a protein to be considered a +Stim-biased (ratio ≥ 3) or −Stim-biased protein (ratio ≤ 0.33) (Table S11, ESI†). Of the 195 proteins detected in both datasets, 139 were always made, 10 were −Stim-biased and 36 were +Stim-biased (Fig. 5(C) and Table S11, ESI†). Taken together these results suggest that +Stim macrophages synthesize more protein than −Stim macrophages.
Having characterized our dataset through high-level analyses, we wanted to determine if specific proteins found to be sensitive to IF-g treatment could be corroborated by previous literature reports. Proteins that were uniquely “Made in +Stim” including, Complement C2 (CO2), C1 inhibitor (IC1) and Caspase-7 (CASP7), among others, have been previously reported to be upregulated in response to IF-g (Table S11, ESI†).39–41 Conversely, IF-g has been shown to downregulate mTOR in macrophages, and this finding is recapitulated in our BONCAT studies (Table S11, ESI†).42
Finally, we cross-referenced our +Stim/−Stim and “Made in +Stim”/”Made in −Stim” datasets, focusing on proteins that were unique to either “Made in +Stim” or “Made in −Stim”. A majority of proteins detected in these data were previously identified in the whole protein analysis (348 of 378 proteins under +Stim conditions and 41 of 47 proteins under −Stim conditions). However, we were intrigued to see that very few proteins that were detectable as both +Stim and “Made In +Stim Only” (17 proteins of 348) or −Stim and “Made in −Stim Only” (2 proteins of 41, Fig. S7A, ESI†). Future investigations involve exploring the possibility of differential protein half-lives in response to macrophage stimulation, which would potentially require increased rates of synthesis to maintain comparable protein levels. These analyses highlight the value of performing both whole-protein mass spectrometry experiments and pulse-type chemical proteomics BONCAT studies over a defined time window. Gratifyingly, statistical over-representation analysis of biological processes in the 17 proteins overlapping as both +Stim and”Made In +Stim Only” revealed enrichment of processes associated with innate immune response (Fig. S7B and Table S12, ESI†).
Conclusions
In this manuscript we have established methods for characterization of the whole proteome of primary human monocyte-derived macrophages and characterized changes to protein synthesis in response to pro-phagocytic stimulation. The established workflow can be applied to other low-yield primary cell types and provides a first-in-class dataset for these cells. This depth of coverage was previously unattainable due to technological limitations. Our findings provide a wealth of hypothesis-generating data and shed light on how macrophages modify their protein repertoire to prepare for PrCR. Identification of previously noted but unexplored targets, such as IDO1, can guide further analysis into protein-level control of phagocytosis. As an immunosuppressive protein, this finding could be part of a delicate balance within macrophages between pro-inflammation and PrCR and remediation of inflammation to prevent nonspecific tissue damage. These two pathways must also coincide with the role of macrophages in antigen processing/presentation to promote the adaptive immune response.
We were somewhat surprised to find that active protein synthesis in macrophages is relatively low, particularly in comparison to immortalized cell lines. Excitingly, due to low incorporation, this workflow will be applicable in co-culture scenarios with cancer cells where a vast majority of HPG labeling will come from the cancer cells. In future work, we aim to profile active synthesis in cancer cells using HPG with and without macrophage co-culture. The resulting characterization of cancer cell protein synthesis could identify novel regulatory proteins and processes on the target cell side of PrCR, thereby providing a deeper understanding of how these cells can efficiently avoid recognition by macrophages. In the future, the workflow could also be used to profile macrophage response to other stimuli as well as other phagocytes known to facilitate PrCR. Due to non-specific targeting by the innate immune system, we anticipate inflammatory stimuli from mammalian or pathogenic origins may result in a similar proteomic signature. This may contrast with anti-inflammatory stimuli which could downregulate proteins identified in this report. In future work we plan to explore a diverse panel of stimuli to identify proteins which may be attractive broad-spectrum therapeutic targets. Additionally, protein expression signatures for a particular macrophage phenotype (for example, pro- or anti-inflammatory) could also serve an experimental readout in future phenotypic screens for innate immunomodulatory molecules.
Experimental
Macrophage generation and cytokine treatment
Primary human donor-derived macrophages generation and cytokine treatment were performed as described previously by Barkal et al. 2019.5 For interferon-gamma, 100 U mL−1 (5 ng mL−1) was selected (R&D Systems), for a total of 50 ng per plate of monocytes, as utilized by Wallet et al.38 In brief, 100 U mL−1 of interferon-gamma was supplemented into macrophage differentiation media (IMDM, GlutaMAX™ Supplement (ThermoFisher), 10% Human Serum AB (Gemini Bio Products)) on day 5 and allowed to incubate at 37 °C for 72 h.
Flow cytometry-based phagocytosis assay
Evaluation of percent phagocytosis of calcein stained SW620s by monocyte derived macrophages was done as described previously by Barkal et al. 2019.5 Macrophage co-incubation was performed with 50
000 macrophages and 100
000 or 500
000 cancer cells to evaluate the effect on percent eating via target cell abundance.
In situ labelling-homopropargylglycine treatment
Selective labeling of actively synthesized proteins was performed as described by Dieterich et al. 2006.28 In brief, cells were lifted from plates and washed of complete media with 3× PBS washes prior to resuspension in methionine or HPG supplemented methionine-free RPMI (Thermo Scientific). Cells were then introduced to 96-well clear round bottom ultra-low attachment microplates (Corning) at 50
000 cells per 100 μL and incubated 2 h at 37 °C. Cells were then washed 2× with PBS to remove excess methionine or HPG before pellets were snap frozen and stored at −80 °C.
Biotin enrichment
Pelleted cells were resuspended in 10 μL of Lysis Buffer 1 (LB1, 0.05% SDS, 10 mM TEA pH 7.4, 150 mM NaCl, and 10 mg/100 μL) protease inhibitor cocktail (Sigma Aldrich). The samples were supplemented with 1 μL benzonase (250 U μL−1, Millipore Sigma) and incubated on ice for 30 minutes. 90 μL of Lysis Buffer 2 (LB2, 4% SDS, 50 mM TEA pH 7.4, 150 mM NaCl) was added and the sample was mixed by pipetting and left at RT for 5 min. This lysis buffer solubilized both cytosolic and membrane proteins. Samples were then centrifuged for 10 min, 10
000 × g, 15 °C. BCA assay (Pierce™) was used to determine protein concentration. 30 μg of sample was reserved for in-gel fluorescence analysis (see below) and the remainder was normalized across each donor and diluted to 1 μg μL−1 before biotin click-chemistry was performed. In brief, samples were incubated at RT for 75 min with Biotin Azide (0.1 mM, Click-chemistry tools), TCEP (1 mM, freshly prepared, EMD Millipore), TBTA (0.102 mM, dissolved in 4
:
1 t-butanol
:
DMSO, TCI America), and CuSO4 (1 mM, Sigma Aldrich). Samples were precipitated using chloroform-methanol extraction: 2× cold MeOH, 0.5 × CHCl3, 1 × H20. The samples were then spun at max speed for 5 minutes yielding a protein disk between phases. Excess reagents were removed by decanting and sample was resuspended in 5 mL MeOH and stored in −20 °C overnight.
Precipitated protein disks were spun at max speed, 5 min, and methanol was removed prior to allowing samples to air dry inverted for 15 min. Proteins were resuspended in urea buffer (6 mM in PBS, Omnipur) and sonicated to resuspend prior to addition of 10%SDS in PBS (5 μL, Fisher Scientific). DTT was added (10 mM, Fisher Scientific) and samples were incubated at 65 °C for 15 min, shaking 500 rpm. Next, iodoacetamide was added (56 mM, Acros) and samples were shaken for 30 min at 37 °C. Samples were supplemented with 65 μL 10%SDS in PBS to ensure everything was solubilized and then diluted with 3 mL of PBS. Next, 50 μL/500 μg protein of Streptavidin-agarose (Thermo Scientific) was added and samples were rotated for 1.5 h at RT. Beads were pelleted by centrifugation (1600 × g, 2 min) and supernatant aspirated. Beads were then washed 1× each with 5 mLs of 0.2%SDS in PBS, 1× PBS, and MilliQ water. Using 2× 500 μL aliquots of MilliQ, beads were transferred to DNA lo-bind Eppendorfs. MilliQ water was removed following centrifugation and beads were resuspended in 50 mM Ammonium Bicarbonate (Alfa Aesar) supplemented with 1 μg Trypsin/500 μg (Promega) protein and incubated at 37 °C for 16 h, shaking. Beads were pelleted by centrifugation and supernatant collected. Beads were then rinsed 2× with 200 μL 50 mM ABC. Collected peptides were cleaned up using C18 columns (Fisher Scientific) and manufacturer protocol. Final resuspension occurred in PreOmics Loading Buffer.
In-gel fluorescence
Reserved protein from lysis (30 μg, see biotin enrichment) was normalized to 1 μg μL−1 in lo-bind Eppendorf. A click chemistry master mix (MM) was prepared 0.6 μL Rhodamine-azide (1.25 mM stock), 0.6 μL TCEP (50 mM, stock made fresh in water), 1.8 μL TBTA (1.7 mM, 4
:
1 t-butanol
:
DMSO), and 0.6 μL CuSO4 (50 mM stock) per sample. 3.6 μL of MM was added to each sample prior to 1 h. incubation at RT in the dark. Samples were precipitated with 5× volume ice-cold methanol and stored in −20 °C overnight to remove excess click reagents. The next day, samples were spun max speed and methanol aspirated away before allowing them to dry inverted for 2 h. Protein pellets were solubilized with 30 μL of 4%SDS in PBS and 10 min of bath sonication. 20 μL of 4× LB + BME was added, and samples were boiled 5 min at 95 °C. For gel analysis, 25 μL of sample was loaded to SDS-PAGE gel and resolved over 1 h at 150 V before imaging in cy3/cy5 on Chemidoc (Biorad).
Whole protein macrophage analysis
Lifted macrophages were washed 3× with dPBS prior to pelleting and snap freeze. Stored pellets were then allowed to thaw on ice before preparation with PreOmics iST96 kit with 10 mg/100 μL protease inhibitor cocktail (Sigma Aldrich). PreOmics iST lysis buffer in combination with boiling solubilizes membrane and soluble proteins.
Western blot validation
Lifted macrophages were washed 3× with dPBS prior to pelleting and snap freeze. Stored pellets were allowed to thaw on ice prior to lysis with PreOmics iST96 lysis buffer with 10 mg/100 μL protease inhibitor cocktail (Sigma Aldrich). Lysed samples were heated at 95 °C for 10 min following PreOmics protocol prior to precipitation in 5× volume of acetone. Samples were resuspended in 4%SDS in PBS and sonicated for 10 min. BCA assay was used to determine protein concentration. Sample concentration was normalized and 4× loading buffer plus BME was added to 1× concentration before heat denature at 95 °C for 5 min. 25 μL of sample was loaded onto TGX Stain-free 4–15% gels (Biorad) and run at 195 V for 45 min. Resulting gels were imaged stain free before transferring to PVDF membrane. Blots were blocked in 5% milk in TBST for 45 min, rinsed 3× with 1× TBST, and incubated overnight at 4 °C with 1
:
1000 CD74 (cell signaling, #77274), CD11b (cell signaling, #49420), or IDO1 (cell signaling, #12006) in 5% BSA supplemented with 0.05% sodium azide (TGI). Blots were rinsed 3× with 1× TBST and incubated with anti-rabbit IgG, HRP-linked secondary (Cell Signaling, #7074) for 1 h at RT. Finally, blots were washed again 3× with 1× TBST and equal amounts of Radiance ECL substrate and peroxide (Azure Biosystems) were added, and blot was allowed to rock for 5 min prior to chemiluminescent imaging on Chemidoc (Biorad).
Mass spectrometry analysis
A nanoElute was attached in line to a timsTOF Pro equipped with a CaptiveSpray Source (Bruker, Hamburg, Germany). Chromatography was conducted at 40 °C through a 25 cm reversed-phase C18 column (PepSep) at a constant flowrate of 0.5 μL min−1. Mobile phase A was 98/2/0.1% water/MeCN/formic acid (v/v/v) and phase B was MeCN with 0.1% formic acid (v/v). During a 108 min method, peptides were separated by a 3-step linear gradient (5% to 30% B over 90 min, 30% to 35% B over 10 min, 35% to 95% B over 4 min) followed by a 4 min isocratic flush at 95% for 4 min before washing and a return to low organic conditions. Experiments were run as data-dependent acquisitions with ion mobility activated in PASEF mode. MS and MS/MS spectra were collected with m/z 100 to 1700 and ions with z = +1 were excluded.
Raw data files were searched using PEAKS Online Xpro 1.6 (Bioinformatics Solutions Inc., Waterloo, Ontario, Canada). The precursor mass error tolerance and fragment mass error tolerance were set to 20 ppm and 0.03 respectively. The trypsin digest mode was set to semi-specific and missed cleavages was set to 2. The human Swiss-Prot reviewed (canonical) database (downloaded from UniProt) and the common repository of adventitious proteins (cRAP, downloaded from The Global Proteome Machine Organization) totaling 20,487 entries were used. Carbamidomethylation was selected as a fixed modification. Oxidation (M) was selected as a variable modification.
Whole protein experiments were performed in biological triplicate, with samples being run in duplicate on the instrument. Resulting combined datasets were subjected to the following filtration criteria:
(1) Database search (−10
log(p-value) ≥ 20, 1% peptide and protein FDR).
(2) Sum of spectral counts across 3 biological replicates in technical duplicate (6 samples total) for each condition.
(3) Generate ratio of +Stim/−Stim.
(4) Proteins differentially detected were 3-fold more detected in +Stim (ratio of ≥3) or −Stim (ratio ≤ 0.33) – Fig. S2B, ESI† generated.
(5) Proteins of interest required to have at ≥10 spectral counts.
HPG experiments were performed in biological triplicate, with samples being run in duplicate on the instrument. Resulting combined datasets were subjected to the following filtration criteria:
(1) Database search (−10
log(p-value) ≥ 20, 1% peptide and protein FDR).
(2) Sum of spectral counts across 3 biological replicates in technical duplicate (6 samples total) for each condition.
(3) Generate ratio of HPG-treated/methionine-treated. Require ≥2 unique peptides and ≥10 spectral counts with HPG treatment.
(4) Proteins differentially detected were 3-fold more detected in HPG-treated sample compared to methionine-treated (ratio of ≥3).
Raw data files and searched datasets are available on the Mass Spectrometry Interactive Virtual Environment (MassIVE), a full member of the Proteome Xchange consortium under the identifier: MSV000089027. The complete searched datasets are also available in our ESI.†
Author contributions
R. F. V. designed and performed experiments. B. W. Z. conceived of the project and designed experiments. B. W. Z. and R. F. V. wrote the manuscript. J. L. M., S. E. W., and K. L. M. provided feedback on experimental design. J. L. M. and S. E. W. contributed to manuscript revisions.
Conflicts of interest
There are no conflicts to declare.
Acknowledgements
This work was supported by the Arnold and Mabel Beckman Foundation's Young Investigator Award and the University of California San Francisco. We thank R. E. Brewer for advice on macrophage generation and M. Krawitzsky on his continued instrumentation support. Cell images in the manuscript were generated on Biorender.com.
Notes and references
-
A. Kelly, A. M. Grabiec and M. A. Travis, Culture of Human Monocyte-Derived Macrophages, Methods in Molecular Biology, 2018, vol. 1784, pp. 1–11 DOI:10.1007/978-1-4939-7837-3_1.
- E. Uribe-Querol and C. Rosales, Phagocytosis: Our current understanding of a universal biological process, Front. Immunol., 2020, 11, 1066, DOI:10.3389/fimmu.2020.01066.
- M. Feng, W. Jiang, B. Y.-S. Kim, C. C. Zhang, Y.-X. Fu and I. L. Weissman, Phagocytosis checkpoints as new targets for cancer immunotherapy, Nat. Rev. Cancer, 2019, 19(10), 568–586, DOI:10.1038/s41568-019-0183-z.
- S. Jaiswal, C. H.-M. Jamieson, W. W. Pang, C. Y. Park, M. P. Chao, R. Majeti, D. Traver, N. van Rooijen and I. L. Weissman, CD47 is upregulated on circulating hematopoietic stem cells and leukemia cells to avoid phagocytosis, Cell, 2009, 138(2), 271–285, DOI:10.1016/j.cell.2009.05.046.
- A. A. Barkal, R. E. Brewer, M. Markovic, M. Kowarsky, S. A. Barkal, B. W. Zaro, V. Krishnan, J. Hatakeyama, O. Dorigo, L. J. Barkal and I. L. Weissman, CD24 signalling through macrophage siglec-10 is a target for cancer immunotherapy., Nature, 2019, 572(7769), 392–396, DOI:10.1038/s41586-019-1456-0.
- S. R. Gordon, R. L. Maute, B. W. Dulken, G. Hutter, B. M. George, M. N. McCracken, R. Gupta, J. M. Tsai, R. Sinha, D. Corey, A. M. Ring, A. J. Connolly and I. L. Weissman, PD-1 expression by tumour-associated macrophages inhibits phagocytosis and tumour immunity, Nature, 2017, 545(7655), 495–499, DOI:10.1038/nature22396.
- A. A. Barkal, K. Weiskopf, K. S. Kao, S. R. Gordon, B. Rosental, Y. Y. Yiu, B. M. George, M. Markovic, N. G. Ring, J. M. Tsai, K. M. McKenna, P. Y. Ho, R. Z. Cheng, J. Y. Chen, L. J. Barkal, A. M. Ring, I. L. Weissman and R. L. Maute, Engagement of MHC Class I by the inhibitory receptor LILRB1 suppresses macrophages and is a target of cancer immunotherapy., Nat. Immunol., 2018, 19(1), 76–84, DOI:10.1038/s41590-017-0004-z.
- M. P. Chao, S. Jaiswal, R. Weissman-Tsukamoto, A. A. Alizadeh, A. J. Gentles, J. Volkmer, K. Weiskopf, S. B. Willingham, T. Raveh, C. Y. Park, R. Majeti and I. L. Weissman, Calreticulin is the dominant pro-phagocytic signal on multiple human cancers and is counterbalanced by CD47., Sci. Transl. Med., 2010, 2(63), 63ra94, DOI:10.1126/scitranslmed.3001375.
- J. Chen, M.-C. Zhong, H. Guo, D. Davidson, S. Mishel, Y. Lu, I. Rhee, L.-A. Pérez-Quintero, S. Zhang, M.-E. Cruz-Munoz, N. Wu, D. C. Vinh, M. Sinha, V. Calderon, C. A. Lowell, J. S. Danska and A. Veillette, SLAMF7 is critical for phagocytosis of haematopoietic tumour cells via Mac-1 integrin., Nature, 2017, 544(7651), 493–497, DOI:10.1038/nature22076.
- A. Veillette and J. Chen, SIRPα-CD47 immune checkpoint blockade in anticancer therapy., Trends Immunol., 2018, 39(3), 173–184, DOI:10.1016/j.it.2017.12.005.
- M. Feng, K. D. Marjon, F. Zhu, R. Weissman-Tsukamoto, A. Levett, K. Sullivan, K. S. Kao, M. Markovic, P. A. Bump, H. M. Jackson, T. S. Choi, J. Chen, A. M. Banuelos, J. Liu, P. Gip, L. Cheng, D. Wang and I. L. Weissman, Programmed cell removal by calreticulin in tissue homeostasis and cancer., Nat. Commun., 2018, 9(1), 3194, DOI:10.1038/s41467-018-05211-7.
- M. C. Tal, L. B. Torrez Dulgeroff, L. Myers, L. B. Cham, K. D. Mayer-Barber, A. C. Bohrer, E. Castro, Y. Y. Yiu, C. Lopez Angel, E. Pham, A. B. Carmody, R. J. Messer, E. Gars, J. Kortmann, M. Markovic, M. Hasenkrug, K. E. Peterson, C. W. Winkler, T. A. Woods, P. Hansen, S. Galloway, D. Wagh, B. J. Fram, T. Nguyen, D. Corey, R. S. Kalluru, N. Banaei, J. Rajadas, D. M. Monack, A. Ahmed, D. Sahoo, M. M. Davis, J. S. Glenn, T. Adomati, K. S. Lang, I. L. Weissman and K. J. Hasenkrug, Upregulation of CD47 is a host checkpoint response to pathogen recognition., mBio, 2020, 11(3), e01293–20, DOI:10.1128/mBio.01293-20.
- S. M. Gheibihayat, R. Cabezas, N. G. Nikiforov, T. Jamialahmadi, T. P. Johnston and A. Sahebkar, CD47 in the brain and neurodegeneration: An update on the role in neuroinflammatory pathways., Molecules, 2021, 26(13), 3943, DOI:10.3390/molecules26133943.
- Y. Kojima, J.-P. Volkmer, K. McKenna, M. Civelek, A. J. Lusis, C. L. Miller, D. Direnzo, V. Nanda, J. Ye, A. J. Connolly, E. E. Schadt, T. Quertermous, P. Betancur, L. Maegdefessel, L. P. Matic, U. Hedin, I. L. Weissman and N. J. Leeper, CD47-blocking antibodies restore phagocytosis and prevent atherosclerosis., Nature, 2016, 536(7614), 86–90, DOI:10.1038/nature18935.
- R. Maute, J. Xu and I. L. Weissman, CD47-SIRPα-targeted therapeutics: Status and prospects, Immuno-Oncol. Technol., 2022, 13, 100070, DOI:10.1016/j.iotech.2022.100070.
- R. Advani, I. Flinn, L. Popplewell, A. Forero, N. L. Bartlett, N. Ghosh, J. Kline, M. Roschewski, A. LaCasce, G. P. Collins, T. Tran, J. Lynn, J. Y. Chen, J.-P. Volkmer, B. Agoram, J. Huang, R. Majeti, I. L. Weissman, C. H. Takimoto, M. P. Chao and S. M. Smith, CD47 blockade by Hu5F9-G4 and rituximab in non-Hodgkin's lymphoma., N. Engl. J. Med., 2018, 379(18), 1711–1721, DOI:10.1056/NEJMoa1807315.
- P. S. Petrova, N. N. Viller, M. Wong, X. Pang, G. H.-Y. Lin, K. Dodge, V. Chai, H. Chen, V. Lee, V. House, N. T. Vigo, D. Jin, T. Mutukura, M. Charbonneau, T. Truong, S. Viau, L. D. Johnson, E. Linderoth, E. L. Sievers, S. Maleki Vareki, R. Figueredo, M. Pampillo, J. Koropatnick, S. Trudel, N. Mbong, L. Jin, J. C.-Y. Wang and R. A. Uger, TTI-621 (SIRPαFc): A CD47-blocking innate immune checkpoint inhibitor with broad antitumor activity and minimal erythrocyte binding, Clin. Cancer Res., 2017, 23(4), 1068–1079, DOI:10.1158/1078-0432.CCR-16-1700.
- S. Eligini, M. Brioschi, S. Fiorelli, E. Tremoli, C. Banfi and S. Colli, Human monocyte-derived macrophages are heterogenous: Proteomic profile of different phenotypes, J. Proteomics, 2015, 124, 112–123, DOI:10.1016/j.jprot.2015.03.026.
- Y. Zhang, Y. Fu, L. Jia, C. Zhang, W. Cao, N. Alam, R. Wang, W. Wang, L. Bai, S. Zhao and E. Liu, TMT-based quantitative proteomic profiling of human monocyte-derived macrophages and foam cells., Proteome Sci., 2022, 20(1), 1, DOI:10.1186/s12953-021-00183-x.
- M. Court, G. Petre, M. E. Atifi and A. Millet, Proteomic signature reveals modulation of human macrophage polarization and functions under differing environmental oxygen conditions., Mol. Cell. Proteomics, 2017, 16(12), 2153–2168, DOI:10.1074/mcp.RA117.000082.
- J. N. Brown, M. A. Wallet, B. Krastins, D. Sarracino and M. M. Goodenow, Proteome bioprofiles distinguish between m1 priming and activation states in human macrophages., J. Leukoc. Biol., 2010, 87(4), 655–662, DOI:10.1189/jlb.0809570.
- A. Kumar, S. Jamwal, M. K. Midha, B. Hamza, S. Aggarwal, A. K. Yadav and K. V.-S. Rao, Dataset generated using hyperplexing and click chemistry to monitor temporal dynamics of newly synthesized macrophage secretome post infection by mycobacterial strains., Data Brief, 2016, 9, 349–354, DOI:10.1016/j.dib.2016.08.055.
- J. Selkrig, N. Li, A. Hausmann, M. S.-J. Mangan, M. Zietek, A. Mateus, J. Bobonis, A. Sueki, H. Imamura, B. El Debs, G. Sigismondo, B. I. Florea, H. S. Overkleeft, N. Kopitar-Jerala, B. Turk, P. Beltrao, M. M. Savitski, E. Latz, W.-D. Hardt, J. Krijgsveld and A. Typas, Spatiotemporal proteomics uncovers cathepsin-dependent macrophage cell death during salmonella infection, Nat. Microbiol., 2020, 5(9), 1119–1133, DOI:10.1038/s41564-020-0736-7.
- V. Piccolo, A. Curina, M. Genua, S. Ghisletti, M. Simonatto, A. Sabò, B. Amati, R. Ostuni and G. Natoli, Opposing macrophage polarization programs show extensive epigenomic and transcriptional cross-talk., Nat. Immunol., 2017, 18(5), 530–540, DOI:10.1038/ni.3710.
- B. W. Zaro, J. J. Noh, V. L. Mascetti, J. Demeter, B. George, M. Zukowska, G. S. Gulati, R. Sinha, R. A. Flynn, A. Banuelos, A. Zhang, A. C. Wilkinson, P. Jackson and I. L. Weissman, Proteomic analysis of young and old mouse hematopoietic stem cells and their progenitors reveals post-transcriptional regulation in stem cells., eLife, 2020, 9, e62210, DOI:10.7554/eLife.62210.
- M. S. Haney, C. J. Bohlen, D. W. Morgens, J. A. Ousey, A. A. Barkal, C. K. Tsui, B. K. Ego, R. Levin, R. A. Kamber, H. Collins, A. Tucker, A. Li, D. Vorselen, L. Labitigan, E. Crane, E. Boyle, L. Jiang, J. Chan, E. Rincón, W. J. Greenleaf, B. Li, M. P. Snyder, I. L. Weissman, J. A. Theriot, S. R. Collins, B. A. Barres and M. C. Bassik, Identification of phagocytosis regulators using magnetic genome-wide CRISPR screens., Nat. Genet., 2018, 50(12), 1716–1727, DOI:10.1038/s41588-018-0254-1.
- F. Meier, A.-D. Brunner, M. Frank, A. Ha, I. Bludau, E. Voytik, S. Kaspar-Schoenefeld, M. Lubeck, O. Raether, N. Bache, R. Aebersold, B. C. Collins, H. L. Röst and M. Mann, DiaPASEF: Parallel accumulation–serial fragmentation combined with data-independent acquisition., Nat. Methods, 2020, 17(12), 1229–1236, DOI:10.1038/s41592-020-00998-0.
- D. C. Dieterich, A. J. Link, J. Graumann, D. A. Tirrell and E. M. Schuman, Selective identification of newly synthesized proteins in mammalian cells using bioorthogonal noncanonical amino acid tagging (BONCAT)., Proc. Natl. Acad. Sci. U. S. A., 2006, 103(25), 9482–9487, DOI:10.1073/pnas.0601637103.
- C. G. Parker and M. R. Pratt, Click chemistry in proteomic investigations., Cell, 2020, 180(4), 605–632, DOI:10.1016/j.cell.2020.01.025.
- A. J.-M. Howden, V. Geoghegan, K. Katsch, G. Efstathiou, B. Bhushan, O. Boutureira, B. Thomas, D. C. Trudgian, B. M. Kessler, D. C. Dieterich, B. G. Davis and O. Acuto, QuaNCAT: Quantitating proteome dynamics in primary cells., Nat. Methods, 2013, 10(4), 343–346, DOI:10.1038/nmeth.2401.
- E. Müller, P. F. Christopoulos, S. Halder, A. Lunde, K. Beraki, M. Speth, I. Øynebråten and A. Corthay, Toll-like receptor ligands and interferon-γ synergize for induction of antitumor M1 macrophages., Front. Immunol., 2017, 8, 1383, DOI:10.3389/fimmu.2017.01383.
- J. M. Riberdy, J. R. Newcomb, M. J. Surman, J. A. Barbosat and P. Cresswell, HLA-DR molecules from an antigen-processing mutant cell line are associated with invariant chain peptides., Nature, 1992, 360(6403), 474–477, DOI:10.1038/360474a0.
- Y. Fukuda, M. A. Bustos, S.-N. Cho, J. Roszik, S. Ryu, V. M. Lopez, J. K. Burks, J. E. Lee, E. A. Grimm, D. S.-B. Hoon and S. Ekmekcioglu, Interplay between soluble CD74 and macrophage-migration inhibitory factor drives tumor growth and influences patient survival in melanoma., Cell Death Dis., 2022, 13(2), 117, DOI:10.1038/s41419-022-04552-y.
- K. Tanese, Y. Hashimoto, Z. Berkova, Y. Wang, F. Samaniego, J. E. Lee, S. Ekmekcioglu and E. A. Grimm, Cell Surface CD74-MIF interactions drive melanoma survival in response to interferon-γ., J. Invest. Dermatol., 2015, 135(11), 2775–2784, DOI:10.1038/jid.2015.204.
- R. Ji, L. Ma, X. Chen, R. Sun, L. Zhang, H. Saiyin and W. Wei, Characterizing the distributions of IDO-1 expressing macrophages/microglia in human and murine brains and evaluating the immunological and physiological roles of IDO-1 in RAW264.7/BV-2 cells., PLoS One, 2021, 16(11), e0258204, DOI:10.1371/journal.pone.0258204.
- P. D. Thomas, A. Kejariwal, N. Guo, H. Mi, M. J. Campbell, A. Muruganujan and B. Lazareva-Ulitsky, Applications for protein sequence-function evolution data: MRNA/protein expression analysis and coding SNP scoring tools., Nucleic Acids Res., 2006, 34(Web Server), W645–W650, DOI:10.1093/nar/gkl229.
- P. D. Thomas, M. J. Campbell, A. Kejariwal, H. Mi, B. Karlak, R. Daverman, K. Diemer, A. Muruganujan and A. Narechania, PANTHER: A library of protein families and subfamilies indexed by function., Genome Res., 2003, 13(9), 2129–2141, DOI:10.1101/gr.772403.
- P. Wallet, S. Benaoudia, A. Mosnier, B. Lagrange, A. Martin, H. Lindgren, I. Golovliov, F. Michal, P. Basso, S. Djebali, A. Provost, O. Allatif, E. Meunier, P. Broz, M. Yamamoto, B. F. Py, E. Faudry, A. Sjöstedt and T. Henry, IFN-γ extends the immune functions of guanylate binding proteins to inflammasome-independent antibacterial activities during Francisella novicida infection., PLoS Pathog., 2017, 13(10), e1006630, DOI:10.1371/journal.ppat.1006630.
- R. C. Strunk, F. S. Cole, D. H. Perlmutter and H. R. Colten, Gamma-Interferon increases expression of class III complement genes C2 and factor B in human monocytes and in murine fibroblasts transfected with human C2 and factor B genes, J. Biol. Chem., 1985, 260, 15280–15285, DOI:10.1016/S0021-9258(18)95732-7.
- G. Heda, S. Mardente, L. Weiner and A. Schmaier, Interferon gamma increases in vitro and in vivo expression of C1 inhibitor., Blood, 1990, 75, 2401–2407, DOI:10.1182/blood.V75.12.2401.2401.
- M. A. Ellison, C. M. Gearheart, C. C. Porter and D. R. Ambruso, IFN-γ alters the expression of diverse immunity related genes in a cell culture model designed to represent maturing neutrophils, PLoS One, 2017, 12, e0185956, DOI:10.1371/journal.pone.0185956.
- X. Su, Y. Yu, Y. Zhong, E. G. Giannopoulou, X. Hu, H. Liu, J. R. Cross, G. Rätsch, C. M. Rice and L. B. Ivashkiv, Interferon-γ regulates cellular metabolism and mRNA translation to potentiate macrophage activation, Nat. Immunol., 2015, 16, 838–849, DOI:10.1038/ni.3205.
|
This journal is © The Royal Society of Chemistry 2022 |
Click here to see how this site uses Cookies. View our privacy policy here.