DOI:
10.1039/D2CB00096B
(Opinion)
RSC Chem. Biol., 2022,
3, 1301-1313
Alternative design strategies to help build the enzymatic retrosynthesis toolbox
Received
6th April 2022
, Accepted 11th September 2022
First published on 13th September 2022
Abstract
Most of the complex molecules found in nature still cannot be synthesized by current organic chemistry methods. Given the number of enzymes that exist in nature and the incredible potential of directed evolution, the field of synthetic biology contains perhaps all the necessary building blocks to bring about the realization of applied enzymatic retrosynthesis. Current thinking anticipates that enzymatic retrosynthesis will be implemented using conventional cell-based synthetic biology approaches where requisite native, heterologous, designer, and evolved enzymes making up a given multi-enzyme pathway are hosted by chassis organisms to carry out designer synthesis. In this perspective, we suggest that such an effort should not be limited by solely exploiting living cells and enzyme evolution and describe some useful yet less intensive complementary approaches that may prove especially productive in this grand scheme. By decoupling reactions from the environment of a living cell, a significantly larger portion of potential synthetic chemical space becomes available for exploration; most of this area is currently unavailable to cell-based approaches due to toxicity issues. In contrast, in a cell-free reaction a variety of classical enzymatic approaches can be exploited to improve performance and explore and understand a given enzyme's substrate specificity and catalytic profile towards non-natural substrates. We expect these studies will reveal unique enzymatic capabilities that are not accessible in living cells.
Introduction
The desire to both understand and control the synthesis of complex molecules began as early as 1828, when Friedrich Wöhler ushered in the era of modern organic chemistry via the first ever synthesis of urea.1 However, it was not until the early 1900s that the elucidation of chemical structure and synthetic processes started to revolutionize the field of organic chemistry. One of the leaders in organic chemistry during this time (ca. 1910–1950) was Sir Robert Robinson who is remembered for his research on anthocyanins, alkaloids, and the famous Robinson annulation. Robinson was one of many early organic chemists who exploited a unique ability to recognize the relationships and patterns which existed between chemical structures and their reactivity. Advances by early organic chemists like Robinson paved the way for later chemists including R. B. Woodward, E. J. Corey, K. C. Nicolaou, and countless others to build on this mechanistic understanding and further grow the field of organic chemistry.2 Seminally, Corey brought retrosynthetic organic chemistry into the mainstream for assembling complex molecules in the 1960s, and chemical synthesis itself has, in turn, contributed to expanding the number of proven retrosynthetic pathways.3,4 Retrosynthesis provides an efficient tool for mitigating problems in planning the synthesis of complex organic molecules. It starts from the complex molecule to be synthesized and works in the reverse direction to sequentially break down the complex molecule into a series of elementary steps of substrate combinations that lead to the desired product. The ‘Network of Organic Chemistry’ now contains almost every reaction published in the literature and hosts 35 million chemicals typically allowing for derivation of multiple suggested pathways towards the synthesis of any new molecule.5
Although incredibly powerful and representing the current ‘state-of-the-art’, multistep organic synthesis typically relies on specific catalysts and requires a sequential and stepwise process (assemble reactants → synthesis → purify product from reactants and undesired byproducts → determine yield/purity/enantiomeric excess (ee) → repeat with next reaction step) that is characterized by diminishing yield as the number of steps and time needed increase, ubiquitous organic waste, sometimes non-trivial product isolation, along with relying on requisite expertise. In contrast, eons of evolution has allowed nature to evolve enzymes and improve their properties allowing them to efficiently catalyze complex multistep syntheses. Enzymes possess the ability to carry out a variety of multistep reactions inside the cell, in a one-pot manner if you will, achieving unparalleled regio- and stereospecificity within the cellular cytosol. Cells have numerous membrane-bound organelles where reactions take place, as well as molecular crowding that create localized regions of function within the cytosol. Further, there are certainly biochemical processes that begin at one location in a cell and end at another. Our use of the descriptive ‘one-pot’ for reaction site refers to the cell as a total synthetic entity here. Depending upon substrain type, it is estimated that an E. coli bacterial cell can carry out >1400 different enzymatic reactions with more than a plurality running concurrently.6 Moreover, most of the complex molecules made by nature still cannot be emulated by current organic chemistry.7 Given the vast number of enzymes that exist in nature, many of which are still waiting to be described but which will nevertheless contribute to expanding the repertoire of available reactions, and the incredible potential of directed evolution, the field of synthetic biology contains perhaps all the necessary building blocks to bring about the realization of applied enzymatic retrosynthesis.8 This would allow the power of enzymatic synthesis to be harnessed in a similar manner to its analogous chemical system while bringing with it remedies for many of the issues that plague organic synthesis. Current thinking anticipates that enzymatic retrosynthesis will be primarily implemented using conventional cell-based synthetic biology where requisite designer and evolved multi-enzyme pathways hosted by chassis organisms carry out an ‘optimized’ designer synthesis.8–11 In relying primarily on cell-based approaches, a significant amount of potential synthetic chemical space is precluded simply due to cellular toxicity issues.
In this perspective, we suggest that there are several other complimentary approaches that can not only contribute to building the enzymatic retrosynthesis toolbox, but which can also help expand its capabilities significantly beyond that of what cells can provide. Beyond enzyme evolution and redesign, we look to see if there are other ways to get Nature's currently available repertoire of enzymes to perform in a more efficient manner ex vivo and to catalyze reactions that they normally would not undertake in a cell. In conjunction with this, we highlight key areas of research and viable alternatives to cell-based systems that are needed to populate the enzyme retrosynthetic database such that it can incorporate substrates that would be toxic to cells along with, perhaps more importantly, those considered non-natural or xenobiotic. We begin by looking at the promise of cell-based synthetic biology along with its limitations as the latter are the key areas we wish to address and overcome.
Synthetic biology and enzymatic synthesis
Synthetic biology is being touted as the next industrial revolution due to its potential to address the many existing socioeconomic problems associated with the exponentially growing demands of an industrially and technologically-dependent planet.12–14 This potential is real and is certainly not under dispute here. For these purposes, the salient points are that the expected way forward will engineer cellular chasses (e.g. bacteria or yeast) to host designer enzymatic pathways to produce the desired molecules from precursors or even generic carbon–nitrogen sources in an efficient manner.8–11 If a particular chemical reaction/step is not available in Nature, then it is hoped that new enzymes can be evolved to accomplish this step.15–17 Cell-based approaches come with many inherent benefits, which also serve to highlight the current interest and enticement in exploiting them, these include: cells replicate themselves; cells can be engineered to tolerate focused enzymatic pathways that produce a given molecule while removing competing pathways; recombinant DNA technology and molecular biology now provide a powerful toolbox for engineering cells and pathways; cells can make and continuously replenish all the enzymes they are directed to use; the confines of a cell can act as a highly concentrated synthetic vessel to facilitate efficient catalysis; and industrial fermenters can host thousands of gallons of an actively producing culture.18
So what is it that cell-based synthetic biology cannot do (at least currently)? Cells typically cannot tolerate the vast majority of unnatural or xenobiotic substrates, especially, if they inhibit a key pathway or adversely alter key cellular molecules or structures downstream. This point is exemplified by amino acid analogs, many of which are highly toxic to cells as they cannot be incorporated into nascent proteins and can poison the cell.19,20 This does not mean that enzymes in a given cascade cannot act on that substrate; in fact, the enzymes may be tolerant or promiscuous to the analog and the chemical transformations still potentially useful, just not in the context of a cell if they are to be exploited. Another key point to be appreciated is the interdependence amongst the vast number of enzymatic pathways present in a cell. Nature is nothing if not efficient and frugal, and so one enzymatic pathway can cross-feed into multiple products from a common intermediary rather than requiring multiple redundant pathways to make the same intermediary. For example, cellular glycolysis feeds into or is otherwise intertwined with amino acid, nucleic acid, and fatty acid synthesis along with many other metabolic processes. Unfortunately, along with these pathways competing with each other, which can be detrimental from a production standpoint, this also now provides multiple points to poison a cell. There may also not be a pathway for cellular internalization of a non-natural analog and similarly, no pathway for export before it builds up and becomes toxic. Moreover, if a natural version of an analog is required for growth, this can make for complex final product separation.
The current solution to some of these issues comes in the form of either major metabolic reengineering or cell-free biosynthesis, where the cellular cytosol and its contents are appropriated, or recombinantly constituted, to do the catalysis in vitro.21 Although clearly useful, this brings with it some of the same issues and a significant financial burden. For example, a single 25 μL reaction from the PURExpress In vitro Protein Synthesis Kit manufactured by New England Biolabs has a current cost of ∼US$25.22,23 In-between the boundaries of purely cell-based approaches versus purely cell-free methods for synthetic biology exist many different potentially viable variants where cell culture, cell lysates, and designer mixtures of enzymes and other components can still be used to carry out a set of reactions of interest.24–29 In cell lysates, the enzyme has been grown and harvested within the cell and subsequent lysis has been performed to release the enzyme from the cell. Therefore, the cell lysate represents a material containing an abundance of the cell-free enzymes of interest along with other cellular components. One undisputed advantage of this approach is the reduction in cost relative to reconstituted/artificial cell-free systems since the cell lysate requires fewer purification steps for production. However, a disadvantage of using cell lysates is that mixture complexity is increased relative to purified enzymes since lysis induces a release of all enzymes present in the cell, such as periplasmic nucleases and peptidases, which then remain in the cell extract potentially reacting with intermediates and/or substrates present.30 A variety of possible hybrid systems exist for performing multi-step reactions such as adding purified enzymes to cell lysates, combining chemical catalysts with enzymes, supplementing cell-based systems with purified enzymes, etc.31–35 If needed, there is no reason why the first part of a reaction sequence couldn’t be done outside a cell with a toxic but cell impermeable substrate or intermediate. Further, two-pot reactions with a cell-free reactor and cell-based reactor can even be linked through chemical engineering methods. It must be appreciated that with each additional component or custom configuration there exists a consequent increase in mixture complexity and a series of benefits and liabilities that will need to be carefully considered.
The true power of synthetic biology will only be unleashed when it can access the chemical space now precluded from cells and move from ‘natural’ substrates and products to those that are not found in nature; in essence, when we can harness enzymes to make almost any molecule we desire in a designer fashion. This will be especially true if we can do this with the knowledge and power of unfettered retrosynthesis driving the field. So how do we get from these issues to a form of enzymatic retrosynthesis that can accommodate such non-natural chemistry and even build on it? We suggest several avenues of research that are needed, which cumulatively have strong potential for positive contribution here. Namely,
(i) Developing focused minimalistic multi-enzyme synthetic systems.
(ii) Undertaking substrate tolerance and specificity studies for major classes of enzymes.
(iii) Optimizing desired catalytic activity outside a cell.
(iv) Determining relevant thermodynamic–kinetic properties.
The concepts behind each of these ideas are briefly developed in the following sections. We note that many of these ideas are not unique to us and have been previously iterated in various different forms by others.8,30
Minimalist multi-enzyme synthetic systems
Broadly speaking, the minimum number of components needed to implement a multi-enzyme biosynthetic cascade are the requisite enzymes, their cofactors, and substrates. The driving concept behind ‘minimalist’ here is to remove all manner of competitive pathways and the presence of any substrate inhibitors such that the only possible reaction is the one desired; this, of course, does not account for reversible or back-reactions. The number of molecules and their derivatives which are known to either be recalcitrant to diffusion through cell walls and/or induce cellular death are both abundant and diverse.36 The net result of this is a vast synthetic chemical space, which simply cannot yet be explored within the constraints of cellular-based systems, and which make this concept of minimalism attractive within the field of synthetic biology.
Within cellular-based systems, an enzyme's promiscuity cannot surmount what the cell itself cannot tolerate. Outside a cell, an individual enzyme's promiscuity can and will increase overall flexibility in the design of biosynthetic pathways.37 By isolating an enzyme which carries out a desired function from the cell, the ability to identify all the promiscuous attributes for a specific enzyme can now be realized (vide infra). In the construction of multistep one-pot enzymatic reactions, working outside the cell also greatly simplifies pathway design to synthesize a product of interest and this is especially true if all manner of competition is removed. The ability to combine enzymes isolated from different organisms to function in the same biosynthetic pathway also offers the potential to truly harness all the reactivity nature has to offer. This also obviates the need, for example, to back engineer eukaryotic enzymes for expression in bacterial systems.38 Further, minimalist approaches allow for rapid detection and identification of bottlenecks and/or degradation pathways.37 By simplifying the number of components within a system to include only what is required, the ability to identify sources to problems such as slow conversion, incomplete conversion, or off target activity/degradation within a biosynthetic pathway become much easier. A great deal of leeway is also available in how such reactions are implemented with easy adjustment to variables such as volume, concentration, temperature, and the like. Moreover, concerted efforts are currently underway to create multifunctional nanoscale scaffolds that stabilize the enzymes, control the placement, order, and stoichiometry of the enzymes, and even allow them to access phenomena such as channeling.39
The potential in this approach is perhaps epitomized by work from the Bowie and Lou Labs towards the synthesis of molecules such as monoterpenes and D-glucaric acid, respectively.40,41 Monoterpenes are a class of isoprenoids widely used within the pharmaceutical, cosmetic, and agricultural industries.42–44 However, within cells, monoterpenes are highly toxic and previous cell-based studies have found that specific types of monoterpenes can cause damage to the plasma membrane leading to decreased downstream ATP concentrations.45 This property has hindered the industrial application of cell-based terpene biosynthesis due to the low inhibitory concentrations that must be maintained in order to sustain cell viability.46 The Bowie Lab reported the design of a multi-enzyme cell-free system to produce monoterpenes from glucose at production yields which were over an order of magnitude greater than cellular toxicity limits.40 They combined 27 enzymes to process glucose into monoterpenes and by switching between specific terpene synthase enzymes at the final step of the pathway, they could vary the product from limonene to pinene and sabinene; see the enzymatic pathway schematic in Fig. 1. This pathway utilized enzymes drawn from 13 different species/strains many of which were optimized mutants. Later work demonstrated a cascaded system that produced the bioplastic polyhydroxybutyrate from glucose along with a ‘purge valve’ node for preventing the buildup of excess reduced nicotinamide adenine dinucleotide phosphate (NADPH) which would become inhibitory.30 Similarly, D-glucaric acid is a targeted high-value molecule derived from biomass due to its potential application in biodegradable detergents and polymers.47 Several cell-based methods for its biosynthesis have been previously described in both yeast and E. coli. However, these strategies are limited in industrial fermentation methods due to low conversion rates, unbalanced pathway fluxes, and issues of cellular acid toxicity.48,49 Cell-free methods were similarly developed by Lou for the production of D-glucaric acid from sucrose whereby addition of a single NAD+ regenerative enzyme was able to significantly increase the overall efficiency of this pathway.41 Beyond the benefits and capabilities described here, such minimalist enzymatic systems also provide a potent format for implementing the next area of discussion.
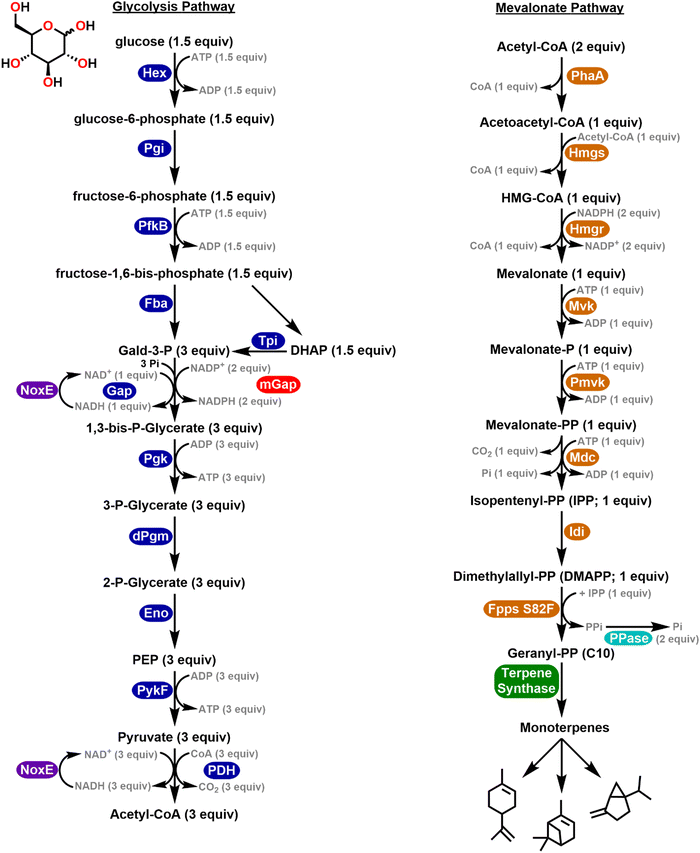 |
| Fig. 1 Multienzymatic pathway converting glucose into monoterpenes. Schematic of the synthetic enzyme system converting glucose to monoterpenes. Glycolytic and mevalonate enzymes are highlighted in blue and orange respectively. Auxiliary enzymes constituting the purge valve, (mGap and NoxE) and phosphate recycling by pyrophosphatase are highlighted in red, purple, and cyan. Different monoterpenes can be made by using alternative terpene synthases (green). Abbreviations: Hex – hexokinase, Pgi – glucose-6-phosphate isomerase, Pfk – phosphofructokinase, Fba – fructose-1,6-bisphosphate aldolase, Tpi – triose phosphate isomerase, Gap – gald-3-P dehydrogenase, mGap – gald-3-P dehydrogenase, Pgk – phosphoglycerate kinase, dPgm – 2,3-bisphosphoglycerate-dependent phosphoglycerate mutase, Eno – phosphoenolpyruvate hydratase, PykF – pyruvate kinase, PDH – pyruvate dehydrogenase, PhaA – acetyl-CoA acetyltransferase, Hmgs – β-hydroxy β-methylglutaryl (HMG)-CoA synthase, Hmgr – HMG-CoA reductase, Mvk – mevalonate kinase, Pmvk – phosphomevalonate kinase, Mdc – mevalonate-PP decarboxylase, Idi – isopentenyl-PP isomerase, Fpps – farnesyl-PP synthase, NoxE – NADH oxidase, PPase – pyrophosphatase, Gald-3-P – glyceraldehyde 3-phosphate, DHAP – dihydroxyacetone phosphate, P – phosphate, PEP – 2-phosphophenolpyruvate, HMG – 3-hydroxy-3-methylglutaryl, PP – diphosphate. Schematic drawn from ref. 40. | |
Substrate tolerance and specificity studies
An enzyme's specificity refers to its ability to activate a specific substrate amongst a pool of structurally similar analogs, while enzyme promiscuity is an enzyme's ability to tolerate a substrate and catalyze a reaction with it, especially when it is not normally part of its functional repertoire.50 In directed evolution, it has been shown that promiscuity simplifies the search for an enzyme that will act on a specific target.16,17 However, in the design of new biosynthetic pathways, past studies suggest that in multistep cascades the extent of enzyme promiscuity may be more inhibitory than helpful because, as reaction pathways become increasingly complex, so too does the requirement for specificity of the enzymes involved.16,17 Accordingly, previous studies have even focused on methods for increasing an enzyme's specificity for a target substrate.51,52 To date, this remains a problem for applications of directed evolution in multistep cascade design.8 In terms of reactivity profiles for different enzymes, the most common approach is to generate a substrate scope for a particular enzymatic pathway and provide the corresponding yields for each substrate.53,54 By determining this for major enzyme classes, and variants of a given enzyme – using panels of substrate analogs that include diverse unnatural or xenobiotic derivatives – enough information can be gleaned to be able to predict which substrate(s) a given enzyme will tolerate and which they will not. This is obviously part of the critical information needed to populate a retrosynthetic enzyme database. However, this only provides information on whether a specific reaction will proceed. More valuable, would be the additional understanding of how the initial rate or the total turnover number (TTN) of an enzyme changes across a series of systematically selected substrates. This information is relevant because it provides insight into the robustness of an enzyme and its overall lifetime, which aids in the selection of enzymes for multistep cascades.55 Similarly, a thorough understanding of the factors which dictate the initial rate of an enzyme are needed in order to iteratively enhance the performance of an individual enzyme.56 It is important to appreciate that the suite of commercial, and in many cases, automated, ‘design, test, build, learn’ tools developed for bioprospecting in synthetic biology can be brought to bear here and allow such studies to be undertaken in a massively-parallel and automated fashion.57,58 The complexity involved for developing a database to aid in enzyme selection methods can be appreciated from the work of Henrissat and co-workers in the development of the CAZy database which compiles carbohydrate-active enzymes into groups based on enzyme sequence and specificity.59–61
Optimize desired catalytic activity outside a cell
While the removal of an enzyme from a cellular environment is often associated with a decrease in enzyme stability, it also provides the opportunity for simplified testing strategies to optimize its activity and analyze its ability to tolerate de novo substrates. By optimization, we stipulate to techniques that do not involve mutagenesis or evolution, which are already well-established ways to optimize enzymatic performance but which are based on different principles and considerable effort.62–64 For a single step enzymatic reaction within a cell-free system, the environmental conditions of the enzyme can be systematically varied such that the accessible operating conditions of the enzyme can be defined and optimized for a specific activity. Similarly, this same strategy can be applied to multistep enzymatic cascades. Here, the ability to optimize conditions whereby the rate of the slowest enzymatic step increases while conditions which are conducive to all enzymes in the system can be maintained is an invaluable tool to optimize the flux through a cascade.55 One elegant example of this approach is the six-enzyme cascade developed for the synthesis of amorpha-4,11-diene, a precursor in the synthesis of the drug artemisinin used to treat malaria. This study demonstrated a methodology to test and analyze enzyme ratios, buffer conditions, pH, and ion choice, which increased the rate limiting step of a multi-enzymatic cascade such that the overall rate of product formation was also optimized.65
For our focus, the goal is to increase the efficiency with which an enzyme catalyzes the reaction of both native and non-native substrates. There are many proven ways to manipulate an enzyme's reaction environment such that some desirable functionality is increased. These include altering buffer composition, pH, ionic concentration, temperature, viscosity, pressure, adding organics and the like, along with adding other exogenous materials.11,30,66 See Table 1 for some representative examples. One of the more prominent successes in this regard is the use of lipases and esterases in reactions with different organic solvents added; this has allowed these enzymes to increase their substrate and prochiral selectivity and, in direct contrast to their expected hydrolytic activity, even catalyze synthetic reactions such as C–C and C–heteroatom bond formation along with Michael addition.67–69 Clearly, some enzymes are latently capable of doing one reaction in one context and a completely different type of chemistry in a different context.
Table 1 Examples of changes to enzyme activity and substrate tolerance obtained by altering select reaction conditions
Enzyme |
Native activity |
Alteration |
Change |
Utility |
Ref. |
Also known as phosphotriesterase.
Pichia pastoris.
Hyperthermophilic eubacterium Thermotoga maritima.
Trametes versicolor.
|
Methyl-parathion hydrolasea |
Organophosphate hydrolysis |
Change divalent cation in catalytic site |
100-fold increase in activity from Ca2+ to Ni2+ |
Increased activity |
70
|
Alcohol oxidaseb |
Convert short chain alcohols to aldehydes/ketones |
High hydrostatic pressure |
Stability to thermal deactivation |
Increased lifetime and extended reaction conditions |
55, 56, 71 and 72
|
Glucose oxidase |
Glucose oxidation to H2O2 and D-glucono-δ-lactone |
High hydrostatic pressure |
Stability to thermal deactivation |
Glucose sensing |
73
|
Thymidine kinasec |
Phosphorylate thymidine |
Change temperature from 82° to 37 °C |
Increased promiscuity for non-natural substrate |
Phosphorylate wide variety of nucleotide analog prodrugs |
74
|
Bovine liver catalase |
Breakdown reactive oxygen species |
Increase temperature |
Tolerated higher H2O2 concentration |
Utility in reactions with high H2O2 present |
75
|
Lipases |
Hydrolyze fatty acids |
Increase organic solvent |
C–C and C–heteroatom bond formation, Michael addition |
Fat removal and industrial synthetic applications |
69
|
Lipases |
Hydrolyze fatty acids |
Change solvent present |
Increase in prochiral selectivity |
Commercial transformations |
76
|
Polygalacturonase |
Hydrolyzes α-1,4 glycosidic bonds of pectic acid |
Change temperature and pressure |
Increased activity |
Increased activity at lower temperatures |
77
|
Alkaline endo-1,4-β-glucanase |
Hydrolyze glucosidic bonds of cellulose |
pH change from alkaline to slightly acidic (pH 6) |
10-fold increase in hydrolysis of cellohexaose and cellopentaitol |
Optimized activity for additional substrates |
78
|
Laccased |
Catalyze oxidation reactions by reducing O2 to water |
Addition of water miscible ionic liquid choline dihydrogen phosphate |
451% rate and 4.5 fold increase in laccase stability at room temperature |
2,2′-Azino-bis(3-ethylbenzothiazoline-6-sulfonic acid) diammonium salt oxidation |
79
|
Another method for optimizing enzyme activity is through various immobilization strategies.80 As this is often associated with increased stability, it offers a potential solution to counteract the decreased stability of an enzyme once it is removed from a cellular matrix and increase its useful lifetime.81 Several immobilization strategies have been developed including enzyme display on metal nanoparticles, encapsulation within biological or polymeric materials, and chemical cross-linking.39,66,81–84 See Fig. 2 for an example of where nanoparticle display on a semiconductor quantum dot (QD) allowed the enzyme phosphotriesterase to function 2× more efficiently (higher catalytic rate – kcat) and further do this in the presence of increased concentrations of a competitive inhibitor, i.e., 3× increase in Ki.85 Interestingly, enzymatic enhancement in this context appears to be dependent upon NP size with smaller diameter materials somewhat counterintuitively displaying the largest enhancement.86 In the specific context of multiple enzymes (two or more) working together in a concerted or coupled biocatalytical cascade, attachment to a NP surface may potentially offer access to other useful phenomena beyond just enzyme stabilization and/or enhancement, in the form of enzymatic channeling. Probabilistic substrate/intermediary channeling can dramatically improve the rates of catalytic flux in multienzyme systems by increasing the rate of intermediary transfer between proximal enzymes in a manner that competes with what is more typically expected to be the much faster rate of product diffusion away from the enzyme.85Fig. 3 highlights some representative data from a recent example where the coupled activity between pyruvate kinase (PykA) and lactate dehydrogenase (LDH) were evaluated when assembled onto QDs.87 In this example, the tetrameric enzymes were found to crosslink with the QDs into nanoaggregated structures which stabilized LDH and significantly increased the rate of coupled PykA–LDH activity by ca. 100-fold versus that of equivalent free enzyme (see Fig. 3C and D). Another example looked at the interaction of a 3-enzyme pathway when attached to the surface of a single but much larger gold NP.88 Here, the enhanced catalytic flux attributable to channeling processes around the same NP was far more modest at ∼3-fold. In a further tantalizing report that hinted at application of this phenomena to far more complex systems, NP addition also enhanced reaction rates in full transcription–translation cell-free reaction mixtures.23,39,66,81–84 Beyond this, even DNA structures are being tested as multienzyme scaffolds due to their ability to directly control enzyme number, order, and sequentiality.39,89 We expect studies of these and related phenomena will help reveal the unique enzymatic capabilities that are not manifest in live cells especially if the substrate is non-natural. Again, such studies can be carried out in a parallel, parametric format to screen for the desired mechanistic information.
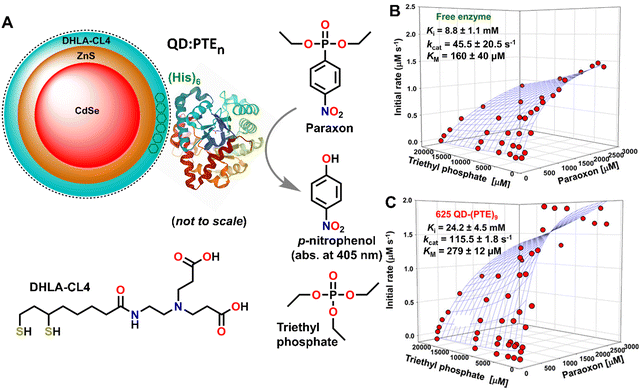 |
| Fig. 2 Nanoparticle enhancement of enzyme activity. (A) Schematic of a CdSe/ZnS core/shell semiconductor quantum dot (QD) surface-functionalized with the DHLA-CL4 ligand to make it colloidally stable in buffer. Phosphotriesterase (PTE) is ratiometrically self-assembled to the QD surface by its terminal hexahistidine (His)6 sequence. The average number of PTE per QD is controlled through the molar stoichiometry added during assembly. PTE hydrolysis of paraoxon substrate to p-nitrophenol product, which absorbs at 405 nm, is also shown schematically. Structure of the PTE competitive inhibitor triethyl phosphate. QD phosphotriesterase bioconjugate triethyl phosphate inhibition assays. Three-dimensional plots of PTE initial rates versus increasing paraoxon concentration in the presence of increasing triethyl phosphate inhibitor for (B) free enzyme and (C) 625 QD nm emitting (diameter 9.3 nm)–(PTE)9 bioconjugates. Estimated Ki, kcat, and KM values shown for each. Figure reproduced with permission from ref. 85 Copyright 2015 American Chemical Society. | |
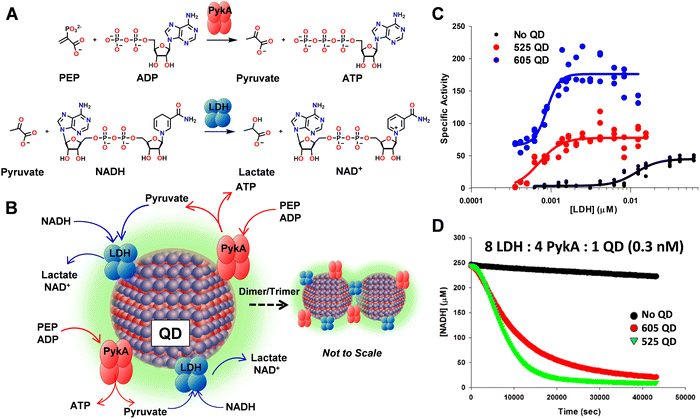 |
| Fig. 3 Coupled enzymatic and channeling activity when assembled on a QD scaffold. (A) Reaction scheme for coupled PykA and LDH activity. Individual and coupled enzyme activity monitored by NADH consumption with loss of its absorption at 340 nm. (B) Schematic of the coupled PykA–LDH enzyme system colocalized on a QD surface. The propensity of the enzymes to form cross-linked QD dimers and, to a lesser extent, trimers via the enzyme's tetrameric polyhistidine tags located at each monomers distal end is also schematically indicated. Note, not to scale. (C) LDH tetramer stability monitored as its concentration is reduced when free in solution and as assembled to QDs. Specific activity of LDH (μM NADH consumed s−1 μM LDH−1) determined at various enzyme concentrations in the absence (black) or presence of 605 nm emitting QD (blue, diameter ∼10.1 nm) or 525 nm emitting QDs (red, diameter 4.3 nm). Data fit to a dissociation equation. Note how LDH activity when assembled to the QDs significantly extends its activity profile below the 10 nM dissociation constant of the wildtype enzyme. (D) Coupled PykA–LDH enzymatic kinetics. NADH consumption in a combined PykA–LDH reaction monitored with enzymes assembled to 605 (red) or 525 QDs (green) and QD-free (black). The reactions were at a concentration of 8 LDH (2.4 nM)/4 PykA (1.2 nM)/1 QD (0.3 nM). Enzyme-only contained the equivalent amount of free enzyme. Figure reproduced with permission from ref. 87 Copyright 2018 American Chemical Society. | |
Determining relevant thermodynamic–kinetic properties
For any single step enzyme catalyzed reaction, the reaction's thermodynamic and kinetic factors control the rate of substrate conversion to product. For successful product formation, the overall difference in energy between the product and reactant under a given set of conditions must be negative (i.e.,
). Additionally, the energy between the transition state (TS) and the reactant (i.e., ΔG‡) must be low enough such that the kinetic barrier for the rate-limiting step of the reaction can be overcome. The more the thermodynamic and kinetic free energy values decrease for the rate-limiting step of a reaction, the more facile or favored a given reaction becomes. Experimentally optimizing thermodynamic parameters to achieve optimal enzyme kinetics has previously shown itself to be a valuable tool for successful biosynthesis. For example, Aitken and Heck showed that the activity of a peroxidase enzyme was related to the homolytic O–H bond dissociation energy (BDE) by analyzing the activity of a peroxidase enzyme across a series of monosubstituted phenols.90 They found phenols with highly electron-donating (hydroxy and amino) substituents led to inactivation of the enzyme with minimal substrate removal via a mechanism that was unrelated to how the enzyme catalyzed other phenolic substrates. Russell and co-workers identified a temperature dependence on the catalytic rate (kcat) for a chymotrypsin enzyme both in its native state and when modified as a polymer based material.91 Increasing hydrostatic pressure has even been directly correlated to the activity of an alcohol oxidase enzyme.72 Lastly, the specific activity of a nitrite reductase was found to correlate with the redox potential of the mediator used in the biocatalytic conversion of nitrite to ammonia.92 In each of these examples, a thermodynamic parameter was varied and correlated with the observed effect on the kinetic activity of the enzyme. Driving reactions to completion by lowering the thermodynamic free energy
is most advantageous to the overall enzymatic reaction as long as the kinetic barrier for the reaction (ΔG‡) decreases, or remains unchanged. Therefore, correlating thermodynamic–kinetic parameters with one another is a simple approach to understanding how to systematically decrease the free energy requirements which dictate product formation. One such useful tool for the determination of thermodynamic values is the eQuilibrator program, which provides estimations of thermodynamic values for small molecules adjusted for experimental pH and ionic strength.93 In developing multienzyme cascades, the obvious requirement is that the difference in energy between product and starting material (i.e., initial reactant) again be negative (i.e.,
). Beyond that, the ability to understand how kinetic parameters for the rate-limiting step of a given enzyme correlate with thermodynamic changes can enable efficient determination of the preeminent enzyme, or homolog thereof, that will be most suitable within a given cascade. Overall, it is our opinion that an understanding of relevant thermodynamic–kinetic parameters which dictate activity across a series of enzyme analogues could be an invaluable tool for iteratively optimized enzyme engineering and selection processes. Most simplistically, it would help to provide a framework for which analogs will be tolerated and which steps in a given enzymatic reaction pathway may or may not work along with what conditions are needed to make it move in the desired direction.
Outlook: towards a framework for assembling retrosynthetic enzymatic pathways
Enzymatic retrosynthetic platforms are starting to be developed with beta versions becoming available and issues with their initial accuracy (≤50%) has strengthened the call to populate them with as much data about each enzyme and their substrate tolerance as possible.94–96 Coming back full circle, contributions from the above ideas become more apparent in the framework of extending enzymatic retrosynthesis to focus on including non-natural substrates and making non-natural or xenobiotic products. Similar to how chemical retrosynthesis is utilized conceptually, when a target molecule is desired, the enzymatic retrosynthetic platform will suggest the best combinations of enzymes and order of enzymatic steps needed to assemble that molecule. Within a minimalist reaction, the best combination of prokaryotic and eukaryotic-sourced enzymes can be jointly incorporated to carry out the desired chemistry without being challenged by competing pathways. The breadth of substrates that a given enzyme can tolerate will inform the suggested pathways and precursors to be used along with the intermediaries that can be accessed. Consideration of each enzyme's thermodynamic and kinetic properties in conjunction with optimization of reaction conditions, environment, enzyme immobilization, and the like can all be exploited to improve and drive the reactions forward and overcome any bottleneck or unfavorable step. See also Fig. 4, which illustrates this concept schematically to highlight the types of information that are potentially useful in this context and how they can contribute to enzymatic retrosynthesis and especially that of de novo products.
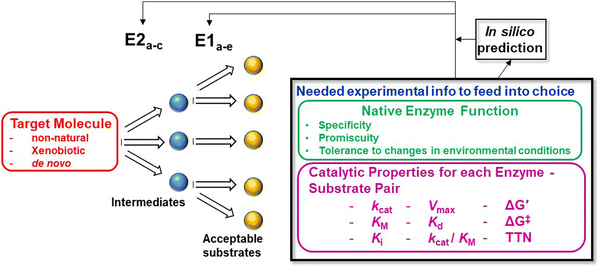 |
| Fig. 4 Schematic highlighting potential contributions from the studies suggested here towards augmenting retrosynthetic enzyme synthesis. The concepts behind each are outlined in the text along with how they can contribute to improving the data that will give rise to retrosynthetic enzymatic capabilities. E1 and E2 represent enzymes in an assembled pathway while the subscripts a–c or a–e represent homologs with different substrate and reactivity profiles. | |
Of course, all of this is meant to happen within the same framework as that used for cell-based synthetic biology. They are not distinct from each other but are rather meant to be complementary. Our approach enriches and expands the attainable product space and provides choices and enzymatic chemistries currently not available within live cell systems. Some enzymatic reactions are just not available and here is where enzyme redesign and evolution will once again be invaluable. Additionally, not every step in a desired synthesis can be accessed enzymatically and so mixed chemo-enzymatic approaches will continue to be a mainstay as well.97 Similarly, not every catalyzed multi-step reaction will be viable in a one-pot minimalist cell-free biosynthetic approach and therefore flow-based systems will continue to be necessary to separate multi-step reactions into viable steps that enable product formation.98–100 One-pot and minimalist are not meant to be all encompassing and immobile approaches, rather they can also be philosophical and conceptual if needed. If one or more steps or reactions can be simplified and minimized to make the overall process more efficient and specific, then the minimalist approach is still contributing to some extent. Advances in artificial intelligence (AI) and machine learning (ML) will clearly be critical to help both compile the exponentially growing library of data describing enzyme properties and characteristics along with mining that data for complex properties and relationships that are not overtly available at a first look to the human eye.101 So is all this easy to achieve? Of course not! A great deal of discovery, enzyme prospecting, experimental testing, meta-analysis, and years of hard work along with overcoming an untold number of problems still remain to see if even some of these concepts prove useful. We look forward to contributing here as well and to learning about other ways to apply enzymes in a similar manner.
Conflicts of interest
There are no conflicts of interest to declare.
Acknowledgements
The authors acknowledge the Office of Naval Research (ONR), the U.S. Naval Research Laboratory (NRL), and the NRL Nanoscience Institute for funding support. S. L. H. acknowledges a National Research Council Fellowship through NRL. I. L. M. and G. A. E. acknowledge the National Institute of Food and Agriculture, U.S. Department of Agriculture, under Award #2020-67021-31254, and the Strategic Environmental Research and Development Program (SERDP), under Award # WP21-1073 New Start Project (W74RDV03497375).
References
- F. Wöhler, Ueber künstliche Bildung des Harnstoffs, Ann. Phys., 1828, 88, 253–256 CrossRef.
- E. J. Corey, Robert Robinson Lecture. Retrosynthetic Thinking—Essentials and Examples, Chem. Soc. Rev., 1988, 17(0), 111–133 RSC.
-
E. J. Corey, General Methods for the Construction of Complex Molecules, The Chemistry of Natural Products, Butterworth-Heinemann, Oxford, UK, 1967, pp. 19–37 Search PubMed.
- E. J. Corey, A. K. Long and S. D. Rubenstein, Computer-Assisted Analysis in Organic Synthesis, Science, 1985, 228(4698), 408–418 CrossRef CAS PubMed.
- P. M. Jacob and A. Lapkin, Statistics of the Network of Organic Chemistry, React. Chem. Eng., 2018, 3(1), 102–118 RSC.
- P. D. Karp, R. Billington, R. Caspi, C. A. Fulcher, M. Latendresse, A. Kothari, I. M. Keseler, M. Krummenacker, P. E. Midford, Q. Ong, W. K. Ong, S. M. Paley and P. Subhraveti, The BioCyc Collection of Microbial Genomes and Metabolic Pathways, Briefings Bioinf., 2019, 20(4), 1085–1093 CrossRef CAS PubMed.
- A. Schmid, J. S. Dordick, B. Hauer, A. Kiener, M. Wubbolts and B. Witholt, Industrial Biocatalysis Today and Tomorrow, Nature, 2001, 409(6817), 258–268 CrossRef CAS PubMed.
- G.-M. Lin, R. Warden-Rothman and C. A. Voigt, Retrosynthetic Design of Metabolic Pathways to Chemicals Not Found in Nature, Curr. Opin. Syst. Biol., 2019, 14, 82–107 CrossRef.
- N. J. Turner and E. O'Reilly, Biocatalytic Retrosynthesis, Nat. Chem. Biol., 2013, 9(5), 285–288 CrossRef CAS PubMed.
- R. Wohlgemuth, Tools and Ingredients for the Biocatalytic Synthesis of Metabolites, Biotechnol. J., 2009, 4(9), 1253–1265 CrossRef CAS PubMed.
- N. J. Claassens, S. Burgener, B. Vögeli, T. J. Erb and A. Bar-Even, A Critical Comparison of Cellular and Cell-Free Bioproduction Systems, Curr. Opin. Biotechnol., 2019, 60, 221–229 CrossRef CAS PubMed.
- M. El Karoui, M. Hoyos-Flight and L. Fletcher, Future Trends in Synthetic Biology—A Report, Front. Bioeng. Biotechnol., 2019, 7(7), 175 CrossRef.
-
National Research Council, National Academy of Sciences USA, Industrialization of Biology: A Roadmap to Accelerate the Advanced Manufacturing of Chemicals, The National Academy Press, Washington, DC, 2015 Search PubMed.
- Redesigning Life. The Promise and Perils of Synthetic Biology. The Economist 2019, April (Technology Quaterly), 3–11.
- J. Nazor, J. Liu and G. Huisman, Enzyme evolution for Industrial Biocatalytic Cascades, Curr. Opin. Biotechnol., 2021, 69, 182–190 CrossRef CAS PubMed.
- N. J. Turner, Directed Evolution Drives the Next Generation of Biocatalysts, Nat. Chem. Biol., 2009, 5(8), 567–573 CrossRef CAS PubMed.
- C. Zeymer and D. Hilvert, Directed Evolution of Protein Catalysts, Annu. Rev. Biochem., 2018, 87(1), 131–157 CrossRef CAS PubMed.
- L. Clarke and R. Kitney, Developing Synthetic Biology for Industrial Biotechnology Applications, Biochem. Soc. Trans., 2020, 48(1), 113–122 CrossRef CAS PubMed.
-
K. J. Rodgers, K. Samardzic and B. J. Main, Toxic Nonprotein Amino Acids, in Plant Toxins, ed. C. R. Carlini, R. LigabueBraun and P. Gopalakrishnakone, 2017, pp. 263–285 Search PubMed.
- P. Staszek, L. A. Weston, K. Ciacka, U. Krasuska and A. Gniazdowska, L-Canavanine: How Does a Simple Non-Protein Amino Acid Inhibit Cellular Function in a Diverse Living System?, Phytochem. Rev., 2017, 16(6), 1269–1282 CrossRef CAS.
- E. D. Carlson, R. Gan, C. E. Hodgman and M. C. Jewett, Cell-Free Protein Synthesis: Applications Come of Age, Biotechnol. Adv., 2012, 30(5), 1185–1194 CrossRef CAS.
- New England Biolabs, I. PURExpress® In Vitro Protein Synthesis Kit, https://www.neb.com/products/e6800-purexpress-invitro-protein-synthesis-kit#Product%20Information (accessed 03/10/2022).
- M. Thakur, J. C. Breger, K. Susumu, K. Oh, J. R. Spangler, I. L. Medintz, S. W. Walper and G. A. Ellis, Self-Assembled Nanoparticle-Enzyme Aggregates Enhance Functional Protein Production in Pure Transcription-Translation Systems, PLoS One, 2022, 17(3), e0265274 CrossRef CAS PubMed.
- J. R. Swartz, Expanding Biological Applications Using Cell-Free Metabolic Engineering: An overview, Metab. Eng., 2018, 50, 156–172 CrossRef CAS PubMed.
- J. E. Kay and M. C. Jewett, Lysate of Engineered Escherichia coli Supports High-Level Conversion of Glucose to 2,3-Butanediol, Metab. Eng., 2015, 32, 133–142 CrossRef CAS PubMed.
- M. Bechtold, E. Brenna, C. Femmer, F. G. Gatti, S. Panke, F. Parmeggiani and A. Sacchetti, Biotechnological Development of a Practical Synthesis of Ethyl (S)-2-Ethoxy-3-(p-methoxyphenyl)propanoate (EEHP): Over 100-Fold Productivity Increase from Yeast Whole Cells to Recombinant Isolated Enzymes, Org. Process Res. Dev., 2012, 16(2), 269–276 CrossRef CAS.
- Q. M. Dudley, K. C. Anderson and M. C. Jewett, Cell-Free Mixing of Escherichia coli Crude Extracts to Prototype and Rationally Engineer High-Titer Mevalonate Synthesis, ACS Synth. Biol., 2016, 5(12), 1578–1588 CrossRef CAS PubMed.
- B. J. Rasor, X. Yi, H. Brown, H. S. Alper and M. C. Jewett, An Integrated In Vivo/In Vitro Framework to Enhance Cell-Free Biosynthesis with Metabolically Rewired Yeast Extracts, Nat. Commun., 2021, 12(1), 5139 CrossRef CAS PubMed.
- Q. M. Dudley, A. S. Karim and M. C. Jewett, Cell-Free Metabolic Engineering: Biomanufacturing Beyond the Cell, Biotechnol. J., 2015, 10(1), 69–82 CrossRef CAS PubMed.
- J. U. Bowie, S. Sherkhanov, T. P. Korman, M. A. Valliere, P. H. Opgenorth and H. Liu, Synthetic Biochemistry: The Bio-inspired Cell-Free Approach to Commodity Chemical Production, Trends Biotechnol., 2020, 38(7), 766–778 CrossRef CAS PubMed.
- N. Cihan, G. Bharath, A. K. Nadda and O. YukselOrhan, A Hybrid Chemo-Biocatalytic System of Carbonic Anhydrase Submerged in CO2-Phillic Sterically Hindered Amines for Enhanced CO2 Capture and Conversion into Carbonates, Int. J. Greenhouse Gas Control, 2021, 111, 103465 CrossRef CAS.
- E. Liardo, N. Ríos-Lombardía, F. Morís, F. Rebolledo and J. González-Sabín, Hybrid Organo- and Biocatalytic Process for the Asymmetric Transformation of Alcohols into Amines in Aqueous Medium, ACS Catal., 2017, 7(7), 4768–4774 CrossRef CAS.
- S. Guo, T. Asset and P. Atanassov, Catalytic Hybrid Electrocatalytic/Biocatalytic Cascades for Carbon Dioxide Reduction and Valorization, ACS Catal., 2021, 11(9), 5172–5188 CrossRef CAS.
- J.-W. Song, E.-Y. Jeon, D.-H. Song, H.-Y. Jang, U. T. Bornscheuer, D.-K. Oh and J.-B. Park, Multistep Enzymatic Synthesis of Long-Chain α,ω-Dicarboxylic and ω-Hydroxycarboxylic Acids from Renewable Fatty Acids and Plant Oils, Angew. Chem., Int. Ed., 2013, 52(9), 2534–2537 CrossRef CAS PubMed.
- S. P. France, S. Hussain, A. M. Hill, L. J. Hepworth, R. M. Howard, K. R. Mulholland, S. L. Flitsch and N. J. Turner, One-Pot Cascade Synthesis of Mono- and Disubstituted Piperidines and Pyrrolidines using Carboxylic Acid Reductase (CAR), ω-Transaminase (ω-TA), and Imine Reductase (IRED) Biocatalysts, ACS Catal., 2016, 6(6), 3753–3759 CrossRef CAS.
- S. Orrenius, P. Nicotera and B. Zhivotovsky, Cell Death Mechanisms and Their Implications in Toxicology, Toxicol. Sci., 2010, 119(1), 3–19 CrossRef PubMed.
- C. E. Hodgman and M. C. Jewett, Cell-Free Synthetic Biology: Thinking Outside the Cell, Metab. Eng., 2012, 14(3), 261–269 CrossRef CAS PubMed.
- G. L. Rosano and E. A. Ceccarelli, Recombinant Protein Expression in Escherichia coli: Advances and Challenges, Front. Microbiol., 2014, 5, 172 Search PubMed.
- G. A. Ellis, W. P. Klein, G. Lasarte-Aragones, M. Thakur, S. A. Walper and I. L. Medintz, Artificial Multienzyme Scaffolds: Pursuing in Vitro Substrate Channeling with an Overview of Current Progress, ACS Catal., 2019, 9(12), 10812–10869 CrossRef CAS.
- T. P. Korman, P. H. Opgenorth and J. U. Bowie, A Synthetic Biochemistry Platform for Cell Free Production of Monoterpenes from Glucose, Nat. Commun., 2017, 8(1), 15526 CrossRef CAS PubMed.
- H.-H. Su, Z.-W. Guo, X.-L. Wu, P. Xu, N. Li, M.-H. Zong and W.-Y. Lou, Efficient Bioconversion of Sucrose to High-Value-Added Glucaric Acid by In Vitro Metabolic Engineering, ChemSusChem, 2019, 12(10), 2278–2285 CrossRef CAS PubMed.
- P. K. Ajikumar, K. Tyo, S. Carlsen, O. Mucha, T. H. Phon and G. Stephanopoulos, Terpenoids: Opportunities for Biosynthesis of Natural Product Drugs Using Engineered Microorganisms, Mol. Pharmaceutics, 2008, 5(2), 167–190 CrossRef CAS PubMed.
- U. Krings and R. G. Berger, Biotechnological Production of Flavours and Fragrances, Appl. Microbiol. Biotechnol., 1998, 49(1), 1–8 CrossRef CAS PubMed.
- S. T. Withers and J. D. Keasling, Biosynthesis and Engineering of Isoprenoid Small Molecules, Appl. Microbiol. Biotechnol., 2007, 73(5), 980–990 CrossRef CAS PubMed.
- S. Uribe, J. Ramirez and A. Peña, Effects of Beta-Pinene on Yeast Membrane Functions, J. Bacteriol., 1985, 161(3), 1195–1200 CrossRef CAS.
- T. C. R. Brennan, C. D. Turner, J. O. Krömer and L. K. Nielsen, Alleviating Monoterpene Toxicity Using a Two-Phase Extractive Fermentation for the Bioproduction of Jet Fuel Mixtures in Saccharomyces Cerevisiae, Biotechnol. Bioeng., 2012, 109(10), 2513–2522 CrossRef CAS PubMed.
-
A. Komesu, J. Oliveira, D. K. T. Moreira, A. H. Khalid, J. Moreira Neto and L. H. da Silva Martins, Biorefinery Approach for Production of Some High-Value Chemicals, in Advanced Biofuel Technologies, ed. D. Tuli, S. Kasture and A. Kuila, Elsevier, 2022, ch. 16, pp. 409–429 Search PubMed.
- A. Pellis, S. Cantone, C. Ebert and L. Gardossi, Evolving Biocatalysis to Meet Bioeconomy Challenges and Opportunities, New Biotechnol., 2018, 40, 154–169 CrossRef CAS PubMed.
- L.-Z. Chen, S.-L. Huang, J. Hou, X.-P. Guo, F.-S. Wang and J.-Z. Sheng, Cell-Based and Cell-Free Biocatalysis for the Production of D-Glucaric Acid, Biotechnol. Biofuels, 2020, 13(1), 203 CrossRef CAS PubMed.
-
A. Cornish-Bowden, Fundamentals of Enzyme Kinetics, Wiley-Blackwell, Weinheim, Germany, 4th edn, 2012 Search PubMed.
- M. A. Chow, K. E. McElroy, K. D. Corbett, J. M. Berger and J. F. Kirsch, Narrowing Substrate Specificity in a Directly Evolved Enzyme:
The A293D Mutant of Aspartate Aminotransferase, Biochemistry, 2004, 43(40), 12780–12787 CrossRef CAS PubMed.
- N. Gupta, F. S. Lee and E. T. Farinas, Laboratory Evolution of Laccase for Substrate Specificity, J. Mol. Catal. B: Enzym., 2010, 62(3), 230–234 CrossRef CAS.
- E. O'Reilly, C. Iglesias and N. J. Turner, Monoamine Oxidase–ω-Transaminase Cascade for the Deracemisation and Dealkylation of Amines, ChemCatChem, 2014, 6(4), 992–995 CrossRef.
- S. P. France, L. J. Hepworth, N. J. Turner and S. L. Flitsch, Constructing Biocatalytic Cascades: In Vitro and in Vivo Approaches to de Novo Multi-Enzyme Pathways, ACS Catal., 2017, 7(1), 710–724 CrossRef CAS.
- R. Siedentop, C. Claaßen, D. Rother, S. Lütz and K. Rosenthal, Getting the Most Out of Enzyme Cascades: Strategies to Optimize In Vitro Multi-Enzymatic Reactions, Catalysts, 2021, 11(10), 1183 CrossRef CAS.
- B. Hauer, Embracing Nature's Catalysts: A Viewpoint on the Future of Biocatalysis, ACS Catal., 2020, 10(15), 8418–8427 CrossRef CAS.
- E. Appleton, C. Madsen, N. Roehner and D. Densmore, Design Automation in Synthetic Biology, Cold Spring Harb. Perspect. Biol., 2017, 9(4), a023978 CrossRef.
- A. Casini, F. Y. Chang, R. Eluere, A. M. King, E. M. Young, Q. M. Dudley, A. Karim, K. Pratt, C. Bristol, A. Forget, A. Ghodasara, R. Warden-Rothman, R. Gan, A. Cristofaro, A. E. Borujeni, M. H. Ryu, J. Li, Y. C. Kwon, H. Wang, E. Tatsis, C. Rodriguez-Lopez, S. O'Connor, M. H. Medema, M. A. Fischbach, M. C. Jewett, C. Voigt and D. B. Gordon, A Pressure Test to Make 10 Molecules in 90 Days: External Evaluation of Methods to Engineer Biology, J. Am. Chem. Soc., 2018, 140(12), 4302–4316 CrossRef CAS PubMed.
- B. Henrissat, A Classification of Glycosyl Hydrolases Based on Amino Acid Sequence Similarities, Biochem. J., 1991, 280(2), 309–316 CrossRef CAS PubMed.
- W. Helbert, L. Poulet, S. Drouillard, S. Mathieu, M. Loiodice, M. Couturier, V. Lombard, N. Terrapon, J. Turchetto, R. Vincentelli and B. Henrissat, Discovery of Novel Carbohydrate-Active Enzymes Through the Rational Exploration of the Protein Sequences Space, Proc. Natl. Acad. Sci. U. S. A., 2019, 116(13), 6063–6068 CrossRef CAS.
- M. Couturier, M. Touvrey-Loiodice, N. Terrapon, E. Drula, L. Buon, C. Chirat, B. Henrissat and W. Helbert, Functional Exploration of the Glycoside Hydrolase Family GH113, PLoS One, 2022, 17(4), e0267509 CrossRef CAS PubMed.
- R. Chowdhury and C. D. Maranas, From Directed Evolution to Computational Enzyme Engineering-A review, AIChE J., 2020, 66, e16847 CrossRef CAS.
- C. Garcia-Galan, A. Berenguer-Murcia, R. Fernandez-Lafuente and R. C. Rodrigues, Potential of Different Enzyme Immobilization Strategies to Improve Enzyme Performance, Adv. Synth. Catal., 2011, 353(16), 2885–2904 CrossRef CAS.
- N. E. Labrou, Random Mutagenesis Methods for In Vitro Directed Enzyme Evolution, Curr. Protein Pept. Sci., 2010, 11(1), 91–100 CrossRef CAS PubMed.
- X. Chen, C. Zhang, R. Zou, K. Zhou, G. Stephanopoulos and H. P. Too, Statistical Experimental Design Guided Optimization of a One-Pot Biphasic Multienzyme Total Synthesis of Amorpha-4,11-diene, PLoS One, 2013, 8(11), e79650 CrossRef PubMed.
- J. N. Vranish, M. G. Ancona, S. A. Walper and I. L. Medintz, Pursuing the Promise of Enzymatic Enhancement with Nanoparticle Assemblies, Langmuir, 2018, 34(9), 2901–2925 CrossRef CAS PubMed.
- B. P. Dwivedee, S. Soni, M. Sharma, J. Bhaumik, J. K. Laha and U. C. Banerjee, Promiscuity of Lipase-Catalyzed Reactions for Organic Synthesis: A Recent Update, ChemistrySelect, 2018, 3(9), 2441–2466 CrossRef CAS.
- A. Kumar, K. Dhar, S. S. Kanwar and P. K. Arora, Lipase Catalysis in Organic Solvents: Advantages and Applications, Biol. Proc. Online, 2016, 18, 2 CrossRef PubMed.
- M. Kapoor and M. N. Gupta, Lipase Promiscuity and its Biochemical Applications, Process Biochem., 2012, 47(4), 555–569 CrossRef CAS.
- D. W. Anderson, F. Baier, G. Yang and N. Tokuriki, The Adaptive Landscape of a Metallo-Enzyme is Shaped by Environment-Dependent Epistasis, Nat. Commun., 2021, 12(1), 3867 CrossRef CAS PubMed.
- M. I. Buchholz-Afari, A. Halalipour, D. Y. Yang and J. I. Reyes-De-Corcuera, Increased Stability of Alcohol Oxidase Under High Hydrostatic Pressure, J. Food Eng., 2019, 246, 95–101 CrossRef CAS.
- D. Y. Yang and J. I. Reyes-De-Corcuera, Increased Activity of Alcohol Oxidase at High Hydrostatic Pressure, Enzyme Microb. Technol., 2021, 145, 109751 CrossRef CAS PubMed.
- D. Y. Yang, H. E. Olstad and J. I. Reyes-De-Corcuera, Increased Thermal Stability of a Glucose Oxidase Biosensor Under High Hydrostatic Pressure, Enzyme Microb. Technol., 2020, 134, 109486 CrossRef CAS PubMed.
- S. Lutz, J. Lichter and L. F. Liu, Exploiting Temperature-Dependent Substrate Promiscuity for Nucleoside Analogue Activation by Thymidine Kinase from Thermotoga Maritima, J. Am. Chem. Soc., 2007, 129(28), 8714–8715 CrossRef CAS.
- M. Ghadermarzi and A. A. Moosavi-Movahedi, The Effects of Temperature and pH on the Kinetics of Reactions Between Catalase and its Suicide Substrate Hydrogen Peroxide, Italian J. Biochem., 1997, 46(4), 197–205 CAS.
- F. Terradas, M. Testonhenry, P. A. Fitzpatrick and A. M. Klibanov, Marked Dependence of Enzyme Prochiral Selectivity on the Solvent, J. Am. Chem. Soc., 1993, 115(2), 390–396 CrossRef CAS.
- F. Abe, H. Minegishi, T. Miura, T. Nagahama, R. Usami and K. Horikoshi, Characterization of Cold- and High-Pressure-Active Polygalacturonases From a Deep-Sea Yeast, Cryptococcus Liquefaciens Strain N6, Biosci. Biotechnol. Biochem., 2006, 70(1), 296–299 CrossRef CAS.
- J. Hitomi, J. S. Park, M. Nishiyama, S. Horinouchi and T. Beppu, Substrate-Dependent Change in the pH-Activity Profile of Alkaline Endo-1,4-Beta-Glucanase from an Alkaline bacillus Sp, J. Biochem., 1994, 116(3), 554–559 CrossRef CAS PubMed.
- S. P. Galai, A. de los Ríos, F. J. Hernández-Fernández, S. Haj Kacem and F. Tomas-Alonso, Over-Activity and Stability of Laccase Using Ionic Liquids: Screening and Application in Dye Decolorization, RSC Adv., 2015, 5(21), 16173–16189 RSC.
- O. Barbosa, C. Ortiz, A. Berenguer-Murcia, R. Torres, R. C. Rodrigues and R. Fernandez-Lafuente, Strategies for the One-Step Immobilization-Purification of Enzymes as Industrial Biocatalysts, Biotechnol. Adv., 2015, 33(5), 435–456 CrossRef CAS PubMed.
- G. A. Ellis, S. A. Díaz and I. L. Medintz, Enhancing Enzymatic Performance with Nanoparticle Immobilization: Improved Analytical and Control Capability for Synthetic Biochemistry, Curr. Opin. Biotechnol., 2021, 71, 77–90 CrossRef CAS PubMed.
- G. A. Ellis, S. N. Dean, S. A. Walper and I. L. Medintz, Quantum Dots and Gold Nanoparticles as Scaffolds for Enzymatic Enhancement: Recent Advances and the Influence of Nanoparticle Size, Catalysts, 2020, 10(1), 83 CrossRef CAS.
- J. Mehta, N. Bhardwaj, S. K. Bhardwaj, K.-H. Kim and A. Deep, Recent Advances in Enzyme Immobilization Techniques: Metal-Organic Frameworks as Novel Substrates, Coord. Chem. Rev., 2016, 322, 30–40 CrossRef CAS.
- M. Bilal and H. M. N. Iqbal, Naturally-Derived Biopolymers: Potential Platforms for Enzyme Immobilization, Int. J. Biol. Macromol., 2019, 130, 462–482 CrossRef CAS PubMed.
- J. C. Breger, M. G. Ancona, S. A. Walper, E. Oh, K. Susumu, M. H. Stewart, J. R. Deschamps and I. L. Medintz, Understanding How Nanoparticle Attachment Enhances Phosphotriesterase Kinetic Efficiency, ACS Nano, 2015, 9(8), 8491–8503 CrossRef CAS PubMed.
- J. C. Breger, E. Oh, K. Susumu, W. P. Klein, S. A. Walper, M. G. Ancona and I. L. Medintz, Nanoparticle Size Influences Localized Enzymatic Enhancement—A Case Study with Phosphotriesterase, Bioconjugate Chem., 2019, 30(7), 2060–2074 CrossRef CAS PubMed.
- J. N. Vranish, M. G. Ancona, E. Oh, K. Susumu, G. Lasarte Aragonés, J. C. Breger, S. A. Walper and I. L. Medintz, Enhancing Coupled Enzymatic Activity by Colocalization on Nanoparticle Surfaces: Kinetic Evidence for Directed Channeling of Intermediates, ACS Nano, 2018, 12(8), 7911–7926 CrossRef CAS.
- S. A. Díaz, P. Choo, E. Oh, K. Susumu, W. P. Klein, S. A. Walper, D. A. Hastman, T. W. Odom and I. L. Medintz, Gold Nanoparticle Templating Increases the Catalytic Rate of an Amylase, Maltase, and Glucokinase Multienzyme Cascade through
Substrate Channeling Independent of Surface Curvature, ACS Catal., 2021, 11(2), 627–638 CrossRef.
- W. P. Klein, R. P. Thomsen, K. B. Turner, S. A. Walper, J. Vranish, J. Kjems, M. G. Ancona and I. L. Medintz, Enhanced Catalysis from Multienzyme Cascades Assembled on a DNA Origami Triangle, ACS Nano, 2019, 13(12), 13677–13689 CrossRef CAS PubMed.
- M. D. Aitken and P. E. Heck, Turnover Capacity of Coprinus Cinereus Peroxidase for Phenol and Monosubstituted Phenols, Biotechnol. Prog., 1998, 14(3), 487–492 CrossRef CAS PubMed.
- H. Murata, C. S. Cummings, R. R. Koepsel and A. J. Russell, Polymer-Based Protein Engineering Can Rationally Tune Enzyme Activity, pH-Dependence, and Stability, Biomacromolecules, 2013, 14(6), 1919–1926 CrossRef CAS PubMed.
- C. M. Silveira, S. Besson, I. Moura, J. J. G. Moura and M. G. Almeida, Measuring the Cytochrome c Nitrite Reductase Activity-Practical Considerations on the Enzyme Assays, Bioinorg. Chem. Appl., 2010, 2010 Search PubMed.
- E. Noor, A. Bar-Even, A. Flamholz, Y. Lubling, D. Davidi and R. Milo, An Integrated Open Framework for Thermodynamics of Reactions that Combines Accuracy and Coverage, Bioinformatics, 2012, 28(15), 2037–2044 CrossRef CAS PubMed.
- L. Howes, Enzymes Enter Automated Synthesis-Planning Picture, Chem. Eng. News, 2022, 100, 11 CrossRef.
- W. Finnigan, L. J. Hepworth, S. L. Flitsch and N. J. Turner, RetroBioCat as a Computer-Aided Synthesis Planning Tool for Biocatalytic Reactions and Cascades, Nat. Catal., 2021, 4(2), 98–104 CrossRef CAS.
- D. Probst, Y. Manica, Y. G. N. Teukam, A. Castrogiovanni, F. Paratore and T. Laino, Biocatalysed Synthesis Planning Using Data-Driven Learning, Nat. Commun., 2022, 13, 964 CrossRef CAS PubMed.
- N. Losada-Garcia, Z. Cabrera, P. Urrutia, C. Garcia-Sanz, A. Andreu and J. M. Palomo, Recent Advances in Enzymatic and Chemoenzymatic Cascade Processes, Catalysts, 2020, 10(11), 1258 CrossRef CAS.
- M. Romero-Fernández and F. Paradisi, Protein Immobilization Technology for Flow Biocatalysis, Curr. Opin. Chem. Biol., 2020, 55, 1–8 CrossRef.
- L. Tamborini, P. Fernandes, F. Paradisi and F. Molinari, Flow Bioreactors as Complementary Tools for Biocatalytic Process Intensification, Trends Biotechnol., 2018, 36(1), 73–88 CrossRef CAS PubMed.
- A. I. Benítez-Mateos, M. L. Contente, D. Roura Padrosa and F. Paradisi, Flow Biocatalysis 101: Design, Development and Applications, React. Chem. Eng., 2021, 6(4), 599–611 RSC.
- E. Callaway, DeepMind's AI Predicts Structures for a Vast Trove of Proteins, Nature, 2021, 595, 635 CrossRef CAS.
|
This journal is © The Royal Society of Chemistry 2022 |
Click here to see how this site uses Cookies. View our privacy policy here.