DOI:
10.1039/D2CP02827A
(Perspective)
Phys. Chem. Chem. Phys., 2022,
24, 28700-28781
DFT exchange: sharing perspectives on the workhorse of quantum chemistry and materials science
Received
22nd June 2022
, Accepted 9th August 2022
First published on 10th August 2022
Abstract
In this paper, the history, present status, and future of density-functional theory (DFT) is informally reviewed and discussed by 70 workers in the field, including molecular scientists, materials scientists, method developers and practitioners. The format of the paper is that of a roundtable discussion, in which the participants express and exchange views on DFT in the form of 302 individual contributions, formulated as responses to a preset list of 26 questions. Supported by a bibliography of 777 entries, the paper represents a broad snapshot of DFT, anno 2022.
1 Introduction
What is the status of DFT? Where is DFT heading? What are the important new developments in DFT and what are the points of contention? What is DFT?
Such questions are discussed whenever developers and users of density-functional theory (DFT) meet – in conferences and workshops, during coffee breaks and over dinners. We do not expect short, clear answers to such questions but the discussions and conversations they give rise to are often informative and entertaining – and different from discussions in publications and presentations. We learn about new ideas and developments and about failed attempts – a casual remark may trigger new research or lead to new collaborations. These discussions are an important reason for travelling to conferences and something we have missed during the pandemic.
This article is an attempt to bring such discussions to the printed format – to let prominent workers in the field exchange views and thoughts about DFT in an open informal manner, mimicking the format of a roundtable discussion, but backing up their statements by arguments and references to the literature. The end result should be a lively guide to DFT and its development.
The format of the present article is an unusual one, resembling most closely the Faraday Discussions but not anchored to the talks presented at a conference. It is to our knowledge the first paper of its kind in PCCP and the first such paper on DFT. Given its unusual format, we here describe how it came about.
The initiative for the article was taken by three of the authors, Andy Teale, Trygve Helgaker, and Andreas Savin. Having received a go-ahead for the project from the publisher, the three initiators compiled an initial list of questions about DFT and some tentative answers. A letter of invitation was then sent out to about hundred workers in the field, inviting them “to participate in what will hopefully be an open, thought provoking and informal discussion about density-functional theory and its applications”. To clarify the format of the article, the invitation contained a link to the document with the preliminary questions and answers. A total of 67 accepted the invitation, bringing the number of authors to 70.
In a process involving all authors, the preliminary questions were revised and preliminary answers removed. A final set of 26 questions was agreed upon: five questions for DFT, nine for Density-Functional Approximations (DFAs), eight for The Future of DFT and DFAs, and four for Communicating and Sharing Our Results.
All authors were then invited by the initiators to contribute to the discussion by providing answers to the questions and also comments to answers over a six-week period, encouraging discussions among the authors. Guidelines were provided to ensure a smooth collaborative process. The end result was an extensive first draft of the manuscript, running over sixty pages and with several hundred references. After a two-week internal review involving all authors, an additional two weeks were allotted for responses to the internal review. The purpose of the internal review was solely to improve clarity of expression – not to restrict in any way the freedom of the authors to express their opinions.
The final draft was edited by the three initiators, with the aim of improving the organization of the manuscript by reordering contributions and comments, reducing, where possible, repetition and ensuring a certain level of uniformity in notation and clarity of presentation. However, to retain the spontaneity of the discussion and reflect the multitude of views presented, reorganization was kept to a minimum. As a consequence, some themes may be revisited in different contexts throughout the paper – much as would happen in a lively roundtable discussion.
Having received a final go-ahead from all co-authors, the final manuscript was submitted to the journal. All work on the paper was carried out with LaTeX, using the Overleaf platform1 for ease of collaboration.
The final manuscript provides an interesting snapshot of where DFT stands today and where it is moving. It covers much of DFT with an extensive bibliography, but coverage is nevertheless not exhaustive – classical DFT and multicomponent DFT are not discussed, for example. The topics covered in the paper reflect the interests of the authors. Also, the views stated are those of the individual authors – as such, the paper has no conclusion. In the spirit of the paper, you are instead encouraged to continue this exchange of views, by contacting the authors.
2 Density-functional theory
2.1 What is DFT?
2.1.1 Savin.
Density-functional theory (DFT) is more than existence theorems. I like to make the distinction between
(1) a density functional, a number obtained from the density;
(2) DFT, the collection of theorems useful for obtaining exact results with procedures using density functionals, without having to solve the exact many-body problem;
(3) the methods using them – for example, the Kohn–Sham method; and
(4) density-functional approximations (DFAs), the approximations (or models). The latter can originate from a choice of a “closed form”, as mentioned in contribution (2.1.4), or from controllable ones, as related to the numerical treatment and discussed in contribution (4.6.7).
2.1.2 Levy.
Federico Zahariev and I have recently shown in ref. 2 that it is useful and variationally valid to employ spin-free wave functions in the constrained-search formulation when deriving certain properties of a functional for the purpose of its approximation.
In the constrained-search formulation of pure-state (or ensemble) DFT, the kinetic plus electron–electron repulsion energy of a density is the expectation value of the wave function (or ensemble) that yields this density and minimizes the kinetic plus electron–electron repulsion expectation value. That is,
|  | (1) |
where, with the use of pure-state wave functions,
|  | (2) |
The wave functions are here spin-free, but antisymmetric in the first
M spatial coordinates and separately antisymmetric in last (
N −
M) spatial coordinates. The generalization of
F[
ρ] to ensembles should be clear. This generalization ensures convexity.
2.1.3 Reining.
One may distinguish different possible aspects in this question: What is the message of DFT? Why has it been successful? How is it used today? What distinguishes it from other theories that deal with the many-body problem? Some are treated later, so I think we should focus on the first aspect here. I also think that, in answering this and many other questions, a glance at other possible theoretical approaches is healthy, because we always learn from comparison, so let us try to have such a point of view whenever possible.
The term DFT expresses the fact that observables in the ground state at zero temperature can be considered as functionals of the ground-state density. This can then be extended to thermal equilibrium, etc., as others point out. So, it means that the density is a sufficient descriptor. It is important to say “can be considered as a functional of the density” and not “is a functional of the density”, because this is a choice: observables can also be considered as functionals of the many-body ground-state wave function, or the one-body Green's function, or many other possible choices. The functional of the many-body ground-state wave function is very simple (whereas the wave function is not, of course), and a density functional will in most cases be exceedingly complicated (whereas the density is simple). Actually, I chose to say “can be considered as”, because this does not imply that there must be an explicit expression.
A second important point: the density is not known a priori but is needed as input to evaluate our density functionals for a given system and observable. So, as a second aspect of DFT, we also have to invoke the variational character of the energy as functional of the density, because it allows us to find the density that is needed to evaluate the functionals for the various observables, without calculating the density from the many-body wave function. Otherwise, DFT could probably not compete with other approaches, not even as an idea – for example, also the external potential is a sufficient descriptor (for given particle number or chemical potential), it is simple, and it has the advantage that we (think we) know it. The variational character also has the benefit that a slightly wrong density may still lead to a reasonable energy (whereas this may not hold for other observables).
So, we may consider DFT as one possibility: one possible way to formulate the calculation of observables in a many-body system. There are many such ways, and we know that for most systems we will never be able to obtain the exact answer. Therefore, once we agree that those various ways are in principle exact, the true question is: how suitable are they as starting points for approximations? And so, for our purpose here: in which way is DFT a good starting point for approximations?
2.1.4 Scheffler.
Since the development of the quantum mechanics of atoms and polyatomic systems, it was clear that inspection of the ground-state electron density ρ(r) provides the information on the total number of electrons, N, the positions of the atoms, {RI}, and from ρ(RI) the nuclear charges.3,4 Thus, ρ(r) determines N, {RI}, {ZI} – that is, the many-electron Hamiltonian, and therefore, it determines everything. This is the algorithm that defines how to go from the ground-state density to the energy.
The theorem of Hohenberg and Kohn5 and the works by Levy6,7 and Lieb8 are beautiful mathematical treatments. Importantly, the basic concept that the ground-state electron density determines everything often enables decisive physical insight. The often misleading assumption is that the above laid out, exact algorithm “ρ(r)
ground-state energy (and even everything)” can be expressed in terms of a closed mathematical expression. Approximating the algorithm by a mathematical functional, i.e., by a DFA, suffers from the severe problem that the range of validity of this functional is typically unclear: We can test its accuracy only by comparing results with experiments or high-level wave-function theories. We trust the reliability for systems that we believe (!) are “similar” to the tested ones, but we don’t know about the accuracy for untested systems. And the term “similar” is not even defined.
Let me add: I am not aware of a proof that the exact exchange–correlation-functional exists, beyond the noted algorithm which requires to solve the many-body Schrödinger equation. However, and most importantly, the works by Hohenberg and Kohn and Kohn and Sham have shown the way to develop density-functional approximations which revolutionized the description and understanding of polyatomic systems.
2.1.5 Kvaal.
I agree with Savin in contribution (2.1.1) – in particular with respect to the claim that a distinction between exact DFT and approximate DFT is useful. In my opinion, they are both conceptually and mathematically different. They share the use of the density and potential as dual basic variables, but otherwise the similarities disappear for me. For instance, a DFA will have much nicer mathematical properties than the exact universal functional, as they are built from simple, explicit ingredients, at least partially necessitated by the need for efficient numerical evaluation and optimization in order to be useful. On the other hand, the exact universal density functional has a complicated implicit definition, leading to a highly complicated functional. A concrete formulation of this is due to Schuch and Verstraete,9 who demonstrated that, if an efficient evaluation of the universal functional could be done, all NP hard problems would be solvable in polynomial time. This is highly unlikely. On the other hand, DFAs are necessarily computable! (It is of course one of the marvels of DFT, that it is even possible to obtain such good results with so little computational effort.)
Thus, approximate and exact density-functionals are mathematically quite different. The noncomputability of the exact functional indicates that systematically improvable DFAs are probably possible, in the sense of mathematical a priori error estimation – that is, mathematical statements towards an approximation's accuracy in terms of its adjustable parameters, such as basis size. Therefore, I would like to go out on a limb and say that approximate density functionals are not really approximations to exact density functionals. They are instead largely independent and, to a variable extent, semiempirical models that have the common use of the density as a basic variable as a characteristic. The latter aspect is for me an answer to the question “What is DFT?”
2.1.6 Savin.
Let me comment on the difficulty of obtaining exact functionals in a (semi)local form by choosing a simpler example. The Hartree density functional, |  | (3) |
is universal, and not only known but also simple. However, I don’t see how to replace it by a (semi)local form.† One can argue that this does not lead to problems, as we compute EH explicitly. However, this argument is not valid if we choose to express the exchange functional, Ex, in a (semi)local form: for one-electron systems, Ex = −EH.
2.1.7 Yang.
I agree with Savin on the difficulty of semilocal functionals. The example of the interaction energy of a one-electron system is a clear case: the exact exchange–correlation energy has to cancel the classical Coulomb energy.10 Otherwise, the functional has a self-interaction error (SIE).
For many years, the SIE had been assumed to be the main systematic error in DFAs, related to the incorrect dissociation of molecular ions, the underestimation of chemical reaction barriers and band gaps of molecules and bulk materials, the overestimation of polymer polarizability, and many other failure of commonly used DFAs.11,12 However, the development of two SIE-free functionals, the Becke0513 and the MCY214 functionals, changed the understanding.15 While these two exchange–correlation functionals, nonlocal and also nonsemilocal, are SIE-free by construction for any one-electron system and perform as well on thermodynamics benchmarks as hybrid functionals, they still retain significant errors in the dissociation of molecular ions, band gaps of molecules, and polymer polarizability problems, much like the hybrid functional B3LYP. The only significant improvement observed is in the prediction of reaction barriers. Thus the systematic error is clearly not the SIE.
To describe the systematic error of DFAs, the concept of the delocalization error has been developed, and it can be understood from the perspective of fractional charges.16,17 For systems of small or moderate physical sizes, conventional DFAs usually have good accuracy in total energies for an integer number of electrons. For a fractional number of electrons, conventional DFAs, however, violate the Perdew–Parr–Levy–Balduz (PPLB) linearity condition,18–20 which states that the exact ground-state energy E(N) is a linear function of the fractional electron numbers connecting adjacent integer points. Inconsistent with the requirement of the PPLB linearity condition, E(N) curves from conventional DFAs are usually convex, with drastic underestimation to the ground-state energies of fractional systems. The convex deviation of conventional DFAs decreases when the systems become larger and vanishes at the bulk limit. However, the delocalization error is exhibited in another way, in which the error manifests itself in too low relative ground-state energies of ionized systems and incorrect linear E(N) curves with wrong slopes at the bulk limit.16,17,21
To reduce or eliminate the delocalization error, enormous efforts have been devoted to the development of new exchange–correlation functionals. None of these developments are based on a semilocal form. All have nonlocal features in the functionals – see the development of the scaling approaches.22–25
In addition to the delocalization error characterized by fractional charges, commonly used DFAs also have a significant systematic static correlation error characterized by the violation of the constancy conditions on fractional spins.17,20,26 The combination of the exact fractional charge condition18 and the exact fractional spin condition20,26 leads to the general flat-plane condition,27 the satisfaction of which is a necessary condition for describing the band gap of strongly correlated Mott insulators. The flat-plane condition also leads to the conclusion that the exact exchange–correlation functional cannot be a continuous functional of the electron density or the density matrix of the noninteracting reference system everywhere.27 To reduce or eliminate the static correlation error, one has to use nonlocal functionals.28
2.1.8 Savin.
Warren Pickett said during a talk (Brisbane, 1996): “True, the density gives the potential, and this makes the Hohenberg–Kohn theorem sound so empty, because the potential, we know it anyhow”. We do not need to start with an unknown function, ρ(r), when it is equivalent to using a known function of the position r – namely, the external potential, v(r).
2.1.9 Trickey.
The Pickett remark quoted by Savin is a paraphrase of the analysis that Per-Olov Löwdin had attributed earlier to E. Bright Wilson.29 The density cusps tell you the nuclear charges, hence the external potential v, hence the Hamiltonian. Also see Krylov's contribution (2.1.22) below.
2.1.10 Yang.
The Hohenberg–Kohn work established the principles for describing a many-electron system from the reduced variable of its electron density and the Kohn–Sham work provided the formulation to use a noninteracting reference system to represent the electron density of a many-electron system. These works are the solid foundation of DFT. However, they do not lead to any systematic pathway to the approximation of the density functional; see contribution (2.1.8). The specific approximations for the density functionals are the key to all applications.
2.1.11 Helgaker.
I suppose the nontrivial result is that (for a given number of electrons) the potential and density are dual variables – what you can calculate from one, you can calculate from the other. In particular, we can calculate the energy directly from the density, bypassing the potential.
2.1.12 Yang.
Indeed, the dual formulation of DFT is the potential-functional theory (PFT).30 PFT establishes two results: the dual of the Hohenberg–Kohn theorem in terms of the external potential as the basic variable and the dual of the Kohn–Sham theorem in terms of the potential of the noninteracting reference system. The first result provides a solution to the v-representability problem in the original Hohenberg–Kohn work. The second result provides the theoretical foundation for the optimized-effective-potential approach for Kohn–Sham calculations with functionals of orbitals.
2.1.13 Helgaker.
I like to think of DFT in terms of Legendre–Fenchel transforms.8,31 In short, from the concavity and continuity of the ground-state energy
as a function of the external potential v ∈ L3/2(
3) + L∞(
3) follows the existence of a universal density functional
as a function of the electron density ρ ∈ L3(
3) ∩ L1(
3) such that5,8 |  | (4) |
|  | (5) |
where
. Since E and F can be calculated from each other, they contain the same information, only expressed in different ways. However, although the Lieb variation principle is a powerful tool for analysis and method development, it is not a practical tool for computation. Instead, the power of DFT derives from Kohn–Sham theory, making it possible to approximate F[ρ] (sufficiently) accurately and inexpensively for densities ρ of interest to us by introducing orbitals.
2.1.14 Levy.
In contribution (2.1.13), Helgaker states that he prefers the Legendre-transform formulation. However, it has been shown that the Legendre-transform formulation is equivalent to the ensemble constrained search.8
2.1.15 Helgaker.
It is of course correct that the ensemble constrained-search functional is identical to Lieb's functional. With respect to the different formulations of DFT, my view is the following.
The Hohenberg–Kohn theorem,5 often thought of as the cornerstone of DFT, is easy to prove (apart from some subtleties) but perhaps not so easy to understand intuitively. Hohenberg and Kohn's original formulation of DFT is therefore not only restrictive in scope (in that it assumes v-representability) but may also appear a little mysterious.
Levy's constrained-search formulation6 took the mystery out of DFT and brought clarity and generality to the field – a major step forward, indeed. Lieb's convex formulation,8 on the other hand, gave DFT beauty and elegance by identifying the density functional with the Legendre transform (convex conjugate) of the ground-state energy, thereby placing DFT in a broader mathematical framework.32
It is an important and nontrivial result in DFT that the ensemble constrained-search functional and the Legendre-transform functional are the same – they are merely complementary formulations of the same thing.8 Together, they constitute the solid foundation of DFT.
2.1.16 Scheffler.
I somehow disagree with the last sentence of contribution (2.1.13). Clearly, Kohn–Sham theory has provided us with significant understanding, for polyatomic systems, mostly for cases where the physics is largely governed by the independent-particle kinetic-energy operator (or its orbitals). However, in general, I would hesitate to call Kohn–Sham theory together with the known DFAs “(sufficiently) accurate”. A key scientific problem is that the range of validity of the known DFAs is unknown, and a reliable estimate of the accuracy and a systematic convergence of the accuracy are not possible. Our own pragmatic approach is to perform calculations with different DFAs, and if the results are similar, we tend to accept them. Otherwise, we are worried. And, if possible, we check final results by a higher-level theory – by, for example, coupled-cluster theory.
2.1.17 Kvaal.
It is interesting to note that Lieb's convex formulation of exact DFT, the essence of which is succinctly described in contribution (2.1.13), does not rely in any way on the classical Hohenberg–Kohn theorems to establish duality of ρ and v. Neither are the theorems necessary for the derivation of exact Kohn–Sham theory. While the original Hohenberg–Kohn theorems are now established rigorously, albeit with mild assumptions on the potential,33 it is my opinion much easier to say that the Legendre transform of E[v] is the essence and foundation of DFT, from both a mathematical and a physical point of view. Lammert has pointed out that the Hohenberg–Kohn density-potential correspondence map is quite ill-behaved.34 Nearby v-representable densities may have wildly different potentials, and thus fundamental arguments that rely on, for example, some kind of differentiation of v as a function of ρ are not useful, at least for exact DFT.34
2.1.18 Laestadius.
With recent development of unique-continuation from sets of measure zero, in particular by Garrigue,35 I regard the Hohenberg–Kohn theorem as rigorous, albeit with some limitations. In particular, certain Lp spaces need to be considered for the potentials – for example, Theorem 30 in ref. 33 is a Hohenberg–Kohn result with all previous gaps filled, although it is not given for L3/2 + L∞.
Furthermore, comparing the situation with paramagnetic-current DFT, where the lack of a (corresponding) Hohenberg–Kohn theorem has been established by Capelle and Vignale,36 it is striking that although (ρ,jp) determines the nondegenerate ground state, if degeneracies are allowed, then the level of degeneracy is not determined.37 A given (ρ,jp) can therefore be associated with two different Hamiltonians (in fact, infinitely many) that may have different numbers of degenerate ground states. (Of course, this doesn’t stop the constrained search, which remains well defined.) In DFT, the extra layer of a Hohenberg–Kohn theorem (not just the first part of a constrained search) rules out such situations. I view the Hohenberg–Kohn theorem as a gold reserve – it is perhaps unexciting and just sits in the vault but is, on the other hand, good to have in certain extreme situations.
2.1.19 Helgaker.
Regarding the Hohenberg–Kohn theorem in DFT, it is interesting to see what role it plays within the Legendre–Fenchel formulation of DFT. The condition for a minimizing density in the Hohenberg–Kohn variation principle as given in contribution (2.1.13) is −v ∈ ∂F[ρ] where ∂F[ρ] is the subdifferential of F at ρ – that is, the collection of potentials with ground-state density ρ. Likewise, the condition for a maximizing potential in the Lieb variation principle is ρ ∈ ∂E[v], where the subdifferential of E at v is the collection of all ground-state densities of v. In fact, the two conditions are equivalent: | E[v] = F[ρ] + (v|ρ) ⇔ −v ∈ ∂F[ρ] ⇔ ρ ∈ ∂E[v]. | (6) |
By the Hohenberg–Kohn theorem, the optimality condition of the Hohenberg–Kohn variation principle takes the form |  | (7) |
This uniqueness of the potential (up to an additive constant) is not mission critical for DFT but tells us that there is a unique maximizing potential in the Lieb variation principle (if any).
The optimality conditions in eqn (6) give some additional insight: the ground-state energy E and the universal density functional F are functions whose subdifferential mappings (“functional derivatives”) are each other's inverses. Loosely speaking, therefore, E and F may be obtained from each other by differentiation followed by inversion and integration.
2.1.20 Salahub.
Savins answer in contribution (2.1.1) to “what is DFT?” appeals to me because of its breadth. DFT appeals to different people for different reasons, from the joy of pure theory, to the satisfying hard work of DFAs, to the romp of applications across disciplines (when it works), to the agony when it doesn't (appealing to masochists, but also affording the possibility of looping back for improvements). So “DFT” is like an excellent marketing logo, as recognizable to scientists as the Nike logo is to the general public. Reasons for buying into DFT are numerous and varied, as reflected in the sections of this paper.
2.1.21 Fuentealba.
The first time I heard about DFT was in the eighties in Germany, and people called it “Density Functional Method”, because the theory is the quantum mechanics and one cannot have a theory into another one.
2.1.22 Krylov.
I first learned about the key ideas behind DFT before its modern incarnation was developed. Back in the eighties, chemists were using the Xα method, which was regarded by ab initio theorists as semiempirical and, therefore, inferior to the then gold standard – the full Hartree–Fock method. We were struggling to understand why an inferior method would give more accurate results. I think the real insight was to understand that the Wilson conjecture – the observation that the one-electron density contains all the information needed to reconstruct the many-body Hamiltonian (and, therefore, to find the exact solution of the Schrödinger equation) – provides a physical justification for the existence of a mapping between the density and the exact energy of the system. The Hohenberg–Kohn theorems inform us that this mapping is unique.
With such justification, one can approach the problem of finding this mapping in a completely different way – not by building approximations to the known exact solution (as done in the wave-function theory), but by parameterizing an empirical representation of the mapping device, the functional. Most DFAs are built upon mathematical representations of the functional grounded in our physical understanding of what it should look like (based on exact results for model systems), but one can envision finding the mapping without any such help from physics – for example, by brute-force training of a neural network (machine learning).38 One can, therefore, think of DFT as an empirical method that can be made exact.
While the blind brute-force (e.g., via ML) discovery of the density-energy mapping is, in principle, possible, it has important limitations compared to physically motivated DFAs. First, without any constraints due to physics, such brute-force search is going to be computationally wasteful. Second, having discovered the mapping between energy and density, one still has no recipe for computing energy derivatives with respect to various perturbations (i.e., properties), unless properties (or various energy derivatives) were included in the training. In contrast, using a physically motivated form of the functional opens access to properties (although the quality is not guaranteed, as illustrated by the developments of magnetic DFAs39).
2.1.23 Helgaker.
I am not so fond of the Wilson conjecture – it works only if we already know that the potential is a Coulomb potential. It is a striking observation, but to some extent it trivializes DFT. The Hohenberg–Kohn theorem makes no such assumptions regarding the potential.
2.1.24 Jones.
A fixation on exact energies appears to be so strong among chemists that it justifies any amount of data fitting, so reducing DFT to a “semiempirical” or “empirical” method. With their focus on extended systems, materials scientists know that new knowledge can result from DFT calculations, even if all the calculated energies are wrong. See also contribution (2.2.23).
2.1.25 Ayers.
Arguably, any electronic structure theory method can be reformulated as a DFA by substituting its associated energy functional into the Legendre transform or its associated wave-function ansatz into the constrained search. So Hartree–Fock may be legitimately considered a DFT (a generalized Kohn–Sham DFT). Is Hartree–Fock theory and its analysis therefore DFT? Clearly, many coupled-cluster and propagator methods are also frequently analysed as DFT. I would not like to define DFT as “the sort of stuff that is done by density-functional theorists” but some work that is marketed as DFT (cf. contribution (2.1.20)) is not presented in the context of the mathematical framework of DFT (cf. contribution (2.1.1)).
To me, only orbital-free DFT is unequivocally DFT; everything else can also be fruitfully viewed from an alternative perspective. Indeed, some theoretical approaches and computational methods can legitimately be considered wave-function theories/methods, density-matrix theories/methods, propagator theories/methods, and density-functional theories/methods. I do not wish to take a hard line and proclaim that these types of theories/methods are not DFT because the philosophy (especially the emphasis on explicitly defining and characterizing the functional that is being approximated), traditions (especially the openness to pragmatic parameterization and approximation), and tools of DFT can be useful even for theories/methods that are “not just DFT”. But other, non-DFT, approaches could sometimes be even more useful.
2.1.26 Görling.
While the electron density certainly is a key quantity in DFT, I feel that there is a too strong focus on it – in particular, on the idea of getting the total energy or other information directly from the density. While this is the idea behind certain flavours of orbital-free DFT, it is not the idea behind the most commonly used DFT approaches, which are the Kohn–Sham or generalized Kohn–Sham methods. For these methods, a quite different view on DFT can be taken: to consider the electron density as the quantity that enables one to associate the real electronic system with a model system that has the same ground-state density, which makes it possible to describe the ground-state energy and other properties of the real system via the model system, i.e., via its orbitals and eigenvalues. From the Kohn–Sham orbitals, traditionally, only the ‘noninteracting’ kinetic energy is calculated exactly, while the exchange–correlation energy is approximated by an explicit functional of the density.
But this is just one strategy. It is possible to determine additionally other contributions to the energy from the orbitals – for example, parts of the exchange energy in hybrid methods – or even to calculate all contributions to the energy exactly from the occupied orbitals, except the correlation energy. The latter can then be approximated by orbital-dependent functionals.40 In the latter case, the density is not needed at all in the calculation of the total DFT energy. If, furthermore, the orbitals are obtained via the optimized-effective-potential (OEP) method40–46 or within an appropriate generalized Kohn–Sham approach, then DFT methods results that do not require at any point the calculation of the density. The density is then only required in the underlying formalism.
I feel that the perception of DFT has been somewhat blurred by a questionable statement that, one way or another, is frequently found in textbooks and articles. This is the statement that DFT is distinguished from wave-function methods by using the electron density instead of a wave function to calculate the total energy of an electronic system. This statement is at least misleading if not wrong because most DFT methods used in practice are Kohn–Sham or generalized Kohn–Sham methods, which require orbitals and thus one-electron wave functions to calculate crucial parts of the total energy.
2.1.27 Gidopoulos.
I believe the distinction in the literature between wave-function methods and DFT is slightly different. In my understanding, the distinction is not that in DFT the energy is actually calculated from the density, once we know the density, because the question remains how to find the density. Rather, the distinction is that in DFT the solution to the electronic-structure problem is obtained by minimizing a total energy as a functional of the density, while in wave-function theory the solution is obtained by solving Schrödinger's equation. So, calculating the energy from the density does not mean literally plugging the density into some orbital-free expression, but the process of minimization of the total-energy density functional to obtain the minimum value, which is the total energy of the interacting system.
2.1.28 Chattaraj.
Any theory that applies density to understand a many-particle system, without using the exact wave function, can be termed as DFT.47–49 According to Hohenberg–Kohn theorems,5 DFT is a theory that legitimizes the use of the density to calculate all possible properties. The Hohenberg–Kohn theorems are just existence theorems and do not provide any know-how for an explicit form of the energy as a functional of the density as well as functional forms of other properties.
2.1.29 Trickey.
The foregoing discussion seems a bit parochial – for example, the identification in contribution (2.1.4) of DFT with “ground state”. That restriction seems to have been accepted by subsequent commentators in this section. But there are several instances of what generically is a DFT. There is, for example, a well-developed classical DFT. Closer to the focus of this discussion (many-fermion systems), there is free-energy DFT (also known as finite-temperature DFT).50 It inexorably involves excited states. There has been progress on free-energy DFAs.51–56 Another ensemble DFT is the Gross–Kohn–Oliveira (GOK) approach for excited states at T = 0 K (see other commentators below).
The common theme of these DFTs is the reduction of the inherent complexity of the direct description of a many-body system to the comparative simplicity of functionals of the density – either explicitly, or implicitly in terms of auxiliary functions such as orbitals. The strategy, in the time-independent case at least, is to obtain the relevant physics (hence also chemistry) by an appropriate minimization procedure on a functional of the density itself (whether it be pure-state or ensemble).
2.1.30 Galli.
In the Hohenberg–Kohn formulation, DFT is an exact theory of ground and excited states, entirely based on the electron density. That is, the density determines uniquely the potential, hence both ground and excited state properties of the system may in principle be derived. However there is no practical recipe on how to derive such potential and hence on how to derive neither ground or excited state properties. The Kohn–Sham formulation, in contrast, is applicable only to ground-state properties, although in practice it is applied also to excited states.
2.1.31 Schwerdtfeger.
We should be reminded that the charge density ρ(r, t) is not Lorentz invariant. As relativistic quantum (field) theory demands a fully covariant formalism, we have to use the four-current density jμ as a function of the four-position xμ instead of the charge density, the latter appearing only as the time-like (first) component of the four-vector (ρ, jx/c, jy/c, jz/c), where c is the speed of light in vacuum. The Hohenberg–Kohn theorem has been generalized to the relativistic domain by Rajagopal and Callaway57 and field-theoretical aspects have also been taken into account by Engel.58 Beside this enormous progress on the theoretical side, it is fair to say that applications in this most rigorous relativistic framework using the current-dependent exchange–correlation energy functional are more or less absent.58 The main reason lies, as one can guess, in the fact that relativistic DFT (RDFT) faces exactly the same fundamental problems as DFT in the nonrelativistic domain. As we know, relativistic effects can be very large for electronic properties of compounds containing heavy elements, often larger than the error introduced by many DFAs, thus justifying the introduction of the exchange–correlation functional into the (no-pair) Dirac–Coulomb (DC) equation (the Douglas–Kroll Kohn–Sham scheme) or into its corresponding two-component (such as exact two-component [X2C]) or scalar relativistic schemes, with or without the relativistic pseudopotential approximation. The latter together with DFT is clearly the main workhorse in solid-state physics. One may however, question the inclusion of smaller radiative QED corrections into RDFT as it cannot compete with more accurate wave-function based methods. On the other hand, we should mention that RDFT approximations based on the density ρ and the (noncollinear) magnetization density m59,60 have now become feasible and useful in many applications.
2.1.32 Tellgren.
In my view, a lot of work remains to be done on the theoretical side of RDFT too. Every rigorous formulation of nonrelativistic ground-state DFT depends on the ground state being identified as a global energy minimum. At the relativistic level, an energy minimization principle strong enough to construct a DFT is missing and the present attempts to establish a relativistic Hohenberg–Kohn theorem are not rigorous.
2.1.33 Gritsenko.
DFT can be formally considered as the result of the simplest exact functional closure of the conventional expression for the nonrelativistic ground-state energy E[ρ, γ, P], which includes the electron density ρ, the first-order reduced density matrix (1RDM) γ, and the diagonal part P of the 2RDM Γ corresponding to a ground-state wave function Ψ. This can be achieved in the spirit of the Bogoliubov–Born–Green–Kirkwood–Yvon (BBGKY) chain61 of the quantum dynamical reduced theories of many-electron systems. Truncation of the BBGKY chain with its exact or approximate closure at the mth level produces theories that operate with the mth (and lower) order RDMs.62 In this sense, DFT can be considered as the result of the exact closure at the “zero” (i.e., only density functional) level of E[ρ, γ, P] with two maps, in complete analogy with those employed in the derivation of time-dependent density-matrix-functional theory (TDDMFT).63 The first map is the evident map P ← Γ
γ
ρ, while the second map ρ
Ψ
Γ employs the Hohenberg–Kohn theorem. It is its simplicity and compactness in the BBGKY sense and also its definite connection with a real world via its exactness that make DFT such a fertile ground for the present wealth of DFAs.
This great success of DFT can be favourably compared with a rather tumultuous development of “higher-order” full 1RDM or density-matrix-functional (DMFT) and 2RDM theories, which still do not enjoy a truly successful “take-off”. The ongoing development (see contribution (4.1.1)) explores a way64 in which DFT can help DMFT with such a “take-off”, while DMFT can help DFAs with the problematic inclusion in the latter of nondynamical or strong electron correlation.
2.2 What is Kohn–Sham DFT?
2.2.1 Perdew.
Often we need to predict the ground-state total energy and electron density of a system of real interacting electrons in a scalar external potential (created, for example, by their attraction to nuclei). Correlated wave-function theory provides “the right answer for the right reason”, but at a high computational price for systems of many electrons. Kohn–Sham DFT65 employs a simpler noninteracting or Coulomb-uncorrelated wave function, but includes a density functional for the exchange–correlation energy that is exact in principle but requires improvable approximations in practice. It often provides “almost the right answer for almost the right reason at almost the right price” for real atoms, molecules, and materials. The noninteracting kinetic energy and the electron density are found by the not-so-expensive self-consistent solution of effective one-electron Schrödinger equations. Indeed, the exchange–correlation energy and exchange–correlation potential “exactify” the Hartree approximation for the ground-state energy and density. The generalization from total density to spin density66 provides more information and enhances the accuracy of the approximations.
2.2.2 Gould.
Kohn–Sham DFT65 typically means any DFT approximation that employs a set of one-body orbitals, usually denoted {ϕi}, to produce a kinetic energy functional, Ts[ρ] := Ts[{ϕi}] that approximates the many-body kinetic energy, T[Ψ] = 〈Ψ|T|Ψ〉. Generalized Kohn–Sham DFT incorporates traditional approaches to DFT as well as “hybrid” functionals, which allow for a nonlocal operator treatment of the Hartree–Fock exchange terms.67
As a result, one can replace a many-body interacting Hamiltonian, H, by a simpler-to-evaluate one-body Kohn–Sham effective Hamiltonian:
|  | (8) |
where
vs is an effective one-body potential (or operator potential). The density may then be calculated as
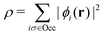
, while the energy is given by
E0[
ρ] =
Ts[
ρ] +
EHxc[
ρ] + (
v|
ρ). We will define
vs and
EHxc below.
Formally, one may define
, where
in the notation defined in contribution (2.1.13).‡ Thus, Ts[ρ] is the lowest kinetic energy of a noninteracting system with density ρ. Kohn–Sham DFT is useful because the Hartree–exchange–correlation (Hxc) energy,
| EHxc[ρ] := F[ρ] − Ts[ρ], | (9) |
is easier to approximate than
F[
ρ]. Here,
EHxc incorporates the energetics of the interacting system, including some kinetic-energy terms. The one-body effective potential that minimizes
E0[
ρ] can be shown to be
vs =
v + δ
EHxc/δ
ρ.
2.2.3 Gritsenko.
A profound physical meaning of the exchange–correlation part of the Kohn–Sham potential vxc is revealed with its partitioning | vxc = holexc + resp | (10) |
into the potential of the exchange–correlation hole
holexc and the response potential
resp. This partitioning emerges from differentiation with respect to ρ of the exchange–correlation energy Exc[ρ] represented via the exchange–correlation pair-correlation function ḡxc, |  | (11) |
where the overbar indicates the coupling strength integrated pair-correlation function. The potential
holexc, the derivative of the ρ functions under the integral, represents the universal interaction (for both occupied and virtual Kohn–Sham orbitals) with the exchange–correlation hole of the unit charge. In turn, the potential
resp, the derivative of the pair-correlation function ḡxc, exhibits the spatial step-like structure, with the individual steps distinguishing various atomic and molecular electron shells.69
2.2.4 Baerends.
The Kohn–Sham method is often cited as the method that made DFT a feasible computational method by offering a decent approximation to (a large part of) the kinetic energy. The latter proved too hard to obtain as a density functional. But more importantly, the Kohn–Sham method has provided DFT with an orbital model. This has greatly facilitated its acceptance in the computational chemistry community. After initial reservations about the Kohn–Sham orbitals (“they are only there to build the density”), it has become evident that these orbitals are not inferior to or more approximate than the Hartree–Fock orbitals, but on the contrary are even more suitable for the qualitative and semiquantitative molecular-orbital (MO) theories of chemistry. If the exact Kohn–Sham orbitals and orbital energies could be obtained, this would be evident. The Kohn–Sham orbitals build the exact electron density, i.e., the exact charge distribution in molecules, so they are perfect for the so-called charge control factor of chemical reactions. The energies of the exact upper valence Kohn–Sham orbitals approximate the first ionization energies exceedingly well: whereas the Hartree–Fock orbital energies, within the frozen orbital approximation for ionization energies (Koopmans’ theorem), deviate typically by more than 1 eV from ionization energies, the exact Kohn–Sham orbital energies have deviations that are typically an order of magnitude smaller.70,71 The virtual orbitals of the Kohn–Sham model are not Koopmans-type approximations to the electron affinities, but the virtual-occupied orbital energy gaps are excellent approximations to excitation energies.72,73 These are properties that have been the basis for the whole edifice of orbital-based explanations in chemistry.
Ultimately, virtually all explanations of chemical behaviour are cast in orbital language, even if the underlying computations are based on the most sophisticated techniques of theoretical chemistry. The ready acceptance of DFT in chemistry has been greatly aided by the availability of the familiar orbital model. As for the old adage that Kohn–Sham orbitals and orbital energies “have no meaning, there is no Koopmans’ theorem like in Hartree–Fock theory”: the opposite is true.70,71
The orbital energies of almost all DFAs do not have the nice properties of the exact Kohn–Sham model, being some 5 eV too high (not negative enough). This is unfortunate and has some adverse consequences, but fortunately the upshift is approximately the same for the upper valence and the lower virtual (valence) orbitals, so the correct relative order is preserved in most DFAs. Nevertheless, more efforts should be made to construct DFAs that obey these exact Kohn–Sham properties (much) more closely.
2.2.5 Krylov.
The orbital picture of Kohn–Sham DFT is indeed of great importance. With the exact functional, the energies of the highest occupied Kohn–Sham orbitals become exact ionization energies (IEs) (as per Janak's theorem). Numerical investigations show that the shapes of the Kohn–Sham orbitals in cases when their IEs are close to the exact IE (such as when tuning the range-separation parameter to make the Koopmans IE match Δ SCF IE) become similar to the shapes of the Dyson orbitals.74,75 Interestingly, the energies of lower-lying Kohn–Sham orbitals provide surprisingly accurate approximations to the exact many-body IEs (when used with appropriate DFAs),76,77 which can be understood by analysing the curvature of the total Kohn–Sham energy with respect to the occupation numbers.76
This endows the theory with the ability to provide physically relevant quantities – for example, Dyson orbitals enter the expressions for photoionization/photodetachment cross-sections and can even be reconstructed from experimental data.74 Moreover, the orbitals provide a link between many-body theories and DFT – for example, one can judge the quality of a particular DFA by how well the shapes and energies of the Kohn–Sham orbitals agree with those from high-level many-body calculations (e.g., equation-of-motion coupled-cluster theory).78 These ideas are already exploited in optimally-tuned range-separated DFAs.76,77 But, perhaps more opportunities exist for using ab initio Dyson orbitals to build better DFAs?
2.2.6 Calaminici and Köster.
To further underline the importance of Kohn–Sham orbitals in chemistry and physics, we mention their interpretative use in cluster science for the definition of so-called superatoms – see, for example, ref. 79 and references therein.
Specifically, the electronic states of small metal clusters are bunched in shells. These shells are experimentally observed in the variations of polarizabilities, ionization energies, and electron affinities – to name a few characteristic observables. Kohn–Sham orbitals, as approximations to Dyson orbitals, reflect these shell structures in a large variety of free and ligand-stabilized clusters. Thus, the now common concept of superatoms in chemistry is based almost exclusively on Kohn–Sham calculations and the corresponding canonical Kohn–Sham orbitals.
2.2.7 Gritsenko.
True, the shape of the accurate Dyson orbital of a primary ionization is very close to that of the corresponding accurate occupied Kohn–Sham orbital ϕi obtained by “reverse engineering” from the correlated density. However, the same is true also for Dyson orbitals of the satellites of this ionization, reflecting the fact that Dyson orbitals are neither orthogonal to one another other nor normalized. This “unfortunate” feature of Dyson orbitals definitely hinders their comparison with other, “normally behaving” sets of orbitals.
Due to this, the Kohn–Sham orbital energies εi differ, in general, from the ionization energies Ii by the spectroscopic average of the satellite ionizations (see contribution (2.4.9)) as well as by the contributions from the response potential (see contribution (2.2.3)), with equality only for the highest occupied Kohn–Sham molecular orbital.71 The “well-behaved” (i.e., orthonormal) Kohn–Sham orbitals are, in no way, the “poor cousins” of the Dyson orbitals, forming a distinctively different set of “optimal” orbitals. Indeed, unlike the Dyson orbitals, the occupied Kohn–Sham orbitals meaningfully accommodate the “electron pairs” of conceptual chemistry, while their energies provide a fair estimate of the potentials of primary ionizations. Furthermore, combined with the virtual Kohn–Sham orbitals and their energies, they form the basis for the successful treatment of electronic excitations in TDDFT (see contribution (2.4.9)).
2.2.8 Staroverov.
The classic Kohn–Sham scheme almost solves the problem of the kinetic-energy functional but its one-determinantal form creates formidable challenges for approximating the exchange–correlation part. These include the difficulty of devising exchange–correlation functionals for strongly correlated systems (see contribution (3.4.1)), limitations imposed by the assumption of noninteracting v-representability by a single Slater determinant, and the intricate behaviour of exact Kohn–Sham potentials (e.g., shifts within nodal surfaces of the highest-occupied Kohn–Sham orbital80), which DFAs somehow have to get just right. Although the existing Kohn–Sham DFAs are amazingly more accurate than the Hartree–Fock method in general, it is sobering that they still inherit most qualitative failures (see Section 3.4) of the mean-field approximation. Ensemble methods (see Section 3.7) seem unavoidable from this perspective.
2.2.9 Reining.
Just to emphasize a few points, more from a solid-state physicist's point of view: first, Kohn–Sham theory seems to be a natural next step when choosing to work with DFT. Certainly, formulating things (or at least, energies) in terms of functionals of the density is very much helped by the fact that the huge Hartree electrostatic energy is known as an explicit functional of the density. It allows us to have a large part that we know exactly and only a small remainder that has to be approximated.
What is more logical than continuing along this line and taking out another part (the kinetic energy of some noninteracting system)? And what is more logical than taking this noninteracting system to be “similar” to the real system – with the same density, in the spirit of DFT? Generalized Kohn–Sham theory is then also very natural, both because we know more pieces and because (like the kinetic energy) we do not know them as explicit density functionals. Making these pieces and the resulting “potentials” more and more complex appears to build a continuous bridge between Kohn–Sham and Green's functions equations. Another generalization is to start with the consideration that the calculation of any observable will in general integrate out certain details of a system, so the same value for the observable might well be found in a simpler system. This holds for the density – with the Kohn–Sham system, for example – but one can also build auxiliary systems for other observables and profit from the Kohn–Sham experience.
Second, further to the discussion about the Kohn–Sham system, we should keep in mind that, for a single electron, the Kohn–Sham excitation energies equal the exact ones, while the Kohn–Sham electron addition energies are different from the exact ones. So we may expect that, for certain systems, there is a reasonable correspondence for the excitation energies. It is far from obvious that this would also hold true in extended systems with many electrons, and, of course, the Kohn–Sham gap does not equal the optical gap in general. The Kohn–Sham band structure is nevertheless a powerful starting point for calculations using, for example, one- and two-body Green's functions.
Third, the sometimes bad reputation of the Kohn–Sham noninteracting system stems from the fact that it is often used in place of the real system – not to yield simply its density, but also any other observable, in particular, spectral functions. Of course, this can lead to strong disagreement with the truth – and the band gap is just one example. Maybe we should just be more precise in saying what we are doing here – namely, that we use the Kohn–Sham expression (which is a functional of the density) for a given observable as an approximate functional because we do not know a better one? This doesn’t change the results, but it sounds a little more fair to the Kohn–Sham noninteracting system.
2.2.10 Draxl.
Indeed, the bad reputation of the Kohn–Sham system may often come from the fact that we either tend to overinterpret results or are not precise enough about what we are doing. Sloppy phrases like “DFT is well known for its notorious band-gap problem” might have been considered appropriate long time ago, but should not be said anymore in 2022. Pointing out the SIE of many functionals is certainly important, but we should always make clear at the very same time that Kohn–Sham eigenvalues are not supposed to provide band gaps.
2.2.11 Baerends.
I would like to endorse the statement in contribution (2.2.10) that Kohn–Sham eigenvalues are not (should not be) supposed to provide band gaps. The fact that in solids the Kohn–Sham band gap is not equal to (or close to) the fundamental gap I–A is extremely frequently cited as the (notorious, infamous,…) band-gap problem. But it is a problem of wrong expectations.
In molecules, it is well known that the Kohn–Sham HOMO–LUMO gap is much below the I–A difference. This is due to the fundamental difference that the Kohn–Sham system has an attractive potential due to the exchange–correlation hole of −1 electron also for the virtual levels, while the Hartree–Fock system lacks this attractive hole potential for the virtual levels. In the same way, the presence of this vholexc potential lowers the LUMO level (bottom of the conduction band, BCB) in solids strongly.81 The exchange–correlation hole in solids is pretty localized – at a given point r, its size is usually well within a unit-cell range around r and therefore its potential is strongly stabilizing. In a delocalized excitation, from an occupied Bloch state to an empty Bloch state, the excited electron does not benefit from this stabilization. Neither does an added electron – the excitation energy to this delocalized state is understandably close to the fundamental gap. So, physically we cannot expect the Kohn–Sham band gap to match approximately the fundamental gap or a delocalized excitation energy. Excitons in a solid (except for Frenkel excitons) typically have a large size, extending over many unit cells. They have excitation energies not much lower than the delocalized excitations, so also for them the attractive Kohn–Sham potential vholexc does not fit reality.81
The situation is different in molecules since there the physical hole that the excited electron leaves behind is roughly mimicked by the attractive exchange–correlation hole in the Kohn–Sham potential. Hence the Kohn–Sham virtual–occupied orbital energy differences have the nice property that they do approximate excitation energies in molecules;72,73 see contribution (2.4.9).
The difference between the Kohn–Sham band gap and the fundamental gap can be cast in the form of expectation values of the response potential part vresp of the Kohn–Sham potential;82 see also contribution (3.8.6).
2.2.12 Vignale.
A question that keeps resurfacing is: Why are the Kohn–Sham orbitals better than the Hartree–Fock orbitals? From the point of view of the variational principle, the Hartree–Fock orbitals should be the best, since they build a Slater determinant which has the lowest energy (defined as expectation value of the Hamiltonian) among all Slater determinants. The Kohn–Sham wave function – also a single Slater determinant – cannot beat that. Nevertheless, we know that the DFT energy is better than the Hartree–Fock energy and also that the Kohn–Sham orbitals, as discussed in contribution (2.2.4), far from being meaningless, are in many ways “better” than the Hartree–Fock orbitals.
The resolution of the apparent paradox is that the Kohn–Sham energy is not calculated as the expectation value of the Hamiltonian in the Kohn–Sham wave function. The moment we adopt the Kohn–Sham approach, the original Hamiltonian of the system is no longer relevant. We are dealing with a reference system that is no longer interacting, but the rules for calculating the energy from the orbitals have also changed and are now expressed in terms of the exchange–correlation energy functional of the density. One could argue that the “particles” of this reference system are the “quasiparticles” of the original system, and this may help to rationalize the a priori surprising success of the Kohn–Sham orbitals in predicting single-particle excitation energies.
2.2.13 Baerends.
So in what sense are Kohn–Sham orbitals better than Hartree–Fock orbitals? When the energy of the determinant of Kohn–Sham orbitals is calculated with the full Hamiltonian, its energy is of course higher than the Hartree–Fock energy, but actually by only a tiny amount.83 On the other hand, the Kohn–Sham density, being equal to the exact one and not so diffuse as the Hartree–Fock one (in molecules), leads to much improved (more negative) electron–nuclear energy. Also the orbital shapes are “better” than the too diffuse Hartree–Fock orbitals (in molecules), so the kinetic energy is also considerably better (higher). The errors of the Hartree–Fock model for these two energy terms are large, in molecules often larger than the bond energy, and they rapidly increase upon bond lengthening.83 They cancel to some extent, which is why they are not so readily recognized. The Hartree term is of course also better (exact) with Kohn–Sham orbitals and density.
A tongue in cheek observation would be that the Hartree–Fock model manages to build a determinant that has a little bit lower expectation value of the Hamiltonian, but it has to distort the orbitals (make them more diffuse) because the lowering of the kinetic energy then just outweighs the energy penalty of the increase in the electron–nuclear energy. The Hartree–Fock model does not care – it just tries to get the lowest energy determinant. As noted in contribution (2.2.12), the true power of Kohn–Sham DFT has to come from accurate approximations of the exchange–correlation energy (defined in the Kohn–Sham context), but the good properties of the Kohn–Sham orbitals are an asset of this model.
2.2.14 Gidopoulos.
To address the recurring question by Vignale in contribution (2.2.12), I would like to point out that the Kohn–Sham orbitals are in fact as “energetically optimal” as the Hartree–Fock ones. Let me first quote Walter Kohn, who said in his Nobel Prize lecture that, while the Hartree–Fock orbitals are “total energy optimal”, the Kohn–Sham orbitals are “density optimal” because they yield the exact density.
Although, undeniably the Hartree–Fock Slater determinant has the lowest energy among all Slater determinants, we now know that the Kohn–Sham determinant can at least match, if not beat that (record), since it is “energy optimal” in a similar sense: in the Hartree–Fock optimization, we use the full interacting N-electron Hamiltonian, H and then seek the lowest energy Slater determinant as the best approximate ground state. For the Kohn–Sham orbitals, we may perform an equivalent, but reverse Rayleigh–Ritz optimization: let us assume that the ground state Ψ of the physical N-electron, interacting system is somehow known (and fixed). Then, we consider all N-electron effective, noninteracting Hamiltonians, Hv, with a local potential v(r). The ground-state wave function and energy of each Hv are Φv and Ev, respectively.
For N > 1, Ψ cannot be the exact ground state of any of these noninteracting Hamiltonians, Ψ ≠ Φv for each v, because Ψ is an interacting state while all Φv are noninteracting states (Slater determinants). Hence, the following Rayleigh–Ritz energy difference on the left-hand side is strictly positive:
This energy difference gives a measure of how well
Ψ approximates the ground state
Φv of the effective Hamiltonian
Hv. The smaller the energy difference, the better the approximation of
Ψ to
Φv. It is elementary to confirm that the energy difference is minimized by the exact Kohn–Sham Hamiltonian
Hvs.
84 Interestingly, the exact density property of the Kohn–Sham state is the result of the Rayleigh–Ritz optimization and the density is not
a priori fixed. Hence, the Kohn–Sham Slater determinant, on top of being “density optimal”, it is also “energetically optimal” in a Rayleigh–Ritz optimization, which physically is equivalent to the total energy minimization of Hartree–Fock theory.
I note that the variational principle in eqn (12) can be used to construct optimally converging power series expansions for the Kohn–Sham potential, without using the adiabatic connection (AC) path formalism.85
2.2.15 Yang.
I would like to address the physical meaning of Kohn–Sham orbitals in calculations with DFAs. Most DFAs to the exchange–correlation energy Exc usually produce reasonable total energies for small and medium-size molecules; however, they have major deficiencies in the orbital energies. As has been known for a long time, for finite systems, the eigenvalue of the HOMO for the exact Kohn–Sham potential is equal to the negative of the first ionization potential (IP), as follows from the asymptotic decay behaviour of the exact electron density and the requirement that the Kohn–Sham effective potential be zero at infinity.86 However, in a Kohn–Sham calculation, the local Kohn–Sham potential can have any additive constant and give the same total energy and density but different orbital energies. Thus, the argument based on the long-range behaviour of density and potential hinges on a particular choice of the additive constant of the potential.
The orbital energies for the frontier HOMO and LUMO were rigorously shown to be the DFA prediction of the negative of the first IP and the first electron affinity (EA) in 2008.21 Three key results were used in the proof. (1) The Janak theorem shows that Kohn–Sham orbital energies are the derivatives of the total energy with respect to the orbital occupation numbers. Note that the Janak theorem does not relate orbital energies to any physical observables.87 (2) The left and right derivatives of the total energy with respect to the total electron number, or the left and right chemical potentials, are the negative of the first IP and the first EA, respectively, of the corresponding energy functional. This follows from the linear condition on the behaviour of the total energy for fractional number of electrons.18 The linearity condition is true for the exact functional, or for a functional without delocalization error for general systems. For infinite bulk systems, however, the linearity condition holds true for any functional approximation.16 (3) The chemical potentials were proved to be the derivatives of the energy with respect to the orbital occupation numbers of HOMO and LUMO in a Kohn–Sham calculation, when the exchange–correlation energy used is a functional of the density. With the use of the Janak theorem, this then establishes that the Kohn–Sham HOMO and LUMO energies are the chemical potentials of the system for the given DFA.21 Similarly, when the exchange–correlation energy is a functional of the noninteracting one-electron density matrix, the chemical potentials were proved to be the derivatives of the energy with respect to the orbital occupation numbers of HOMO and LUMO in a generalized Kohn–Sham calculation.21 Therefore, the HOMO and LUMO orbital energies are the DFA prediction of the negative of the first IP and the first EA. This interpretation of the HOMO and LUMO energies holds true for molecular and bulk systems, for any given DFA.
Indeed, DFAs with minimal delocalization error23–25 have excellent predictions of IPs and EAs from the HOMO and LUMO of generalized Kohn–Sham calculations, comparable to the accuracy of GW approaches.88 In addition, the orbital energies above the LUMO and below the HOMO approximate the corresponding quasi-particle energies, with similar accuracy as the HOMO/LUMO for the IP/EA. This has been explored to describe accurately the excitation energies and conical intersections of molecular systems in the quasi-energy DFT approach based on ground-state generalized Kohn–Sham calculations.88,89
2.2.16 Baerends.
In relation to contributions (2.2.15) and (2.2.11), it should be stressed that it is very important to distinguish between the properties of, on the one hand, the exact (original) Kohn–Sham model of noninteracting electrons in a local potential such that the exact density is reproduced and, on the other hand, the currently popular DFAs – in particular, those of the generalized Kohn–Sham family with nonlocal potentials. The local Kohn–Sham potential is unique by application of the Hohenberg–Kohn theorem to the noninteracting electron system, and so are the orbitals and orbital energies.
The attractive properties of the exact Kohn–Sham orbitals and orbital energies have been expounded in some contributions; see contributions (2.4.9), (2.2.4), (2.2.13), and (2.2.11). A salient feature of the exact Kohn–Sham model is that the LUMO is not at −A (given that the HOMO is at −I) but much lower: the HOMO–LUMO gap is approximately equal to the first excitation energy.72,73,90 It should be made clear that contribution (2.2.15) does not contradict these properties of the exact Kohn–Sham model. It refers to a different family of Kohn–Sham models, usually called the generalized Kohn–Sham models. These generalized models make it possible to include, for instance, part of the exchange operator (a nonlocal potential) of the Hartree–Fock model and adjust the local part of the potential so that the density remains exact and adjust the exchange–correlation functional so that the energy also remains exact.67 In such a scheme, the orbital energies are different from those generated by the exact local Kohn–Sham potential. In such a generalized Kohn–Sham model, one may strive to obtain that the HOMO is again at −I and that the LUMO is now at −A, as is also done in the Koopmans-compliant functionals.91,92 The LUMO then becomes more diffuse and one loses the simple representation of excitations in TDDFT with just one or a few orbital transitions.73
2.2.17 Yang.
In relation to the discussion in contributions (2.2.15) and (2.2.11) on the physical meaning of the HOMO and LUMO in DFT, it is important to separate the two types of one-electron Kohn–Sham Hamiltonians. The first one is from the ground-state calculation with a given DFA EDFAxc, which yields the density, orbitals and orbital energies of the noninteracting reference system, as developed in the original Kohn–Sham paper.65 This is called the direct approach.93 The second one is from an inverse calculation, generating the local potential vσs(r) that reproduces a given ground-state electron density, which can be the exact density or an accurate density from high-level calculations. We called this the inverse Kohn–Sham or inverse OEP approach,93 the potential so obtained is also called the “exact Kohn–Sham” potential by Baerends in contribution (2.2.16).
In an inverse approach, the local potential is determined up to an arbitrary constant. Thus, in principle, the absolute values of the orbital energies are not defined. However, if the correct asymptotic condition on the potential is satisfied, which also sets the constant, then εH = −I is obtained, where I is the experimental ionization energy, if an exact density is given (row 1 Table 1). Similarly, a good approximation to the experimental −I is expected if a good approximation to the density is given from a DFA calculation (row 1 in Table 1). However, the corresponding LUMO energy has not been shown to relate to the ionization energy and is not a good approximation to the experimental −I, as discussed in contribution (2.2.16). In atomic calculations, the unoccupied-orbital energies, {εa}, obtained from inverse Kohn–Sham calculations, have been shown to represent electronic excitations, with εa − εH describing excitation energies of the system with the same number of electrons. Using εa − εH to approximate excitation energies for molecules is less successful.
Table 1 Properties of the electron density ρσs(r) and HOMO and LUMO orbital energies, εH and εL, of the noninteracting reference systems in exact DFT (EDFT) and various DFA models. The DFA models include all continuous functionals of the density EDFAxc[ρσs(r)], continuous functionals of the non-interacting density matrix EDFAxc[ρσs(r′,r)], and continuous functionals of the noninteracting orbitals and the external potential EDFAxc[{ϕpσ},vext(r)]. Computational approaches for ρσs(r), εH and εL include inverse calculations from a given (accurate) electron density and direct calculation methods based on the original Kohn–Sham approach (KS), the optimized effective potential (OEP), the generalized Kohn–Sham (GKS) and the generalized optimized effective potential (GOEP, which has been shown to be equivalent to orbital optimization (OO); see Jin et al.94). Three properties of each quantity are considered for each computational approach: (1) agreement of ρσs(r), the density of the noninteracting reference system, with ρσ(r), the density of the physical system consistent with the exact DFT, or the density of the DFA as defined by the linear response
; see Chen et al.,95 Voora et al.;96 (2) agreement of the HOMO orbital energy εH with
the chemical potential of electron removal for the functional employed; (3) agreement of the LUMO orbital energy εL with
the chemical potential of electron addition for the functional employed. No entry indicates that it is impossible or not yet known how to conduct the corresponding calculation. (Table provided by Yang, extended from ref. 93)
Noninteracting system |
Type |
|
E
DFT
|
E
DFAxc[ρσs(r)] |
E
DFAxc[ρσs(r′,r)] |
E
DFAxc[{ϕpσ}, vext(r)] |
In an inverse calculation, the potential is determined up to an arbitrary constant and the absolute values of the orbital energies are therefore undefined. However, if the correct asymptotic condition on the potential is imposed, which also sets the constant, then εH = −I, is obtained, where I is the experimental ionization energy.86
If the correct asymptotic condition on the potential is imposed, and if a good electron density is obtained from the DFA, then the inverse OEP calculation will leads to εH that is a good approximation to the experimental −I.
The agreement between ρσs(r) with is only true at the complete basis set limit for the basis set expansion of vσs(r), and not so for any finite basis set.93
Similar to (b), if the correct asymptotic condition on the potential is imposed, then the direct OEP calculation will lead to εH that is a good approximation to the experimental −I.
For explicit functionals of the density, or the density matrix, GOEP/OO gives the same total energies and density matrix as in regular SCF. But the orbitals obtained in general are no longer the canonical orbitals and thus have no orbital energies directly. However, a unitary rotation can bring them to the canonical orbitals with proper orbital energies in agreement with the corresponding chemical potentials.
In GOEP or OO calculations, the Hamiltonian for the noninteracting system is not available, so neither are the noninteracting orbital energies.
|
Inverse KS/inverse OEP vσs(r) |
Inverse |
ρ
σ
s(r) |
Yes |
Yes |
Yes |
Yes |
|
|
ε
H
|
|
|
|
|
|
|
ε
L
|
No |
No |
No |
No |
KS vσs(r) |
Direct |
ρ
σ
s(r) |
|
Yes |
|
|
|
|
ε
H
|
|
Yes |
|
|
|
|
ε
L
|
|
Yes |
|
|
OEP vσs(r) |
Direct |
ρ
σ
s(r) |
|
Yes |
Yes/noc |
No |
|
|
ε
H
|
|
Yes |
|
|
|
|
ε
L
|
|
Yes |
No |
|
GKS vσs(r,r′) |
Direct |
ρ
σ
s(r) |
|
Yes |
Yes |
|
|
|
ε
H
|
|
Yes |
Yes |
|
|
|
ε
L
|
|
Yes |
Yes |
|
GOEP/OO vσs(r,r′) |
Direct |
ρ
σ
s(r) |
|
Yes |
Yes |
No |
|
|
ε
H
|
|
Yese |
Yese |
|
|
|
ε
L
|
|
Yese |
Yese |
|
In a direct calculation with a DFA – that is, when the energy is minimized with respect to its variables, as discussed in contribution (2.2.15) – the HOMO energy of the noninteracting reference system has been shown to be equal to the chemical potential for electron removal
| 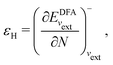 | (13) |
and LUMO energy of the noninteracting reference system has been established as being equal to the chemical potential for electron addition
| 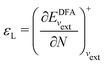 | (14) |
for a Kohn–Sham calculation with
EDFAxc[
ρσs(
r)] and also for a generalized Kohn–Sham calculation with
EDFAxc[
ρσs(
r′,
r)] in the work of Cohen, Moris-Sanchez and Yang
21 (rows 2 and 4 in
Table 1). Note that these identifications are based on the assumption that
EDFAxc[
ρσs(
r)] and
EDFAxc[
ρσs(
r′,
r)] have an explicit and continuous dependence on its variables
ρσs(
r) and
ρσs(
r′,
r). But no locality is assumed. With these identifications, the use of HOMO/LUMO energy to approximate −
I/−
A was then established,
21 building on the PPLB condition for fractional number of electrons and its results for chemical potentials.
18 The quality of the approximation of
εH to −
I and/or
εL to −
A just reflects the quality of the DFAs used, where the delocalization error of the DFA plays a key role;
16 see contribution (2.2.15).
There are other approaches to direct calculation, using as the basic computational variable either a local potential vσs(r) in an OEP approach or a nonlocal potential vσs(r,r′) in the direct generalized OEP (GOEP) approach.94 The meaning of HOMO and LUMO energies in direct OEP calculations was established in ref. 21; see also Row 3 in Table 1.
In Table 1, we also list the results on the agreement of the electron density of the noninteracting reference system with the density of the physical system as defined by the linear response.93
2.2.18 Trickey.
The pervasive emphasis on the Kohn–Sham orbitals to this point in the discussion is striking and, from the perspective of my interest in orbital-free DFT, a bit overbalanced. From that perspective, the Kohn–Sham orbitals and eigenvalues are not the crucial insight provided by the Kohn–Sham decomposition – that crucial insight is the existence (assuming v-representable densities) of a noninteracting system with the same density as the many-body system. With that assumption, existence is provable by application of Levy6–Lieb8 for the ground-state and Runge–Gross97 (as updated by Ruggenthaler et al.98,99) for the time-dependent case and Mermin50 for the temperature-dependent case. The orbitals (and eigenvalues) are a valuable, exploitable by-product.
Particularizing to the ground state, Kohn–Sham DFT is, at base, the decomposition of the Levy–Lieb functional (putting aside to a separate discussion the issues associated with the original Hohenberg–Kohn and later Levy–Lieb functionals) into physically recognizable, interpretable, and computable parts. Orbital-free DFT (better called one-orbital DFT) exploits only the decomposition, while conventional Kohn–Sham DFT also uses the Kohn–Sham orbitals explicitly. Both variants (to use a currently prominent word) are fundamentally Kohn–Sham theory. Both have the same definitions of kinetic energy, Hartree energy, exchange energy, and correlation energy. All those definitions depend upon the Kohn–Sham determinant.
The distinction between those two variants is operational – namely, what is done to exploit the Kohn–Sham decomposition computationally. This is crucial because of the many statements that one sees to the effect that orbital-free DFT is an “alternative formulation of DFT” that avoids the problems of Kohn–Sham theory, etc. That completely ignores the underlying Kohn–Sham logic. That logic is in fact crucial to constraints on approximate kinetic-energy density functionals (KEDFs).
2.2.19 Gritsenko.
The unique feature of the exchange–correlation part of the local Kohn–Sham potential is the richness of the physical information on the local effects of electron correlation, as reflected in the shape of the potential. This can be favourably compared with the nonlocal Hartree–Fock and self-energy potentials of the wave-function theory produced from the corresponding kernels. The shape of the latter potentials is “ruined” with singularities related to the orbital nodes. Contrary to this, the steps of the Kohn–Sham exchange–correlation potential meaningfully distinguish the local correlation effects in adjacent atomic and molecular shells with the corresponding “gauges” (see contribution (2.2.3)), while its integer discontinuity “jumps” signal occupation of (previously virtual) Kohn–Sham orbitals.
Then, instead of complaining about “the idiosyncratic behaviour” of the Kohn–Sham exchange–correlation potential, one should fruitfully explore and employ this meaningful information – see, for example, contributions (3.1.12) and (3.8.6). Moreover, one should not attempt to “wash away” this precious true information by constructing artificially too smooth Kohn–Sham exchange–correlation potentials by “reverse engineering” techniques.
As to the generalized Kohn–Sham scheme, the term ‘Kohn–Sham’ seems to be misused in this case. Indeed, out of desire to get electron affinities as the energies of virtual orbitals, the original Kohn–Sham theory is forcefully “crashed” in some (out of infinitely many) variants of the generalized Kohn–Sham “landscape” by mixing different theories both globally and with range-separation techniques.
2.2.20 Görling and Kronik.
With respect to the term ‘generalized Kohn–Sham’, we feel that it is appropriate. The generalized Kohn–Sham approach67 relies on the basic idea of the original Kohn–Sham formalism by exploiting the Hohenberg–Kohn theorem to introduce a model system with the same ground-state density, in order to have access to quantities that help in the description of the electronic system. Such quantities can be energies, typically the ‘noninteracting’ kinetic energy or the exchange energy, but can also be orbital eigenvalues. The generalized Kohn–Sham approach generalizes the Kohn–Sham one in the sense that it extends the range of possible model systems. Like all proper generalizations, it contains the original Kohn–Sham approach as a special case. As also discussed in contribution (2.4.8), the generalized Kohn–Sham approach provides more flexibility and establishes a firm formal foundation for frequently used methods that do not calculate the exchange–correlation potential as a functional derivative with respect to the electron density, notably hybrid functional methods. And, as also discussed in contribution (4.1.5), a specific generalized-Kohn–Sham map need not be “crashed”, but rather can be judiciously chosen, nonempirically, based on physical constraints.
2.2.21 Görling.
It is instructive to define which electronic-structure approaches are Kohn–Sham methods. Such a definition reveals the key characteristics of the Kohn–Sham formalism and shows the scope and perspective that the Kohn–Sham formalism provides. By a quite wide definition, those methods are Kohn–Sham methods that rely on a model system of noninteracting ‘electrons’ with the same ground-state electron density as the true physical electronic system and with a local multiplicative potential. The noninteracting ‘electrons’ are particles that are identical to electrons – in particular, they have the spin of electrons – but do not interact among themselves. Given that the particles of the Kohn–Sham system are noninteracting, the Kohn–Sham equation for the Kohn–Sham orbitals and their eigenvalues in eqn (8) emerges immediately.
Traditionally, the Kohn–Sham orbitals are used only to evaluate the kinetic energy of the Kohn–Sham model system, which represents the bulk of the full kinetic energy, taking into account the fermionic nature of electrons. The Kohn–Sham orbitals, however, contain much more information than their kinetic energy. The occupied Kohn–Sham orbitals, for example, enable an exact calculation of the exchange energy. This means that all parts of the total energy except the correlation energy can be easily calculated exactly, technically by evaluating the Hartree–Fock energy with Kohn–Sham orbitals. Indeed, approximating only the remaining small part of the energy, the correlation energy, is a natural and systematic approach. For individually approximating the correlation energy, orbital-dependent functionals40 can be constructed that use occupied as well as unoccupied Kohn–Sham orbitals and their orbital energies, in this way exploiting much more of the information contained in the Kohn–Sham model system.
Historically, this route was not pursued for three reasons:
(1) to avoid the high cost of evaluating the exact exchange energy, which nowadays is not really a problem for molecules up to a size of several hundred atoms. For larger systems or when very many electronic-structure calculations are required, in ab initio dynamics simulations, for example, the cost of exact exchange remains an issue.
(2) to benefit from error cancellation between exchange and correlation contributions. While this is a valid reason, the cancellation is not complete, limiting the accuracy that can be reached by traditional Kohn–Sham methods.
(3) to avoid the problem that the exchange potential is not directly accessible in terms of the Kohn–Sham orbitals. With the OEP method, functional derivatives of orbital-dependent energy expressions, including – for example, the Kohn–Sham exchange potential – are accessible.40–46
While basis-set OEP methods were numerically problematic in the past, robust, numerically stable basis-set OEP methods are now available.46 Moreover, orbital-dependent functionals can be evaluated in a post-self-consistent-field (post-SCF) manner, avoiding the need to take functional derivatives of orbital-dependent functionals with respect to the electron density. Alternatively, functional derivatives can be taken with respect to orbitals instead of the electron density, leading to generalized Kohn–Sham methods.
Meta-GGA and hybrid functionals are established functionals that depend on the occupied orbitals. Correlation functionals based on the adiabatic connection fluctuation-dissipation (ACFD) theorem100,101 depend on unoccupied as well as occupied orbitals and their eigenvalues. The simplest example of such a functional is the correlation energy within the random-phase approximation (RPA).102–104 All these methods are Kohn–Sham methods or, depending on the way the exchange–correlation potential is obtained, generalized Kohn–Sham methods.
2.2.22 Trickey.
The remark by Görling about the computational cost of exact exchange deserves emphasis. He observes that the cost “nowadays is not really a problem for molecules up to a size of several hundred atoms. For larger systems or when very many electronic-structure calculations are required, in ab initio dynamics simulations, for example, the cost of exact exchange remains an issue.”
This is a crucial distinction between gas-phase chemistry and materials physics and chemistry. For those with access to significant computing resources, exact exchange is not prohibitive for the comparatively small number of calculations needed to study an isolated molecule of up to a few hundred atoms. But that is manifestly not true for ab initio molecular dynamics (AIMD) of several thousand molecular-dynamics (MD) steps used to screen tens of different but kindred condensed-phase systems, for each of which the constituents are molecules with 300 or more non-hydrogen atoms. This distinction illustrates the compelling importance of continued effort to improve lower-rung DFAs. It also is but one example that there is more than gas-phase chemistry at stake in the development of DFT methodology and algorithms.
2.2.23 Jones.
I agree with Trickey in contribution (2.2.22) and Görling in contribution (2.2.21). The computational effort required in many “real-world” applications is often underestimated – see also Trickey in contribution (3.2.12), concerning other problems of extended systems. A single MD simulation of nanoseconds with a time step of femtoseconds can mean millions (!) of self-consistent DFT calculations of a system with hundreds of atoms.105 A factor of ten (or even of two) in computer time per time step can mean the difference between completing the calculation and abandoning it.
2.2.24 Savin.
The Hohenberg–Kohn theorem is valid for many Hamiltonians, including those with no interaction between particles. The latter case shows already the difficulty of constructing closed-form approximations to an energy density functional. Kohn and Sham decided to alleviate the treatment of electronic systems by treating accurately a (model) noninteracting system and by using density-functional corrections only for the difference between the energy of this system and the system of interest, with interacting electrons. Note that this idea is easily extended to other model Hamiltonians, making it possible to go beyond the use of a single Slater-determinant reference within DFT – see, for example, ref. 106.
2.2.25 Tozer.
A feature of regular Kohn–Sham calculations using common exchange–correlation functionals is that the electronic energy does not in general equal the sum of the occupied orbital energies. Recently, Levy and Zahariev107 proposed the direct energy Kohn–Sham (DEKS) scheme, whereby the Hartree-exchange–correlation potential is shifted by a constant, in order to make the electronic energy equal to the sum of the orbital energies. This shifted potential has attractive theoretical characteristics and so it is desirable to try to model it directly for use in DEKS calculations. The use of density-scaling homogeneity considerations is one possible way forward.108
2.2.26 Arbuznikov.
The remarks of Schwerdtfeger in contribution (2.1.31) have prompted me to add a few words on relativistic exchange–correlation functionals.
Despite the lack of a rigorous theory that would allow one to construct them in a systematic way, a potentially useful pragmatic solution within the Dirac–Coulomb–Breit framework has been known for a long time. Since relativistic effects become important at high densities – that is, in exchange-dominated core regions – one could, in a first approximation, restrict oneself to an appropriate treatment of the exchange energy. For the exchange energy of the relativistic homogeneous electron gas (RHEG),57,59,109 a multiplicative correction (a kind of “enhancement factor”) has been derived as a simple analytic function Φ(β), where β = (3π2ρ)1/3/c (in atomic units). This function satisfies Φ(β) > 1 and tends to one at the low-density limit; it is a sum of both Coulomb (longitudinal) and Breit (transverse) contributions. This scheme has been implemented and tested for atoms at the LDA level110 and subsequently extended to the GGA level111via data on the linear response of the RHEG to a weak perturbing potential.57 Data for several small diatomics are available as well.112
While valence-shell related properties turned out not to be sensitive with respect to these corrections,112 a high sensitivity of core one-electron energies of heavy atoms has been clearly demonstrated.111 For heavy atoms, these corrections seem to be of the same order of magnitude as atomic (nonrelativistic) correlation energies.110 So far, it appears that these corrections have not yet been implemented into a molecular or solid-state code. Obviously, studies of the impact on core-related properties will be of interest. Recently, short-range LDA and GGA exchange functionals have been developed and implemented in a similar way,113,114 but again only for atoms and ions so far.
A very recent development of a potentially useful relativistic local hybrid functional115 within an X2C code should be mentioned as well.
2.3 What can be described with DFT?
2.3.1 Helgaker.
Pure (non-Kohn–Sham) DFT provides the ground-state density and the ground-state energy. We can then (in principle) obtain rigorously all properties that can be expressed as functions of the density and the energy – for example, derivatives of the energy with respect to nuclear displacements or nuclear magnetic moments (provided DFT has been extended to deal with magnetism as discussed in contribution (4.3.1)). We can in principle also calculate excitation energies, from equiensembles.
In practice, we do Kohn–Sham DFT, which in addition to the density and the ground-state energy (in principle, both exact) also gives us the Kohn–Sham noninteracting wave function, from which many more properties of the system can be obtained, but only approximately, given that the Kohn–Sham wave function is a noninteracting approximation to the exact many-body wave function.
We are of course free to use the Kohn–Sham wave function as a zero-order starting point for a many-body treatment – but we are then leaving the domain of DFT.
2.3.2 Görling.
The ground-state electron density yields the electron number and the Hohenberg–Kohn theorem tells us that it determines furthermore the external potential and thus the Hamiltonian operator which determines all properties of an electronic system. Therefore the ground-state electron density determines the energy and the properties of the ground state and of all excited states. In practice, we typically use DFT to get information on ground-state properties and for excited states we switch to TDDFT in the linear response regime. However, it might be worthwhile to devote more effort to explore how excited-state energies and properties can be obtained in DFT without invoking TDDFT.
2.3.3 Krylov.
I would like to see some thoughts of how to approach the problem of extracting properties that cannot be formally expressed in terms of the electron density or one-particle density matrix. The value of S2 is such a property.
2.3.4 Reining.
I agree, in principle, that we should get from the density all properties that are determined by the external potential and the number of electrons. Why do we then feel that we have so little diversity in the observables that are traditionally dealt with in DFT? First, this statement is actually not true, if we consider the Kohn–Sham observables as approximations to the true density functional of, for example, spectra – there are many such calculations around. The Kohn–Sham expressions are of course not explicit functionals of the density, but implicit ones, via the orbitals. But why is it so difficult to go beyond the Kohn–Sham approximation and find better ones for these observables?
Again, this is actually not completely the case. Take the polarizability – we do go beyond the Kohn–Sham independent particle polarizability, by adding Hartree (i.e., the bare Coulomb interaction in the integral kernel of the Dyson equation) effects in the RPA, and even exchange–correlation effects through the exchange–correlation kernel, which is also a density functional. Like Görling in contribution (2.3.2), you might object that this is TDDFT, but I would say it is linear response in the ground state, so we are talking about functionals of the ground-state density. Simply, we have derived this ground-state density functional using TDDFT, but who cares how we derived it once we have it? We could of course dream of finding simpler functionals for the polarizability, maybe even explicit functionals of the ground-state density, but since even the kinetic energy is so difficult, I wouldn’t bet on this in the near future.
2.3.5 Yang.
An exact DFT calculation for the ground state of an N electron system provides directly the ground state total energy Ev(N) and electron density. It also provides the ground state energies for the corresponding (N − 1) and (N + 1) electron systems directly through the chemical potentials of the N electron system. The extension of a similar connection to the excited states of the corresponding (N − 1) and (N + 1) electron systems has recently been made.88,89,116,117 However, since the exact functional is not available in an explicit form, neither is the method for the associated chemical potential calculations. We now focus on the discussion on explicit functional forms that include most existing DFAs.
For an N-electron system, a Kohn–Sham calculation with an exchange–correlation functional that is an explicit and continuous functional of the electron density leads directly to Ev(N − 1) and Ev(N + 1), the ground-state energies of the corresponding (N − 1) and (N + 1) electron systems. Similar connections follow for a generalized Kohn–Sham calculation with an exchange–correlation functional that is an explicit and continuous functional of the noninteracting reference density matrix. This is true because of the following: (1) it has been proved that the HOMO/LUMO energy is the chemical potential for electron removal/addition,21 (see Table 1) (2) the PPLB condition shows that the chemical potential of the N-electron system is −I and −A.18 Thus the band gap can be predicted from the HOMO–LUMO gap, in either Kohn–Sham calculations with an explicit functional of the electron density or generalized Kohn–Sham calculations with an explicit functional of the noninteracting reference density matrix. This connection is independent of the functional approximation. However, the accuracy of the prediction depends on the quality of the functional used.21 For functionals with minimal delocalization error, the prediction is comparable to, or better than, that of GW approaches.25,88,89
Similarly to the access to the ground state information of the corresponding (N − 1) and (N + 1) electron systems, it has been argued recently that ε(N), the orbital energies of orbitals above LUMO and below HOMO also approximate the corresponding quasiparticle energies ω+/−(N) as follows: εm(N) ≈ ωm+(N) = Em(N + 1) − E0(N), and εn(N) ≈ ωn−(N) = E0(N) − En(N − 1). This then links directly to the excited-state energies of the corresponding (N + 1) and (N − 1) systems.88,89,116,117 Extensive numerical evidence supports this claim.88,89,116,117 Thus, the excited-state energies of N electron systems can be obtained from ground-state calculations on the (N − 1) or (N + 1) electron systems.88,89,116,117
2.4 What concepts are useful for the development and understanding of DFT?
2.4.1 Perdew.
An open subsystem of fluctuating (and thus on average noninteger) electron number is a surprisingly useful concept. Real atoms have integer electron numbers, but local and semilocal approximations to the DFA for the exchange–correlation energy spuriously predict the transfer (delocalization) of a fraction of an electron between two different well-separated open-shell atoms (or between two open subsystems of a combined system). Nature's integer preference is explained by invoking an ensemble description of each separated open quantum subsystem that is equivalent to making a wave-function description of the combined system.18 When the electron number in the open quantum subsystem is varied between two adjacent integers, its exact total energy and electron density vary linearly with the electron number (piecewise linearity), so the exact energy minimizes at an integer electron number.
This has important practical consequences. In particular, local and semilocal approximations predict incorrect energies and densities for a diatomic molecule AB in the dissociation limit. In fact, these approximations are much more accurate for integer than for fractional electron numbers. This problem still plagues density functional approximations. A non-self-consistent cure is to evaluate the approximate functionals on Hartree–Fock densities, which localize an integer charge around each separated nucleus.118 Doing that also cures some related problems, such as spurious charge transfers at smaller internuclear separations.
2.4.2 Perdew and Savin.
In many cases, the energy and wave function of the interacting system can be connected smoothly to those of the Kohn–Sham noninteracting system of the same electron density. Then the exact exchange–correlation energy for that density becomes an integral over the strength of the electron–electron interaction, which subsumes both the potential energy of exchange and correlation and the kinetic energy of electron correlation. The AC and the idea of modelling the pair density associated with it100,119–121 served as the key inspiration not only for passing from LDA to GGAs,122,123 but also for making the step to hybrid functionals.124 Note that it is not necessary to use the pair density in the adiabatic coupling; one can use the first-order density matrix as well – see, for example, ref. 125.
2.4.3 Sun.
Related to the concepts mentioned above – that is, the AC and fractional charges – the concept of the exchange–correlation hole has been useful for the development and understanding of DFT. For example, the sum rules for the exchange and correlation holes have been used to explain the successes of LDA, while the successful PW91 GGA functional was constructed by enforcing the sum rules for the exchange and correlation holes on the gradient expansion approximation of slowly varying densities. The construction of the SCAN meta-GGA was guided by the understanding of the exchange and correlation holes. In particular, prototypical systems with very localized exchange correlation holes can be used as appropriate norms, whose exchange–correlation energies can be exactly or nearly exactly predicted by a semilocal density-functional approximation. Semilocal approximations, whose underlying exchange–correlation-hole models are necessarily semilocal, must fail to describe systems with delocalized exchange correlation holes – for example, systems characterized by fractional charges.
2.4.4 Xu.
The AC path mentioned in contribution (2.4.2), which bridges the fictitious noninteracting Kohn–Sham system to the fully interacting real system, is one of the most important concepts in the development and understanding of DFT.100,101 The coupling-constant integration along the AC path defines the Kohn–Sham exchange–correlation functional, which also accounts for the kinetic energy of correlation.125 The more we know about the AC path, the better DFAs we can construct.
The first widely recognized hybrid DFA is Becke's half-and-half functional.124 It was derived based on a linear model for the AC path, which was then empirically extended, leading eventually to the widely used B3LYP functional.126–129 More sophisticated AC models have been used to develop and rationalize the popular “nonempirical” PBE0 functional,130 as well as some other hybrid functionals.131
The AC formalism has provided an important playground for the development of the advanced DFAs that involve the unoccupied Kohn–Sham orbitals. The random-phase approximation (RPA) was introduced to the DFT community via the ACFD formalism.100,132 Görling–Levy (GL) perturbation theory133 shows that the initial slope of the AC path is twice the second-order GL perturbation energy (GL2). For systems with a linear AC path, the exact exchange–correlation functional is therefore nothing but the exact exchange plus GL2 correlation energy.134 The AC formalism has motivated the initial developments of several successful double-hybrid approximations by further mixing the second-order perturbation (PT2) energy with the already successful hybrid functionals.134–137
2.4.5 Gori-Giorgi.
The AC can be mathematically extended outside the usual range between the Kohn–Sham and the physical systems – for example, to negative coupling strengths (attractive electrons)138 or, more interestingly, to very large positive coupling strengths (electrons repelling each other infinitely strongly, or, equivalently, the Levy–Lieb functional in the ℏ → 0 limit139,140). This latter case defines the limit of strictly correlated electrons (SCE),141–144 which yields the functional complementary to the Kohn–Sham kinetic energy – that is, the minimum possible electron–electron interaction of a system with given one-electron density ρ(r); see eqn (76) in contribution (4.5.8). The SCE functional also yields the exact low-density limit of the exchange–correlation functional of Kohn–Sham DFT. Although chemical systems are usually very far from this limiting situation, the SCE functional sheds light on the nonlocal nature of the exact exchange–correlation functional and can inspire the construction of new approximations to handle strong correlation.145–147
Another way to use the SCE limit in chemistry is to build interpolation models of the AC between the Kohn–Sham limit (which may include exact exchange and second-order perturbation theory) and the expansion at strong coupling strength.142,148–151 The interpolation strategy based on global quantities (integrated over all space, a strategy that can be viewed as creating nonlinear hybrids and double hybrids) was abandoned for some time because of its lack of size consistency. However, more recently, it has been shown that size consistency can be easily restored for these functionals at no extra computational cost.150
2.4.6 Teale and Helgaker.
The AC is certainly a powerful tool for understanding the universal density functional. Using the Lieb variation principle (see contribution (2.1.13)), the AC can be calculated to high accuracy using many-body wave-function techniques.152–155 As well as the usual linear AC path, generalized AC paths, such as those based on the error function, can be calculated and are relevant for range-separated hybrid functionals.11,156,157
Such calculations can also be used to extract the coupling-constant-dependent one- and two-particle density matrices. The one-particle density matrices may be used to define an AC focusing on the kinetic component of the DFT correlation energy – see, for example, ref. 125 and 158, as alluded to in contribution (2.4.8) and calculated in ref. 159. The two-particle density matrices can be used to give direct access to the exchange–correlation hole and its coupling-constant average.149,160 All these quantities can be determined using high-level ab initio methods, giving valuable insight into the near exact behaviour of F[ρ]. The challenge is to parameterize simple models to construct useful DFAs – work that is still an active area of research.
All of the AC pathways mentioned above focus on the density-fixed case, relevant to Kohn–Sham DFT. However, if one notes the conjugate relationship between F[ρ] and E[v], a natural alternative is a potential-fixed AC, a possibility that has also been explored numerically.154,161 Since the density is no longer fixed, the calculations of the AC pathway are in the potential-fixed case much simpler to perform, but the noninteracting reference system (the bare nucleus system) is farther from a realistic electronic system than its Kohn–Sham counterpart. Recently, other ACs have been developed that do not insist on a fixed density along the AC pathway – see, for example, ref. 162 for an AC that recovers the Møller–Plesset series as its low coupling-strength expansion. The utility of the AC as a concept for understanding new theories based on these alternative pathways and their relative pros and cons compared with the Kohn–Sham approach underlines its importance as a concept in electronic-structure method development.
2.4.7 Kaupp and Arbuznikov.
The AC, which has already been invoked in contributions (2.4.2)–(2.4.6) as an important principle for the development of DFAs, is usually applied to the energy functional, where its existence is well established.163,164
Increasingly, however, interpolations along local ACs have been used, meaning that the coupling-strength (λ) integration is applied to the corresponding energy density or even to the exchange–correlation hole followed by integration over one and two spatial coordinates, respectively. While the existence of a “local AC” has never been proven rigorously, Becke argued that such an approach does not violate any basic principles and is just a matter of changing the order of integration that is valid for continuous functions165 – see also, for example, ref. 149.
One of the first applications of the local AC to the development of DFAs was to derive the B88 correlation functional166 Given that the global AC is the founding principle underpinning (global) hybrid functionals (see contributions (2.4.2), (2.4.4) and (2.4.6) above),124,128,167 a local AC should be relevant for local hybrid functionals (LHs) with position-dependent exact-exchange admixture. Let us mention in passing our early attempts to derive local mixing functions for LHs from local AC interpolation.168 Other important hyper-GGA functionals simulate strong correlation effects and also make use of local interpolations.165,169
Most notable in this context are recent efforts to include the SCE limit (λ → ∞) of the AC by local AC interpolation.149,170–172 Importantly, the local AC approach has advantages compared to the global AC in terms of achieving size-consistency for DFAs in the presence of degeneracies.173
2.4.8 Kronik.
An important concept that I have found to be very useful is that of generalized Kohn–Sham theory, introduced by Seidl et al.67 This involves mapping of the many-electron system onto a partially interacting model system, represented by a single Slater determinant, such that the ground-state electron density is conserved. The original Kohn–Sham theory then emerges as a special case of generalized Kohn–Sham theory, where the partial interaction is set to zero.
Generalized Kohn–Sham theory, recently extended to both TDDFT174 and ensemble DFT,175 provides a useful viewpoint that rigorously justifies the use of nonmultiplicative potentials. In particular, this means that the use of Fock-exchange potential operators (and variants thereof) in hybrid functionals (both global and range-separated), originally viewed as an ad hoc and theoretically unjustified merger of Kohn–Sham and Hartree–Fock theories, are rigorously derived and justified within generalized Kohn–Sham theory.176 While, for a given system, there is only one exact Kohn–Sham map, there are infinitely many partially-interacting systems to which an exact generalized Kohn–Sham map may be formed.68 This added flexibility has been found to be useful for spectroscopy – in particular, for choosing generalized-Kohn–Sham maps in which the derivative discontinuity is eliminated; see elaboration in contribution (4.1.5).
2.4.9 Gritsenko.
A useful concept of Kohn–Sham DFT is the meaning of the energies of the Kohn–Sham orbitals. According to the Kohn–Sham analogue of Koopmans’ theorem,70 the energy εi of the occupied Kohn–Sham orbital ϕi can be interpreted as approximate relaxed vertical potential Ii of the primary ionization, εi ≈ −Ii. The quality of this approximation is better for the outer-valence Kohn–Sham orbitals, with equality for the HOMO. The deviation of εi of the lower-lying Kohn–Sham orbitals from −Ii is, primarily, due to the spectroscopically-averaged contributions from ionization of the corresponding “shake-up” satellites.71 The energies εa of virtual Kohn–Sham orbitals ϕa include the “excitonic” type particle–hole interaction; see contribution (2.2.3). The difference εa − εi therefore serves as a good-quality zero-order estimate of the corresponding excitation energy from TDDFT. With the electron affinity provided by the energy of the anionic Kohn–Sham HOMO, the Kohn–Sham orbitals deliver all the important one-electron quantities.
2.4.10 Baerends.
The formal theory – beginning with the Hohenberg–Kohn theorem – is clear enough. It offers the prospect of finding important properties, notably the energy, as functionals of the electron density. However, the functional relation between the density and the energy remains obscure. The theory tells us that the density uniquely determines the energy (or rather, that each ground-state density is associated with a specific energy), but it tells us nothing about the precise relation. When two densities are close to each other (given some topological definition of distance between densities), there is no guarantee that the corresponding energies are also close. In other words, we do not (yet) know how to derive from the one-electron density information on the pair density, which determines the (correlation) energy. It is a fundamental weakness of the theory that it provides no clue to the solution of this problem.
In ab initio quantum chemistry, the route that is followed, in many different ways, consists of finding computationally feasible and sufficiently accurate approximations to the full configuration-interaction (FCI) solution. One may call this a mathematically-oriented approach. One can view DFT as an attempt – maybe often unconsciously – to follow the route of finding physical models for the pair-correlation function. The largest part of the LDA functional is the exchange functional, which is practically the same as Slater's original ρ4/3 approximation. Slater derived his “exchange hole” from a simple model (local hole of constant depth integrating to −1) which leads to practically the same exchange energy expression (with ρ4/3 density dependence) as the homogeneous electron gas of LDA. But the Slater construction shows that the interpretation is not necessarily that of an electron-gas exchange approximation. Indeed, it has been realized that this simple local hole is much more like an exchange–correlation hole – for instance, accounting for considerable left–right correlation in the chemical bond (which is why it soon was called an exchange–correlation approximation).69 The Slater (or LDA) hole yields much better bond energies for prototypical diatomic molecules than the exchange hole of the Hartree–Fock model: bonding changes from severe underbinding in Hartree–Fock theory to some overbinding in LDA. Also, the systematic errors in frequencies and bond distances that characterize the Hartree–Fock model disappear. This tells us that modelling of the exchange–correlation energy may not be so difficult after all.
The major improvement in the step from LDA to GGA comes from improved modelling of the exchange approximation. Becke's parameterization was fitted to reproduce the exchange energies of the rare-gas atoms, and Perdew's nonempirical GGA approximation of the exchange hole likewise considerably improved atomic exchange energies. The main contribution to the success of GGA (improvement over LDA) for bonds in simple diatomic molecules originates from these exchange improvements. This is mystifying since one would expect the better exchange approximation of GGA to reproduce more closely the poor Hartree–Fock results. Apparently, the GGA exchange improvements have turned the exchange holes into better exchange–correlation holes. So, there is still a considerable lack of precise understanding why the most successful models work, which perhaps explains the lack of consistent improvement beyond the GGA level. On the other hand, the “physical route” to the correlation problem by modelling of the exchange–correlation hole is hopefully a fruitful way forward that can be pursued independently of the clarification of the mathematical intricacies of DFT.
2.4.11 Gori-Giorgi.
Besides the argument that the exchange–correlation hole is much more localized than the exchange hole,69 other possible ways to understand the interesting point made in contribution (2.4.10) about fitting exchange on atoms and getting correlation in molecules could be:
(i) the exchange energy functional (and the exchange hole) changes linearly under uniform coordinate scaling. The correlation energy (and hole), by contrast, does not exhibit any simple scaling. However, when the electron–electron interaction becomes dominant with respect to the kinetic energy (see contributions (2.4.5) and (4.5.8)), then the exchange–correlation energy scales again as the exchange energy.170,177,178 It might thus make sense to have an exchange-like functional to capture (at least part of the) static (left–right) correlation.
(ii) More recent work by Burke, Perdew and coworkers (see ref. 179 for a recent review) has clarified the sense in which LDA is a universal limit for coulombically bound systems, with exchange as the leading term.
2.4.12 Krylov.
Reduced quantities, such as state and transition density matrices, natural orbitals, natural transition orbitals (NTOs), and Dyson orbitals, are very useful for understanding what DFT can and cannot do.74 These objects are also useful for making rigorous connections between DFT and wave-function theories, as well as for interpretation.
For example, NTOs afford a unified and rigorous description of electronic transitions in terms of MO theory, which is also experimentally verifiable, noting that observables such as absorption cross-sections can be rigorously expressed in terms of matrix elements between hole and particle NTOs. By using natural orbitals and their occupations, one can compare such properties as diradical character and the number of effectively unpaired electrons;180 although not observable, these quantities are useful for understanding the underlying electronic structure and for judging whether DFT captures the physics of the problem.
2.4.13 Krylov.
The observation that the response of the density of one electronic state (e.g., the ground state) contains the information about the entire spectrum of the system is both an opportunity for useful extensions and a liability in the context of the applicability of the theory. This observation has been used to extend Kohn–Sham DFT to describe excited states via TDDFT.
The same observation, coupled with the fact that the quality of the response of the density depends on the quality of the density, also enabled the extension of Kohn–Sham DFT to electronic structures that have multiconfigurational character and, therefore, are not well described by the single determinant. Although with exact Kohn–Sham DFT, we should be able to treat any type of electronic structure, current DFAs implicitly rely on the expectation that a single Slater determinant provides a good zero-order representation of the wave function; consequently, most Kohn–Sham DFAs fail when the electronic structure is multiconfigurational.
In the spin-flip DFT (SF-TDDFT) approach, the “difficult” multiconfigurational states (such as diradicals, molecules with broken bonds, transition metals) are described by means of spin-flipping excitations from a well-behaved high-spin reference state.181–183 In exact DFT, this approach should yield identical results to the traditional Kohn–Sham treatment – however, with the current incarnations of Kohn–Sham DFT, the SF-TDDFT method provides an effective solution to certain types of multiconfigurational states.
Thinking in terms of response properties also helps to understand when to anticipate potential problems. For example, while a TDDFT calculation may yield excellent excitation energies for a few valence states of interest, it may fail miserably in describing nonlinear properties, such as two-photon cross-sections, if the chosen functional does not treat (higher-lying) Rydberg states correctly.
2.5 What useful concepts of electronic structure theory have emerged from DFT?
2.5.1 Gill.
Inspection of the electronic Schrödinger equation for a large molecule does not lead one to anticipate that most of its interesting properties can be partitioned into almost-additive contributions from its various parts. As a result, there is an apparent inconsistency between the baroque complexity of quantum chemistry's many-body framework and the simplicity and predictive power of chemistry's “functional group” paradigm. Kohn–Sham DFT models, in which the exchange–correlation energy is approximated by an integral over all space of a function of the electron density, have partially bridged that conceptual gap.
2.5.2 Kronik.
Regarding contribution (2.5.1): interestingly, decades after his seminal DFT work, Walter Kohn formalized the idea of almost-additive local contributions by introducing the concept of “nearsightedness” of electrons in many-atom systems; see also contribution (5.4.7). Nearsightedness means that (with some caveats), for a fixed chemical potential, local electronic properties, such as the density, ρ(r), depend significantly on the effective external potential only at nearby points.184,185
2.5.3 Ayers, Chattaraj, Chermette, De Proft, Fuentealba, Geerlings, Liu, Vela, and Yang.
In the variational equation of DFT, the Lagrange multiplier μ was identified by Parr and coworkers in 197847,186 as the partial derivative of the energy E with respect to the number of electrons N, at constant external potential v(r), |  | (15) |
The chemical potential is the negative electronegativity χ = −μ by the Iczkowski–Margrave definition187 and reduces to the Mulliken electronegativity in a finite-difference approximation. As the electron density ρ(r) can be shown to be equal to the functional derivative of the energy with respect to the external potential, | 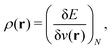 | (16) |
two basic quantities μ and ρ(r) can thereby be seen as responses of the energy to perturbations in N and v, respectively.
This observation forms the basis of conceptual DFT where, starting from the energy functional E[N,v] for atoms, molecules, and the solid state, derivatives of the type
are identified as response functions of the system's energy to perturbations in N and v, important for chemical reactions, with μ and ρ being the first-order (n = m + m′ = 1) responses. Second-order properties (n = 2) like the chemical hardness,
|  | (17) |
and its inverse, the chemical softness
S = 1/
η, the Fukui function
| 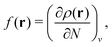 | (18) |
the linear response function
| 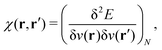 | (19) |
and even third order properties, with
| 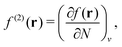 | (20) |
as the most representative member the dual descriptor, followed. All of them have proven their merits as concepts in the electronic-structure theory of atoms, molecules and the solid state,
188,189 emerging in a natural way in conceptual DFT which, on the basis of the initial identifications, forms an integral part of DFT.
All these response functions and some others derived from the energy function E(N), of which the electrophilicity ω is the most eminent representative,190,191 were shown to bear chemical relevance. To give some examples: the chemical hardness η in eqn (17) was identified with Pearson's hardness, while the Fukui function f (r) was recognized as a generalization of Fukui's frontier MO concept, its product with the total softness, the local softness s(r) = Sf(r) being a local indicator for soft regions in a molecule.
The first conceptual development of the chemical potential μ was based on the assumption that the fundamental functional E[N,v(r)] is differentiable everywhere for both variables.186 Subsequently, the exact piecewise linear conditions at fractional particle numbers were established originally by Perdew, Parr, Levy, and Baldus (PPLB)18 based on grand canonical ensembles at zero temperature and later by Yang, Zhang, and Ayers based on pure states with degeneracy.20
The piecewise linearity of E[N,v(r)] with respect to N means that the derivatives at integer electron numbers are discontinuous. In particular, at a given integer N, the chemical potential μ = (∂E/∂N)v, the Fukui function f (r) = (∂ρ(r)/∂N))v and other related quantities are discontinuous, the corresponding left and right derivatives being different. In view of this discontinuity, use of the derivative notation is best understood with an underlying finite-difference mathematical definition,47,192 where e.g. the right derivative is obtained by evaluating E(N + 1) − E(N). This interpretation is particularly important for second derivatives such as the chemical hardness in eqn (17).47 Since the chemical hardness describes the change in the first derivative at an integer electron number N, it will be zero or infinite at N and have no physical meaning unless interpreted in the above finite-difference manner. The PPLB condition is thus the foundation for the discussion of derivatives. It leads to the identification of the left and right chemical potentials with the ionization energy I and the electron affinity A, respectively.18 This identification was used to establish the physical meaning of the HOMO and LUMO orbital energies as the density-functional prediction of −I and −A, respectively, associated with the functional approximation used.21
2.5.4 Liu.
The use of density functionals to quantify and rationalize traditional chemical concepts and physiochemical properties is an ongoing research topic in DFT.193 The first example was by Nalewajski and Parr,194 who proved that the Hirshfeld partitioning (the Hirshfeld charge) arises from the constrained minimization of information gain (the Kullback–Leibler divergence, an explicit density functional), subject to the normalization condition of the total electron density.
Steric effects have been quantified in DFT by the Weizsäcker kinetic energy functional.195 Its functional derivative has been employed to predict stereoselectivity.196 Pauli energy has been validated as a robust identifier for double, triple, quadruple, and even higher covalent bonds.197
2.5.5 Staroverov.
The electron localization function (ELF)198,199 and related tools for analysing the nature of chemical bonds200 come from DFT and are now ubiquitous in computational chemistry. Other examples include the average local ionization energy201–203 and classical turning surfaces of atoms and molecules.204
2.5.6 Pernal.
The concept of the AC, conceived within the DFT framework and successfully used to develop approximations to exchange–correlation functionals – see contributions (2.4.2)–(2.4.7) – has inspired the development of methods for calculating the dynamical correlation energy in wave-function theories.205 In the general AC theory developed in ref. 205 and elsewhere, one is not restricted to adopting a noninteracting Kohn–Sham system as a reference system, corresponding to a vanishing coupling constant. If, instead, the reference wave function consists of a combination of Slater determinants and orbitals are partitioned into noninteracting groups (most commonly into doubly occupied (inactive), fractionally occupied (active), and unoccupied (virtual) orbitals, as in multiconfigurational self-consistent-field (MCSCF) theory) then, by following the AC path, the limit of no correlation is smoothly connected with the full electron-correlation limit.
A difference between AC-DFT and multiconfigurational AC theory is that, in the former theory, the electron density is fixed to the exact density by a local one-body potential varying along the AC path, while in the latter, the condition of a constant density is imposed as an approximation. AC-based correlation energy approximations have been used with MCSCF, complete-active-space SCF (CASSCF), density-matrix-renormalization group (DMRG) and geminal theories.205,206 These multiconfigurational AC approximations rely on the ACFD formalism and the (extended) RPA. An appealing feature of the multiconfigurational AC methods is that only one- and two-electron reduced density matrices are needed, as opposed to perturbation approaches such as complete-active-space second-order perturbation theory (CASPT2) or N-electron valence-state second-order perturbation theory (NEVPT2), which require three- and four-body RDMs.
It has been recognized that, in the general AC theory, the reference state need not be an electronic ground state as long as it is not degenerate. This has motivated the development of AC methods for excited states, which recover the dynamical correlation energy for a specific state.207 It may be worth exploring if a similar approach could be developed for Kohn–Sham DFT, taking an excited Kohn–Sham determinant as the noninteracting system. The clear advantage over TDDFT would then be the description of states of double-excitation character.
2.5.7 Pernal.
There has always been an intuitive understanding that short-range correlation relates to the electron cusp in the wave-function description, while long-range correlation plays a role when electron pairs dissociate or when van der Waals bonds are formed. Range separation of electron correlation has gained mathematical rigour in the range-separated multiconfigurational formulation of DFT.208,209 In range-separate multiconfiguational DFT (RS MC-DFT), only the long-range part of the electron interaction operator, which is bounded at electron coalescence and is characterized by a proper Coulomb tail, is retained in the many-body Hamiltonian. Consequently, a wave function in RS MC-DFT has no electron cusp, which greatly simplifies the many-body problem – approximate wave functions call for shorter configuration-interaction (CI) expansions than when the full Coulomb interaction operator is used.
The long-range electron correlation energy naturally emerges as the difference between the energies of the FCI wave function and the chosen model (CI, CASSCF, etc.).210,211 The short-range correlation energy is rigorously defined as
| 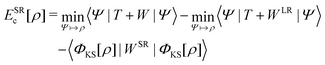 | (21) |
and depend on the underlying range-separation of the electron interaction operator,
W =
WLR +
WSR. Approximations for the short- and long-range correlation energies can be developed independently. One of the appealing features of RS MC-DFT is that wave-function models and existing approximate exchange–correlation functionals may be adapted to a range-separated electron interaction.
The rigorous range separation of electron correlation has led to a proliferation of wave-function methods using short-range exchange–correlation functionals as an inexpensive way of accounting for dynamical (short-range) correlation, thereby improving their accuracy and/or efficiency.212 A promising direction of development of DFT via its merger with wave-function theory is enabled by gaining access to a correlated two-particle local function – the on-top pair density – which can be used as a variable in correlation functionals in addition to the electron density; see, for example, ref. 213 and contribution (4.1.1).
2.5.8 Grimme.
In the early days of quantum-chemical method development for electronic structure, drastically simplified methods for large systems termed “semiempirical” like MNDO, AM1 or PM6214 were derived as approximations to Hartree–Fock theory.214 Usually, minimal atom-centred atomic-orbital (AO) basis sets and severe integral (multipole) approximations were applied, enabling a reasonably accurate, extremely fast treatment of mostly organic molecules. Because of the applied zero-differential-overlap (ZDO) approximation and their Hartree–Fock origin, these methods are not robust for more complicated electronic systems like, for example, the important class of organometallic catalysts.
This situation changed in the mid 1990s when the tight-binding (TB) semiempirical theory was proposed as an approximation to Kohn–Sham DFT,215,216 based on previous work of Foulkes and Haydock.217 The current theoretical view on TB methods, which in the meantime have been consistently parameterized for the whole periodic table,218 is based on a Taylor expansion of the total energy E around a reference density ρ0, constructed as a sum of atomic valence densities:
| E[ρ] = E(0)[ρ0] + E(1)[ρ0,δρ] + E(2)[ρ0,(δρ)2] + E(3)[ρ0,(δρ)3] + … | (22) |
where the fluctuations δ
ρ are expressed in terms of multipoles and the series is usually truncated at third order. Short-range repulsive, exchange–correlation as well as dispersion effects are typically described using empirical pairwise potentials.
The speed-up of a TB calculation compared to, for example, a regular GGA(PBE) DFT calculation, is about three orders of magnitude, at little loss of accuracy for common properties like electronic and geometric structures. Thermochemical data and, in particular, conformational energies are generally not so well described, which is at least partially attributed to the small (mostly minimal) AO basis sets employed.218 The development of more accurate, but still sufficiently fast, TB methods is an important future field that should take advantage of more advanced DFAs.
2.5.9 Aradi and Frauenheim.
The efficient DFT-based TB methods are not restricted to “classical” DFT and to ground-state properties only. Several DFT extensions have been successfully ported into the density-functional tight-binding (DFTB) framework215 and implemented in various program packages. The DFTB version of those extensions (hybrid functionals219 TDDFT,220 Ehrenfest dynamics,221 Green's-function-based electron transport,222etc.) are typically several orders of magnitude faster than their DFT counterparts, allowing for a more efficient treatment of large systems and/or long time scales.
2.5.10 Köster.
For decades, Xα and Kohn–Sham DFT methods have served as a playground for the development of density-fitting methods.223,224 Commonly used approaches are the variational fitting of the Coulomb225 and Fock226 potentials. With these fitting approaches, the formal scaling of first-principles Hartree–Fock and Kohn–Sham calculations is reduced by one order of magnitude without lowering the accuracy of the underlying methodology. To avoid linear-algebra bottlenecks associated with variational density fitting, iterative Krylov subspace solvers are advocated.227
A further simplification of Kohn–Sham DFT implementations can be achieved by using the approximate density from the variational fitting of the Coulomb potential for the evaluation of the exchange–correlation energy and potential.228,229 The resulting energy expression remains variational and yields optimized structures and relative energies that are almost indistinguishable from standard Kohn–Sham approaches, but at a substantially reduced computational effort. The extension of this auxiliary DFT (ADFT) approach to perturbation theory permits first-principles molecular property calculations of systems with up to thousand atoms – for example, second-order analytic energy derivatives.230 Most recently, ADFT also serves as platform for the development of new DFAs.
2.5.11 Galli.
DFT in its approximate Kohn–Sham formulation has been key in the description of chemical bonding in condensed systems, including solids and liquids, in different phases and under different thermodynamic conditions. It has been especially critical for understanding trends in chemical bonding in solids as a function of temperature, pressure and, more recently, even external fields, although we are still far from having accurate descriptions in many cases. It is also important to note that the use of approximate DFT (beginning with LDA) is at the basis of the development of first-principles molecular dynamics and hence the ability to study finite-temperature properties of materials.
Orbitals obtained from the solution of the Kohn–Sham equations are also at the basis of most many-body perturbation theories solving, in approximate manners, the Dyson and Bethe–Salpeter equations (GW and BSE methods). These approaches have brought tremendous progress in understanding properties of solids, in spite of some lack of accuracy, and almost all of them (for solids) are based on DFT.
2.5.12 Reining.
Further to the usefulness of DFT as starting point for Green's functions methods, I would like to point out that combinations, for example, approximations for vertex corrections beyond the GW method are derived from DFT and TDDFT.
3 Density functional approximations
3.1 What strategies have been useful in constructing DFAs?
3.1.1 Chermette.
It is worth recalling that DFAs span a wide range, from quasi ab initio to fully semiempirical status. The first category, promoted by Perdew and collaborators, introduces parameters that are almost all fixed by theoretical constraints. This approach, which allows us to use the resulting exchange–correlation functionals in exotic systems with some confidence (assuming universality of the functional), may, however, involve constraints that can be questioned, as being not necessarily appropriate for molecular systems – for example, the uniform-gas limit. This constraint was removed by Handy et al.231 in the OPTX exchange functional and is a reminiscent of the X-alpha functional. This approach, coupled to a correlation functional (e.g., in the OPBE functional), may lead to a good description of spin states.232
The second category, promoted by the Minnesota team, has led to functionals involving up to 64 parameters. These functionals may be very accurate for – but limited to – small classes of molecular systems and properties. In a paper involving 200 combinations of exchange and correlation functionals,233 Mardirossian and Head-Gordon compared the performance of these exchange–correlation functionals applied to 82 data sets, with and without dispersion corrections, and documented the scattering of the performance among the properties for given classes of molecular systems.
3.1.2 Perdew.
The original local density approximation (LDA)65 for the exchange–correlation energy was based upon fitting to an appropriate norm or system for which the approximation is exact: the electron gas of uniform density. Nonempirical generalizations of LDA have been constructed by satisfying additional exact constraints or mathematical properties derived from exact but impractical expressions (see, for example, ref. 6, 100 and 120) for the functional. For example, the PBE functional234 satisfied 11 exact constraints, and the SCAN functional235 was constructed to satisfy all 17 known exact constraints that a meta-GGA can satisfy. The SCAN functional also fits generalized appropriate norms, such as the hydrogen atom and neutral atoms of large atomic number. By contrast, empirical constructions are fitted to experimental or higher-level computational data (usually for molecules), which can make them more reliably interpolative and less widely predictive than the nonempirical functionals.
Of course, these two approaches are often combined. The most accurate functionals (including meta-GGAs, hybrids, and RPA-like functionals) generalize Kohn–Sham theory67 by employing as arguments of the energy density not only the electron density and its gradient, but also the occupied or even the unoccupied orbitals or one-electron wave functions, and by optimizing those arguments. A sometimes important but seldom discussed step in the development of a functional is “deconstruction”: removing what is wrong or unnecessary, as in the transition from gradient expansions to generalized gradient expansions.123
3.1.3 Chermette.
As suggested in contribution (3.1.2), in case of semiempirical functionals (see contribution (3.1.1)) which may involve dozens of parameters, it is especially important to remove all parameters with statistically insignificant weights in the fits. The reason is that these parameters introduce noise in the calculations and restrict severely the application domain to the classes of molecular systems that have been used in the training set. Approaches like variance analysis spring to mind, but more elaborate methods may also be used – for example, Mardirossian and Head-Gordon236 have detailed the strategy they used for a combinatorial approach to handle the problem, which is made more complicated by the fact that the objective function to be optimized (usually a least-squares sum) is a (linear) combination of inhomogeneous quantities (energies, structural data, other physical properties) that are combined with ad hoc weights in the objective function.
3.1.4 Adamo and Ciofini.
The terms “empirical” and “nonempirical” used above deserve some clarification. For us, the term “nonempirical” denotes those DFAs whose internal coefficients are not determined by an error minimization relative to external reference data sets (experimental or theoretical), but instead are fixed using only constraints derived by theory. The term “empirical” denotes, by contrast, those functionals whose coefficients are determined by a parameterization procedure. However, since these latter functionals may also respect some theoretical constraints, we prefer to use the term “semiempirical” to underline their theoretical foundation. In our opinion, these two terms, “nonempirical” and “semiempirical”, are not measures of quality, but rather indicate how the functional has been developed. Between these two classes, the term “minimally parameterized” is also used, to underline that an effort has been made to reduce the number of functional parameters, as mentioned above.
3.1.5 Loos.
The uniform electron gas, a hypothetical infinite substance where an infinite number of electrons “bathe” in a (uniform) positively charged jelly of infinite volume, is one of the success stories of DFT and, in particular, the parameterization of its correlation energy as a function of the density has been enormously useful for the construction of DFAs.237 From a more general point of view, model systems (especially the ones with uniform electron densities) provide new ways for improving and testing DFAs.238 In this regard, finite uniform electron gases (where electrons are confined to the surface of a sphere) can be seen as an extension of the conventional “infinite” version thanks to additional degrees of freedom coming from the tunable “finiteness” of the electron gas.239,240
3.1.6 Reining.
I would like to elaborate on contribution (3.1.5) of Loos: using results from the uniform electron gas has been invaluable for the success of DFT. Here, we should stress how much DFT has profited from other people's work and methods – in particular, from the quantum Monte Carlo calculations of Ceperley and Alder.241 This is important: trying to use the strong points of other methods – and trying to use the knowledge of model systems for the real materials we are interested in. This strategy could be extended much further.242
3.1.7 Savin.
An important decision in constructing DFAs is the choice of parts to be approximated by a closed form. Hohenberg and Kohn already considered it necessary to treat exactly the Hartree term, thus treating the electrostatic contribution to the energy correctly.5 Kohn and Sham decided to leave the DFA for exchange and correlation (Section II.A of their paper), or for correlation only (Section II.B).65 One can discuss having a part of exchange treated by orbitals and a part by DFAs, as done in hybrid DFT.124 One can also decide to treat only a part of correlation by a DFA – see, for example, ref. 243.
3.1.8 Staroverov.
The analytic derivations of density functionals for model systems that gave us the Dirac exchange and Thomas–Fermi theory, as well as derivations of DFAs from model exchange–correlation holes166,244 have been seminal. The success of this analytic approach seems difficult to sustain in DFT, but that is almost certainly because not everything has been tried. Attempts to connect DFT with wave-function methods explicitly can also result in effective practical methods, especially for calculations of accurate Kohn–Sham potentials.245–247
3.1.9 Johnson.
Explicit modelling of the separate exchange, dynamical, and nondynamical correlation holes has been a very successful strategy in functional development. The (exact) exchange–correlation energy can be written in terms of the exchange–correlation hole as: | 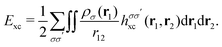 | (23) |
The total exchange–correlation hole can be decomposed into separate exchange, parallel-spin correlation, and opposite-spin correlation holes. Real space models can then be proposed that obey known constraints, such as normalization, as well as density and curvature constraints at a reference point.244
Another useful strategy in the development of GGAs is to enforce a large-gradient limit of the enhancement factor,248,249 which ensures an accurate treatment of nonbonded repulsion in van der Waals complexes.250,251 Such functionals are capable of high accuracy for modelling intermolecular interactions in both gas-phase and solid-state systems, when paired with a density-functional dispersion correction.252
3.1.10 Adamo and Ciofini.
It is worth underlining how the respect of known theoretical constraints can help in the development of DFA approximations. In this sense, we should first mention Becke's half-and-half model, which introduces the AC at the heart of functional construction.124 Another example is the PBE0 functional,167,253 defined based on the ansatz of Perdew and co-workers for the form of the AC path.130 The relationship between the AC ansatz and numerical performance has been explored by Yang and co-workers.131 Later, the introduction of the GL limit (see contribution (2.4.4))133 in functional development has led to the definition of double-hybrid functionals, including some nonempirical approaches.254–256
Interestingly, since the introduction of Becke's half-and-half model, constraints derived from properties of the AC have been used for functional development, thereby avoiding introducing variables to be fitted to external (not theoretical) data. In other words, increasing the number of theoretical constraints in going from local to hybrid functionals leads to improved numerical performance (at least within the same functional family) for a large number of chemical properties.257
3.1.11 Sun.
In the approach of using exact constraints to construct DFAs mentioned in contribution (3.1.2), two different levels of exact constraints have been successfully used. For example, the PW91 GGA functional was constructed to satisfy the exact constraints of the exchange–correlation hole, while the very similar PBE GGA functional was constructed to satisfy exact constraints of the exchange–correlation energy. The SCAN meta-GGA functional was constructed by satisfying the exact constraints of the exchange–correlation energy but guided also by properties of the exchange–correlation hole.
3.1.12 Gritsenko.
A useful strategy in constructing approximations to the Kohn–Sham exchange–correlation potential is the statistical averaging of (different) orbital potentials (SAOP). The SAOP exchange–correlation potential, which statistically averages the potential with the correct Coulombic asymptotics and the potential arising from the step structure of the atomic and molecular electron shells, produces a good-quality estimate of vertical ionization potentials and yields a high-quality zero-order estimate of excitation energies within TDDFT.258
3.1.13 Romaniello.
The link between DFT and many-body perturbation theory (MBPT) based on Green's functions has been particularly beneficial. The Sham–Schlüter equation (SSE),259 which relates the Kohn–Sham potential of DFT to the self-energy of MBPT, has given several insights into approximations to vxc. As an example, one can easily retrieve the OEP equations from the linearized version of the SSE.260,261
Also, the time-dependent version of the SSE262 has been very useful in the context of TDDFT. For example, one can show that the TDDFT exchange–correlation kernel fxc can be written exactly as two contributions, one responsible for the shift of the Kohn–Sham band gap to the fundamental gap and the other accounting for excitonic effects.263 This splitting has been recently used to calculate accurate optical spectra of semiconductors and insulators within a pure Kohn–Sham TDDFT framework – that is, without invoking empirical information nor theory beyond Kohn–Sham DFT (e.g., GW theory) to correct the Kohn–Sham gap.264
3.1.14 Galli.
One of the outstanding open problems in defining approximate density functionals pertains to the description of the electronic properties of solid–solid and solid–liquid interfaces. When systems with different dielectric properties are interfaced – for example, a metal with an insulator or a semiconductor such as silicon with an insulating liquid such as water – none of the existing functionals can accurately describe band offsets and other electronic properties. This issue can be mitigated by carrying out GW calculations starting from DFT orbitals (for nonmetallic systems) However, this GW@DFT approach does not work when the underlying wave function provided by DFT turns out to be too inaccurate as a starting point – for example, for some transition-metal oxides.
A useful strategy for deriving approximate functionals for interfaces may be based on an approximate treatment of the screened Coulomb interaction and of dielectric matrices; the latter may then be used to derive approximate hybrid functionals with parameters that capture how the dielectric screening varies in different parts of the system (see, e.g., ref. 265 and references therein).
3.2 How accurate do we need DFAs to be?
3.2.1 Jones.
Table 2 shows that “accuracy” has different meanings in different contexts. If one is interested in properties such as cohesive energies and structures in different phases of extended systems, then it is impossible in practice to determine accurate total energies using DFT methods. If the goal, however, is to shed new light on a problem or to make unbiased predictions, then DFT calculations can be a reliable partner. They share with other approaches the benefits of error cancellation, and users of molecular dynamics welcome the fact that forces are straightforward to calculate and consistent with variations in the energy.
Table 2 Common accuracy objectives
Property |
Accuracy required |
Savin: mean value of the experimental uncertainties compiled in ref. 266 for over 500 molecules containing elements with Z < 18. See also ref. 267.
Savin: Q95, cf. contribution (3.3.12), obtained from the experimental uncertainties compiled in ref. 266 for over 500 molecules containing elements with Z < 18.
Savin: heat of formation divided by (the number of atoms −1), justified by the mean of the values obtained by detaching successively one atom after the other.
Grimme: molecular total energy difference for the same covalently bound structure but with different three-dimensional shape normally obtained by rotation around covalent bonds.
Schwerdtfeger: based on ref. 267.
Kronik: an experimental accuracy of 0.1 eV in band gap measurements is possible, as well as desirable, but not at all trivial and may require the combination of several measurement techniques. Many reported experimental results, especially for insulators, do not necessarily reach this level of accuracy. Also, some reported band gaps arise from correction terms to optical gap values. Furthermore, experimental band gaps are also influenced by electron-nucleus coupling, sometimes quite significantly. This should be taken into account when comparing to results of electronic structure theory that do not include such coupling.
Helgaker: the uncertainties in experimental bond lengths depend strongly on the experimental technique used – an accuracy of 1 pm for covalent bonds of first-row atoms is a reasonable target for computation. For benchmark data of wave-function methods, see ref. 268.
Draxl: for vibrational frequencies, even semilocal DFT does already very well, if computed consistently (i.e., for the optimized geometry269). The situation is more tricky for intensities, as these are typically not measured for solids. The situation may be different for molecules; thus a distinction would be needed. Note that intensities can’t be obtained by DFT alone.
Kaupp: the necessary and achievable accuracy for shieldings and relative shifts differs from nucleus to nucleus and for different applications. The best way to report the accuracy that allows a comparison between different nuclei, is to give relative deviations in %, normalized to the shielding or shift range of a given nucleus (either computed or experimental). For meaningful accuracy, this should not exceed a few percent, sub-percent accuracy is better, and is achievable at least for light main-group systems. This is not yet the case for transition-metal nuclei.
Schwerdtfeger: these accuracies are expected from any decent ab initio calculation. For comparable accuracies for EFGs achieved by coupled-cluster methods see ref. 270, for DFT see ref. 271 and 272.
|
Heats of formation |
1a kcal mol−1 |
|
3b kcal mol−1 |
Heats of formation (“intensive”)c |
0.3a kcal mol−1 |
|
1.6b kcal mol−1 |
Conformational energies |
0.1d kcal mol−1 |
Barrier heights |
1e kcal mol−1 |
Ionization potentials |
1e kcal mol−1 |
Band gaps |
0.1f eV |
Excitation energies |
0.1f eV |
Bond lengths |
1g pm |
Vibrational frequencies |
<3h cm−1 |
Shielding constants |
0.5–5%i |
Dipole moments |
0.1–0.2j D |
Dipole polarizabilities |
0.5–1j a.u. |
Electric field gradients |
0.1–0.2j a.u. |
In extended systems, it is often impractical to repeat calculations with different functional approximations [see contribution (2.1.16)], and it is essential to develop a level of “trust” [see contribution (3.2.7)] in the approximations one uses and a feeling for their limitations. My own applications over several decades show a clear preference for main-group elements, which might imply less trust in the ability of particular DFAs to describe transition and rare-earth elements. This is perhaps not surprising, since some DFAs describe energy differences in the corresponding atoms very poorly.
3.2.2 Schwerdtfeger.
The accuracy really depends on the property in question, on whether the corresponding operators sample the density more in the outer region or in the region close to the nucleus. I find Table 2 quite useful, but we should be reminded of some more problematic cases for properties such as polarizabilities or hyper-polarizabilities.
Moreover, if we have an incorrect long-range behaviour of the one-particle density, then the region close to the nucleus will also suffer because of charge conservation. As a result, properties like electric-field gradients (EFGs) are not so well described by currently available DFAs – the worst results are perhaps obtained for the late transition metals.271 To illustrate, the Cu EFG in CuF at the experimental bond distance is measured to be (in atomic units) −0.31(2), while some representative DFAs give +0.495 (LDA), +0.444 (PW91), and +0.146 (B3LYP). At the coupled-cluster CCSD(T) level, we have −0.439 (−0.341 if relativistic effects are included).270 To address this problem, the parameters in the CAMB3LYP functional can be tailored such that accurate results for EFG (and other short-range properties) are produced,272 but this is not a nice solution and no unique functional exists that performs well for all properties concerned.
One should also mention that, as for molecules,273 the performance of various DFAs for the solid state has been extensively analysed in the past – see, for example, ref. 274–276. Here, a few percent error range is typical for solid-state properties such as lattice constants, cohesive energies and bulk moduli if (for the heavier elements) relativistic effects and (for the lighter elements) phonon contributions are included. For finite temperatures, thermal effects need to be included as well.
3.2.3 Fuentealba.
Let me illustrate Schwerdtfeger's point regarding polarizabilities with some numbers. The dipole polarizability of a Li4 cluster has been calculated using the B3LYP and PW91 functionals, the values being (in atomic units) 355 and 394, respectively.277 But the experimental value is 327 – no explanation. More dramatically, for the Cu9 cluster, the calculated value is 295, while the experimental value is 984.278 Pathetic.
3.2.4 Calaminici.
The accuracies of static Kohn–Sham DFT dipole polarizabilities are usually in the range of 1%. However, the errors in dynamic dipole polarizabilities can be catastrophic – in particular, for planar conjugated systems. Here, the long-range behaviour of the functional used is critical.279 The polarizabilities of small metal clusters can be significantly influenced by temperature effects.280 Furthermore, the experimental references for static polarizabilities of such clusters are not always reliable. In particular, this is the case for the available measured copper-cluster polarizabilities in the literature.
3.2.5 Chermette.
As far as bond lengths are concerned, the accuracy can be better than 1 pm (perhaps has small as 0.3 pm) if the aim of the calculation is to compare bond lengths of organic isomers and conformers. On the other hand, if heavy atoms are present, 3 pm or even 5 pm can be considered fine, even with relativistic corrections added. There is room for improvement.
3.2.6 Grimme.
Chemically relevant energies span a huge range – from one tenth to hundreds of kcal mol−1. The often cited “chemical accuracy” of 1 kcal mol−1 usually refers to bond or atomization energies, which (for small systems) are on the order of a few hundred kcal mol−1. While this definition is appropriate for some thermochemical problems, it is inappropriate for others. For example, the very relevant conformational energies of typical pharmaceutical drug molecules with about 50 atoms are on the order of the thermal energy at room temperature (0.6 kcal mol−1). Hence, in practical applications, only errors less than about 0.1–0.2 kcal mol−1 are acceptable.281
Another aspect to consider here is that the most important primary application of current Kohn–Sham DFT in chemistry is probably the determination of equilibrium structures (Re) including those of chemical transition states. Even with rather simple DFAs (e.g., GGAs), basically no severe outliers are obtained, even in electronically difficult cases – a fact that, in retrospect, was extremely important for the development of computational chemistry. As such, newly proposed, empirical DFAs, should be carefully tested not only for energies but additionally for the computation of Re structures. Similar considerations hold for the computation of vibrational frequencies, which are of utmost importance for thermostatistical properties – for example, Gibbs free energies.
3.2.7 Savin.
It seems to me that in most cases, calculations – like experiments – are not carried out to obtain specific numbers, but to answer some questions. Furthermore, the methods of quantum chemistry do not provide error bars for our calculations. The expected accuracy is therefore what we have from our experience with methods, which may not apply to a specific case. This experience may be tainted by trust acquired over years and not revised by an active following of progress in the field.
Another aspect is that we may overemphasize the accuracy of what we take as a reference. Herbstein discusses several factors that may affect the measurement of such basic data as single-crystal unit-cell dimensions.282 Cioslowski et al. show that experimental error bars are often missing or can be quite large.266 Sometimes advanced wave-function calculations are not pushed far enough to be used as a reference.283
3.2.8 Adamo and Ciofini.
In some cases, determining how accurate DFAs need to be is probably even more difficult than determining how accurate existing DFAs are in fact for a given property. Indeed, even for a single, well-defined property, the target accuracy will depend on which question we aim to answer (as already pointed out by Savin in contribution (3.2.7)). The necessary accuracy will depend strongly on the type of “interaction with the real world” is desired, following the excellent classification given by Kronik in contribution (3.8.5): confirmation, interpretation, or prediction. In our experience, this is particularly true for the interpretation and prediction of excited-state properties of molecular systems.
There have been a huge number of publications assessing the performance of different DFAs within TDDFT for the prediction of excitation energies both using theoretical and experimental reference data – see, for instance, ref. 284–288. Nonetheless, two difficulties are becoming nowadays evident: the reliability of affordable theoretical reference methods for large molecular systems may be difficult to assess, and the fact that excitation energies may not be the only property needed to provide a full answer to a given chemical question.
Concerning the first point, thanks to a number of detailed studies that compare DFAs results with those obtained using different reference methods, it has become evident that, especially for complex molecular systems, assessing the accuracy of DFAs is also dependent on the choice of reference. By targeting an accuracy below a certain threshold in the excitation energy, one is probably simply targeting the error bar of the methods used as reference.289 Furthermore, errors depend on the type of excitations considered – for instance, local or charge-transfer excitations. Due to the different impact of the approximation used in a given DFA on the different types of excitation, it is very difficult to assess a global accuracy for this DFA in predicting excitation energies. More severely, (vertical) excitation energies are often not what one aims for as a chemical answer since the quantitative description of the photophysical properties of a given molecular system are related to the prediction of its entire spectrum (absorption or emission), practically manifested in the observed colour.290 In this case, the accuracy we would like to reach – and which is asked for in industrial application, for instance – is the sensitivity of human colour perception.291
To achieve this objective, one needs to combine a very high (and energy-dependent) accuracy in the excitation energy with a very good description of the band shape. This latter is mostly obtained using approaches enabling the description of the vibrational broadening, that is the vibronic coupling between ground and excited states. Previous studies291 have nonetheless demonstrated that the same DFA can seldom reproduce with the same accuracy both electronic excitation energies and vibrational broadening. Finally, the comparison with experimental data can become even more complicated if environment effects, usually modelled with approximate methods, have to be considered.292 This latter point is of course of relevance, for any theoretical approach used and not exclusively limited to DFT.
3.2.9 Barone.
Sufficiently accurate molecular structures are a prerequisite for the computation of thermodynamic, kinetic, and spectroscopic properties. In this connection, the latest-generation DFAs (hybrid and, especially, double hybrids) with dispersion corrections added perform a remarkable job for main-group elements, in noncovalent complexes and for transition states.293–295 Furthermore, the remaining errors are rather systematic and can be corrected for by linear regression, depending only on the atomic numbers of the involved atoms.296,297 As a matter of fact, energies and properties can usually be calculated very accurately at DFT geometries with negligible errors provided that the functional and the basis set are properly selected. The situation is more involved for transition metals, where comprehensive benchmarks are still missing.
From another point of view, comparison with experiment requires vibrational corrections to geometric parameters and zero-point energies. The situation for main-group elements is again very satisfactory, with latest-generation DFAs in conjunction with second-order vibrational perturbation theory (or anharmonic treatments) providing remarkably accurate results without the need for any scaling factor.294,296 The above remarks concern isolated molecules (or low-pressure gas-phase). The situation is more involved in condensed phases, where much work to improve the accuracy of the results is ongoing.
3.2.10 Piecuch.
While it is important to set accuracy targets for any quantum-chemistry approach, not only for methods based on DFT, it may be useful to keep in mind that some quantities, such as binding energies in weakly bound clusters, activation energies, and vibrational frequencies, to name a few examples, vary so much among the various systems (in the case of vibrational frequencies, even within a given system) that setting up fixed error limits is not necessarily helpful. In all such cases, the relative (percent) errors may be more informative when setting up accuracy standards.
For example, it is commonly accepted that achieving a 1 kcal mol−1 (chemical) accuracy for binding energies involving covalently bound molecular species is often desirable, but setting a similar accuracy target for activation energies, which can be on the order of 1 kcal mol−1 in some processes and more than 10 kcal mol−1 in others, may be misleading. Furthermore, one can have reaction mechanisms that involve larger and smaller barriers along the same reaction pathway or along multiple competing pathways. A 1 kcal mol−1 accuracy level does not work well for noncovalent interactions either. In fact, even the frequently mentioned value of about 0.1 kcal mol−1 may not be adequate in this case.
If we replace the error criteria for the binding energies in weakly bound species and activation energies by relative errors of, say, 5%, then we may be in a better position to judge and make recommendations regarding what method to use. Indeed, if the activation energies along the reaction pathway of interest are on the order of 10 kcal mol−1 or more, as in ref. 298, then ∼5% relative errors translate into total errors on the order of 0.5–1 kcal mol−1, which is good enough to understand the reaction mechanism. However, if the activation barriers are on the order of 1–5 kcal mol−1, or if we must decide if a particular reaction has a barrier or is barrierless, as in ref. 299, then a fixed accuracy criterion may be insufficient to make a proper recommendation regarding what method to use. Similarly, a fixed 0.1 kcal mol−1 criterion might be of little use for some noncovalent interactions, where there are many cases in which the interaction energies are as small as 1 kcal mol−1 or less and equally many cases in which the interaction energies are on the order of a few kcal mol−1 or more. A good illustration of the former situation is the magnesium dimer, which is an important weakly bound system in studies of ultracold and collisional phenomena and which is characterized by a binding energy of about 430 cm−1; see ref. 300 and 301. To properly understand this system, one must be able to reach an accuracy on the order of a few cm−1, which is a major undertaking, even for the highest levels of ab initio wave-function theory.301,302
The magnesium-dimer example is also a reminder that in setting accuracy targets, we should be careful about treating wave-function methods, including those based on coupled-cluster theory, as providers of reliable reference information. As is very well known, wave-function methods exhibit a much slower convergence with the basis set than methods based on DFT. Also, the treatment of core electrons is usually different in DFT and wave-function calculations. Wave-function calculations are often carried out with frozen core electrons, whereas DFT (putting aside the issue of relativistic effective core potentials) is an all-electron theory. Thus, judging DFAs by comparison with wave-function approaches may sometimes be misleading or questionable.303 Finally, the CCSD(T) approach, often regarded as a standard for high-accuracy calculations, fails not only in multireference situations, such as covalent bond stretching and biradicals, but also in many cases of noncovalent interactions, including the aforementioned magnesium dimer, where the CCSD(T) binding energy extrapolated to the complete basis-set limit has a substantial error.301,302 While the development of ab initio wave function methods can be well served by comparisons with FCI, the development of DFAs may be better served by comparisons with reliable experimental data.
The idea of setting up accuracy targets using relative (percent) errors may easily be extended to other properties in Table 2. For example, the aforementioned 5% error limit would work well for vibrational frequencies, including low-frequency and high-frequency modes. Clearly, depending on the nature of the application, one may replace the 5% target by a different target.
3.2.11 Kaupp and Arbuznikov.
Several contributions in this section indicate that accuracy depends on the type of property one looks at. While highly empirical DFAs have concentrated on relative energies relevant for chemical processes, a wide-ranging recent discussion has put electron densities into focus.304–311 Here, we should clearly distinguish different spatial regions in an atom, molecule or solid, as different requirements hold for the core, valence, asymptotic, and intermediate regions. For example, many (albeit not all!) empirical Minnesota functionals, which give excellent valence energies and probably reasonable valence densities, produce highly erratic hyperfine couplings for transition-metal nuclei312–314 and also perform poorly for NMR shifts and spin–spin coupling constants.315–317 A position-dependent admixture of Hartree–Fock exchange in local hybrid functionals318,319 seems to be one way to improve specifically properties of operators that act near the nuclei or far away from them – see contribution (4.1.10).
3.2.12 Trickey.
One of the most striking features of Table 2 is what is not there. Except for band gaps, there is nothing about solids, no cohesive energies, no bulk moduli, no crystalline phase-transition pressures. (We here assume that “bond lengths” can be interpreted generously as including lattice constants.) With the disclaimer that what follow are simply values that seem to be fitting from experience but not from study, plausible useful accuracy values seem to be 0.015 Å for cell constants, about 0.1 eV per atom for cohesive energies, ±4% for bulk moduli, and ±2% for transition pressures (assuming the crystal structures are correct). The main point is that work is needed on such criteria.
Add to that something little discussed in this roundtable – namely, that predictive screening of materials requires even-handed accuracy across states of aggregation. One must have the same computationally affordable functional and protocols for both the isolated molecular constituents and the condensed phases, with correspondingly consistently appropriate accuracy for both constituents and aggregates. It is of little or no use in first-principles computational materials physics to prescribe a highly sophisticated DFA of great accuracy for the molecular constituents that cannot be afforded in condensed-phase studies or is deliberately tuned (e.g., OPTX) to be accurate for molecules only.
3.2.13 Baerends.
A striking deficiency of almost all DFAs is the error of about 5 eV in the orbital energies. This is a much larger error (more than 100 kcal mol−1!) than in the total energy and unacceptable in view of the desired chemical accuracy. Its origin can be clarified using the partitioning of the exchange–correlation potential in the hole potential part and response part, vxc =
holexc +
resp, where the overbar indicates that we are dealing with coupling constant integrated quantities; see Section 2.4. The exchange–correlation hole potential is directly related to the exchange–correlation energy density,
holexc(r) = 2εxc(r) with
. The response part originates from the functional derivative of the εxc factor in the total energy.
Given DFAs with good total energies, the error in the orbital energies should not come from the hole part of the exchange–correlation potential – indeed, it has been argued that the error is in the response potential.320 It is quite common that an approximation to an integrand is decent in the sense that the integral (the energy) is well approximated, while the derivative of the integrand (the potential) is still very poor.
The response part of the current DFA potentials is too repulsive over the bulk molecular region, causing the 4–6 eV upshift of the orbital levels. A better approximation to the response potential is called for, rather than just the derivative that arises from existing LDA or (meta-)GGA energy density approximations. Indeed, replacing the LDA/GGA response part of the potential with the approximate response potential from ref. 321 (a local potential determined from nonlocal input) already improves the orbital energy spectrum a lot.320 Better approximations to the Kohn–Sham potential of course also improve response properties such as (hyper)polarizabilities and excitation energies.258 Note that this improvement is not primarily an effect of the correct asymptotic behaviour since the orbital energies are mostly determined by the potential in regions where the orbitals have a large amplitude (i.e., the region where the bulk molecular density resides). Obviously, the accuracy of the DFA potential has been lagging far behind that of the energy. It needs to be improved, preferably in a more fundamental way than by pragmatically admixing some percentage of a nonlocal exchange potential.
3.2.14 Görling.
The origin of the errors that most DFAs exhibit for the Kohn–Sham orbital energies is the presence of unphysical self-interactions. A solution to this problem has been around for a long time – namely, an exact treatment of the Kohn–Sham exchange potential, which requires the OEP method.40–46 If the exact local Kohn–Sham exchange potential – that is, the OEP exchange potential – is used, then the HOMO eigenvalue immediately is close to the IP as it should, whereas, in conventional GGA calculations, it is typically several eV too high. Moreover, the Kohn–Sham eigenvalue spectrum changes qualitatively: an exact-exchange (EXX-OEP) calculation gives a Rydberg series as it should, while a GGA calculation does not. Thus, if the self-interaction contained in the Hartree potential is properly cancelled by the exact Kohn–Sham exchange potential, then a qualitatively correct and quantitatively much more accurate spectrum of Kohn–Sham orbital energies is obtained.42–46
The OEP method has a bad reputation because of numerical problems. However, these problems have been solved and computationally efficient, numerically stable OEP methods are now available.46 An exact treatment of exchange requires correlation functionals that go along with it. Such correlation functionals exist – for example, RPA-based functionals102–104 – but are so far not very popular. The poor orbital energies are thus the price to pay for approximating exchange and correlation together in most DFAs, in order to exploit error cancellations.
3.2.15 Neese.
I very much welcome this discussion. In practice, there is a large SIE. This error is large and profoundly influences the localization/delocalization of the Kohn–Sham orbitals and consequently, the properties derived from them and the associated electron density. We know that removing the SIE using established methods like the Perdew–Zunger scheme10 destroys much of the accuracy of Kohn–Sham DFT. The development of physically based correlation functionals becomes challenging with this error in the background.
I am aware of brilliant attempts to develop correlation functionals on top of self-interaction free references (discussed in the preceding contribution by Görling). I would be delighted to see this approach receive even more attention in functional development.
3.2.16 Baerends.
To further this discussion, let me note that it is indeed generally accepted that the origin of the poor orbital energies of presently available DFAs are the unphysical self-interactions. However, it is not completely clear what is meant by SIE. The one-electron SIE is felt to be evident: the exchange–correlation energy of a one-electron system like the H atom (in this case just the exchange energy) should cancel the Hartree energy. Actually, LDA is not so bad for the H atom: the Hartree energy of 8.01 eV is cancelled to a reasonable degree by the LDA exchange energy of −6.89 eV, yielding an error of only 1.12 eV. If we add the B88 GGA gradient correction for the exchange energy, then the SIE is reduced to 0.04 eV. The same very small SIE is observed for the H2 molecule at the equilibrium distance.320
This should give us pause for thought when we want to blame the SIE for failures of DFAs. How much SIE is there really in the current DFAs? The DFA error in the orbital energy is of a different order of magnitude: 6.88 eV above the exact H-atom value with LDA and 6.20 eV when the B88 gradient correction is added – clearly, not the same effect as the tiny SIE in the total energy. The effect on the orbital energies that we call SIE arises when we take the functional derivative of the energy (it is in vresp).
A general definition of SIE is not so easy to formulate. In the original Perdew–Zunger work, the total energy was taken as starting point for constructing the correction.10 The most important effect of the Perdew–Zunger correction was, however, to change the potential and most of their discussion was focused on orbital energies. A straightforward definition of the SIE (also in the many-electron case) would be the error incurred by the exchange–correlation hole not integrating to −1 electron. Now, the first exact property that is required of the model holes in DFT is that they do integrate to −1 electron, as is already true for Slater's ρ1/3 approximation. This does indeed lead to reasonable results for the total energy but does not guarantee a good potential because of deficiencies that appear in the step to the corresponding potential.320
Using exact exchange (100% or at least a very large percentage) in either a generalized Kohn–Sham or EXX-OEP manner also provides a large improvement in the orbital energies, as noted in contribution (3.2.14). The improvement is due to the way the step to the potential works out in that case, as it does for the Hartree–Fock-like correction in the Perdew–Zunger self-interaction correction.
3.2.17 Gidopoulos.
In our group, we share the view that self-interactions are behind the errors of Kohn–Sham orbital energies, an opinion advocated strongly also by Rod Bartlett.322 As Baerends explains, the effects of self-interaction are not evident in the DFA total energy and the error in the Kohn–Sham orbital energies is about an order of magnitude greater than the error in the total energy. So why blame self-interaction for the Kohn–Sham orbital error? Our reasoning for arguing that the errors in the Kohn–Sham orbital energies are due to self-interaction, when even the definition of self-interaction in the total energy is unclear, is simple.
Görling was the first to point out that we can use Poisson's equation to define an effective charge density from the Kohn–Sham potential.43 Then, the Laplacian of the Kohn–Sham Hartree-exchange–correlation (Hxc) potential vHxc(r) defines unambiguously an effective charge density ρrep(r) whose Coulomb potential is the Hxc potential:
|  | (24) |
The “repulsion” or “screening” density
ρrep(
r) effectively mimics the repulsion felt by each electron. For a system of
N electrons in a self-interaction-free theory, the integrated charge
Qrep of
ρrep(
r) should be
Qrep =
N − 1, because each electron is repelled by the other
N − 1 electrons but not by itself. However, in local and semilocal DFAs,
Qrep =
N, which we interpret to imply that each Kohn–Sham electron is effectively repelled by all electrons of the system, including itself, and so self-interaction is present.
We agree with Baerends that the quality of the total energy in local and semilocal DFAs is (far) superior to the quality of the Kohn–Sham potential and hence we have decided not to interfere with the total energy of the DFA. Instead, we impose constraints on the effective local potential to reduce the self-interaction errors from it. These constraints, Qrep = N − 1 and ρrep(r) ≥ 0, are enforced with the OEP method, whose mathematical (rather than numerical) problems with finite basis sets are now well understood.323,324
The computational cost of these OEP calculations is determined by the matrix elements of the DFA functional derivative, a local potential, and is comparable to performing a small number (about ten) of DFA calculations. Imposing these constraints, the error of the HOMO Kohn–Sham orbital energies for local and semilocal DFAs reduces to about 1 eV. For one-electron systems, the two constraints give correctly a zero Hxc potential.325,326
3.2.18 Kronik.
Following the important points raised in contributions (2.1.7) and (3.2.17), I think it is worthwhile to emphasize that piecewise linearity, freedom from self-interaction, and an asymptotically correct Kohn–Sham potential – all three of which are important principles for DFA construction – are somewhat related yet inequivalent properties of the exact density functional.327
3.2.19 Xu.
The IP and EA are fundamental properties of atoms, molecules and solids, which are often associated with the orbital energies via Koopmans' theorem328 in Hartree–Fock theory or Janak's theorem87 in Kohn–Sham DFT; see contributions (2.2.4), (2.4.9), and (3.2.13). However, it is well-known that relaxation and correlation effects are often important in electron detachment and attachment processes, calling for extensions to the theory.16,329–334
From the perspective of fractional charges,16 an integration approach has been developed for the double-hybrid functionals,331 whose justification lies in the fact that they are found to fulfil better the piecewise linearity condition (see contribution (2.4.1)) and suffer less from delocalization error.332 Furthermore, the extended Koopmans' theorem333 (EKT) can be applied to the double-hybrid (DH) functionals, leading to the EKT-DH methods,334 which are shown to be capable of describing the breakdowns of the quasi-particle approximations for the inner-valence IPs, at a relatively low computational cost and a high accuracy.
3.3 How should we validate the quality of DFAs?
3.3.1 Staroverov.
In ab initio methods, quality is synonymous with overall accuracy. The current state of DFT suggests that one may need at least two interconnected criteria to characterize the quality of DFAs: accuracy and mathematical rigour (i.e., the extent to which the DFA satisfies exact constraints), which is a proxy for universality. Of course, the choice and relative importance of various exact constraints, test sets, metrics of accuracy, etc. are subjective, but some consensus may not be impossible to reach.
3.3.2 De Proft and Geerlings.
A remark aside from the validation itself is the use of a certain DFA after its validation. It can be asked, once a given DFA has proven its merits in a certain domain, that this DFA should additionally be benchmarked for problems (compounds, properties, reactions) that do not differ markedly from those for which the DFA has been successfully validated in the literature and for which one can reasonably expect that it will perform well for the problem at hand (cf. the notion “level of trust” introduced in contribution (3.2.1)).
This procedure, sometimes requested by reviewers, is often time consuming. In addition, if one asks authors to benchmark each DFA for every type of compound in every type of reaction, it can be argued that one is perhaps approaching a new level of parameterization.
3.3.3 Gill.
The development of a small number of data sets of unimpeachable experimental data, against which the predictions of new DFAs can be compared objectively and reproducibly, is essential. Both the data sets and the software used to evaluate DFA performance should be freely accessible and entirely transparent. It was the publication of such comparisons in the early 1990s that led to the widespread adoption of DFT in the chemical community.335
3.3.4 Gould.
While not quite as robust as experimental data, we now have access to some impressively large quantum-chemical benchmark sets (about 5000 energy differences in MGCDB84273 and 1500 in GMTKN55336) against which to validate DFAs. However, key overall quality metrics can be reproduced almost perfectly by about 150 entries of the original benchmark sets (less than 10%).337 This means that a large part of these sets contain redundant information. It is therefore important that validation protocols test DFAs across diverse physics and chemistry – we should not just assume that testing against more systems will automatically do a better job. New applications of statistical techniques may be required to develop robust validation protocols.
3.3.5 Grimme.
Validation of DFAs on benchmark sets outside the common chemical compound space is essential if we are to find universal and practically robust methods. Automatically generated molecular structures – as employed, for example, in the “mindless benchmarking” scheme proposed in ref. 338 – may offer a solution to this problem.
3.3.6 Krylov.
Extending the validation studies to more properties is important – for example, what works well for dipole moments may not give you good polarizabilities, and so on.
3.3.7 Neese.
I completely agree with the comment of Krylov. It seems to me that enormous efforts are directed towards developing functionals that provide good total energies and significant progress in this direction is undeniable. Yet, there are many other properties of chemical interest. In addition, accuracy in total energies does not translate to accuracy in other properties – for example, we have frequently seen that some popular functionals that provide good total energies fail spectacularly in the computation of hyperfine couplings, excitation energies or other spectroscopic properties (as eluded to in contribution (3.2.11)). At this point, co-convergence of energy and properties appears to be exclusive to wave-function-based ab initio methods.
The development of standardized test sets has been very beneficial for the development of DFT. It would seem beneficial, yet challenging, to include a wide range of additional data in the development of new functionals to come closer to co-convergence.
3.3.8 Trickey.
Regarding validation construed broadly: what constitutes a meaningful, hence valid, improvement in a DFA? If a DFA improves over another by 0.2 kcal mol−1 mean absolute error (MAE) on atomization energies and 0.02 Å on bond lengths, etc., is that really an improvement or is it in the noise of the data sets themselves? If my group produces a DFA that gives essentially the same errors on a large collection of canonical data sets as the best older DFA on the same Perdew–Schmidt rung,339 but the new DFA is much more stable numerically or 20% faster, wouldn’t such improvements be validation themselves?
3.3.9 Savin.
True, the superiority of one DFA over another can be significantly diminished after taking into account the uncertainty in the reference data.340
3.3.10 Barone.
Ideally, a DFA should provide accurate results for a broad set of molecular properties. However, from a pragmatic point of view, there is a difference between specialized and broadly applicable DFAs and the choice between the two classes depends on the problem at hand. My personal view is that the most suitable strategy is to enforce the largest possible number of formal constraints that a DFA should obey while leaving a few parameters free for improving accuracy. However, at present, DFA benchmark is severely biased towards energies and (perhaps) first-order properties of molecules containing atoms belonging to the first three rows of the periodic table.
3.3.11 Pernot.
To answer the question of Trickey about the intercomparison of DFAs, a small difference in MAEs between two DFAs certainly cannot be relied upon without additional information. This difference might be an artefact of the limited size of a nonexhaustive reference data set. As a result, there may be a high probability of rank inversion when the data set is perturbed by adding or suppressing a few points. A set of tools has recently been proposed to address this problem – for example, by estimating a rank inversion probability, Pinv, or by using statistics based on system-wise comparisons, such as the systematic improvement probability.341,342
3.3.12 Pernot.
Validation requires the comparison of calculated values with a set of high-quality reference data. The resulting errors are used to estimate validation statistics. An important fact to have in mind is that the distribution of errors has no reason to be normal,343 essentially because the errors are dominated by systematic contributions from all the approximations involved in a calculation – level of theory, basis set quality, values of parameters, and so on.344
This is why statistics such as the MAE should not be used to estimate the confidence level of a DFA. There are more pertinent metrics such as Q95 (the 95th quantile of the absolute error distribution), on which a level of confidence can be based.343,345 We then know that there is a 5% risk to get an absolute error above Q95. By comparison, the probability for absolute errors to be above the MAE has been observed to vary between 0.2 and 0.45.346 From a MAE value of let us say 0.5 kcal mol−1, one cannot estimate the probability/risk for the errors to exceed 1 kcal mol−1.
The confidence we have in the prediction capabilities of a given method has something to do with probabilistic forecasting; this however, may depend on factors difficult to quantify, such as deciding about the probability we consider good enough to take a decision. Ideally, a DFA should be judged on its prediction uncertainty, like any measurement method, but this requires the correction of systematic errors, which goes beyond the realm of the DFA itself.347–349
3.3.13 Savin.
Could it be that the importance of accuracy is overemphasized? Let us take as an example the intensive atomization energies (i.e., per atom) as obtained with the B3LYP functional on a widely used benchmark data set.350 Let us now consider what the measures mentioned in contribution (3.3.12) provide.343 The often quoted “chemical accuracy” of 1 kcal mol−1 corresponds to the mean absolute error of the B3LYP functional. However, this accuracy is reached by only about half of the systems in the data set.
Let us now ask ourselves what is smallest target accuracy that is reached by 95% of the systems in this data set. It turns out that, to satisfy this condition, the target accuracy must be as large as 3–5 kcal mol−1, the presence of the interval arising from the finite size of the data set. Nevertheless, the B3LYP functional is a very successful DFA.
3.3.14 Neese.
It appears to me that “sufficient accuracy” is something that depends largely on the context of the computational problem at hand. Take a very exothermic reaction, with a free-energy change of around −50 kcal mol−1. I don’t think I would understand anything about this reaction any better whether I compute the energy change to be −47 or −53 kcal mol−1, nor would it change any conclusion. On the other hand, if one wants to correctly predict the enantiomeric excess of a bifurcating reaction that may lead to different stereochemical outcomes, then even one half of kcal mol−1 matters.
Related to this problem of adjusting the “useful” accuracy to the problem at hand is the question of what else is needed for a successful chemical prediction or interpretation? For example, we have learned the hard way that getting accurate electronic energies from coupled-cluster theory will not necessarily lead to more accurate chemical predictions. In real-life chemical applications, there are other important error sources, for example coming from solvation or entropy effects. In addition one can not stress enough how important it is to carefully construct the computational model. In studying complex molecules (or enzyme active sites), one needs to pay a great deal of attention to possible alternative conformers, alternative protonation states, possible hydrogen bonds or potentially functionally important solvent molecules, to name only a few important aspects of model construction. In our experience, the errors stemming from failing to treat any of the mentioned effects correctly can easily overwhelm the error in the electronic energies. If this is the case, focusing on computing accurate electronic energies on irrelevant chemical models or with a large, possibly unrecognized solvation energy error in the background appears rather pointless – the conclusions drawn from the calculations will likely be wrong or will fail to properly explain the experimental findings. These aspects require a great deal of chemical common sense on the part of the computational chemist and are independent of the intrinsic accuracy of the chosen theoretical method.
3.3.15 Galli.
The validation of DFAs requires first a serious verification effort. This verification should include comparisons of properties obtained with different codes, taking care to ensure convergence of all numerical parameters involved in the calculations.
Sometimes statements on the validity of DFAs made in the literature are inaccurate or just not correct because a detailed analysis of numerical approximations has not been carried out before assessing the accuracy of the DFA. It is important to realize that the impact of poorly converged numerical calculations on the assessment of the validity of the theory is not the same for all properties and hence numerical verification should be done for each property of interest separately. It is also important to keep in mind that many comparisons of various DFAs in the solid state are made using pseudopotentials that are not consistent with the level of theory adopted for the valence electrons. For example, almost all hybrid DFT calculations are carried out with PBE pseudopotentials; and all comparisons are thus tainted by this inconsistency.
3.3.16 Galli.
We should push for a much more concerted effort of the different communities using DFT, aimed towards the verification and validation of properties calculated with DFAs. Different communities of DFT users, with different “cultures”, still exist: the quantum-chemistry community, the solid-state and materials science community, and the community carrying out first-principles MD and interested in finite-temperature properties. It would be very important for these communities to come together and establish a list of properties, comparing results for molecular and solid-state systems.
3.4 Where do existing DFAs work and where do they fail?
3.4.1 Johnson.
Existing Kohn–Sham DFAs fail for systems where the orbital occupations cannot be represented by a single Slater determinant. The classic example of a multireference system that cannot be represented by a single Slater determinant is the stretched H2 molecule. The ground-state wave function in the dissociation limit is an equal mixture of two determinants. A simple single-determinant wave function from MO theory incorrectly includes both covalent and ionic terms and, consequently, gives in the dissociation limit an energy of only −11/16Eh, compared to the exact energy of −1Eh. Despite not using an explicit wave function, Kohn–Sham DFAs are valid only for single-determinant states and suffer from similar errors as Hartree–Fock-based wave-function methods for multireference systems.121 This can be understood by examination of the pair density. For a Slater-determinant wave function, the pair density is | 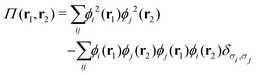 | (25) |
where the sums run over pairs of occupied orbitals ϕi of spin σi. The exact pair density is the probability of finding a pair of electrons simultaneously at two points in space; it determines the proper form of the exchange–correlation energy via the AC.
Notably, the form of the pair density gives rise to the exchange hole in the case of parallel spins (δσi,σj = 1) and leads to a depletion of probability of finding a second, same-spin electron near a reference electron. However, for multideterminental wave functions, the pair density behaves quite differently. Instead of leading to a Fermi (exchange) hole, with a depletion of parallel-spin electron density around a reference point, multireference systems can instead have a Fermi “heap” around the reference point,351 with an accumulation of parallel-spin electron density. This physics is not captured by Kohn–Sham DFAs, which model a localized exchange hole.
While there are other types of systems (such as those exhibiting significant delocalization error) where a particular DFA or class of DFAs may fail, all Kohn–Sham DFAs fail for multireference systems. Examples include stretched covalent bonds,352 organic biradicals,353 and solid Au2S.354
3.4.2 Rebolini.
Although Kohn–Sham theory is in principle valid for all systems, in practice existing DFAs mostly fail at describing multireference systems. However, one may want to distinguish between properties that depend on the total density of the system, which may still be properly described, and properties which depend specifically on the strongly-correlated electrons where DFAs are almost “expected” to fail – for instance, DFAs can be used to study the equilibrium structure, phonon spectrum, and polarization of strongly-correlated materials but fail to describe most magnetic properties.
3.4.3 Neese.
The first thing that comes to my mind in this context is the multiplet problem – that is, the simple fact that a single electron configuration (meaning a distribution of electrons among orbitals with occupation 0, 1 or 2) gives, in general, a number of different many-particle states with different spin couplings among the unpaired electrons.
This is not an esoteric formal remark. In open-shell transition-metal complexes, for example, the multiplet problem is extremely prevalent and affects all of their physical (spectroscopic) properties in a profound way. Take a simple L-edge (2p → 3d) excitation. In a d5 system, this excitation leads to as many as 1512 different final states that all contribute to the L-edge absorption spectrum. Yet, in a particle–hole theory such as TDDFT, one only has 15 particle–hole pairs to work with. How to describe 1512 states with only 15 particle–hole pairs is not clear. In practice, the failures are dramatic. Another example are the d–d spectra of these ions, which frequently show low-lying double excitations that are completely absent from the DFT-computed spectra.355
Similar remarks hold for many multideterminental (as opposed to multiconfigurational) problems, like spin-coupled open-shell ions. Surely, broken-symmetry DFT is a highly useful tool, yet it is a bit of a crutch and I am not aware of a satisfactory formal solution that would also be practical.
I find it important to distinguish between multideterminental and multiconfiguration problems. In the former case, there is a single electronic configuration but spin coupling of the unpaired electrons leads to a multideterminental wave function. In the latter case, there is an actual mixing of configurations with different orbital occupations. The former case is far easier and far more frequent. Recognizing this distinction may help (and, in fact, has already helped) to design more tailored approximations – for example, spin-flip methods.
3.4.4 Ayers.
Multireference effects are obviously problematic in single-determinant theories like Kohn–Sham DFT. But even if one changed to a different starting point (perhaps by moving to an extended DFT), there would still be (different) types of correlation that would be difficult to describe. Indeed, for every practical electronic-structure method I know, I can think of some type of electron correlation that it struggles to describe, so ab initio DFT356 is no solution. I would not say the situation is hopeless, but I accept that different flavours of DFAs will be needed for different types of properties and systems.
3.4.5 Piecuch.
This clearly is a rich topic, and all of us could find examples of situations (molecular systems, solids, selected properties other than energy, etc.) where the existing functionals used in conjunction with Kohn–Sham DFT and TD-DFT struggle. Bond breaking, doubly excited and charge-transfer states, strong correlations, and dispersion forces require additional – sometimes a lot of additional – effort beyond conventional Kohn–Sham DFT computations. However, from my point of view, which is the point of view of an ordinary user of DFT codes, a larger issue is the lack of transferability of DFT-based recommendations.
Focusing on my own experiences, the widely used B3LYP functional is among the best DFAs for studies of the activation barriers that determine aerobic oxidations of alcohols by gold nanoparticles,298 the BP86 and B97-D functionals performing considerably worse. At the same time, B3LYP and other hybrid functionals work poorly and the BP86 (especially when corrected for dispersion) and B97-D functionals are impressively accurate in applications of DFT to methyl–cobalt bond dissociation and low-lying excited states of methylcobalamin.357,358
The latter situation is similar to that created by the application of various DFAs to dicopper–dioxygen structural motifs. For example, when examining isomerization curves connecting the bis(μ-oxo) and μ-η2:η2-peroxo isomers of Cu2O2 cores supported by 0, 2, 4, and 6 ammonia ligands, hybrid functionals fail, the magnitude of the error being directly proportional to the percentage Hartree–Fock exchange in the functional.359 Pure GGAs work well in this case.
There is nothing new in the observation that pure GGAs may work better in situations involving static correlation. However, improving predictability of the outcome of DFT computations, so that one could, for example, avoid calibrating DFT functionals every time a new system is studied, while addressing fundamental issues such as the issue of SIE, would be useful. I realize that there has been great progress in addressing these and related issues in all sorts of interesting ways, but an additional effort toward improving the situation in this area would be helpful for the users of DFT methods.
3.4.6 Gagliardi.
Another application where Kohn–Sham DFT encounters some challenges is the determination of the spin ladder in multimetallic compounds. Some of these compounds are molecular magnets with potential applications in information storage, quantum computation, and molecular electronics.360 In compounds containing several magnetic centres, the spin carriers can magnetically interact in many ways. A prototypical system is a tris-hydroxo-bridged Cr(III)–Cr(III) system (Kremers dimer),361 which consists of two antiferromagnetically coupled Cr(III) metal centres with a d3–d3 electron configuration.
Pantzasis362 pointed out that Kohn–Sham DFT generally fails to reproduce the experimental spin ladder for such systems (unless some ad hoc spin purification is performed), and thus the calculation of the magnetic coupling constant, and a more physical representation of the low-spin states requires a multireference treatment based on restricted-active-space SCF (RASSCF) or DMRG wave functions. However, also within the context of a large active space, a post wave-function treatment is needed. Multireference pair-density functional theory (MC-PDFT) starting from a large active space (DMRG or RASSCF active space with 30 electrons in 22 orbitals) gives encouraging results.363
3.4.7 Kaupp and Arbuznikov.
In the context of the failures for multireference cases, it seems important to mention attempts to account for strong-correlation effects and minimize fractional-spin errors.26,27,364 An important direction of development are Becke's real-space models of nondynamical correlation (initially the B05 functional13), which have been extended to account for strong-correlation terms by relying on the AC (Becke's B13 model165 and the related KP16/B13 model169 by Kong and Proynov). One interesting aspect of these functionals is that they are based on full exact exchange and model nondynamical correlation without using semilocal exchange.
Another important direction towards including strong-correlation effects also makes use of the AC but extends it to the strongly-correlated (λ → ∞) limit.149,170,172 While the question of how to best represent the noninteracting reference system of Kohn–Sham theory and the validity of the AC for multireference cases is still open, these approaches provide some hope of obtaining functionals that incorporate such effects.
3.4.8 Romaniello.
Kohn–Sham band structures are widely employed in solid-state physics. However, whereas this may be a reasonable approximation to the true charged excitation energies in the limit of weak correlation, it completely fails in the limit of strong correlation, as pointed out in contribution (3.4.2).
The paramagnetic phase of transition-metal oxides – which is systematically described as metallic, contrary to experiment – is a paradigmatic example. These systems are a challenge also for more advanced methods such as the GW method.365 However, this problem arises since we are modelling the paramagnetic phase as nonmagnetic. Trimarchi et al.366 have recently shown that band-structure theories can give a correct description of these systems provided that one models the spin-disordered paramagnetic phase using a larger supercell.
In fact, this is routinely done to model the spin-ordered antiferromagnetic phase: the nonmagnetic unit cell is doubled so that a different spin can be specified for the transition-metal atom. In this case, a band-gap opening is usually obtained in band-structure theories, reflecting the fact that, the more physical information is put into the problem, the less accurate a theory needs to be.
Maybe I can clarify this point with the simple example of the Hubbard dimer at one-half filling, which can give insight into a paramagnetic or an antiferromagnetic spin structure, depending on whether or not the spin symmetry is broken. In the atomic limit (where the electron–electron interaction dominates over the kinetic energy, hence we are in the regime of strong correlation) the two electrons, one with spin up and the other with spin down, are localized one on one site and the other on the other site with equal probability – that is, the ground state is the spin singlet
. The spectral function (which is related to photoemission spectra) thus shows, for each spin, two peaks with the same spectral weight 1/2 – one for the removal of an electron (peak at ε0, which is the orbital energy), and one for the addition of a second electron (peak at ε0 + U, with U the on-site electron–electron interaction), representing, respectively, the removal and addition energies, of an isolated atom with one electron.
Even the GW method cannot reproduce this spectrum. The GW method gives only one peak at ε0 + U/2, in line with the fact that this method describes many paramagnetic insulators as metallic. This happens because the GW method treats the charge/spin density as a classical charge distribution, with half an electron with spin up and half an electron with spin down on each atom that respond to the additional electron or hole added to the system in a photoemission experiment. If one considers instead the spin-symmetry broken state |Ψ0〉 = |↑↓〉 (or, equivalently, |↓↑〉), which is also an eigenstate of the system in this limit, then the GW method gives the correct spectral function. In this case, the electrons have fixed positions and one does not need to consider explicitly the correlation between two particles. One may therefore think that there is little correlation in this state. In reality, the system is correlated, but part of the correlation is included in the symmetry breaking.
3.4.9 Galli.
As indicated earlier, interfaces between metallic and insulating phases and interfaces between low-gap and wide-band gap semiconductors remain challenging to describe with existing DFAs – namely, band offsets of these interfaces are not accurate with most functionals and even structural properties in some cases367 turn out to be inaccurate. The electronic properties of several transition-metal oxides are equally challenging to describe with existing DFAs, especially those considered to be highly correlated materials. Empirical fixes have been proposed and used, as in the DFT+U method, but their predictive power is yet unclear, especially in cases where different values of U must be used for different oxidation states of the metal in the same oxide. An outstanding open problem is, for example, the metal-to-insulator transition in vanadium oxide (just to name one transition-metal oxide) as a function of oxygen composition or temperature.
3.5 What type or level of spatial nonlocality is required in explicit DFAs for the energy?
3.5.1 Perdew.
Given the exact electron density for a real system, and excluding exotic cases like the strongly stretched hydrogen molecule ion, the meta-GGA level of nonlocality can often give an accurate energy.368 But the level of nonlocality of the exchange–correlation potential (functional derivative of the exchange–correlation energy) can be much more critical for the electron density. The meta-GGA density is not sufficiently localized around the nuclear centres for some chemical problems, where the Hartree–Fock density, which comes from a more nonlocal exchange–correlation potential, is better.118 In a solid metal, however, the Hartree–Fock and even the meta-GGA density may be too localized in comparison with the exact density. Importantly, an approximate functional that is accurate for the energies on the exact electron densities of a wide class of real systems can still have inaccurate functional derivatives and thus inaccurate self-consistent densities in that class.
3.5.2 Chermette.
To generalize Perdew's statement in contribution (3.5.1), a given approximate functional can be very accurate for a given property, such as density, (everywhere or at nuclei), energy, or properties involving functional derivatives, but not for other ones. Indeed, this is just a consequence of the approximate nature of a DFA.
3.5.3 Baerends.
One consequence of the orbital levels for most DFAs being much too high (see contribution (3.2.13)) is a much too high-lying LUMO level. Adding an extra electron to the system then causes the LUMO level (containing the added electron) to be so close to zero that the LUMO becomes very diffuse, or even above zero, with an infinitely extended LUMO. This is a case where the Hartree–Fock method yields a much improved density.369 A similar effect can be achieved by using a more accurate model Kohn–Sham potential.370,371
On the other hand, while the Hartree–Fock density is typically very good for atoms, the Hartree–Fock model often yields poor bonding densities for molecules, being too diffuse around nuclear centres. For instance, for H2 it has been demonstrated that, due to this diffuse character, the errors in the one-electron energy terms (not sufficiently negative electron–nuclear energy and too low kinetic energy) are comparable to the error in the electron–electron energy.69 Upon stretching H2, the errors in the one-electron energy terms soon exceed the two-electron energy errors. For N2,83 the one-electron errors due to the too diffuse Hartree–Fock density are at the equilibrium distance already larger than the total bond energy of about 10 eV!
These errors can be understood from the limited flexibility of the Hartree–Fock wave function – that is, they arise from the lack of electron correlation. This gives a strong incentive to develop accurate model Kohn–Sham potentials that do better for the density and for the orbital energies and is also very important for the MO-theoretical explanations in chemistry.
3.5.4 Xu.
The electron density and the electronic energy are two quantities of fundamental importance. While an accurate description of the density allows for correct physical insight from the charge distribution, accurate determination of energies and their changes allows for precise quantification of the properties of a system of interest. The Hohenberg–Kohn theorems,5,6 which state that there exists a mapping from the ground-state electron density of a many-body system to its total energy, lay the foundation of modern DFT. To put DFT in practical use, the central questions are then (Q1) how to find the ground-state density of a physical system, and (Q2) how to set up a mapping from the density to the total energy. The Kohn–Sham scheme65 answers Q1 and Q2 simultaneously in a self-consistent way, using a local exchange–correlation potential, obtained by taking the derivative directly from a given DFA.
However, Q1 and Q2 can also be pursued separately.372 In cases where the Hartree–Fock method yields a much improved density (see contributions (3.5.1) and (3.5.3)), evaluation of the energy using a GGA functional on the Hartree–Fock density yields a much improved energy.373–375 It seems impractical, or even impossible, to demand that all properties be calculated accurately using a single, low-rung DFA; see contribution (3.5.2). It may eventually be possible for top-rung DFAs to give good densities, energies, and other properties, simultaneously.332,376,377 On the other hand, it is important to take both accuracy and efficiency into account. Hence, one can use a low-rung DFA to generate good orbitals and a good density efficiently, while using a top-rung functional to evaluate the energy accurately, as in the XYG3 double-hybrid functional.134
3.5.5 Ayers.
If one wishes to describe strong/static correlation using a Kohn–Sham DFA, then it is clear that enormous (even infinite) spatial nonlocality is required, because the (spherically-averaged) exchange–correlation hole can have a significant long-range structure. Moreover, when the multireference character is strong, that structure is exquisitely sensitive to small perturbations.
3.5.6 Gill.
Local DFAs are intrinsically incapable of capturing dispersion energy, which arises from long-range correlation effects between electrons.
3.5.7 Grimme.
The fact that semilocal DFAs yield an inconsistent or even unbound description of small van der Waals complexes was discovered in the mid-90s by Becke, Hobza, and Pulay.378–380 Noble gas dimers have been investigated several times as difficult cases for Kohn–Sham DFT, with large errors and sometimes qualitatively wrong behaviour being found. However, this “DFT failure” is actually a failure of the usual semi-local approximations and not of the theory itself. Around the same time, Meijer and Sprik presented an analysis of the problem for the typical case of the benzene dimer and noted related errors in the computed lattice energy or mass density of molecular crystals.381 General claims that semilocal DFAs cannot describe nonlocal long-range correlation (London forces) were occasionally made,382 but without further theoretical explanation – in particular, regarding the role of the correlation functional. Even as late as in 2002, the situation was not clear as indicated by a study of van Mourik and Gdanitz, which identified over-repulsive as well as over-binding functionals.383 For a more detailed historic account of the development of the dispersion problem in Kohn–Sham DFT, which cannot be solved simply by including nonlocal Fock exchange as is done in hybrid functionals, see ref. 384.
In those early days, the simple but incomplete picture prevailed that the dispersion energy is only relevant for the intermolecular situation – that is, for van der Waals complexes and condensed phases. The modern notion – namely, that intramolecular dispersion effects are especially important in large systems and in standard thermochemical applications – emerged only over the last ten years.385 Nowadays, newly proposed and accurate DFAs account for dispersion, which is mandatory for quantitative calculations and often even to obtain qualitatively correct results.
The most prominent dispersion correction schemes, which can be added to established DFAs, can be classified into the four groups:384 (i) nonlocal, density-based functionals (e.g., vdW-DF or VV10), (ii) C6-based, atom-pairwise semiclassical models (e.g., D3/D4, XDM, TS/MBD), (iii) one-electron effective potentials, and (iv) highly parameterized density functionals (e.g., M06). Some of these methods, which mostly contain empirical components, yield very accurate long-range interactions, close to coupled-cluster accuracy (with a typical relative error of less than 5%) at low, often negligible computational cost.
Problems for particular systems or seemingly large differences between dispersion-corrected DFAs can often be attributed to an inaccurate description of short-range exchange–correlation effects, which are more difficult to describe than the long-range regime, dominated by 1/R−6 interactions. Dispersion effects can also be hidden by exaggerated charge-transfer interactions induced by the SIE in GGAs.387 Note further that, although London dispersion as a nonlocal correlation effect is omnipresent, it can be partly quenched in typical condensed-phase chemistry applications. In such systems, intramolecular noncovalent interactions compete with intermolecular solvent interactions and their subtle balance requires a sophisticated theoretical treatment of both dispersion and solvation.
3.5.8 Tozer.
The electrostatic theorem of Feynman (obtained by applying the differential Hellmann–Feynman theorem to a nuclear perturbation) states that the force on a nucleus equals the classical electrostatic force due to the electrons and nuclei in the system.388 This has great physical appeal since it relates the force on a nucleus directly to the electron density, in the true spirit of DFT.
The electrostatic theorem is formally exact, but breaks down for nonvariational methodologies and/or finite basis sets, meaning that it is of limited use in practice. However, for small systems where variational methodologies can be used with very large basis sets, the theorem is quantitatively applicable, meaning it provides an alternative perspective for viewing the “dispersion problem” of local functionals.389 Errors in dispersion forces can be understood in terms of errors in electron densities, which in turn can be understood in terms of errors in the exchange–correlation potential in the Kohn–Sham equations. Similar arguments can be applied to other problems, such as static correlation or delocalization errors.390
3.5.9 Gori-Giorgi.
The relevance of errors in electron densities for capturing dispersion interactions may need some reconsideration, or at least needs to be better understood, especially in the DFT setting. Pragmatically, poor densities can give very good dispersion energies – as an extreme example, it has been shown that it is possible to get exact dispersion energies between two one-electron systems up to and including orders R−10 without any deformation of the monomer densities.391
The subtle point with the electrostatic theorem of Feynman388,392 is that the result depends on whether one performs the derivative with respect to the nuclear position in the original coordinates or in the coordinates in which the electrons are centred on their respective nuclei.393 In the first case, the interaction energy depends only on the density distortion at order R−7 (for which the underlying wave function must be accurate to second order in the dipole–dipole and dipole–quadrupole interaction); in the second case, the interaction energy depends only on the distortion of the interfragment pair density at order R−3 (for which the underlying wave function must be accurate only to first order in the dipole–dipole interaction).393,394 These observations may suggest a route to build approximate exchange–correlation functionals by considering a simplified real-space mechanism, in which dispersion is reduced to the competition between kinetic energy and monomer–monomer interaction (thus keeping the density and pair density of the monomers unchanged, but producing an accurate interfragment pair density).394
3.5.10 Dobson.
Much relevant physics can be included in energy functionals via use of generalized “densities” assembled from Kohn–Sham orbitals, such as the positive local kinetic-energy density τ(r) used in meta-GGAs. Here, however, attention will be focused, as per the title question for this section, on strictly explicit functionals of the electron number density ρ and its space derivatives such as ∇ρ and ∇2ρ.
It may be useful to consider nonlocal functionals as a sum of “one-point”, “two-point”, “three-point”… contributions, where the nth term involves a 3n-dimensional space integral of a function Fn of the density and its derivatives, sampled at n different spatial points:
|  | (26) |
with
| F1 ≡ F1(r, ρ(r), ∇ρ(r),…), | (27) |
| F2 ≡ F2(r1,ρ(r1),∇ρ(r1),…, r2,ρ(r2),∇ρ(r2),…), | (28) |
and so on. Here, three dots … represent possibly a small finite number of additional space derivatives of
ρ. Keeping an infinite number of derivatives would probably be equivalent to knowing the density everywhere
via a three-dimensional Taylor series, at least for smooth densities. Then perhaps even the first term on the right-hand side of
eqn (26) would represent the most general nonlocal density functional.
The LDA and GGA functionals correspond to the first term of the expansion in eqn (26). Examples of the second-order term (“two-point functionals”) are the naive Hartree energy and the vdW-DF energy functional of Langreth, Lundqvist, and co-workers.395
The expansion in eqn (26) may be relevant in the quest for explicit density functionals for the kinetic energy, a topic that has seen revived interest recently in the context of orbital-free DFT. Here, however, I will confine my remarks to the theory of van der Waals interactions (London dispersion),396 with which I am more familiar.
For dispersion interactions, the second-order term in eqn (26) has already had considerable success via the vdW-DF functional395 and its extensions.397 The third-order term would be needed, for example, for a strictly explicit density functional to capture the Axilrod–Teller–Muto interaction – that is, the van der Waals interaction between three atoms, taken beyond the summed interaction between pairs of atoms).
It has been known for some time that widely-spaced low-dimensional metals have van der Waals interactions that are qualitatively different from those between nonmetallic structures with a similar geometry;398–400 for some discussion, see Chapter 11 of ref. 396. Recently, it has been found that this specific metallic van der Waals physics is important beyond the asymptotic region, indeed right down to contact, for metallic nanotubes and doped graphene sheets. This behaviour was captured by calculations401 of the electron correlation energy in direct RPA (dRPA). Methods like dRPA start from an electronic band-structure calculation and are thereby sensitive to the presence or absence of a zero HOMO–LUMO gap (band gap). I fear that a very high order in the functional expansion in eqn (26) might be needed to capture such physics. I wonder, though, whether one could use a close examination of the ground-state electron density in the tunnelling region between atoms, in order to recognize the band gap. Certainly, this region determines the overlap (tunnelling) energy integral t in the tight-binding description of electronic band structure. In that case, perhaps the first few terms on the right-hand side of eqn (26) might be sufficient.
3.6 What is the role of symmetry breaking/restoration for DFAs?
3.6.1 Perdew.
Symmetry breaking reveals strong correlations that are present in a symmetry-preserving correlated wave function but “freeze out” in the total density or spin density of a Kohn–Sham DFT calculation.402 This often provides real information about the system being studied, and sometimes enhances the accuracy of the approximate functional. For example, when the bond length of the hydrogen molecule is strongly stretched, the symmetry-preserving ground-state wave function is a spin-unpolarized singlet state, whose energy most standard DFAs cannot get right, but the symmetry-broken solution reveals the correct dissociation to two separate hydrogen atoms, one spin up and the other spin down. In this way, symmetry breaking in approximate Kohn–Sham theory can capture what is a strong correlation in wave-function theory. What is strongly-correlated for one reference state can be weakly-correlated or even uncorrelated for another reference state. Kohn–Sham theory can also be re-interpreted as a theory not for the up- and down-spin densities but for the total density and on-top pair density.403
It is only via symmetry breaking that the interaction of the electrons with the nuclei can be regarded as a static external potential. In a symmetry-unbroken wave function for electrons and nuclei, all potentials are internal and all effects are correlations. The quantum theory of measurement requires a symmetry-broken or classical observer. The measured antiferromagnetism of solids is a physical symmetry breaking: a fluctuation or correlation that persists for a long time even on the human scale.404 Thus, condensed matter physicists tend to be more comfortable with symmetry breaking than many quantum chemists are. While the symmetry of the ground state of a finite system remains unbroken when averaged over an infinite time interval, the time interval over which the symmetry remains broken in a fluctuation can grow rapidly as the spatial extent of the system grows. The macroscopic world as we perceive it is symmetry-broken and classical.
3.6.2 Gould.
It is worth noting that, although symmetry breaking is extremely useful and often physically reasonable for the reasons mentioned in contribution (3.6.1), there are cases where preserving symmetries is important. A prime example is when we are interested in spectroscopic properties that are meant to be degenerate, but where the degeneracy is “spoiled” by symmetry breaking. Such cases can be dealt with by careful application of ensemble theories, as discussed in Section 3.7.
3.6.3 Vignale.
With reference to contribution (3.6.2), a good case in point is that of open-shell atoms, where rotational symmetry demands the existence of a degenerate multiplet of ground states when the magnitude of the orbital angular momentum L is nonzero. There is no functional that I know that can guarantee that this degeneracy is respected when the densities of the degenerate states are not trivially related to each other by a rotation. Years ago, Becke attempted to solve this problem by introducing a current-dependent functional, but could not achieve rigorous degeneracy.405
3.6.4 Gould.
It is worth noting that ensemble DFT, which invokes multiple Kohn–Sham states via ensemble density matrices, can restore all degeneracy. This is briefly discussed in Section 3.7.
3.6.5 Savin.
Spin-symmetry breaking is related to the general problem of degeneracy, as is the localization/delocalization problem.173 Note that the two-body density does not have the ensemble property used for the one-particle density. However, the real problem (not only for DFAs) is to deal with near degeneracy.
3.6.6 Loos.
In the DFT context, symmetry breaking might be seen as a signature of the approximate nature of a given exchange–correlation functional. Taking as an example the dissociation of the hydrogen molecule discussed in contribution (3.6.1), one might expect to never see any symmetry breaking if one employs the exact exchange–correlation functional within Kohn–Sham DFT, which is true for the Hubbard dimer.406 Thus, the ability of a given functional not to break the (spin and spatial) symmetries could be potentially seen as a diagnostic of its quality.
3.6.7 Gould.
While this is almost certainly true for exact spin-free DFT, there is an important consideration in Kohn–Sham DFT with spin densities. Even if we fix calculations to the exact total density, ρ = ρ↑ + ρ↓, the Kohn–Sham kinetic energy Ts[ρ↑,ρ↓] can depend on ζ = |ρ↑ − ρ↓|/ρ (or, rather, on its Kohn–Sham equivalent, which may not be the same), and this dependence must be mirrored by Exc[ρ↑,ρ↓]. Since Kohn–Sham spin DFT seeks to minimize Ts[ρ↑,ρ↓], it might be the case that Ts[ρ↑,ρ↓] is minimized for a broken symmetry. It would be nice to determine if there is any exact symmetry breaking, or if spin DFT also preserves symmetries.
3.6.8 Gori-Giorgi.
Kohn–Sham DFT that uses the SCE limit (see contributions (2.4.5) and (4.5.8)) to approximate the exchange–correlation functional is able to stretch the H2 molecule without spin symmetry breaking.172,407 The SCE functional is also able to capture charge localization in very low-density systems without spatial symmetry breaking.408 However, the SCE functional is a very nonlocal approximation to the exchange–correlation functional (which is exact in a certain limit) and, at present, rather involved to evaluate. It also strongly overestimates correlation, so that a better strategy could be to design functionals that are inspired by the mathematical SCE structure but simplify and renormalize it.145–147
Apart from the reasons mentioned in contribution (3.6.2), further efforts to avoid symmetry breaking might be worthwhile in order to obtain potential-energy surfaces without kinks.
3.6.9 Görling.
To understand symmetry in Kohn–Sham DFT, it is necessary to look not only at the symmetry of the density or spin density but at the symmetry of the Hamiltonian operator of the true electronic system and of the Kohn–Sham Hamiltonian operator. The nonrelativistic Hamiltonian operator of the true electronic system is rotationally invariant in spin space even for a spin-polarized system with an odd number of electrons. Therefore spin is a good quantum number.
In the Kohn–Sham treatment, we then have a choice: (i) we can require the spin density to be identical in the Kohn–Sham and true electronic systems. This choice amounts to a spin-polarized Kohn–Sham calculation with a Kohn–Sham Hamiltonian operator that is no longer rotationally invariant in spin space. Spin is then no longer a good quantum number for the Kohn–Sham determinant – that is, spin poisoning occurs. (ii) Alternatively, we can require the total density but not the individual spin densities of the Kohn–Sham system to be identical to the true electronic system. The Kohn–Sham Hamiltonian operator then remains rotationally invariant in spin space and we get identical spin-up and -down orbitals. As a result, the orbitals and the Kohn–Sham wave function can be chosen to have well-defined spin but the individual Kohn–Sham spin-up and -down densities are no longer identical to those of the true electronic system. In practice, approach (i) is typically taken – however, approach (ii) is equally correct from a formal perspective, a point Walter Kohn made from time to time.
A similar choice can be made with respect to symmetries in real space. In open-shell atoms, for example, one can either require that the total density or the spin densities for the Kohn–Sham system and true electronic systems are identical or require that only their totally symmetric (i.e., spherical) real-space components are identical.409 Depending on the choice made, the Kohn–Sham Hamiltonian either has a symmetry lower than the spherical symmetry of the true Hamiltonian in real space or is spherically symmetric in real space like the Hamiltonian of the true electronic system.
These choices, leading to different but formally correct Kohn–Sham approaches, must be distinguished from symmetry breaking of the type observed in a dissociating hydrogen molecule. The Kohn–Sham Hamiltonian has been shown to exhibit at least the symmetry of the total density or spin density of the true electronic system.409 In the dissociating hydrogen molecule, the true density is non-spin-polarized at all distances. Therefore, an exact spin-polarized Kohn–Sham calculation always reduces to the non-spin-polarized case. If this reduction does not occur, then it is an artefact of the employed approximate exchange–correlation functional, pointing to a shortcoming in the description of static correlation.
Finally, it should be pointed out that symmetry-breaking contributions in the Hamiltonian of the real system necessarily lead to corresponding terms in the Kohn–Sham Hamiltonian. Spin–orbit interactions, for example, require from a formal point of view the presence of terms in the Kohn–Sham potentials that couple to spin or magnetization currents.410 In practice, these terms are often neglected. It is interesting to note that, from a formal point of view, terms in the Kohn–Sham potential that couple to noncollinear spin are not required in the presence of spin–orbit interactions.410
3.6.10 Chermette.
Taking approach (ii) of contribution (3.6.9), a powerful, although limited, method of use for spectroscopy is ligand-field DFT (LFDFT) developed by Daul et al.411,412 This semiempirical method uses all the symmetry constraints included in the ligand-field formalism. Its parameters are extracted from a standard (usually restricted GGA) Kohn–Sham calculation. The spherical symmetry of the atomic densities is obtained by fractional occupation of the involved orbitals.
For instance, for a f7 → d1 transition in a lanthanide compound (here europium), the GGA Kohn–Sham MO occupations corresponding to the 4f6d1 configuration is 6/7e for the (7) MOs strongly localized on the Eu/4f orbital, and 1/5e for the (5) MOs mostly localized on the Eu/5d orbital. See, for example, the case of the Eu(η9-C9H9)2 complex, for which the 30
030 multiplet energy levels have been calculated.413
3.6.11 Krylov.
To properly deal with symmetry breaking, we need to look at properties that are rigorously defined – for example, it is difficult to discuss what spin symmetry means within DFT because S2 is a two-electron operator.414
So spin-contaminated (as traditionally computed) Kohn–Sham DFT or TDDFT solutions might, in fact represent the correct spin densities of the spin-pure correlated many body wave functions – for example, an open-shell doublet radical (such as CH3) in which the unpaired electron has alpha spin, is known to have areas with an excess beta-spin density.414 This cannot be reproduced by a spin-adapted (ROHF) Kohn–Sham determinant (which only allows for an excess alpha density), hence suggesting that a spin-polarized Kohn–Sham determinant provides a more appropriate description.
Because we do not know how to compute the S2 value in DFT, we should formulate this question – whether or not we have unphysical symmetry breaking – in terms of finding molecular properties that could report on it. The same concerns apply to spatial symmetry breaking. One example of how one may approach this problem is a charge-transfer system, such as (He)3+ or the charged ethylene dimer. Charge localization is very sensitive to Hartree–Fock-like symmetry breaking and also to the SIE. The comparison of charge localization patterns against high-level reference data can inform us whether symmetry breaking is real or artificial; some useful examples can be found in ref. 415.
3.6.12 Baerends.
In contribution (3.6.6), the “challenge” is put forward that, with the dissociating H2 molecule as an example, one would never see any symmetry breaking if one employs the exact exchange–correlation functional within Kohn–Sham DFT. Actually, the dissociating H2 molecule is a simple enough system that such a functional can be formulated.416 This functional becomes exact in the dissociation limit and does not lead to any symmetry breaking. Not unexpectedly, it is orbital dependent and employs in addition to the σg orbital the σu orbital. Since such involvement of “unoccupied” orbitals can be regulated via the natural orbital occupations, this ushers in reduced-density-matrix-functional theory. For heavier systems, such functionals become approximate, but can still provide good dissociation curves without symmetry breaking.417 In the Kohn–Sham context, the temperature-assisted occupation DFT (TAO-DFT) of Chai418 is an example of attempts to involve virtual Kohn–Sham orbitals via 1RDM-like occupation schemes.
3.7 What is the role of ensemble methods for DFAs?
3.7.1 Savin.
When discussing ensembles, we should be careful to distinguish between the different cases. Are we interested in describing ensembles associated with degenerate states? For example, do we want to construct universal density functionals that have the same value for all the densities of the ensemble? Do we mean errors that show up due to the locality in our approximations419 – for example, at dissociation? Do we mean ensembles that show up in (even accurate) Kohn–Sham calculations (cf. the pure-state v-representability discussion in ref. 7)? Do these ensembles survive at weak interactions? If a multireference treatment is needed in wave-function theory, can we use ensembles with (semi)local DFAs in DFT? We should keep in mind that the same classical ensemble may correspond to different wave functions, by ignoring the effect of the interference term produced by the sign (phase) of the coefficients. Ensembles are introduced for very different reasons for ground states, excited states and high temperature.
3.7.2 Gould.
Ensemble DFT420 extends the Kohn–Sham method and related theorems to a much wider range of problems – everything including thermal states,50 degenerate states,6,8 “partial” electrons,18 excited states,421,422 and states that give direct access to fundamental gaps.423 DFAs based on ensemble principles should be able to inherit this generality and thereby offer insights into systems that cannot be described by standard DFAs.
Thermal ensembles are rather different to the other types of ensembles. The following discussion thus focuses on other types of ensembles, which give insight into spectroscopic properties of electronic systems, like fundamental and optical gaps.
Despite representational issues in some systems, there are a wide variety of problems for which ensembles can be described cleanly, and for which mappings are one-to-one.424 In such cases, key ensemble functionals may be defined as,6,8
|  | (29) |
|  | (30) |
where
T and
W are the kinetic-energy and two-electron repulsion operators, respectively. Here, the energy is found by a constrained minimization over density matrices
Γ, with different constraints leading to different types of ensemble theories. One may then define an ensemble Kohn–Sham theory, using,
| s[ρ] := 0[ρ], Hxc[ρ] := 1[ρ] − 0[ρ], | (31) |
where
s and
Hxc serve the same role as in conventional DFT. “Constraints” are henceforth implied by the use of calligraphic letters.
Eqn (31) defines an ensemble Kohn–Sham system with orbitals obeying,
|  | (32) |
The density,

, is defined using orbitals with allowed fractional occupation factors,
fi, that reflect the nature of the ensemble. Ensembles are thus amenable the same machinery as standard DFT – that is, by approximating
Hxc[
ρ] and then finding a set of self-consistent orbitals and density. Accommodating ensembles in Kohn–Sham DFT not only extends approximations to new problems (like excited states), but can also remedy deficiencies in standard approximations.
425,426
A major difficulty in treating ensembles is that the minimizing wave functions and ensembles are not guaranteed to be unique and cannot be used explicitly to define functionals – the “nonuniqueness disaster”.427 This is related to issues raised in contribution (3.7.1). One must thus resort to more foundational relationships to further break
Hxc into useful pieces that may then be approximated. A rigorous separation into Hartree–exchange and correlation terms is achieved by using,
|  | (33) |
which gives the usual results for pure states. In the special case of ensembles that preserve fundamental symmetries by equally weighting states related by symmetry operations,
424 one may also rigorously define the Hartree term
FDTH[
ρ] and exchange term
FDTx[
ρ] using the fluctuation-dissipation theorem.
428 The resulting orbital functionals reduce to their usual definitions in pure states. Hybrid functionals formed on these orbital functionals (
e.g., by using
s +
FDTH +
α
FDTx, where
α is a HF mixing parameter) may be defined using ensemble generalized Kohn–Sham theory.
175
An additional challenge in ensemble DFT is that the correlation energy is more complicated than its standard (pure-state) DFT counterpart. Firstly, because it must address multiple states at once. Secondly, because it contains density-driven (DD) correlations,429 which are a consequence of the fact that Kohn–Sham states reproduce the correct total density, but not the correct densities of the individual interacting states included in the ensemble. Gould and Pittalis defined DD correlations in special types of ensembles.429 Fromager then provided a rigorous general scheme for understanding DD correlations.430
3.7.3 Fromager.
Just for the sake of clarity, it is probably good to explain why a distinction has to be made between thermal ensembles and other types of ensembles like, for example, the Gross–Oliveira–Kohn (GOK) ensemble422 or the more recent N-centred ensembles,423 which are (somehow artificially) constructed in order to compute neutral or charged excitation energies in a completely time-independent formalism.
The discussion that follows focuses on the latter type of ensembles, which we could refer to as “pre-defined” ensembles. Indeed, unlike in thermal DFT,431,432 the ensemble weights ξ = (ξ0,ξ1,…, ξI,…) that are assigned to each state within the ensemble will always be known before the ensemble DFT calculation is carried out. They are chosen (in principle, arbitrarily) and fixed. In other words, in the exact theory, the Hohenberg–Kohn theorem is established for a given ensemble or, equivalently, for a given set ξ of weight values. The one-to-one correspondence between local potentials and ensemble densities relies on the extension (from pure ground states to ensembles) of the Rayleigh–Ritz variational principle,421,423
|  | (34) |
where {
EI} are the targeted (ground- and excited-state) energies. The ensemble Hartree-exchange–correlation (Hxc) energy functional
| Hxc[ρ] ≡ EξHxc[ρ] | (35) |
is said to be universal because it does not depend on the external (local) potential. However, it is expected to depend on the ensemble under study, through its weight dependence. The latter originates from the fact that a density
ρ that integrates to a fixed integer number
N of electrons can be both pure-state and ensemble
N-representable at the same time:
423,433 | 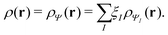 | (36) |
A simple example is provided by the hydrogen atom. The effective 1s orbital
|  | (37) |
which represents the ground state of −∇
r2/2 +
vξ(
r) in the potential
vξ(
r) = ∇
r2[
ϕξ1s(
r)]/(2
ϕξ1s(
r)), has the same density as the GOK ensemble constructed from the regular 1s and 2s orbitals with weights (1 −
ξ) and
ξ, respectively.
In the general many-electron case, the ensemble Hxc functional needs to know if it has to compute the Hxc energy of a pure ground state or of an ensemble consisting of ground and excited states, hence the ξ dependence in EξHxc [ρ]. The extraction of excitation energies from an ensemble DFT calculation reveals the importance of this weight dependence.422,423,434,435 In particular, it has been shown that ensemble density-functional weight derivatives ∂EξHxc[ρ]/∂ξI are directly connected to the derivative discontinuities that the Hxc potential exhibits when a given excited state is incorporated into the ensemble.436–438
In DFT for (canonical, for simplicity) thermal ensembles,431,432,439 the ensemble weights are controlled by a single parameter – the temperature or, equivalently, the inverse temperature β. In thermal DFT, the variational principle is extended to the total (Helmholtz) energy, which contains an entropic contribution:
|  | (38) |
Note that, unlike in GOK or
N-centred ensemble DFT, the minimizing ensemble weights
are energy dependent. Therefore, they are unknown when the calculation starts. Moreover, even though both interacting and noninteracting Kohn–Sham systems are described at the same temperature and share the same (ensemble) thermal density, their ensemble weights are different, simply because Kohn–Sham energies do not match the true interacting ones.
With these major differences in mind, the discussion on ensemble DFAs that follows essentially applies also to thermal DFT. The ensemble-weight dependence of the Hxc functional simply reduces to a temperature dependence.
3.7.4 Gould.
The last few years have seen significant development of new ensemble DFAs – especially for excited states. There are two main approaches: (i) explicit functionals of the density and ensemble weights (i.e., constraints); (ii) ensemble-adaptation of existing functionals.
3.7.5 Loos.
Concerning point (i) of contribution (3.7.4), different strategies have been followed. In ref. 440, Loos and Fromager constructed a weight-dependent LDA (correlation) functional for GOK DFT422 using both finite and infinite uniform electron gases. This functional was employed to compute single and double excitations in one-dimensional systems. In ref. 441, Marut et al. designed, in the spirit of optimally-tuned range-separated hybrid functionals, a two-step system-dependent procedure (resulting in the construction of a weight-dependent exchange functional) to obtain accurate double excitations for two-electron atomic and molecular systems. The transferability of these weight-dependent functionals remains questionable.
3.7.7 Loos.
Several current limitations of ensemble DFT are worth mentioning here:
(1) Self-consistent ensemble DFT calculations still lack a well-defined computational protocol (usual or generalized Kohn–Sham schemes, OEP-type algorithms, CASSCF-type orbital optimization techniques, etc.). How best to correct the ghost-interaction error at an affordable cost is also an open question.444
(2) In GOK DFT,421 one is supposed to know in advance the energy ordering of the excited states, which is far from being straightforward.
(3) Different flavours of ensemble DFT are used depending on the type of excitations targeted. In this context, a unified theory for charge and neutral excitations would be desirable in order to be able to compute both the fundamental and optical gaps from a single calculation.
3.7.8 Fromager.
I would like to complement the discussion in contributions (3.7.4), (3.7.6) and (3.7.7) from the perspective of state-averaged (usually multiconfigurational) wave-function-based methods.445 The incorporation of ensemble-weight dependencies into DFAs is probably the most challenging task in ensemble DFT. Defining Hartree, exchange, and correlation ensemble energies is not as straightforward as in regular ground-state DFT. Various decompositions have actually been proposed.427–430,438 They all have their advantages and drawbacks. A dilemma already appears at the Hartree-only level of approximation. In the original formulation of GOK422 and N-centred423 ensemble DFTs, the ensemble Hartree energy is evaluated from the regular ground-state Hartree density functional | 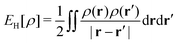 | (39) |
as follows: | 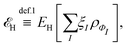 | (40) |
where {ΦI} are trial Kohn–Sham wave functions. While the above definition is formally convenient because it ensures that the Kohn–Sham orbitals are obtained from a single (local) ensemble-density-functional potential (Hartree-only in this case), it is, from a practical point of view, a very poor choice. The reason is that it contains unphysical “ghost” interaction terms between the states.444 The Hartree energy defined in this way also varies quadratically with the ensemble weights, by construction, while the exact ensemble energy varies linearly. At first sight, it seems better to opt for the following definition, | 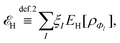 | (41) |
where individual Hartree energies are used instead. The above ensemble Hartree energy is an implicit functional of the ensemble density. If we want to preserve the original formulation of ensemble DFT, where a single local ensemble Kohn–Sham potential is employed, OEP techniques must be employed.175,446
Nevertheless, it is possible to tackle the problem differently. Indeed, an orbital-dependent Hartree-only density functional can be defined using Lieb's maximization (see eqn (29)) and the following approximate expression for the potential-functional ensemble energy:438
|  | (42) |
This procedure can be seen as the Hartree-only version for single-configuration (Kohn–Sham) wave functions of the state-averaged CASSCF (SA-CASSCF) method.
445 Its practical disadvantage is that standard SCF routines cannot be used in this context. Indeed, as each Kohn–Sham state generates its own Hartree potential, there is no single ensemble potential from which the minimizing Kohn–Sham orbitals can be determined (by diagonalization).
175,438,447,448 If we want to avoid the use of OEPs, this is essentially the price to pay for constructing ghost-interaction-free ensemble energies in a systematic and general way. Mapping the true interacting ensemble density onto such an approximate Hartree-only state-averaged ensemble leads to an alternative (in principle, exact) formulation of ensemble DFT.
438 An exact ensemble exchange scheme is obtained along the same lines from the following approximate ensemble energy expression (note that, in practice, complementary fractions of exact and approximate density-functional expressions for the ensemble exchange energy are usually combined
438,448):
| 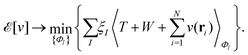 | (43) |
In this case, the individual (nonlocal) exchange potentials are functionals of the individual one-electron reduced density matrices.
Electron correlation can be introduced (approximately) into the theory by recycling the regular (weight-independent) ground-state correlation functional Ec[ρ] as follows:
|  | (44) |
Mapping the true ensemble density onto such an (approximate) ensemble leads to another exact formulation of ensemble DFT. In order to recover the exact ensemble energy, a density-functional correction should then in principle be designed,
|  | (45) |
where {
ΨI} and {
ΦI} are, respectively, the true interacting and auxiliary (generalized Kohn–Sham) density-functional ensembles with density
|  | (46) |
This is perhaps where the challenge in ensemble DFT lies – indeed, in computational studies, Δ
Eξc[
ρ] is usually neglected.
448 It is far from clear how accurate such an approximation is and if error cancellations systematically occur in this context; hence, the (urgent) need for a clearer hierarchy of approximations – that is, a Jacob's ladder for ensembles.
Let us give further insight into the approximation of eqn (44). From the more explicit expression
|  | (47) |
where
Ψ0[
ρ] and
ΦKS0[
ρ] are the standard interacting and Kohn–Sham noninteracting density-functional ground-state wave functions, respectively, we can rewrite the exact ensemble density-functional correlation correction to
eqn (44) as follows:
| 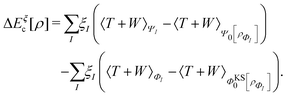 | (48) |
At this point, we stress that the density constraint of
eqn (46) does not imply that, within the ensemble, interacting and Kohn–Sham densities match individually – in general, they do not.
429,430 This can be seen in the regular ground-state limit of the theory, when the weights assigned to the excited states are equal to zero.
438 This specific feature of ensemble DFT is reflected in the implicit weight-dependence of the Kohn–Sham wave functions {
ΦI}.
430 It is related to the concept of density-driven (DD) correlation recently introduced by Gould and Pittalis;
429 see contribution (3.7.2). Moreover, even if the exact individual densities
ρΨI (which can be extracted, in principle exactly, from the Kohn–Sham ensemble
430) were used instead of the bare Kohn–Sham densities
ρΦI, one would still not recover the exact ensemble correlation energy simply because, for a given excited-state density
ρΨI,
Ψ0[
ρΨI] is always a ground-state wave function. The fact that the true excited-state wave function
ΨI differs from
Ψ0[
ρΨI] can be related to the concept of state-driven (SD) correlation.
429 In the light of this analysis, the following decomposition may be used as a guideline for the development of ensemble correlation DFAs:
| 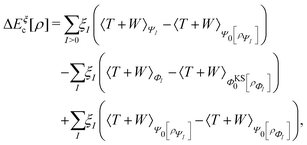 | (49) |
where the ground-state (
I = 0) interacting contributions in the first summation rigorously cancel out.
Let us finally mention that ensemble DFT does not give a direct access to response properties such as oscillator strengths or to Dyson orbitals (in the case of N-centred ensemble DFT423). The extension of Görling–Levy perturbation theory133,449,450 to ensembles should probably be explored for that purpose.
3.7.9 Grimme.
Finite-(electronic)-temperature Kohn–Sham DFT, even with standard GGA or hybrid DFAs, can be used routinely to approximately describe difficult static-correlation problems in large systems even if the resulting energies need to be taken with caution. Such calculations often improve SCF convergence and can be employed, for example, in an MD treatment of high-energy chemistry (mass spectrometry451) or for the analysis of static-correlation effects.452
3.7.10 Chermette.
Most of the ensemble approaches discussed here are for “true” ground states or excited states, which naturally involve an integer (total) number of electrons. However, reactions or excitation processes may be described with a noninteger number of electrons, which mimics the approach of a charged or simply polarized species or particles. The relaxation of the MOs that occurs through the addition or depletion of a small amount of an electron is a tool not (yet) widely used. Organometallic complexes are sensitive to this because of their partially filled d orbitals. As a result, static (quasi-degeneracy) correlation may play a significant role – see, for instance, ref. 453.
3.7.11 Gao.
I would like to add an alternative formulation of the density functional of an ensemble of states in multistate DFT (MSDFT).454,455 We have been experimenting with this approach in the past few years, with excellent results in a variety of applications – including applications to singlet fission,456 proton-coupled electron transfer,455 conical intersections,454 local-valence and charge-transfer excited states,457 and core-level excitation energies.458
Recently, my coworker Dr Yangyi Lu and I proved that MSDFT is an exact DFT in the subspace
N spanned by the lowest N eigenstates of the Hamiltonian.459 It is proved (1) that the Hamiltonian projected onto
N is a matrix functional H[D(r)] of the multistate matrix density D(r) and (2) that variational minimization of the multistate energy, EMS[D] = trV[S−1H(D)], gives the exact energies and densities of all N eigenstates. The second theorem corresponds to an extension of the Theophilou variational principle for the whole subspace460 in terms of H[D(r)], ensuring that the energies and vectors of individual states are obtained simultaneously. In these expressions, D(r) is a matrix of electron densities and transition densities of a set of basis states that represent the ensemble density ρV(r) = trV[S−1D(r)] of the subspace
N, where S is the overlap matrix of the basis states; D(r) is not to be confused with the one-electron density matrix.
The multistate matrix density D(r) can be sufficiently represented by N2 nonorthogonal (necessary) determinants.459 In a departure from Kohn–Sham DFT for the ground state, we introduce in MSDFT an “active space” of N interacting states {ΦA; A = 1,…, N}, each of which is written as a linear combination of N2 nonorthogonal determinants,
, to completely represent D(r) of the real (fully interacting) system. Its matrix elements are computed from one-electron orbitals {χApj(r)} in the manner
| 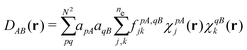 | (50) |
where
fpA,qBjk is the overlap between two Slater determinants of the corresponding (
ne − 1) orbitals. Unlike the diagonal state densities, the transition density
DAB(
r) with
A ≠
B can be positive, negative or complex.
Analogous to the ground-state DFT, we can define a Lieb-like subspace energy functional
|  | (51) |
where
H0 =
T +
W =
H −
v(
r). The constrained minimization in
eqn (51) imposes the condition that the total density of the
N eigenstates of primary interest is identical to the subspace density. The optimal subspace density
ρV(
r) is found by minimizing the multistate energy functional, an implicit functional of
ρV(
r), with respect to
D(
r),
|  | (52) |
The energies of all
N eigenstates of
H within the subspace
N are thus simultaneously determined.
| 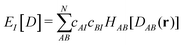 | (53) |
where
I = 1,…,
N and
E1 is the ground state energy. The matrix functional of the full Hamiltonian
H in the subspace
N is given by
|  | (54) |
where
![[scr F, script letter F]](https://www.rsc.org/images/entities/char_e141.gif)
[
D(
r)] is the universal matrix functional, whose elements in terms of one-body orbitals are
| [D(r)] = TMS[D(r)] + EHx[D(r)] + Ec[D(r)] | (55) |
The first and second terms in
eqn (55) are, respectively, the multistate (active space) matrix functionals of the kinetic energy and of the Hartree–exchange energy:
|  | (56) |
| 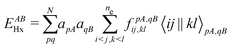 | (57) |
where 〈
ij||
kl〉
Ap,Bq is the two-electron Coulomb-exchange integral with
fAp,Bqij,kl being the coefficient. The multistate exchange–correlation matrix function
Exc[
D(
r)] is defined by
eqn (55), accounting for the remaining correlation energy not included in the multistate active space.
Notice that I have introduced a new class of density functional, the transition density functional (TDF) EABc[D(r)] between states A and B.458 The physical interpretation of the TDF is the dynamic correlation contribution to the electronic coupling between two basis states.457,458 Although the functional form of the TDF is not known (similar to the exchange–correlation functional in Kohn–Sham DFT), in special cases such as spin-coupling interactions, it can be determined with the constraint of spin-multiplet degeneracy with the high-spin state determined separately using Kohn–Sham DFT.457,458 Obviously, as in Kohn–Sham DFT, EABc[D(r)] also includes the corresponding residual kinetic energy not expressed in the orbital term.
One way to construct the multistate active space is to use constrained Kohn–Sham determinants for the states of interest. These non-Aufbau configurations can be optimized via a ΔSCF procedure,461 or by the targeted orbital optimization method.462 If we do not simultaneously optimize {cAI,apA} in eqn (52), the orbitals in each determinant will be separately optimized, and they are generally nonorthogonal. Then, the procedure is equivalent to nonorthogonal state interaction (NOSI), a convenient approximation to the full MSSCF solution.454,455 In this case, one first carries out the optimization of each determinant configuration as a constrained Kohn–Sham DFT calculation.455,463 Then, this is followed by a single step of diagonalization of the Hamiltonian matrix functional (eqn (54)), with the approximations for the off-diagonal elements HAB given in ref. 454, 455 and 457 to yield the energies of the adiabatic states. Consequently, all adiabatic states in the subspace, including the ground state, are treated on an equal footing in the course of minimizing the multistate energy functional.458
Since state interactions are explicitly included in the active space that defines the multistate energy, the effect of interference highlighted in contribution (3.7.1) is naturally included in MSDFT.
3.8 What has DFT told us about the real world?
3.8.1 Jones.
DFT would not be “the workhorse of quantum chemistry and materials science” if it had not provided much information about the real world. This is obvious in areas of particular interest to me, where its ability to make useful predictions of interesting physical properties broke new ground.
However, this is not the main lesson that DFT has taught me about the “real world”. I participated in (and survived) the struggle of a small number of scientists to convince the overwhelming majority of theoretical chemists that density-functional calculations could play an important role in chemistry. This struggle (from the mid-1970s to the breakthrough to general acceptance in the early 1990s) was against a conservative community that, with few exceptions, did not hesitate to “vilify” (Baerends, personal communication), ridicule, or ignore a development that everyone now knows was in its own interests. Such long-running rejection of unfamiliar ideas is certainly not unique in science, but I hope that it will not be repeated in the density-functional community.
3.8.2 Salahub.
Jones's answer about DFT being vilified in the early days reminds me of a tongue-in-cheek paper I wrote in 1999 as part of a Theor. Chem. Acta series reviewing contributions of DFT to end-of-the-century applications.464 Here is the beginning:
“1 am. A faint knock on the downstairs door. Or was it? Then the unmistakable thump of a heavy boot against the door and the crack of the door jamb as it shattered. Had his sordid past caught up with him? The interrogation would be swift and on the spot. Where did that wooden chair come from? And the bare light bulb slowly swaying above it? Whose face was that, almost invisible behind the glare? Inquisitor: Are you now or have you ever been a member of the Xalpha party? Mild-mannered respectable density-functional-theory practitioner (MMRDFTP): What? (Where had he heard that voice before?) Inquisitor: Are you now or have you ever been a member of the Xalpha party? MMRDFTP: I’m a Mild-mannered respectable density-functional-theory practitioner (MMRDFTP). What do you mean by breaking into my house in the middle of the night and hauling me out of bed like that? I was just in the middle of a great dream about an exchange–correlation functional that had the right asymptotic form and took care of dispersion seamlessly. Could have done excited states too, and eminently parallelizable. And now I’ve forgotten what it looked like”
Perhaps this has something to do with the “real world”.
3.8.3 Schwerdtfeger.
I remember the days when John Pople and Walter Kohn each argued their case of what the future will be, wave-function or density-functional based. It is clear that we can reach unprecedented accuracy in wave-function-based theory, testing even the standard model of physics. A nice example here is the accurate determination the fine structure constant from QED.465
And to make it clear: wave-function-based theory should be used wherever it can be used. But this is exactly the point. DFT is applied for large systems because of its low computational scaling law, O(n3), with the number of particles involved n, and where wave-function-based theory has (and in future will still have) real problems – for example, in describing electron correlation for strongly correlated and metallic systems. The electron-correlation problem for metallic systems in wave-function-based theory was already pointed out in early days by Fulde.466 Here, DFT gave us many useful results of the “real world” where ab initio theory is just not able (yet) to do the same job. It has become therefore an invaluable tool for materials science, solid-state physics, and the simulation of biomolecules.
What is perhaps a bit annoying (at least to me) are the “quick fixes” applied to DFT when one does not get reasonably accurate results – I just mention here the better description of electron pairing due to the on-site Coulomb repulsion by the use of the Hubbard term in DFT + U.467,468 On the other hand, many-body theory can be used successfully within a DFT formalism as the many applications in solid-state physics show – for example, by using GW and Bethe–Salpeter theory leading to quite accurate solid-state properties. So the two worlds come together somehow.
3.8.4 Chermette.
Young researchers may be reminded of the difficult youth of DFT in chemistry, even though interesting results were obtained469,470 and interpreted471 in the 1970s and 1980s. A similar situation occurred in other domains, such as the quasi-crystal discovery, not accepted by the crystallographers for a while.472
3.8.5 Kronik.
DFT interacts with the “real world” in three ways:
Confirmation.
Sometimes experimental findings can be conflicting or controversial, owing to sample quality, complexity of measurement, difficulty of interpretation, or all of the above. “Reproducing” the experiment on the computer, using DFT, allows theorists to weigh in on such controversies.
Interpretation.
Often the experimental result is beyond dispute, but it is poorly understood. With DFT, we can easily test for the effect of, for example, adding, moving, or removing an atom; we can examine the role of transition states and metastable states; we can assess what individual (Kohn–Sham) electron orbitals do and more. By doing so, we can explain experiment. The same tasks would range from the exceedingly difficult to the a priori impossible if attempted experimentally.
Prediction.
Suggesting new mechanisms and properties before they have been examined experimentally, or indeed even suggesting new useful molecules or materials before they have been synthesized, once seemed like a distant “holy grail”. It is a testament to the quality of modern DFAs that such predictions are becoming increasingly successful.
3.8.6 Gritsenko.
DFT provides an astonishing example of how, arguably, the most exotic ultra-nonlocal feature of Kohn–Sham theory supplies the missing piece of information about one of the most important experimental characteristics of solid-state physics: the fundamental band gap Eg. The feature in question is a finite upward jump Δxc of the Kohn–Sham exchange–correlation potential of a bulk crystal with a finite Eg, when just a single electron is added to the conductance band. Addition to the too low Kohn–Sham band gap EKSg of a simple estimate of Δxc extracted from the Gritsenko–van Leeuwen–van Lenthe–Baerends (GLLB)321 or Becke–Johnson (BJ)473 model exchange–correlation potentials produced surprisingly good-quality Eg for many extended systems.474
3.8.7 Galli.
DFT has told us about trends in properties and chemical bonding in numerous molecular and condensed phases, in spite of inherent inaccuracies of existing DFAs, and has shown predictive power and great usefulness in interpreting experiments. DFT has also been overused and those instances should of course be corrected. It should be emphasized that DFT is at the basis of all MBPT studies and even of quantum Monte Carlo calculations of solids, where the starting wave function is in most cases constructed from DFT orbitals.
3.8.8 Neese.
There is no doubt in my mind that computational chemistry would not be nearly as popular and important in chemistry as it is today without the huge success that DFT has enjoyed. While linear-scaling wave-function-based approaches have a come a very long way and can now be routinely used in most computational chemistry studies, I do not foresee that even the best linear-scaling approaches will make DFT obsolete in any shape or form. For example, it is difficult to see how correlated wave functions could compete with the speed and accuracy of DFT for geometries and harmonic frequencies. On the other hand, correlated wave functions are conceptually and numerically superior to DFT for a number of properties, for example magnetic properties. Personally, I hope for a fruitful interplay and co-existence, in which computational chemists make the best use of the available computational tools, no matter what theoretical framework they are based on.
3.8.9 Barone.
What is the meaning of the “real world”? All computations are performed on model systems, so that comparison with experiment requires the definition of both the mathematical (here the DFA) and the physical (the system investigated). Since DFT allows us to increase the dimensions of the physical model more than is possible with wave-function methods, we come closer to the “real system”. As a result, the discrepancies with experimental results are more probably related to deficiencies of the mathematical model. Of course, here multilayer (QM/QM′) models play a significant role, at least for nonperiodic systems.
3.8.10 Helgaker.
I wonder whether we as a community would have known less about the role of dispersion in chemistry and physics if DFT had not struggled to describe it. By being able to turn on and off dispersion as described for example by Grimme,384 we have observed the effects of dispersion in a very transparent manner – this was for me, at least, an eye-opener. In general, DFT forces us to discuss the real world in a different manner than wave-function theory.
3.9 What is the status of DFT-based tools for interpretation of chemical phenomena?
3.9.1 Ayers, Chattaraj, Chermette, De Proft, Fuentealba, Geerlings, Liu, Vela, and Yang.
The role of conceptual DFT in this endeavour has been very important: its past and recent accomplishments have been summarized in earlier reviews188,189 and a recent “status” paper475 where also its present status, prospects and issues are scrutinized. The present status can be best understood by considering the aim of conceptual DFT and the philosophy behind its realization.
The aim of conceptual DFT was clarified at a conference in Changsha City, China in 2018, attended by almost all of the most influential workers in the field, and formulated in the above mentioned status paper as “to develop a nonempirical, mathematically and physically sound, density-based, quantum-chemical theory for interpreting and predicting chemical phenomena, especially chemical reactions”.475 This aim should be realized with a philosophy based on three fundamental precepts: observability (our understanding of chemical observations should be based on quantum-mechanical observables – in particular, the energy, the density and their derivatives); universality (the results should not depend on the type of calculations) and mathematical rigour (aiming at a well-defined mathematical framework).
Based on these precepts, conceptual DFT has introduced a number of molecular reactivity descriptors, mostly response functions or descriptors derived from the E(N) curve, that – either alone or in combination with the electronegativity-equalization principle, the hard/soft-acid/base principle, the maximum-hardness principle, or the minimum-electrophilicity principle, for example – have served as valuable tools for the interpretation of experimental and theoretical (computational) data for a wide variety of reactions. Its scope comprises “generalized” acid–base, complexation and redox reactions and a multitude of “classical” organic reaction types including pericyclic reactions, with substrates varying from inorganic to organic and organometallic molecules, to polymers and the solid state. The success of conceptual DFT in pervading a broad range of chemical subdisciplines – from inorganic, organic, and organometallic chemistry to biochemistry and materials chemistry – can be described as getting “insight from numbers”, experimental or theoretical.
Pitfalls and shortcomings are still to be coped with, however, both on the more fundamental issues (e.g., the nature of the E(N) function and the issue of differentiability, the convergence of the E = E[N, v] perturbation series in N and v) and on more applied aspects (e.g., the delineation of the scope of the various principles) before the next step, from interpretation to prediction, can safely be taken; see Section 4.8.
3.9.2 Baerends.
On the topic of differentiability of E(N) hinted at in contribution (3.9.1), let us note that there truly is a fundamental problem.476 In the Euler–Lagrange equation for the optimization of the density, |  | (58) |
the total functional derivative of Ev[ρ] has to be broken down into its partial derivatives | 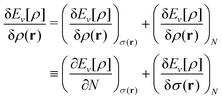 | (59) |
Here, the density is written as a product ρ(r) = Nσ(r) of the particle number N with a shape function σ(r) that integrates to 1.477 According to the theory of optimization under constraints, the Lagrange multiplier μ at the optimum density ρN is equal to the partial derivative with the shape function held constant, ∂E/∂N for short. But the Hohenberg–Kohn theorem does not define the energy for densities with a noninteger number of electrons. So the energy for a density with noninteger N in a neighbourhood of ρN is not defined and the derivative does not exist.
The typical solution in the theory of variations under constraints is to choose some extension of the functional into the domain where it is not defined. This extension is essentially arbitrary, the only requirement is that the extension obeys continuity properties so that the derivative exists. At this point, the Lagrange multiplier, which is the force of constraint keeping the density at integer N, is undetermined. It is determined by the chosen extension of E[ρ] into the noninteger domain. This is not a problem, it is directly related to the well-known gauge freedom of the Kohn–Sham potential, to which an arbitrary constant may be added.476 In a widely cited paper by Parr et al.186 (see also contribution (2.5.3)), the Lagrange multiplier μ has been described as “a characteristic constant for a system”, but without any proof or arguments. This contradicts the essential arbitrariness of the constant μ, and therefore of ∂E/∂N. Atoms and molecules have an ionization energy and an electron affinity – there is no additional physical quantity μ = ∂E/∂N.
The best known choice for extension of Ev[ρ] into the noninteger domain is the one of ref. 18 – namely, forming an ensemble of the ground-state density matrix of the N-electron system with either the density matrix of the ground state of the (N + 1) system or the ground state of the (N − 1) system. This procedure leads to piecewise linear energy behaviour. This choice precludes application of the Euler–Lagrange variation method because the derivative ∂E/∂N does not exist (is discontinuous at the integer point). More extensive discussion of these matters is given in ref. 478.
3.9.3 Liu.
Two schemes for partitioning the total energy in DFT have been applied to understand different chemical processes and transformations.193 From these schemes, a unified view of molecular conformational stability has emerged, in which the electrostatic interaction plays the dominant role, while the contributions of steric repulsion and quantum effects are minor yet indispensable.193 This was also recently utilized to analyse the effects of cooperativity,479 frustration,480 and homochirality.481 Regioselectivity, nucleophilicity, and electrophilicity have also been quantified by information gain and Hirshfeld charge.193 Recent studies of density-based quantities for aromaticity and antiaromaticity yielded two opposite propensities, one for aromaticity and the other for antiaromaticity, depending on the number of π-electrons.193
3.9.4 Chermette.
As pointed out in contribution (3.9.3), for most molecular systems, the electrostatic interactions dominate over steric repulsion and other quantum effects. Accordingly, an analysis of the molecular perturbation introduced by a small charge (typically ±0.1e) leads to interesting insights in the understanding of reactivity.
The perturbed energy can be analysed as a contribution from excited configurations, whose importance may be estimated by their oscillator strengths. In most cases, it appears that only a limited number of excitations contribute significantly to the overall response to the perturbation, suggesting that chemical reactivity can be predicted by analysing the reshuffling of electron density upon excitation.482 The stabilization energy due to interaction between the polarization density and the electrostatic potential δv(r) is given by
|  | (60) |
where
ck2[δ
v(
r)] is the oscillator strength of the
kth excited state and
E0 −
Ek is minus the
kth excitation energy.
483 Therefore,
eqn (60) can be viewed as a minus the energy required to rearrange the electron configuration so that
ck2 electrons are promoted from the ground state to the
kth state. Following the same line of thought, the plot of
ck2versus (
E0 −
Ek) can be considered as a polarization spectrum. The polarization density can be computed as
| 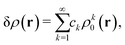 | (61) |
where
ρk0(
r) is the transition density coupling the
kth state to the ground state.
483
From a link between conceptual DFT and statistical thermodynamics, it has been shown that the perturbation energy due to intermolecular electrostatic interactions can be understood in terms of effective work and heat exchange,484 the first-order correction
being the effective work and the second-order correction in eqn (60) being the heat exchange. A polarization entropy and a polarization temperature can also been defined by this analogy. Therefore, using the external electrostatic potential as a probe and the polarization energy, entropy, and density as electronic responses, one can get qualitative and quantitative insight into the reactivity and the selectivity of molecular fragments.483
3.9.5 Fuentealba.
One should not forget that, along with density functionals, one has density functions as a special case. Ramon Carbó-Dorca Carre has studied the mathematical structure of such functions.485
3.9.6 Ayers.
One advantage of the popularity of DFT is that it gave publicity to methods based on the direct analysis of the electron density (and higher-order electron distribution functions), some of which developed concurrently with, or even predated, the emergence of modern DFT. Simply stated, DFT is a useful method not only for predicting reactivity as discussed in contribution (3.9.1) but also for describing and characterizing molecular electronic structure. Indeed, the framework of the quantum theory of atoms in molecules (QTAIM)486 and more generally quantum chemical topology (QCT)487 were largely developed alongside DFT, and use the same quantities (notably the density and its derivatives, various energy densities, and various strategies for characterizing, representing, and approximating the exchange–correlation hole) to obtain insight into molecular structure and chemical bonding.
4 The Future of DFT and DFAs
4.1 What are the important lines of development in DFT and for DFAs?
4.1.1 Gritsenko and Pernal.
Importantly, DFT can resolve a bottleneck problem of wave-function theory and DMFT regarding the reliable description of dynamical electron correlation. Indeed, nondynamical correlation can be efficiently accounted for with the small CAS CI and DMRG ab initio approaches or with DMFT functionals of the extended Löwdin–Shull (ELS) type,64 all in relatively small basis sets. It is the description of the residual dynamical correlation, which requires the inclusion of prohibitively many CI excitations in a sufficiently large basis, or many very weakly occupied natural orbitals, which is difficult to achieve with approximate DMFT functionals.
This bottleneck problem has been efficiently resolved in the CASΠDFT488 and ELS+ (the extension of the above mentioned ELS method)64 methods, which share the following master formula for the electronic energy:
|  | (62) |
Here
Erefe is the CAS or ELS electronic energy,
εc is a standard DFT correlation energy density functional, while
P[
X] is a scaling factor depending on the CAS or ELS on-top pair density
Π(
r,
r) and the density
ρ(
r):
| 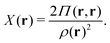 | (63) |
Effectively, the dynamical correlation energy is a functional of
Π(
r,
r) and
ρ(
r) and the method works because
X(
r) locally probes the effect of nondynamical correlation exerted on the dynamical correlation. More precisely, the region where
X(
r)≤1 represents suppression of dynamical correlation by nondynamical correlation, while the region where
X(
r) > 1 indicates enhancement of dynamical correlation in excited states of ionic type.
488 The CASΠDFT and ELS+ methods reproduce well, in a relatively small orbital basis, the accurate potential-energy curves in the complete basis-set limit; also, the CASΠDFT method yields good-quality vertical excitation energies for prototypical molecules.
489 This development shows a promising new direction of combining
ab initio methods with DFAs
via the on-top pair density correlation functional.
4.1.2 Gagliardi.
The on-top pair density is the diagonal part of the two-body density matrix in the coordinate representation. It plays a very general role in wave-function theory and has also been used in many contexts in DFT and DMFT with both single- and multiconfigurational reference states. Many examples of multiconfigurational DFT have shown that use of the pair density gives superior results. One recent utilization of the pair density has occurred in multiconfigurational pair-density functional theory (MC-PDFT).490,491 In MC-PDFT, the energy is computed by combining wave-function theory for the classical components of the electronic energy (kinetic energy, electron–nuclear attraction, and classical electron–electron repulsion) with a functional for the nonclassical components of the energy (exchange and correlation). MC-PDFT is a special case of multiconfigurational nonclassical functional theory (MC-NCFT).492 The expression for the MC-NCFT energy is | EMC-NCFT[ψMC] = EMCclass + Enc[f[ψMC]] | (64) |
where the classical energy EMCclass contains the nucleus–nucleus repulsion, nucleus–electron attraction, classical electron–electron repulsion, and electronic kinetic energy. The nonclassical functional Enc depends on a featurization f of the reference wave function ψMC, which may be the density, on-top density, and their gradients, or other attributes of the wave function – it can, for example, be the on-top functional Eot. MC-PDFT does not use wave-function theory for the internal correlation energy. The method has shown promising performances in several applications involving ground and excited states of multireference systems.493,494 Analytic gradients for the state-specific and state-average formulations are available.495,496
There are two main issues that should be addressed in the future, if MC-PDFT is to become a routine method for multireference systems:
1. How should one choose reference wave functions for these calculations and make them affordable for extended systems? Instead of using the CASSCF wave function, one can use RASSCF or generalized-active-space SCF (GASSCF) wave functions or DMRG wave functions.497 Related to this challenge is the task of automating active-space selections to make these methods more user friendly.
2. The second direction of development is towards the functional form. Currently, functionals are borrowed from the Kohn–Sham world, but specific functionals for this theory should eventually be developed. One possibility is to develop multiconfigurational density-driven functional methods that correct the classical or total energy of a multiconfigurational wave-function method through the use of a machine-learned functional.492
4.1.3 Jensen.
A promising alternative to variants of single-determinantal Kohn–Sham DFT, including hybrid and long-range corrected DFT, is to use range separation not only for the exchange energy but also for the correlation energy, as originally suggested by Savin158 and mentioned by him in contribution (2.2.24); see also contributions (2.5.6) and (2.5.7) from Pernal.
The separation of the electron–electron repulsion into a long-range (lr) part and a short-range (sr) part is usually achieved with the error function erf(−μr12) where μ is an interaction-strength parameter. By means of the AC, one obtains a continuous range of possible DFA models defined by different values of μ ≥ 0. In particular, one obtains Kohn–Sham DFT for μ = 0 and pure wave-function theory (WFT) for μ →∞; the AC from μ = 0 to μ = ∞ thus provides an alternative path to Kohn–Sham DFT.
The more interesting case is to use the AC from a partially interacting system at finite μ > 0 to μ = ∞. One then obtains a hybrid lrWFT–srDFT model, which, for a sufficiently large μ, can describe nondynamical long-range correlations adequately and also give correct spin symmetry of open-shell molecules498 by means of a multideterminant reference wave function. This approach is much simpler than attempting to describe them with a complicated Kohn–Sham DFA functional based on a single determinant. On the other hand, the dynamical short-range correlation effects can be described efficiently with a semilocal srDFT functional connecting to a Kohn–Sham DFT functional in the μ = 0 limit.
In the hypothetical case of short-range exact density functionals and long-range FCI wave functions for any μ value, the total energy would be the same for all μ values. The idea is to use the μ value for which the least computational work is needed to obtain good-quality energies and properties.
The computationally most efficient lrWFT–srDFT model will be for the smallest μ value for which long-range and spin correlations can be described to the desired accuracy, as this leads to the most compact lrWFT part with the smallest number of active orbitals. By comparison with accurate wave-function calculations, it has been found that a value around μ = 0.4 can be considered universally applicable for valence properties of singlet molecules.499–501 Preliminary investigations of transition-metal complexes indicate that a value around μ = 1.0 may be needed to describe their spin correlation sufficiently well.502
A particularly promising choice for the lrWFT part is to use the variational multiconfigurational self-consistent field description, leading to an lrMCSCF–srDFT (MC–srDFT) model. Because it is variational, the MC–srDFT model can also be used for molecular response properties, just like TDDFT in Kohn–Sham DFT – not only for excitation energies and transition moments, but in general for optical, electrical, and magnetic perturbations. For molecules with strong nondynamical correlation, the kinetic energy will be better described with the MC–srDFT model than with Kohn–Sham-DFT, thus a smaller kinetic-energy correction needs to be described by the correlation functional.
Note also that the MC–srDFT approach can be used not only for electronic ground states, but also in state-specific models for excited electronic states of any spin multiplicity and spatial symmetry.
4.1.4 Loos.
Recent developments by Giner, Toulouse, and coworkers on DFT-based basis-set corrections for wave-function theory (based on the range-separation of the electron interaction) are particularly promising with respect to removing, at a low computational cost, the basis-set incompleteness error in high-level calculations.503
4.1.5 Kronik.
The concept of “optimal tuning” has proven to be highly useful for extracting accurate one- and two-electron excitation energies from (relatively) simple DFAs.91 In DFT research, we typically seek an increasingly general DFA, which can come as close as possible to the ideal of a universal functional. But this comes at an increasingly large computational cost. Optimal tuning deviates from this paradigm. It seeks to retain a reasonably simple, low-cost general functional form, in which one or two parameters remain undetermined. The remaining freedom affords enhanced accuracy, with the parameter(s) determined nonempirically, but in a system-specific way, by demanding that a physical constraint be obeyed.
The most successful practical incarnation of this idea has been based on another highly successful idea – namely, that of range-separated hybrid functionals.106,504 These functionals can exhibit an asymptotically correct long-range (free91 or screened505) Coulomb potential, while retaining a useful balance between exchange and correlation in the short range. The range-separation parameter is then tuned, per system, by enforcing the ionization-potential theorem18 (or variants thereof). The approach has been successful in overcoming issues considered very challenging for DFAs, notably the infamous band-gap problem506,507 and the prediction of charge-transfer excitation energies,508,509 through systematic elimination of derivative discontinuity errors. Importantly, this approach restores the physical picture of single- and two-quasi-particle excitation thresholds, by reliably predicting them from the HOMO–LUMO eigenvalue difference of a DFT calculation and the lowest eigenvalue of a TDDFT calculation, respectively, using the same exchange–correlation functional.91 Extensions of these ideas can be expected to continue to play a role in DFT applications to spectroscopy.
4.1.6 Johnson.
Development of a DFA that can eliminate delocalization error is needed. The delocalization error, also known as the many-electron SIE, refers to the tendency of many DFAs to overstabilize systems with highly delocalized electrons or fractional charges on separated moieties.17,510,511 This error affects charge-transfer complexes, extended hydrogen-bonding networks, halogen bonds, organic salt crystals, systems with extended π-conjugation, and transition states of many radical reactions, to list a few examples. It is also responsible for the notorious band-gap problem.21,67,512
While many approaches to reducing delocalization error have been proposed, none is a panacea. Typically, one can reduce delocalization error through a (frequently range-dependent) mixing of local, DFA exchange and nonlocal, Hartree–Fock exchange. However, the optimal mixing is known to be highly system and size dependent.513,514 Development of a practical and universally applicable DFA with minimal delocalization error remains an outstanding challenge and would represent a significant advance.
4.1.7 Gould.
It is likely that any advance on the delocalization-error front would also help to resolve some of the issues with strong correlation, given how closely linked the two problems are. DFAs from Gori-Giorgi and Vuckovic that are based on the SCE limit (see contribution (2.4.5)) offer some innovative ways of thinking about both problems.
4.1.8 Görling.
A promising line of work in DFT is the development of correlation functionals based on the ACFD theorem.100,101 Such correlation functionals are used in conjunction with an exact treatment of all other parts of the total energy, obtained by simply evaluating the expression of the Hartree–Fock total energy with Kohn–Sham orbitals. The simplest of these correlation functionals is based on the RPA.102–104 Already the RPA yields competitive reaction and transition-state energies but can in addition treat noncovalent interactions.
There are various ways to go beyond the RPA. Highly promising are σ-functionals,515,516 which are technically closely related to the RPA but formally rooted in many-body perturbation theory along the AC. Methods using σ-functionals are distinctively more accurate than RPA-based or conventional functionals – they reach chemical accuracy for main-group chemistry and treat noncovalent interactions accurately.
While some methods based on the ACFD theorem are computationally expensive, this is not at all true for functionals within the RPA or for σ-functionals. These are typically evaluated in a post-SCF way, ideally using orbitals and eigenvalues from hybrid DFT methods. In this case, the post-SCF calculation of the total energy using the RPA or the σ-functional requires less computational time than the preceding hybrid calculation and thus can be easily carried out routinely.
There is much room for further developing correlation functionals based on the ACFD theorem and these functionals open up a new area for DFT – the field of highly accurate electronic-structure calculations, so far dominated by wave-function methods like the hierarchy of coupled-cluster methods.
4.1.9 Xu.
Despite being highly successful for main-group chemistry,273,336,517 the PT2-based double-hybrid approximations inherit the intrinsic deficiency of the PT2 correlation model for nondynamical correlation, which hinders their application to some of the challenging problems of DFT, such as stretched H2 and other molecules (see contribution (3.4.1)) without symmetry breaking (see 3.6) and transition-metal complexes (see contributions (3.2.2) and (4.1.11)).
A simple replacement of standard PT2 by more sophisticated correlation models from wave-function theory does not seem to lead to a notable improvement in accuracy despite the higher cost.518 Some recent efforts to develop efficient models that go beyond PT2 for double-hybrid approximations have led to some encouraging schemes for further progress.519–522
4.1.10 Kaupp and Arbuznikov.
One generalization of the concept of hybrid functionals that tries to account for local differences in the relative importance of exchange and correlation as well as for the differing spatial demands of different property operators is to use position-dependent Hartree–Fock exchange admixture in local hybrid functionals (LHs).318,319,523 While this introduces the ambiguities of locally mixing exchange-energy densities (the “gauge-problem” of LHs524–527) and some (manageable) additional requirements regarding two-electron integrals compared with standard (“global”) hybrids, the advantages of position-dependent exchange admixtures for various properties depending on different regions of space have been demonstrated.312,523,528,529
These advantages extend also to TDDFT computations of various types of excitations, including core, valence, and Rydberg excitations, with particularly good performances for triplet excitations.530,531 In general, LHs give extra flexibility to balance minimal self-interaction or delocalization errors in some regions of space with the simulation of left–right correlation in bonds. LHs can be further extended in various directions by combining with range separation (e.g., local range separation532–534 and range-separated LHs535,536), by adding dispersion either via correction terms or via nonlocal van der Waals functionals, potentially by adding nonlocal rung-5 correlation contributions, or by adding corrections for strong correlation.
4.1.11 Xu.
There is an ongoing effort devoted to the development of reference data sets that are sufficiently accurate for benchmarking functional performance.537,538 A recent progress in the community is the emergence of large data sets for the main-group chemistry – for example, the MGCDB84 set with about 5000 data points maintained by Head-Gordon's group273 and the GMTKN55 set of Grimme's group with about 1500 data points.336 Comprehensive benchmarking of existing DFAs for main-group chemistry has become a reality, numerically validating the concept of Jacob's ladder of Kohn–Sham DFT by demonstrating that a higher-rung DFA is, in general, more accurate than a lower-rung DFA.336
For transition-metal systems, the situation is more complex and less developed than for main-group systems.539–541 Large transition-metal test sets with accurate reference data are urgently needed. It is not merely important for benchmarking Kohn–Sham DFAs, but also fundamentally important for understanding the limitations of current Kohn–Sham DFAs for strongly-correlated systems as mentioned in contribution (3.4.1), since transition-metal systems often have a strong multireference character.
4.1.12 Ruzsinszky.
Density functionals at the meta-GGA level harbour a great potential that has not been fully exploited. The excellent performance of the SCAN and r2SCAN functionals and the deorbitalized meta-GGA versions542,543 work very well for structural and energetic properties. Less is known in practice about the capability of some meta-GGAs for fundamental band gaps512 and excited states. While the TB-mBJ potential544 delivers accurate band gaps, its accuracy originates from fitting.
The recent TASK545 and modified (mTASK)546 meta-GGA DFAs are energy functionals developed explicitly for band gaps. Both DFAs excelled in accuracy for band gaps of some important but limited test sets of bulk solids and two-dimensional materials. However, more tests should be done in order to reveal the strengths (and limitations as well) and applicability of these meta-GGAs. With more information, the TASK and mTASK approximations can compete with available hybrid functionals such as the HSE06 functional in accuracy, at a more favourable computational cost. Such applications for the fundamental band gap could initiate the development of exchange–correlation kernels for optical response properties constructed from these meta-GGA functionals. This latter possibility is an obvious advantage of DFAs that possess functional derivatives. The static exchange–correlation kernel from a DFA is its second functional derivative with respect to the density, which needs to exist for this approximation.
4.1.13 Chattaraj.
Orbital-free DFT with approximate interacting kinetic-energy functionals should be explored further.547,548
4.1.14 Fuentealba.
The question is: Is there any hope to get a relatively accurate kinetic-energy functional? It must be highly nonlocal. Machine learning (ML) may help.
4.1.15 Trickey.
Apropos ML and orbital-free DFT, a warning is in order about good answers for bad reasons. There have been several instances of machine-learning manuscripts that purported to provide a kinetic-energy density functional (KEDF) but the functional dependence on the density ρ was such that ordinary uniform scaling of Ts[ρ] was violated.
4.1.16 Carter.
Orbital-free DFT simulations that utilize existing nonlocal KEDFs are already quite accurate for a number of properties of solid and liquid main-group metals549 – see, for example, an orbital-free DFT MD study of the dynamics of liquid tin in ref. 550 using our open-source orbital-free DFT code PROFESS 3.0.551 These nonlocal KEDFs – see ref. 552 for a software library – are successful for such nearly-free electron-like systems because they are directly derived from the physics of the perturbed free-electron gas (the Lindhard function); for recent analysis, see ref. 553 and 554.
The real challenge is to develop KEDFs that can describe molecules and transition metals (similar problems will exist for f-block elements). The inherent angular momentum dependence of the electron distributions – captured by orbitals but not by densities – makes KEDF development for far-from-uniform densities truly a grand challenge. Self-consistent all-electron calculations, without pseudopotentials/effective core potentials, are also a huge challenge due to the same issues. We have shown how difficult this is to accomplish in several papers, where we can achieve small wins but easily break our models as well; see, for example, ref. 555–559, as I believe strongly – as discussed later in contribution (4.4.4) – in the value of publishing failures to gain insight into how to advance the field.
4.1.17 Trickey.
We too have been working on orbital-free DFT with emphasis on one-point KEDFs designed to satisfy constraints. One can get surprisingly good forces from those, but at the cost (so far) of inaccurate (too high) energies.560,561 An oft-ignored requirement on KEDFs is their N-representability; see Ayers and Liu.562 See the preceding remarks by Carter about nonlocal functionals. Also note her remark about local pseudopotentials. It may be that orbital-free DFT is forced into all-electron calculations of a modified projected-augmented wave (PAW) type.
A crucial point for the orbital-free DFT agenda that often goes undiscussed is to get rid of the orbital dependence in DFAs. This is the antithesis of much of the activity in the quantum chemistry community, as much of the discussion in this roundtable confirms. We have made considerable progress on de-orbitalization of meta-GGA DFAs542,543,563 by inclusion of a dimensionless ∇2ρ dependence, though the success of that approach is quite dependent upon the numerical stability of the parent meta-GGA DFA.
4.1.18 Carter.
We also did some work on single-point KEDFs, based on pointwise kinetic energy density and ELF analyses, emphasizing approaches that enable self-consistent calculations.564,565 Most single-point GGA KEDFs are unable to converge densities self-consistently, with the VT84F KEDF of Trickey et al. being a notable exception,560,561,564 rendering most of them impractical for most applications.
Our single-point KEDFs have no problems converging and yield properties in good agreement with Kohn–Sham DFT for the usual materials – simple metals – but again, we can easily break them. Just study a vacancy or an alloy, both of which by contrast are handled well by our 1999 nonlocal WGC KEDF.566,567 Our 2015 pointwise analysis of our single-point KEDFs compared to the WGC KEDF indicates at least some of what is needed to help improve such single-point KEDFs565 – namely, reproducing of the inherent multivaluedness of the non-von-Weizsäcker component of the GGA enhancement factor when plotted against the reduced density gradient. The WGC KEDF, remarkably, does so, indicating yet another fundamental reason it is so accurate for simple metals.
4.1.19 Carter.
Regarding N-representability in contribution (4.1.17): this is more difficult than it seems – we worked on this for quite some time, without much success; see Chapter 7 of ref. 568.
4.1.20 Liu.
Relevant to KEDFs in orbital-free DFT is the Pauli energy, which has recently been employed to identify strong covalent interactions.197 If approximate KEDFs are utilized for the same purpose, miserable results are obtained.569 This quality appraisal test for approximate KEDFs shows that they are unable to accurately account for the kinetic energy distribution in the medium range away from nuclei, where chemical bonding takes place and the Pauli energy plays a crucial role.
4.1.21 Teale and Helgaker.
Recently, we addressed the issue of attempting to solve the Euler–Lagrange equation of orbital-free DFT in the all-electron context.570 Using a second-order optimization method based on the trust-region image method (TRIM),386 we could robustly solve the equation for many systems by simultaneously optimizing the density and the chemical potential in the saddle function
. An interesting finding is that more complicated GGA-type functionals often show an erroneous nonconvex behaviour for the model
s[ρ] (where tilde indicates an approximate quantity). As a result, many solutions (rather than one solution) to the Euler–Lagrange problem with a given particle number N are found. Since Ts[ρ] is the noninteracting limit of F[ρ] and since both functionals are convex with respect to the density variations, it would be interesting to explore techniques to impose convexity on approximate
s[ρ] and
[ρ] =
s[ρ] + EH[ρ] + Ẽxc[ρ].
4.1.22 Trickey.
This remark from Teale and Helgaker is interesting because it suggests a different kind of constraint to impose in the construction of better KEDFs. It also will be important to see what the TRIM method does on a modern, constraint-based (all-electron) generalized gradient approximation such as our VT84F.561 By the way, since GGA KEDFs are inherently singular, a direction of interest to us is nonsingular combinations of reduced density derivatives.
4.1.23 Vignale.
Orbital-free approaches can be valuable not only in static DFT but also in time-dependent DFT. I would like to point out the existence of an orbital-free quantum-continuum-mechanics (QCM) approach,571–573 which offers an alternative to the time-dependent Kohn–Sham approach in calculating the dynamics of interacting electronic systems. This approach is based on the observation that the density ρ(r, t) and current j(r, t) of the many-body system obey the exact equations of motion | 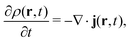 | (65) |
and |  | (66) |
where me is the electron mass, v(r, t) is the potential and
(r, t) is the force density arising from interactions between the particles. The calculation of the force density from the expectation value of the corresponding operator is a prohibitively difficult task: however, to the extent that we trust the basic tenets of time-dependent density and current DFT, we can assume that
is a functional of the basic variables ρ and j. If an approximate form of this functional is adopted, then eqn (65) and (66) become a closed set of partial differential equations, akin to the equations of fluid mechanics, which can yield a huge amount of information about the evolution of the system without invoking the exact wave function. A particularly simple and appealing approximation to the force functional was proposed in ref. 571 and 572 for the linear response regime – that is, when the system is assumed to remain close to the ground state. The approximate force is then given by | 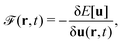 | (67) |
where E[u] is the energy (kinetic plus potential) of the state obtained from the ground-state wave function by applying a position- and time-dependent translation operator with displacement vector u(r, t). The displacement field is related to the current and the density by the relation | 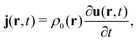 | (68) |
where ρ0(r) is the ground-state density and the functional derivative is evaluated to first order in u. This approximation was dubbed the “elastic approximation” in ref. 571 and 572 and reduces the problem of finding excitation energies to a standard eigenvalue problem with a dynamical matrix that is constructed from ground-state properties such as the pair correlation function and the one-particle density matrix. Only a few applications of this theory have been reported to date – see ref. 574 for a very recent one.
The elastic approximation is expected to work well for systems whose dynamics is dominated by collective modes, but not so well for independent-particle dynamics. There is much room for improvement, which makes this an exciting direction of research. Just to mention one possibility, the elastic approximation assumes that the force is instantaneously determined by the current: this leads to infinitely sharp excitation energies. We could go beyond the elastic approximation by introducing a physically motivated form of time retardation, which would immediately lead to more realistic spectra in extended systems.
4.2 What role will DFT play in multiscale and embedding methods?
4.2.1 Salahub.
I think Perdew's comment in contribution (2.2.1) situates DFT appropriately for multiscale modelling applications as providing “almost the right answer for almost the right reason at almost the right price”. QM/MM or embedding models have a “high-accuracy” method embedded in a “low-accuracy” method. In situations where DFT accuracy is good enough and if the speed is adequate, then DFT can be the high-accuracy method, usually coupled with an MM force field or various solvation models for the low-accuracy method. If DFT speed is an issue, then DFT can be used to calibrate faster semiempirical methods, like DFTB, again combined with an MM force field, for example. DFT can also be the embedding method as with frozen-density embedding theory, requiring kinetic-energy functionals.
4.2.2 Carter.
Beyond chemical applications, I want to remind readers of early work done to develop multiscale methods coupling quantum mechanics to higher-length-scale methods for studying materials properties, in order to simulate phenomena that cannot be handled properly by one scale alone – see, for example, this brief review from 15 years ago.575 While the coupled quantum–atomistic methods will be familiar to this readership (very much in the spirit of QM/MM and/or ab initio molecular dynamics/Monte Carlo), there are examples of coupling quantum mechanics (typically DFT) to continuum solid-mechanics methods, with feedback between scales that could offer ideas to build upon in the chemistry/physics realm going forward.
4.2.3 Galli.
Embedding techniques based on DFT are having an increasingly high impact in the study of highly correlated materials. There are many interesting problems that naturally lend themselves to a quantum-embedding description – for example, spin defects in solids or more generally point defects in materials, active site of catalysts, molecular adsorbates on surfaces, and nanostructures embedded in condensed systems, including solvents, to name a few. The great majority of embedding theories used in the literature today have some DFT component (e.g., wave-function-method embedding in DFT, DMFT, and Green's-function-based embedding). In addition, using embedding theories, one may define second-quantized Hamiltonians and devise frameworks to carry out quantum-mechanical calculations for solids on near-term quantum computers – see, for example, ref. 576.
4.2.4 Wesolowski.
The Hohenberg–Kohn theorems and Kohn–Sham formulation of DFT are crucial for multiscale and/or embedding methods that apply multiplicative embedding operators (embedding potentials). The formal framework of frozen-density embedding theory (FDET) establishes the exact relations between the optimal embedded wave function, the embedding potential, and the Hohenberg–Kohn energy functional, for any nonnegative real function ρB(r) used as the only quantum descriptor for the environment of an embedded system. For embedded wave functions obtained variationally, the FDET energy functional
satisfies the following equality by construction:577 | 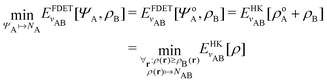 | (69) |
where
. Recently, an expression for the total energy that (similarly to eqn (69)) is consistent with the Hohenberg–Kohn energy functional, was derived also for methods in which the correlation energy is obtained as a nonvariational correction to variationally obtained wave functions.578 For whatever form of the embedded wave function, including the one introduced by Wesolowski and Warshel,579 FDET represents a bottom-up approach to deal with the quantum embedding problem in multilevel/multiscale simulations.
The optimal total density is the sum of individual components ρB(r) and ρoA(r) considered as independent variables in the total energy expression. Such a choice of independent variables makes it possible to use the information about the environment of the embedded species obtained from any physical model capable of delivering ρB(r). Generating ρB(r) using a wave-function description of the environment is one of several possible choices. Other choices include ρB(r) obtained from continuum models of the environment,580 from X-ray diffraction data581 generated using a library of molecular electron densities,582 or from a simplified treatment of the electronic polarization.583 A particular version of FDET, where a noninteracting reference system is used for both ρA(r) and ρB(r) and where both densities are subject to optimization, is equivalent to Cortona's formulation of DFT.584
4.2.5 Carter.
The earliest FDET actually precedes Wesolowski and Warshel's 1993 paper; that credit should go to Warshel's, who proposed the first DFT-in-DFT embedding using KEDFs.584 As far as I am aware, the first WFT-in-DFT embedding utilizing this idea of an embedding potential containing a KEDF potential, as in FDET, was introduced by my group in 1998 in ref. 585, where we carried out, for example, MP4-in-DFT calculations for CO adsorbed on Cu(111).585 A follow-up, more detailed paper discussing our KEDF-based embedded correlated wave-function (ECW) theory also provides in the introduction important context of earlier embedded wave-function theories, for those interested in the history of the field.586 A 2008 review article summarizes the state of embedding (and other) electronic-structure methods for solids at that time.587 As Wesolowski points out in contribution (4.2.4), the FDET formalism subsequently was generalized by Wesolowski and coworkers to encompass methods beyond DFT, including a correlated wave-function treatment of subsystems.
However, for all the reasons summarized above, when discussing orbital-free DFT, the KEDF potentials introduce errors one would like to avoid, since we do not yet have KEDFs that reliably work across the periodic table. Because of this, more than 10 years ago we proposed density-functional embedding theory (DFET),588 in which one eschews use of KEDF potentials and instead uses OEP theory to solve for an exact (within a given DFA) embedding potential that describes the interaction between the embedded region and its environment. (Note that DFET is fully generalizable to more than two subsystems but, since we are mostly interested in fairly localized phenomena, typically two subsystems of a cluster of atoms embedded in a periodic slab background is sufficient.) This embedding potential thus solved for is then “frozen”, with no approximation other than the choice of exchange–correlation functional used to perform the OEP calculation. Since exchange–correlation functionals in use today are much more accurate and transferable across the periodic table than KEDFs, the embedding potential thus derived is much more accurate as well. This frozen embedding potential then is added as a one-electron operator to the cluster Hamiltonian. Thereafter, one can exploit readily any quantum-chemistry method for conducting the ECW calculations.
See ref. 589 for a brief review and ref. 590 for a more in-depth review of DFET591 and its cousin, potential-functional embedding theory (PFET).592 The latter can deliver self-consistent embedding potentials for hybrid ECW/DFT systems,593–595 albeit at considerable cost. Frankly, we have yet to find cases in which such self-consistency was terribly important, although I imagine such cases will emerge. If one is careful to include sufficient numbers of atoms in the embedded region such that the embedding potential does not overlap the phenomenon of interest but instead is essentially a physical boundary condition, then the frozen exact embedding potential we derive from DFET works very well. Thus we have continued to use DFET/ECW theory rather than PFET – with considerable success – to study problems where conventional DFAs fail, such as for phenomena involving electron transfer596 and excited states involved in electrochemistry and photochemistry on metals; for a recent review on the latter, see ref. 597. You are welcome to utilize our codes that compute embedding potentials and the AO-integrals in a variety of formats (see ref. 598) to try these calculations for yourself. There you can also find codes for a generalization of DFET to nonlocal embedding potentials that can also describe covalently bonded systems, in what we refer to as density-matrix-functional embedding theory (DMFET), where the same idea of using OEP is applied to density matrices rather than densities.599,600
Recent benchmarks that we have conducted comparing electrochemical carbon dioxide reduction modelled by a conventional DFA versus DFET/ECW theory reveals critical insights for modellers: for qualitative conclusions regarding reactions that do not involve electron transfer, the DFA is acceptable.601 By contrast, for any step involving electron transfer, specifically proton-coupled electron transfer (which we find to be the most favourable pathway), the DFA fails on multiple fronts (specifically it yields results inconsistent with experiments) due to too facile electron transfer (as expected from self-interaction error and the lack of a derivative discontinuity) whereas DFET/ECW predictions agree with experiments and produces qualitatively different products than the DFA602 – a cautionary tale for DFT modellers of electrochemistry.
Finally, we recently extended DFET/ECW theory to ionic/covalent materials; nearly all our previous work was done on metals. Before solving for the embedding potential with OEP theory, we cap the dangling bonds that were created at fragment edges by initial covalent bond cleavage, while partitioning atoms into subsystems. The capping eliminates potential spin-polarization artefacts that unpaired electrons at fragment edges would produce. The DFET theory is modified to account for the density of the capping atoms while solving for the embedding potential.603 An interesting sustainable energy application using this new theory examined metal-to-ligand charge-transfer states in a Ru-bpy dye attached to a titania cluster, as a model for such excitations in a dye-sensitized solar cell. The ECW calculations were conducted at the embedded CASPT2 level, predicting both lifetimes of singlet excited states and the positioning of triplet excited states, in order to consider the competition between fluorescent and phosphorescent decay.604
4.2.6 Wesolowski.
Owing to the consistency with the Hohenberg–Kohn energy functional, the formal framework of FDET provides a convenient tool for identifying the approximations/assumptions in any method that uses a multiplicative embedding operator. Multiplicative embedding operators are used commonly in QM/MM approaches, where they represent the classical electrostatic interactions, but also in various quantum embedding methods including those reviewed in contribution (4.2.5). A direct comparison of the expressions for the energy and the embedded potential of these methods with their FDET counterparts for each method discussed in contribution (4.2.5) is straightforward. For most of the methods, the approximations are easy to identify.
Concerning the potential-functional embedding theory (PDFT), FDET expressions for the total energy and the embedding potential admit also the embedded wave function and the environment density obtained from PDFT. In such a case, the total energies and embedding potentials of PFET and FDET are expected to be the same, in the absence of additional assumptions and approximations. In their exact form, both approaches target the same solutions. The identity of the corresponding quantities, one given as an explicit functional of the environment density (FDET) and one not (self-consistent PFET), might lead to a better understanding of the relevant density functionals.
Concerning Cortona's formulation of DFT applied originally to atoms in solids, it is worthwhile to recall an intriguing observation regarding LDA made when it was used for intermolecular complexes in our exploratory works.605–607 For such complexes, LDA is known to be inadequate for approximating the exchange–correlation energy. However, when applied simultaneously for both the exchange–correlation and non-additive kinetic-energy functionals, LDA yields surprisingly good interaction energies. The reasons for this apparent compensation of errors remains an open question.
4.2.7 Piecuch.
I have no doubt that DFT will continue to play a major role in the development of multiscale and embedding methods. I have been impressed by the ability of the FDET approach of Wesołowski and Warshel579 to compete with the considerably more expensive high-level equation-of-motion coupled-cluster calculations with singles, doubles, and noniterative completely renormalized triples (δ-CR-EOMCC(2,3)) in accurately reproducing the experimentally observed shifts of excitation energies due to hydrogen bonding.608
While this question may not belong to this section, and it may very well be that it is even ill-defined, our results obtained in ref. 608, especially the failure of the supermolecular TDDFT approach to produce accurate results for the same spectral shifts, made me wonder if practical implementations of TDDFT, which invoke a variety of approximations, satisfy the property of size intensivity of excitation energies (satisfied by properly developed methods based on equation-of-motion coupled-cluster theory). One might say that FDET and other embedding techniques are size intensive by design, which is yet another argument in their favour in applications involving excitation spectra in condensed phases.
We recently used the ab initio embedding scheme called the effective-fragment-potential approach609 combined with the aforementioned δ-CR-EOMCC(2,3) calculations, properly calibrated DFT and TDDFT methods to optimize geometries, and the DFT continuum solvation model based on the solute-electron-density approach610 to accurately model photochemistry of the strongest known super photobase abbreviated as FR0-SB in various alcohol solutions.611 This would not be possible without mixing DFT and TDDFT with embedding and ab initio approaches.
4.2.8 Fromager.
An alternative approach to quantum embedding initiated with the seminal work of Knizia and Chan612 on density-matrix embedding theory (DMET) has been intensively developed in the last few years in both condensed-matter physics613–615 and quantum chemistry.616,617 At first sight, DMET looks more like a wave-function-based method that has nothing to do with DFT. Nevertheless, connections can be made when the convergence criteria involve diagonal elements of the (one-electron reduced) density matrix only.615,618,619 In this context, the localized “impurity” orbital occupations
play the role of the density and the correlation embedding potential,616 which is used in the full-size system, is a collection of one-electron (“on-site” in lattice models615) energies vi. The latter are adjusted so that the corresponding embedded impurity orbitals have the same occupation as in the full-size system. Referring to a noninteracting Kohn–Sham (full-size) system in this context is appealing because it is a way to “exactify” the embedding procedure.
In regular DMET, the bath orbitals (which exchange electrons with the impurity orbitals) are constructed from the Schmidt decomposition of the approximate mean-field wave function (which is computed for the full system). The resulting reduced-in-size “impurity + bath” cluster is then treated in wave-function theory as a closed system.
Sekaran et al. have recently shown that this procedure is equivalent to a (much simpler) density matrix-functional Householder transformation when the density matrix is idempotent.615 They have also shown that, when the transformation is applied to a correlated density matrix, the cluster becomes an open subsystem. Therefore, if the full system is described at the noninteracting Kohn–Sham level (which is still exact density-wise), then the usual separation of the cluster from its environment is perfectly justified. The correlation potential then learns from the cluster (in which interactions are reintroduced, after applying the Householder transformation) through the density constraint.
Thus, we obtain a new type of density-functional approximation (with an implicit dependence on the density) where we can afford an accurate description of strong electron correlation. Obviously, in general, the cluster's environment (which is usually neglected) contributes to the total correlation energy. A formally exact density-functional embedding theory would in principle be obtained by deriving, in this context, a multireference version of Görling–Levy perturbation theory.133,449,450,615
4.2.9 Grimme.
While Kohn–Sham-DFT calculations with accurate DFAs in combination with good one-particle basis sets are feasible for molecules with a few hundreds of atoms, they are still computationally too demanding for many purposes – for example in large scale screening applications, for the combinatorial problem of conformational sampling of flexible molecules, or the computation of vibrational Gibbs free energies of large systems. While the initial steps in typical multilevel approaches can be conducted routinely at a semiempirical or force-field level,281 at some point in the applied filtering procedures, higher accuracy is required – in particular, for relative (chemical) energies.
This motivated the development of composite Kohn–Sham-DFT methods – for example, from the 3c-family B97-3c or r2SCAN-3c620,621 at the (meta)GGA level. The sought-after compromise between computational effort and accuracy is achieved here by applying tailored, medium-sized atom-centred AO basis sets on top of standard or slightly modified DFAs and adding appropriate atom pairwise potentials to account for dispersion and basis-set incompleteness. The recently proposed r2SCAN-3c method outperforms some hybrid-DFT/QZ approaches for reaction and conformational energies as well as for noncovalent interactions at a speed-up of two to three orders of magnitude.621
4.2.10 Köster.
For QM/MM MD applications, Kohn–Sham DFT with density fitting or auxiliary DFT (ADFT) are very promising QM methods.622 In combination with DFT-optimized basis sets and automatically generated auxiliary functions, ADFT Born–Oppenheimer MD simulations on the nanosecond timescale are possible. These calculations permit the simulation of finite-system melting, to determine the corresponding melting temperatures and latent heats.623 The extension of these calculations to QM/MM models will allow the simulation of finite-system phase transitions in MM environments within the NVT and NPT ensembles.
4.2.11 Gao.
A general approach that goes beyond QM/MM are the fragment-based methods.624 In 2013, we edited a special issue of Accounts of Chemical Research on this topic.625
Fragment-based methods such as the explicit polarization (X-Pol) model can be designed as general QM/QM embedding approaches in which each fragment can be individually represented by any electronic-structure method, with the inclusion of the instantaneous environmental effects through Hartree, Pauli exchange, and dispersion potentials.626 Importantly, X-Pol and other fragment-based methods provide a framework for the development of next-generation quantum-mechanics force fields (QMFFs) for condensed-phase and biomolecular simulations.627 In a QMFF, QM effects such as polarization, charge transfer, and the change of the potential-energy surface itself due to dynamical fluctuations as well as chemical reactions are naturally included. These effects would be very difficult, if not impossible, to describe using the current MM force fields.
4.3 In what areas of application are improvements needed?
4.3.1 Helgaker, Teale, and Laestadius.
Current DFT (CDFT), in which the density functional depends on both the charge density ρ and the paramagnetic component of the current density jp, was introduced in 1987 by Vignale and Rasolt.628 The initial works assumed a Hohenberg–Kohn-type theorem, but it was later recognized that no such theorem had been rigorously established.36 Moreover, the conclusion in ref. 36 was that the pair of densities ρ and jp cannot determine the scalar and vector potentials v and A since a wave function can be the ground state of infinitely many systems when the flexibility of a vector potential is added. This observation rules out a Hohenberg–Kohn theorem for the paramagnetic current density. Regarding the total (as opposed to paramagnetic) current, no Hohenberg–Kohn-type theorem has so far been established but it is not precluded either since no counterexamples have been found.
Nevertheless, the relationship between E[v,A] and F[ρ,jp] is sufficient to establish the Vignale–Rasolt formulation as a rigorous extension of DFT to systems in an external magnetic field. To obtain a convex formulation, however, the change of variables u = v + |A|2/2 is needed, imposing a formulation of the theory where the potential space can absorb the norm squared of the vector potential.629 Such a formulation of CDFT inherits the mathematical structure of standard DFT, only lacking the uniqueness provided by a Hohenberg–Kohn result.
The lack of a Hohenberg–Kohn theorem for CDFT has led to confusion in the literature and some (erroneous) claims questioning the validity of the Vignale–Rasolt formulation – see ref. 630 and 631 for a discussion of these points. Aside from these controversies, questions regarding the mathematical properties of F[ρ,jp] remained unclear, particularly regarding whether a formulation analogous to Lieb's treatment of DFT could be established for CDFT.
Such a Legendre–Fenchel formulation of CDFT was developed in ref. 631–633 but the equivalence of the Vignale–Rasolt constrained-search functional and the Lieb-type functional was only very recently established with the proof of the lower semicontinuity and expectation-valued nature of F[ρ,jp].634 The expectation-valuedness is important for the AC since it allows the energy to be partitioned (into exchange and correlation parts) in terms of the minimizing density matrix (or wave function) that satisfies the density constraint.
The Kohn–Sham formulation of CDFT was introduced already in 1987628,635 and recently several practical implementations of this approach for general molecular systems using London atomic orbitals for gauge-origin independence have appeared.636–638 As CDFT becomes a more widely applicable and practical tool for molecular simulations, several open questions remain both from a theoretical and a numerical point of view:
Representability.
For CDFT, representability issues may be more acute.639,640 To what extent may ensemble approaches play a role in this context?
Current-dependent functionals.
Approximate current-dependent exchange–correlation functionals Exc[ρ,jp] are still in an early stage of development although some approaches have been presented for extending existing DFAs.641–645 Those based on meta-GGA functionals have shown some promise in strong fields.39 However, improvements for low-field properties such as NMR shieldings, central to chemistry, are more modest.646 What is the optimal gauge-invariant parameterization of Exc[ρ,jp]? How may new functionals be developed and tested?
Alternative formulations of DFT in a magnetic field.
CDFT is not the only way to extend DFT to systems in a magnetic field. The magnetic DFT (BDFT) formulation of Grayce and Harris requires functionals of the form E[v;B] and F[ρ;B], which simplifies numerical implementation and avoids an explicit functional dependence on jp, at the cost of losing some degree of universality.647,648 Could the simplifications outweigh the loss of universality in practical implementations? Another alternative would be to consider the coupling of internal magnetic fields with the external field via a Maxwell–Kohn–Sham approach, which does feature a Hohenberg–Kohn result for the total current density.649 Such an extension may be important in strong-field time-dependent light–matter interactions650 and leads to a more appealing functional dependence on the total current density, rather than only on its paramagnetic component. Finally, we mention linear-vector-potential DFT (LDFT), a simplified formulation of CDFT suitable for uniform magnetic fields.651
Given recent strides in better understanding the theoretical foundations of CDFT, and the construction of several practical implementations, addressing these challenges should lead to further progress and improvements in the accuracy of magnetic response properties with DFAs.
4.3.2 Görling.
As pointed out in contribution (4.3.1), the development of approximate exchange–correlation functionals in CDFT is not an easy task and is still in its early stage. It is, however, possible to the treat the exchange contribution to these functionals exactly by generalizing the OEP method to spin-current DFT.652 By this generalization, exact exchange vector potentials coupling to density currents and exact exchange magnetic fields coupling to noncollinear spin components arise (in addition to the usual exchange potential coupling to the electron density) and can actually be calculated.
4.3.3 Tellgren.
I agree with contribution (4.3.1) and want to elaborate that the development of practical CDFT functionals has only reached a crude stage of development compared with conventional DFT functionals. Pure CDFT functionals should depend only on the density and the paramagnetic vorticity ν = ∇ × ρ−1jp.628
At least for molecular systems, pure functionals are not yet practically useful. Instead, the more pragmatic meta-GGA functionals are presently much better at capturing the response to magnetic fields. However, recent work has shown that the kinetic energy density employed in these meta-GGA functionals only builds in the correct gauge correction, but not any vorticity dependence.
One way forward is to employ a local tensor, akin to a stress-energy tensor, that encodes both the vorticity and a gauge-invariant kinetic energy in a natural way;653 see also the current dependent meta-GGA form in ref. 654. This tensor furthermore obeys strong N-representability conditions that enable discrimination of regions with one, two, three, and four-or-more Kohn–Sham orbitals. The isoorbital indicators that underlie many standard approximations discriminate rigorously only between one and two-or-more orbitals.
4.3.4 Vignale.
Following up on contributions (4.3.1) and (4.3.2), I would say that all generalizations of the original DFT of Hohenberg, Kohn, and Sham require a firmer mathematical basis. The absence of a strict Hohenberg–Kohn theorem is a problem not only for CDFT but also for spin-DFT, and, probably, also for DFT of superconductors.
Fortunately, there are many indications that the “nonuniqueness” of the potential is harmless for Kohn–Sham applications because the wave function remains unique even when the potential is not. However, I do feel that all multivariable DFTs have another hidden problem – namely, to what extent can the intensive variables of the theory be varied arbitrarily and independently of each other? This problem may be particularly severe when there are global constraints enforced by symmetry – for example, the total angular momentum of a rotationally symmetric system. Or there may be inequalities whereby the maximum value of a density (say, the spin density) can never exceed some maximum value that is controlled by another density (say, the particle density). Such constraints affect the intensive variables but not their conjugate fields, which can always be varied independently. This is one of the main reasons (if not the only one) why the map from densities to potentials is generally not invertible.
4.4 What extensions are needed to get ground- and excited-state properties and observables in DFT?
4.4.1 Vignale and Ullrich.
Interest in noncollinear spin magnetism in systems with strong spin–orbit coupling has greatly increased since the emergence of spintronics and the discovery of topological materials. The SU(2) formulation of spin DFT for a noncollinear spin density seems to be nearing a “phase transition” with the appearance of new DFAs. There exist several interesting ideas for the construction of noncollinear spin functionals using gauge-invariant blocks655 and orbital functionals:656 they should be pursued.
4.4.2 Gidopoulos.
The expectation value of any observable quantity is a functional of the ground-state density. As far as I am aware, there is little progress in developing exact or approximate functionals for general observables, except for the total energy and the density itself. The definition of the density functional of any observable is known – for example, if
is the operator for the observable Q, then the density functional Q[ρ] is given by | 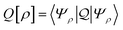 | (70) |
where Ψρ is the minimizing state in the definition of the universal internal energy density functional |  | (71) |
Since we do not have a good approximation to Ψρ, the Kohn–Sham state Φs[ρ] is sometimes employed |  | (72) |
but this approximation is often not accurate enough.
Since the definition of the density functional of a general observable is known, the development of an approximate or exact density functional Q[ρ] means trying to find an exact or approximate expression that does not depend on the unknown interacting state Ψρ, but which depends only on the density and also on quantities that can be obtained from a Kohn–Sham calculation (e.g., the Kohn–Sham state, orbitals, and eigenvalues) and which is more accurate than the obvious approximation in eqn (72). Recently, we managed to write down such a density functional for the magnetization density in DFT (not spin DFT) for open-shell systems in the absence of an external magnetic field.657
4.4.3 Gould.
Much recent work on ensemble DFT (see Section 3.7) and DFAs is focused on excited states.421,422 Ensemble DFT has the useful feature that differentiation with respect to ensemble weights gives access to Kohn–Sham wave functions (which can be multireference,427) densities, and energies of excited states.430 It has already been shown that ensemble DFAs can outperform their DFT or TDDFT counterparts in some difficult cases.442,658 Moreover, ensemble DFT can do so without breaking any symmetries424,443 and thus preserves spectroscopic features such as degeneracies.
4.4.4 Maitra and Ullrich.
Ensemble DFT is an elegant way to obtain excitation energies; however, we do not know how to obtain oscillator strengths from it. A more versatile approach to the calculation of spectroscopic properties such as optical spectra, excited-state forces, excited-state dipole moments and transition-dipole moments is via TDDFT.97,659–661 More than that, TDDFT gives access to a wealth of properties and observables in the nonlinear and real-time regimes, including high-harmonic generation or transient absorption, and, in general, electron dynamics on the attosecond time-scale. TDDFT can also be coupled with ionic dynamics, allowing practical calculations of the photochemistry of complex systems in mixed quantum–classical approaches. Needless to say, all these applications, while based on a theory that is in principle exact, involve approximations.
TDDFT has been overwhelmingly successful for a wide range of excited-state properties, but there are also spectacular failures. In the linear response regime, for example, it is now well understood that caution should exercised when standard DFAs are used to study states of double-excitation or charge-transfer character, and that these standard DFAs fail to yield excitonic spectra of semiconductors. However, such failures provide us with an opportunity to learn and improve our DFAs – much work remains to be done, but progress has been steady. It is also worth noting that the effort is well spent, given that TDDFT computations have a far smaller carbon footprint than alternative methods.
4.4.5 Romaniello.
Jacob and collaborators have recently proposed a scheme to extract the many-body spectral function of an interacting many-electron system from an equilibrium DFT calculation.662,663 This has been achieved by using an extension of DFT, called steady-state DFT (i-DFT).664
4.4.6 Jensen.
Excitation energies and transition properties may also be calculated using the long-range MCSCF – short-range DFT (MC–srDFT) method, which offers improved accuracy compared to TDDFT for ground states characterized by significant long-range nondynamical correlation and excited states with double-excitation character.500,501 The possibility of performing a state-specific optimization of an excited state with the MC–srDFT method offers another direct path to modelling excited states of any spin symmetry and any spatial symmetry; see contribution (4.1.3). Improvements to currently available short-range DFAs are needed to model the spin densities accurately, most likely by using the on-top pair density.
4.4.7 Romaniello.
An elegant but not often used (I do not know why) method for calculating the linear response of finite and extended systems is TD-current-DFT (TDCDFT).665–667 In TDCDFT, the basic quantity is the total current–density of the system, rather than the density as in TDDFT, that has a one-to-one mapping with an external vector potential. There are three main reasons for using TDCDFT:
1. for extended systems, it allows a well-defined expression for the macroscopic polarization of the system in terms of the induced current–density in the bulk;668,669
2. it allows one to treat the response to transverse fields;670,671
3. instead of looking for frequency-dependent approximations to the exchange–correlation kernel that are nonlocal functionals of the density (as done in TDDFT), one can look for consistent frequency-dependent approximations that are local functionals of the current–density, such as the Vignale–Kohn (VK) functional.672
Recently, Berger proposed a functional in the context of TDCDFT that can describe excitons in 3D materials.673 Linear response of 2D materials by contrast remains a challenge in TD(C)DFT.674
4.4.8 Savin.
As recalled in contribution (2.2.24), the universal functional, F[ρ] does not depend only on the density, but also on the Hamiltonian used. Bauer added operators to the Hamiltonian and, by exploiting the Hellmann–Feynman theorem, showed that properties can be obtained in this manner (even if not only the density is needed to get the expectation value of the operator).675 Bauer's approach requires density functionals that are specific for each property and new, property-specific DFAs must therefore be generated – see, for example, ref. 676.
This approach of DFT to molecular properties is not only interesting from a fundamental point of view; it can also show how far the present ideas for generating approximations can be taken – or help us understand why our current ideas work for the energy but not for a given property.
4.4.9 De Proft and Geerlings.
In conceptual DFT, the extension to excited states should certainly also be considered for use in, for example, photochemical reactions; see also Section 4.8. Its status and prospects have recently been commented on in ref. 475.
4.5 How can DFT further benefit from rigorous developments?
4.5.1 Chattaraj.
As systematic improvement is not possible in DFT (unlike in ab initio wave-function theory), research on fundamental aspects should continue. To achieve the goal of chemical accuracy in DFT, different approaches should be pursued side-by-side/in parallel, including the development of improved parameter-free functionals.
4.5.2 Lewin.
Rigorous mathematical results have played an important role in DFT. The most celebrated work is that of Elliott Lieb from 1983, who introduced the correct functional analysis setting for the ground-state problem.8 Several exact constraints have also been found, which could then be used in the construction of nonempirical DFAs, as mentioned by Perdew in contribution (3.1.2). This includes, for instance, the Lieb–Oxford bound,677 which provides an exact lower bound on the smallest possible Coulomb energy of N electrons, expressed only in terms of their density ρ. A recent review of known rigorous results for DFT can be found in ref. 33.
Several mathematical problems are still open and it would be nice to discuss here which of those could have an impact in DFT. I will only mention three problems which, in my opinion, deserve attention in the future.
The first is to better understand the Kohn–Sham potential. We have no rigorous proof that vs, which appears in eqn (8) and (32), for example, exists and, to my knowledge, no efficient numerical tool to construct an approximate one exists. Let me try to be a bit more precise.
Recall that a density ρ is (ensemble) v-representable when it arises from an N-electron (mixed) ground state with an external potential v. Let me emphasize that there are two notions of v-representability, for the interacting and noninteracting cases, respectively. The question is whether a v-representable density (for the interacting system) is v-representable by the noninteracting system. In other words, we need to study the set of densities that are simultaneously v-representable for the two cases. At the moment, nothing is known rigorously about this set, to my knowledge – in principle, it could even be empty! Of course, to properly discuss this problem, it is important to first fix a class of admissible potentials v that we wish to consider in DFT. This class should be large in order to increase the probability of being v-representable, but probably not too crazy either. Lieb considered all potentials belonging to L3/2(
3) + L∞(
3) because the energy is always bounded-below for such potentials.8 However, many physical cases do not appear in this class, such as the harmonic potential for instance. This is definitely a too small class.
One can understand v-representability in several other equivalent ways, all described by Lieb.8 My preference goes towards the Legendre–Frenchel point of view, which requires the use of mixed states and ensemble v-representability and was already discussed above in contributions (2.1.13) and (3.7.2). We know that the corresponding lowest total energy and the lowest kinetic energy for a given density ρ satisfy the duality principles8
|  | (73) |
|  | (74) |
where
EN[
v] and
E0N[
v] are the interacting and noninteracting ground-state energies in an external potential
v, respectively. Again, one should specify the set of potentials
v in the two suprema, but any reasonable class will yield the same final value. The question is whether these suprema are attained (our desired potential
vs is a maximizer for
Ts[
ρ]) and then the chances that this happens are much higher if the allowed class of potentials
v is larger. Let me recall in passing that the existence of a dual potential is well understood in classical DFT. At zero temperature this follows from methods in multimarginal optimal transportation.
678 At positive temperature, this result was proved by Chayes, Chayes, and Lieb.
679 The quantum kinetic energy is thus the main obstacle here. Discretized quantum systems are studied in ref.
34,
680 and
681.
Another point of view has been mentioned above in contributions (2.2.2) and (3.7.2) and involves a kind of differentiability of F[ρ] and Ts[ρ]. Any potential solving a maximum principle such as in eqn (74) is, formally at least, a derivative of the corresponding functional.8 To be able to treat the difference F[ρ] − Ts[ρ], we thus need both to be differentiable at the same time. Although the notion of differentiability looks natural and intuitive, it is in fact not so easy. The reason is that the natural domain of F[ρ] is the set of densities with a finite von Weizäcker energy,8 which is not such a nice set.
Lieb proved that the two sets of v-representable densities are dense in the space L1(
3) ∩ L3(
3).8 The problem is that a dense set can, in principle, be extremely small – think of the rational numbers, which are dense but form a set of zero measure in the set of real numbers. Even worse, we need to look at the intersection of these two dense sets, which can be arbitrarily small or even empty. Very little is thus known mathematically about this problem.681
Let me now quickly mention the other two problems I had in mind. The second one is to improve existing exact constraints. For instance, I already mentioned the Lieb–Oxford bound,677 of which the best constant is believed to be that of the uniform electron gas.177,682,683 But at present, we have no idea on how to justify this rigorously.
Finally, I would like to mention that, unlike ground-state DFT, TDDFT is very poorly understood mathematically.684
4.5.3 Kvaal.
Moreau–Yosida regularized Kohn–Sham theory does not suffer from the nondifferentiability of F[ρ] − Ts[ρ] and the problem of nonrepresentability therefore does not arise; see remarks in contribution (4.5.6). Also, Lammert has made an interesting attempt to coarse-grain exact DFT, where this issue is to a large extent resolved.34
4.5.4 Laestadius.
An important work addressing differentiability of the density functional F[ρ] is Lammert's work in ref. 685. Lammert provides a counterexample of a convex and lower semicontinuous function with a (unique) subdifferential that is not differentiable. Thus, this illustrates that convexity and lower semicontinuity are not enough to establish “F′ = −v”, even for variations that stay within the domain of the density functional F[ρ] – that is, Lieb's set of N-representable densities
N.
4.5.5 Helgaker.
With regard to contribution (4.5.4), subdifferentiability of F on a dense subset of the N-representable densities
N (namely, on the set of ensemble v-representable densities
N) follows from the convexity and lower semicontinuity of the universal density functional F. It is a general result of convex analysis that a proper lower semicontinuous convex function (here F) is subdifferentiable on a dense subset (here
N) of its effective domain (here
N) and everywhere in the interior of its effective domain (here the empty set since
N has no interior).
4.5.6 Helgaker, Teale, Laestadius, and Kvaal.
As pointed out in contribution (4.5.2), a central problem of DFT is the representability problem of Kohn–Sham theory. In short, what one would like to have is a simultaneous solution to the interacting and noninteracting Euler–Lagrange equations: | 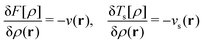 | (75) |
where ρ is simultaneously the ground-state density of the interacting system in the external potential v and the ground-state density of the noninteracting system in the external potential vs. The problem is that both F and Ts are everywhere discontinuous and therefore not differentiable685 – more precisely, they are subdifferentiable but only on a (dense) subset of their domains8 and there is no reason to believe that these subsets are the same for the interacting and noninteracting problems. In short, we cannot hope to find a Kohn–Sham noninteracting system with exactly the same ground-state density as the interacting system.
However, imagine that we change the ground-state energy in the manner
where
γ > 0 can be arbitrarily small.
686 The density functional then becomes
and likewise for (
Ts)
γ. Importantly,
Fγ and (
Ts)
γ are both everywhere differentiable, meaning that the interacting and noninteracting Euler–Lagrange equations can now be solved simultaneously.
99,686 In convex analysis, such a procedure is known as Moreau–Yosida regularization.
687 Once the regularized energy
Eγ[
v] has been calculated, it is trivial to obtain
E[
v] and nothing is lost – that is, the Moreau–Yosida regularization of
F has the curious property of being lossless with respect to the calculation of the ground-state energy
E[
v]. The only caveat is that ‖
v‖ must be finite, which is only satisfied for Coulomb potentials by constraining the system to an arbitrarily large box.
In the regularized setting, every density is both interacting and noninteracting representable – a rigorous exact Kohn–Sham theory is thereby established. However, such a density need not be a “physical” density.
The Moreau–Yosida regularization of DFT may also be of practical interest as a tool for guaranteeing and improving convergence of the Kohn–Sham iterations.99,688
4.5.7 Laestadius.
Representability of a given density ρ can also be understood as the Lieb functional
(see contribution (2.1.13)) attaining its maximum, such that ρ is representable by its maximizing potential. In analogy with the above discussion of the Moreau-Yosida procedure, a maximizing potential can here be guaranteed by a regularization of
using fixed weight functions αi ∈ L∞. Such a scheme only makes use of partial information of the density constraint, i.e.,
, for all i (for more details see ref. 689).
4.5.8 Gori-Giorgi.
The ℏ → 0 limit of the Levy–Lieb functional (see contribution (2.4.5)) establishes a link690,691 between DFT and the mathematical field of optimal transport; see, for example, ref. 678. When ℏ → 0, the Levy–Lieb functional tends to the SCE functional139,140 | 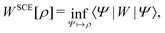 | (76) |
which defines an optimal-transport multimarginal problem with the repulsive Coulomb interaction as cost function.690–692 Techniques from optimal transport made it possible, for example, to prove693 that the exact SCE functional for one-dimensional systems is provided by the solution first guessed by Seidl141 on physical grounds. Another application is on the Lieb–Oxford bound (see contribution (4.5.2)), where optimal-transport methods can be used to improve bounds on the optimal constant.694,695
Some open questions that remain on the rigorous side concern the next leading term in the ℏ → 0 expansion, whose form was conjectured in ref. 144. A first step in this direction has been recently taken.696 I do have the feeling that there is still a lot to learn from the connection with optimal transport and by further analysing this limit, which provides complementary information with respect to perturbation theory. Although it is a semiclassical limit, it may perhaps be corrected for exchange (spin) effects,697 and could be combined with Kohn–Sham DFT.408,698
4.5.9 Lewin.
I fully agree with contribution (4.5.8). Related to the above discussion in contribution (4.5.2), one interesting question could be to understand what can be said about the Kohn–Sham potential vs in the limit ℏ → 0. As I have mentioned, all densities are v-representable in the SCE limit eqn (76). So, more v-representable densities seem to exist when ℏ gets sufficiently small.
4.5.10 Gori-Giorgi.
A first (brute-force) attempt to get insight into this intriguing question has been made by Grossi et al.,699 who explicitly computed the functional derivative of the next leading term144 in the ℏ → 0 expansion, in the special one-dimensional case (for which we now know696 that the functional proposed in ref. 144 is exact). However, Kohn–Sham self-consistent calculations that include the functional derivative of this next leading term700 make the density poorer relative to the bare Kohn–Sham SCE result – even for very low-density systems, where inclusion of this term improves the energy. This suggests that this is not the right route to take to answer the question on vs, or at least that we need to better understand the expansion.
4.5.11 Arbuznikov and Kaupp.
Regarding the discussion in contribution (4.5.2), we would like to make a point about the difference between global and local exact constraints. Most of the extremely important and useful known and proven exact constraints pertain to global (integral) energy functionals. Yet most DFAs are designed with local (exchange and/or correlation) energy densities in mind. Apart from the issue of the nonuniqueness of any energy density (only defined up to a real-space function whose integral vanishes), constructions often apply known “global” constraints locally, even though the local versions of the constraints are usually ill-defined or unknown.
A point in case is the Lieb–Oxford bound.677,695 Becke argued that any reasonable exchange functional globally satisfies the Lieb–Oxford bound for any real chemical or physical system, irrespective of whether the underlying energy density obeys or violates it locally.701 In other words, a local Lieb–Oxford bound seems to be a sufficient but not a necessary constraint in the design of functionals – the exact-exchange energy density, in particular, violates the local Lieb–Oxford bound in the tail of any finite system. A local enforcement of the Lieb–Oxford bound gives enhancement factors of some widely used semilocal exchange functionals (e.g., PBE234 and SCAN235 functionals) that are somewhat too low to describe finite systems adequately (in combination with an appropriate correlation functional), thus hampering thermochemical accuracy.
4.5.12 Vignale.
Concerning the mathematical foundation of TDDFT, it seems to me that much progress has been made recently by Ruggenthaler and coworkers in establishing the existence and uniqueness of the density-potential map; see ref. 98 for a review. This goes significantly beyond the original Runge–Gross theorem. There are more radical forms of TDDFT (e.g., time-dependent DFT for the calculation of thermal currents, reviewed in ref. 702) that still lack a rigorous mathematical foundation.
4.5.13 Ullrich.
To follow up on contribution (4.5.12): the requirements for proving a rigorous mathematical structure of TDDFT are vastly different from those of ground-state DFT. In recent years, a consensus seems to have developed that the most promising avenue is to find a fixed-point proof,98via the force-balance equation. The latter is an equation of motion for the density, involving its second time derivative
, external forces, and internal kinetic and many-body stresses. The fixed-point technique is mathematically very difficult and the TDDFT proofs based on it are still not fully rigorous.98
It has recently been shown that TDDFT can be reformulated using
instead of ρ(r, t) as the basic variable, which has the advantage that the causal structure of the theory becomes more transparent.703 This result may provide a new way forward in the ongoing attempts to solidify the foundations of TDDFT.
4.6 How can DFT further benefit from numerical analysis and algorithmic developments?
4.6.1 Johnson.
In finite-molecule calculations, use of hybrid functionals is routine. However, for plane-wave DFT calculations on periodic solids, use of hybrid functionals remains prohibitively expensive for most systems. A more efficient algorithm for evaluation of the exact exchange energy in plane-wave codes would benefit the application of hybrid DFAs to solid-state systems. Algorithms for efficient evaluation of the exact exchange-energy density in both finite-molecule and periodic-boundary codes would also aid the implementation of local hybrid functionals319 and of Becke's real-space correlation functionals.13
4.6.2 Kronik.
The overwhelming majority of DFT-based calculations are performed using either atom-centred basis sets or plane waves. While calculations based on a real-space grids have been available for a long time,704,705 their importance can be expected to increase – first and foremost, since this approach lends itself easily to massive parallelization across a large number of processors.
4.6.3 Cancès.
I agree. This also applies to finite-element706 and wavelet707 discretization methods.
4.6.4 Cancès.
Efficient SCF algorithms are available for a variety of systems of practical interest.708,709 However, SCF convergence remains problematic in some cases – for instance, for large, heterogeneous, systems such as metal-insulator interfaces. Progress has been made recently,710 based on a better understanding of the mathematical properties of the Kohn–Sham model. Some particularly difficult systems are still resisting, motivating further work in this direction.
4.6.5 Cancès.
Another numerical issue encountered in materials science, as well as in chemistry in the liquid phase (with explicit solvent molecules), is the choice of suitable supercells. The smaller the supercell, the lower the computational cost. On the other hand, the supercell must be large enough to limit spurious interactions from the artificial periodic boundary conditions. Finite-size corrections for point defects in periodic crystals have been proposed in the physics literature711 and analysed mathematically.712 Selecting optimal supercells and associated random configurations for disordered systems (alloys, glassy materials, and liquids) is a notoriously difficult problem.
Let us emphasize that the apparently simple case of a genuine, periodic crystal can be challenging also when the crystal is a metal. Recall that, for periodic crystals, using a supercell is mathematically equivalent to sampling the Brillouin zone with the regular k-point grid;713 the advantage of the latter approach is that it is far more efficient from a computational viewpoint. In most calculations, a relatively coarse k-point grid is used to further reduce the computational burden (say, 3 × 3 × 3 for insulators and 7 × 7 × 7 for metals). This approach is usually sufficient for insulators because the integrands are periodic, analytic, and weakly oscillating over the Brillouin zone for all relevant physical observables, but far from sufficient714 for metals with complicated Fermi surfaces. Smearing techniques715 at a fictitious positive temperature (possibly higher than the melting temperature of the metal) help to some extent,716 but do not fully solve the problem. It appears that many computational results on metals reported in the literature cannot be considered as converged with respect to k-point discretization.
4.6.7 Cancès.
What is the error in the output of DFT codes relative to the exact value of a chemical or physical quantity of interest (e.g., the dissociation energy of a molecule, the bulk modulus of a material)? This question is obviously of major importance and is usually addressed by comparing experimental and computational results on large databases. However, such statistical analyses do not really answer legitimate questions of most users, which can be formulated as follows: “What will be the error for the specific system I am interested in if I use this code, with these numerical parameters (basis set/energy cutoff, convergence thresholds, etc.)? How should these parameters be chosen to obtain the accuracy I need, at the lowest computational cost?”
Providing partial answers to these questions is the purpose of a field of applied mathematics called a posteriori error analysis. This field has reached its maturity in, for example, finite-element based computational mechanics, where most academic and commercial codes provide numerical results (e.g., the lift and drag of an aircraft) complemented by error bars. To understand what can or cannot be done in this direction for DFT, it is useful to decompose the overall error in several pieces:
1. the model error, coming from replacing the reference very accurate model (the N-body Schrödinger model or one of its relativistic counterparts) by a DFT approximation (LDA, PBE, B3LYP, etc.), possibly with pseudopotentials;
2. the discretization error due to the use of a finite basis set;
3. the algorithmic error due to finite convergence thresholds;
4. the finite-arithmetic error (computations are usually done in double precision);
5. execution error (negligible for current computers but an issue for future exascale719,720 and quantum computers).
It is already possible to estimate the discretization, algorithmic, and finite-arithmetic errors for linear Schrödinger equations discretized in plane-wave basis sets.721,722 The more recently developed error estimators are
1. guaranteed: mathematical theorems prove that the exact value indeed lies in the confidence interval;
2. accurate: the actual error is of the same order of magnitude as the error bar;
3. cheap to compute: evaluating the error bars requires only a moderate computational extra cost; and
4. systematically improvable: provide detailed information on how to increase the accuracy at the lowest cost.
Extending these techniques to the nonlinear Schrödinger and Kohn–Sham equations is work in progress.723 Such estimators would allow the computer program to choose adaptively, in a black-box manner, the best numerical parameters to reach a given numerical accuracy at the lowest computational cost (error balancing). Error balancing would be particularly useful for building large databases for ML, requiring hundreds of millions of single-point DFT calculations.724
Let us finally discuss the model error. For wave-function methods, it is in principle possible to estimate this component of the error by a careful mathematical analysis of the residual HNΨappN − ENΨappN, where HN is the N-electron Hamiltonian, and ΨappN and ENapp are the computed approximations to the ground-state wave function and energy; this is a topic of ongoing research. In the DFT setting, a promising approach is the use of (non-guaranteed) estimates based on a statistical analysis of the model error – see, for example, ref. 341–343 and 346. Whether nonstatistical, guaranteed, accurate, and cheap-to-compute model error estimators can be constructed using DFT is a completely open question.
4.7 What role will machine learning play in the future of DFAs and DFT?
4.7.1 Scheffler.
Artificial intelligence (AI) accepts that there are relationships or correlations that cannot be expressed in terms of a closed mathematical form. Thus, in principle, AI is more flexible than the theory of the past. The algorithm outlined in (2.1.4) “ρ(r)
ground-state energy (and even everything)” may be a case where an AI model can capture the relationship better than a mathematical functional. However, at present, the flexibility of AI comes together with a lack of interpretability, and the missing knowledge of the domain of applicability is probably an even more severe problem for AI models than it is for DFAs. AI can only predict aspects that were included in the training. If this is considered sufficient, then AI is a numerically efficient approach, once the laborious training has been performed with appropriate care.
4.7.2 Salahub.
Recent progress in AI/ML has been so astounding that even the “old guard” need to be aware and beware. As far as DFT is concerned, ML can have a great impact from (at least) three perspectives: (1) improving DFAs, (2) accelerating DFT calculations, evaluation of potential-energy surfaces and (Born–Oppenheimer) MD or, catastrophically for DFT, (3) obviating the need for DFT if ML-accelerated wave-function calculations become “infinitely” efficient (of course the advantages of a (Kohn–Sham) orbital picture would remain). I think the next few years will be very dynamic on all of these fronts, and others. And we, DFT aficionados, should also be paying attention to advances in quantum computers.
4.7.3 De Proft and Geerlings.
ML may be a valuable tool to inspire researchers in conceptual DFT to look for possible relationships between reactivity and conceptual-DFT descriptors that are not obvious when a limited number of cases are explored “by hand”. Care should of course be taken that, in the end, the researcher comes to a point where the link proposed by the ML ansatz provides him/her with a reasonable insight into the problem.
4.7.4 Grimme.
The primary purpose of Kohn–Sham DFT for AI/ML approaches seems to be the efficient generation of the required huge amount of reasonably accurate reference data (mostly energy and forces).
4.7.5 Chermette, Adamo, and Ciofini.
AI will be also involved in the building of exchange–correlation functionals – for example, by following Perdew's approach of satisfying 17 theoretical constraints; see contribution (3.1.2). A paper in this vein by Kirkpatrick and Cohen appeared recently.725
4.7.6 Galli.
I would like to mention DFT-based deep-potential MD (DeePMD) by Robert Car and Weinan E's group in Princeton717 and machine learning dielectric screening for the simulation of excited state properties of molecules and materials,726 which may eventually be used also to derive dielectric-dependent hybrid DFAs.
4.7.7 Trickey.
It is important to scrutinize ML results to see if they actually match the DFT on which they are supposed to be founded. We recently found an example in which such a match does not occur – namely, the liquid–liquid phase transition of hydrogen727 (in which H2 dissociates to atomic H). A machine-learned potential (MLP) had been developed by Cheng et al.,728 primarily by training on small (mostly 108-atom) and some intermediate-sized (512 atom) AIMD-DFT calculations. The resulting MLP-AIMD simulations for systems of 1728 atoms has a qualitatively different continuous transition from the first-order transition found by all the prior AIMD-DFT simulations (which were on smaller systems). Supposedly, the MLP-AIMD allowed bigger systems, hence overcame finite-size effects in the earlier AIMD-DFT studies. So we redid the brute-force AIMD-DFT calculations not only for 512 atoms but also 1024 and 2048 atoms. Our results were consistent with the earlier AIMD-DFT ones, a first-order transition. They do not confirm the MLP-AIMD results. Something artefactual remains in the MLP such that it is not a faithful extrapolation of the AIMD-DFT potential. I suspect that there is much yet to learn about the limitations of ML regarding extrapolation toward the thermodynamic limit, particularly in the treatment of phase transitions. Scrutiny, if not outright scepticism, is warranted.
4.8 How should interpretive tools based on DFT evolve?
4.8.1 Ayers, Chattaraj, Chermette, De Proft, Fuentealba, Geerlings, Liu, Vela, and Yang.
In the case of conceptual DFT, the prospects and concomitant issues were summarized in the status paper475 mentioned in Section 3.9. Regarding first the interpretive aspect, some issues should clearly be communicated by the experts to the practising chemist using conceptual DFT. For example:
1. Is the use of a temperature-dependent version of conceptual DFT729 necessary to avoid the E(N) differentiability problem when considering temperatures typical for laboratory conditions in synthetic work (leaving high-temperature chemistry aside)?
2. Can the pros and cons of going from the canonical ensemble, with the associated E[N, v] functional, to the grand canonical ensemble, with a state function Ω[μ, v] that allows fluctuations in the number of electrons,730 be clarified for the practising chemist? See also contribution (2.4.1).
3. Can one expect that the perturbation series of E[N, v] of a given reactant upon interaction with a perturbing reaction partner (the second reactant) converges properly in most cases?
4. Can the proper use of the principles mentioned in contribution (3.9.1) be supported by delineating their domain of applicability – that is, by formulating the conditions under which a meaningful application of the different principles is possible?
5. Can potential pitfalls in using conceptual DFT when going from kinetic (reactivity) to thermodynamic (stability) reasoning be identified?
On the other hand, the theory is in need of extensions – for example:
1. the inclusion of new variables in the E[N, v] functional such as electric and magnetic fields, mechanical forces, pressure to cope with the increasing variety of reaction conditions encountered in present-day chemistry;731,732
2. a proper and possibly elegant inclusion of spin733 to extend the theory to transition metals, of crucial importance in catalysis, and to radical reactions;
3. a generally applicable extension to excited-state reactivity482,734,735 to deepen the insight into photochemical phenomena and, in the same vein, an extension to time-dependent conceptual DFT;736
4. a deeper connection between conceptual DFT and information theory and its density functionals193,737 and with the reaction-force ansatz when considering reaction mechanisms.738
Recognizing that the ultimate goal of conceptual DFT is prediction, it was realized that the one reactant approach on the basis of the above mentioned E[N, v] expansion should be scrutinized to identify what terms in the expansion of E[N, v] are most likely to be efficient for a given problem, thereby challenging chemical intuition. Characteristics of the second reactant most probably should be explicitly introduced at various levels of refinement for quantifying the ΔN and Δv perturbations.475
4.8.2 Gao.
In contribution (3.7.11), I described MSDFT as a hybrid wave-function and DFT method in the context of ensemble DFT. The basis states used to generate the energies of the adiabatic states in the ensemble can be viewed as effective valence-bond configurations. These configurations, obtained either through fragmental block-localization or by local electronic excitations, correspond to well-defined Lewis resonance structures, whose variational optimization can be directly used for block-localized wave-function interaction energy-decomposition analysis (BLW-EDA) to provide a quantitative interpretation of DFT results, such as aromaticity, hyperconjugation, and the Dewar–Chatt–Duncanson σ-dative donation and π-backbonding in transition-metal complexes.739 Furthermore, these localized electronic structures can be used to define diabatic states by orthogonal projection,740 suitable for dynamics simulations of nonadiabatic processes, including electron transfer, excited-state energy transfer, and photochemical reactions.
Recently, a general approach was introduced for treating spin-coupling interactions of open-shell molecules by MSDFT.458 The TDF energies that determine spin coupling are obtained by enforcing the multiplet degeneracy of the S + 1 state in the MS = S manifold. Spin-adapted configuration states were used as the active space in MSDFT calculations of core excitations of open-shell molecules.
4.8.3 Ayers.
While existing density-based tools can provide deep insight into chemical bonding, molecular electronic structure, and even the thermodynamic driving forces for chemical processes,741 there are still outstanding issues. I am not entirely convinced that there is any fully satisfactory definition of bond order, atomic partial charge, etc. I am not even convinced that the canonical Parr–Pearson definition of chemical hardness is the best one.742 Perhaps some of these concepts must be discarded. However, the power of DFT is that, unlike most (but not all) other approaches, it is mathematically rigorous and grounded on physical observables. This allows one to attempt to approach chemical concepts axiomatically: first one lists the key properties/tests one expects a concept to possess/satisfy, then one tries to find a mathematical definition.743
5 Communicating and sharing our results
5.1 How should the DFT community organize and share information?
5.1.1 Loos.
No one should have to code the B3LYP functional again: the democratization of open-source software and libraries such as libxc,744 xcfun,745 and numgrid,746 are a big step in this direction. The availability of the source code should be strongly encouraged, especially for research funded by public money.
5.1.2 Krylov.
I would like to distinguish between open sharing of ideas and basic tools useful for prototyping versus open-sourcing production-level codes. Yes, we should openly exchange ideas and share basic tools of development, or some libraries. But, as described in this Viewpoint article,747 sustainability of code development cannot be ensured by present funding models and license income then provides a way to sustain scientific developments and software maintenance. We should remember that our ultimate goal is to provide chemists with software that is robust, effective, and usable. The commercial software-development model provides a vehicle for achieving this goal.
5.1.3 Trickey.
Most of the issues associated with this section seem to be generic to the practice of scientific research and not specific to DFT. Those issues include the sociology of science, national funding policies (note Krylov's contribution (5.1.2)), priorities, and mandates (e.g., data management plans in the USA), institutional practices and policies (e.g., tenure and promotion in the USA compared to say China or Germany or México). Given that enormously variegated setting and given the sprawling utilization of many-fermion DFT in myriad diverse specialities and technologies, one might ask whether there are DFT-specific aspects of dissemination, communication, and/or data management, for which the DFT development community has explicit responsibilities and/or opportunities.
One such DFT-specific aspect has been pointed out by Loos in his contribution (5.1.1). But as usual there is a hitch. First, just because a DFA (or KEDF) is implemented for some kind of Gaussians, this doesn’t mean that it will work for a plane-wave PAW code. (It may not even work for another kind of Gaussians without some fiddling.) Secondly, there are mis-implementations. (The B3LYP DFA itself is a kind of mis-implementation and there are the notorious VWN versions.) Postdocs in my group have found several mis-implementations in popular codes and one of our finite-temperature DFAs was mis-implemented in libxc. Especially for intricate DFAs (and KEDFs), it seems healthier to have several independent implementations.
Another step would be for presentation of a new DFA to include an explicit, unambiguous statement near the outset of whether it was intended for only a certain class of molecules or only for molecules but not condensed phases, etc. and on what class of systems it had been tested.
5.1.4 Draxl.
I agree, for example, with contribution (5.1.1) that we should share as much as possible to avoid that many people are doing the same again and again. We should use our human resources for going beyond what is done already. This also implies, however, that many of us contribute to open-source libraries; testing and feedback is another issue. It is also good to have alternative implementations for comparison. Very important, we as a community also need to appreciate much more the work that some people are putting into developing codes and tools. It often happens that such work is considered “nonscientific” or “programming jobs only”. Also, when papers on implementations are submitted, referees reject these because of lack of novelty. People dedicating months and years to develop tools that are used by the community should not have a disadvantage when being considered (or not) for a job because they published less during this time.
5.1.5 Ayers.
I see no drawback to being aggressively open. Indeed, I believe we should aspire to share so aggressively that reproducing, and even extending, a study is not only possible but feasible. This requires more than FAIR sharing of content/data;748 it requires more than releasing open-source software; it requires a high standard of communication/documentation for theory, data, algorithms, and code. I concur with contribution (5.1.3) that some of these issues are very broad, and we can certainly learn from other researchers in the computational mathematical sciences.
5.1.6 Savin.
One aspect related to sharing information is improving the condensed information we share – for example, the way we summarize the results obtained from benchmarks. This means that we need good tools to analyse the existing and ever-increasing amount of data.
5.1.7 Draxl.
The need of benchmarks is also emphasized in contribution (5.2.3). A very first step was made by Lejaeghere et al. in a true community effort, known as the Delta test.749 From the beginning of the initiative to publication, it took several years during which codes and pseudopotentials were significantly improved. Still, this is only a very first step as this work only concerns total energies for elemental solids and a single semilocal exchange–correlation functional (PBE). We need comparative studies for very different properties (barriers, band gaps, spectra, etc.) and very different types of materials (organic, inorganic, surfaces, interfaces, hybrid materials, etc.), carried out on different levels of methodology. Even the Delta test data, though appearing extremely consistent across many codes with regard to the total energy, exhibits an unacceptable spread when it comes to properties, as revealed in a subsequent analysis.750
5.1.8 Crawford.
I fully agree that the broad dissemination of both established and emerging DFAs and related DFA technologies is to the benefit of the scientific community, and optimized libraries such as libxc,751 xcfun,745 and numgrid746 provide superb examples of the added value of such an approach. The impact of libxc, in particular, is noteworthy in that it provides more than 600 density functionals (LDA, GGA, and meta-GGA) to dozens of community quantum-chemistry and materials-science software packages, both open-source (e.g., PySCF,752 Psi4,753 Quantum ESPRESSO754) and commercial (e.g., ORCA,755 ADF,756 Molpro757). Furthermore, the library is applicable not only to Gaussian basis sets, but also to plane waves, adaptive grids, and finite-element representations.
Libraries and modules such as these not only provide high performance, but also improved reproducibility and standardization, both of which are becoming more vital as the complexity of our models advances. To that end, emerging standards and tools for sharing computational results will similarly grow in importance. In the materials-science domain, for example, this has long been underway with community-driven resources such as the Materials Project758 as a paradigm, although the standardization of the content of materials databases is still under development within that community. In the computational-chemistry domain, new tools such as the Quantum Chemistry Schema (QCSchema) and Quantum Chemistry Archive (QCArchive)759 (an open, public-facing database of computational results) developed by the Molecular Sciences Software Institute (MolSSI)760,761 would allow much greater interoperability between codes by facilitating standards for data sharing. In addition, the Simulation Environment for Atomistic and Molecular Modeling (SEAMM),762 also under development by the MolSSI, provides a lightweight, Python-based plug-in environment for complex, shareable workflows, which will permit sophisticated computations involving multiple community code components in a fully reproducible and publishable manner. The broader the adoption of standards and tools such as these, the faster our community will be equipped to handle more complex and important scientific challenges.
5.1.9 Reining.
Since data from models, such as the quantum Monte Carlo data for the homogeneous electron gas,241 have turned out to be so precious for DFT, we should think about the best way to share such data. Some of us think that models more complex than the homogeneous electron gas may contribute to better approximations,242,763 not only finite models with uniform electron density as Loos mentions in contribution (3.1.5), but truly inhomogeneous, still simple, systems. To tabulate and/or interpolate such model data will be hard work on its own, and sharing the results will be crucial.
5.2 How and what should we publish?
5.2.1 Loos.
Publishing negative results should be encouraged much more in our community because they may be as valuable as the positive ones and may provide important insights.
5.2.2 Loos.
Hopefully, the popularity of open-access repositories for electronic preprints and postprints (such as arXiv or ChemRxiv) will keep growing in our community so that researchers have rapid access to free, new science. I personally believe that the present model where researchers seek funding, supervise students/postdocs, write articles, and review them is a broken, unsustainable model.
5.2.3 Draxl.
I agree with both – we should change our publication culture, including also publication of negative results. But we also need more “positive results”. While in chemistry, verification/validation and benchmarking are a matter of course and have been for many years, or even decades, in computational physics this is still in a very early stage. All this is, however, crucial for assessing methodology and distinguishing between accuracy (of a method) and (numerical) precision.
5.2.4 Jones.
I have participated in countless discussions over many years concerning scientific publication, mostly in physics. Common conclusions have been that too much is published in too many journals, and improved refereeing is needed to reduce the number of publications whose quality is borderline or below. The world has gone in the opposite direction. Open access publication has some advantages, but it has contributed to the continuing proliferation of journals and can result in a lowering of standards (accepted papers bring income, rejected papers do not). The widespread use of electronic archives increases the number of articles that are not reviewed at all. I see little hope for change and have depended for years on private communications about new developments.
Identifying something as “broken” and/or “unsustainable” as in contribution (5.2.2) could be the first step towards repairing it, but I am not optimistic. I am reminded of the alleged response of a local in rural Ireland: “If I wanted to get to Dublin, I wouldn’t start here.” Ever-increasing pressure to obtain external funding will both hamper risk-taking and increase focus on “fashionable” topics.
5.2.5 Savin.
I agree with contribution (5.2.4). I feel drowned in the publication flood. Finally, the question is about transmitting information. Maybe we should try to establish ways to present essential findings that is incremental, and can be updated, in the style of Wikipedia. The numerical support could be put in a database that can be searched by automatic tools.
5.2.6 Trickey.
Again we are faced with generic challenges in physical science. Funding pressures are one. Competition among publishers to have the most exclusive journals is another. Emphasis is on the allegedly spectacular. These influences combine to make it hard to publish careful, incremental advances, let alone negative results. Within the DFT community, maybe we should urge editors to accept the publication (hence, also, respectful refereeing) of careful presentation of negative results about well-motivated, well-grounded attempts at advancement?
5.2.7 Gori-Giorgi.
We should also not forget that the pressure to publish goes hand in hand not only with the competition to obtain funding but also with how we evaluate (young) scientists. There is now (finally) an attempt to shift from criteria based on quantity (like the number of publications, the h-index, etc.) to move towards quality.764 How to define the latter is of course a big challenge – although experts usually recognize quality in their field, any definition has exceptions. There is also more focus on collaboration and team science, which are positive developments.764 But without appropriate funding and reasonable career perspectives for young people, the situation regarding overpublishing and overselling results (writing artificial success stories) will probably remain dire.
5.2.8 Romaniello.
I agree with contribution (5.2.4). In particular, open-access publication seemed a nice idea at the start but, as with most human activities where money circulates, the system got corrupted. The publishing fees are now so high that it is much cheaper for the scientific community to keep the standard subscription model. Moreover, nowadays everybody can wake up in the morning and create their own journal, which makes it difficult for the institutions to keep track of “serious” journals. I like the idea proposed in contribution (5.2.5), which puts back in the spotlight the importance of sharing knowledge and not of increasing the h-index.
5.2.9 Galli.
I would like to alert the community on one of our efforts to make data available on a per-publication basis, which could be used also for DFT publications. Please see Qresp, a tool for curating, discovering and exploring reproducible scientific papers.765,766 For an example of a curated paper, see ref. 767.
5.2.10 Ayers.
For traditional electronic-structure calculations on molecules and materials, there are existing platforms like QC-Archive768 and the NOMAD repository769 for securely storing and sharing data. These databases provide good search capabilities, support most popular electronic-structure packages, use the well-defined JSON schema, which can be directly accessed/used (especially NOMAD), and at least partly fulfil the goals of making data findable, accessible, interoperable, and reusable (FAIR).
5.3 What format should workshops and conferences take in the future?
5.3.1 Maitra and Ullrich.
In the past 15 years or so, there have been a number of schools and workshops geared towards graduate students and postdocs – notably, the DFT/TDDFT tutorials at the March Meeting of the American Physical Society, the biyearly TDDFT series at the Benasque Center for Science since 2004, a similar series in the US since 2017, and the CECAM workshops on learning the theory of DFT. Despite the positive feedback these events have received, their impact is limited to those who can travel to their locations. The Zoom activities that arose out of necessity during the pandemic (e.g., the international PhD student seminar series on (TD)DFT theory development770) offer us the possibility to think about establishing hybrid schools and workshops routinely: not only to reduce our carbon footprint at the heights of the climate crisis, but also to enable students truly from all over the world to attend.
5.3.2 Romaniello.
We should not go back to the pre-pandemic model. In this last year, we have learned that we can easily follow a workshop/conference from our office/home. Of course, we also need real interactions, but we could select one or two events per year in which to participate in person and the rest online. Together with the advantages mentioned in contribution (5.3.1), let me add that the possibility of hybrid events will be beneficial also for female scientists just back from maternity leave, for whom it is usually complicated to leave home for several days.
5.3.3 Reining.
I would like to advance two more arguments in favour of using online tools in general: First, if we talk about family matters, this should concern not only women, but also, and equally, men who care for their family. Second, we are scientists and know about the climate – so, let us make an effort to travel less and shorter distances, preferably by train.
We should work out new formats that do not force us to choose between taking a plane and having coffee with colleagues, or just sitting in front of a screen. We could work out, for example, a delocalized physical conference, where smaller hubs are connected by internet and people can travel to the nearest hub. Such a format would necessitate new forms of discussion but, if we are not too conservative, we can certainly come up with solutions. Besides, online tools also allow us to make material available in advance, such that newcomers in the field can be better prepared and profit more.
5.4 How can we best teach and communicate DFT?
5.4.1 Helgaker.
I believe too much is made of the Hohenberg–Kohn theorem – expressing the ground-state energy as a function of the density alone in the manner E[ρ] is unhelpful and obfuscates the theory. We do not ever attempt to obtain the energy is this manner, by some miraculous use of the Hohenberg–Kohn theorem. The constrained-search approach is a much more intuitive and transparent introduction to DFT and the theory of Lieb provides an elegant mathematical framework that captures the essence of DFT.
5.4.2 Jones.
In my experience, lecture courses and seminars involving DFT usually give a rather boring view of its history. The world began in 1964 with Hohenberg–Kohn, Kohn–Sham made DFT usable, and so on. The listener learns nothing about the excitement people working in DFT experienced during the bleak years up to 1990, perhaps because the speaker does not know or care. Here are some points for consideration.
The Hohenberg–Kohn theorem5 is ubiquitous, but little used in practice. Its proper place today is in review articles and textbooks, and we should focus on the constrained-search approach6 and the formulation of DFT in terms of Legendre transforms.8 Kutzelnigg's “beginner's” guide to the latter is accessible to most in the DFT field.771
As noted in contribution (2.1.13), “the power of DFT derives from Kohn–Sham theory,” but the successes of Kohn–Sham theory are linked closely to the ability of local density approximations to the exchange–correlation energy Exc to give useful results in most cases. Kohn noted: “I believe that formal DFT would have been of very little interest if there had not been a simple and very practical approximation for Exc, the LDA, which has yielded surprisingly accurate results.”772
Kohn and Sham proposed using an LDA for Exc that is exact in two limits (slowly varying densities and high densities).65 These are far from the density distributions found in atoms, molecules, and condensed matter. Kohn and Sham, and many others, were therefore convinced that LDAs would not describe chemical bonding well. Nevertheless, they gave “reasonable” answers in early tests of energy differences (including small molecules120,469,773 and jellium half-spaces100,119) and remain the basis of many approximations for Exc. The initial successes of LDA were so surprising that they motivated work to understand why it could provide useful energy differences for systems with densities far from the regions of obvious validity. This work led to “adiabatic coupling” and studies of the exchange and correlation holes, their spherical averages and related sum rules,100,119,120 which have been of lasting value in DFT studies. This surprisingly satisfactory description of reality often provided by LDAs was essential to the ultimate success of DFT.
5.4.3 Chattaraj.
The density (its advantage over the wave function), density matrices (writing energy in terms of them), and density functionals (a map from a function to a number) should be introduced. DFT highlights the fact that, as N and v(r) fix the Hamiltonian, the Schrödinger equation is a map from these quantities to the density, whereas the corresponding inverse map (along with normalization) is DFT. Of course, Kato's cusp condition4 for the ground state of any system provides v(r) through nuclear positions and the charges, when the density is known. Various techniques (Kohn–Sham, Levy–Lieb, orbital-free DFT, etc.) exist for calculating the density. A recapitulation of Hartree–Fock theory may be helpful.
5.4.4 Maitra and Ullrich.
DFT is taught in many ways and at many levels: in addition to the tutorials, workshops, and summer schools mentioned in contribution (5.3.1), there are online courses,774 regular courses at universities, and pedagogical textbooks.659,775–777 The targeted audience often tends to be at an advanced level (graduate students, postdocs, researchers). However, there is an urgent need to teach DFT at a more basic, introductory level, to make it accessible to undergraduate students and to those who may not have a strong background in quantum mechanics, and who wish to understand and learn how to use DFT.
When teaching DFT to beginners, we face similar choices as in other fields of physics (e.g., quantum mechanics, electrodynamics): we can follow the historical path in which the field was established, or we can start with the most fundamental theorems and then build up the formalism, or we can introduce the subject through examples, case studies, and hands-on applications. In our experience, students tend to learn DFT better when the latter approach is taken; if we start with the theorems or with many-body theory, students often fail to see the connection to the “real world”. Thus, as a community, we should make an effort to make DFT more accessible and inclusive, and to do this it will be helpful to develop (and share) simple numerical examples and hands-on exercises.
5.4.5 Grimme.
The title of this paper contains the word “workhorse” and hence we should not forget to teach this aspect – that DFT really works every day in thousands of applications. DFT is a theory that is generally robust but students should also know when it fails (rarely) and why and how this is related to the DFAs (and other) approximations involved. Therefore, it is especially important to teach the basics of DFT in the context of real-world applications to illustrate that scientists are able to use it as a versatile tool to solve chemical and physical problems in many disciplines. The perception of DFT as a valuable component of today's fundamental chemistry–physics method toolbox should be promoted accordingly, through practicals and lectures with a pronounced hands-on mentality.
5.4.6 Gori-Giorgi.
I fully agree with everything said above, especially on using the constrained-search approach instead of introducing the Hohenberg–Kohn theorem. Obstacles I often see students facing when trying to learn DFT are:
1. To understand the theory behind DFT, you need to have a good understanding of many-electron wave functions and reduced density matrices, especially in real space, which they often lack.
2. Most pedagogical material focuses on DFT without spin densities, while in applications the latter are used.
3. Modern exchange–correlation functionals are very complicated and look obscure to them.
4. The language is often ambiguous – for example, in the literature and in conferences the term “local” is sometimes used to indicate a multiplicative potential (the local Kohn–Sham potential, as opposed to the nonlocal Hartree–Fock potential) and sometimes to indicate a local dependence on the density.
5. The role of symmetry breaking is very important and often neglected in pedagogical material.
Finally, I believe it would be useful to teach LDA in a more modern way, by including the recent works on the large – N limit of neutral atoms, which show in which sense LDA is a universal limit for Coulombically bound systems and how gradient expansions arise; see, for example, ref. 179 for a recent review.
5.4.7 Reining.
Good teaching should help the learner to take a step back and understand the essential elements, rather than the technical details. A crucial question that is in my opinion often neglected but merits deep thought, is the motivation: Why should we choose to work with DFT? The reason cannot just be that so many people do it successfully. In other words, we should (in general, and also for DFT) talk more about how we make choices in science. The answer may include a historical component and should mention alternatives – not for a detailed comparison, but to highlight some basic choices that may distinguish or be common to different methods. For DFT, I would insist on the following points:
• We know in principle how to calculate observables in terms of many-body wave functions, but we cannot do it in practice in most cases. The choice of DFT, instead, is to express ground-state observables as functionals of the ground-state density. The density is an object that is more compact (depending on fewer variables) than the many-body wave function;
• We could also express observables as functionals of other quantities that are more compact than many-body wave functions – DFT uses the ground-state density, but we could also choose density matrices, for example, as a good descriptor;
• As a rule of thumb, the more compact we make the descriptor, the fewer observables can be calculated as explicit functionals. We can therefore discuss when and why the density is a convenient choice – for example, when the Hartree energy is important;
• The idea to use an auxiliary system to determine one or more, but perhaps not all, observables exactly should be exposed clearly;
• In many-body physics, we know from the very start that approximations will be needed. It is then crucial to discuss why DFT is a good starting point for approximations;
• We should also discuss the choice of strategies for developing approximations, rather than the technical details. One example in DFT is to calculate the kinetic energy from a noninteracting system with the same density as the interacting one. Another example is to use the intuitive concept of nearsightedness.184,185
These are very general ideas that can be found – maybe under different names – also in contexts other than DFT, but they have all been important for the success of DFT.
Conflicts of interest
There are no conflicts to declare.
Appendices
A Acronyms
AC | Adiabatic connection |
ACFD | Adiabatic-connection fluctuation-dissipation |
ADFT | Auxiliary DFT |
AI | Artificial intelligence |
AIMD |
Ab initio MD |
AM1 | Austin Model 1 |
BBGKY | Bogoliubov–Born–Green–Kirkwood–Yvon |
BCB | Bottom of the conduction band |
BDFT | Magnetic DFT |
BLW | Block-localized wave function |
BOMD | Born–Oppenheimer molecular dynamics |
BSE | Bethe–Salpeter equation |
CASPiDFT/CASΠDFT | CAS pair-density functional theory |
CASSCF | Complete-active-space SCF |
CASPT2 | Complete-active-space PT2 |
CCSD | Coupled-cluster singles–doubles |
CCSD(T) | CCSD with perturbative triples |
CDFT | Current DFT |
CI | Configuration interaction |
DC | Dirac–Coulomb |
DD | Density driven |
DEKS | Direct-energy Kohn–Sham |
DF | Density functional |
DFA | Density-functional approximation |
DFET | Density-functional embedding theory |
DFT | Density-functional theory |
DFTB | Density-functional tight binding |
DFT+U | DFT with the Hubbard U correction |
DK | Douglas–Kroll |
DMET | Density-matrix embedding theory |
DMFT | Density-matrix functional theory |
DMRG | Density-matrix renormalization group |
dRPA | Direct RPA |
EA | Electron affinity |
ECW | Embedded correlated wave function |
EDA | Energy decomposition analysis |
EDFT | Ensemble DFT |
EFG | Electric field gradient |
ELS | Extended Löwdin–Shull |
ELS + | Augmented ELS |
EXX-OEP | Exact exchange – optimized effective potential |
FCI | Full CI |
FDET | Frozen-density embedding theory |
FPMD | First-principles molecular dynamics |
GASSCF | Generalized-active-space SCF |
GGA | Generalized gradient approximation |
GKS | Generalized Kohn–Sham |
GL | Görling–Levy |
GL2 | Second-order GL |
GMTKN55 | General main-group thermochemistry, kinetics, and non-covalent interactions dataset |
GW
| Green's function G and screened Coulomb interaction W |
GOEP | Generalized OEP |
GOK | Gross–Oliveira–Kohn |
HEG | Homogeneous electron gas |
HF | Hartree–Fock |
HK | Hohenberg–Kohn |
HOMO | Highest occupied MO |
Hxc | Hartree–exchange–correlation |
IP | Ionization potential |
i-DFT | DFT for steady-state transport |
KE | Kinetic energy |
KEDF | Kinetic-energy density functional |
KS | Kohn–Sham |
LFDFT | Ligand-field DFT |
LD | Local density |
LDA | Local density approximation |
LH | Local hybrid |
LOB | Lieb–Oxford bound |
LR/lr | Long range |
lrMCSCF–srDFT | Long-range MCSCF with short-range DFT |
lrWFT–srDFT | Long-range WFT with short-range DFT |
LUMO | Lowest occupied MO |
MAE | Mean absolute error |
MC | Multiconfigurational |
MBPT | Many-body perturbation theory |
MC-DFT | Multiconfiguration DFT |
MC-NCFT | Multiconfiguration nonclassical functional theory |
MC-PDFT | Multiconfiguration pair-density functional theory |
MCSCF | Multiconfigurational SCF |
MC-srDFT | MCSCF theory with short-range DFT |
MD | Molecular dynamics |
MD/MC | Molecular dynamics/Monte Carlo |
MGCDB84 | Main-group chemistry database with 84 data sets |
ML | Machine learning |
MLP | Machine-learned potential |
MM | Molecular mechanics |
MMRDFTP | Mild-mannered respectable DFT practitioner |
MNDO | Modified neglect of diatomic overlap |
MO | Molecular orbital |
MP4 | 4th-order Møller–Plesset |
MSDFT | Multistate DFT |
MSSCF | Multistate SCF |
NEVPT2 |
N-Electron valence state PT2 |
NOSI | Nonorthogonal state interaction |
NTO | Natural transition orbital |
OEP | Optimized effective potential |
OO | Orbital optimization |
OT | Optimal transport |
PAW | Projected augmented wave |
PD | Pair density |
PFET | Potential-functional embedding theory |
PFT | Potential-functional theory |
PM6 | Parameterization Method 6 |
PPLB | Perdew–Parr–Levy–Balduz |
PT2 | Second-order perturbation theory |
PZ | Perdew–Zunger |
QCT | Quantum-chemical topology |
QE-DFT | Quasi-energy DFT |
QED | Quantum electrodynamics |
QM | Quantum mechanics/mechanical |
QMC | Quantum Monte Carlo |
QMFF | Quantum-mechanical force field |
QM/MM | Quantum-mechanics/molecular-mechanics |
QM/QM | Quantum-mechanics/quantum-mechanics |
QTAIM | Quantum theory of atoms in molecules |
QZ | Quadruple zeta |
RASSCF | Restricted-active-space SCF |
RDF | Relativistic DF |
RDM | Reduced density matrix |
RHEG | Relativistic HEG |
RPA | Random-phase approximation |
RS | Range separation |
SA-CASSCF | State-averaged CASSCF |
SAOP | Statistical averaging of orbital potentials |
SCE | Strictly-correlated electrons |
SCF | Self-consistent field |
SF-TDDFT | Spin-flip TDDFT |
SIC | Self-interaction correction |
SIE | Self-interaction error |
SR/sr | Short range |
srDFT | Short-range DFT |
SSE | Sham–Schlüter equation |
SU(n) | Special unitary group of degree n |
TAO-DFT | Thermally-assisted-occupied DFT |
TB | Tight binding |
TDCDFT | Time-dependent CDFT |
TDDFT | Time-dependent DFT |
TDDMFT | Time-dependent DMFT |
TDF | Transition-density functional |
TRIM | Trust-region image minimization |
UEG | Uniform electron gas |
vdW | van der Waals |
WF | Wave function |
WFT | Wave-function theory |
XC | Exchange–correlation |
X-Pol | Explicit polarization |
X2C | Exact two-component |
Xα | X-alpha method |
ZDO | Zero differential overlap |
ΔSCF | Delta-SCF |
1RDM | First-order reduced density matrix |
2RDM | Second-order reduced density matrix |
B Mathematical symbols
A
| Electron affinity |
N | Set of ensemble v-representable densities |
E
| Total electronic energy |
E
H
| Hartree density functional |
E
xc
| Exchange–correlation density functional |
E
Hxc
|
E
H + Exc |
F
| Universal density functional |
f
| Fukui function |
H
| Hamiltonian |
I
| Ionization potential |
N | Set of N-representable densities |
j
μ
| Four-current-density |
j
| Total current-density |
j
p
| Paramagnetic current-density |
L
| Angular momentum |
L
p
( 3) | Set of Lebesgue p-integrable functions on 3 |
m
| Magnetization density |
m
e
| Electron mass |
N
| Number of electrons |
R
| Nuclear positions |
r
| Electron positions |
s
| Local softness |
S
2
| Total spin squared |
S
| Chemical softness |
t
| Time |
V
|
|
v
| External potential |
W
|
|
w
| Potential of interaction between electrons |
v
s
| Kohn–Sham potential |
v
xc
| Exchange–correlation potential |
T
| Kinetic energy |
T
s
| Kinetic energy of the Kohn–Sham noninteracting system |
W
| Two-particle interaction |
Z
| Nuclear charges |
γ
| First-order reduced density matrix |
Γ
| Two-particle reduced density matrix |
λ
| Coupling coefficient in adiabatic connection |
μ
| Chemical potential |
ε
| Orbital energy |
ζ
| Spin polarization |
Φ
| Slater determinant |
ϕ
| Orbital |
Π
| Pair density |
ρ
| Density |
ρ
Ψ
|
ρ given by Ψ |
σ
| Spin index |
ξ
| Ensemble weight |
Ψ
|
N-Electron wave function |
η
| Chemical hardness |
χ
| Linear response function |
ω
| Electrophilicity |
Acknowledgements
The authors are indebted to Steven Berry, Durham University, for preparation of the cover art graphics associated with this paper. AK acknowledges support from the U.S. National Science Foundation (NSF) through Grant No. CHE-2154482. AL acknowledges support from the Norwegian Research Council through Grant No. 287906 (CCerror) and 262695 (CoE Hylleraas Center for Quantum Molecular Sciences). AMK acknowledges support from the Mexican Consejo Nacional de Ciencia y Tecnología through Grant No. A1-S-11929. AMT acknowledges financial support from the European Research Council under H2020/ERC Consolidator Grant topDFT (Grant No. 772259). CAU is supported by DOE grant No. DE-SC0019109 and NSF grants No. DMR-1810922 and DMR-2149082. CD acknowledges funding by the German Research Foundation (DFG) through projects 460197019, 424709454, and 182087777 as well as by the European Union's Horizon 2020 research and innovation program under the grant agreement No. 951786 (NOMAD CoE). EAC acknowledges financial support from the Advanced Scientific Computing Research Program funded by the U.S. Department of Energy, Office of Science, Basic Energy Sciences, under Award No. DE-AC02-05CH11231. EC acknowledges support from the European Research Council (ERC) under H2020/ERC Grant EMC2 810367. EF thanks LabEx CSC (grant number: ANR-10-LABX-0026-CSC) and ANR (CoLab project, grant number: ANR-19-CE07-0024-02) for funding. ERJ thanks the Natural Sciences and Engineering Research Council of Canada (NSERC) for financial support. FDP and PG want to acknowledge the Vrije Universiteit Brussel for the support through a Strategic Research Program (SRP). GG acknowledges DOE/BES support through the Computational Materials Science Center MICCoM. HCh thanks gratefully C. Morell for his continuous support and for thorough discussions. JG acknowledges support from the U.S. National Institutes of Health under grant no. GM046736. JPP acknowledges support from the U.S. National Science Foundation under grant no. DMR-1939528 and from the U.S. Department of Energy under grant no. DE-SC0018331. LK is supported by the Helen and Martin Kimmel Award for Innovative Investigation and the Aryeh and Mintzi Katzman Professorial Chair. ML acknowledges support from the European Research Council (ERC) under H2020/ERC Grant MDFT 725528. MLe acknowledges support from the Julian Schwinger Foundation. MS acknowledges funding from the European Unions Horizon 2020 Research and Innovation Programme (No. 951786, the NOMAD Center of Excellence) and from the ERC Advanced Grant TEC1P (No. 740233). NG acknowledges financial support from The Leverhulme Trust, through a Research Project (Grant No. RPG-2016-005). PFL thanks the European Research Council (ERC) under the European Union's Horizon 2020 research and innovation programme (Grant agreement No. 863481) for financial support. PKC acknowledges DST, New Delhi, India for the J. C. Bose National Fellowship, grant number SR/S2/JCB-09/2009. PPi acknowledges support from the Chemical Sciences, Geosciences and Biosciences Division, Office of Basic Energy Sciences, Office of Science, U.S. Department of Energy under award no. DE-FG02-01ER15228. SBT is supported by U.S. DOE grant DE-SC0002139 and NSF grant DMR-1912618. TDC was supported by the Molecular Sciences Software Institute under U.S. National Science Foundation grants ACI-1547580 and CHE-2136142. TG acknowledges support from the Australian Research Council (grant no. DP200100033). TH acknowledges support from the Research Council of Norway for the CoE Hylleraas Centre for Molecular Sciences (Grant No. 262695). XX acknowledges support from the National Natural Science Foundation of China (Grant No. 21688102).
Notes and references
- Overleaf, an open-source online real-time collaborative LaTeX editor, https://www.overleaf.com.
- F. Zahariev and M. Levy, Phys. Rev. A, 2019, 100, 062507 CrossRef CAS.
- E. Fermi, Z. Phys., 1928, 48, 73–79 CrossRef CAS.
- T. Kato, Commun. Pure Appl. Math., 1957, 10, 151–177 CrossRef.
- P. Hohenberg and W. Kohn, Phys. Rev., 1964, 136, B864–B871 CrossRef.
- M. Levy, Proc. Natl. Acad. Sci. U. S. A., 1979, 76, 6062–6065 CrossRef CAS PubMed.
- M. Levy, Phys. Rev. A: At., Mol., Opt. Phys., 1982, 26, 1200–1208 CrossRef CAS.
- E. H. Lieb, Int. J. Quantum Chem., 1983, 24, 243–277 CrossRef CAS.
- N. Schuch and F. Verstraete, Nat. Phys., 2009, 5, 732–735 Search PubMed.
- J. P. Perdew and A. Zunger, Phys. Rev. B: Condens. Matter Mater. Phys., 1981, 23, 5048–5079 CrossRef CAS.
-
A. Savin, Theoretical and Computational Chemistry, Elsevier, 1996, pp. 327–357 Search PubMed.
- Y. Zhang and W. Yang, J. Chem. Phys., 1998, 109, 2604–2608 CrossRef CAS.
- A. D. Becke, J. Chem. Phys., 2005, 122, 064101 CrossRef PubMed.
- P. Mori-Sánchez, A. J. Cohen and W. Yang, J. Chem. Phys., 2006, 124, 091102 CrossRef.
- P. Mori-Sánchez, A. J. Cohen and W. Yang, J. Chem. Phys., 2006, 125, 201102 CrossRef PubMed.
- P. Mori-Sánchez, A. J. Cohen and W. T. Yang, Phys. Rev. Lett., 2008, 100, 146401 CrossRef PubMed.
- A. J. Cohen, P. Mori-Sánchez and W. Yang, Science, 2008, 321, 792–794 CrossRef CAS PubMed.
- J. P. Perdew, R. G. Parr, M. Levy and J. L. Balduz, Phys. Rev. Lett., 1982, 49, 1691–1694 CrossRef CAS.
- Y. Zhang and W. Yang, Theor. Chem. Acc., 2000, 103, 346–348 Search PubMed.
- W. Yang, Y. Zhang and P. W. Ayers, Phys. Rev. Lett., 2000, 84, 5172–5175 CrossRef CAS PubMed.
- A. J. Cohen, P. Mori-Sánchez and W. Yang, Phys. Rev. B: Condens. Matter Mater. Phys., 2008, 77, 115123 CrossRef.
- X. Zheng, A. J. Cohen, P. Mori-Sánchez, X. Hu and W. Yang, Phys. Rev. Lett., 2011, 107, 026403 CrossRef PubMed.
- C. Li, X. Zheng, N. Q. Su and W. Yang, Natl. Sci. Rev., 2018, 5, 203–215 CrossRef CAS.
- N. Q. Su, A. Mahler and W. Yang, J. Phys. Chem. Lett., 2020, 11, 1528–1535 CrossRef CAS PubMed.
- Y. Mei, Z. Chen and W. Yang, J. Phys. Chem. Lett., 2020, 11, 10269–10277 CrossRef CAS PubMed.
- A. J. Cohen, P. Mori-Sánchez and W. Yang, J. Chem. Phys., 2008, 129, 121104 CrossRef PubMed.
- P. Mori-Sánchez, A. J. Cohen and W. Yang, Phys. Rev. Lett., 2009, 102, 066403 CrossRef PubMed.
- N. Q. Su, C. Li and W. Yang, Proc. Natl. Acad. Sci. U. S. A., 2018, 115, 9678–9683 CrossRef CAS PubMed.
- P.-O. Löwdin, Int. J. Quantum Chem., 1985, 28, 19–37 CrossRef.
- W. Yang, P. W. Ayers and Q. Wu, Phys. Rev. Lett., 2004, 92, 146404 CrossRef PubMed.
- R. F. Nalewajski and R. G. Parr, J. Chem. Phys., 1982, 77, 399–407 CrossRef CAS.
-
T. Helgaker and A. M. Teale, The physics and mathematics of Elliott Lieb, European Mathematical Society Press, London, 2022, vol. I, pp. 527–559 Search PubMed.
-
M. Lewin, E. H. Lieb and R. Seiringer, 2020, arXiv e-prints, arXiv:1912.10424.
- P. E. Lammert, Phys. Rev. A: At., Mol., Opt. Phys., 2010, 82, 012109 CrossRef.
- L. Garrigue, Math. Phys. Anal. Geom., 2018, 21, 27 CrossRef.
- K. Capelle and G. Vignale, Phys. Rev. B: Condens. Matter Mater. Phys., 2002, 65, 113106 CrossRef.
- A. Laestadius and E. I. Tellgren, Phys. Rev. A, 2018, 97, 022514 CrossRef CAS.
- A. E. Clark, H. Adams, R. Hernandez, A. I. Krylov, A. M. N. Niklasson, S. Sarupria, Y. Wang, S. M. Wild and Q. Yang, ACS Cent. Sci., 2021, 7(8), 1271–1287 CrossRef CAS PubMed.
- J. W. Furness, J. Verbeke, E. I. Tellgren, S. Stopkowicz, U. Ekström, T. Helgaker and A. M. Teale, J. Chem. Theory Comput., 2015, 11, 4169–4181 CrossRef CAS PubMed.
- A. Görling, J. Chem. Phys., 2005, 123, 062203 CrossRef PubMed.
- R. T. Sharp and G. K. Horton, Phys. Rev., 1953, 90, 317 CrossRef.
- J. D. Talman and W. F. Shadwick, Phys. Rev. A: At., Mol., Opt. Phys., 1976, 14, 36–40 CrossRef CAS.
- A. Görling, Phys. Rev. Lett., 1999, 83, 5459–5462 CrossRef.
- S. Ivanov, S. Hirata and R. J. Bartlett, Phys. Rev. Lett., 1999, 83, 5455–5458 CrossRef CAS.
- S. Kümmel and L. Kronik, Rev. Mod. Phys., 2008, 80, 3–60 CrossRef.
- E. Trushin and A. Görling, J. Chem. Phys., 2021, 155, 054109 CrossRef CAS PubMed.
-
R. G. Parr and W. Yang, Density Functional Theory of Atoms and Molecules, Oxford University Press, Oxford, 1989 Search PubMed.
-
N. H. March and B. M. Deb, The single-particle density in physics and chemistry, Academic Press, London, 1987 Search PubMed.
-
Chemical Reactivity Theory: A Density Functional View, ed. P. K. Chattaraj, CRC Press, Taylor & Francis Group, Florida, 2009 Search PubMed.
- N. D. Mermin, Phys. Rev., 1965, 137, A1441–A1443 CrossRef.
- V. V. Karasiev, T. Sjostrom, J. Dufty and S. B. Trickey, Phys. Rev. Lett., 2014, 112, 076403 CrossRef PubMed.
- T. Sjostrom and J. Daligault, Phys. Rev. Lett., 2014, 113, 155006 CrossRef PubMed.
-
V. Karasiev, T. Sjostrom, D. Chakraborty, J. W. Dufty, F. E. Harris, K. Runge and S. B. Trickey, Frontiers and Challenges in Warm Dense Matter, Springer, 2014, pp. 61–85 Search PubMed.
- V. V. Karasiev, J. W. Dufty and S. B. Trickey, Phys. Rev. Lett., 2018, 120, 076401 CrossRef CAS PubMed.
-
J. C. Smith, F. Sagredo and K. Burke, Frontiers of Quantum Chemistry, Springer, 2018, pp. 249–271 Search PubMed.
- V. V. Karasiev, S. B. Trickey and J. W. Dufty, Phys. Rev. B, 2019, 99, 195134 CrossRef CAS.
- A. K. Rajagopal and J. Callaway, Phys. Rev. B: Solid State, 1973, 7, 1912–1919 CrossRef CAS.
-
E. Engel, Relativistic Electronic Structure Theory: Part 2. Applications, Elsevier, Amsterdam, 2004, pp. 523–621 Search PubMed.
- A. H. MacDonald and S. H. Vosko, J. Phys. C: Solid State Phys., 1979, 12, 2977–2990 CrossRef CAS.
- M. V. Ramana and A. K. Rajagopal, J. Phys. C: Solid State Phys., 1981, 14, 4291–4302 CrossRef.
-
N. N. Bogoliubov, Problems of Dynamical Theory in Statistical Physics (in Russian) [Studies in Statistical Mechanics, edited by J. de Boer and G. E. Uhlenbeck, North-Holland, Amsterdam, 1961, vol. 1)], Gostekhisdat, Moscow, 1946 Search PubMed.
-
E. R. Davidson, Reduced Density Matrices in Quantum Chemistry, Academic Press, London, 1976 Search PubMed.
- K. Pernal, O. Gritsenko and E. J. Baerends, Phys. Rev. A: At., Mol., Opt. Phys., 2007, 75, 012506 CrossRef.
- R. Van Meer, O. Gritsenko and J.-D. Chai, Phys. Rev. A, 2020, 102, 032815 CrossRef CAS.
- W. Kohn and L. J. Sham, Phys. Rev., 1965, 140, A1133–A1138 CrossRef.
- U. von Barth and L. Hedin, J. Phys. C: Solid State Phys., 1972, 5, 1629–1642 CrossRef CAS.
- A. Seidl, A. Görling, P. Vogl, J. A. Majewski and M. Levy, Phys. Rev. B: Condens. Matter Mater. Phys., 1996, 53, 3764–3774 CrossRef CAS PubMed.
- R. Garrick, A. Natan, T. Gould and L. Kronik, Phys. Rev. X, 2020, 10, 021040 CAS.
- E. J. Baerends and O. V. Gritsenko, J. Phys. Chem. A, 1997, 101, 5383–5403 CrossRef CAS.
- D. P. Chong, O. V. Gritsenko and E. J. Baerends, J. Chem. Phys., 2002, 116, 1760–1772 CrossRef CAS.
- O. V. Gritsenko, B. Braida and E. J. Baerends, J. Chem. Phys., 2003, 119, 1937–1950 CrossRef CAS.
- A. Savin, C. J. Umrigar and X. Gonze, Chem. Phys. Lett., 1998, 288, 391–395 CrossRef CAS.
- R. van Meer, O. V. Gritsenko and E. J. Baerends, J. Chem. Theory Comput., 2014, 10, 4432–4441 CrossRef CAS PubMed.
- A. I. Krylov, J. Chem. Phys., 2020, 153, 080901 CrossRef CAS PubMed.
- J. V. Ortiz, J. Chem. Phys., 2020, 153, 070902 CrossRef CAS PubMed.
- U. Salzner and R. Baer, J. Chem. Phys., 2009, 131, 231101 CrossRef PubMed.
- R. Baer, E. Livshits and U. Salzner, Annu. Rev. Phys. Chem., 2010, 61, 85–109 CrossRef CAS PubMed.
- A. I. Krylov, Annu. Rev. Phys. Chem., 2008, 59, 433–462 CrossRef CAS PubMed.
- V. M. Medel, A. C. Reber, V. Chauhan, P. Sen, A. M. Köster, P. Calaminici and S. N. Khanna, J. Am. Chem. Soc., 2014, 136, 8229–8236 CrossRef CAS PubMed.
- P. Gori-Giorgi, T. Gál and E. J. Baerends, Mol. Phys., 2016, 114, 1086–1097 CrossRef CAS.
- E. J. Baerends, O. V. Gritsenko and R. van Meer, Phys. Chem. Chem. Phys., 2013, 15, 16408–16425 RSC.
- E. J. Baerends, Phys. Chem. Chem. Phys., 2017, 19, 15639–15656 RSC.
- O. V. Gritsenko, P. R. T. Schipper and E. J. Baerends, J. Chem. Phys., 1997, 107, 5007–5015 CrossRef CAS.
- N. I. Gidopoulos, Phys. Rev. A: At., Mol., Opt. Phys., 2011, 83, 040502 CrossRef.
- T. J. Callow and N. I. Gidopoulos, Eur. Phys. J. B, 2018, 91, 1–13 CrossRef.
-
R. G. Parr and W. Yang, Density-Functional Theory of Atoms and Molecules, Oxford University Press, New York, 1989 Search PubMed.
- J. F. Janak, Phys. Rev., 1978, B18, 7165–7168 Search PubMed.
- Y. Mei, C. Li, N. Q. Su and W. Yang, J. Phys. Chem. A, 2019, 123, 666–673 CrossRef CAS PubMed.
- Y. Mei and W. Yang, J. Phys. Chem. Lett., 2019, 2538–2545 CrossRef CAS PubMed.
- E. J. Baerends, J. Chem. Phys., 2018, 149, 054105 CrossRef PubMed.
- L. Kronik, T. Stein, S. Refaely-Abramson and R. Baer, J. Chem. Theory Comput., 2012, 8, 1515–1531 CrossRef CAS PubMed.
- G. Borghi, A. Ferretti, N. L. Nguyen, I. Dabo and N. Marzari, Phys. Rev. B: Condens. Matter Mater. Phys., 2014, 90, 075135 CrossRef CAS.
- Y. Jin, N. Q. Su, Z. Chen and W. Yang, Faraday Discuss., 2020, 224, 9–26 RSC.
- Y. Jin, D. Zhang, Z. Chen, N. Q. Su and W. Yang, J. Phys. Chem. Lett., 2017, 8, 4746–4751 CrossRef CAS PubMed.
- Z. Chen, D. Zhang, Y. Jin, Y. Yang, N. Q. Su and W. Yang, J. Phys. Chem. Lett., 2017, 4479–4485 CrossRef CAS PubMed.
- V. K. Voora, S. G. Balasubramani and F. Furche, Phys. Rev. A, 2019, 99, 012518 CrossRef CAS.
- E. Runge and E. K. U. Gross, Phys. Rev. Lett., 1984, 52, 997–1000 CrossRef CAS.
- M. Ruggenthaler, M. Penz and R. van Leeuwen, J. Phys.: Condens. Matter, 2015, 27, 203202 CrossRef PubMed.
- A. Laestadius, M. Penz, E. I. Tellgren, M. Ruggenthaler, S. Kvaal and T. Helgaker, J. Chem. Phys., 2018, 149, 164103 CrossRef PubMed.
- D. C. Langreth and J. P. Perdew, Solid State Commun., 1975, 17, 1425–1429 CrossRef.
- D. C. Langreth and J. P. Perdew, Phys. Rev. B: Solid State, 1977, 15, 2884–2901 CrossRef.
- A. Heßelmann and A. Görling, Mol. Phys., 2011, 109, 2473–2500 CrossRef.
- H. Eshuis, J. E. Bates and F. Furche, Theor. Chem. Acc., 2012, 131, 1084 Search PubMed.
- X. Ren, P. Rinke, C. Joas and M. Scheffler, J. Mater. Sci., 2012, 47, 7447–7471 CrossRef CAS.
- J. Kalikka, J. Akola and R. O. Jones, Phys. Rev. B, 2016, 94, 134105 CrossRef.
- A. Savin and H.-J. Flad, Int. J. Quantum Chem., 1995, 56, 327–332 CrossRef CAS.
- M. Levy and F. Zahariev, Phys. Rev. Lett., 2014, 113, 113002 CrossRef PubMed.
- D. J. Sharpe, M. Levy and D. J. Tozer, J. Chem. Theory Comput., 2018, 14, 684–692 CrossRef CAS PubMed.
- A. K. Rajagopal, J. Phys. C: Solid State Phys., 1978, 11, L943–L948 CrossRef CAS.
- E. Engel, S. Keller, A. Facco Bonetti, H. Müller and R. M. Dreizler, Phys. Rev. A: At., Mol., Opt. Phys., 1995, 52, 2750–2764 CrossRef CAS PubMed.
- E. Engel, S. Keller and R. M. Dreizler, Phys. Rev. A: At., Mol., Opt. Phys., 1996, 53, 1367–1374 CrossRef CAS PubMed.
- S. Varga, E. Engel, W.-D. Sepp and B. Fricke, Phys. Rev. A: At., Mol., Opt. Phys., 1999, 59, 4288–4294 CrossRef CAS.
- J. Paquier and J. Toulouse, J. Chem. Phys., 2018, 149, 174110 CrossRef PubMed.
- J. Paquier, E. Giner and J. Toulouse, J. Chem. Phys., 2020, 152, 214106 CrossRef CAS PubMed.
- T. M. Maier, Y. Ikabata and H. Nakai, J. Chem. Phys., 2020, 152, 214103 CrossRef CAS PubMed.
-
Y. Mei, C. Li, N. Q. Su and W. Yang, 2018, arXiv.1810.09906.
- R. L. A. Haiduke and R. J. Bartlett, J. Chem. Phys., 2018, 149, 131101 CrossRef PubMed.
- S. Vuckovic, S. Song, J. Kozlowski, E. Sim and K. Burke, J. Chem. Theory Comput., 2019, 15, 6636–6646 CrossRef CAS PubMed.
- J. Harris and R. O. Jones, J. Phys. F: Met. Phys., 1974, 4, 1170–1186 CrossRef.
- O. Gunnarsson and B. I. Lundqvist, Phys. Rev. B: Solid State, 1976, 13, 4274–4298 CrossRef CAS.
- T. Ziegler, A. Rauk and E. J. Baerends, Theor. Chim. Acta, 1977, 43, 261–271 CrossRef CAS.
- A. D. Becke, Int. J. Quantum Chem., 1983, 23, 1915–1922 CrossRef CAS.
- J. P. Perdew, Phys. B, 1991, 172, 1–6 CrossRef CAS.
- A. D. Becke, J. Chem. Phys., 1993, 98, 1372–1377 CrossRef CAS.
- M. Levy and A. Görling, Phys. Rev. A: At., Mol., Opt. Phys., 1995, 52, R1808–R1810 CrossRef CAS PubMed.
- A. D. Becke, Phys. Rev. A: At., Mol., Opt. Phys., 1988, 38, 3098–3100 CrossRef CAS PubMed.
- C. T. Lee, W. T. Yang and R. G. Parr, Phys. Rev. B: Condens. Matter Mater. Phys., 1988, 37, 785–789 CrossRef CAS PubMed.
- A. D. Becke, J. Chem. Phys., 1993, 98, 5648–5652 CrossRef CAS.
- P. J. Stephens, F. J. Devlin, C. F. Chabalowski and M. J. Frisch, J. Phys. Chem., 1994, 98, 11623–11627 CrossRef CAS.
- J. P. Perdew, M. Ernzerhof and K. Burke, J. Chem. Phys., 1996, 105, 9982–9985 CrossRef CAS.
- A. J. Cohen, P. Mori-Sánchez and W. Yang, J. Chem. Phys., 2007, 127, 034101 CrossRef PubMed.
- F. Furche, Phys. Rev. B: Condens. Matter Mater. Phys., 2001, 64, 195120 CrossRef.
- A. Görling and M. Levy, Phys. Rev. A: At., Mol., Opt. Phys., 1994, 50, 196–204 CrossRef PubMed.
- Y. Zhang, X. Xu and W. A. Goddard, Proc. Natl. Acad. Sci. U. S. A., 2009, 106, 4963–4968 CrossRef CAS PubMed.
- S. Grimme, J. Chem. Phys., 2006, 124, 034108 CrossRef PubMed.
- A. Tarnopolsky, A. Karton, R. Sertchook, D. Vuzman and J. M. L. Martin, J. Phys. Chem. A, 2008, 112, 3–8 CrossRef CAS PubMed.
- J. D. Chai and M. Head-Gordon, J. Chem. Phys., 2009, 131, 174105 CrossRef PubMed.
- M. Seidl and P. Gori-Giorgi, Phys. Rev. A: At., Mol., Opt. Phys., 2010, 81, 012508 CrossRef.
- M. Lewin, C. R. Math., 2018, 356, 449–455 CrossRef.
- C. Cotar, G. Friesecke and C. Klüppelberg, Arch. Ration. Mech. Anal., 2018, 228, 891–922 CrossRef.
- M. Seidl, Phys. Rev. A: At., Mol., Opt. Phys., 1999, 60, 4387–4395 CrossRef CAS.
- M. Seidl, J. P. Perdew and M. Levy, Phys. Rev. A: At., Mol., Opt. Phys., 1999, 59, 51–54 CrossRef CAS.
- M. Seidl, P. Gori-Giorgi and A. Savin, Phys. Rev. A: At., Mol., Opt. Phys., 2007, 75, 042511 CrossRef.
- P. Gori-Giorgi, G. Vignale and M. Seidl, J. Chem. Theory Comput., 2009, 5, 743–753 CrossRef CAS PubMed.
- S. Vuckovic and P. Gori-Giorgi, J. Phys. Chem. Lett., 2017, 8, 2799–2805 CrossRef CAS PubMed.
- S. Vuckovic, J. Chem. Theory Comput., 2019, 15, 3580–3590 CrossRef CAS PubMed.
- T. Gould and S. Vuckovic, J. Chem. Phys., 2019, 151, 184101 CrossRef PubMed.
- M. Seidl, J. P. Perdew and S. Kurth, Phys. Rev. Lett., 2000, 84, 5070–5073 CrossRef CAS PubMed.
- S. Vuckovic, T. J. P. Irons, A. Savin, A. M. Teale and P. Gori-Giorgi, J. Chem. Theory Comput., 2016, 12, 2598–2610 CrossRef CAS PubMed.
- S. Vuckovic, P. Gori-Giorgi, F. Della Sala and E. Fabiano, J. Phys. Chem. Lett., 2018, 9, 3137–3142 CrossRef CAS PubMed.
- S. Giarrusso, P. Gori-Giorgi, F. Della Sala and E. Fabiano, J. Chem. Phys., 2018, 148, 134106 CrossRef PubMed.
- F. Colonna and A. Savin, J. Chem. Phys., 1999, 110, 2828–2835 CrossRef CAS.
- Q. Wu and W. Yang, J. Chem. Phys., 2003, 118, 2498–2509 CrossRef CAS.
- A. M. Teale, S. Coriani and T. Helgaker, J. Chem. Phys., 2009, 130, 104111 CrossRef CAS PubMed.
- A. M. Teale, S. Coriani and T. Helgaker, J. Chem. Phys., 2010, 132, 164115 CrossRef CAS PubMed.
- W. Yang, J. Chem. Phys., 1998, 109, 10107–10110 CrossRef CAS.
- A. M. Teale, S. Coriani and T. Helgaker, J. Chem. Phys., 2010, 133, 164112 CrossRef CAS PubMed.
- A. Savin, Phys. Rev. A: At., Mol., Opt. Phys., 1995, 52, R1805–R1807 CrossRef CAS PubMed.
- A. M. Teale, T. Helgaker and A. Savin, J. Chin. Chem. Soc., 2015, 63, 121–128 CrossRef.
- T. J. P. Irons and A. M. Teale, Mol. Phys., 2015, 1–14 CrossRef.
- E. K. U. Gross and C. R. Proetto, J. Chem. Theory Comput., 2009, 5, 844–849 CrossRef CAS PubMed.
- T. J. Daas, J. Grossi, S. Vuckovic, Z. H. Musslimani, D. P. Kooi, M. Seidl, K. J. H. Giesbertz and P. Gori-Giorgi, J. Chem. Phys., 2020, 153, 214112 CrossRef CAS PubMed.
-
E. Engel and R. M. Dreizler, Density Functional Theory. Theoretical and Mathematical Physics, Springer, Berlin, Heidelberg, 2011, pp. 11–56 Search PubMed.
- B. G. Giraud, B. K. Jennings and B. R. Barrett, Phys. Rev. A: At., Mol., Opt. Phys., 2008, 78, 032507 CrossRef.
- A. D. Becke, J. Chem. Phys., 2013, 138, 074109 CrossRef PubMed.
- A. D. Becke, J. Chem. Phys., 1988, 88, 1053–1062 CrossRef CAS.
- C. Adamo and V. Barone, J. Phys. Chem., 1999, 110, 6158–6170 CrossRef CAS.
- A. V. Arbuznikov and M. Kaupp, J. Chem. Phys., 2008, 128, 214107 CrossRef PubMed.
- J. Kong and E. Proynov, J. Chem. Theory Comput., 2016, 12, 133–143 CrossRef CAS PubMed.
- P. Gori-Giorgi and M. Seidl, Phys. Chem. Chem. Phys., 2010, 12, 14405–14419 RSC.
- H. Bahmann, Y. Y. Zhou and M. Ernzerhof, J. Chem. Phys., 2016, 145, 124104 CrossRef PubMed.
- S. Vuckovic, L. Wagner, A. Mirtschink and P. Gori-Giorgi, J. Chem. Theory Comput., 2015, 11, 3153–3162 CrossRef CAS PubMed.
- A. Savin, Chem. Phys., 2009, 356, 91–97 CrossRef CAS.
- R. Baer and L. Kronik, Eur. Phys. J. B, 2018, 91, 170 CrossRef.
- T. Gould and L. Kronik, J. Chem. Phys., 2021, 154, 094125 CrossRef CAS PubMed.
- A. Görling and M. Levy, J. Chem. Phys., 1997, 106, 2675–2680 CrossRef.
- M. Levy and J. P. Perdew, Phys. Rev. B: Condens. Matter Mater. Phys., 1993, 48, 11638–11645 CrossRef CAS PubMed.
- S. Vuckovic, M. Levy and P. Gori-Giorgi, J. Chem. Phys., 2017, 147, 214107 CrossRef PubMed.
-
P. Okun and K. Burke, 2021, arXiv.2105.04384.
- N. Orms, D. R. Rehn, A. Dreuw and A. I. Krylov, J. Chem. Theory Comput., 2018, 14, 638–648 CrossRef CAS PubMed.
- Y. Shao, M. Head-Gordon and A. I. Krylov, J. Chem. Phys., 2003, 118, 4807–4818 CrossRef CAS.
- Y. A. Bernard, Y. Shao and A. I. Krylov, J. Chem. Phys., 2012, 136, 204103 CrossRef PubMed.
- D. Casanova and A. I. Krylov, Phys. Chem. Chem. Phys., 2020, 22, 4326–4342 RSC.
- W. Kohn, Phys. Rev. Lett., 1996, 76, 3168–3171 CrossRef CAS PubMed.
- E. Prodan and W. Kohn, Proc. Natl. Acad. Sci. U. S. A., 2005, 102, 11635–11638 CrossRef CAS.
- R. G. Parr, R. Donnelly and W. Palke, J. Chem. Phys., 1978, 68, 3801–3807 CrossRef CAS.
- R. P. Iczkowski and J. L. Margrave, J. Am. Chem. Soc., 1961, 83, 3547–3551 CrossRef CAS.
- H. Chermette, J. Comput. Chem., 1999, 20, 129–154 CrossRef CAS.
- P. Geerlings, F. De Proft and W. Langenaeker, Chem. Rev., 2003, 103, 1793–1873 CrossRef CAS PubMed.
- R. Parr, L. Szentpaly and S. Liu, J. Am. Chem. Soc., 1999, 121, 1922–1924 CrossRef CAS.
- P. K. Chattaraj, U. Sarkar and D. R. Roy, Chem. Rev., 2006, 106, 2065–2091 CrossRef CAS PubMed.
- W. Yang, A. J. Cohen, F. De Proft and P. Geerlings, J. Chem. Phys., 2012, 136, 144110 CrossRef PubMed.
- C. Rong, B. Wang, D. Zhao and S. Liu, Wiley Interdiscip. Rev.: Comput. Mol. Sci., 2020, 10, e1461 CAS.
- R. Nalewajski and R. Parr, Proc. Natl. Acad. Sci. U. S. A., 2000, 97, 8879–8882 CrossRef CAS PubMed.
- S. Liu, J. Chem. Phys., 2007, 126, 244103 CrossRef PubMed.
- S. Liu, C. Rong and T. Lu, Phys. Chem. Chem. Phys., 2017, 19, 1496–1503 RSC.
- S. Liu, C. Rong, T. Lu and H. Hu, J. Phys. Chem. A, 2018, 122, 3087–3095 CrossRef CAS PubMed.
- A. D. Becke and K. E. Edgecombe, J. Chem. Phys., 1990, 92, 5397–5403 CrossRef CAS.
- B. Silvi and A. Savin, Nature, 1994, 371, 683–686 CrossRef CAS.
- E. R. Johnson, S. Keinan, P. Mori-Sánchez, J. Contreras-García, A. J. Cohen and W. Yang, J. Am. Chem. Soc., 2010, 132, 6498–6506 CrossRef CAS PubMed.
- P. Politzer, J. S. Murray and F. A. Bulat, J. Mol. Model., 2010, 16, 1731–1742 CrossRef CAS PubMed.
- I. G. Ryabinkin and V. N. Staroverov, J. Chem. Phys., 2014, 141, 084107 CrossRef PubMed.
- S. V. Kohut, R. Cuevas-Saavedra and V. N. Staroverov, J. Chem. Phys., 2016, 145, 074113 CrossRef PubMed.
- E. Ospadov, J. Tao, V. N. Staroverov and J. P. Perdew, Proc. Natl. Acad. Sci. U. S. A., 2018, 115, E11578–E11585 CrossRef CAS PubMed.
- K. Pernal, Phys. Rev. Lett., 2018, 120, 013001 CrossRef CAS PubMed.
- E. Pastorczak and K. Pernal, J. Chem. Theory Comput., 2018, 14, 3493–3503 CrossRef CAS PubMed.
- D. Drwal, E. Pastorczak and K. Pernal, J. Chem. Phys., 2021, 154, 164102 CrossRef CAS PubMed.
-
A. Savin, Recent Developments of Modern Density Functional Theory, Elsevier, Amsterdam, 1996, pp. 327–357 Search PubMed.
- J. Toulouse, F. Colonna and A. Savin, Phys. Rev. A: At., Mol., Opt. Phys., 2004, 70, 062505 CrossRef.
- J. Toulouse, W. Zhu, J. G. Angyán and A. Savin, Phys. Rev. A: At., Mol., Opt. Phys., 2010, 82, 032502 CrossRef.
- M. Hapka, E. Pastorczak, A. Krzemińska and K. Pernal, J. Chem. Phys., 2020, 152, 094102 CrossRef CAS PubMed.
- K. Pernal and M. Hapka, Wiley Interdiscip. Rev.: Comput. Mol. Sci., 2021, e1566 Search PubMed.
- A. Ferté, E. Giner and J. Toulouse, J. Chem. Phys., 2019, 150, 084103 CrossRef PubMed.
- N. Otte, M. Scholten and W. Thiel, J. Phys. Chem. A, 2007, 111, 5751–5755 CrossRef CAS PubMed.
- G. Seifert, D. Porezag and T. Frauenheim, Int. J. Quantum Chem., 1996, 58, 185–192 CrossRef CAS.
- A. S. Christensen, T. Kubař, Q. Cui and M. Elstner, Chem. Rev., 2016, 116, 5301–5337 CrossRef CAS PubMed.
- M. C. Foulkes and R. Haydock, Phys. Rev. B: Condens. Matter Mater. Phys., 1989, 39, 12520–12536 CrossRef PubMed.
- C. Bannwarth, E. Caldeweyher, S. Ehlert, A. Hansen, P. Pracht, J. Seibert, S. Spicher and S. Grimme, Wiley Interdiscip. Rev.: Comput. Mol. Sci., 2021, 11, e1493 CAS.
- V. Lutsker, B. Aradi and T. A. Niehaus, J. Chem. Phys., 2015, 143, 184107 CrossRef CAS PubMed.
- J. J. Kranz, M. Elstner, B. Aradi, T. Frauenheim, V. Lutsker, A. D. Garcia and T. A. Niehaus, J. Chem. Theory Comput., 2017, 13, 1737–1747 CrossRef CAS PubMed.
- F. P. Bonafé, B. Aradi, B. Hourahine, C. R. Medrano, F. J. Hernández, T. Frauenheim and C. G. Sánchez, J. Chem. Theory Comput., 2020, 16, 4454–4469 CrossRef PubMed.
- A. Pecchia, G. Penazzi, L. Salvucci and A. Di Carlo, New J. Phys., 2008, 10, 065022 CrossRef.
- E. Baerends, D. E. Ellis and P. Ros, Chem. Phys., 1973, 2, 41–51 CrossRef CAS.
- H. Sambe and R. H. Felton, J. Chem. Phys., 1975, 62, 1122–1126 CrossRef CAS.
- B. I. Dunlap, J. W. D. Connolly and J. R. Sabin, J. Chem. Phys., 1979, 71, 4993–4999 CrossRef CAS.
- D. Mejía-Rodríguez and A. M. Köster, J. Chem. Phys., 2014, 141, 124114 CrossRef PubMed.
- J. N. Pedroza-Montero, J. L. Morales, G. Geudtner, A. Álvarez-Ibarra, P. Calaminici and A. M. Köster, J. Chem. Theory Comput., 2020, 16, 2965–2974 CrossRef CAS PubMed.
- D. N. Laikov, Chem. Phys. Lett., 1997, 281, 151–156 CrossRef CAS.
- A. M. Köster, J. U. Reveles and J. M. del Campo, J. Chem. Phys., 2004, 121, 3417–3424 CrossRef PubMed.
- R. I. Delgado-Venegas, D. Mejía-Rodríguez, R. Flores-Moreno, P. Calaminici and A. M. Köster, J. Chem. Phys., 2016, 145, 224103 CrossRef PubMed.
- W.-M. Hoe, A. J. Cohen and N. C. Handy, Chem. Phys. Lett., 2001, 341, 319–328 CrossRef CAS.
- M. Swart, A. Ehlers and K. Lammertsma, Mol. Phys., 2004, 102, 2467–2474 CrossRef CAS.
- N. Mardirossian and M. Head-Gordon, Mol. Phys., 2017, 115, 2315–2372 CrossRef CAS.
- J. P. Perdew, K. Burke and M. Ernzerhof, Phys. Rev. Lett., 1996, 77, 3865–3868 CrossRef CAS PubMed.
- J. Sun, A. Ruzsinszky and J. P. Perdew, Phys. Rev. Lett., 2015, 115, 036402 CrossRef PubMed.
- N. Mardirossian and M. Head-Gordon, J. Chem. Phys., 2016, 144, 214110 CrossRef PubMed.
- P.-F. Loos and P. M. W. Gill, Wiley Interdiscip. Rev.: Comput. Mol. Sci., 2016, 6, 410–429 CAS.
- M. Seidl, Phys. Rev. A: At., Mol., Opt. Phys., 2007, 75, 062506 CrossRef.
- P. M. W. Gill and P.-F. Loos, Theor. Chem. Acc., 2012, 131, 1069 Search PubMed.
- P.-F. Loos, J. Chem. Phys., 2017, 146, 114108 CrossRef PubMed.
- D. M. Ceperley and B. J. Alder, Phys. Rev. Lett., 1980, 45, 566–569 CrossRef CAS.
- A. Aouina, M. Gatti and L. Reining, Faraday Discuss., 2020, 224, 27–55 RSC.
- G. C. Lie and E. Clementi, J. Chem. Phys., 1974, 60, 1275–1287 CrossRef CAS.
- A. D. Becke and M. R. Roussel, Phys. Rev. A: At., Mol., Opt. Phys., 1989, 39, 3761–3767 CrossRef CAS PubMed.
- I. G. Ryabinkin, S. V. Kohut and V. N. Staroverov, Phys. Rev. Lett., 2015, 115, 083001 CrossRef PubMed.
- E. Ospadov, I. G. Ryabinkin and V. N. Staroverov, J. Chem. Phys., 2017, 146, 084103 CrossRef PubMed.
- I. G. Ryabinkin, E. Ospadov and V. N. Staroverov, J. Chem. Phys., 2017, 147, 164117 CrossRef PubMed.
- A. D. Becke, J. Chem. Phys., 1986, 85, 7184–7187 CrossRef CAS.
- J. Perdew and Y. Wang, Phys. Rev. B: Condens. Matter Mater. Phys., 1986, 33, 8800–8802 CrossRef PubMed.
- D. J. Lacks and R. G. Gordon, Phys. Rev. A: At., Mol., Opt. Phys., 1993, 47, 4681–4690 CrossRef CAS PubMed.
- Y. Zhang, W. Pan and W. Yang, J. Chem. Phys., 1997, 107, 7921–7925 CrossRef CAS.
- A. J. A. Price, K. R. Bryenton and E. R. Johnson, J. Chem. Phys., 2021, 154, 230902 CrossRef CAS PubMed.
- M. Ernzerhof and G. E. Scuseria, J. Phys. Chem., 1999, 110, 5029–5036 CrossRef CAS.
- J. Toulouse, K. Sharkas, E. Bremond and C. Adamo, J. Chem. Phys., 2011, 135, 101102 CrossRef PubMed.
- E. Bremond and C. Adamo, J. Chem. Phys., 2011, 135, 024106 CrossRef PubMed.
- E. Bremond, J. C. Sancho-Garcia, A. J. Perez-Jimenez and C. Adamo, J. Chem. Phys., 2014, 141, 031101 CrossRef PubMed.
- E. Bremond, M. Savarese, A. J. Perez-Jimenez, J. Sancho-Garcia and C. Adamo, J. Phys. Chem. Lett., 2015, 6, 3540–3545 CrossRef CAS PubMed.
- P. R. T. Schipper, O. V. Gritsenko, S. J. A. van Gisbergen and E. J. Baerends, J. Chem. Phys., 2000, 112, 1344–1352 CrossRef CAS.
- L. J. Sham and M. Schlüter, Phys. Rev. Lett., 1983, 51, 1888–1891 CrossRef.
- M. E. Casida, Phys. Rev. A: At., Mol., Opt. Phys., 1995, 51, 2005–2013 CrossRef CAS PubMed.
- U. von Barth, N. E. Dahlen, R. van Leeuwen and G. Stefanucci, Phys. Rev. B: Condens. Matter Mater. Phys., 2005, 72, 235109 CrossRef.
- R. van Leeuwen, Phys. Rev. Lett., 1996, 76, 3610–3613 CrossRef CAS PubMed.
- F. Sottile, V. Olevano and L. Reining, Phys. Rev. Lett., 2003, 91, 056402 CrossRef PubMed.
- S. Cavo, J. A. Berger and P. Romaniello, Phys. Rev. B, 2020, 101, 115109 CrossRef CAS.
- H. Zheng, M. Govoni and G. Galli, Phys. Rev. Mater., 2019, 3, 073803 CrossRef CAS.
- J. Cioslowski, M. Schimeczek, G. Liu and V. Stoyanov, J. Chem. Phys., 2000, 113, 9377–9389 CrossRef CAS.
- J. A. Pople, Rev. Mod. Phys., 1999, 71, 1267–1274 CrossRef CAS.
- T. Helgaker, J. Gauss, P. Jørgensen and J. Olsen, J. Chem. Phys., 1997, 106, 6430–6440 CrossRef CAS.
- R. Kouba, C. Ambrosch-Draxl and B. Zangger, Phys. Rev. B: Condens. Matter Mater. Phys., 1999, 60, 9321–9324 CrossRef CAS.
- P. Schwerdtfeger, M. Pernpointner and J. K. Laerdahl, J. Chem. Phys., 1999, 111, 3357–3364 CrossRef CAS.
- R. Bast and P. Schwerdtfeger, J. Chem. Phys., 2003, 119, 5988–5994 CrossRef CAS.
- C. Thierfelder, P. Schwerdtfeger and T. Saue, Phys. Rev. A: At., Mol., Opt. Phys., 2007, 76, 034502 CrossRef.
- N. Mardirossian and M. Head-Gordon, J. Chem. Phys., 2018, 148, 241736 CrossRef PubMed.
- G. I. Csonka, J. P. Perdew, A. Ruzsinszky, P. H. T. Philipsen, S. Lebègue, J. Paier, O. A. Vydrov and J. G. Ángyán, Phys. Rev. B: Condens. Matter Mater. Phys., 2009, 79, 155107 CrossRef.
- P. Haas, F. Tran and P. Blaha, Phys. Rev. B: Condens. Matter Mater. Phys., 2009, 79, 085104 CrossRef.
- K. Lejaeghere, V. V. Speybroeck, G. V. Oost and S. Cottenier, Crit. Rev. Solid State Mater. Sci., 2014, 39, 1–24 CrossRef CAS.
-
P. Fuentealba, Atoms, Molecules and Clusters in electric Fields, ICP, London, 2006, p. 75 Search PubMed.
- M. B. Knickelbein, J. Chem. Phys., 2004, 120, 10450 CrossRef CAS PubMed.
- F. A. Delesma, R. I. Delgado-Venegas, D. R. Salahub, J. M. del Campo, J. N. Pedroza-Montero, P. Calaminici and A. M. Köster, J. Chem. Theory Comput., 2021, 17, 6934–6946 CrossRef CAS PubMed.
- G. U. Gamboa, P. Calaminici, G. Geudtner and A. M. Köster, J. Phys. Chem. A, 2008, 112, 11969–11971 CrossRef CAS PubMed.
- S. Grimme, F. Bohle, A. Hansen, P. Pracht, S. Spicher and M. Stahn, J. Phys. Chem. A, 2021, 125, 4039–4054 CrossRef CAS PubMed.
- F. Herbstein, Acta Crystallogr., Sect. B: Struct. Sci., Cryst. Eng. Mater., 2000, 56, 547–557 CrossRef CAS PubMed.
- J. C. Grossman, J. Chem. Phys., 2002, 117, 1434–1440 CrossRef CAS.
- Y. Zhao and D. G. Truhlar, J. Phys. Chem. A, 2006, 110, 13126–13130 CrossRef CAS PubMed.
- M. Schreiber, M. R. Silva-Junior, S. P. A. Sauer and W. Thiel, J. Chem. Phys., 2008, 128, 134110 CrossRef PubMed.
- D. Jacquemin, V. Wathelet, E. A. Perpete and C. Adamo, J. Chem. Theory Comput., 2009, 5, 2420–2435 CrossRef CAS PubMed.
- S. S. Leang, F. Zahariev and M. S. Gordon, J. Chem. Phys., 2012, 136, 104101 CrossRef PubMed.
- L. Goerigk, J. Moellmann and S. Grimme, Phys. Chem. Chem. Phys., 2009, 11, 4611–4620 RSC.
- P. Loos, A. Scemama and D. Jacquemin, J. Phys. Chem. Lett., 2020, 11, 2374–2383 CrossRef CAS PubMed.
- D. Jacquemin, E. Bremond, I. Ciofini and C. Adamo, J. Phys. Chem. Lett., 2012, 3, 468–471 CrossRef CAS PubMed.
- S. Di Tommaso, D. Bousquet, D. Moulin, F. Baltenneck, P. Riva, H. David, A. Fadli, J. Gomar, I. Ciofini and C. Adamo, J. Comput. Chem., 2017, 38, 998–1004 CrossRef CAS PubMed.
- C. Adamo and D. Jacquemin, Chem. Soc. Rev., 2013, 42, 845–856 RSC.
- V. Barone, G. Ceselin, M. Fusè and N. Tasinato, Front. Chem., 2020, 8, 584203 CrossRef CAS PubMed.
- F. Xie, M. Fusè, S. Hazrah, A. W. Jaeger, V. Barone and Y. Xu, Angew. Chem., Int. Ed., 2020, 59, 22427–22430 CrossRef CAS PubMed.
- V. Barone, J. Lupi, Z. Salta and N. Tasinato, J. Chem. Theory Comput., 2021, 17, 4913–4928 CrossRef CAS PubMed.
- E. Penocchio, M. Piccardo and V. Barone, J. Chem. Theory Comput., 2015, 11, 4689–4707 CrossRef CAS PubMed.
- G. Ceselin, V. Barone and N. Tasinato, J. Chem. Theory Comput., 2021, 17, 7290–7311 CrossRef CAS PubMed.
- J. A. Hansen, M. Ehara and P. Piecuch, J. Phys. Chem. A, 2013, 117, 10416–10427 CrossRef CAS PubMed.
- G. R. Magoon, J. Aguilera-Iparraguirre, W. H. Green, J. J. Lutz, P. Piecuch, H.-W. Wong and O. O. Oluwole, Int. J. Chem. Kinet., 2012, 44, 179–193 CrossRef CAS.
- H. Knöckel, S. Rühmann and E. Tiemann, J. Chem. Phys., 2013, 138, 094303 CrossRef PubMed.
- S. H. Yuwono, I. Magoulas and P. Piecuch, Sci. Adv., 2020, 6, eaay4058 CrossRef CAS PubMed.
- S. H. Yuwono, I. Magoulas, J. Shen and P. Piecuch, Mol. Phys., 2019, 117, 1486–1506 CrossRef CAS.
- X. Xu, W. Zhang, M. Tang and D. G. Truhlar, J. Chem. Theory Comput., 2015, 11, 2036–2052 CrossRef CAS PubMed.
- M. G. Medvedev, I. S. Bushmarinov, J. Sun, J. P. Perdew and K. A. Lyssenko, Science, 2017, 355, 49–52 CrossRef CAS PubMed.
- K. P. Kepp, Science, 2017, 356, 496 CrossRef CAS PubMed.
- M. G. Medvedev, I. S. Bushmarinov, J. Sun, J. P. Perdew and K. A. Lyssenko, Science, 2017, 356, 496 CrossRef CAS PubMed.
- K. P. Kepp, Phys. Chem. Chem. Phys., 2018, 20, 7538–7548 RSC.
- K. R. Brorsen, Y. Yang, M. V. Pak and S. Hammes-Schiffer, J. Phys. Chem. Lett., 2017, 8, 2076–2081 CrossRef CAS PubMed.
- M. Korth, Angew. Chem., Int. Ed., 2017, 56, 5396–5398 CrossRef CAS PubMed.
- P. D. Mezei, G. I. Csonka and M. Kállay, J. Chem. Theory Comput., 2017, 13, 4753–4764 CrossRef CAS PubMed.
- E. Sim, S. Song and K. Burke, J. Phys. Chem. Lett., 2018, 9, 6385–6392 CrossRef CAS PubMed.
- C. Schattenberg, T. M. Maier and M. Kaupp, J. Chem. Theory Comput., 2018, 14, 5653–5672 CrossRef CAS PubMed.
- G. L. Stoychev, A. A. Auer and F. Neese, J. Chem. Theory Comput., 2018, 14, 4756–4771 CrossRef CAS PubMed.
- G. L. Stoychev, A. A. Auer, J. Gauss and F. Neese, J. Chem. Phys., 2021, 154, 164110 CrossRef CAS PubMed.
- C. J. Schattenberg and M. Kaupp, J. Chem. Theory Comput., 2021, 17, 1469–1479 CrossRef CAS PubMed.
- B. Pudasaini and B. G. Janesko, J. Chem. Theory Comput., 2013, 9, 1443–1451 CrossRef CAS PubMed.
- J. San Fabián, S. Omar and J. M. Garca de la Vega, J. Chem. Phys., 2016, 145, 084301 CrossRef PubMed.
- J. Jaramillo, G. E. Scuseria and M. Ernzerhof, J. Chem. Phys., 2003, 118, 1068–1073 CrossRef CAS.
- T. M. Maier, A. V. Arbuznikov and M. Kaupp, Wiley Interdiscip. Rev.: Comput. Mol. Sci., 2019, 9, e1378 Search PubMed.
- O. V. Gritsenko, L. Mentel and E. J. Baerends, J. Chem. Phys., 2016, 144, 204114 CrossRef CAS PubMed.
- O. V. Gritsenko, R. van Leeuwen, E. van Lenthe and E. J. Baerends, Phys. Rev. A: At., Mol., Opt. Phys., 1995, 51, 1944–1954 CrossRef CAS PubMed.
- R. J. Bartlett, J. Chem. Phys., 2019, 151, 160901 CrossRef PubMed.
- N. I. Gidopoulos and N. N. Lathiotakis, Phys. Rev. A: At., Mol., Opt. Phys., 2012, 85, 052508 CrossRef.
- T. J. Callow, B. J. Pearce, T. Pitts, N. N. Lathiotakis, M. J. Hodgson and N. I. Gidopoulos, Faraday Discuss., 2020, 224, 126–144 RSC.
- N. I. Gidopoulos and N. N. Lathiotakis, J. Chem. Phys., 2012, 136, 224109 CrossRef PubMed.
- T. Pitts, N. I. Gidopoulos and N. N. Lathiotakis, Eur. Phys. J. B, 2018, 91, 130 CrossRef.
- L. Kronik and S. Kümmel, Phys. Chem. Chem. Phys., 2020, 22, 16467–16481 RSC.
- T. Koopmans, Physica, 1934, 1, 104–113 CrossRef.
- D. W. Smith and O. W. Day, J. Chem. Phys., 1975, 62, 113–114 CrossRef CAS.
- M. M. Morrell, R. G. Parr and M. Levy, J. Chem. Phys., 1975, 62, 549–554 CrossRef CAS.
- N. Q. Su and X. Xu, J. Phys. Chem. Lett., 2019, 10, 2692–2699 CrossRef CAS PubMed.
- N. Q. Su and X. Xu, Wiley Interdiscip. Rev.: Comput. Mol. Sci., 2016, 6, 721–747 CAS.
- J. Cioslowski, P. Piskorz and G. Liu, J. Chem. Phys., 1997, 107, 6804–6811 CrossRef CAS.
- Y. H. Gu and X. Xu, J. Chem. Phys., 2020, 153, 044109 CrossRef CAS PubMed.
- B. G. Johnson, P. M. W. Gill and J. A. Pople, J. Chem. Phys., 1993, 98, 5612–5626 CrossRef CAS.
- L. Goerigk, A. Hansen, C. Bauer, S. Ehrlich, A. Najibi and S. Grimme, Phys. Chem. Chem. Phys., 2017, 19, 32184–32215 RSC.
- T. Gould, Phys. Chem. Chem. Phys., 2018, 20, 27735–27739 RSC.
- M. Korth and S. Grimme, J. Chem. Theory Comput., 2009, 5, 993–1003 CrossRef CAS PubMed.
- J. P. Perdew and K. Schmidt, AIP Conf. Proc., 2001, 577, 1–20 CrossRef CAS.
- A. Savin and P. Pernot, Z. Anorg. Allg. Chem., 2020, 646, 1042–1045 CrossRef CAS.
- P. Pernot and A. Savin, J. Chem. Phys., 2020, 152, 164108 CrossRef CAS PubMed.
- P. Pernot and A. Savin, J. Chem. Phys., 2020, 152, 164109 CrossRef CAS PubMed.
- P. Pernot and A. Savin, J. Chem. Phys., 2018, 148, 241707 CrossRef PubMed.
-
K. Lejaeghere, Uncertainty Quantification in Multiscale Materials Modeling, Woodhead Publishing, 2020, pp. 41–76 Search PubMed.
- A. J. Thakkar and T. Wu, J. Chem. Phys., 2015, 143, 144302 CrossRef PubMed.
- P. Pernot, B. Huang and A. Savin, Mach. Learn.: Sci. Technol., 2020, 1, 035011 Search PubMed.
- P. Pernot, B. Civalleri, D. Presti and A. Savin, J. Phys. Chem. A, 2015, 119, 5288–5304 CrossRef CAS PubMed.
- S. De Waele, K. Lejaeghere, M. Sluydts and S. Cottenier, Phys. Rev. B, 2016, 94, 235418 CrossRef.
- J. Proppe and M. Reiher, J. Chem. Theory Comput., 2017, 13, 3297–3317 CrossRef CAS PubMed.
- L. A. Curtiss, K. Raghavachari, P. C. Redfern and J. A. Pople, J. Chem. Phys., 2000, 112, 7374–7383 CrossRef CAS.
-
P. W. Atkins, Molecular quantum mechanics, Oxford University Press, New York, NY, New York, 2nd edn, 1983, p. 227 Search PubMed.
- S. M. Valone and M. Levy, Phys. Rev. A: At., Mol., Opt. Phys., 2009, 80, 042501 CrossRef.
- L. V. Slipchenko and A. I. Krylov, J. Chem. Phys., 2002, 117, 4694–4708 CrossRef CAS.
- D. Santamaria-Pérez, D. Daisenberger, J. Ruiz-Fuertes, T. M. no, R. Chulia-Jordan, C. Muehle, M. Jansen, P. Rodriguez-Hernadez, A. Munoj, E. R. Johnson and A. Otero-de-la-Roza, Chem. Sci., 2019, 10, 6467–6475 RSC.
- F. A. Neese, J. Biol. Inorg. Chem., 2006, 11, 702–711 CrossRef CAS PubMed.
- R. J. Bartlett, V. F. Lotrich and I. V. Schweigert, J. Chem. Phys., 2005, 123, 062205 CrossRef PubMed.
- P. M. Kozlowski, M. Kumar, P. Piecuch, W. Li, N. P. Bauman, J. A. Hansen, P. Lodowski and M. Jaworska, J. Chem. Theory Comput., 2012, 8, 1870–1894 CrossRef CAS PubMed.
- K. Kornobis, N. Kumar, P. Lodowski, M. Jaworska, P. Piecuch, J. J. Lutz, B. M. Wong and P. M. Kozlowski, J. Comput. Chem., 2013, 34, 987–1004 CrossRef CAS PubMed.
- C. J. Cramer, M. Włoch, P. Piecuch, C. Puzzarini and L. Gagliardi, J. Phys. Chem. A, 2006, 110, 1991–2004 CrossRef CAS PubMed.
- M. N. Leuenberger and D. Loss, Nature, 2001, 410, 789–793 CrossRef CAS PubMed.
- D. E. Bolster, P. Guetlich, W. E. Hatfield, S. Kremer, E. W. Mueller and K. Wieghardt, Inorg. Chem., 1983, 22, 1725–1729 CrossRef CAS.
- D. A. Pantazis, J. Chem. Theory Comput., 2019, 15, 938–948 CrossRef CAS PubMed.
- P. Sharma, D. G. Truhlar and L. Gagliardi, J. Am. Chem. Soc., 2020, 142, 16644–16650 CrossRef CAS PubMed.
- W. Yang, P. Mori-Sánchez and A. J. Cohen, J. Chem. Phys., 2013, 139, 104114 CrossRef PubMed.
- S. Di Sabatino, J. A. Berger, L. Reining and P. Romaniello, Phys. Rev. B, 2016, 94, 155141 CrossRef.
- G. Trimarchi, Z. Wang and A. Zunger, Phys. Rev. B, 2018, 97, 035107 CrossRef CAS.
- K. J. Harmon, K. Letchworth-Weaver, A. P. Gaiduk, F. Giberti, M. Chan, F. Gygi, P. Fenter and G. Galli, Phys. Rev. Mater., 2020, 4, 113805 CrossRef CAS.
- G. Santra and J. M. L. Martin, J. Chem. Theory Comput., 2021, 17, 1368–1379 CrossRef CAS PubMed.
- M. C. Kim, E. J. Sim and K. Burke, J. Chem. Phys., 2011, 134, 171103 CrossRef PubMed.
- R. van Leeuwen and E. J. Baerends, Phys. Rev. A: At., Mol., Opt. Phys., 1994, 49, 2421–2431 CrossRef CAS PubMed.
- M. Amati and E. J. Baerends, J. Chem. Theory Comput., 2020, 16, 443–452 CrossRef CAS PubMed.
- N. Q. Su and X. Xu, Int. J. Quantum Chem., 2015, 115, 589–595 CrossRef CAS.
- P. Verma, A. Perera and R. J. Bartlett, Chem. Phys. Lett., 2012, 524, 10–15 CrossRef CAS.
- B. G. Janesko and G. E. Scuseria, J. Chem. Phys., 2008, 128, 244112 CrossRef PubMed.
- M.-C. Kim, E. Sim and K. Burke, J. Chem. Phys., 2014, 140, 18A528 CrossRef PubMed.
- N. Q. Su, Z. Y. Zhu and X. Xu, Proc. Natl. Acad. Sci. U. S. A., 2018, 115, 2287–2292 CrossRef CAS PubMed.
- Y. H. Gu and X. Xu, J. Chem. Theory Comput., 2021, 17, 4860–4871 CrossRef CAS PubMed.
- S. Kristyán and P. Pulay, Chem. Phys. Lett., 1994, 229, 175–180 CrossRef.
- J. Pérez-Jordá and A. Becke, Chem. Phys. Lett., 1995, 233, 134–137 CrossRef.
- P. Hobza, J. Sponer and T. Reschel, J. Comput. Chem., 1995, 16, 1315–1325 CrossRef CAS.
- E. J. Meijer and M. Sprik, J. Chem. Phys., 1996, 105, 8684–8689 CrossRef CAS.
- T. Ziegler, Chem. Rev., 1991, 91, 651–667 CrossRef CAS.
- T. van Mourik and R. J. Gdanitz, J. Chem. Phys., 2002, 116, 9620–9623 CrossRef CAS.
- S. Grimme, A. Hansen, J. G. Brandenburg and C. Bannwarth, Chem. Rev., 2016, 116, 5105–5154 CrossRef CAS PubMed.
- J. P. Wagner and P. R. Schreiner, Angew. Chem., Int. Ed., 2015, 54, 12274–12296 CrossRef CAS PubMed.
- T. Helgaker, Chem. Phys. Lett., 1991, 182, 503–510 CrossRef CAS.
- S. Song, S. Vuckovic, E. Sim and K. Burke, J. Phys. Chem. Lett., 2021, 12, 800–807 CrossRef CAS PubMed.
- R. P. Feynman, Phys. Rev., 1939, 56, 340–343 CrossRef CAS.
- M. J. Allen and D. J. Tozer, J. Chem. Phys., 2002, 117, 11113–11120 CrossRef CAS.
- A. D. Dwyer and D. J. Tozer, J. Chem. Phys., 2011, 135, 164110 CrossRef PubMed.
- D. P. Kooi and P. Gori-Giorgi, J. Phys. Chem. Lett., 2019, 10, 1537–1541 CrossRef CAS PubMed.
- K. L. C. Hunt, J. Chem. Phys., 1990, 92, 1180–1187 CrossRef CAS.
- E. Steiner, J. Chem. Phys., 1973, 59, 2427–2429 CrossRef CAS.
- D. P. Kooi and P. Gori-Giorgi, Faraday Discuss., 2020, 224, 145–165 RSC.
- M. Dion, H. Rydberg, E. Schröder, D. C. Langreth and B. I. Lundqvist, Phys. Rev. Lett., 2004, 92, 246401 CrossRef CAS PubMed.
-
J. G. Ángyán, J. F. Dobson, G. Jansen and T. Gould, London Dispersion Forces in Molecules, Solids and Nanostructures, The Royal Society of Chemistry, Cambridge, 2020 Search PubMed.
- O. A. Vydrov and T. Van Voorhis, Phys. Rev. Lett., 2009, 103, 063004 CrossRef PubMed.
- D. B. Chang, R. L. Cooper, J. E. Drummond and A. C. Young, Phys. Lett. A, 1971, 174, 311–312 CrossRef.
- M. Boström and B. E. Sernelius, Phys. Rev. B: Condens. Matter Mater. Phys., 2000, 61, 2204 CrossRef.
- J. F. Dobson, A. White and A. Rubio, Phys. Rev. Lett., 2006, 96, 073201 CrossRef PubMed.
- J. F. Dobson, Electron. Struct., 2021, 3, 044001 CrossRef CAS.
- J. P. Perdew, A. Ruzsinszky, J. Sun, N. K. Nepal and A. D. Kaplan, Proc. Natl. Acad. Sci. U. S. A., 2021, 118, e2017850118 CrossRef CAS PubMed.
- J. P. Perdew, A. Savin and K. Burke, Phys. Rev. A: At., Mol., Opt. Phys., 1995, 51, 4531–4541 CrossRef CAS PubMed.
- P. W. Anderson, Science, 1972, 177, 393–396 CrossRef CAS PubMed.
- A. D. Becke, J. Chem. Phys., 2002, 117, 6935–6938 CrossRef CAS.
- D. J. Carrascal, J. Ferrer, J. C. Smith and K. Burke, J. Phys.: Condens. Matter, 2015, 27, 393001 CrossRef CAS PubMed.
- H. Chen, G. Friesecke and C. B. Mendl, J. Chem. Theory Comput., 2014, 10, 4360–4368 CrossRef CAS PubMed.
- C. B. Mendl, F. Malet and P. Gori-Giorgi, Phys. Rev. B: Condens. Matter Mater. Phys., 2014, 89, 125106 CrossRef.
- A. Görling, Phys. Rev. A: At., Mol., Opt. Phys., 1993, 47, 2783–2799 CrossRef PubMed.
- E. Trushin and A. Görling, Phys. Rev. B, 2018, 98, 205137 CrossRef CAS.
-
M. Atanasov, C. A. Daul and C. Rauzy, in A DFT Based Ligand Field Theory, ed. D. M. P. Mingos and T. Schönherr, Springer, Berlin, Heidelberg, Berlin, Heidelberg, 2004, pp. 97–125 Search PubMed.
- H. Ramanantoanina, W. Urland, F. Cimpoesu and C. Daul, Phys. Chem. Chem. Phys., 2013, 15, 13902–13910 RSC.
- H. Ramanantoanina, L. Merzoud, J. T. Muya, H. Chermette and C. Daul, J. Phys. Chem. A, 2020, 124, 152–164 CrossRef CAS PubMed.
- J. Pople, P. Gill and N. Handy, Int. J. Quantum Chem., 1995, 56, 303–305 CrossRef CAS.
-
A. I. Krylov, Reviews in Computational Chemistry, John Wiley & Sons, Ltd, 2017, vol. 30, pp. 151–224 Search PubMed.
- E. J. Baerends, Phys. Rev. Lett., 2001, 87, 133004 CrossRef CAS PubMed.
- D. R. Rohr, K. Pernal, O. V. Gritsenko and E. J. Baerends, J. Chem. Phys., 2008, 129, 164105 CrossRef PubMed.
- J. D. Chai, J. Chem. Phys., 2012, 136, 154104 CrossRef PubMed.
-
J. P. Perdew, Density Functional Methods in Physics, Plenum, New York, 1985, pp. 265–308 Search PubMed.
- S. M. Valone, J. Chem. Phys., 1980, 73, 4653–4655 CrossRef CAS.
- E. K. U. Gross, L. N. Oliveira and W. Kohn, Phys. Rev. A: At., Mol., Opt. Phys., 1988, 37, 2805–2808 CrossRef PubMed.
- E. K. U. Gross, L. N. Oliveira and W. Kohn, Phys. Rev. A: At., Mol., Opt. Phys., 1988, 37, 2809–2820 CrossRef CAS PubMed.
- B. Senjean and E. Fromager, Phys. Rev. A, 2018, 98, 022513 CrossRef CAS.
- T. Gould and S. Pittalis, Aust. J. Chem., 2020, 73, 714–723 CrossRef CAS.
- E. Kraisler and L. Kronik, Phys. Rev. Lett., 2013, 110, 126403 CrossRef PubMed.
- E. Kraisler and L. Kronik, J. Chem. Phys., 2014, 140, 18A540 CrossRef PubMed.
- T. Gould and S. Pittalis, Phys. Rev. Lett., 2017, 119, 243001 CrossRef PubMed.
- T. Gould, G. Stefanucci and S. Pittalis, Phys. Rev. Lett., 2020, 125, 233001 CrossRef CAS PubMed.
- T. Gould and S. Pittalis, Phys. Rev. Lett., 2019, 123, 016401 CrossRef CAS PubMed.
- E. Fromager, Phys. Rev. Lett., 2020, 124, 243001 CrossRef CAS PubMed.
- S. Pittalis, C. R. Proetto, A. Floris, A. Sanna, C. Bersier, K. Burke and E. K. U. Gross, Phys. Rev. Lett., 2011, 107, 163001 CrossRef CAS PubMed.
- A. Pribram-Jones and K. Burke, Phys. Rev. B, 2016, 93, 205140 CrossRef.
- O. Franck and E. Fromager, Mol. Phys., 2014, 112, 1684–1701 CrossRef CAS.
- B. Senjean and E. Fromager, Int. J. Quantum Chem., 2020, e26190 CAS.
- K. Deur and E. Fromager, J. Chem. Phys., 2019, 150, 094106 CrossRef PubMed.
- M. Levy, Phys. Rev. A: At., Mol., Opt. Phys., 1995, 52, R4313–R4315 CrossRef CAS PubMed.
- M. J. P. Hodgson, J. Wetherell and E. Fromager, Phys. Rev. A, 2021, 103, 012806 CrossRef CAS.
- F. Cernatic, B. Senjean, V. Robert and E. Fromager, Top. Curr. Chem., 2022, 380, 4 CrossRef CAS PubMed.
- E. Pastorczak, N. I. Gidopoulos and K. Pernal, Phys. Rev. A: At., Mol., Opt. Phys., 2013, 87, 062501 CrossRef.
- P.-F. Loos and E. Fromager, J. Chem. Phys., 2020, 152, 214101 CrossRef CAS PubMed.
- C. Marut, B. Senjean, E. Fromager and P.-F. Loos, Faraday Discuss., 2020, 224, 402–423 RSC.
- T. Gould, J. Phys. Chem. Lett., 2020, 11, 9907–9912 CrossRef CAS PubMed.
- T. Gould, L. Kronik and S. Pittalis, Phys. Rev. A, 2021, 104, 022803 CrossRef CAS.
- N. I. Gidopoulos, P. G. Papaconstantinou and E. K. U. Gross, Phys. Rev. Lett., 2002, 88, 033003 CrossRef CAS PubMed.
-
T. Helgaker, P. Jorgensen and J. Olsen, Molecular electronic-structure theory, John Wiley & Sons, 2014 Search PubMed.
- Z.-h Yang, A. Pribram-Jones, K. Burke and C. A. Ullrich, Phys. Rev. Lett., 2017, 119, 033003 CrossRef PubMed.
- M. Filatov, Wiley Interdiscip. Rev.: Comput. Mol. Sci., 2015, 5, 146–167 CAS.
- M. Filatov, S. Lee and C. H. Choi, J. Chem. Theory Comput., 2021, 17, 5123–5139 CrossRef CAS PubMed.
- A. Görling and M. Levy, Int. J. Quantum Chem., 1995, 56, 93–108 CrossRef.
- S. Ivanov and M. Levy, J. Chem. Phys., 2002, 116, 6924–6929 CrossRef CAS.
- S. Grimme, Angew. Chem., Int. Ed., 2013, 52, 6306–6312 CrossRef CAS PubMed.
- S. Grimme and A. Hansen, Angew. Chem., Int. Ed., 2015, 54, 12308–12313 CrossRef CAS PubMed.
- W. Lamine, S. Boughdiri, L. Christ, L. Merzoud, C. Morell and H. Chermette, Theor. Chem. Acc., 2019, 139, 7 Search PubMed.
- J. Gao, A. Grofe, H. Ren and P. Bao, J. Phys. Chem. Lett., 2016, 7, 5143–5149 Search PubMed.
- A. Cembran, L. Song, Y. Mo and J. Gao, J. Chem. Theory Comput., 2009, 5, 2702–2716 CrossRef CAS PubMed.
- W. L. Chan, T. C. Berkelbach, M. R. Provorse, N. R. Monahan, J. R. Tritsch, M. S. Hybertsen, D. R. Reichman, J. Gao and X. Y. Zhu, Acc. Chem. Res., 2013, 46, 1321–1329 CrossRef CAS PubMed.
- A. Grofe, X. Chen, W. Liu and J. Gao, J. Phys. Chem. Lett., 2017, 8, 4838–4845 CrossRef CAS PubMed.
- R. Zhao, A. Grofe, Z. Wang, P. Bao, X. Chen, W. Liu and J. Gao, J. Phys. Chem. Lett., 2021, 12, 7409–7417 CrossRef CAS PubMed.
- Y. Lu and J. Gao, J. Phys. Chem. Lett., 2022, 13, 7762–7769 CrossRef CAS PubMed.
- A. K. Theophilou, J. Phys. C: Solid State Phys., 1979, 12, 5419–5430 CrossRef CAS.
- P. Bao, C. P. Hettich, Q. Shi and J. Gao, J. Chem. Theory Comput., 2021, 17, 240–254 CrossRef CAS PubMed.
- A. Grofe, R. Zhao, A. Wildman, T. F. Stetina, X. Li, P. Bao and J. Gao, J. Chem. Theory Comput., 2021, 17, 277–289 CrossRef CAS PubMed.
- Y. Mo and J. Gao, J. Phys. Chem. A, 2000, 104, 3012–3020 CrossRef CAS.
- D. R. Salahub, Theor. Chem. Acc., 2000, 103, 311–312 Search PubMed.
- G. Gabrielse, D. Hanneke, T. Kinoshita, M. Nio and B. Odom, Phys. Rev. Lett., 2006, 97, 030802 CrossRef CAS PubMed.
-
P. Fulde, Electron correlations in molecules and solids, Springer Science & Business Media, Berlin, 1995, vol. 100 Search PubMed.
- V. I. Anisimov, J. Zaanen and O. K. Andersen, Phys. Rev. B: Condens. Matter Mater. Phys., 1991, 44, 943–954 CrossRef CAS PubMed.
- V. I. Anisimov, F. Aryasetiawan and A. I. Lichtenstein, J. Phys.: Condens. Matter, 1997, 9, 767–808 CrossRef CAS.
- E. J. Baerends and P. Ros, Chem. Phys. Lett., 1973, 23, 391–393 CrossRef CAS.
- H. Chermette, P. Pertosa and F. M. Michel-Calendini, Chem. Phys. Lett., 1980, 69, 240–245 CrossRef CAS.
- M. A. Buijse and E. J. Baerends, Mol. Phys., 2002, 100, 401–421 CrossRef CAS.
-
D. Shechtman, Nobel lecture, 2012, https://www.youtube.com/watch?v=ga71v4nofVM.
- A. D. Becke and E. R. Johnson, J. Chem. Phys., 2006, 124, 221101 CrossRef PubMed.
- F. Tran, S. Ehsan and P. Blaha, Phys. Rev. Mater., 2018, 2, 023802 CrossRef CAS.
- P. Geerlings, E. Chamorro, P. K. Chattaraj, F. D. Proft, J. L. Gázquez, S. Liu, C. Morell, A. Toro-Labbé, A. Vela and P. W. Ayers, Theor. Chem. Acc., 2020, 139, 36 Search PubMed.
- E. J. Baerends, Mol. Phys., 2020, 118, e1612955 CrossRef.
- R. G. Parr and L. J. Bartolotti, J. Phys. Chem., 1983, 87, 2810–2815 CrossRef CAS.
- E. J. Baerends, Phys. Chem. Chem. Phys., 2022, 6430–6440, 10.1039/d2cp01585d.
- C. Rong, D. Zhao, T. Zhou, S. Liu, D. Yu and S. Liu, J. Phys. Chem. Lett., 2019, 10, 1716–1721 CrossRef CAS PubMed.
- S. Liu and C. Rong, J. Phys. Chem. A, 2021, 125, 4910–4917 CrossRef CAS PubMed.
- S. Liu, J. Phys. Chem. Lett., 2020, 11, 8690–8696 CrossRef CAS PubMed.
- F. Guégan, T. Pigeon, F. De Proft, V. Tognetti, L. Joubert, H. Chermette, P. W. Ayers, D. Luneau and C. Morell, J. Phys. Chem. A, 2020, 124, 633–641 CrossRef PubMed.
- S. Menant, F. Guégan, V. Tognetti, L. Merzoud, H. Chermette and M. Christophe, Molecules, 2021, 26, 6218 CrossRef CAS PubMed.
- F. Guégan, V. Tognetti, J. I. Martínez-Araya, H. Chermette, L. Merzoud, A. Toro-Labbé and C. Morell, Phys. Chem. Chem. Phys., 2020, 22, 23553–23562 RSC.
-
R. Carbó-Dorca, E. Besalú and X. Gironés, Advances in Quantum Chemistry, Academic Press, New York, 2000, pp. 1–63 Search PubMed.
-
R. F. W. Bader, Atoms in molecules: A quantum theory, Clarendon, 1990 Search PubMed.
-
P. L. A. Popelier, Atoms in Molecules: An Introduction, Pearson, 2000 Search PubMed.
- O. V. Gritsenko, R. van Meer and K. Pernal, Phys. Rev. A, 2018, 98, 062510 CrossRef CAS.
- M. Hapka, K. Pernal and O. V. Gritsenko, J. Phys. Chem. Lett., 2020, 11, 5883–5889 CrossRef CAS PubMed.
- G. L. Manni, R. K. Carlson, S. Luo, D. Ma, J. Olsen, D. G. Truhlar and L. Gagliardi, J. Chem. Theory Comput., 2014, 10, 3669–3680 CrossRef PubMed.
- L. Gagliardi, D. G. Truhlar, G. L. Manni, R. K. Carlson, C. E. Hoyer and J. L. Bao, Acc. Chem. Res., 2016, 50, 66–73 CrossRef PubMed.
- D. S. King, D. G. Truhlar and L. Gagliardi, J. Phys. Chem. Lett., 2021, 12, 7761–7767 CrossRef CAS PubMed.
- R. K. Carlson, D. G. Truhlar and L. Gagliardi, J. Chem. Theory Comput., 2015, 11, 4077–4085 CrossRef CAS PubMed.
- C. E. Hoyer, S. Ghosh, D. G. Truhlar and L. Gagliardi, J. Phys. Chem. Lett., 2016, 7, 586–591 CrossRef CAS PubMed.
- T. R. Scott, M. R. Hermes, A. M. Sand, M. S. Oakley, D. G. Truhlar and L. Gagliardi, J. Chem. Phys., 2020, 153, 014106 CrossRef CAS PubMed.
- A. M. Sand, C. E. Hoyer, K. Sharkas, K. M. Kidder, R. Lindh, D. G. Truhlar and L. Gagliardi, J. Chem. Theory Comput., 2017, 14, 126–138 CrossRef PubMed.
- P. Sharma, V. Bernales, S. Knecht, D. G. Truhlar and L. Gagliardi, Chem. Sci., 2019, 10, 1716–1723 RSC.
- E. D. Hedegård, J. Toulouse and H. J. Aa Jensen, J. Chem. Phys., 2018, 148, 214103 CrossRef PubMed.
- E. Fromager, J. Toulouse and H. J. Aa Jensen, J. Chem. Phys., 2007, 126, 074111 CrossRef PubMed.
- M. Hubert, E. D. Hedegård and H. J. Aa Jensen, J. Chem. Theory Comput., 2016, 12, 2203–2213 CrossRef CAS PubMed.
- E. R. Kjellgren, E. D. Hedegård and H. J. Aa Jensen, J. Chem. Phys., 2019, 151, 124113 CrossRef PubMed.
- E. R. Kjellgren and H. J. Aa Jensen, J. Chem. Phys., 2021, 155, 084102 CrossRef CAS PubMed.
- E. Giner, B. Pradines, A. Ferté, R. Assaraf, A. Savin and J. Toulouse, J. Chem. Phys., 2018, 149, 194301 CrossRef PubMed.
- T. Leininger, H. Stoll, H. J. Werner and A. Savin, Chem. Phys. Lett., 1997, 275, 151–160 CrossRef CAS.
- L. Kronik and S. Kümmel, Adv. Mater., 2018, 30, 1706560 CrossRef PubMed.
- T. Stein, H. Eisenberg, L. Kronik and R. Baer, Phys. Rev. Lett., 2010, 105, 266802 CrossRef PubMed.
- D. Wing, G. Ohad, J. B. Haber, M. R. Filip, S. E. Gant, J. B. Neaton and L. Kronik, Proc. Natl. Acad. Sci. U. S. A., 2021, 118, e2104556118 CrossRef CAS PubMed.
- T. Stein, L. Kronik and R. Baer, J. Am. Chem. Soc., 2009, 131, 2818–2820 CrossRef CAS PubMed.
- Z. Zheng, D. A. Egger, J.-L. Brédas, L. Kronik and V. Coropceanu, J. Phys. Chem. Lett., 2017, 8, 3277–3283 CrossRef CAS PubMed.
- O. A. Vydrov, G. E. Scuseria and J. P. Perdew, J. Chem. Phys., 2007, 126, 154109 CrossRef PubMed.
- M.-C. Kim, E. Sim and K. Burke, Phys. Rev. Lett., 2013, 111, 073003 CrossRef PubMed.
- J. Perdew, W. Yang, Z. Yang, E. Gross, M. Scheffler, G. Scuseria, T. Henderson, Y. Zhang, A. Ruzsinszky, H. Peng, J. Sun, E. Trushin and A. Goerling, Proc. Natl. Acad. Sci. U. S. A., 2017, 114, 2801–2806 CrossRef CAS PubMed.
- T. Koerzdoerfer, J. S. Sears, C. Sutton and J. L. Bredas, J. Chem. Phys., 2011, 135, 204107 CrossRef PubMed.
- S. R. Whittleton, X. A. S. Vazquez, C. M. Isborn and E. R. Johnson, J. Chem. Phys., 2015, 142, 184106 CrossRef PubMed.
- E. Trushin, A. Thierbach and A. Görling, J. Chem. Phys., 2021, 154, 014104 CrossRef CAS PubMed.
- S. Fauser, E. Trushin, C. Neiss and A. Görling, J. Chem. Phys., 2021, 155, 134111 CrossRef CAS PubMed.
- I. Y. Zhang and X. Xu, J. Phys. Chem. Lett., 2021, 12, 2638–2644 CrossRef CAS PubMed.
- B. Chan, L. Goerigk and L. Radom, J. Comput. Chem., 2016, 37, 183–193 CrossRef CAS PubMed.
- I. Y. Zhang, P. Rinke and M. Scheffler, New J. Phys., 2016, 18, 073026 CrossRef.
- I. Y. Zhang, P. Rinke, J. P. Perdew and M. Scheffler, Phys. Rev. Lett., 2016, 117, 133002 CrossRef PubMed.
- I. Y. Zhang and X. Xu, J. Phys. Chem. Lett., 2019, 10, 2617–2623 CrossRef CAS PubMed.
- I. Y. Zhang and X. Xu, Wiley Interdiscip. Rev.: Comput. Mol. Sci., 2021, 11, e1490 Search PubMed.
- M. Haasler, T. M. Maier, R. Grotjahn, S. Gückel, A. V. Arbuznikov and M. Kaupp, J. Chem. Theory Comput., 2020, 16, 5645–5657 CrossRef CAS PubMed.
- F. G. Cruz, K.-C. Lam and K. Burke, J. Phys. Chem. A, 1998, 102, 4911–4917 CrossRef CAS.
- J. Tao, V. N. Staroverov, G. E. Scuseria and J. P. Perdew, Phys. Rev. A: At., Mol., Opt. Phys., 2008, 77, 012509 CrossRef.
- A. V. Arbuznikov and M. Kaupp, J. Chem. Phys., 2014, 141, 204101 CrossRef PubMed.
- T. M. Maier, M. Haasler, A. V. Arbuznikov and M. Kaupp, Phys. Chem. Chem. Phys., 2016, 18, 21133–21144 RSC.
- F. Mack, C. Schattenberg, M. Kaupp and F. Weigend, J. Phys. Chem. A, 2020, 124, 8529–8539 CrossRef CAS PubMed.
- C. J. Schattenberg and M. Kaupp, J. Phys. Chem. A, 2021, 125, 2697–2707 CrossRef CAS PubMed.
- T. M. Maier, H. Bahmann, A. V. Arbuznikov and M. Kaupp, J. Chem. Phys., 2016, 144, 074106 CrossRef PubMed.
- R. Grotjahn and M. Kaupp, J. Phys. Chem. A, 2021, 125, 7099–7110 CrossRef CAS PubMed.
- A. V. Krukau, G. E. Scuseria, J. P. Perdew and A. Savin, J. Chem. Phys., 2008, 129, 124103 CrossRef PubMed.
- S. Klawohn and H. Bahmann, J. Chem. Theory Comput., 2020, 16, 953–963 CrossRef CAS PubMed.
- T. M. Maier, Y. Ikabata and H. Nakai, J. Chem. Phys., 2021, 154, 214101 CrossRef CAS PubMed.
- B. G. Janesko, A. V. Krukau and G. E. Scuseria, J. Chem. Phys., 2008, 129, 124110 CrossRef PubMed.
- A. V. Arbuznikov and M. Kaupp, J. Chem. Phys., 2012, 136, 014111 CrossRef PubMed.
- L. Curtiss, P. Redfern and K. Raghavachari, Wiley Interdiscip. Rev.: Comput. Mol. Sci., 2011, 1, 810–825 CAS.
- Y. Zhao and D. G. Truhlar, Theor. Chem. Acc., 2008, 120, 215–241 Search PubMed.
- T. Weymuth, E. P. A. Couzijn, P. Chen and M. Reiher, J. Chem. Theory Comput., 2014, 10, 3092–3103 CrossRef CAS PubMed.
- S. Dohm, A. Hansen, M. Steinmetz, S. Grimme and M. P. Checinski, J. Chem. Theory Comput., 2018, 14, 2596–2608 CrossRef CAS PubMed.
- C. Zhe-Ning, S. Tonghao, W. Yizhen and Z. I. Ying, CCS Chem., 2021, 3, 136–143 CrossRef.
- D. Mejía-Rodríguez and S. B. Trickey, Phys. Rev. A, 2017, 96, 052512 CrossRef.
- D. Meja-Rodrguez and S. B. Trickey, Phys. Rev. B, 2018, 98, 115161 CrossRef.
- P. Tran and F. Blaha, Phys. Rev. Lett., 2009, 102, 226401 CrossRef PubMed.
- S. Aschebrock and T. Kuemmel, Phys. Rev. Res., 2019, 1, 033082 CrossRef.
- B. Neupane, H. Tang, N. Nepal, S. Adhikari and A. Ruzsinszky, Phys. Rev. Mater., 2021, 5, 063803 CrossRef CAS.
- D. Chakraborty, S. Kar and P. K. Chattaraj, Phys. Chem. Chem. Phys., 2015, 17, 31516–31529 RSC.
- M. Levy, J. Mol. Model., 2018, 24, 311 CrossRef PubMed.
- W. C. Witt, B. G. del Rio, J. M. Dieterich and E. A. Carter, J. Mater. Res., 2018, 33, 777–795 CrossRef CAS.
- B. G. del Rio, M. Chen, L. E. González and E. A. Carter, J. Chem. Phys., 2018, 149, 094504 CrossRef PubMed.
- M. Chen, J. Xia, C. Huang, J. M. Dieterich, L. Hung, I. Shin and E. A. Carter, Comput. Phys. Commun., 2015, 190, 228–230 CrossRef CAS.
- J. M. Dieterich, W. C. Witt and E. A. Carter, J. Comput. Chem., 2017, 38, 1552–1559 CrossRef CAS PubMed.
- W. C. Witt and E. A. Carter, Phys. Rev. B, 2019, 100, 125106 CrossRef CAS.
- W. C. Witt and E. A. Carter, Phys. Rev. B, 2019, 100, 125107 CrossRef CAS.
- J. Xia, C. Huang, I. Shin and E. A. Carter, J. Chem. Phys., 2012, 136, 084102 CrossRef PubMed.
- J. Xia and E. A. Carter, Phys. Rev. B: Condens. Matter Mater. Phys., 2012, 86, 235109 CrossRef.
- B. Zhou and E. A. Carter, J. Chem. Phys., 2005, 122, 184108 CrossRef PubMed.
- C. Huang and E. A. Carter, Phys. Rev. B: Condens. Matter Mater. Phys., 2012, 85, 045126 CrossRef.
- Y. Ke, F. Libisch, J. Xia and E. A. Carter, Phys. Rev. B: Condens. Matter Mater. Phys., 2014, 89, 155112 CrossRef.
- K. Luo, V. V. Karasiev and S. B. Trickey, Phys. Rev. B, 2018, 98, 041111 CrossRef CAS.
- V. V. Karasiev, D. Chakraborty, O. A. Shukruto and S. B. Trickey, Phys. Rev. B: Condens. Matter Mater. Phys., 2013, 88, 161108 CrossRef.
- P. W. Ayers and S. Liu, Phys. Rev. A: At., Mol., Opt. Phys., 2007, 75, 022514 CrossRef.
- D. Mejía-Rodríguez and S. B. Trickey, Phys. Rev. B, 2020, 102, 121109R CrossRef.
- J. Xia and E. A. Carter, Phys. Rev. B: Condens. Matter Mater. Phys., 2015, 92, 117102 CrossRef.
- J. Xia and E. A. Carter, Phys. Rev. B: Condens. Matter Mater. Phys., 2015, 91, 045124 CrossRef.
- Y. A. Wang, N. Govind and E. A. Carter, Phys. Rev. B: Condens. Matter Mater. Phys., 1999, 60, 16350–16358 CrossRef CAS.
- Y. A. Wang, N. Govind and E. A. Carter, Phys. Rev. B: Condens. Matter Mater. Phys., 2001, 64, 089903 CrossRef.
-
W. C. Witt, PhD thesis, Quantum Mechanics Without Wave Functions: Advancing Orbital-Free Methods for Materials Research, Princeton University, ProQuest Dissertations Publishing, https://www.proquest.com/openview/c1fc6f14650438aeeabb45bada186676/1?pq-origsite=gscholarcbl=18750diss=y (accessed on 26/08/2021), 2019 Search PubMed.
- S. Liu, D. Zhao, C. Rong, T. Lu and S. Liu, J. Chem. Phys., 2019, 150, 204106 CrossRef PubMed.
- M. S. Ryley, M. Withnall, T. J. P. Irons, T. Helgaker and A. M. Teale, J. Phys. Chem. A, 2020, 125, 459–475 CrossRef PubMed.
- J. Tao, X. Gao, G. Vignale and I. Tokatly, Phys. Rev. Lett., 2009, 103, 086401 CrossRef PubMed.
- X. Gao, J. Tao, G. Vignale and I. Tokatly, Phys. Rev. B: Condens. Matter Mater. Phys., 2010, 81, 195106 CrossRef.
- T. Gould, G. Jansen, I. Tokatly and J. Dobson, J. Chem. Phys., 2012, 136, 204115 CrossRef PubMed.
-
S. D. Palo, P. E. Trevisanutto, G. Senatore and G. Vignale, 2021, arXiv.2107.12063.
- A. Ramasubramaniam and E. A. Carter, MRS Bull., 2007, 32, 913–918 CrossRef CAS.
-
N. Sheng, C. Vorwerk, M. Govoni, B. Huang and G. Galli, 2021, arXiv.2105.04736.
- T. A. Wesołowski, Phys. Rev. A: At., Mol., Opt. Phys., 2008, 77, 012504 CrossRef.
- T. A. Wesolowski, J. Chem. Theory Comput., 2020, 16, 6880–6885 CrossRef CAS PubMed.
- T. A. Wesolowski and A. Warshel, J. Phys. Chem., 1993, 97, 8050–8053 CrossRef CAS.
- J. W. Kaminski, S. Gusarov, T. A. Wesolowski and A. Kovalenko, J. Phys. Chem. A, 2010, 114, 6082–6096 CrossRef CAS PubMed.
- N. Ricardi, M. Ernst, P. Macchi and T. A. Wesolowski, Acta Crystallogr., Sect. A: Found. Adv., 2020, 76, 571–579 CrossRef CAS PubMed.
- T. Wesolowski and A. Warshel, J. Phys. Chem., 1994, 98, 5183–5187 CrossRef CAS.
- M. Zbiri, M. Atanasov, C. Daul, J. M. Garcia-Lastra and T. A. Wesolowski, Chem. Phys. Lett., 2004, 397, 441–446 CrossRef CAS.
- P. Cortona, Phys. Rev. B: Condens. Matter Mater. Phys., 1991, 44, 8454–8458 CrossRef PubMed.
- N. Govind, Y. Wang, A. da Silva and E. Carter, Chem. Phys. Lett., 1998, 295, 129–134 CrossRef CAS.
- N. Govind, Y. A. Wang and E. A. Carter, J. Chem. Phys., 1999, 110, 7677–7688 CrossRef CAS.
- P. Huang and E. A. Carter, Annu. Rev. Phys. Chem., 2008, 59, 261–290 CrossRef CAS PubMed.
- C. Huang, M. Pavone and E. A. Carter, J. Chem. Phys., 2011, 134, 154110 CrossRef PubMed.
- F. Libisch, C. Huang and E. A. Carter, Acc. Chem. Res., 2014, 47, 2768–2775 CrossRef CAS PubMed.
-
K. Yu, C. M. Krauter, J. M. Dieterich and E. A. Carter, Density and Potential Functional Embedding: Theory and Practice, John Wiley & Sons, Ltd, 2017, ch. 2, pp. 81–117 Search PubMed.
- K. Yu, F. Libisch and E. A. Carter, J. Chem. Phys., 2015, 143, 102806 CrossRef PubMed.
- C. Huang and E. A. Carter, J. Chem. Phys., 2011, 135, 194104 CrossRef PubMed.
- J. Cheng, F. Libisch, K. Yu, M. Chen, J. M. Dieterich and E. A. Carter, J. Chem. Theory Comput., 2017, 13, 1067–1080 CrossRef CAS PubMed.
- J. Cheng, K. Yu, F. Libisch, J. M. Dieterich and E. A. Carter, J. Chem. Theory Comput., 2017, 13, 1081–1093 CrossRef CAS PubMed.
- Q. Ou and E. A. Carter, J. Chem. Theory Comput., 2018, 14, 5680–5689 CrossRef CAS PubMed.
- F. Libisch, C. Huang, P. Liao, M. Pavone and E. A. Carter, Phys. Rev. Lett., 2012, 109, 198303 CrossRef PubMed.
- J. M. P. Martirez, J. L. Bao and E. A. Carter, Annu. Rev. Phys. Chem., 2021, 72, 99–119 CrossRef CAS PubMed.
-
Carter group codes, https://github.com/EACcodes, accessed: 2022-01-12.
- X. Zhang and E. A. Carter, J. Chem. Theory Comput., 2018, 15, 949–960 CrossRef PubMed.
- K. Yu and E. A. Carter, Proc. Natl. Acad. Sci. U. S. A., 2017, 114, E10861–E10870 CAS.
- Q. Zhao and E. A. Carter, J. Chem. Theory Comput., 2020, 16, 6528–6538 CrossRef CAS PubMed.
- Q. Zhao, J. M. P. Martirez and E. A. Carter, J. Am. Chem. Soc., 2021, 143, 6152–6164 CrossRef CAS PubMed.
- J. M. P. Martirez and E. A. Carter, J.
Chem. Theory Comput., 2021, 17, 4105–4121 CrossRef CAS PubMed.
- J. M. P. Martirez and E. A. Carter, J. Phys. Chem. A, 2021, 125, 4998–5013 CrossRef CAS PubMed.
- T. A. Wesolowski and F. Tran, J. Chem. Phys., 2003, 118, 2072–2080 CrossRef CAS.
- M. Dułak and T. A. Wesołowski, J. Mol. Model., 2007, 13, 631–642 CrossRef PubMed.
- M. Dułak, J. W. Kamiński and T. A. Wesołowski, J. Chem. Theory Comput., 2007, 3, 735–745 CrossRef PubMed.
- G. Fradelos, J. J. Lutz, T. A. Wesołowski, P. Piecuch and M. Włoch, J. Chem. Theory Comput., 2011, 7, 1647–1666 CrossRef CAS.
-
M. S. Gordon, L. Slipchenko, H. Li and J. H. Jensen, Annual Reports in Computational Chemistry, Elsevier, 2007, vol. 3, pp. 177–193 Search PubMed.
- A. V. Marenich, C. J. Cramer and D. G. Truhlar, J. Phys. Chem. B, 2009, 113, 6378–6396 CrossRef CAS PubMed.
- J. Lahiri, M. Moemeni, J. Kline, I. Magoulas, S. H. Yuwono, M. Laboe, J. Shen, B. Borhan, P. Piecuch, J. E. Jackson, G. J. Blanchard and M. Dantus, J. Chem. Phys., 2020, 153, 224301 CrossRef CAS PubMed.
- G. Knizia and G. K.-L. Chan, Phys. Rev. Lett., 2012, 109, 186404 CrossRef PubMed.
- T. Ayral, T.-H. Lee and G. Kotliar, Phys. Rev. B, 2017, 96, 235139 CrossRef.
- P. V. Sriluckshmy, M. Nusspickel, E. Fertitta and G. H. Booth, Phys. Rev. B, 2021, 103, 085131 CrossRef CAS.
- S. Sekaran, M. Tsuchiizu, M. Saubanère and E. Fromager, Phys. Rev. B, 2021, 104, 035121 CrossRef CAS.
- S. Wouters, C. A. Jiménez-Hoyos, Q. Sun and G. K.-L. Chan, J. Chem. Theory Comput., 2016, 12, 2706–2719 CrossRef CAS PubMed.
- M. R. Hermes and L. Gagliardi, J. Chem. Theory Comput., 2019, 15, 972–986 CrossRef PubMed.
- I. W. Bulik, G. E. Scuseria and J. Dukelsky, Phys. Rev. B: Condens. Matter Mater. Phys., 2014, 89, 035140 CrossRef.
- U. Mordovina, T. E. Reinhard, I. Theophilou, H. Appel and A. Rubio, J. Chem. Theory Comput., 2019, 15, 5209–5220 CrossRef CAS PubMed.
- J. G. Brandenburg, C. Bannwarth, A. Hansen and S. Grimme, J. Chem. Phys., 2018, 148, 064104 CrossRef PubMed.
- S. Grimme, A. Hansen, S. Ehlert and J.-M. Mewes, J. Chem. Phys., 2021, 154, 064103 CrossRef CAS PubMed.
- A. de la Lande, A. Álvarez-Ibarra, K. Hasnaoui, F. Cailliez, X. Wu, T. Mineva, J. Cuny, P. Calaminici, L. López-Sosa, G. Geudtner, I. Navizet, C. Garcia Iriepa, D. R. Salahub and A. M. Köster, Molecules, 2019, 24, 1653 CrossRef CAS PubMed.
- J. M. Vásquez-Pérez, G. U. Gamboa, D. Mejía-Rodríguez, A. Álvarez-Ibarra, G. Geudtner, P. Calaminici and A. M. Köster, J. Phys. Chem. Lett., 2015, 6, 4646–4652 CrossRef PubMed.
- J. Gao, J. Phys. Chem. B, 1997, 101, 657–663 CrossRef CAS.
- J. Gao, J. Z. H. Zhang and K. N. Houk, Acc. Chem. Res., 2014, 47, 2711 CrossRef CAS PubMed.
- X. Chen and J. Gao, J. Phys. Chem. Lett., 2020, 11, 4008–4016 CrossRef CAS PubMed.
- W. Xie and J. Gao, J. Chem. Theory Comput., 2007, 3, 1890–1900 CrossRef CAS PubMed.
- G. Vignale and M. Rasolt, Phys. Rev. Lett., 1987, 59, 2360–2363 CrossRef CAS PubMed.
- A. Laestadius, M. Penz, E. I. Tellgren, M. Ruggenthaler, S. Kvaal and T. Helgaker, J. Chem. Theory Comput., 2019, 15, 4003–4020 CrossRef CAS PubMed.
- G. Vignale, C. A. Ullrich and K. Capelle, Int. J. Quantum Chem., 2012, 113, 1422–1423 CrossRef.
- E. I. Tellgren, S. Kvaal, E. Sagvolden, U. Ekström, A. M. Teale and T. Helgaker, Phys. Rev. A: At., Mol., Opt. Phys., 2012, 86, 062506 CrossRef.
- A. Laestadius and M. Benedicks, Int. J. Quantum Chem., 2014, 114, 782–795 CrossRef CAS.
- A. Laestadius, Int. J. Quantum Chem., 2014, 114, 1445–1456 CrossRef CAS.
- S. Kvaal, A. Laestadius, E. Tellgren and T. Helgaker, J. Phys. Chem. Lett., 2021, 12, 1421–1425 CrossRef CAS PubMed.
- G. Vignale and M. Rasolt, Phys. Rev. B: Condens. Matter Mater. Phys., 1988, 37, 10685–10696 CrossRef PubMed.
- E. I. Tellgren, A. M. Teale, J. W. Furness, K. K. Lange, U. Ekström and T. Helgaker, J. Chem. Phys., 2014, 140, 034101 CrossRef CAS PubMed.
- T. J. P. Irons, J. Zemen and A. M. Teale, J. Chem. Theory Comput., 2017, 13, 3636–3649 CrossRef CAS PubMed.
- A. Pausch and W. Klopper, Mol. Phys., 2020, 118, e1736675 CrossRef.
- E. H. Lieb and R. Schrader, Phys. Rev. A: At., Mol., Opt. Phys., 2013, 88, 032516 CrossRef.
- E. I. Tellgren, S. Kvaal and T. Helgaker, Phys. Rev. A: At., Mol., Opt. Phys., 2014, 89, 012515 CrossRef.
- G. Vignale, M. Rasolt and D. J. W. Geldart, Phys. Rev. B: Condens. Matter Mater. Phys., 1988, 37, 2502–2507 CrossRef PubMed.
- J. Tao and J. P. Perdew, Phys. Rev. Lett., 2005, 95, 196403 CrossRef PubMed.
- W. Zhu and S. B. Trickey, J. Chem. Phys., 2006, 125, 094317 CrossRef PubMed.
- J. E. Bates and F. Furche, J. Chem. Phys., 2012, 137, 164105 CrossRef PubMed.
- W. Zhu, L. Zhang and S. B. Trickey, Phys. Rev. A: At., Mol., Opt. Phys., 2014, 90, 022504 CrossRef.
- S. Reimann, U. Ekström, S. Stopkowicz, A. M. Teale, A. Borgoo and T. Helgaker, Phys. Chem. Chem. Phys., 2015, 17, 18834–18842 RSC.
- C. J. Grayce and R. A. Harris, Phys. Rev. A: At., Mol., Opt. Phys., 1994, 50, 3089–3095 CrossRef CAS PubMed.
- F. R. Salsbury and R. A. Harris, J. Chem. Phys., 1997, 107, 7350–7359 CrossRef CAS.
- E. I. Tellgren, Phys. Rev. A, 2018, 97, 012504 CrossRef CAS.
- N. Tancogne-Dejean, M. J. T. Oliveira, X. Andrade, H. Appel, C. H. Borca, G. L. Breton, F. Buchholz, A. Castro, S. Corni, A. A. Correa, U. D. Giovannini, A. Delgado, F. G. Eich, J. Flick, G. Gil, A. Gomez, N. Helbig, H. Hübener, R. Jestädt, J. Jornet-Somoza, A. H. Larsen, I. V. Lebedeva, M. Lüders, M. A. L. Marques, S. T. Ohlmann, S. Pipolo, M. Rampp, C. A. Rozzi, D. A. Strubbe, S. A. Sato, C. Schäfer, I. Theophilou, A. Welden and A. Rubio, J. Chem. Phys., 2020, 152, 124119 CrossRef CAS PubMed.
- E. I. Tellgren, A. Laestadius, T. Helgaker, S. Kvaal and A. M. Teale, J. Chem. Phys., 2018, 148, 024101 CrossRef PubMed.
- S. Rohra and A. Görling, Phys. Rev. Lett., 2006, 97, 013005 CrossRef PubMed.
- S. Sen and E. I. Tellgren, J. Chem. Phys., 2018, 149, 144109 CrossRef PubMed.
- J. Tao, Phys. Rev. B: Condens. Matter Mater. Phys., 2005, 71, 205107 CrossRef.
- S. Pittalis, G. Vignale and F. Eich, Phys. Rev. B, 2017, 96, 035141 CrossRef.
- C. A. Ullrich, Phys. Rev. B, 2018, 98, 035140 CrossRef CAS.
- T. J. Callow, B. Pearce and N. I. Gidopoulos, J. Chem. Phys., 2022, 156, 111101 CrossRef CAS PubMed.
- T. Gould, L. Kronik and S. Pittalis, J. Chem. Phys., 2018, 148, 174101 CrossRef PubMed.
-
C. A. Ullrich, Time-dependent density-functional theory: concepts and applications, Oxford University Press, Oxford, 2012 Search PubMed.
-
Fundamentals of Time-dependent density-functional theory, ed. M. A. L. Marques, N. T. Maitra, F. M. S. Nogueira, E. K. U. Gross and A. Rubio, Springer, Heidelberg, 2012 Search PubMed.
- N. T. Maitra, J. Chem. Phys., 2016, 144, 220901 CrossRef PubMed.
- D. Jacob and S. Kurth, Nano Lett., 2018, 18, 2086–2090 CrossRef CAS PubMed.
- D. Jacob, G. Stefanucci and S. Kurth, Phys. Rev. Lett., 2020, 125, 216401 CrossRef CAS PubMed.
- G. Stefanucci and S. Kurth, Nano Lett., 2015, 15, 8020–8025 CrossRef CAS PubMed.
- A. K. Dhara and S. K. Ghosh, Phys. Rev. A: At., Mol., Opt. Phys., 1987, 35, 442–444 CrossRef CAS PubMed.
- S. K. Ghosh and A. K. Dhara, Phys. Rev. A: At., Mol., Opt. Phys., 1988, 38, 1149–1158 CrossRef CAS PubMed.
- G. Vignale, Phys. Rev. B: Condens. Matter Mater. Phys., 2004, 70, 201102 CrossRef.
- F. Kootstra, P. L. de Boeij and J. G. Snijders, J. Chem. Phys., 2000, 112, 6517–6531 CrossRef CAS.
- P. Romaniello and P. L. de Boeij, Phys. Rev. B: Condens. Matter Mater. Phys., 2005, 71, 155108 CrossRef.
- N. Raimbault, P. L. de Boeij, P. Romaniello and J. A. Berger, Phys. Rev. Lett., 2015, 114, 066404 CrossRef CAS PubMed.
- N. Raimbault, P. L. de Boeij, P. Romaniello and J. A. Berger, J. Chem. Theory Comput., 2016, 12, 3278–3283 CrossRef CAS PubMed.
- G. Vignale and W. Kohn, Phys. Rev. Lett., 1996, 77, 2037–2040 CrossRef CAS PubMed.
- J. A. Berger, Phys. Rev. Lett., 2015, 115, 137402 CrossRef CAS PubMed.
- S. Di Sabatino, J. A. Berger and P. Romaniello, Faraday Discuss., 2020, 224, 467–482 RSC.
- G. E. W. Bauer, Phys. Rev. B: Condens. Matter Mater. Phys., 1983, 27, 5912–5918 CrossRef.
- L. Lam and P. Platzmann, Phys. Rev. B: Solid State, 1974, 9, 5122–5127 CrossRef.
- E. H. Lieb and S. Oxford, Int. J. Quantum Chem., 1981, 19, 427–439 CrossRef CAS.
-
S. Di Marino, A. Gerolin and L. Nenna, in Optimal Transportation Theory with Repulsive Costs, ed. F. Santambrogio, T. Champion, G. Carlier, M. Rumpf, É. Oudet and M. Bergounioux, De Gruyter, 2017, ch. 9, vol. Topological Optimization and Optimal Transport in the Applied Sciences, pp. 204–256 Search PubMed.
- J. T. Chayes, L. Chayes and E. H. Lieb, Commun. Math. Phys., 1984, 93, 57–121 CrossRef.
- J. T. Chayes, L. Chayes and M. B. Ruskai, J. Stat. Phys., 1985, 38, 497–518 CrossRef.
- L. Garrigue, Commun. Math. Phys., 2021, 1803–1844 CrossRef , online first.
- M. M. Odashima and K. Capelle, J. Chem. Phys., 2007, 127, 054106 CrossRef PubMed.
- E. Räsänen, S. Pittalis, K. Capelle and C. R. Proetto, Phys. Rev. Lett., 2009, 102, 206406 CrossRef PubMed.
- S. Fournais, J. Lampart, M. Lewin and T. Østergaard Sørensen, Phys. Rev. A, 2016, 93, 062510 CrossRef.
- P. E. Lammert, Int. J. Quantum Chem., 2007, 107, 1943–1953 CrossRef CAS.
- S. Kvaal, U. Ekström, A. M. Teale and T. Helgaker, J. Chem. Phys., 2014, 140, 18A518 CrossRef PubMed.
-
H. H. Bauschke and P. L. Combettes, Convex Analysis and Monotone Operator Theory in Hilbert Spaces, Springer, New York, 2011 Search PubMed.
- M. Penz, A. Laestadius, E. I. Tellgren and M. Ruggenthaler, Phys. Rev. Lett., 2019, 123, 037401 CrossRef CAS PubMed.
-
L. Garrigue, 2021, arXiv.2101.01127.
- G. Buttazzo, L. De Pascale and P. Gori-Giorgi, Phys. Rev. A: At., Mol., Opt. Phys., 2012, 85, 062502 CrossRef.
- C. Cotar, G. Friesecke and C. Klüppelberg, Commun. Pure Appl. Math., 2013, 66, 548–599 CrossRef.
-
S. D. Marino, A. Gerolin and L. Nenna, in Optimal transportation theory with repulsive costs, ed. M. Bergounioux, É. Oudet, M. Rumpf, G. Carlier, T. Champion and F. Santambrogio, De Gruyter, 2017, pp. 204–256 Search PubMed.
- M. Colombo, L. De Pascale and S. Di Marino, Can. J. Math., 2015, 67, 350–368 CrossRef.
- E. Räsänen, M. Seidl and P. Gori-Giorgi, Phys. Rev. B: Condens. Matter Mater. Phys., 2011, 83, 195111 CrossRef.
- M. Seidl, S. Vuckovic and P. Gori-Giorgi, Mol. Phys., 2016, 114, 1076–1085 CrossRef CAS.
-
M. Colombo, S. Di Marino and F. Stra, 2021, arXiv.2106.06282.
- J. Grossi, D. P. Kooi, K. J. H. Giesbertz, M. Seidl, A. J. Cohen, P. Mori-Sánchez and P. Gori-Giorgi, J. Chem. Theory Comput., 2017, 13, 6089–6100 CrossRef CAS PubMed.
- F. Malet and P. Gori-Giorgi, Phys. Rev. Lett., 2012, 109, 246402 CrossRef PubMed.
- J. Grossi, M. Seidl, P. Gori-Giorgi and K. J. H. Giesbertz, Phys. Rev. A, 2019, 99, 052504 CrossRef CAS.
- J. Grossi, Z. H. Musslimani, M. Seidl and P. Gori-Giorgi, J. Phys.: Condens. Matter, 2020, 32, 475602 CrossRef CAS PubMed.
- A. D. Becke, J. Chem. Phys., 2014, 140, 18A301 CrossRef PubMed.
- F. Eich, M. Di Ventra and G. Vignale, J. Phys.: Condens. Matter, 2017, 29, 063001 CrossRef CAS PubMed.
- W. Tarantino and C. A. Ullrich, J. Chem. Phys., 2021, 154, 204112 CrossRef CAS PubMed.
- J. R. Chelikowsky, N. Troullier and Y. Saad, Phys. Rev. Lett., 1994, 72, 1240–1243 CrossRef CAS PubMed.
- T. L. Beck, Rev. Mod. Phys., 2000, 72, 1041–1080 CrossRef CAS.
- P. Motamarri, S. Das, S. Rudraraju, K. Ghosh, D. Davydov and V. Gavini, Comput. Phys. Commun., 2020, 246, 106853 CrossRef CAS.
- L. E. Ratcliff, W. Dawson, G. Fisicaro, D. Caliste, S. Mohr, A. Degomme, B. Videau, V. Cristiglio, M. Stella, M. D’Alessandro, S. Goedecker, T. Nakajima, T. Deutsch and L. Genovese, J. Chem. Phys., 2020, 152, 194110 CrossRef CAS PubMed.
- L. Lin, J. Lu and L. Ying, Acta Numer., 2019, 28, 405–539 CrossRef.
-
E. Cancès, A. Levitt, Y. Maday and C. Yang, Numerical methods for Kohn-Sham models: discretization, algorithms, and error analysis, in Density Functional Theory, ed. E. Cancès and G. Friesecke, Springer, 2023, ch. 7, in press Search PubMed.
- M. F. Herbst and A. Levitt, J. Phys.: Condens. Matter, 2020, 33, 085503 CrossRef PubMed.
- G. Makov and M. C. Payne, Phys. Rev. B: Condens. Matter Mater. Phys., 1995, 51, 4014–4022 CrossRef CAS PubMed.
- D. Gontier and S. Lahbabi, Appl. Math. Res. Express, 2017, 1, 1–64 Search PubMed.
- H. J. Monkhorst and J. D. Pack, Phys. Rev. B: Solid State, 1976, 13, 5188 CrossRef.
- G. L. W. Hart, J. J. Jorgensen, W. S. Morgan and R. W. Forcade, J. Phys. Commun., 2019, 3, 065009 CrossRef CAS.
- M. Methfessel and A. Paxton, Phys. Rev. B: Condens. Matter Mater. Phys., 1989, 40, 3616 CrossRef CAS PubMed.
- É. Cancès, V. Ehrlacher, D. Gontier, A. Levitt and D. Lombardi, Numer. Math., 2020, 1–48 Search PubMed.
- L. Zhang, H. Wang, R. Car and W. E, Phys. Rev. Lett., 2021, 126, 236001 CrossRef CAS PubMed.
- S. Mohr, L. E. Ratcliff, L. Genovese, D. Caliste, P. Boulanger, S. Goedecker and T. Deutsch, Phys. Chem. Chem. Phys., 2015, 17, 31360–31370 RSC.
-
S. Li, K. Chen, M.-Y. Hsieh, N. Muralimanohar, C. D. Kersey, J. B. Brockman, A. F. Rodrigues and N. P. Jouppi, SC ‘11: Proceedings of 2011 International Conference for High Performance Computing, Networking, Storage and Analysis, 2011, pp. 1–12.
- M. Snir, R. W. Wisniewski, J. A. Abraham, S. V. Adve, S. Bagchi, P. Balaji, J. Belak, P. Bose, F. Cappello and B. Carlson,
et al.
, Int. J. High Perform. Comput. Appl., 2014, 28, 129–173 CrossRef.
- E. Cancès, G. Dusson, Y. Maday, B. Stamm and M. Vohralk, Numer. Math., 2018, 140, 1033–1079 CrossRef.
- M. F. Herbst, A. Levitt and E. Cancès, Faraday Discuss., 2020, 224, 227–246 RSC.
- M. Herbst, A. Levitt and E. Cancès, Proc. JuliaCon Conf., 2021, 3, 69 CrossRef.
- L. E. A. Chanussot, ACS Catal., 2021, 11, 6059–6072 CrossRef CAS.
- J. Kirkpatrick, B. McMorrow, D. H. P. Turban, A. L. Gaunt, J. S. Spencer, A. G. D. G. Matthews, A. Obika, L. Thiry, M. Fortunato, D. Pfau, L. R. Castellanos, S. Petersen, A. W. R. Nelson, P. Kohli, P. Mori-Sánchez, D. Hassabis and A. J. Cohen, Science, 2021, 374, 1385–1389 CrossRef CAS PubMed.
- S. Dong, M. Govoni and G. Galli, Chem. Sci., 2021, 12, 4970–4980 RSC.
- V. V. Karasiev, J. Hinz, S. X. Hu and S. B. Trickey, Nature, 2021, 600, E12–E14 CrossRef CAS PubMed.
- B. Cheng, G. Mazzola, C. J. Pickard and M. Ceriotti, Nature, 2020, 585, 217–220 CrossRef CAS PubMed.
- J. Gazquez, M. Franco-Perez, P. Ayers and A. Vela, Int. J. Quantum Chem., 2019, 119, e25797 CrossRef.
- W. Yang and R. Parr, Proc. Natl. Acad. Sci. U. S. A., 1985, 82, 6723–6726 CrossRef CAS PubMed.
- T. Bettens, M. Alonso, P. Geerlings and F. De Proft, Phys. Chem. Chem. Phys., 2019, 21, 7378–7388 RSC.
- T. Clarys, T. Stuyver, F. De Proft and P. Geerlings, Phys. Chem. Chem. Phys., 2021, 23, 990–1005 RSC.
- R. Miranda-Quintana and P. Ayers, Theor. Chem. Acc., 2016, 135, 1–18 Search PubMed.
- P. K. Chattaraj and A. Poddar, J. Phys. Chem. A, 1998, 102, 9944–9948 CrossRef CAS.
- C. Morrel, V. Labet, A. Grand, P. Ayers, F. De Proft, P. Geerlings and H. Chermette, J. Chem. Theory Comput., 2009, 9, 2274–2283 CrossRef PubMed.
- U. Sarkar and P. K. Chattaraj, J. Phys. Chem. A, 2021, 125, 2051–2060 CrossRef CAS PubMed.
- X. Zhou, C. Rong, T. Lu and S. Liu, J. Phys. Chem. A, 2016, 120, 3634–3642 CrossRef CAS PubMed.
- N. Villegas-Escobar, S. Vogt Geisse, S. Gutierrez-Oliva and A. Toro-Labbe, Theor. Chem. Acc., 2020, 135, 191 Search PubMed.
- J. Han, A. Grafe and J. Gao, Inorg. Chem., 2021, 60, 14060–14071 CrossRef CAS PubMed.
- M. Liu, X. Chen, A. Grofe and J. Gao, J. Phys. Chem. Lett., 2018, 9, 6038–6046 CrossRef CAS PubMed.
- Q. Wu, P. W. Ayers and Y. K. Zhang, J. Chem. Phys., 2009, 131, 164112 CrossRef PubMed.
- R. G. Parr and R. G. Pearson, J. Am. Chem. Soc., 1983, 105, 7512–7516 CrossRef CAS.
- P. W. Ayers, S. Fias and F. Heidar-Zadeh, Comput. Theor. Chem., 2018, 1142, 83–87 CrossRef CAS.
-
Libxc: a library of exchange and correlation functionals for density functional theory, https://www.tddft.org/programs/libxc/, accessed: 2022-01-12.
-
XCFun: A library of exchange-correlation functionals with arbitrary-order derivatives, https://github.com/dftlibs/xcfun, accessed: 2022-01-12.
-
Numgrid: a library that produces numerical integration grid for molecules based on atom coordinates, atom types, and basis set information, https://github.com/dftlibs/numgrid, accessed: 2022-01-12.
- A. Krylov, J. Herbert, F. Furche, M. Head-Gordon, P. Knowles, R. Lindh, F. Manby, P. Pulay, C.-K. Skylaris and H.-J. Werner, J. Phys. Chem. Lett., 2015, 6, 2751–2754 CrossRef CAS PubMed.
-
The FAIR data principles, https://www.force11.org/group/fairgroup/fairprinciples, accessed: 2022-01-12.
- K. Lejaeghere, G. Bihlmayer, T. Björkman, P. Blaha, S. Blügel, V. Blum, D. Caliste, I. E. Castelli, S. J. Clark, A. D. Corso, S. de Gironcoli, T. Deutsch, J. K. Dewhurst, I. D. Marco, C. Draxl, M. Dułak, O. Eriksson, J. A. Flores-Livas, K. F. Garrity, L. Genovese, P. Giannozzi, M. Giantomassi, S. Goedecker, X. Gonze, O. Grånäs, E. K. U. Gross, A. Gulans, F. Gygi, D. R. Hamann, P. J. Hasnip, N. A. W. Holzwarth, D. Iuşan, D. B. Jochym, F. Jollet, D. Jones, G. Kresse, K. Koepernik, E. Küçükbenli, Y. O. Kvashnin, I. L. M. Locht, S. Lubeck, M. Marsman, N. Marzari, U. Nitzsche, L. Nordström, T. Ozaki, L. Paulatto, C. J. Pickard, W. Poelmans, M. I. J. Probert, K. Refson, M. Richter, G.-M. Rignanese, S. Saha, M. Scheffler, M. Schlipf, K. Schwarz, S. Sharma, F. Tavazza, P. Thunström, A. Tkatchenko, M. Torrent, D. Vanderbilt, M. J. van Setten, V. V. Speybroeck, J. M. Wills, J. R. Yates, G.-X. Zhang and S. Cottenier, Science, 2016, 351, aad3000 CrossRef PubMed.
- A. Gulans, A. Kozhevnikov and C. Draxl, Phys. Rev. B, 2018, 97, 161105 CrossRef CAS.
- S. Lehtola, C. Steigemann, M. J. Oliveira and M. A. Marques, SoftwareX, 2018, 7, 1–5 CrossRef.
- Q. Sun, X. Zhang, S. Banerjee, P. Bao, M. Barbry, N. S. Blunt, N. A. Bogdanov, G. H. Booth, J. Chen, Z.-H. Cui, J. J. Eriksen, Y. Gao, S. Guo, J. Hermann, M. R. Hermes, K. Koh, P. Koval, S. Lehtola, Z. Li, J. Liu, N. Mardirossian, J. D. McClain, M. Motta, B. Mussard, H. Q. Pham, A. Pulkin, W. Purwanto, P. J. Robinson, E. Ronca, E. R. Sayfutyarova, M. Scheurer, H. F. Schurkus, J. E. T. Smith, C. Sun, S.-N. Sun, S. Upadhyay, L. K. Wagner, X. Wang, A. White, J. D. Whitfield, M. J. Williamson, S. Wouters, J. Yang, J. M. Yu, T. Zhu, T. C. Berkelbach, S. Sharma, A. Y. Sokolov and G. K.-L. Chan, J. Chem. Phys., 2020, 153, 024109 CrossRef CAS PubMed.
- D. G. A. Smith, L. A. Burns, A. C. Simmonett, R. M. Parrish, M. C. Schieber, R. Galvelis, P. Kraus, H. Kruse, R. Di Remigio, A. Alenaizan, A. M. James, S. Lehtola, J. P. Misiewicz, M. Scheurer, R. A. Shaw, J. B. Schriber, Y. Xie, Z. L. Glick, D. A. Sirianni, J. S. O’Brien, J. M. Waldrop, A. Kumar, E. G. Hohenstein, B. P. Pritchard, B. R. Brooks, H. F. Schaefer, A. Y. Sokolov, K. Patkowski, A. E. DePrince, U. Bozkaya, R. A. King, F. A. Evangelista, J. M. Turney, T. D. Crawford and C. D. Sherrill, J. Chem. Phys., 2020, 152, 184108 CrossRef CAS PubMed.
- P. Giannozzi, O. Baseggio, P. Bonfà, D. Brunato, R. Car, I. Carnimeo, C. Cavazzoni, S. de Gironcoli, P. Delugas, F. Ferrari Ruffino, A. Ferretti, N. Marzari, I. Timrov, A. Urru and S. Baroni, J. Chem. Phys., 2020, 152, 154105 CrossRef CAS PubMed.
- F. Neese, F. Wennmohs, U. Becker and C. Riplinger, J. Chem. Phys., 2020, 152, 224108 CrossRef CAS PubMed.
- G. te Velde, F. M. Bickelhaupt, E. J. Baerends, C. Fonseca Guerra, S. J. A. van Gisbergen, J. G. Snijders and T. Ziegler, J. Comput. Chem., 2001, 22, 931–967 CrossRef CAS.
- H.-J. Werner, P. J. Knowles, F. R. Manby, J. A. Black, K. Doll, A. Heßelmann, D. Kats, A. Köhn, T. Korona, D. A. Kreplin, Q. Ma, T. F. Miller, A. Mitrushchenkov, K. A. Peterson, I. Polyak, G. Rauhut and M. Sibaev, J. Chem. Phys., 2020, 152, 144107 CrossRef CAS PubMed.
- A. Jain, S. P. Ong, G. Hautier, W. Chen, W. D. Richards, S. Dacek, S. Cholia, D. Gunter, D. Skinner, G. Ceder and K. A. Persson, APL Mater., 2013, 1, 011002 CrossRef.
- D. G. A. Smith, D. Altarawy, L. A. Burns, M. Welborn, L. N. Naden, L. Ward, S. Ellis, B. P. Pritchard and T. D. Crawford, Wiley Interdiscip. Rev.: Comput. Mol. Sci., 2020, e1491 Search PubMed.
-
Molecular scicences software institute (MolSSI), https://molssi.org/, accessed: 2022-01-12.
- A. I. Krylov, T. Windus, T. Barnes, E. Marin-Rimoldi, J. Nash, B. Pritchard, D. G. A. Smith, D. Altarawy, P. Saxe, C. Clementi, T. D. Crawford, R. J. Harrison, S. Jha, V. Pande and T. Head-Gordon, J. Chem. Phys., 2018, 149, 180901 CrossRef PubMed.
-
Simulation Environment for Atomistic and Molecular Modelling (SEAMM), https://github.com/molssi-seamm, accessed: 2022-01-12.
-
M. Vanzini, A. Aouina, M. Panholzer, M. Gatti and L. Reining, Connector theory for reusing model results to determine materials properties, 2022 Search PubMed.
-
Declaration on Research Assessment, (DORA), https://sfdora.org.
- M. Govoni, M. Munakami, A. Tanikanti, J. H. Skone, H. B. Runesha, F. Giberti, J. de Pablo and G. Galli, Sci. Data, 2019, 6, 190002 CrossRef PubMed.
-
Qresp, Curation and Exploration of Reproducible Scientific Papers, https://qresp.org/, accessed: 2022-01-12.
-
Photoelectron spectra of aqueous solutions from first principle, https://paperstack.uchicago.edu/paperdetails/594c50671bd40f5e9b5c043b?server=https.
-
The MolSSI Quantum Chemistry Archive, https://qcarchive.molssi.org/, accessed: 2022-01-12.
-
NOMAD Repository and Archive, https://nomad-lab.eu/services/repo-arch, accessed: 2022-01-12.
- PhD Student Seminar Series organized at Rutgers-Newark, Summer 2021, https://sites.rutgers.edu/dft-student-seminar.
- W. Kutzelnigg, THEOCHEM, 2006, 768, 163–173 CrossRef CAS.
-
W. Kohn, Density Functional Theory, Plenum, New York, 1995, pp. 3–10 Search PubMed.
- O. Gunnarsson, J. Harris and R. O. Jones, Phys. Rev. B: Solid State, 1977, 15, 3027–3038 CrossRef CAS.
-
https://www.coursera.org/learn/density-functional-theory
.
-
K. Burke, The ABC of DFT, https://dft.uci.edu/doc/g1.pdf.
-
W. Koch and M. C. Holthausen, A Chemist's Guide to Density Functional Theory, Wiley-VCH, Weinheim, 2001 Search PubMed.
-
F. Giustino, Materials Modelling using Density Functional Theory, Oxford University Press, Oxford, 2014 Search PubMed.
Footnotes |
† Note that there is a (semi)local form for short-range interactions, e.g., δ(r1 − r2), . |
‡ Ts[ρ] has a slightly different meaning in hybrid DFT, where the Slater determinant Φ in Ts = 〈Φ|T|Φ〉 minimizes T + αW with 0 < α < 1 rather than T; see Garrick et al.68 |
|
This journal is © the Owner Societies 2022 |