Tropical cyclone-induced cold wakes in the northeast Indian Ocean†
Received
14th August 2021
, Accepted 11th February 2022
First published on 11th February 2022
Abstract
We present a detailed long-term (1982–2020) analysis of cyclone-induced surface cooling (i.e., cold wake) in the northeast Indian Ocean, which hosts about 6% of the annual tropical cyclones in the world. Here, the analysis is performed for 92 cyclones that triggered significant cooling at the sea surface in two different seasons: the post and pre-monsoons. It is found that these cyclones generally cool the sea surface from 0.5 °C to 4.81 °C after their passage; depending on the cyclones. The pre-monsoon cyclones show stronger cooling, but the post-monsoon cyclones exhibit a longer duration of cooling and recovery time. Slow-moving cyclones (translational speed less than 3 m s−1) impose more stress on the ocean surface and produce stronger cooling, due to upwelling and associated vertical mixing. Furthermore, the cyclones that occurred during La Niña years show relatively more intensity than those in the El Niño years. Since the sea surface temperature (SST) drop impedes cyclones from further intensification, our study gives new insights into the cyclone intensity in the Bay of Bengal. Additionally, the range of surface cooling derived from this analysis can help coupled numerical models to improve the forecast of intensity and landfall of cyclones, which can save millions of lives and the coastal ecosystem.
Environmental significance
We present the first long-term assessment of cyclone-triggered cold water wakes in an oceanic region, where 6% of the total cyclones in the world occur every year, the Bay of Bengal. The analyses show that the cooling can be up to 3.5°, which can significantly impede the intensification of cyclones. The long-term study with two different data sets provides new insights into the cyclone intensity in the climate change context. The results would also help the relevant physical and microphysical parameterizations in numerical models and aid in forecasting the cyclones to save millions of lives and the ecosystem in and around the oceanic region.
|
1. Introduction
Tropical cyclones (TCs) generally cause great damage to the life and property of people and ecosystems. TCs are extreme weather systems that form over oceans in the tropical regions with well-defined convection and cyclonic circulation of surface winds.1 There are certain conditions that should be met for the genesis of TCs such as the sea surface temperature (SST) should be greater than 26.5 °C, winds should converge near the ocean surface, low vertical wind shear and the system should be away from the equator to spin.2 Although TCs are mostly catastrophic, they occasionally bring rain and water to drought-hit and water-scarce areas.
Recent studies indicate that the damage caused by TCs is increasing.3 Factors governing the strength of TCs are yet to be clearly understood4 as they are mostly influenced by both synoptic scale and small-scale features in the ocean and atmosphere.5 In general, TCs can push the subsurface colder water to the warm surface by the strong winds associated with them and cause cooling there.6 The SST cooling has a negative feedback on the intensity of TCs as it suppresses the exchange of heat flux between the atmosphere and oceans.7,8 The TC-triggered cooling at the surface (this process is also referred to as cold water-wakes or cold wakes) is thus closely related to TC intensity.
Studies have shown different scales of SST drop associated with TCs across the world oceans.9,10 For example, cold wakes were evident during Hurricanes Fabian (2003) and Frances (2004) in the Atlantic, and there was a significant cooling at the sea surface of up to 4 °C.11 Similarly, a range of the magnitude of surface cooling is reported in earlier studies on individual cyclones, e.g., Hurricane Gilbert cooled the surface up to 4 °C (ref. 12) and Hurricane Hilda up to 6 °C.13 In the Pacific, Typhoons Kalmargi and Sarika induced a cooling of 6 °C and 4.2 °C, respectively,14 but Kai-Tak cooled by 9 °C,15 and Lingling cooled by 11 °C.16,17 It is also postulated that TC induced cold wakes can reduce the number of weak to moderate TCs, but may contribute to more severe TCs.18
The north Indian Ocean (NIO) houses two oceanic basins: the Bay of Bengal (BoB or the bay) and Arabian Sea. The bay and Arabian Sea have two peaks in the annual cycle of cyclones. The bay is vulnerable to intense TCs during March–May (MAM, pre-monsoon) and October through December (DJF, post-monsoon) seasons, with noticeable inter-annual variability in both frequency and intensity of TCs.19 In general, there will be an increase in relative humidity over the oceanic region in the pre-monsoon season, which is the preceding feature for the first cyclone season.20 The low vertical wind shear due to the absence of upper-level jet streams accounts for the second cyclone season in the post-monsoon.20–22 The weaker winds over the bay force comparatively slower ocean circulation, and these winds are also incapable of surpassing strong stratification near the surface due to the low salinity there. This brings about a shallow mixed layer, and the prevailing SST (≥28 °C) in the bay favours the development of large-scale deep convective systems such as TCs,23 which is why the bay assists the formation of about 6% of the total annual worldwide TCs there.24 Nevertheless, the stratification is stronger in the post-monsoon season, leading to smaller cyclone-induced cooling compared to that in the pre-monsoon, which is characterised by a higher SST drop due to weaker stratification. The TC-induced reduction in the SST over the bay ranges from 0.3 °C to 3 °C, depending on the cyclone track and strength.25,26
Currently, satellite measurements with microwave sensors that are less sensitive to clouds are available and facilitate extensive description of SST during the period of TCs.15,27 In the case of Bay of Bengal, several studies have examined the impact of TCs on SSTs using satellite measurements,21,25,28–31 but mostly for individual cyclones. Therefore, we present a comprehensive evaluation of the SST drop, surface cooling or cold wakes associated with TCs that occurred in the bay in the satellite measurement era, 1982–2020. The cooling at the surface has important implications for the growth of cyclones as it prevents their intensification. In addition, the analyses presented in this study would help the numerical modelling community to diagnose the best and worst cooling scenarios to parameterise relevant processes in the TC models to make more accurate track prediction and intensity forecasts. The analysis also gives better insights into the air–sea exchange processes.
2. Methods
We have taken the cyclone track data from the Joint Typhoon Warning Centre (JTWC).32 The data include the position and strength of the cyclones at 6 h intervals for their complete life cycle. The data also have location-wise cyclone tracks together with the highest wind speed in that period. We have considered the Saffir–Simpson scale to differentiate the category of cyclones, and considered the maximum sustained wind speed (MSW) as a scale of cyclone intensity. As our work focuses on the cyclones that occurred since 1982 in accordance with the availability of satellite SST measurements, we have used the high resolution AVHRR-only (advanced very high-resolution radiometer) SST observations, which utilise infrared measurements from the AVHRR sensor. These daily SST data have a resolution of 0.25° latitude × 0.25° longitude and have the longest record (from late 1981 to date) from a single instrument. Infrared instruments, such as the AVHRR, can deliver high-resolution observations, but it is difficult to get measurements through clouds.33 Therefore, we have also used the microwave OISST (optimum interpolation sea surface temperature) measurements (from 1998 to 2020) produced by remote sensing systems34 to compare with the AVHRR analyses. The microwave radiometers provide a valuable data set, and the 25 km OISST data include SST measurements from multiple sensors.
The study region, the northeast Indian Ocean or BoB (4°–24° N, 78°–98° E), is separated to southwest (4°–14° N, 78°–88° E), southeast (4°–14° N, 88–98° E), northwest (14°–24° N, 78°–88° E) and northeast (14°–24° N, 88°–98° E) bay. The SST cooling or cold wake is considered as the difference in the SST between the pre- and post-cyclone periods. The pre-cyclone period is considered as 3 days (72 h) prior to the formation of cyclones, and the post-cyclone period is the day in which the maximum cooling is observed, although the peak cooling occurred during the cyclone period itself in some cases. To examine the time evolution of the SST drop associated with cyclones, the daily analysis is done on a 1° × 1° spatial grid, and is taken adjacent to the track of cyclones in which the maximum cooling is observed. The SST drop is considered significant, when it is >0.5 °C, as these SST data have an uncertainty of about 0.5 °C.35 The lifetime of a cyclone is defined as the number of days in which the cyclone sustains in subsequent stages, from its development to the weakening stage as demonstrated by its (JTWC) track.
The physical mechanisms responsible for TC-induced SST cooling are entrainment, air-sea exchange and heat advection. The translational speed of a cyclone is calculated by dividing the sum of the respective distance the cyclone moves 6 h before and 6 h after reaching its current position by the total time interval of 12 h.36 The distance between the track points is derived using the Haversine formula. That is,
p = sin2(ΔΦ/2) + cos(Φ1)·cos(Φ2)·sin2(Δλ/2) |
q = 2·ptan2(√p·√(1 − p)); d = R·q |
where
λ = longitude,
Φ = latitude, Δ
λ =
λ1 − λ
2 (difference of longitude), Δ
Φ =
Φ1 −
Φ2 (difference of latitude),
R is the radius of the earth (6371 km) and
d is the distance computed between the track points. According to Chan and Gray,
37 if the translational speed of a cyclone is lower than or equal to 3 m s
−1, then it is slow moving. The translational speed lies in the range of 3–7 m s
−1 for a “moderately fast” or normal cyclone, and if the translational speed is greater than 7 m s
−1, then it is considered as “fast moving”. Nevertheless, the effect of translational speed on TC intensity has not been examined in detail for TCs over different oceanic regions. The Ekman pumping velocity (EPV) is estimated over a 1° × 1° latitude–longitude area where the cyclone shows its peak intensity. The EPV parameter is used as a basis for indicating the upwelling (positive values) and downwelling (negative values). Many studies used EPV as a measure to indicate the upwelling caused by strong winds during TCs.
2,21,38 The 10 m daily winds with a resolution of 0.25° × 0.25° from the ERA5 reanalysis (European Centre for Medium Range Weather Forecast (ECMWF)) atmospheric ReAnalysis version 5 (ref.
39) are considered to compute EPV.
40 That is,
where
f is the Coriolis parameter,
ρ is the seawater density and ∇ ×
τ is the wind stress curl. In addition to translational speed and EPV, we examine the roles of wind stress, latent heat flux, sensible heat flux and surface net heat flux in modifying the magnitude of SST cooling. The daily data of sensible, latent and net heat fluxes, and wind stress are considered from the TropFlux analyses
41,42 and are analysed on a 1° × 1° latitude–longitude space adjacent to the track of each cyclone, where the peak cooling is observed. The wind stress draws energy and momentum away from cyclones and often limits their intensity.
43 We have used the data of global physics analysis and Copernicus Marine Service (CMEMS) for the mixed layer depth (MLD) for the period 1993–2020. We performed correlation analyses of different parameters with SST drop and examined their statistical significance with respect to the
p-test, at the 95% confidence level. To understand the influence of El Niño and La Niña events on cyclone-induced SST drop, we used the Oceanic Niño Index (ONI) to classify El Niño and La Niña events. If ONI ≥ 0.5, we classify it as an El-Niño year and if ONI ≤ −0.5, we consider it as a La-Niña year. Values of the ONI between −0.5 and 0.5 are considered as normal years. We have taken the difference in SST between three days before and after a cyclone. The cooling days listed in Tables S1 and S2
† are considered to estimate the SST cooling induced by the cyclones during El Niño, La Niña and normal years.
3. Results and discussion
A total of 112 cyclones occurred across all seasons in BoB during the 1982–2020 period. Out of these, 102 cyclones happened in the pre- and post-monsoon seasons, and we restrict our analysis to these seasons. Among the 102 cyclones, 92 cyclones showed a significant drop in SST. The track of all cyclones in these seasons with the respective seasonal climatology of SST is shown in Fig. 1. It is evident that the pre-monsoon season has a greater SST than that in post-monsoon. The frequency of cyclone occurrence in both seasons follows a bimodal distribution with peaks in November and May.44 The number of cyclones is very small in pre-monsoon, as the stronger vertical wind shear disrupts the vertical structure of convection, which inhibits the cyclone formation. In October, the higher SST of about 30 °C–31 °C indicates the secondary warming period in BoB.45 The onset of northeast monsoon winds in late October significantly changes the SST during the October–December period. In winter (January and February), the average SST over the bay is the lowest, about 25 °C–28 °C. Nonetheless, it remains in the range of 28.5 °C–29 °C in other seasons46 with the highest values in May and November.47
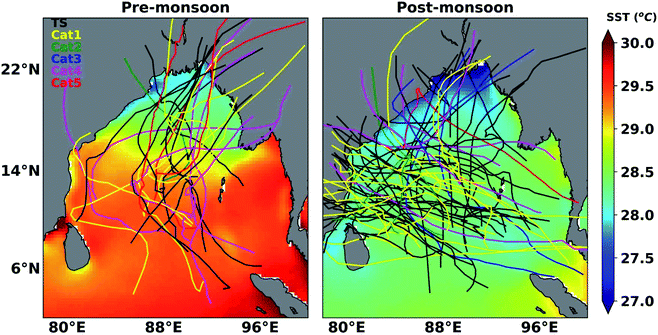 |
| Fig. 1 The cyclone landfall in different seasons. The cyclone tracks from the JTWC are considered for the pre- and post-monsoon seasons. The shade represents average sea surface temperature (SST) from the AVHRR (°C) for each season in the respective regions. | |
3.1 Pre-monsoon cyclones
Twenty-nine cyclones showed a significant SST drop during the pre-monsoon seasons in 1982–2020 and most of them were category 1 cyclones, as shown in Table S1.†Fig. 2 shows that cyclones with high translational speed have smaller EPV and smaller SST drop in most cases.6,36 Out of these 29 cyclones, most had their landfall in the northeastern bay and very few in the northwestern bay (Fig. 1). The cyclone BoB02 in 1982 showed the highest (4.81 °C) cooling and BoB01 in 2004 showed the smallest (0.78 °C) cooling among the pre-monsoon TCs as analysed from the AVHRR data. These cyclones are in category 2 and 1 with translational speeds of 2.54 m s−1 and 6.24 m s−1, respectively. The tropical storm (TS) category cyclones show the highest value of translation speed, yet some exhibit a cooling greater than 1 °C. For example, Viyaru and Maarutha are in the TS category with translational speeds of 7.34 m s−1 and 7.23 m s−1, respectively, and they show more cooling than that by BoB01 in 2004. Therefore, other factors could also influence the cold wakes produced by TCs. When analysing the EPV values of each cyclone, it is found that a higher EPV can produce higher cooling even though the cyclones are in the lower category. The average cooling estimate for the pre-monsoon cyclones is about 2.05 °C (2.24 °C), as derived from the AVHRR (OISST) measurements. The SST drop is largely influenced by the translational speed and EPV, and the cyclones have an average translational speed of 4.86 m s−1, a MLD (as analysed from 1994 to 2020) of 14.6 m and an EPV of 1.94 × 10−4 m s−1 (i.e., they are mostly moderately fast moving).48 The complete analysis for all cyclones is presented in Tables S1–S3.†
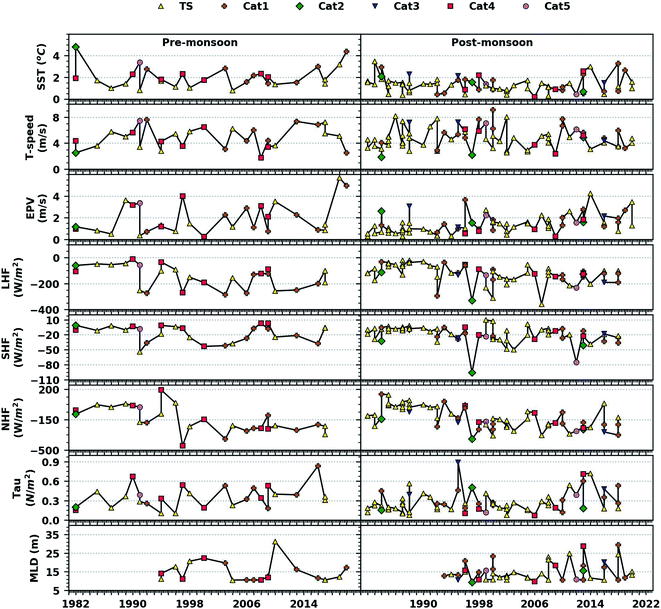 |
| Fig. 2 Sea surface cooling induced by tropical cyclones. The observed SST drop (°C) occurred due to the cyclone passage. The translational speed (T-speed) of TCs when the cyclone reached its maximum intensity (m s−1), EPV when cyclone reached its maximum intensity (10−4 m s−1), and latent heat flux, sensible heat flux, net heat flux, wind stress (Tau) and mixed layer depth (MLD) over the 1° × 1° (latitude–longitude) region where the cooling is observed are shown. | |
Table S3† lists the translational speed, EPV, wind stress and different fluxes during each cyclone period. The EPV is greater than or equal to 1 × 10−4 m s−1 for 61 cyclones. The impact of EPV on surface cooling occurs underneath the cyclones with lower translational speed, as the cyclones with high translational speed can pass the region before the upwelling can influence the temperature in the surface layer. This is because the translational speed denotes the forward speed of cyclones, and if TCs are slow (fast) moving, then they tend to stay longer (shorter) over the oceans and induce higher (smaller) SST drop.36 This is also consistent with the analyses presented for the cyclones here. According to geostrophic adjustment theory, a minimum geostrophic adjustment time of 2π/f (Coriolis parameter)49 is required for the EPV to reach its maximum potential. Henceforth, if the above said time is not sufficiently long, the upwelling velocity will be much smaller than the maximum potential EPV. Note that a positive value of EPV along the track indicates upwelling at the cyclone centre. That is, cooling can occur at the surface due to the movement of surface water by strong winds after the cyclone passes. The surface winds exert stress on the sea surface that lead to vertical mixing.50–52 During the cyclones, the currents generated in the TC centre tend to move the water away from that region due to wind stress. The cooler subsurface water reaches the surface to maintain the equilibrium. The higher category cyclones (Fig. 2) show relatively strong wind stress as evidenced by the pre-monsoon TCs. The heat transfer from the ocean to the atmosphere through evaporation is enhanced by the cyclone-induced surface winds. The net heat flux is negative for most cyclones, which is mainly due to the strong winds and dense cloud cover during the cyclone period. The surface cooling impact due to the surface heat loss is followed by the negative heat flux.53 The surface cooling is produced generally by the entrainment of subsurface cold water and turbulent mixing, and therefore, the role of surface heat flux is insignificant in the case of deep oceans.54–56 Although heat flux influences sea surface cooling, it is subtle in contrast to that caused by the entrainment of cold-water and turbulent mixing in the mixed layer.
We have performed an analysis with AVHRR-only SST averaged for 5 days before the cyclone, the entire cyclone period, 5 days after the cyclone and the next 5 days of three selected cyclones. These cyclones are selected based on the region of landfall, (a–d) Nargis in 2008, (e–h) Aila in 2009 and (i–l) Laila in 2010, and are shown in Fig. 3 (the analyses with OISST is shown in the ESI document Fig. S2†). The daily evolution of SST is shown in the ESI document Fig. S3–S5† for AVHRR and Fig. S6–S8† for OISST, respectively. Nargis was a category 4 cyclone that formed during 27 April–3 May over the warm waters with an SST of about 30 °C (AVHRR). It attained the maximum sustained wind speed (MSW) of 59.16 m s−1, and the SST dropped to 28 °C (AVHRR) during the cyclone passage (as also illustrated in the Graphical Abstract). The cyclone Aila that occurred during 23–26 May was a category 1 TC and was short-lived with a MSW of 33.4 m s−1. The cyclone Laila, a category 1 storm occurred during 17–21 May with a MSW of 33.4 m s−1. The translational speed of Nargis, Aila and Laila was about 1.77, 3.43 and 3.63 m s−1, respectively. Nargis was slow moving, and Aila and Laila were moderately fast-moving cyclones. Nargis showed more cooling than the other two cyclones. Aila and Laila were category 1 cyclones, and they produced a cooling of 2.03 °C and 1.35 °C (with AVHRR data), respectively. The MLD for cyclones Nargis, Aila and Laila is 10.57, 11.91 and 31.3 m, respectively. In general, the BoB is characterised by a smaller MLD during the pre-monsoon season.57 The cyclone Laila had higher translational speed and smaller net heat flux as compared to those of Aila. As the wind stress was higher (0.53 N m−2 for Aila as compared to 0.4 and 0.34 N m−2 for Laila and Nargis, respectively) and the net flux was smaller (−256.4 W m−2 for Laila, and in turn −219.6 and −250 W m−2 for Aila and Nargis), Alia showed relatively higher cooling. When the fluxes are examined, the values of sensible heat flux are 3.03, 3.06 and −24.53 W m−2 and those of latent heat flux are −122.33, −88.63 and −256.39 W m−2 in turn for Nargis, Aila and Laila.
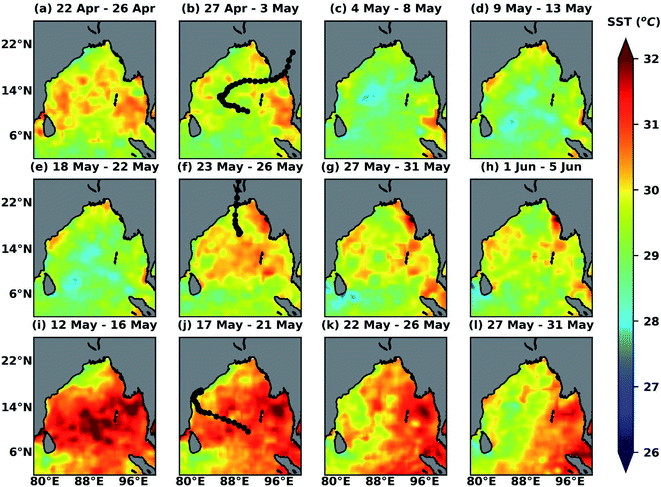 |
| Fig. 3 Evolution of SST during the cyclone passage. The SST (AVHRR) averaged for five days before the cyclone, during the entire cyclone period, five days just after the cyclone and the next five days respectively for (a–d) Nargis (2008), (e–h) Aila (2009) and (i–l) Laila (2010). The complete tracks of the cyclone are also shown in the respective analysis. | |
During the pre-monsoon season, the monsoon trough passes through the northernmost Bay of Bengal.58 Convective systems mostly form within the trough, and if they stay for a longer period over the ocean, they are likely to become a cyclone. In the north and north-western bay, the potential for convection is the highest, with relatively larger surface-based convective available potential energy (CAPE).58 During May, i.e., in pre-monsoon, the solar heating increases SST, which affects the vertical gradient of potential temperature, causing larger values of CAPE.59,60 Our analysis shows that most TCs recurve toward the north or north-eastern bay, and is clear from the TC tracks given in Fig. 1 and Table S1; † consistent with analyses of Sengupta et al.25 As shown for other cyclones, the analyses with OISST data exhibit slightly higher values when compared to those of AVHRR for most cases. Therefore, the maximum and minimum cooling cases are slightly different for the AVHRR-based analyses.
Note that, except Laila, all other cyclones deflected towards the northern bay, but the former propagated along the Andhra coast towards the west. In pre-monsoon, the average recovery time of SST to its pre-cyclone condition is about 10 days, and the average maximum lifetime cyclone intensity is about 31 m s−1. The pre-monsoon cyclones show an average lifetime of 5 days. The peak cooling is generally found one day after passage of the cyclone.61 We also find a similar timing for the SST drop for most cases, but some show the highest cooling during the cyclone period itself. The role of latent heat in cyclones is that its enhancement normally favours TC intensification,62 and these fluxes are lower than those in post-monsoon.
3.2 Post-monsoon cyclones
The monsoon trough crosses the centre of BoB during post-monsoon, and the convective system within the trough will favour the cyclone activity when they are over the ocean for longer periods.58 The primary control for the formation of TCs in the southern basin is the meeting point of the monsoon trough and the region of low vertical wind shear. For instance, it was shown that about 70–80% of TCs form within the monsoon trough in the western North Pacific.63 Stronger near-surface haline stratification is found during the post-monsoon due to the huge influx of freshwater following the monsoon rains.64 In addition, the northern bay has a barrier layer (BL), a deeper isothermal or warm subsurface layer underneath a shallow fresh water layer, which may further impede the cooling during the period. Salinity stratification and the BL trap heat in the subsurface waters. The BL also helps to maintain the lower SST in some cases as the thin surface water column loses heat through air–sea exchanges. In contrast, the southern bay has a relatively very shallow BL throughout the year, and note that stratification plays an important role in cold wakes.22,25,65,66 Furthermore, the difference in the stratification in different seasons is also a factor that decides the magnitude of SST drop instigated by TCs.
The MLD, BLT (BL thickness), ILD (isothermal layer depth) and D23 (depth of the 23° isotherm) of selected cyclones during pre (left) and post (right) – monsoons are shown in Fig. S1.† In accordance with the availability of buoy data (location of buoy data in Table S4†), MLD and BLT are estimated and are listed in Table S5.† The analysis shows a very shallow BL in the pre-monsoon cyclone season (e.g., Aila and Bijli) as compared to that in post-monsoon. It has already been shown that a pre-existing BLT can reduce the TC driven SST drop,67 whereas a strong BLT can energise TCs.7
Out of the 102 cyclones analysed, 73 occurred (71.56%) in the post-monsoon season, as shown in Table S2.† Among these 73 cyclones, 63 showed (86.3%) significant cooling as estimated from the AVHRR SST (35 cyclones or 47.94%, as analysed from the OISST) data. Most cyclones formed in the post-monsoon seasons are TS and category 1. The cyclones Phailin and BoB06 (Odisha super cyclone) are category 5 cyclones of this season. Although they are higher category cyclones, the cold wakes observed are 1.23 °C and 2.25 °C, respectively. The Odisha super cyclone with a translational speed of 4.64 m s−1 was slow moving compared to Phailin (5.39 m s−1). The highest translational speed (9.12 m s−1) is estimated for BoB05 in 2000, a category 1 cyclone that showed a cooling of 0.85 °C. Fig. 2 suggests a higher SST drop driven by the post-monsoon cyclones, particularly for strong and slow-moving, resulting in weakening of cyclones due to the oceanic negative feedback (see ESI Fig. S2 and Table S3†). Earlier studies demonstrated that the northern bay has a deeper BL.68Fig. 1 and Table S2† show that the cyclones that occurred in recent years have a tendency to move northwards and reach up to the northern BoB. The EPV during the cyclone period is positive in all cases, and the strong cyclone Hudhud shows the highest EPV of 4.24 × 10−4 m s−1. The average MLD for cyclones from 1994 to 2020 is 15 m. The cyclones with higher EPV show stronger cold-wakes even though they are fast moving. The lowest EPV of 0.27 × 10−4 m s−1 was associated with BOB03 in 1982 and Ward in 2009, with a cooling of about 1.67 °C and 0.89 °C, respectively.
During post-monsoon, BoB experiences cooling, and negative net heat flux is important in cyclone induced SST cold wakes. The evaporative cooling due to the northeasterly winds coupled with the reduction in solar radiation cause the net heat flux to be negative.69 The advection of dry air originates over the cold-wake and goes past the TC centre to the right side of the track, which is due to the enhanced evaporation driven by the near surface winds. In net fluxes, latent heat flux plays a key role in cyclone-induced cooling, and larger values of latent heat flux are shown by the post-monsoon cyclones. The sensible heat fluxes show lower values as compared to the latent heat flux, as shown in Table S3† and Fig. 2. The fundamental source of energy for the intensification of TCs is provided by the latent heat flux, because TCs withdraw energy from the underlying ocean through latent heat flux and increase the available potential energy by the release of latent energy through convection of clouds during TCs.56,70,71 The sensible heat flux values are usually several times smaller than those of latent heat fluxes and of minor importance compared to latent heat in determining TC intensification.72,73 The higher category cyclones show a larger value of latent heat flux. As shown in Fig. 2, the wind stress during the post-monsoon cyclones is predominant for most higher category TCs. Sometimes high evaporation may not be observed during the cyclone passage due to intense cooling at the surface, although strong winds are present. The surface heat flux facilitates the SST drop by means of vertical mixing and evaporation. The oceanic feedback to the surface heat flux is nearly one-dimensional, owing to rapid mixing in the mixed layer.
The complete analyses of the cyclones occurred over the bay during post-monsoon are given in Tables S2 and S3.† They show the largest cooling during the cyclones BOB04 in 1983 (3.46 °C), Titli (3.28 °C), Hudhud (2.99 °C), BOB04 in 1984 (2.94 °C), Madi (2.57 °C), BOB04 in 1988 (2.28 °C) and Phailin (2.25 °C), and most show a drop greater than 0.5 °C, except for the ten cyclones as marked in Table S3.† The MLD for these cyclones was 10.53 m, 28.73 m, 11.65 m and 10.56 m, and the highest MLD was for the cyclone Phethai, about 29.45 m. Since Chacko31 has taken the value (2.9 °C) from a latitude–longitude region closer to the track, our analysis given in Table S2† (2.99 °C) shows a good agreement for Hudhud. Our results are consistent with those found in previous studies too. For instance, Vidya et al.,21 Navaneeth et al.,74 and Sarangi et al.75 documented a cooling of about 2 °C by Phailin, as also shown in our analysis. Vidya et al.21 reported 2.5 °C cooling by Thane, which shows a difference of almost 1 °C with our estimates. However, Reddy et al.76 reported a cooling of 2.5 °C by the cyclone BoB06 (1999), although we find a slightly lower cooling (1.23 °C) here. Rao et al.65 observed a SST drop of 2.08 °C by BoB05 (2000), but we found it to be 0.85 °C during the same period. Note that Phailin, Helen, Lehar and Madi were the four consecutive cyclones that occurred in 2013, and they showed SST drops of 2.25 °C, 0.50 °C, 0.67 °C and 2.57 °C, respectively, as analysed by Sarangi et al.,75 and are in agreement with our estimates. Conversely, Ye et al.77 reported a cooling of 4 °C by Vardah, four times higher cooling than that found in our study. The difference in estimated cooling among different analyses can be due to the differences in data (e.g., Buoy data, AVHRR and OISST), period of the analyses (e.g., different dates of surface and satellite measurements) and spatial and temporal averaging of the data.
The average SST cooling for post-monsoon cyclones is about 1.31 °C/1.44 °C (AVHRR/OISST). During post-monsoon, the SST cooling is smaller than that in pre-monsoon. Therefore, the time period is not long enough to get back to the pre-cyclone SST conditions (i.e., recovery period). The average cold wake recovery time for post-monsoon cyclones is about 8.5 days (Table S3†). The average translational speed of post-monsoon cyclones is about 4.81 m s−1. It is evident (Fig. 2) that the slow-moving cyclones induce more cooling. The lifetime of post-monsoon cyclones is 5.25 days, and most are moderately fast. These results are slightly different from those reported for Sidr (2.5 °C) by Maneesha et al.78 and for Thane (2.5 °C) by Vidya et al.,21 which can be due to the differences in the data used and the period of analyses in both studies. However, the values are comparable with those derived from the OISST data in the case of Sidr.
Our analyses show that the cyclones that occurred in the northern bay during post-monsoon have a near absence of noticeable SST drop along the track.25 In addition, the slow progressing TCs produce greater cooling as they impart stronger momentum on the surface of the ocean that finally leads to vigorous vertical mixing of warm surface water with colder deep water.27,79 This gives rise to a sustained negative SST feedback, which can effectively limit further intensification of TCs. Therefore, the information presented in this study is useful for TC-forecasting models as we have analysed the TCs that occurred over the bay in the past 39 years in two different cyclone seasons.
3.3 El Niño, La Niña and cyclone activity
Since the SST and cyclogenesis in BoB also depend on the El Niño and La Niña events, it is expected that there will be modifications in cyclone frequency and intensity due to these events.80–86 Wang et al.87 showed that tropical cyclones tend to be more powerful in El Niño years over the South China Sea. According to Hsu et al.88 the lengths of the track, lifetime and area coverage of typhoons are longer/larger in the El Niño years than those in the La Niña years in the western Pacific. To examine the impact of ENSO conditions on TC activity, we analysed the cyclones (pre- and post-monsoon) in the normal, El Niño and La Niña years using the Oceanic Niño Index.89 Out of the 102 cyclones, 27 occurred during El Niño, 38 in La Niña and 37 in normal years. Fig. 4 shows the cold wake composite of SST for the cyclones that occurred in the El Niño, La Niña and normal years. The cyclone induced cooling is more regional, and this could be also due to the influence of cyclone tracks as shown in the figure. For instance, the La Niña composite shows more cooling towards the north and northwestern bay. Therefore, it is very likely that the lower SST can be cyclone-induced. Conversely, the SST composite for normal years shows more cooling towards the central bay. The contrasting features in the SST cooling composite during El Niño and La Niña are due to the difference in the number of cyclones. Similarly, the SST is higher in BoB than that in AS, and TC activity is enhanced/suppressed in accordance with the changes in SST during El Niño, La Niña and normal years. In general, the number of cyclones is more (less) in La Niña (El Niño) years. The variability in the MLD is also affected by these events, and the average MLD during the El Niño, La Niña and normal years is 14.78, 15.92 and 14.28 m, respectively, although these differences in MLD are not statistically significant.
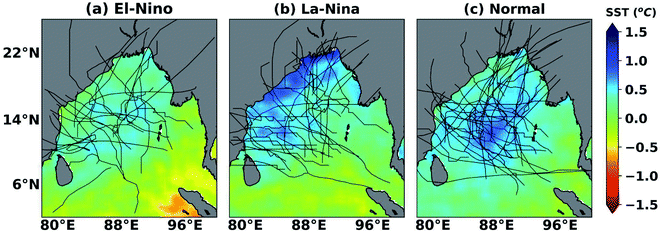 |
| Fig. 4 The sea surface-cooling composite of cyclones during El Niño, La Niña and neutral years including both pre-monsoon and post-monsoon seasons. The SST (AVHRR) composite of the study period for El Niño, La Niña and normal years (the respective cyclone tracks are also shown). | |
According to Bhardwaj et al.,85 about 83% of the total land-falling TCs have a westward motion and make landfall in the eastern coast of India during the El Niño years. In the La Niña years, 73% of the total land-falling TCs curved towards northwest and northward, and made landfall north of 17° N in the east coast of India, Bangladesh and Myanmar, as also shown in our analyses. The frequency of intense cyclones (category 2, 3, 4 and 5) is higher in the La Niña years (Table S3†), as compared to that in the El Niño years.84,90 The cause of more frequent cyclones in the La Niña years might be the resultant of remnants of TCs formed in the northwest Pacific.85 TCs that occur during La Niña travel longer distances and usually mature before their landfall, but an opposite scenario is observed for those in the El Niño years. The average lifetime of TCs in the La Niña year is about 8 days, which is longer than that of TCs in El Niño (5 days) and normal (4 days) years. The lifetime of all cyclones is given in Table S3.† The average translational speed in the La Niña years is about 4.965 m s−1, whereas it is 4.435 m s−1 and 5.087 m s−1 for El Niño and normal years, respectively.
The SST anomalies are slightly higher in the El Niño (>0.25 °C) years,82 and the average SST is higher (>28 °C) than the cyclogenesis threshold of 26.5 °C in both the El Niño and La Niña conditions over BoB. In the La Niña years, the comparatively strong winds of cyclones can cause high evaporation and enhanced convection that lead to an increased latent flux and higher SST drop.81 Nevertheless, the La Niña years favour more cyclonic activity over BoB, because of the low-level cyclonic vorticity and higher moisture content. On the other hand, the comparatively weaker winds in the El Niño years do not cool the southern BoB.81 The convective activity over the eastern BoB is reduced (enhanced) in response to the eastward (westward) motion of the rising limb of the Walker Cell due to the higher (lower) SST in the central Pacific during El Niño (La Niña) years. This acts as a precondition for TC development through increased moisture and its uplift there.81
Table 1 shows that in the El Niño years, SST and EPV and SST and TC intensity show a high positive correlation of 0.5 and 0.5, respectively, whereas the net heat flux and MLD show a high negative correlation. In the La Niña years, all parameters show a weaker correlation with SST. However, the wind stress and EPV show higher positive correlations of 0.53 and 0.55, respectively, in the normal years, but other parameters except latent heat flux (0.63) show a weaker correlation with SST. In both seasons, SST and translational speed exhibit a high negative correlation; reiterating the stronger relationship between the SST drop and translational speed. In addition, there is also a high negative correlation between the net flux and SST, as larger fluxes would reduce the SST drop associated with cold wakes.55 The analysis shows that there is a strong inter-annual variability in translational speed, and the pre-monsoon season exhibits an increasing trend in the translational speed. The other parameters show a weak correlation with SST in post-monsoon.
Table 1 Correlation of parameters influencing SST cooling. The correlation of SST with different parameters such as translational speed (TS), Ekman pumping velocity (EPV), mixed layer depth (MLD), wind stress (Tau), net heat flux (NHF), latent heat flux (LHF), sensible heat flux (SHF) and intensity (INT). The statistically significant values are given in bold
Correlation |
El-Niño |
La-Niña |
Normal |
Pre-monsoon |
Post-monsoon |
SST vs. TS |
−0.21 |
−0.21 |
−0.04 |
−0.21 |
−0.21 |
SST vs. EPV |
0.50
|
0.02 |
0.55
|
0.42
|
0.42
|
SST vs. MLD |
−0.31 |
−0.07 |
0.20 |
−0.09 |
−0.09 |
SST vs. NHF |
−0.19 |
0.008 |
−0.20 |
−0.04 |
−0.04 |
SST vs. LHF |
−0.04 |
−0.01 |
0.63 |
0.07 |
0.07 |
SST vs. SHF |
0.15 |
−0.11 |
−0.16 |
0.17 |
0.17 |
SST vs. INT |
0.51
|
0.32 |
0.05
|
0.48
|
0.48
|
SST vs. Tau |
0.24 |
0.31 |
0.52
|
0.27 |
0.27
|
4. Conclusions and implications
We have analysed the magnitude of cyclone-induced surface cooling in the bay for the past four decades (1982–2020). Our analysis shows that the degree of SST drop strongly depends on the TC intensity, translational speed and ocean mixed layer. (i) The SST cooling along the track of pre-monsoon cyclones is larger than that of the post-monsoon season. (ii) The frequency of occurrence of TCs is higher during post-monsoon. In the post-monsoon, the peak cooling is observed over the southern bay, because the barrier layer is shallow or absent in almost all months unlike in the northern bay where the absence of the barrier layer is found only during pre-monsoon; indicating a possible reason for more frequent landfall of cyclones in the northern bay in pre-monsoon. (iii) Translational speed has a crucial role in the evolution of tropical cyclones, as the cyclone-induced cooling is small for the cyclones with higher translational speed. (iv) During the cyclone period, the EPV is positive, and tropical storms and category 1 cyclones imply higher upwelling than that of higher category cyclones. (v) The La Niña years exhibit more intense cyclones than those in the El Niño years, as assessed from the available measurements presented in this study. Our results have the potential to improve cyclone forecasting using coupled weather prediction models and climate change impact studies, as TCs are expected to change in frequency and intensity in the context of climate change. This is crucial for a region in which about 6% of the total annual cyclones in the world occur and eventually affect millions of people in the coastal regions of India and its neighbourhood.
Data availability
The data used in this study are publicly available. The analysis codes can be provided on request. The ERA5 atmospheric reanalysis and global physical analysis data are available at the Copernicus Climate Change Service's Climate Data Store (http://cds.climate.copernicus.eu) and https://resources.marine.copernicus.eu/; JTWC best-track data are available on http://www.usno.navy.mil/JTWC/best-track-archive, and the AVHRR – Only are acquired from http://las.incois.gov.in. The Ocean Niño Index is available on (https://origin.cpc.ncep.noaa.gov/products/analysis_monitoring/ensostuff/ONI_v5.php). The authors are happy to share the data used in the manuscript on request. Since the satellite data used are already freely available on public domains, the analysed data can also be provided for any scientific study.
Author contributions
JK: conceptualization, methodology, software analysis, validation, formal analysis, investigation, resources, data curation, writing – original draft, writing – review & editing, visualization, supervision, project administration, and funding acquisition. AR: methodology, software analysis, validation, formal analysis, investigation, resources, data curation, writing – original draft, writing – review & editing, and visualization. MVM, GK, MM, BBS, KM, and SN: methodology, software analysis, validation, formal analysis, investigation, resources, data curation, writing – original draft, writing – review & editing, and visualization. AC: supervision, project administration, funding acquisition, and writing – review & editing.
Conflicts of interest
The authors confirm that there are no known conflicts of interest associated with this article.
Acknowledgements
We thank the Department of Science and Technology for the SPLICE project, Ministry of Education, and Indian Institute of Technology Kharagpur, National Institute of Ocean Technology, Chennai, National Centre for Ocean Information Services, Hyderabad and Ministry of Earth Sciences for facilitating the study. We also thank all the data managers and the scientists who made available the data for this study.
References
-
G. J. Holland, Global Guide to Tropical Cyclone Forecasting, WMO/TD-No. 560, Report No, TCP-31, WMO, Geneva, Switzerland, 1993 Search PubMed.
- J. Kuttippurath, N. Sunanda, M. V Martin and K. Chakraborty, Tropical storms trigger phytoplankton blooms in the deserts of north Indian Ocean, npj Clim. Atmos. Sci., 2021, 4, 11 CrossRef CAS.
- P. Peduzzi, B. Chatenoux, H. Dao, A. De Bono, C. Herold, J. Kossin, F. Mouton and O. Nordbeck, Global trends in tropical cyclone risk, Nat. Clim. Change, 2012, 2, 289–294 CrossRef.
- K. Balaguru, G. R. Foltz, L. R. Leung, E. D'Asaro, K. A. Emanuel, H. Liu and S. E. Zedler, Dynamic Potential Intensity: An improved representation of the ocean's impact on tropical cyclones, Geophys. Res. Lett., 2015, 42, 6739–6746 CrossRef.
- K. A. Emanuel, An air-sea interaction theory for tropical cyclones. Part I: Steady-state maintenance, J. Atmos. Sci., 1986, 43, 585–605 CrossRef.
- J. F. Price, Upper ocean response to a typhoon, J. Phys. Oceanogr., 1981, 11, 153–175 CrossRef.
- K. Balaguru, P. Chang, R. Saravanan, L. R. Leung, Z. Xu, M. Li and J. S. Hsieh, Ocean barrier layers' effect on tropical cyclone intensification, Proc. Natl. Acad. Sci. U. S. A., 2012, 109(36), 14343–14347 CrossRef CAS PubMed.
- K. S. Mogensen, L. Magnusson and J.-R. Bidlot, Tropical cyclone sensitivity to ocean coupling in the ECMWF coupled model, J. Geophys. Res.: Oceans, 2017, 122, 4392–4412 CrossRef.
- M. A. Bender, I. Ginis and Y. Kurihara, Numerical simulations of tropical cyclone-ocean interaction with a high-resolution coupled model, J. Geophys. Res., 1993, 98, 245–268 Search PubMed.
- M. F. Jansen, R. Ferrari and T. A. Mooring, Seasonal versus permanent thermocline warming by tropical cyclones, Geophys. Res. Lett., 2010, 37, L03602 CrossRef.
- J. F. Price, J. Morzel and P. P. Niiler, Warming of SST in the cool wake of a moving hurricane, J. Geophys. Res., 2008, 113, C07010 CrossRef.
- L. K. Shay, P. G. Black, A. J. Mariano, J. D. Hawkins and R. L. Elsberry, Upper Ocean Response to Hurricane Gilbert, J. Geophys. Res.: Oceans, 1992, 97(C12), 20227–20248 CrossRef.
- D. F. Leipper, Observed ocean conditions and Hurricane Hilda, 1964, J. Atmos. Sci., 1967, 24, 182–186 CrossRef.
- X. Yue, B. Zhang, G. Liu, X. Li, H. Zhang and Y. He, Upper ocean response to typhoon Kalmaegi and Sarika in the South China Sea from multiple-satellite observations and numerical simulations, Remote Sens., 2018, 10, 348 CrossRef.
- T.-L. Chiang, C.-R. Wu, L.-Y. Oey and T. Kai-Tak, An ocean's perfect storm, J. Phys. Oceanogr., 2011, 41, 221–233 CrossRef.
- S. Shang, L. Li, F. Sun, J. Wu, C. Hu, D. Chen, X. Ning, Y. Qiu, C. Zhang and S. Shang, Changes of temperature and bio-optical properties in the South China Sea in response to Typhoon Lingling, 2001, Geophys. Res. Lett., 2008, 35, L10602 CrossRef.
- I. Lin, W. T. Liu, C.-C. Wu, G. T. F. Wong, C. Hu, Z. Chen, W.-D. Liang, Y. Yang and K.-K. Liu, New evidence for enhanced ocean primary production triggered by tropical cyclone, Geophys. Res. Lett., 2003, 30(13), 1718 Search PubMed.
- K. Emanuel, Evidence that hurricanes are getting stronger, Proc. Natl. Acad. Sci., 2020, 117, 13194–13195 CrossRef CAS PubMed.
- G. O. P. Obasi, WMO's programme on tropical cyclone, Mausam, 1997, 48, 103–112 CrossRef.
-
X. Li, S. Yang, H. Wang, X. Jia, A. Kumar, X. Li, S. Yang, H. Wang, X. Jia and A. Kumar, A Dynamical-statistical Forecast Model for the Annual Frequency of Western Pacific Tropical Cyclones Based on the NCEP Climate Forecast System Version 2, Wiley Online Libr., 2013, vol. 118, pp. 61–73 Search PubMed.
- P. J. Vidya, S. Das and M. Murali, Contrasting Chl-a responses to the tropical cyclones Thane and Phailin in the Bay of Bengal, J. Mar. Syst., 2017, 165, 103–114 CrossRef.
- S. Neetu, M. Lengaigne, E. M. Vincent, J. Vialard, G. Madec, G. Samson, M. R. Ramesh Kumar and F. Durand, Influence of upper-ocean stratification on tropical cyclone-induced surface cooling in the Bay of Bengal, J. Geophys. Res.: Oceans, 2012, 117, 12020 CrossRef.
- S. S. C. Shenoi, Differences in heat budgets of the near-surface Arabian Sea and Bay of Bengal: Implications for the summer monsoon, J. Geophys. Res., 2002, 107, 1–14 CrossRef.
-
D. Longshore, Encyclopedia of Hurricanes, Typhoons, and Cyclones, Infobase Publishing. 2008 Search PubMed.
- D. Sengupta, B. R. Goddalehundi and D. S. Anitha, Cyclone-induced mixing does not cool SST in the post-monsoon north Bay of Bengal, Atmos. Sci. Lett, 2008, 9, 1–6 CrossRef.
- B. Subrahmanyam, V. S. N. Murty, R. J. Sharp and J. J. O'Brien, Air-sea coupling during the tropical cyclones in the Indian Ocean: A case study using satellite observations, Pure Appl. Geophys., 2005, 162, 1643–1672 CrossRef.
- I. I. Lin, C. C. Wu, I. F. Pun and D. S. Ko, Upper-Ocean Thermal Structure and the Western North Pacific Category 5 Typhoons. Part I: Ocean Features and the Category 5 Typhoons' Intensification, Mon. Weather Rev., 2008, 136, 3288–3306 CrossRef.
- R. K. Sarangi, Impact of cyclones on the Bay of Bengal chlorophyll variability using remote sensing satellites, Indian J. Geo-Mar. Sci., 2011, 40, 794–801 Search PubMed.
- R. K. Sarangi, M. K. Mishra and P. Chauhan, Remote Sensing Observations on Impact of Phailin Cyclone on Phytoplankton Distribution in Northern Bay of Bengal, IEEE J. Sel. Top. Appl. Earth Obs. Remote Sens., 2015, 8, 539–549 Search PubMed.
- E. M. Vincent, M. Lengaigne, G. Madec, J. Vialard, G. Samson, N. C. Jourdain, C. E. Menkes and S. Jullien, Processes setting the characteristics of sea surface cooling induced by tropical cyclones, J. Geophys. Res.: Oceans, 2012, 117, C02020 Search PubMed.
- N. Chacko, Chlorophyll bloom in response to tropical cyclone Hudhud in the Bay of Bengal: Bio-Argo subsurface observations, Deep Sea Res., Part I, 2017, 124, 66–72 CrossRef CAS.
-
J.-H. Chu, C. R. Sampson, A. S. Levine and E. Fukada, The Joint Typhoon Warning Center Tropical Cyclone Best-Tracks, 1945–2000, ref. NRL/MR/7540-02, 2002 Search PubMed.
- R. W. Reynolds, T. M. Smith, C. Liu, D. B. Chelton, K. S. Casey and M. G. Schlax, Daily high-resolution-blended analyses for sea surface temperature, J. Clim., 2007, 20, 5473–5496 CrossRef.
- R. W. Reynolds and T. M. Smith, Improved global sea surface temperature analyses using optimum interpolation, J. Clim., 1994, 7, 929–948 CrossRef.
- H.-M. Zhang, Bias characteristics in the AVHRR sea surface temperature, Geophys. Res. Lett., 2004, 31, L01307 Search PubMed.
- W. Mei, C. Pasquero and F. Primeau, The effect of translation speed upon the intensity of tropical cyclones over the tropical ocean, Geophys. Res. Lett., 2012, 39, L07801 CrossRef.
- J. C. L. Chan and W. M. Gray, Tropical cyclone movement and surrounding flow relationships, Mon. Weather Rev., 1982, 110, 1354–1374 CrossRef.
- V. Ch and A. A. Deo, Study of upper ocean parameters during passage of tropical cyclones over Indian seas, Int. J. Remote Sens., 2019, 40, 4683–4723 CrossRef.
- H. Hersbach, B. Bell, P. Berrisford, S. Hirahara, A. Horányi, J. Muñoz-Sabater, J. Nicolas, C. Peubey, R. Radu, D. Schepers, A. Simmons, C. Soci, S. Abdalla, X. Abellan, G. Balsamo, P. Bechtold, G. Biavati, J. Bidlot, M. Bonavita, G. Chiara, P. Dahlgren, D. Dee, M. Diamantakis, R. Dragani, J. Flemming, R. Forbes, M. Fuentes, A. Geer, L. Haimberger, S. Healy, R. J. Hogan, E. Hólm, M. Janisková, S. Keeley, P. Laloyaux, P. Lopez, C. Lupu, G. Radnoti, P. Rosnay, I. Rozum, F. Vamborg, S. Villaume and J. Thépaut, The ERA5 global reanalysis, Q. J. R. Meteorol. Soc., 2020, 146, 1999–2049 CrossRef.
- R. L. Smith, Upwelling, Ocean. Mar. Biol. Ann. Rev., 1968, 6, 11–46 Search PubMed.
- B. Praveen Kumar, J. Vialard, M. Lengaigne, V. S. N. Murty and M. J. McPhaden, TropFlux: Air-sea fluxes for the global tropical oceans-description and evaluation, Clim. Dyn., 2012, 38, 1521–1543 CrossRef.
- B. Praveen Kumar, J. Vialard, M. Lengaigne, V. S. N. Murty, M. J. McPhaden, M. F. Cronin, F. Pinsard and K. Gopala Reddy, TropFlux wind stresses over the tropical oceans: Evaluation and comparison with other products, Clim. Dyn., 2013, 40, 2049–2071 CrossRef.
- K. A. Emanuel, Sensitivity of Tropical Cyclones to Surface Exchange Coefficients and a Revised Steady-State Model incorporating Eye Dynamics, J. Atmos. Sci., 1995, 52, 3969–3976 CrossRef.
- M. M. Alam, M. A. Hossain and S. Shafee, Frequency of Bay of Bengal cyclonic storms and depressions crossing different coastal zones, Int J Climatol, 2003, 23, 1119–1125 CrossRef.
- V. S. N. Murty, B. Subrahmanyam, L. V. G. Rao and G. V Reddy, Seasonal variation of sea surface temperature in the Bay of Bengal during 1992 as derived from NOAA-AVHRR SST data, Int. J. Remote Sens., 1998, 19, 2361–2372 CrossRef.
- A. K. Jaswal, V. Singh and S. R. Bhambak, Relationship between sea surface temperature and surface air temperature over Arabian Sea, Bay of Bengal and Indian Ocean, J. Indian Geophys. Union, 2012, 16, 41–53 Search PubMed.
- O. P. Singh, T. M. Ali Khan and M. S. Rahman, Changes in the frequency of tropical cyclones over the North Indian Ocean, Meteorol. Atmos. Phys., 2000, 75, 11–20 CrossRef.
- U. P. Gayan Pathirana, G. Chen, T. Priyadarshana and D. Wang, Importance of vertical mixing and barrier layer variation on seasonal mixed layer heat balance in the Bay of Bengal, Ocean Sci. Discuss., 2017, 1–27 Search PubMed.
- L. Sun, Y. J. Yang, T. Xian, Z. M. Lu and Y. F. Fu, Strong enhancement of chlorophyll a concentration by a weak typhoon, Mar. Ecol.: Prog. Ser., 2010, 404, 39–50 CrossRef.
- R. L. Korty, K. A. Emanuel and J. R. Scott, Tropical cyclone-induced upper-ocean mixing and climate: Application to equable climates, J. Clim., 2008, 21, 638–654 CrossRef.
- G. E. Manucharyan, C. M. Brierley and A. V Fedorov, Climate impacts of intermittent upper ocean mixing induced by tropical cyclones, J. Geophys. Res.: Oceans, 2011, 116, C11038 CrossRef.
- L. Stoney, K. Walsh, A. V Babanin, M. Ghantous, P. Govekar and I. Young, Simulated ocean response to tropical cyclones: The effect of a novel parameterization of mixing from unbroken surface waves, J. Adv. Model. Earth Syst., 2017, 9, 759–780 CrossRef.
- V. Pant and K. R. Prakash, Response of Air–Sea Fluxes and Oceanic Features to the Coupling of Ocean–Atmosphere–Wave During the Passage of a Tropical Cyclone, Pure Appl. Geophys., 2020, 177, 3999–4023 CrossRef.
- W. Shen and I. Ginis, Effects of surface heat flux-induced sea surface temperature changes on tropical cyclone intensity, Geophys. Res. Lett., 2003, 30(18), 1933 Search PubMed.
- S. Raj Parampil, G. N. Bharathraj, M. Harrison and D. Sengupta, Observed subseasonal variability of heat flux and the SST response of the tropical Indian Ocean, J. Geophys. Res.: Oceans, 2016, 121, 7290–7307 CrossRef.
- Z. Ma, J. Fei, X. Huang and X. Cheng, The effects of ocean feedback on tropical cyclone energetics under idealized air-sea interaction conditions, J. Geophys. Res.: Atmos., 2013, 118, 9778–9788 CrossRef.
- M. M. Uddin, R. Chowdhury, S. Ahammed and S. C. Basak, Seasonal variability of mixed layer depth (MLD) in the Bay of Bengal, Indian J. Geo-Mar. Sci., 2014, 43, 400–407 Search PubMed.
- N. Akter and K. Tsuboki, Role of synoptic-scale forcing in cyclogenesis over the Bay of Bengal, Clim. Dyn., 2014, 43, 2651–2662 CrossRef.
- S. K. Sasamal, Pre-monsoon Indian Ocean SST in contrasting years of Indian summer monsoon rainfall, Int. J. Remote Sens., 2007, 28, 4403–4407 CrossRef.
- D. P. Alappattu and P. K. Kunhikrishnan, Premonsoon estimates of convective available potential energy over the oceanic region surrounding the Indian subcontinent, J. Geophys. Res., 2009, 114, D08108 CrossRef.
- R. A. Dare and J. L. McBride, Sea surface temperature response to tropical cyclones, Mon. Weather Rev., 2011, 139, 3798–3808 CrossRef.
- Z. Ma, J. Fei, X. Huang and X. Cheng, Contributions of Surface Sensible Heat Fluxes to Tropical Cyclone. Part I: Evolution of Tropical Cyclone Intensity and Structure, J. Atmos. Sci., 2015, 72, 120–140 CrossRef.
- L. Wu, Z. Wen, R. Huang and R. Wu, Possible linkage between the monsoon trough variability and the tropical cyclone activity over the western North Pacific, Mon. Weather Rev., 2012, 140, 140–150 CrossRef.
- P. K. Pothapakula, K. K. Osuri, S. Pattanayak, U. C. Mohanty, S. Sil and R. Nadimpalli, Observational perspective of SST changes during life cycle of tropical cyclones over Bay of Bengal, Nat. Hazards, 2017, 88, 1769–1787 CrossRef.
- K. H. Rao, A. Smitha and M. M. Ali, A study on cyclone induced productivity in south-western Bay of Bengal during November-December 2000 using MODIS (SST and chlorophyll-a) and altimeter sea surface height observations, Indian J. Mar. Sci., 2006, 35(2), 153–160 Search PubMed.
- M. J. McPhaden, G. R. Foltz, T. Lee, V. S. N. Murty, M. Ravichandran, G. A. Vecchi, J. Vialard, J. D. Wiggert and L. Yu, Ocean-atmosphere interactions during cyclone nargis, Eos, 2009, 90(7), 53–54 CrossRef.
- X. Wang, G. Han, Y. Qi and W. Li, Impact of barrier layer on typhoon-induced sea surface cooling, Dyn. Atmos. Oceans, 2011, 52, 367–385 CrossRef.
- N. K. Vissa, A. N. V Satyanarayana and B. P. Kumar, Response of upper ocean and impact of barrier layer on Sidr cyclone induced sea surface cooling, Ocean Sci. J., 2013, 48, 279–288 CrossRef.
- S.-H. Chou, W. Zhao and M.-D. Chou, Surface Heat Budgets and Sea Surface Temperature in the Pacific Warm Pool during TOGA COARE, J. Clim., 2000, 13, 634–649 CrossRef.
- D. S. Nolan, Y. Moon and D. P. Stern, Tropical cyclone intensification from asymmetric convection: Energetics and efficiency, J. Atmos. Sci., 2007, 64, 3377–3405 CrossRef.
- W. Hogsett and D.-L. Zhang, Numerical simulation of hurricane bonnie (1998). Part III: Energetics, J. Atmos. Sci., 2009, 66, 2678–2696 CrossRef.
- S. K. Kimball and F. C. Dougherty, The Sensitivity of Idealized Hurricane Structure and Development to the Distribution of Vertical Levels in MM5, Mon. Weather Rev., 2006, 134, 1987–2008 CrossRef.
- K. A. Hill and G. M. Lackmann, Analysis of idealized tropical cyclone simulations using the weather research and forecasting model: Sensitivity to turbulence parameterization and grid spacing, Mon. Weather Rev., 2009, 137, 745–765 CrossRef.
- K. N. Navaneeth, M. V Martin, K. J. Joseph and R. Venkatesan, Contrasting the upper ocean response to two intense cyclones in the Bay of Bengal, Deep Sea Res., Part I, 2019, 147, 65–78 CrossRef CAS.
- R. K. Sarangi, S. K. Shrinidhi, P. Chauhan and B. R. Raghavan, Remote sensing and in situ platform based study on impact of Bay of Bengal cyclones (Phailin, Helen, Lehar, and Madi) on ocean chlorophyll and associated physical parameters, Nat. Hazards, 2018, 93, 413–451 CrossRef.
- P. R. C. Reddy, P. S. Salvekar and S. Nayak, Super cyclone induces a mesoscale phytoplankton bloom in the bay of bengal, IEEE Geosci. Remote Sens. Lett., 2008, 5, 588–592 Search PubMed.
- H. J. Ye, M. A. Kalhoro, J. Sun and D. Tang, Chlorophyll blooms induced by tropical cyclone vardah in the Bay of Bengal, Indian J. Geo-Mar. Sci., 2018, 47, 1383–1390 Search PubMed.
- K. Maneesha, Y. Sadhuram and K. Prasad, Role of upper ocean parameters in the genesis, intensification and tracks of cyclones over the Bay of Bengal, J. Oper. Oceanogr., 2015, 8, 133–146 Search PubMed.
- S. E. Zedler, Simulations of the ocean response to a hurricane: Nonlinear processes, J. Phys. Oceanogr., 2009, 39, 2618–2634 CrossRef.
- E. K. W. Ng and J. C. L. Chan, Interannual variations of tropical cyclone activity over the north Indian Ocean, Int. J. Climatol., 2012, 32, 819–830 CrossRef.
- C. S. Felton, B. Subrahmanyam and V. S. N. Murty, ENSO-modulated cyclogenesis over the Bay of Bengal, J. Clim., 2013, 26, 9806–9818 CrossRef.
- M. S. Girishkumar and M. Ravichandran, The influences of ENSO on tropical cyclone activity in the Bay of Bengal during October–December, J. Geophys. Res.: Oceans, 2012, 117, C02033 CrossRef.
- M. S. Girishkumar, K. Suprit, S. Vishnu, V. P. T. Prakash and M. Ravichandran, The role of ENSO and MJO on rapid intensification of tropical cyclones in the Bay of Bengal during October–December, Theor, Appl. Climatol., 2015, 120, 797–810 CrossRef.
- B. K. Mahala, B. K. Nayak and P. K. Mohanty, Impacts of ENSO and IOD on tropical cyclone activity in the Bay of Bengal, Nat. Hazards, 2015, 75, 1105–1125 CrossRef.
- P. Bhardwaj, D. R. Pattanaik and O. Singh, Tropical cyclone activity over Bay of Bengal in relation to El Niño-Southern Oscillation, Int. J. Climatol., 2019, 39, 5452–5469 CrossRef.
- R. Rahul, J. Kuttippurath, A. Chakraborty and R. S. Akhila, The inverse influence of MJO on the cyclogenesis in the north Indian Ocean, Atmos. Res., 2021, 265, 105880 CrossRef.
- C. Wang and X. Wang, Classifying El Niño Modoki I and II by different impacts on rainfall in southern China and typhoon tracks, J. Clim., 2013, 26, 1322–1338 CrossRef.
- P.-C. Hsu, C.-R. Ho, S.-J. Liang and N.-J. Kuo, Impacts of two types of El Niño and La Niña events on typhoon activity, Adv. Meteorol., 2021, 99(4), 1071–1088 Search PubMed.
- K. E. Trenberth and D. P. Stepaniak, Indices of el Niño evolution, J. Clim., 2001, 14, 1697–1701 CrossRef.
- M. Mohapatra and V. Vijay Kumar, Interannual variation of tropical cyclone energy metrics over North Indian Ocean, Clim. Dyn., 2017, 48, 1431–1445 CrossRef.
Footnote |
† Electronic supplementary information (ESI) available. See DOI: 10.1039/d1ea00066g |
|
This journal is © The Royal Society of Chemistry 2022 |