Pre-monsoon submicron aerosol composition and source contribution in the Kathmandu Valley, Nepal†
Received
29th January 2022
, Accepted 28th May 2022
First published on 14th June 2022
Abstract
The Kathmandu Valley in Nepal suffers from unhealthy air quality, with mean pre-monsoon submicron particulate matter concentration (PM1) of 40 μg m−3 and daily peaks over 75 μg m−3. A high-resolution time-of-flight aerosol mass spectrometer (HR-ToF-AMS) measured ambient non-refractory submicron PM (NR-PM1) in a Kathmandu suburb in April 2015. The mean NR-PM1 composition was 15.8 μg m−3 (39.7%) organic aerosol (OA), 10.5 μg m−3 (25.0%) BC, 8.4 μg m−3 (21.1%) SO42−, 2.96 μg m−3 (7.4%) NH4+, 1.22 μg m−3 (3.1%) NO3−, and 0.92 μg m−3 (2.3%) Cl−. NR-PM1 exhibited a diurnal pattern with concentration maxima at 08:00 local standard time. Afternoon winds and an elevated planetary boundary layer then mitigated NR-PM1. Positive matrix factorization (PMF) of OA mass spectral data identified four primary source components: hydrocarbon-like OA (HOA, 15%), biomass burning OA (BBOA, 15%), trash burning OA (TBOA, 11%), a local sulfur-containing OA (sLOA, 7%), and three oxidized OA (OOA, 50%) factors. sLOA contains the ion C3HSO+, associated with emissions from coal used in brick kilns. Secondary aerosols account for 50% of all OA mass within the valley. Gas-phase carbon monoxide (CO), carbon dioxide (CO2), sulfur dioxide (SO2), nitrogen dioxide (NO2), ozone (O3), methane (CH4), and black and brown carbon (BC, BrC) measurements also characterized pollution in the valley. Composition, concentration, and source analysis from AMS-PMF and filter-based chemical mass balance measurements agree well (OC slope = 1.05, R2 = 0.47). This work identifies and quantifies significant sources of PM1 in the Kathmandu Valley and provides insight into the impact of these sources on overall air quality throughout South Asia.
Environmental significance
Air pollution is a global problem that significantly impacts the health of the majority of the world's population. Aerosol particles are one of the dominant forms of air pollution impacting human health, and it is therefore important to quantify not only the concentration, but also the main sources of aerosols in urban areas so that informed mitigation strategies can be designed. This work is focused on the measurement of the chemical composition of particulate matter and the subsequent identification and quantification of the sources of aerosols in the Kathmandu Valley of Nepal. During the pre-monsoon period we measure submicron aerosol mass and identify contributions to aerosol concentration from direct emissions such as traffic, brick kilns, biomass, and trash burning to organic aerosol and black carbon concentrations.
|
Introduction
The Kathmandu Valley in the Himalayan mountains of Nepal had a population of over three million people in 2011 (10% of the country's total population) and is subject to unhealthy air pollution with a mean daily maximum of particulate matter (PM) less than 10 microns (PM10) up to 294 μg m−3 and PM less than 2.5 microns (PM2.5) up to 207 μg m−3.1,2 The impact from numerous poorly controlled local pollution sources combines with regional transport from outside the Kathmandu Valley, including the heavily populated Indo-Gangetic Plain. This combination leads to high concentrations of PM, including black carbon (BC) and organic carbon (OC) (ref. 3–7) and gaseous air pollutants like volatile organic compounds (VOC), ozone (O3), and carbon monoxide (CO).5,8–12 Aerosol concentrations have increased continuously over the last 15 years in the Kathmandu Valley,13 and these elevated concentrations have contributed to severe adverse health impacts.14 Over 10% of Nepali fatalities are from lung disorders; this is the most common cause of death in the country.15
Inadequate supply chains promote the use of locally available fuel sources. Wood, charcoal, agricultural residue, and dung are all used as a principal energy source for cooking and home heating throughout South and Southeast Asia.16–20 These residential combustion sources increase indoor exposure for the population. This increase in indoor exposure is a significant cause of aerosol-related mortality in the region.18,21–24 Indoor pollutants are transported to the outdoor environment and contribute to ambient concentrations, which have regional air quality and climate impacts.25–28 Industry in the region uses biofuels19 that impact regional air quality and climate.
The relative contributions of distinct, local sources across South Asia need to be better characterized to develop reliable emission inventories and drive mitigation strategies and reduce exposure to harmful air pollutants. Understanding the regional and local sources, such as the impact from known primary sources like coal, garbage, or agricultural burning, generators, and vehicle fleets, is a major step in developing mitigation strategies.19,28,29
The Nepal Ambient Monitoring and Source Testing Experiment of 2015 (NAMaSTE1) characterized under-sampled pollution sources and their impact on overall air quality in the Kathmandu Valley. The deployment of a high-resolution time-of-flight aerosol mass spectrometer (HR-ToF-AMS)30,31 in the Kathmandu Valley during the NAMaSTE1 campaign was among the first such deployments in the Himalayan mountains and South Asia region. Previously published NAMaSTE1 studies quantified and characterized sources and analyzed emissions from brick kilns, generators, diesel pumps, motorcycles, cookstoves, and garbage, agricultural, and biomass burning. These studies also measured aerosol optical properties, PM2.5 mass and composition, mass spectra, emission factors (EF), size distribution, and ∼100 trace gases.32–34 The properties of the source emissions and ambient aerosol composition were essential in the analysis of this dataset. Organic molecular marker-based chemical mass balance (OMM-CMB) modelling estimated the contribution of various sources to ambient PM2.5 and PM10 from multiple-hour filter samples.35 As another part of the fixed-site ambient air quality component of NAMaSTE1, this work presents the first detailed high time resolution investigation of daily cycles of aerosol constituents and source contributions and the impact of shorter time frame events in the Kathmandu Valley. In this approach, characteristic mass spectra generated from the NAMaSTE1 source measurements help identify the significant sources in ambient measurements.
NAMaSTE1 sampled ambient air at Bode in the eastern suburban part of the Kathmandu Valley in April (pre-monsoon) 2015. The Bode site was also where the Sustainable Atmosphere for the Kathmandu Valley (SusKAT) campaign was centered in winter 2012.10,12,36 Prior studies have established that westerly and southwesterly winds in the valley drive diurnal pollutant concentrations in the late morning to afternoon.5,8,37–39 The westerly wind is the primary removal method but also introduces pollutants formed outside of the Kathmandu Valley. Overnight, there is confinement by the shallow boundary layer and stagnation due to temperature inversions, leading to air quality degradation.6,8,37,38,40 Analysis of bulk aerosol samples found that most primary fine aerosols were from burning solid fuels like biomass and trash.35,41 Secondary OA (SOA) makes up the bulk of PM2.5 in the Kathmandu Valley.35 The abundance of O3 in Kathmandu is a major factor in SOA formation.5
This study's primary goals are to measure the concentration, characterize the daily cycles in composition, and apportion OA for ambient PM1 in the Kathmandu Valley, Nepal, based on measurements during the pre-monsoon month of April 2015. A regular afternoon wind of 3 m s−1, minimal rain, and moderate temperatures (19.2 °C) dominated the meteorology during this period. These characterizations will provide context for emissions inventories, relate ambient monitoring to existing source measurements, and identify prominent PM1 sources to support the scientific basis for mitigation.
Experimental methods
NAMaSTE1 campaign
The ambient measurement portion of the NAMaSTE1 campaign took place in the Kathmandu Valley in Nepal. This work characterized emissions from various local sources and monitored ambient concentrations at Bode in Madhyapur, in the Kathmandu Valley, during April 13–24 of 2015. The magnitude 8.1 Ms Ghorka earthquake on April 25, 2015, forced the ambient and emissions characterization measurements of NAMaSTE1 to end prematurely. Although measurements ended early, there are sufficient data to conduct analyses and present results. Further experiments, NAMaSTE2 in Winter 2017–2018, supplement these measurements with ambient monitoring across the Kathmandu Valley.42,43
Location description
Bode is approximately 8 km to the east north-east of the Kathmandu Valley center (27.689°N, 85.395° E, 1345 m a.s.l.). Bode is a suburban locale situated in a mixed residential, industrial, and agricultural region (Fig. 1). The Bode site is between three major urban centers, approximately 3.75 km west north-west of Bhaktapur, 8 km north-east of Lalitpur, and 10 km south-east of Kathmandu urban districts. The Araniko Highway is 1.75 km south of Bode. The Tribhuvan international airport is approximately 3.5 km west of Bode. The largest metropolitan area, Kathmandu, is about 7 km west of Bode. The Duwakot industrial region is approximately 2.25 km east-south-east of the site, a region dense with brick kilns. The Bhaktapur industrial Estate is 2 km southeast of the Bode site, which houses small-scale plastic, pharmaceutical, electronics, and textile industries.
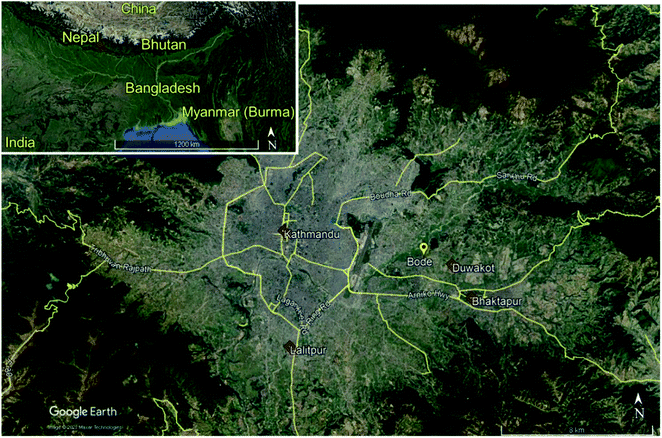 |
| Fig. 1 Map of Bode Thimi location in the Kathmandu Valley of Nepal along with important nearby locations. Subset shows the larger region of south Asia, detailing Nepal's geographical location. Background images from Google Earth. | |
The Bode location is not close to high vehicle traffic areas; the closest significant roadway is more than 500 m from the site. This distance provides time for the dilution of the roadway vehicular emissions prior to sampling. This municipality has several paved roads; however, the majority were compacted soil. Adjacent to this site were many small-scale agriculture fields and a few more extensive vegetable and wheat farms. Pronounced clusters of brick kilns are in Bhaktapur, Lalitpur, and Kathmandu, with additional sites scattered throughout the Kathmandu Valley. Most industry in the valley is within the Kathmandu or Bhaktapur industrial areas.
Instrumentation
The laboratory suite used three separate sample lines for one gas-phase and two aerosol inlets, as shown in the ESI (Fig. SI1†). The inlets sampled from an unobstructed path into both prevalent wind directions, on the third and fourth floor at approximately 12 and 15 meters above ground level (m a.g.l.). The particle sampling line was a 10 meter long ½′′ inner diameter copper line. The flow rate through this inlet was 16.67 liter per minute (LPM). This inlet had a 2.5 μm cyclone (sharp cut cyclone, Mesa Laboratories Inc., USA). This inlet stepped down to ¼′′ and split into a sample flow to instruments and a bypass exhaust flow. A 24′′ Nafion dryer (MD-070, Perma Pure LLC., USA) was installed on the aerosol sampling line to reduce relative humidity (RH) below 30%.44
Aerosol sampling and analysis
The high-resolution time-of-flight aerosol mass spectrometer (HR-ToF-AMS, Aerodyne Research Inc. USA; hereafter referenced by AMS) measured real-time non-refractory submicron PM (NR-PM1) mass loading, particle size distribution in vacuum aerodynamic diameter, and chemical composition.30,31 The AMS data were saved at 1 minute intervals from the 13th to the 24th of April 2015. Due to extended power outages and transient voltages across building infrastructure there are occasional data gaps.
Before sampling, the AMS lens was aligned and calibrated for ionization efficiency (IE) with an ammonium nitrate solution atomized and size selected at 400 nm. Two IE calibrations were performed during the campaign, on the 19th and again on the 21st. We assume a collection efficiency (CE) of 0.5 for all ambient measurements.45,46 The CE of 0.5 is assumed to be constant for the duration of the experiment, based on previous cross instrument comparisons (e.g. (ref. 31 and 47–51)). CE uncertainty is the largest source of uncertainty for individual species. We estimate that the total AMS mass concentration's overall uncertainty is 30%.52 The AMS detection limit was measured daily with 30 minutes periods of sampling through a clean, high-efficiency particulate air (HEPA) filter. This instrument zeroing period allows for calculating the detection limit, defined as 3σ of the total mass of combined filter periods.51 The detection limit for NO3− NAMaSTE1 is 63 ng m−3.
We processed data using Igor64 7.08 (Wavemetrics, Lake Oswego, OR) with HR-ToF-AMS analysis software SQUIRREL v1.62 and PIKA 1.22 A (v.1.03 E).53 We use the standard mass spectral fragmentation table to separate unit mass resolution (UMR) species.54 This fragmentation table is adjusted from default settings at mass to charge (m/z) 15, 29, and 44 to ensure that filter periods have zero particle concentration. High-resolution spectra are peak fit using PIKA.30 All regressions are performed using orthogonal distance regression.
The NR-PM1 measurements from the AMS are summed with BC measurements to determine total online combined PM1 (C-PM1) and account for refractory PM1 mass not measured by the AMS.31 All data are reported at ambient pressure and temperature.
Positive matrix factorization (PMF) was performed on the organic aerosol mass spectral matrix to differentiate organic aerosol sources.55–59 OA Mass spectral matrices were analyzed using PMF Evaluation Tool (PET version 3.04a) to differentiate sources.57 Both high resolution (HR) and unit mass resolution (UMR) solutions were processed (m/z < 200).60 Due to similarity in solution and increased resolution,57,61 only the HR results are presented here.
Inorganic species are excluded from PMF analysis and retained for a posteriori comparison of the factor time series.57 PMF factors were chosen based on the ratio of the total sum of the squares of the scaled residuals (Q) to the expected ratio (Qexp) Q/Qexp values, minimized residuals, external tracers (Fig. SI2†), and prior knowledge of the emissions profiles from AMS measurements of likely sources.
The optimal PMF solution determination involves selecting the solutions space with factor mass spectral profiles that match existing spectra, correlation with time series external to the PMF calculation, PMF diagnostics, and additional independent evidence. The mathematical parameter that allows for the rotation of the solution matrix is called the FPEAK.62 HR AMS-PMF analysis was performed from a factor size space from 1 to 10 factors and FPEAK parameter ranging from −1 to +1, in intervals of 0.1. FPEAKs outside that scope failed to converge on a solution. This PMF model error was 5%. Interpretation of a PMF solution and determination of the ideal solution is made by users. Available source profiles and time series of external tracers provide evidence to justify the selected solution space.57 PMF solutions' uncertainty can be challenging to ascertain, as addressed in previous works.57,62 A comparison between FPEAK solutions can indicate uncertainty; however, this solution had limited FPEAKs that converged to a solution.63,64
Co-located measurements
Simultaneous measurements of brown carbon (BrC) and BC concentrations (Aethalometer AE 33, Magee Scientific, USA) were performed from a rooftop measurement location in the same building. The Aethalometer (AE33) is a dual-spot, filter-based monitor with seven wavelengths (370, 470, 525, 590, 660, 880, and 950 nm) to measure light attenuation by particles collected on a filter.65 The AE33 data was recorded at 1 minute intervals and zero calibrated using a daily HEPA filter to zero-calibrate BC mass. BC calculations derive concentration from light absorption at the wavelength of 880 nm.65 BC mass is assumed to be submicron based on previous morphology emissions studies.66–68 BrC is estimated using the difference in mass calculated from the absorption of light at the 370 nm wavelength and the mass calculated using 880 nm. BrC absorption is assumed to be the source of this difference.69,70 We applied the same mass absorption coefficient (MAC) for BrC as for BC at all wavelengths. This likely underestimates BrC mass as BrC absorption is normally weaker than BC, especially after aging69,71–73 but allows for direct comparison of absorption contributions.
An environmental dust monitor measured PM1, PM2.5, and PM10 through a dedicated sample line (EDM164, GRIMM Aerosol Technik, Germany). The EDM164 measures PM1, PM2.5, and PM10 with a light scattering laser at 660 nm. The instrument samples at 1.2 L min−1 through an integrated heated inlet with Nafion dryer.
An independent 5 meter Teflon inlet for gas-phase measurements sampled the mixing ratios of (CO2), methane (CH4), CO, O3, water vapor (H2O), and nitrogen oxides (NOx). All gas-phase measurements are presented at standard temperature and pressure. Power redundancy from a lead-acid battery backup system maintained continuous operation across daily scheduled multi-hour power outages. CO, CO2, CH4, and H2O mixing ratios were measured with cavity ring-down spectroscopy (G2401, Picarro Inc, USA) and saved at 5 seconds intervals. A scrubber-less ozone monitor (model 211, 2BTechnologies, USA) measured the O3 mixing ratio using light absorption at the wavelength of 254 nm. This instrument self-zeros using nitrous oxide (N2O) gas to generate nitric oxide (NO); this removes O3 for zero measurements in a parallel cell. A nitrogen dioxide (NO2) monitor (model 405, 2BTechnologies, USA) measured light absorption of NO2 at 405 nm wavelength. This instrument measures both NO and NO2. NO is converted to NO2 by reaction with excess O3, and the difference between only NO2 and NO2 + NO(NOx) channels provided the NO concentration. We report odd oxygen (Ox) as the sum of O3 and NO2.
A weather station (Vantage Vue, Davis Instruments, USA) and wind vane (05
103, Campbell Scientific, UK) recorded measurements of ambient temperature, pressure, dew point, relative humidity, rain rate, wind speed, and wind direction at 1 minute intervals on the laboratory's rooftop at approximately 25 m a. g. l. and 7 m from instrument inlets.
Results
Meteorology
The meteorology at the Bode site for NAMaSTE1 followed the typical daily patterns for the Kathmandu Valley during the pre-monsoon season.6,37,74 The mean temperature of 19.2 °C for the sample period, ranged from a minimum of 12.9 °C to a maximum of 28.6 °C. Weather metrics time series and diurnals for the campaign are in the ESI (Table SI1, Fig. SI3 and SI4).† Temperature showed a consistent daily pattern, with cool overnights dropping to around 15 °C just before sunrise. As solar radiation heated the ground, temperatures increased to a noontime high of about 26 °C. The mean ambient pressure was 760 hPa and ranged from 755 to 767 hPa. Pressure peaked at noon Nepal standard time (NST; UTC + 05:45) with an average daily maximum of 763 hPa and a daily minimum of 758 hPa at 17:00 NST. Mean relative humidity (RH) was 80%, ranging from 44% to 100%. RH and temperature had opposite diurnal patterns. Overnight the median RH was around 90%, dropping to 55% during peak temperature and strongest wind at 12:00–14:00 NST. Meteorological data are in the ESI.†
Diurnal aerosol liquid water content (LWC) fluctuations drive partitioning between the gas or particle phase and impact aerosol pH. High overnight RH can lead to hygroscopic uptake of water and an increase in ambient particle size. Overnight increases of RH also corresponded to an increase of partitioning towards the particulate phase, increasing the formation of SOA and PM2.5 concentrations at Bode.35
Wind vectors at Bode for the duration of NAMaSTE1 were typical for the pre-monsoon season, as previously reported in Kathmandu74 and at Bode.11 The average wind speed at Bode was 1.5 m s−1 ranging from stagnant to a maximum of 10.23 m s−1 (Fig. SI5†). The wind speed diurnal minimum at 04:00 NST was 0.38 m s−1, and the daily average maximum at 15:00 NST was 4.06 m s−1. Calm mornings with weak ESE winds at 0.5 m s−1 were typical. In mid-morning, the wind direction changed, and the wind speed increased to a more significant mean 4 m s−1 from the West. This westerly wind persisted between 10:00 and 18:00 NST and was the primary method of transporting emissions out of the Kathmandu Valley.37–39,74,75 In the predawn morning, cold air stagnated, allowing pollutants from the highways, industrial areas, and residential activities near the site to accumulate.8,40,74,76 Overnight low wind speed with continuing emissions caused an increase in pollutant concentrations.6 Early morning pollutants were from the previous night's emission accumulated within a shallow boundary layer.
Aerosol mass concentrations
The mean C-PM1 concentration at the Bode site for the campaign was 39.7 ± 20.5 μg m−3 and ranged from 8 μg m−3 to 160 μg m−3 (Fig. 2). The mean filter-based PM2.5 was 68.2 ± 34.7 μg m−3, and PM10 was 119.7 ± 55.2 μg m−3 at Bode for the same period of NAMaSTE1.35 The NAMaSTE1 PM10 measurements show similarity in magnitude with the previous total suspended particulate (TSP) measurements at Bode of 155 ± 124 μg m−3 for April 2013 to March 2014.77
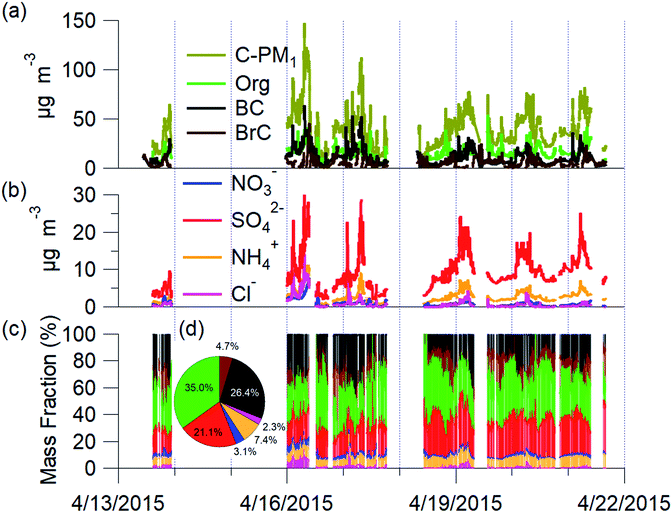 |
| Fig. 2 Time series of PM1 mass concentration and mass fraction during April 13–22, 2015 in Bode, Kathmandu Valley, Nepal: (a) AMS total, OA, refractory BC, and BrC mass concentration; (b) AMS inorganic sulfate, ammonium, chloride, and nitrate mass concentration; (c) fraction of total PM1 mass; and (d) average PM1 mass fraction. | |
The mean PM2.5 concentration at the Bode site was twice the mean PM2.5 concentration observed in the Kathmandu Valley's outskirts in the nearby foothills in Godavari in 2006 (34 μg m−3).4 PM1 alone was thirteen times higher at Bode during NAMaSTE1 than TSP was near the remote background high-altitude Mt. Everest base camp at 5057 m a.s.l. in 2006.78 Diurnal variations in C-PM1 concentration correspond to wind vector changes, photochemistry, sources, and removal processes. Overnight concentrations were consistently elevated due to confinement until the mixing layer height increased after sunrise.
The main species contributing to C-PM1 mass in the Kathmandu Valley are OA, sulfates, and nonrefractory BC aerosols. Mean PM1 was composed by mass, of 35.0% OA, 26.4% BC, 21.1% SO42−, 7.4% NH4+, 3.1% NO3−, 2.3% chloride (Cl−). OA dominated the mass fraction. All BrC was assumed real and a portion of the OA. However, Increased absorption at the 370 nm wavelength due to dust may artificially decrease the measurement used calculate to infer BrC mass. BrC was an average 4.7% of the total C-PM1 mass. C-PM1 mass concentrations time series and average mass fraction for the study duration are in Fig. 2. OA ranged daily from 28.8% to 56%, and BC, the second largest fraction, was 16.7% to 36.6% of PM1 mass. These fractions are consistent with previous AMS studies globally, where OA is often the dominant component of PM1 (e.g., ref. 79). These two species combined accounted for 66% of all C-PM1.
The ratio of C-PM1 concentration to filter-based PM2.5 concentration is 0.61 (R2 = 0.67) (Fig. SI6†). C-PM1 covers a smaller size range and does not include refractory dust. Refractory dust measured in the winter of 2018 comprised 11% of PM2.5 in urban Kathmandu.42 PM1 derived from the light-scattering optical technique (GRIMM164) showed roughly 15% more total mass than C-PM1. A comparison of C-PM1 and optical PM1 measurement showed good correlation with an R2 of 0.85. Optical measurements with the GRIMM164 of PM2.5 and PM1 showed PM2.5 as 1.18 times higher than PM1 and 1.33 times higher than C-PM1. These mass differences across techniques and size ranges are within the uncertainty range expected due to differences in mass and instrumental techniques between C-PM1 and PM2.5. This work will focus on the composition of C-PM1 measured during NAMaSTE1.
Time series of major submicron particulate species
C-PM1 in Kathmandu Valley during NAMaSTE1 had a regular daily pattern. The mean mass concentration (Table 1) and the diurnal aerosol mass fraction, concentration and composition are in Fig. 3. The average sunrise and sunset time for this experiment's duration was 5:39 and 18:34 NST.80 The diurnal pattern of C-PM1 had a daily increase from evening until morning. The C-PM1 concentration increased from late evening to an early morning maximum around 08:00 NST to an average value over 60 μg m−3 C-PM1. A rapid increase of C-PM1 concentration in the morning peaked after sunrise due to additional emissions into a shallow boundary layer.38 This enhancement happens before the mixing height rises in elevation and wind speed increases. In the afternoon when dilution from higher wind speed and the elevated boundary layer was greater than the flux of fresh emissions there is a decrease in concentrations.38 PM1 concentration dropped quickly to reach a daily minimum under 25 μg m−3 around 16:00 NST. BC reached a morning maximum of 25 μg m−3 at 08:00 NST and low concentration of 5 μg m−3 at 16:00 NST. The mass fractions of the various NR-PM1 components were relatively stable throughout the day, except for chloride. Cl− accumulated overnight, and rapidly decreased from meteorological factors noted above. All AMS species had significantly elevated concentrations in the morning.
Table 1 Summary of PM1 chemical composition in the Kathmandu Valley during the pre-monsoon, April of 2015
|
Mean |
25th ‰ |
75th ‰ |
(μg m−3) |
(μg m−3) |
(μg m−3) |
BrC is not included in the sum totals.
|
Org |
15.8 |
11.0 |
18.5 |
NH4+ |
3.0 |
1.7 |
3.4 |
Cl− |
0.9 |
0.1 |
1.3 |
NO3− |
1.2 |
0.3 |
1.14 |
SO42− |
8.4 |
6.2 |
11.2 |
BC |
10.5 |
4.5 |
14.0 |
BrCa |
5.4 |
2.3 |
7.2 |
NR-PM1 |
28.6 |
23.8 |
35.54 |
C-PM1 |
39.4 |
28.3 |
49.54 |
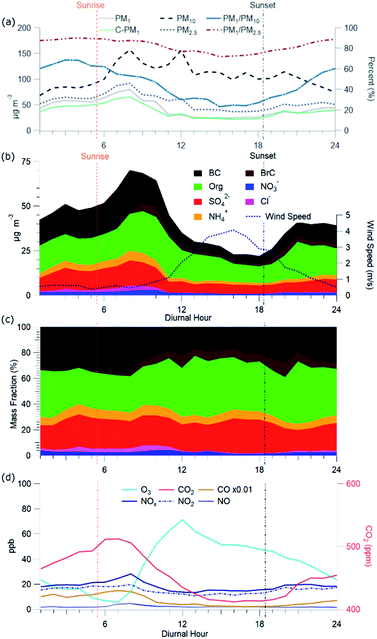 |
| Fig. 3 Diurnal pattern of (a) aerosol mass concentrations and fractions, (b) mass concentrations of AMS species, refractory BC and wind speed, (c) AMS species mass fraction of total C-PM1, (d) gas-phase species at Bode in Kathmandu Valley, Nepal in April 2015. | |
Individual components of C-PM1 have discernible diurnal patterns. Organic species had two distinct peaks, one in the morning when all species are at their highest levels and a second peak at around 20:00 NST. OA's peaks coincide with typical peak traffic hours and mealtimes. A further discussion of OA components and PMF analysis is in Section 3.4.
Nitrate and sulfate concentrations decreased rapidly between 11:00 NST to 12:00 NST. This abrupt change occurred coincident with the increase in the wind speed. Sulfate concentrations had an average of 9 μg m−3 enhancement during the morning peak over the mid-day minimum. The diurnal low concentration of sulfate was 4.7 μg m−3. This elevated background concentration is consistent with the non-volatile nature of sulfate and the more regional character of this species. The observed increase in the morning is linked to industrial emissions from brick kilns overnight as discussed later.
Cl− averages 2% of total PM1 with the lowest concentrations observed between 10:00 NST and 16:00 NST. Concentrations of Cl− increase overnight to almost 3 μg m−3; the campaign maximum concentration of Cl− of 16.13 μg m−3 occurred on the evening of the 16th. The AMS measured a relatively high mass fraction of inorganic and organic chloride in the Kathmandu Valley. Source measurements in Nepal have previously observed organic chloride as gas-phase CH3Cl.32
Chlorides in the atmosphere have numerous natural sources, primarily seawater, as well as agricultural and garbage burning, dust storms, and volcanoes. Emissions of Cl− from seawater are typically 100 times higher than other sources.81 However, as Nepal is landlocked, seawater has negligible influence in this region. Coal combustion, the open burning of garbage or grass, and residential use of dung and hardwood fuels are anthropogenic sources in South Asia.19 Crop residue burning is an agricultural method in the region.19 This practice is a potential source of inorganic and organic chlorine emissions.33 The elevated chloride concentration suggests the condensation of hydrogen chloride with counter ions such as ammonium or potassium to form inorganic salts (e.g., NH4Cl).33 The elevated RH overnight, and abundance of gas phase HCl, means there is potential for repartitioning of particulate Cl−.34
The aerosol neutralization ratio (ANR) is the ratio of aerosol cations to anions, assuming that ammonium is the only cation present. ANR is defined as:82
| 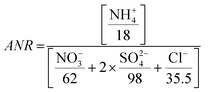 | (1) |
Aerosols in Kathmandu Valley are generally not neutralized, with an average ANR of 0.79 ± 0.04, with a 10th/90th percentile from 0.73 to 0.83 (Fig. SI7†). Despite the abundance of NH4+, elevated chloride emissions may have led to the lack of aerosols' neutralization at this location. Alternatively, the sulfate ions may be bisulfate ions (HSO4−). K+ from biomass burning or other cations can contribute to the ion balance but are not incorporated in the traditional definition of ANR. Due to these complications ANR has significant uncertainty, and therefor is only an indicator of aerosol acidity. The pH of PM2.5 aerosols during NAMaSTE1, derived from the phase partitioning of NH3, ranged from 2.2 to 3.3.35 The lack of neutralization and low pH in Kathmandu agrees with previous urban studies showing acidic pH.83,84
Organic nitrates (OrgNO3) contribute to PM and produce NO+ and NO2+ fragments in the AMS similar to inorganic nitrate but in a different ratio.85 The average ratio of NO2+ to NO+ (Rmeasured) was 0.50 ± 0.07 ranging from 0.18 to 0.72 (Fig. SI7†). This average value is like the 0.42 ± 0.02 ratio of NO2+
:
NO+ for pure NH4NO3 from calibrations (RCalib). The concentration of OrgNO3 is calculated using this ratio, assuming a detection limit of 0.1 μg m−3.87 The diurnal ratio of NO2+
:
NO+ was well correlated with wind speed (R2 = 0.77), suggesting that the formation of OrgNO3 could be related to driven by meteorological conditions. After sunset there is a decrease in ANR and increase in total concentration. Periods of low NO2+
:
NO+ under the calibration factor of 0.4, would suggest the presence of OrgNO3.86 However, while night-time conditions, with the abundance of oxidants (Fig. 3d) could favour the formation of OrgNO3 there is minimal evidence of OrgNO3 in Bode.87 OrgNO3 was below the detection for the majority of the campaign, with a maximum of 0.72 μg m−3 just after at noon on the 17th. OrgNO3 is only significant for the afternoon of the 22nd (Fig. SI7†). When present, OrgNO3 is the majority of NO3. The calculation of OrgNO3 in this situation may be driven instrumental noise during the low background within which OrgNo3 is observed. The low ambient concentration of OrgNO3 despite elevated NO3 and VOC precursors may be due to condensation onto existing SOA or aqueous particles.87 OrgNO3 is defined here as:87
|  | (2) |
Fig. 3c shows the diurnal patterns of the gas-phase species measured during NAMaSTE1. The complete time series of gas-phase species is in the ESI (Fig. SI3†). Gas-phase mixing ratio measurements of O3, NO2, and CO2 during NAMaSTE1 are similar in value to those in March 2013 to March 2014 at the same site.12,76 We observed the highest mixing ratios of combustion-related species, NOx, CO and CO2, in the morning. An early morning increase in elevation of the planetary boundary layer and afternoon ventilation from higher wind speeds mitigated overnight buildup from stagnation. O3 and NO2 are anti-correlated, as expected with daytime photochemical cycling. O3 averaged 36.1 ± 20.6 ppbv during NAMaSTE1, similar to the 43.5 ± 26.6 ppbv measured in April 2013.12 For the study duration, O3 mixing ratios peaked at a mean 71.4 ppbv around 12:00 NST and dropped to a mean 5.8 ppbv at 07:00 NST. The gas-phase NO2 mixing ratio average of 16.4 ± 4.2 ppbv ranged from 10.7 ppbv at 09:00 to 19.7 ppbv at 17:00 NST during NAMaSTE1. The NO2 mixing ratio had the same diurnal patterns, with similarly peaks, and average concentrations within 5% from the previous study at the same location.12
The CO mixing ratio was 719.2 ± 533.6 ppbv for this study, higher than in April 2013, at 667 ± 372 ppbv. CO had an average daily minimum of 229 ppbv at 17:00 NST and an average daily maximum of 1493 ppbv at 07:00 NST. CO2 for NAMaSTE1 was 450.3 ± 41.9 ppmv, ranging from 411.9 ppmv at 16:00 NST to 512.3 ppmv at 07:00 NST. These CO2 measurements are 8% higher, with a greater distribution than the average 419.3 ± 6.0 ppmv in April 2013.11
Organic aerosol source apportionment
Effective air quality improvement schemes involve understanding the overall impact of dominant pollution sources. OA is the most significant fraction of C-PM1; determining OA's primary emission sources or origins will help identify the bulk of aerosols sources. Sources of emissions that influence OA have unique mass spectral fingerprints in the AMS data. With sufficient knowledge of the constituent sources in an area, PMF can identify source factors in ambient OA.
We find that a 7-factor solution with a Q/Qexp of 2.03 accounts for 97% of total mass (Fig. SI2†). While the number of factors is higher than is typically found in PMF solutions, this is due to the higher prevalence and contribution of primary sources in the Kathmandu Valley. We found that solutions with fewer than seven factors have much higher residuals at m/z 60 and 57, and HOA is not resolved, despite being a known primary source in this region. The 8-factor solution splits C3HSO+ across two solutions, with irregular zeros in the two factor's time series and no significant reduction in residuals or Q/Qexp. The 7-factor PMF solution space both minimized the Q/Qexp and the number of distinct factors. FPEAK 0 was selected since other FPEAK values did not decrease Q/Qexp or increase correlation with tracers. The 7-factor solution at FPEAK 0 factors a residual of 1.6% ± 2.3. PMF factors are identified and linked to sources via a posteriori comparison of known m/z emissions signatures, correlations with tracer species, and time-based evidence.57,84Fig. 4 shows the seven OA components' mass spectral profiles and Fig. 5 shows the time series identified by PMF for the NAMaSTE1 campaign. Several profiles show similarity to those reported in previous studies (e.g., ref. 61 and 88).
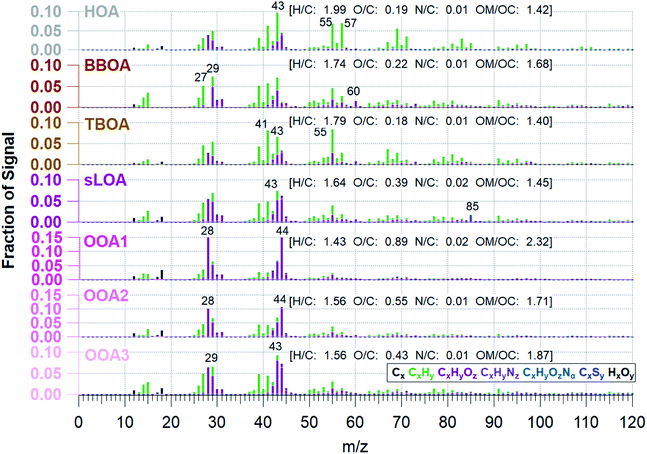 |
| Fig. 4 PMF mass spectra of the seven PMF source components and atomic ratios for AMS OA hydrocarbon-like (HOA), biomass burning (BBOA), trash burning (TBOA), sulfur-containing local OA (sLOA), oxygenated OA 1 (OOA1), oxygenated OA 2 (OOA2), and oxygenated OA 3 (OOA3), from NAMaSTE1, in the Kathmandu Nepal, in April 2015, are shown. The identifying mass to charge ratios are labeled. HR signals are colored by family. | |
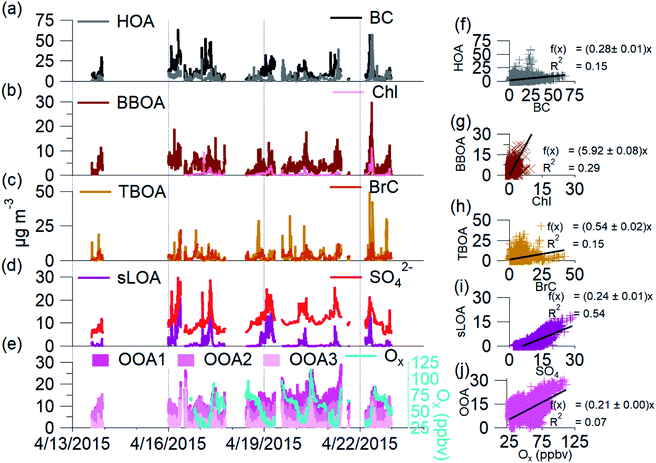 |
| Fig. 5 Time series and scatter plots of AMS-PMF OA sources and non OA tracers: (a and f) HOA, BC; (b and g) BBOA, Chl; (c and h) TBOA, BrC; (d and i) sLOA, SO4; and (e and j) OOA1, OOA2, OOA3, with Ox, for NAMaSTE1, April 2015, Kathmandu, Nepal. | |
The seven factors identified by PMF include both primary and secondary components. The primary organic aerosol factors include urban primary emissions of a hydrocarbon-like OA (HOA), a biomass burning OA (BBOA), and a trash-burning OA (TBOA). There is also a distinct local primary sulfate-containing source (sLOA) with a molar sulfur-to-carbon ratio (S/C) of 0.013, four times higher than other factors. While this S/C relationship is not calibrated, and can only roughly be compared to external measurements, internally to our measurements it is a useful indicator. For external context, this sLOA S/C is 60 times higher than that found in wintertime fresno CA, USA in 2010 (∼8 × 10−5).89 The S/C ratio from sLOA is elevated similar to that as from marine environments (0.013).90 Three distinct oxygenated OA (OOA) factors, OOA1, OOA2, OOA3, which sum to OOA, act as surrogates of SOA at different levels of oxidation detailed below.
Primary organic aerosol source factors
The primary organic aerosol factor's mass spectra at Bode, determined by PMF, are shown in Fig. 4 (Table SI3†). Source mass spectrum characterized by Goetz et al.91 and previously determined m/z ratios88 identify the PMF factors. The HOA mass spectra found in this work is like HOA found in previous works, where the ratio of CxHy ions found at m/z 55 and m/z 57 (m/z 55
:
m/z 57) was typically less than one. The m/z 55
:
m/z 57 for this HOA factor is 0.95. This HOA has a characteristic mass spectrum and a ratio of m/z 55 and m/z 57 (C4H7+ to C4H9+) less than one, indicating traffic-related emissions.92 HOA correlates to the emission spectra of motorcycles from the NAMaSTE1 emission study91 with a slope of 0.94 ± 0.037 and an R2 of 0.81 (Fig. SI8†). HOA mass spectra are traditionally associated with lubricating oil and partial combustion of hydrocarbons.59,91,93–95 HOA compared with BC has a slope of 0.276 and R2 of 0.15. HOA compared to NOx has a slope of 0.213 and an R2 of 0.07. The low R2 indicates that BC, and NOx have significant non-traffic sources.
The O/C ratio for the HOA factor is 0.19, which is similar to that of vehicles reported for Kanpur, India (0.15),96 and urban centers in Mexico and China.61,97 The O/C for HOA at Bode measured was higher than previous motor vehicle exhaust observations in laboratory experiments (0.03).98 The mean HOA/CO ratio observed in the Kathmandu Valley, 23.4 μg m−3 ppmv−1 was significantly higher than the observed mean value in Mexico City (5.7 μg m−3 ppmv−1).61,95,99
The biomass burning related organic aerosol (BBOA) has a characteristically enhanced signal at m/z 60 and m/z 73 (101). In situations where the BBOA temporal variation was similar to other factors, UMR PMF analysis of AMS data can contain residual components from BBOA; this is less of a factor for HR data due to the separation of individual m/z components.88 Biomass-burning emissions are a known source of PM2.5 in the Kathmandu Valley.35 These sources include local biomass combustion in industrial enterprise and residential food cooking101 and agricultural and regional wildfires.4,102 The region's abundance of biomass-burning sources constrains the BBOA with m/z 60, m/z 29, and a high m/z 55:57 ratio (1.79). The ion m/z 60 (C2H4O2+) is a key marker for BBOA, which comes from levoglucosan and other anhydrous sugars.100 BBOA correlates to BC with a slope of 0.274 and R2 of 0.31, UMR levoglucosan with a slope of 113.15 and R2 of 0.64, NO3 (slope = 4.99, R2 = 0.37), and HCl (slope = 8.91, R2 = 0.25). BBOA corresponds to the diurnal patterns of CO2 (slope = 0.03 R2 = 0.51), NOx (slope = 0.09, R2 = 0.74), and BrC (slope = 0.64, R2 = 0.47). BBOA correlates to emissions spectra of mixed agricultural burning (slope = 0.998, R2 = 0.78) and hardwood fuelled cookstoves (slope = 1.504, R2 = 0.76) (Fig. SI8†).
The burning of household refuse is a common waste removal technique in Nepal and other South Asian countries.103 Trash contains organic waste, paper, packaging, plastics, and metalized plastics.32 Here the TBOA is well constrained due to the abundance of immediate source plumes and similarity to source emission spectra found in NAMaSTE1.91 The AMS signal at m/z 60 used previously for BBOA is also found in small quantities from sources such as meat cooking, agricultural burning, or mixed refuse combustion.33,98 This ion is present in TBOA, likely as the pyrolysis product of cellulose from paper and cardboard in the garbage.104 TBOA correlates to diurnal patterns of BrC with a slope of 0.671 and R2 of 0.42. TBOA correlates to emission spectra of open burning of mixed garbage (slope = 0.947, R2 = 0.76), chip bags (slope = 0.933, R2 = 0.89) and plastics (slope = 0.939, R2 = 0.81) (Fig. SI9†).
Sulfur containing local organic aerosol (sLOA) is so named due to abundance of the unique ion of C3HSO+ at m/z 85. Brick kilns are a known sulfur source,105 and this exact same ion is found in brick kilns emissions from the source work in NAMaSTE1.91 sLOA is similar to other known combustion sources and includes a small contribution of m/z 60. sLOA correlates well with BC (slope = 0.224, R2 = 0.52), HCl (slope = 6.269, R2 = 0.58), NH4+ (slope = 1.149, R2 = 0.54), and SO42−(slope = 0.247, R2 = 0.54). sLOA correlates to diurnal patterns of NO (slope = 0.405, R2 = 0.58), NOx (slope = 0.041, R2 = 0.74), O3 (slope = 0.006, R2 = 0.52), CO2 (slope = 0.018, R2 = 0.76), and BC (slope = 0.095, R2 = 0.93). sLOA correlates well with emission spectra from charcoal cooking (slope = 0.902, R2 = 0.70), clamp style brick kilns (slope = 0.624, R2 = 0.43), and zig-zag style brick kilns (slope = 0.717, R2 = 0.35) (Fig. SI9†).
BBOA and TBOA mass spectra observed here may also contain contributions from cooking organic aerosol (COA). Both factors have an elevated contribution of the oxidized fragment (C3H3O+) of m/z 55, usually associated with COA.58,106 Previous studies explore the difficulty in differentiating COA from other OA factors with similar temporal trends.107
Oxygenated organic aerosols
The three OOAs in the PMF solution are typical of oxidized organic aerosol from secondary chemistry in the atmosphere with differing oxidation levels (e.g., ref. 79 and 88). The O
:
C ratios of OOA1, OOA2, and OOA3 are 0.89, 0.55, and 0.43. This ratio indicates aerosol age; the higher O
:
C, the more aged the aerosol. The three OOA mass spectra are similar to those in previous studies49,88,94 with distinct aging profiles and differences in diurnal patterns suggesting an evolution of OOA with time (e.g., ref. 79 and 95). Different processes can lead to the formation of OOA. The relationships between gas-phase emissions, key trace ions, photochemistry, and meteorological parameters drive nucleation. Comparisons with Ox, NO3, NOx, time of day, and oxygen to carbon (O
:
C) ratios can indicate OOA formation. OOA is a PMF factor identified as a close proxy of SOA49,94,108–111 and is formed very readily in urban areas with high O3.109,112,113
The diurnal sum total of OOA (OOA1 + OOA2 + OOA3) at Bode exhibits an initial increase after sunrise and continued enhancement along with NOx and O3. There is a daytime OOA correlation with odd oxygen ([Ox] = [O3] + [NO2]),114 suggesting a photochemical production of this OOA factor. OOAs have better correlations with Ox than with O3 (Fig. SI10†). O3 was the major component of Ox, 89%, during the afternoon. NO2 accounts for between 11 and 72% of Ox. NO2 contributes the most to Ox during the morning, compared to the rest of the day.
Previous studies have a significant variety of slopes of OOA to Ox. OOA/Ox, which increases with both time of day and ambient temperature, is an indicator of photochemical age.111 A lower ratio of OOA/Ox is typical within the first 10 hours of photochemistry.114 The OOA/Ox during a clean period in Pittsburgh was as low as 38 μg m−3 ppmv−1.49 Observations of a clean period in Mexico City were 30 μg m−3 ppmv−1 up to 156 μg m−3 ppmv−1.61,95,114 The elevated clean period ratio for Mexico City is likely a well-aged but diluted sample, is close to the maximum ratio of 180 μg m−3 ppmv−1 OOA/Ox previously observed in Tokyo (R2 = 0.74).115 The ratio of OOA to Ox in the Kathmandu Valley was 491 μg m−3 ppmv−1 in the daytime (R2 = 0.52). The OOA
:
Ox slope observed in the Kathmandu Valley is 2.7-fold greater than the most extreme value from Tokyo. The daytime slope of OOA1
:
Ox was 10 μg m−3 ppmv−1; OOA2
:
Ox was 275 μg m−3 ppmv−1, and OOA3
:
Ox was 185 μg m−3 ppmv−1. Only daytime OOA2 is well correlated with Ox (R2 = 0.79) and O3 (R2 = 0.72) (Fig. SI10†). The ratio of OOA
:
Ox for the more oxidized, “low volatility” and the less oxidized, “semi-volatile” PMF OOA factors in Mexico City and Zurich was 2
:
3.56,114 The relationship of OOA2 to OOA3 at Bode is the same 2
:
3 relationship. OOA2, OOA3, and Ox have a similar rise in the early AM, likely from photochemistry and vertical mixing after sunrise. OOA1 increases after these species, with a maximum several hours after the OOA2 and OOA3 peak. OOA1 increased mid-day and overnight, likely due to transport of OOA1 from outside the Kathmandu Valley and from confinement.
OOA1 is the most oxidized OOA factor resolved by PMF and appears to be a regional background OA. It has a relatively constant mass despite source and meteorological changes. The combination of a high O
:
C ratio and the stability in concentration suggests a regional aged aerosol that persists due to its lower volatility.116 The O
:
C ratio is highest for OOA1 at 0.89.
OOA2 had a slightly elevated signal at C2H4O2+ (m/z 60) compared to the other OOA factors, indicating this may be related to processed biomass burning aerosol. This ion persists for thousands of km of transport and subsequent aging.117 The increase in OOA2 occurs shortly after daily BBOA, sLOA, and TBOA peaks and in the presence of daylight and high O3 mixing ratios, suggesting potential aging of primary biomass burning aerosols. The diurnal pattern of OOA2 and Ox is the most similar and indicative of photochemical formation. The O
:
C ratio for OOA2 is 0.55. The slope of the OOA2 to Ox is 50% higher than previous observations of this relationship. The high correlation of OOA2 to Ox indicates that the duration of formation for the two is similar.114
OOA3 is the least oxidized OOA species with an O/C ratio of 0.43, implying this factor was due to early generation oxidation products partitioning into the aerosol phase. NO3− correlates highest to OOA3. Rapid NO3− formation within the plume is evidence of the volatility of OOA3.56,118 OOA3 decreases from its morning peak to the late afternoon and drives a decrease in OOA3/Ox. The slope of OOA3/Ox is among the high end of urban measurements, while the correlation of 0.23 is much lower than in other urban environments.57 Evaporation to maintain the particle-gas phase equilibrium and concentration changes from lofting due to the elevated mixing height likely drive the decrease in OOA3/Ox correlation.99
The numerous primary combustion sources in the region emit high concentrations of semi-volatile organic compounds (SVOC), fuelling SOA formation.10 The increase of ambient temperature in the afternoon drives the partitioning of semi-volatile compounds towards the gas phase. NOx is elevated overnight due to confinement and NO2–HONO conversion119 and increases after sunrise due to increased emissions. After this initial spike, concentrations diluted from increased mixing height and temperature, resulting in particulate ammonium nitrate evaporation. The mixing ratio of NOx is influential in SOA formation from VOCs.120 Overnight accumulation of OOA and NOx in the low boundary layer could decrease SOA production if SOA precursors are consumed significantly faster than Ox precursors.114
Organic aerosol source factor temporal trends
The AMS-PMF factors, gas-phase species, aerosols, and tracer ions time series (Fig. 5) have a distinct daily pattern (Fig. 6). The average contribution to OA from these factors is: 14.7% HOA, 15.2% BBOA, 11.2% TBOA, 8.7% sLOA, 20.6% OOA1, 12.3% OOA2, and 17.4% OOA3. The average mass fraction of the PMF OA components (Fig. 6c) is over half (50.3%) total OOA. More than a quarter of OA (26.4%) was combined biomass and trash burning OA.
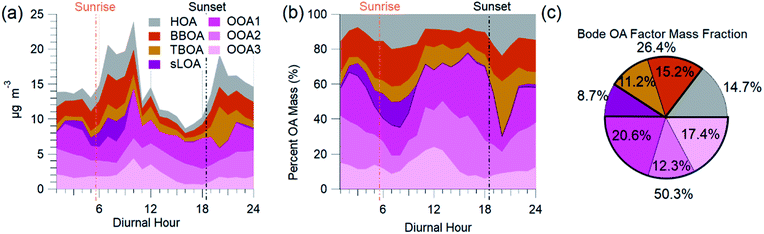 |
| Fig. 6 AMS-PMF OA source factor average diurnal mass concentration (a), the diurnal mass fraction of OA (b), and the average mass fraction (c) are from NAMaSTE1 in Kathmandu, Nepal, April 2015. | |
HOA exhibited a morning and evening increase in concentration and mass fraction that correspond to daily rush hours. BBOA had a build-up in concentrations from evening until late morning when the basin's ventilation from increased windspeed reduced the overall concentration. The concentration of TBOA was high throughout daytime hours, with a significant spike around 20:00 NST.
sLOA was three times higher in the early morning compared to typical daytime concentrations. During the afternoon, the concentration of sLOA was negligible, whereas early morning pre-sunrise sLOA could make up nearly 20% of the total OA mass. This sLOA increase corresponds with overnight stagnation and confinement to the surface due to the shallow boundary layer height. The monitoring site for NAMaSTE1 was downwind of several clusters of brick kilns in Bhaktapur during overnight periods. The sLOA factor is likely the impact of coal burning in brick kilns, the fraction of which enhanced significantly overnight. This factor decreased at sunrise from the increased elevation of the mixing height. The increased ventilation in the afternoon minimized the concentration. OOA peaked after sunrise. The sum of OOA factors accounts for ∼70% of OA mass from 10:00 to 18:00 NST. Photochemical activity is highest during this daylight period, driving increased SOA production. OOA3 mass fraction increased by over 100% after sunrise as sLOA diminishes and accounts for a significant afternoon OOA mass fraction increase. OOA1 increases in mass fraction as the air volume becomes more photochemically aged. OOA1 increased in concentration at 11:00 NST and had a minimum after sunset. OOA2 had less daily variation than the other OOA factors. The morning increase in OOA1 and OOA3 corresponds to the planetary boundary layer's extension and dissolution.6 This atmospheric change may introduce aged aerosol from the residual layer and free troposphere to the surface level.
Co-located instrument apportionment intercomparison
A comparison of the OC source apportionment derived from AMS based PMF results (AMS-PMF) and organic molecular marker-based chemical mass balance (OMM-CMB) for NAMaSTE1 shows temporal agreement and very similar overall source apportionment of OC. In contrast to AMS-PMF, OMM-CMB source apportionment requires an a priori understanding of molecular tracers to identify sources (e.g., ref. 35 and 121). These tracers are identified before analysis and define the resulting sources.
OC for PM1 (OC1) is calculated for AMS-PMF factors and converted using organic aerosol to organic carbon (OA
:
OC) ratios.122 We calculate OA
:
OC for each OA factor (Table SI4†) from the linear relationship between OA
:
OC and O
:
C derived from PMF.16 The values OA
:
OC ratios used agree with previously measured values (Table SI4†).33,123
OA calculations from OMM-CMB PM2.5 (OC2.5) filter measurements in Islam et al.35 used an OA
:
OC factor of 1.7. This value was obtained from AMS measurements and converted from OC2.5 to OA. Fig. 7 shows the OC time-series for these two methods, AMS-PMF and OMM-CMB, on the filter samples' time scale and the average mass fraction for each method. Filter-based OC2.5 concentrations averaged 17.6 ± 9.6 μg m−3, or 26% of the total PM2.5 mass.35 OC2.5 directly measured by filters exhibited 1.05 times greater mass than calculated OC1 from HR-AMS results. There is agreement for total OC between the two techniques (R2 = 0.67) (Fig. SI6†). This mass discrepancy is insignificant.
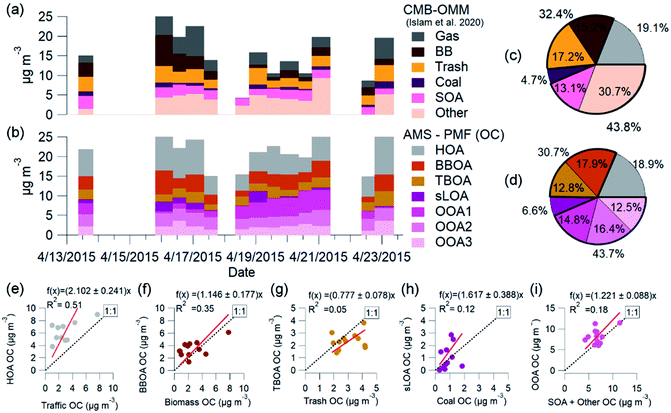 |
| Fig. 7 AMS-PMF and OMM-CMB factors (Islam et al., 2020) OC averages from overlapping sample periods are from NAMaSTE1, Kathmandu Nepal, April 2015. OMM-CMB OC (a) and AMS-PMF OC average factors (b) shown are on the CMB time scale. Scatter plots of OMM-CMB (c) and AMS-PMF OC (d) average mass fractions compare well PMF and CMB factors HOA and traffic (e), BBOA and biomass (f), TBOA and trash (g), sLOA and coal (h), OOA and SOA with other (i) are shown as AMS-PMF OA results converted to OC. | |
Molecular markers from the filter OC identify and apportion OC2.5 to five major primary sources through OMM-CMB in the Kathmandu Valley, including biomass burning, garbage burning, coal combustion, engines, vegetative detritus, and secondary aerosols.35
The OMM-CMB factors somewhat differ from AMS-PMF factors, we combine similar sources to bulk factors to compare the two different methods' results (Fig. 8). The relative source contributions to OC found by each method are very similar. Vehicular traffic OMM-CMB OC was comparable to OC from the AMS-PMF HOA factor. Similarly, the OC from AMS-PMF sLOA was like the coal combustion OC derived from OMM-CMB. However, the secondary sources for the two methods differ, but the sum of all these components agree well. OC for the three AMS-PMF OOA factors sums to a total OOA-related OC compared to OMM-CMB SOA plus the ‘other’ factor. The CMB model is presumed to identify and represent all primary sources.124 All PM2.5 unaccounted for by this model are summed to the ‘other’ OMM-CMB category to compare with total OOA OC. OMM-CMB ‘other’ is assumed to be primarily secondary OC. Islam et al.35 describe this ‘other’ source as from emissions which did not have measured marker species, such as volatile industrial emissions, dust, secondary formation and cooking. None of the possible PMF solutions separated a distinct cooking factor, implying that it is a negligible influence in ambient conditions. As dust is primarily inorganic, uncharacterized VOCs oxidation products from industrial emissions and other secondary organic aerosol species are the main contribution to this source.35
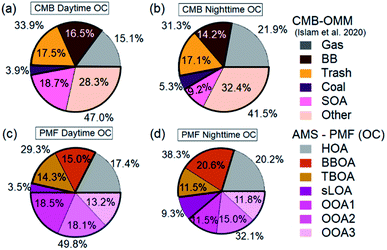 |
| Fig. 8 Mass fractions of OMM-CMB factors and AMS-PMF OC are averaged to filter sample periods for NAMaSTE1, April 2015, Kathmandu, Nepal. Displayed average fractions are AMS-PMF OC daytime (a), AMS-PMF OC night-time (b), OMM-CMB daytime (c), and OMM-CMB night-time (d). | |
AMS-PMF non-traffic combustion factors TBOA and BBOA combine to a non-traffic open combustion factor. This open combustion OC and the sum of OMM-CMB garbage and biomass factors are similarly combined and compared.
Independent of measurement and analysis methodology, vehicular traffic contributed about 19%, open combustion 30%, and SOA 44% of total OC. The combined overall source components from each method, shown in Fig. 7e–i, compare very well in aggregate across the measurement period. The two methods, AMS-PMF and OMM-CMB, show a similar change in mass fraction for daytime and overnight periods, shown in Fig. 8. The total OOA and implied CMB SOA factors are near 50% in the daytime and 40% at night. HOA or gas makes up around 16% in the daytime and 20% at night. The open combustion factor is consistently around 31%. The complementary nature of the methods is shown by the discrepancy between sLOA and Coal.
sLOA/coal shows the highest slope and lowest correlation, likely due to the relatively large dynamic range and fuel inconsistency. sLOA from AMS-PMF has a nearly three-fold increase, 3.5% to 9.3%, overnight, whereas coal from OMM-CMB only increases from 4% to 5%. The AMS-PMF sLOA component has additional mass as compared to the OMM-CMB coal. This discrepancy is likely because brick kilns, a source of sLOA, co-fire coal, and biomass for fuel. OMM-CMB would split kiln emissions across the biomass and coal factors. The temporally distinct nighttime increase of sLOA makes kilns emissions straightforward for PMF to identify.
The average results show agreement between the two independent source apportionment methodologies for organic aerosol. These results, therefore, provide important source information for understanding the air quality impacts of various activities within the Kathmandu Valley despite the uncertainty in this region and the local sources' complexity.
Black carbon source estimation
BC comes from combustion sources, and each source contributes a fraction to the total BC mass. We utilize source profile information to estimate the contribution of each combustion-related PMF factor to the total BC. The estimated BC calculation utilizes organic aerosol PMF factor concentrations converted to organic carbon (OC) and OC to BC ratio (OC
:
BC) from the literature and source measurements. Explicitly the calculation of estimated BC for combustion-related factors is the following: where BCest is the estimated BC determined from the sum of combustion-related PMF factors (HOA, sLOA, BBOA, TBOA) times the reciprocal of the OM
:
OC for each factor to convert to OC, and times the reciprocal of the OC
:
BC ratio for each factor to yield the estimated contribution of BC from each source (Table SI6†). We identified, when possible, the range of measured values for OC
:
BC (this BC reconstruction, uses OC
:
BC and OC
:
EC values interchangeably) for each of these sources and we chose a representative value. Brick kilns emissions show a range of OC
:
BC in literature from 0.1 (ref. 125) to over 50,33 dependent on kiln type and the fuel used. For this calculation, we use an OC
:
BC for sLOA of 0.5, corresponding to the emissions of zig-zag brick kilns.33 Biomass burning OC
:
BC ranges from 1.8 to 6 and greater.34,126 Values for OC
:
BC from trash burning ranged from 1 to 5.5 (ref. 33). The OC
:
BC ratios from NAMaSTE1 emissions used are for mixed trash (1.0) and mixed agricultural residue burning (3.7).33 NAMaSTE1 does not adequately characterize the OC
:
BC for HOA. Vehicular traffic emissions exhibit a range of OC
:
BC from 0.25 to 6.127 The measured HOA
:
BC ratio of 0.25 was lower than the 0.4 HOA
:
EC measured in European studies.128 The HOA
:
BC ratio is near the low end of 0.25 reported for Europe during enhanced photochemistry periods.129 There is significant uncertainty in the OC to BC ratio from vehicles in South Asia. A sensitivity analysis of BCest with respect to HOA resulted in an HOA OC
:
BC of 0.4 (Fig. 9c).
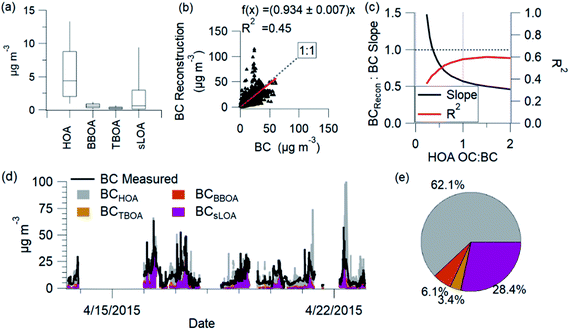 |
| Fig. 9 BC reconstruction variance for individual factors (a), regression of BC reconstructed and measured (b), sensitivity analysis of HOA OC : BC (c), time series of BC measured, and each reconstructed factor (d), and the average mass fraction of BC (e) shown are from AMS-PMF factors, for NAMaSTE1, in April 2015, Kathmandu, Nepal. | |
The reconstructed BC and measured BC correlate with an R2 of 0.45. HOA is the largest source of BC (62%). sLOA is the second largest contributor to BC (28%), followed by TBOA (3%) and BBOA (6%). The sLOA and HOA factors emit more BC than OC and significantly contribute to elevated C-PM1 concentrations.
Individual spikes of the BC reconstruction reach concentrations well above the measured levels. These peaks, driven by BC from HOA, show the uncertainty of this estimation. The OC
:
BC ratio for sources likely changes, and this reconstruction does not account for all possible sources. The vehicle fleet's diversity and differences in OC
:
BC emission ratios create uncertainty in these estimations.
Conclusions
The ambient measurement component of NAMaSTE1 characterized the chemical composition of particulate matter and, identified significant OA source factor mass spectra and gas-phase species for April 2015 in the Kathmandu Valley, Nepal. We identified the constituent parts of PM1 from in situ AMS measurements which compared well with OMM-CMB based source apportionment, gas-phase measurements, and emission spectra. The average results show agreement between the two independent source apportionment methodologies for organic aerosol. These results, therefore, provide important source information for understanding the air quality impacts of various activities and sources within the Kathmandu Valley despite the uncertainty in this region and the local sources' complexity. The general agreement in the inter-comparison between complementary techniques gives additional confidence in the source attribution results; this provides a stronger basis for discussions on policies to reduce air pollution impacts in the Kathmandu Valley.
The C-PM1 averaged a mean of 41.03 ± 20.48 μg m−3, which was comprised of 15.79 μg m−3 (39.7%) OA, 10.5 μg m−3 (25.0%) BC, 8.4 μg m−3 (21.1%) SO42−, 2.96 μg m−3 (7.4%) NH4+, 1.22 μg m−3 (3.1%) NO3−, 0.92 μg m−3 (2.3%) Cl−. BrC comprised a lower limit 1.87 μg m−3 portion of the OA, 4.7% of total mass.
Emissions, boundary layer dynamics, wind patterns, wet deposition, and photochemistry are the prominent factors that impact PM1 concentrations. Diurnal wind speed acted as the primary means of transport to remove and dilute pollutants from the Kathmandu Valley during mid-afternoon when wind speeds were highest.
AMS-PMF analysis of high-resolution organic aerosol mass spectra resulted in the identification of seven distinct source factors. These factors are consistent with previous studies in the Kathmandu Valley. Each OA factor mass spectra correlates to a known source, tracer correlation, and diurnal trends. The OC from PM1 PMF factors correlates well to OC factors from OCC-CMB from PM2.5 (ref. 35) for this location and period.
The dominant primary factors contributing to organic PM1 are associated with traffic (HOA), biomass burning (BBOA), trash burning (TBOA), and local sulfate-containing OA (sLOA) sources. HOA had the most significant impact, accounting for 19% of OA and 62% of BC. The concentration of HOA increased significantly during rush hour periods. These vehicle emissions are a well-known but under-sampled source, especially given the region's vehicle fleet diversity. Limited maintenance and fuel quality lead to increased uncertainty in understanding regional traffic emissions.
Biomass burning is responsible for about 18% of OA and 6% of BC. Trash burning provides 13% of OA and 3% of BC, a significant fraction of total aerosol. Trash burning OA was strongly associated with BrC. sLOA contains an organic sulfur species (C3HSO+), a tracer likely from coal cofired in brick kilns.91 Overall, sLOA contains 7% of total OA mass and 28% of all BC.
Household combustion of biomass and trash can be mitigated through increased access to efficient fuels and improving waste management practices. Biomass emissions have both primary and secondary impacts in the region, so control could significantly reduce aerosols. Adopting a more modern vehicle standard would control traffic emissions, leading to a significant reduction of PM. A reduction in vehicle emissions would also result in a reduction in SOA by limiting NOx emissions. Reducing the density of brick kilns in the Kathmandu Valley would decrease OA emissions and, in parallel, decrease BC emissions. Controlling these primary particulate sources has the additional benefit of reducing VOCs from combustion, SO2 and aerosol sulfate from brick kiln sulfur, and NOx from vehicles, further reducing sources for secondary aerosol production.
Funding
This work was funded in part by the National Science Foundation grants entitled: “Ambient and Source Characterization of Aerosol Size and Composition in Nepal and Bhutan using High-Resolution Aerosol Mass Spectrometry” via award number AGS1461458 to Drexel University, and “Collaborative Research: Measurements of Selected Combustion Emissions in Nepal and Bhutan Integrated with Source Apportionment and Chemical Transport Modelling for South Asia,” award number AGS1351616 to the University of Iowa, AGS-0003865 to the University of Virginia (UVA), AGS-1349976 to the University of Montana, and AGS1350021 to Emory University. The Institute for Advanced Sustainability Studies (IASS) is funded by the German Ministry of Education and Research (BMBF) and the Brandenburg State Ministry of Science, Research and Culture (MWFK). Additional support for NAMaSTE was provided by ICIMOD, which is contributed to by the governments of Afghanistan, Australia, Austria, Bangladesh, Bhutan, China, India, Myanmar, Nepal, Norway, Pakistan, Switzerland, and the United Kingdom. Further funds come from the Government of Sweden for ICIMOD's Atmosphere Initiative.
Author contributions
E. A. S., R. J. Y., A. K. P., E. S., and P. F. D. are the principal investigators of NAMaSTE project. Field measurements were conducted by B. S. W., J. D. G., M. R. G., M. R., and P. F. D. In-country support and logistics were contributed by A. K. P., P. V. B. and P. S. P. Processing, analysis, and interpretation were completed by B. S. W. and P. F. D. All authors contributed to the writing of this paper.
Conflicts of interest
The authors declare that they have no conflict of interest.
Acknowledgements
Special thanks to Sagar Adhikari, Pratik Man Singdan, Bhogendra Kathayat, and Shyam Kumar Newar for their work in the field.
References
- R. K. Aryal, B.-K. Lee, R. Karki, A. Gurung, J. Kandasamy, B. K. Pathak, S. Sharma and N. Giri, Seasonal PM10 dynamics in Kathmandu Valley, Atmos. Environ., 2008, 42, 8623–8633 CrossRef CAS.
- R. K. Aryal, B.-K. Lee, R. Karki, A. Gurung, B. Baral and S.-H. Byeon, Dynamics of PM2.5 concentrations in Kathmandu Valley, Nepal, J. Hazard. Mater., 2009, 168, 732–738 CrossRef CAS PubMed.
- E. A. Stone, T. T. Nguyen, B. B. Pradhan and P. M. Dangol, Assessment of biogenic secondary organic aerosol in the Himalayas, Environ. Chem., 2012, 9, 263–272 CrossRef CAS.
- E. A. Stone, J. J. Schauer, B. B. Pradhan, P. M. Dangol, G. Habib and C. Venkataraman, Characterization of emissions from South Asian biofuels and application to source apportionment of carbonaceous aerosol in the Himalayas, J. Geophys. Res.: Atmos., 2010, 115, D06301, DOI:10.1029/2009JD011881.
- D. Putero, P. Cristofanelli, A. Marinoni, B. Adhikary, R. Duchi, S. D. Shrestha, G. P. Verza, T. C. Landi, F. Calzolari, M. Busetto, G. Agrillo, F. Biancofiore, P. Di Carlo, A. K. Panday, M. Rupakheti and P. Bonasoni, Seasonal variation of ozone and black carbon observed at Paknajol, an urban site in the Kathmandu Valley, Nepal, Atmos. Chem. Phys., 2015, 15, 13957–13971 CrossRef CAS.
- A. Mues, M. Rupakheti, C. Münkel, A. Lauer, H. Bozem, P. Hoor, T. Butler and M. G. Lawrence, Investigation of the mixing layer height derived from ceilometer measurements in the Kathmandu Valley and implications for local air quality, Atmos. Chem. Phys., 2017, 17, 8157–8176 CrossRef CAS.
- X. Wan, S. Kang, M. Rupakheti, Q. Zhang, L. Tripathee, J. Guo, P. Chen, D. Rupakheti, A. K. Panday, M. G. Lawrence, K. Kawamura and Z. Cong, Molecular characterization of organic aerosols in the Kathmandu Valley, Nepal: insights into primary and secondary sources, Atmos. Chem. Phys., 2019, 19, 2725–2747 CrossRef CAS.
- A. K. Panday and R. G. Prinn, Diurnal cycle of air pollution in the Kathmandu Valley, Nepal: Observations, J. Geophys. Res.: Atmos., 2009, 114, D09305, DOI:10.1029/2008JD009777.
- F. Kiros, K. M. Shakya, M. Rupakheti, R. P. Regmi, R. Maharjan, R. M. Byanju, M. Naja, K. Mahata, B. Kathayat and R. E. Peltier, Variability of Anthropogenic Gases: Nitrogen Oxides, Sulfur Dioxide, Ozone and Ammonia in Kathmandu Valley, Nepal, Aerosol Air Qual. Res., 2017, 16, 3088–3101 CrossRef.
- C. Sarkar, V. Sinha, B. Sinha, A. K. Panday, M. Rupakheti and M. G. Lawrence, Source apportionment of NMVOCs in the Kathmandu Valley during the SusKat-ABC international field campaign using positive matrix factorization, Atmos. Chem. Phys., 2017, 17, 8129–8156 CrossRef CAS.
- K. S. Mahata, M. Rupakheti, A. K. Panday, P. Bhardwaj, M. Naja, A. Singh, A. Mues, P. Cristofanelli, D. Pudasainee, P. Bonasoni and M. G. Lawrence, Observation and analysis of spatiotemporal characteristics of surface ozone and carbon monoxide at multiple sites in the Kathmandu Valley, Nepal, Atmos. Chem. Phys., 2018, 18, 14113–14132 CrossRef CAS.
- P. Bhardwaj, M. Naja, M. Rupakheti, A. Lupascu, A. Mues, A. K. Panday, R. Kumar, K. S. Mahata, S. Lal, H. C. Chandola and M. G. Lawrence, Variations in surface ozone and carbon monoxide in the Kathmandu Valley and surrounding broader regions during SusKat-ABC field campaign: role of local and regional sources, Atmos. Chem. Phys., 2018, 18, 11949–11971 CrossRef CAS.
- P. S. Mahapatra, S. P. Puppala, B. Adhikary, K. L. Shrestha, D. P. Dawadi, S. P. Paudel and A. K. Panday, Air quality trends of the Kathmandu Valley: A satellite, observation and modeling perspective, Atmos. Environ., 2019, 201, 334–347 CrossRef CAS.
-
M. Dhimal, L. Bhattarai and C. Bhusal, Situation Analysis of Environmental Health in Nepal 2009, 2009 Search PubMed.
- A. Gurung and M. L. Bell, The state of scientific evidence on air pollution and human health in Nepal, Environ. Res., 2013, 124, 54–64 CrossRef CAS PubMed.
- P. Sadavarte, M. Rupakheti, P. Bhave, K. Shakya and M. Lawrence, Nepal emission inventory – Part I: Technologies and combustion sources (NEEMI-Tech) for 2001–2016, Atmos. Chem. Phys., 2019, 19, 12953–12973 CrossRef CAS.
- D. G. Streets, K. F. Yarber, J.-H. Woo and G. R. Carmichael, Biomass burning in Asia: Annual and seasonal estimates and atmospheric emissions, Global Biogeochem. Cycles, 2003, 17(4), 10-1–10-20 CrossRef.
-
WHO: World Health Organization, Fuel for Life: Household Energy and Health, retrieved from: https://www.who.int/indoorair/publications/fuelforlife/en/(last access: September 2017), 2006 Search PubMed.
- P. Pandey, A. Sadavarte, B. Rao and C. Venkataraman, Trends in multi-pollutant emissions from a technology-linked inventory for India: II. Residential, agricultural and informal industry sectors, Atmos. Environ., 2014, 99, 341–352 CrossRef.
- E. Winijkul and T. C. Bond, Emissions from residential combustion considering end-uses and spatial constraints: Part II, emission reduction scenarios, Atmos. Environ., 2016, 124, 1–11 CrossRef CAS.
- D. G. Fullerton, N. Bruce and S. B. Gordon, Indoor air pollution from biomass fuel smoke is a major health concern in the developing world, Trans. R. Soc. Trop. Med. Hyg., 2008, 102, 843–851 CrossRef PubMed.
- S. S. Lim, T. Vos, A. D. Flaxman, G. Danaei, K. Shibuya, H. Adair-Rohani, M. A. AlMazroa, M. Amann, H. R. Anderson, K. G. Andrews, M. Aryee, C. Atkinson, L. J. Bacchus, A. N. Bahalim, K. Balakrishnan, J. Balmes, S. Barker-Collo, A. Baxter, M. L. Bell, J. D. Blore, F. Blyth, C. Bonner, G. Borges, R. Bourne, M. Boussinesq, M. Brauer, P. Brooks, N. G. Bruce, B. Brunekreef, C. Bryan-Hancock, C. Bucello, R. Buchbinder, F. Bull, R. T. Burnett, T. E. Byers, B. Calabria, J. Carapetis, E. Carnahan, Z. Chafe, F. Charlson, H. Chen, J. S. Chen, A. T.-A. Cheng, J. C. Child, A. Cohen, K. E. Colson, B. C. Cowie, S. Darby, S. Darling, A. Davis, L. Degenhardt, F. Dentener, D. C. D. Jarlais, K. Devries, M. Dherani, E. L. Ding, E. R. Dorsey, T. Driscoll, K. Edmond, S. E. Ali, R. E. Engell, P. J. Erwin, S. Fahimi, G. Falder, F. Farzadfar, A. Ferrari, M. M. Finucane, S. Flaxman, F. G. R. Fowkes, G. Freedman, M. K. Freeman, E. Gakidou, S. Ghosh, E. Giovannucci, G. Gmel, K. Graham, R. Grainger, B. Grant, D. Gunnell, H. R. Gutierrez, W. Hall, H. W. Hoek, A. Hogan, H. D. Hosgood, D. Hoy, H. Hu, B. J. Hubbell, S. J. Hutchings, S. E. Ibeanusi, G. L. Jacklyn, R. Jasrasaria, J. B. Jonas, H. Kan, J. A. Kanis, N. Kassebaum, N. Kawakami, Y.-H. Khang, S. Khatibzadeh, J.-P. Khoo, C. Kok, F. Laden, R. Lalloo, Q. Lan, T. Lathlean, J. L. Leasher, J. Leigh, Y. Li, J. K. Lin, S. E. Lipshultz, S. London, R. Lozano, Y. Lu, J. Mak, R. Malekzadeh, L. Mallinger, W. Marcenes, L. March, R. Marks, R. Martin, P. McGale, J. McGrath, S. Mehta, Z. A. Memish, G. A. Mensah, T. R. Merriman, R. Micha, C. Michaud, V. Mishra, K. M. Hanafiah, A. A. Mokdad, L. Morawska, D. Mozaffarian, T. Murphy, M. Naghavi, B. Neal, P. K. Nelson, J. M. Nolla, R. Norman, C. Olives, S. B. Omer, J. Orchard, R. Osborne, B. Ostro, A. Page, K. D. Pandey, C. D. Parry, E. Passmore, J. Patra, N. Pearce, P. M. Pelizzari, M. Petzold, M. R. Phillips, D. Pope, C. A. Pope, J. Powles, M. Rao, H. Razavi, E. A. Rehfuess, J. T. Rehm, B. Ritz, F. P. Rivara, T. Roberts, C. Robinson, J. A. Rodriguez-Portales, I. Romieu, R. Room, L. C. Rosenfeld, A. Roy, L. Rushton, J. A. Salomon, U. Sampson, L. Sanchez-Riera, E. Sanman, A. Sapkota, S. Seedat, P. Shi, K. Shield, R. Shivakoti, G. M. Singh, D. A. Sleet, E. Smith, K. R. Smith, N. J. Stapelberg, K. Steenland, H. Stöckl, L. J. Stovner, K. Straif, L. Straney, G. D. Thurston, J. H. Tran, R. V. Dingenen, A. van Donkelaar, J. L. Veerman, L. Vijayakumar, R. Weintraub, M. M. Weissman, R. A. White, H. Whiteford, S. T. Wiersma, J. D. Wilkinson, H. C. Williams, W. Williams, N. Wilson, A. D. Woolf, P. Yip, J. M. Zielinski, A. D. Lopez, C. J. Murray and M. Ezzati, A comparative risk assessment of burden of disease and injury attributable to 67 risk factors and risk factor clusters in 21 regions, 1990–2010: a systematic analysis for the Global Burden of Disease Study 2010, Lancet, 2012, 380, 2224–2260 CrossRef.
- Z. A. Chafe, M. Brauer, Z. Klimont, R. V. Dingenen, S. Mehta, S. Rao, K. Riahi, F. Dentener and K. R. Smith, Household Cooking with Solid Fuels Contributes to Ambient PM2.5 Air Pollution and the Burden of Disease, Environ. Health Perspect., 2014, 122(12), 1315–1320 CrossRef PubMed.
- S. Agrawal and S. Yamamoto, Effect of Indoor air pollution from biomass and solid fuel combustion on symptoms of preeclampsia/eclampsia in Indian women, Indoor Air, 2015, 25, 341–352 CrossRef CAS PubMed.
- B. H. Chen, C. J. Hong, M. R. Pandey and K. R. Smith, Indoor air pollution in developing countries, Pollution de l’ air à l’ intérieur des habitations
dans les pays en développement : résumé, World Health Stat. Quart., 1990, 43(3), 127–138 CAS.
- V. Ramanathan, C. Chung, D. Kim, T. Bettge, L. Buja, J. T. Kiehl, W. M. Washington, Q. Fu, D. R. Sikka and M. Wild, Atmospheric brown clouds: Impacts on South Asian climate and hydrological cycle, Proc. Natl. Acad. Sci. U. S. A., 2005, 102, 5326–5333 CrossRef CAS PubMed.
- C. Venkataraman, Residential Biofuels in South Asia: Carbonaceous Aerosol Emissions and Climate Impacts, Science, 2005, 307, 1454–1456 CrossRef CAS PubMed.
- M. G. Lawrence and J. Lelieveld, Atmospheric pollutant outflow from southern Asia: a review, Atmos. Chem. Phys., 2010, 10, 11017–11096 CrossRef CAS.
- M. S. Reddy and C. Venkataraman, Inventory of aerosol and sulphur dioxide emissions from India: I—Fossil fuel combustion, Atmos. Environ., 2002, 36, 677–697 CrossRef CAS.
- P. F. DeCarlo, J. R. Kimmel, A. Trimborn, M. J. Northway, J. T. Jayne, A. C. Aiken, M. Gonin, K. Fuhrer, T. Horvath, K. S. Docherty, D. R. Worsnop and J. L. Jimenez, Field-Deployable, High-Resolution, Time-of-Flight Aerosol Mass Spectrometer, Anal. Chem., 2006, 78, 8281–8289 CrossRef CAS PubMed.
- M. R. Canagaratna, J. T. Jayne, J. L. Jimenez, J. D. Allan, M. R. Alfarra, Q. Zhang, T. B. Onasch, F. Drewnick, H. Coe, A. Middlebrook, A. Delia, L. R. Williams, A. M. Trimborn, M. J. Northway, P. F. DeCarlo, C. E. Kolb, P. Davidovits and D. R. Worsnop, Chemical and microphysical characterization of ambient aerosols with the aerodyne aerosol mass spectrometer, Mass Spectrom. Rev., 2007, 26, 185–222 CrossRef CAS PubMed.
- C. E. Stockwell, T. J. Christian, J. D. Goetz, T. Jayarathne, P. V. Bhave, P. S. Praveen, S. Adhikari, R. Maharjan, P. F. DeCarlo, E. A. Stone, E. Saikawa, D. R. Blake, I. J. Simpson, R. J. Yokelson and A. K. Panday, Nepal Ambient Monitoring and Source Testing Experiment (NAMaSTE): emissions of trace gases and light-absorbing carbon from wood and dung cooking fires, garbage and crop residue burning, brick kilns, and other sources, Atmos. Chem. Phys., 2016, 16, 11043–11081 CrossRef CAS.
- J. D. Goetz, M. R. Giordano, C. E. Stockwell, T. J. Christian, R. Maharjan, S. Adhikari, P. V. Bhave, P. S. Praveen, A. K. Panday, T. Jayarathne, E. A. Stone, R. J. Yokelson and P. F. DeCarlo, Speciated online PM1 from South Asian combustion sources – Part 1: Fuel-based emission factors and size distributions, Atmos. Chem. Phys., 2018, 18, 14653–14679 CrossRef CAS.
- T. Jayarathne, C. E. Stockwell, P. V. Bhave, P. S. Praveen, C. M. Rathnayake, Md. R. Islam, A. K. Panday, S. Adhikari, R. Maharjan, J. D. Goetz, P. F. DeCarlo, E. Saikawa, R. J. Yokelson and E. A. Stone, Nepal Ambient Monitoring and Source Testing Experiment (NAMaSTE): emissions of particulate matter from wood- and dung-fueled cooking fires, garbage and crop residue burning, brick kilns, and other sources, Atmos. Chem. Phys., 2018, 18, 2259–2286 CrossRef CAS.
- Md. R. Islam, T. Jayarathne, I. J. Simpson, B. Werden, J. Maben, A. Gilbert, P. S. Praveen, S. Adhikari, A. K. Panday, M. Rupakheti, D. R. Blake, R. J. Yokelson, P. F. DeCarlo, W. C. Keene and E. A. Stone, Ambient air quality in the Kathmandu Valley, Nepal, during the pre-monsoon: concentrations and sources of particulate matter and trace gases, Atmos. Chem. Phys., 2020, 20, 2927–2951 CrossRef CAS.
- C. Cho, S.-W. Kim, M. Rupakheti, J.-S. Park, A. Panday, S.-C. Yoon, J.-H. Kim, H. Kim, H. Jeon, M. Sung, B. M. Kim, S. K. Hong, R. J. Park, D. Rupakheti, K. S. Mahata, P. S. Praveen, M. G. Lawrence and B. Holben, Wintertime aerosol optical and radiative properties in the Kathmandu Valley during the SusKat-ABC field campaign, Atmos. Chem. Phys., 2017, 17, 12617–12632 CrossRef CAS.
- R. P. Regmi, T. Kitada and G. Kurata, Numerical Simulation of Late Wintertime Local Flows in Kathmandu Valley, Nepal: Implication for Air Pollution Transport, J. Appl. Meteorol. Climatol., 2003, 42, 389–403 CrossRef.
- A. Mues, A. Lauer, A. Lupascu, M. Rupakheti, F. Kuik and M. G. Lawrence, WRF and WRF-Chem v3.5.1 simulations of meteorology and black carbon concentrations in the Kathmandu Valley, Geosci. Model Dev., 2018, 11, 2067–2091 CrossRef CAS.
- R. P. Regmi, T. Kitada, S. Maharjan, S. Shrestha, S. Shrestha and G. Regmi, Wintertime Boundary Layer Evolution and Air Pollution Potential Over the Kathmandu Valley, Nepal, J. Geophys. Res.: Atmos., 2019, 124, 4299–4325 CrossRef CAS.
- C. Sarkar, V. Sinha, V. Kumar, M. Rupakheti, A. Panday, K. S. Mahata, D. Rupakheti, B. Kathayat and M. G. Lawrence, Overview of VOC emissions and chemistry from PTR-TOF-MS measurements during the SusKat-ABC campaign: high acetaldehyde, isoprene and isocyanic acid in wintertime air of the Kathmandu Valley, Atmos. Chem. Phys., 2016, 16, 3979–4003 CrossRef CAS.
- K. M. Shakya, L. D. Ziemba and R. J. Griffin, Characteristics and Sources of Carbonaceous, Ionic, and Isotopic Species of Wintertime Atmospheric Aerosols in Kathmandu Valley, Nepal, Aerosol Air Qual. Res., 2010, 10, 219–230 CrossRef CAS.
- Md. R. Islam, T. Li, K. Mahata, N. Khanal, B. Werden, M. R. Giordano, P. S. Praveen, N. B. Dhital, A. Gurung, A. K. Panday, I. B. Joshi, S. P. Poudel, Y. Wang, E. Saikawa, R. J. Yokelson, P. F. DeCarlo and E. A. Stone, Wintertime Air Quality in Lumbini, Nepal: Sources of Fine Particle Organic Carbon, ACS Earth Space Chem., 2021, 5(2), 226–238, DOI:10.1021/acsearthspacechem.0c00269.
-
B. Werden, M. R. Giordano, M. R. Islam, K. Mahata, P. S. Puppala, A. K. Panday, R. J. Yokelson, E. A. Stone, and P. F. DeCarlo, Wintertime Aerosol Composition, Source Contribution, and Spatial Variation across the Kathmandu Valley, Nepal, 2022.
- C. Mohr, P. F. DeCarlo, M. F. Heringa, R. Chirico, R. Richter, M. Crippa, X. Querol, U. Baltensperger and A. S. H. Prévôt, Spatial Variation of Aerosol Chemical Composition and Organic Components Identified by Positive Matrix Factorization in the Barcelona Region, Environ. Sci. Technol., 2015, 49, 10421–10430 CrossRef CAS PubMed.
- J. A. Huffman, P. J. Ziemann, J. T. Jayne, D. R. Worsnop and J. L. Jimenez, Development and Characterization of a Fast-Stepping/Scanning Thermodenuder for Chemically-Resolved Aerosol Volatility Measurements, Aerosol Sci. Technol., 2008, 42, 395–407 CrossRef CAS.
- B. M. Matthew, A. M. Middlebrook and T. B. Onasch, Collection Efficiencies in an Aerodyne Aerosol Mass Spectrometer as a Function of Particle Phase for Laboratory Generated Aerosols, Aerosol Sci. Technol., 2008, 42, 884–898 CrossRef CAS.
- F. Drewnick, S. S. Hings, P. DeCarlo, J. T. Jayne, M. Gonin, K. Fuhrer, S. Weimer, J. L. Jimenez, K. L. Demerjian, S. Borrmann and D. R. Worsnop, A New Time-of-Flight Aerosol Mass Spectrometer (TOF-AMS)—Instrument Description and First Field Deployment, Aerosol Sci. Technol., 2005, 39, 637–658 CrossRef CAS.
- N. Takegawa, Y. Miyazaki, Y. Kondo, Y. Komazaki, T. Miyakawa, J. L. Jimenez, J. T. Jayne, D. R. Worsnop, J. D. Allan and R. J. Weber, Characterization of an Aerodyne Aerosol Mass Spectrometer (AMS): Intercomparison with Other Aerosol Instruments, Aerosol Sci. Technol., 2005, 39, 760–770 CrossRef CAS.
- Q. Zhang, D. R. Worsnop, M. R. Canagaratna and J. L. Jimenez, Hydrocarbon-like and oxygenated organic aerosols in Pittsburgh: insights into sources and processes of organic aerosols, Atmos. Chem. Phys., 2005, 5, 3289–3311 CrossRef CAS.
- D. Salcedo, T. B. Onasch, K. Dzepina, M. R. Canagaratna, Q. Zhang, J. A. Huffman, P. F. DeCarlo, J. T. Jayne, P. Mortimer, D. R. Worsnop, C. E. Kolb, K. S. Johnson, B. Zuberi, L. C. Marr, R. Volkamer, L. T. Molina, M. J. Molina, B. Cardenas, R. M. Bernabé, C. Márquez, J. S. Gaffney, N. A. Marley, A. Laskin, V. Shutthanandan, Y. Xie, W. Brune, R. Lesher, T. Shirley and J. L. Jimenez, Characterization of ambient aerosols in Mexico City during the MCMA-2003 campaign with Aerosol Mass Spectrometry: results from the CENICA Supersite, Atmos. Chem. Phys., 2006, 6, 925–946 CrossRef CAS.
- M. Middlebrook, R. Bahreini, J. L. Jimenez and M. R. Canagaratna, Evaluation of Composition-Dependent Collection Efficiencies for the Aerodyne Aerosol Mass Spectrometer using Field Data, Aerosol Sci. Technol., 2012, 46, 258–271 CrossRef.
- R. Bahreini, E. J. Dunlea, B. M. Matthew, C. Simons, K. S. Docherty, P. F. DeCarlo, J. L. Jimenez, C. A. Brock and A. M. Middlebrook, Design and Operation of a Pressure-Controlled Inlet for Airborne Sampling with an Aerodynamic Aerosol Lens, Aerosol Sci. Technol., 2008, 42, 465–471 CrossRef CAS.
- D. Sueper, J. D. Allan, E. J. Dunlea, J. Crosier, J. R. Kimmel, P. F. DeCarlo, A. C. Aiken, J. L. Jimenez and D. R. Worsnop, Rendezvous Science, poster presentation, 2008 Search PubMed.
- J. D. Allan, A. E. Delia, H. Coe, K. N. Bower, M. R. Alfarra, J. L. Jimenez, A. M. Middlebrook, F. Drewnick, T. B. Onasch, M. R. Canagaratna, J. T. Jayne and D. R. Worsnop, A generalised method for the extraction of chemically resolved mass spectra from Aerodyne aerosol mass spectrometer data, J. Aerosol Sci., 2004, 35, 909–922 CrossRef CAS.
- P. Paatero and U. Tapper, Positive matrix factorization: A non-negative factor model with optimal utilization of error estimates of data values, Environmetrics, 1994, 5, 111–126 CrossRef.
- V. A. Lanz, M. R. Alfarra, U. Baltensperger, B. Buchmann, C. Hueglin and A. S. H. Prévôt, Source apportionment of submicron organic aerosols at an urban site by factor analytical modelling of aerosol mass spectra, Atmos. Chem. Phys., 2007, 7, 1503–1522 CrossRef CAS.
- M. Ulbrich, M. R. Canagaratna, Q. Zhang, D. R. Worsnop and J. L. Jimenez, Interpretation of organic components from Positive Matrix Factorization of aerosol mass spectrometric data, Atmos. Chem. Phys., 2009, 9, 2891–2918 CrossRef.
- J. D. Allan, P. I. Williams, W. T. Morgan, C. L. Martin, M. J. Flynn, J. Lee, E. Nemitz, G. J. Phillips, M. W. Gallagher and H. Coe, Contributions from transport, solid fuel burning and cooking to primary organic aerosols in two UK cities, Atmos. Chem. Phys., 2010, 10, 647–668 CrossRef CAS.
- Q. Zhang, J. L. Jimenez, M. R. Canagaratna, I. M. Ulbrich, N. L. Ng, D. R. Worsnop and Y. Sun, Understanding atmospheric organic aerosols via factor analysis of aerosol mass spectrometry: a review, Anal. Bioanal. Chem., 2011, 401, 3045–3067 CrossRef CAS PubMed.
- K. S. Docherty, E. A. Stone, I. M. Ulbrich, P. F. DeCarlo, D. C. Snyder, J. J. Schauer, R. E. Peltier, R. J. Weber, S. M. Murphy, J. H. Seinfeld, B. D. Grover, D. J. Eatough and J. L. Jimenez, Apportionment of primary and secondary organic aerosols in southern California during the 2005 study of organic aerosols in riverside (SOAR-1), Environ. Sci. Technol., 2008, 42, 7655–7662 CrossRef CAS PubMed.
- C. Aiken, D. Salcedo, M. J. Cubison, J. A. Huffman, P. F. DeCarlo, I. M. Ulbrich, K. S. Docherty, D. Sueper, J. R. Kimmel, D. R. Worsnop, A. Trimborn, M. Northway, E. A. Stone, J. J. Schauer, R. M. Volkamer, E. Fortner, B. de Foy, J. Wang, A. Laskin, V. Shutthanandan, J. Zheng, R. Zhang, J. Gaffney, N. A. Marley, G. Paredes-Miranda, W. P. Arnott, L. T. Molina, G. Sosa and J. L. Jimenez, Mexico City aerosol analysis during MILAGRO using high resolution aerosol mass spectrometry at the urban supersite (T0) – Part 1: Fine particle composition and organic source apportionment, Atmos. Chem. Phys., 2009, 9, 6633–6653 CrossRef.
- A. Reff, S. I. Eberly and P. V. Bhave, Receptor Modeling of Ambient Particulate Matter Data Using Positive Matrix Factorization: Review of Existing Methods, J. Air Waste Manage. Assoc., 2007, 57, 146–154 CrossRef CAS PubMed.
- E. Nemitz, J. L. Jimenez, J. A. Huffman, I. M. Ulbrich, M. R. Canagaratna, D. R. Worsnop and A. B. Guenther, An Eddy-Covariance System for the Measurement of Surface/Atmosphere Exchange Fluxes of Submicron Aerosol Chemical Species—First Application Above an Urban Area, Aerosol Sci. Technol., 2008, 42, 636–657 CrossRef CAS.
-
P. Paatero, User's Guide for Positive Matrix Factorization Programs PMF2.EXE and PMF3.EXE, University of Helsinki, Finland, 2007 Search PubMed.
- L. Drinovec, G. Močnik, P. Zotter, A. S. H. Prévôt, C. Ruckstuhl, E. Coz, M. Rupakheti, J. Sciare, T. Müller, A. Wiedensohler and A. D. A. Hansen, The dual-spot Aethalometer: an improved measurement of aerosol black carbon with real-time loading compensation, Atmos. Meas. Tech., 2015, 8, 1965–1979 CrossRef CAS.
- S. China, C. Mazzoleni, K. Gorkowski, A. C. Aiken and M. K. Dubey, Morphology and mixing state of individual freshly emitted wildfire carbonaceous particles, Nat. Commun., 2013, 4, 2122 CrossRef PubMed.
- T. Torvela, J. Tissari, O. Sippula, T. Kaivosoja, J. Leskinen, A. Virén, A. Lähde and J. Jokiniemi, Effect of wood combustion conditions on the morphology of freshly emitted fine particles, Atmos. Environ., 2014, 87, 65–76 CrossRef CAS.
- X. Gong, C. Zhang, H. Chen, S. A. Nizkorodov, J. Chen and X. Yang, Size distribution and mixing state of black carbon particles during a heavy air pollution episode in Shanghai, Atmos. Chem. Phys., 2016, 16, 5399–5411 CrossRef CAS.
- A. Lack and C. D. Cappa, Impact of brown and clear carbon on light absorption enhancement, single scatter albedo and absorption wavelength dependence of black carbon, Atmos. Chem. Phys., 2010, 10, 4207–4220 CrossRef.
- M. R. Olson, M. Victoria Garcia, M. A. Robinson, P. Van Rooy, M. A. Dietenberger, M. Bergin and J. J. Schauer, Investigation of black and brown carbon multiple-wavelength-dependent light absorption from biomass and fossil fuel combustion source emissions: BC AND BRC LIGHT ABSORPTION FROM SOURCES, J. Geophys. Res.: Atmos., 2015, 120, 6682–6697 CrossRef CAS.
- A. Lack, C. D. Cappa, D. S. Covert, T. Baynard, P. Massoli, B. Sierau, T. S. Bates, P. K. Quinn, E. R. Lovejoy and A. R. Ravishankara, Bias in Filter-Based Aerosol Light Absorption Measurements Due to Organic Aerosol Loading: Evidence from Ambient Measurements, Aerosol Sci. Technol., 2008, 42, 1033–1041 CrossRef.
- D. A. Lack and J. M. Langridge, On the attribution of black and brown carbon light absorption using the Ångström exponent, Atmos. Chem. Phys., 2013, 13, 10535–10543 CrossRef.
- R. P. Pokhrel, N. L. Wagner, J. M. Langridge, D. A. Lack, T. Jayarathne, E. A. Stone, C. E. Stockwell, R. J. Yokelson and S. M. Murphy, Parameterization of single-scattering albedo (SSA) and absorption Ångström exponent (AAE) with EC/OC for aerosol emissions from biomass burning, Atmos. Chem. Phys., 2016, 16, 9549–9561 CrossRef CAS.
- A. K. Panday, R. G. Prinn and C. Schär, Diurnal cycle of air pollution in the Kathmandu Valley, Nepal: 2. Modeling results, J. Geophys. Res., 2009, 114, D21308 CrossRef.
- T. Kitada and R. P. Regmi, Dynamics of Air Pollution Transport in Late Wintertime over Kathmandu Valley, Nepal: As Revealed with Numerical Simulation, J. Appl. Meteorol. Climatol., 2003, 42, 1770–1798 CrossRef.
- K. S. Mahata, A. K. Panday, M. Rupakheti, A. Singh, M. Naja and M. G. Lawrence, Seasonal and diurnal variations in methane and carbon dioxide in the Kathmandu Valley in the foothills of the central Himalayas, Atmos. Chem. Phys., 2017, 17, 12573–12596 CrossRef CAS.
- P. Chen, S. Kang, C. Li, M. Rupakheti, F. Yan, Q. Li, Z. Ji, Q. Zhang, W. Luo and M. Sillanpää, Characteristics and sources of polycyclic aromatic hydrocarbons in atmospheric aerosols in the Kathmandu Valley, Nepal, Sci. Total Environ., 2015, 538, 86–92 CrossRef CAS PubMed.
- P. Bonasoni, P. Laj, F. Angelini, J. Arduini, U. Bonafè, F. Calzolari, P. Cristofanelli, S. Decesari, M. C. Facchini, S. Fuzzi, G. P. Gobbi, M. Maione, A. Marinoni, A. Petzold, F. Roccato, J. C. Roger, K. Sellegri, M. Sprenger, H. Venzac, G. P. Verza, P. Villani and E. Vuillermoz, The ABC-Pyramid Atmospheric Research Observatory in Himalaya for aerosol, ozone and halocarbon measurements, Sci. Total Environ., 2008, 391, 252–261 CrossRef CAS PubMed.
- J. L. Jimenez, M. R. Canagaratna, N. M. Donahue, A. S. H. Prevot, Q. Zhang, J. H. Kroll, P. F. DeCarlo, J. D. Allan, H. Coe, N. L. Ng, A. C. Aiken, K. S. Docherty, I. M. Ulbrich, A. P. Grieshop, A. L. Robinson, J. Duplissy, J. D. Smith, K. R. Wilson, V. A. Lanz, C. Hueglin, Y. L. Sun, J. Tian, A. Laaksonen, T. Raatikainen, J. Rautiainen, P. Vaattovaara, M. Ehn, M. Kulmala, J. M. Tomlinson, D. R. Collins, M. J. Cubison, E. J. Dunlea, J. A. Huffman, T. B. Onasch, M. R. Alfarra, P. I. Williams, K. Bower, Y. Kondo, J. Schneider, F. Drewnick, S. Borrmann, S. Weimer, K. Demerjian, D. Salcedo, L. Cottrell, R. Griffin, A. Takami, T. Miyoshi, S. Hatakeyama, A. Shimono, J. Y. Sun, Y. M. Zhang, K. Dzepina, J. R. Kimmel, D. Sueper, J. T. Jayne, S. C. Herndon, A. M. Trimborn, L. R. Williams, E. C. Wood, A. M. Middlebrook, C. E. Kolb, U. Baltensperger and D. R. Worsnop, Evolution of Organic Aerosols in the Atmosphere, Science, 2009, 326, 1525–1529 CrossRef CAS PubMed.
-
Sunrise-sunset.org: Sunset and Sunrise Times[online] available from: https://www.sunrise-sunset.org (accessed 11 February 2021), 2021.
- L. Luo, Y.-Y. Zhang, H.-Y. Xiao, H.-W. Xiao, N.-J. Zheng, Z.-Y. Zhang, Y.-J. Xie and C. Liu, Spatial Distributions and Sources of Inorganic Chlorine in PM2.5 across China in Winter, Atmosphere, 2019, 10, 505 CrossRef CAS.
- Q. Zhang, J. L. Jimenez, M. R. Canagaratna, J. D. Allan, H. Coe, I. Ulbrich, M. R. Alfarra, A. Takami, A. M. Middlebrook, Y. L. Sun, K. Dzepina, E. Dunlea, K. Docherty, P. F. DeCarlo, D. Salcedo, T. Onasch, J. T. Jayne, T. Miyoshi, A. Shimono, S. Hatakeyama, N. Takegawa, Y. Kondo, J. Schneider, F. Drewnick, S. Borrmann, S. Weimer, K. Demerjian, P. Williams, K. Bower, R. Bahreini, L. Cottrell, R. J. Griffin, J. Rautiainen, J. Y. Sun, Y. M. Zhang and D. R. Worsnop, Ubiquity and dominance of oxygenated species in organic aerosols in anthropogenically-influenced Northern Hemisphere midlatitudes: UBIQUITY AND DOMINANCE OF OXYGENATED OA, Geophys. Res. Lett., 2007, 34(13), L13801, DOI:10.1029/2007GL029979.
- D. M. San Martini, E. J. Dunlea, R. Volkamer, T. B. Onasch, J. T. Jayne, M. R. Canagaratna, D. R. Worsnop, C. E. Kolb, J. H. Shorter, S. C. Herndon, M. S. Zahniser, D. Salcedo, K. Dzepina, J. L. Jimenez, J. M. Ortega, K. S. Johnson, G. J. McRae, L. T. Molina and M. J. Molina, Implementation of a Markov Chain Monte Carlo method to inorganic aerosol modeling of observations from the MCMA-2003 campaign PartII: Model application to the CENICA, Pedregal and Santa Ana sites, Atmos. Chem. Phys., 2006, 6, 4889–4904 CrossRef.
- Q. Zhang, J. L. Jimenez, D. R. Worsnop and M. Canagaratna, A Case Study of Urban Particle Acidity and Its Influence on Secondary Organic Aerosol, Environ. Sci. Technol., 2007, 41, 3213–3219 CrossRef CAS PubMed.
- D. K. Farmer, A. Matsunaga, K. S. Docherty, J. D. Surratt, J. H. Seinfeld, P. J. Ziemann and J. L. Jimenez, Response of an aerosol mass spectrometer to organonitrates and organosulfates and implications for atmospheric chemistry, Proc. Natl. Acad. Sci. U. S. A., 2010, 107, 6670–6675 CrossRef CAS PubMed.
- J. L. Fry, A. Kiendler-Scharr, A. W. Rollins, P. J. Wooldridge, S. S. Brown, H. Fuchs, W. Dubé, A. Mensah, M. dal Maso, R. Tillmann, H.-P. Dorn, T. Brauers and R. C. Cohen, Organic nitrate and secondary organic aerosol yield from NO3 oxidation of β-pinene evaluated using a gas-phase kinetics/aerosol partitioning model, Atmos. Chem. Phys., 2009, 9, 1431–1449 CrossRef CAS.
- A. Kiendler-Scharr, A. Mensah, E. Friese, D. Topping, E. Nemitz, A. S. H. Prevot, M. Äijälä, J. Allan, F. Canonaco, M. Canagaratna, S. Carbone, M. Crippa, M. D. Osto, D. A. Day, P. D. Carlo, C. F. D. Marco, H. Elbern, A. Eriksson, E. Freney, L. Hao, H. Herrmann, L. Hildebrandt, R. Hillamo, J. L. Jimenez, A. Laaksonen, G. McFiggans, C. Mohr, C. O'Dowd, R. Otjes, J. Ovadnevaite, S. N. Pandis, L. Poulain, P. Schlag, K. Sellegri, E. Swietlicki, P. Tiitta, A. Vermeulen, A. Wahner, D. Worsnop and H.-C. Wu, Ubiquity of organic nitrates from nighttime chemistry in the European submicron aerosol, Geophys. Res. Lett., 2016, 43, 7735–7744 CrossRef CAS.
- N. L. Ng, M. R. Canagaratna, Q. Zhang, J. L. Jimenez, J. Tian, I. M. Ulbrich, J. H. Kroll, K. S. Docherty, P. S. Chhabra, R. Bahreini, S. M. Murphy, J. H. Seinfeld, L. Hildebrandt, N. M. Donahue, P. F. DeCarlo, V. A. Lanz, A. S. H. Prévôt, E. Dinar, Y. Rudich and D. R. Worsnop, Organic aerosol components observed in Northern Hemispheric datasets from Aerosol Mass Spectrometry, Atmos. Chem. Phys., 2010, 10, 4625–4641 CrossRef CAS.
- X. Ge, A. Setyan, Y. Sun and Q. Zhang, Primary and secondary organic aerosols in Fresno, California during wintertime: Results from high resolution aerosol mass spectrometry, J. Geophys. Res.: Atmos., 2012, 117, D19301, DOI:10.1029/2012JD018026.
- M. Crippa, I. El Haddad, J. G. Slowik, P. F. DeCarlo, C. Mohr, M. F. Heringa, R. Chirico, N. Marchand, J. Sciare, U. Baltensperger and A. S. H. Prévôt, Identification of marine and continental aerosol sources in Paris using high resolution aerosol mass spectrometry, J. Geophys. Res.: Atmos., 2013, 118, 1950–1963, DOI:10.1002/JGRD50151.
-
J. D. Goetz, M. R. Giordano, C. E. Stockwell, T. J. Christian, R. Maharjan, S. Adhikari, P. V. Bhave, P. S. Praveen, A. K. Panday, T. Jayarathne, E. A. Stone, R. J. Yokelson, and P. F. DeCarlo: Speciated On-line PM1 from South Asian Combustion Sources: Part II, AMS Mass Spectral Profiles and Wavelength Dependence, 2022.
- C. Mohr, P. F. DeCarlo, M. F. Heringa, R. Chirico, J. G. Slowik, R. Richter, C. Reche, A. Alastuey, X. Querol, R. Seco, J. Peñuelas, J. L. Jiménez, M. Crippa, R. Zimmermann, U. Baltensperger and A. S. H. Prévôt, Identification and quantification of organic aerosol from cooking and other sources in Barcelona using aerosol mass spectrometer data, Atmos. Chem. Phys., 2012, 12, 1649–1665 CrossRef CAS.
-
C. Aiken, P. F. DeCarlo, J. H. Kroll, D. R. Worsnop, J. A. Huffman, K. S. Docherty, I. M. Ulbrich, C. Mohr, J. R. Kimmel, D. Sueper, Y. Sun, Q. Zhang, A. Trimborn, M. Northway, P. J. Ziemann, M. R. Canagaratna, T. B. Onasch, M. R. Alfarra, A. S. H. Prevot, J. Dommen, J. Duplissy, A. Metzger, U. Baltensperger and J. L. Jimenez, O/C and OM/OC Ratios of Primary, Secondary, and Ambient Organic Aerosols with High-Resolution Time-of-Flight Aerosol Mass Spectrometry, https://pubs.acs.org/doi/abs/10.1021/es703009q, (accessed 8 December 2020) Search PubMed.
- Q. Zhang, M. R. Alfarra, D. R. Worsnop, J. D. Allan, H. Coe, M. R. Canagaratna and J. L. Jimenez, Deconvolution and Quantification of Hydrocarbon-like and Oxygenated Organic Aerosols Based on Aerosol Mass Spectrometry, Environ. Sci. Technol., 2005, 39, 4938–4952 CrossRef CAS PubMed.
- P. F. DeCarlo, I. Ulbrich, J. Crounse, B. de Foy, E. Dunlea, A. Aiken, D. Knapp, A. Weinheimer, T. Campos, P. Wennberg and J. Jimenez, Investigation of the sources and processing of organic aerosol over the Central Mexican Plateau from aircraft measurements during MILAGRO, Atmos. Chem. Phys. Discuss., 2010, 10(12), 5257–5280, DOI:10.5194/acp-10-5257-2010.
- A. Chakraborty, D. Bhattu, T. Gupta, S. N. Tripathi and M. R. Canagaratna, Real-time measurements of ambient aerosols in a polluted Indian city: Sources, characteristics, and processing of organic aerosols during foggy and nonfoggy periods, J. Geophys. Res.: Atmos., 2015, 120, 9006–9019 CrossRef CAS.
- X. F. Huang, L. Y. He, M. Hu, M. R. Canagaratna, Y. Sun, Q. Zhang, T. Zhu, L. Xue, L. W. Zeng, X.-G. Liu, Y.-H. Zhang, J. T. Jayne, N. L. Ng and D. R. Worsnop, Highly time-resolved chemical characterization of atmospheric submicron particles during 2008 Beijing Olympic Games using an Aerodyne High-Resolution Aerosol Mass Spectrometer, Atmos. Chem. Phys., 2010, 10, 8933–8945 CrossRef CAS.
- C. Mohr, J. A. Huffman, M. J. Cubison, A. C. Aiken, K. S. Docherty, J. R. Kimmel, I. M. Ulbrich, M. Hannigan and J. L. Jimenez, Characterization of Primary Organic Aerosol Emissions from Meat Cooking, Trash Burning, and Motor Vehicles with High-Resolution Aerosol Mass Spectrometry and Comparison with Ambient and Chamber Observations, Environ. Sci. Technol., 2009, 43, 2443–2449 CrossRef CAS PubMed.
- K. Dzepina, R. M. Volkamer, S. Madronich, P. Tulet, I. M. Ulbrich, Q. Zhang, C. D. Cappa, P. J. Ziemann and J. L. Jimenez, Evaluation of recently-proposed secondary organic aerosol models for a case study in Mexico City, Atmos. Chem. Phys., 2009, 9, 5681–5709 CrossRef CAS.
- M. R. Alfarra, A. S. H. Prevot, S. Szidat, J. Sandradewi, S. Weimer, V. A. Lanz, D. Schreiber, M. Mohr and U. Baltensperger, Identification of the Mass Spectral Signature of Organic Aerosols from Wood Burning Emissions, Environ. Sci. Technol., 2007, 41, 5770–5777 CrossRef CAS PubMed.
-
S. Pariyar, T. Das and T. Ferdous, Environment And Health Impact For Brick Kilns In Kathmandu Valley, https://paper/Environment-And-Health-Impact-For-Brick-Kilns-In-Pariyar-Das/0aa991db45983451bbc0df80ed321758c1e1bc78, (accessed 8 December 2020).
- S. Khanal, Wildfire trends in Nepal based on MODIS burnt-area data, Banko Janakari, 2015, 25, 76–79 CrossRef.
- C. Wiedinmyer, R. J. Yokelson and B. K. Gullett, Global Emissions of Trace Gases, Particulate Matter, and Hazardous Air Pollutants from Open Burning of Domestic Waste, Environ. Sci. Technol., 2014, 48, 9523–9530 CrossRef CAS PubMed.
- T. J. Christian, R. J. Yokelson, B. Cárdenas, L. T. Molina, G. Engling and S.-C. Hsu, Trace gas and particle emissions from domestic and industrial biofuel use and garbage burning in central Mexico, Atmos. Chem. Phys., 2010, 10, 565–584 CrossRef CAS.
- M. Zhong, E. Saikawa, A. Avramov, C. Chen, B. Sun, W. Ye, W. C. Keene, R. J. Yokelson, T. Jayarathne, E. A. Stone, M. Rupakheti and A. K. Panday, Nepal Ambient Monitoring and Source Testing Experiment (NAMaSTE): emissions of particulate matter and sulfur dioxide from vehicles and brick kilns and their impacts on air quality in the Kathmandu Valley, Nepal, Atmos. Chem. Phys., 2019, 19, 8209–8228 CrossRef CAS.
- Y. J. Zhang, L. L. Tang, Z. Wang, H. X. Yu, Y. L. Sun, D. Liu, W. Qin, F. Canonaco, A. S. H. Prévôt, H. L. Zhang and H. C. Zhou, Insights into characteristics, sources, and evolution of submicron aerosols
during harvest seasons in the Yangtze River delta region, China, Atmos. Chem. Phys., 2015, 15, 1331–1349 CrossRef.
- L. Y. He, X.-F. Huang, L. Xue, M. Hu, Y. Lin, J. Zheng, R. Zhang and Y.-H. Zhang, Submicron aerosol analysis and organic source apportionment in an urban atmosphere in Pearl River Delta of China using high-resolution aerosol mass spectrometry, J. Geophys. Res.: Atmos., 2011, 116, D12304, DOI:10.1029/2010JD014566.
- N. Takegawa, T. Miyakawa, Y. Kondo, J. L. Jimenez, Q. Zhang, D. R. Worsnop and M. Fukuda, Seasonal and diurnal variations of submicron organic aerosol in Tokyo observed using the Aerodyne aerosol mass spectrometer, J. Geophys. Res.: Atmos., 2006, 111, D11, DOI:10.1029/2005JD006515.
- R. Volkamer, J. L. Jimenez, F. S. Martini, K. Dzepina, Q. Zhang, D. Salcedo, L. T. Molina, D. R. Worsnop and M. J. Molina, Secondary organic aerosol formation from anthropogenic air pollution: Rapid and higher than expected, Geophys. Res. Lett., 2006, 33, L17811, DOI:10.1029/2006GL026899.
- Y. Kondo, Y. Miyazaki, N. Takegawa, T. Miyakawa, R. J. Weber, J. L. Jimenez, Q. Zhang and D. R. Worsnop, Oxygenated and water-soluble organic aerosols in Tokyo, J. Geophys. Res.: Atmos., 2007, 112, D01203, DOI:10.1029/2006JD007056.
- S. C. Herndon, T. B. Onasch, E. C. Wood, J. H. Kroll, M. R. Canagaratna, J. T. Jayne, M. A. Zavala, W. B. Knighton, C. Mazzoleni, M. K. Dubey, I. M. Ulbrich, J. L. Jimenez, R. Seila, J. A. de Gouw, J. Fast, L. T. Molina, C. E. Kolb and D. R. Worsnop, Correlation of secondary organic aerosol with odd oxygen in Mexico City, Geophys. Res. Lett., 2008, 35(15), L15804, DOI:10.1029/2008GL034058.
- L. I. Kleinman, S. R. Springston, P. H. Daum, Y.-N. Lee, L. J. Nunnermacker, G. I. Senum, J. Wang, J. Weinstein-Lloyd, M. L. Alexander, J. Hubbe, J. Ortega, M. R. Canagaratna and J. Jayne, The time evolution of aerosol composition over the Mexico City plateau, Atmos. Chem. Phys., 2008, 8, 1559–1575 CrossRef CAS.
- J. A. de Gouw, D. Welsh-Bon, C. Warneke, W. C. Kuster, L. Alexander, A. K. Baker, A. J. Beyersdorf, D. R. Blake, M. Canagaratna, A. T. Celada, L. G. Huey, W. Junkermann, T. B. Onasch, A. Salcido, S. J. Sjostedt, A. P. Sullivan, D. J. Tanner, O. Vargas, R. J. Weber, D. R. Worsnop, X. Y. Yu and R. Zaveri, Emission and chemistry of organic carbon in the gas and aerosol phase at a sub-urban site near Mexico City in March 2006 during the MILAGRO study, Atmos. Chem. Phys., 2009, 9, 3425–3442 CrossRef CAS.
- E. C. Wood, M. R. Canagaratna, S. C. Herndon, T. B. Onasch, C. E. Kolb, D. R. Worsnop, J. H. Kroll, W. B. Knighton, R. Seila, M. Zavala, L. T. Molina, P. F. DeCarlo, J. L. Jimenez, A. J. Weinheimer, D. J. Knapp, B. T. Jobson, J. Stutz, W. C. Kuster and E. J. Williams, Investigation of the correlation between odd oxygen and secondary organic aerosol in Mexico City and Houston, Atmos. Chem. Phys., 2010, 10, 8947–8968 CrossRef CAS.
- Y. Kondo, Y. Morino, M. Fukuda, Y. Kanaya, Y. Miyazaki, N. Takegawa, H. Tanimoto, R. McKenzie, P. Johnston, D. R. Blake, T. Murayama and M. Koike, Formation and transport of oxidized reactive nitrogen, ozone, and secondary organic aerosol in Tokyo, J. Geophys. Res.: Atmos., 2008, 113, D21310, DOI:10.1029/2008JD010134.
- J. A. Huffman, K. S. Docherty, C. Mohr, M. J. Cubison, I. M. Ulbrich, P. J. Ziemann, T. B. Onasch and J. L. Jimenez, Chemically-Resolved Volatility Measurements of Organic Aerosol from Different Sources, Environ. Sci. Technol., 2009a, 43(14), 5351–5357, DOI:10.1021/es803539d.
- M. J. Cubison, A. M. Ortega, P. L. Hayes, D. K. Farmer, D. Day, M. J. Lechner, W. H. Brune, E. Apel, G. S. Diskin, J. A. Fisher, H. E. Fuelberg, A. Hecobian, D. J. Knapp, T. Mikoviny, D. Riemer, G. W. Sachse, W. Sessions, R. J. Weber, A. J. Weinheimer, A. Wisthaler and J. L. Jimenez, Effects of aging on organic aerosol from open biomass burning smoke in aircraft and laboratory studies, Atmos. Chem. Phys., 2011, 11, 12049–12064 CrossRef.
- R. J. Yokelson, J. D. Crounse, P. F. DeCarlo, T. Karl, S. Urbanski, E. Atlas, T. Campos, Y. Shinozuka, V. Kapustin, A. D. Clarke, A. Weinheimer, D. J. Knapp, D. D. Montzka, J. Holloway, P. Weibring, F. Flocke, W. Zheng, D. Toohey, P. O. Wennberg, C. Wiedinmyer, L. Mauldin, A. Fried, D. Richter, J. Walega, J. L. Jimenez, K. Adachi, P. R. Buseck, S. R. Hall and R. Shetter, Atmos. Chem. Phys., 2009, 9, 5785–5812 CrossRef CAS.
- Y. Yu, B. Galle, A. Panday, E. Hodson, R. Prinn and S. Wang, Observations of high rates of NO2-HONO conversion in the nocturnal atmospheric boundary layer in Kathmandu, Nepal, Atmos. Chem. Phys., 2009, 9, 6401–6415 CrossRef CAS.
- J. Kroll and J. Seinfeld, Chemistry of secondary organic aerosol: Formation and evolution of low-volatility organics in the atmosphere, Atmos. Environ., 2008, 42, 3593–3624 CrossRef CAS.
- J. Yin, S. A. Cumberland, R. M. Harrison, J. Allan, D. E. Young, P. I. Williams and H. Coe, Receptor modelling of fine particles in southern England using CMB including comparison with AMS-PMF factors, Atmos. Chem. Phys., 2015, 15, 2139–2158 CrossRef CAS.
- N. L. Ng, M. R. Canagaratna, J. L. Jimenez, Q. Zhang, I. M. Ulbrich and D. R. Worsnop, Real-Time Methods for Estimating Organic Component Mass Concentrations from Aerosol Mass Spectrometer Data, Environ. Sci. Technol., 2011, 45, 910–916 CrossRef CAS PubMed.
- M. R. Canagaratna, J. L. Jimenez, J. H. Kroll, Q. Chen, S. H. Kessler, P. Massoli, L. Hildebrandt Ruiz, E. Fortner, L. R. Williams, K. R. Wilson, J. D. Surratt, N. M. Donahue, J. T. Jayne and D. R. Worsnop, Elemental ratio measurements of organic compounds using aerosol mass spectrometry: characterization, improved calibration, and implications, Atmos. Chem. Phys., 2015, 15, 253–272 CrossRef.
- E. A. Stone, D. C. Snyder, R. J. Sheesley, A. P. Sullivan, R. J. Weber and J. J. Schauer, Source apportionment of fine organic aerosol in Mexico City during the MILAGRO experiment 2006, Atmos. Chem. Phys., 2008, 8, 1249–1259 CrossRef CAS.
- C. Weyant, V. Athalye, S. Ragavan, U. Rajarathnam, D. Lalchandani, S. Maithel, E. Baum and T. C. Bond, Emissions from South Asian Brick Production, Environ. Sci. Technol., 2014, 48, 6477–6483 CrossRef CAS PubMed.
- M. O. Andreae and P. Merlet, Emission of trace gases and aerosols from biomass burning, Global Biogeochem. Cycles, 2001, 15, 955–966 CrossRef CAS.
- Y. Zhu, W. C. Hinds, S. Kim, S. Shen and C. Sioutas, Study of ultrafine particles near a major highway with heavy-duty diesel traffic, Atmos. Environ., 2002, 36, 4323–4335 CrossRef CAS.
- R. Chirico, P. F. DeCarlo, M. F. Heringa, T. Tritscher, R. Richter, A. S. H. Prévôt, J. Dommen, E. Weingartner, G. Wehrle, M. Gysel, M. Laborde and U. Baltensperger, Impact of aftertreatment devices on primary emissions and secondary organic aerosol formation potential from in-use diesel vehicles: results from smog chamber experiments, Atmos. Chem. Phys., 2010, 10, 11545–11563 CrossRef CAS.
- I. El Haddad, B. D'Anna, B. Temime-Roussel, M. Nicolas, A. Boreave, O. Favez, D. Voisin, J. Sciare, C. George, J.-L. Jaffrezo, H. Wortham and N. Marchand, Towards a better understanding of the origins, chemical composition and aging of oxygenated organic aerosols: case study of a Mediterranean industrialized environment, Marseille, Atmos. Chem. Phys., 2013, 13, 7875–7894 CrossRef.
Footnotes |
† Electronic supplementary information (ESI) available. See https://doi.org/10.1039/d2ea00008c |
‡ Now at: Aerodyne Research Inc, Billerica, MA, USA. |
§ Now at: Univ Paris Est Creteil and Université de Paris, CNRS, LISA, Créteil, France. |
¶ Now at: Laboratory for Atmospheric and Space Physics, University of Colorado at Boulder, Boulder, CO, USA. |
|| Now at: Duke University, Durham, NC, USA. |
** Now at: Ullens Education Foundation, Khumaltar, Lalitpur, Nepal. |
|
This journal is © The Royal Society of Chemistry 2022 |
Click here to see how this site uses Cookies. View our privacy policy here.