DOI:
10.1039/D2FO02739A
(Paper)
Food Funct., 2022,
13, 12219-12233
Saffron extract (Safr'Inside™) improves anxiety related behaviour in a mouse model of low-grade inflammation through the modulation of the microbiota and gut derived metabolites†
Received
15th September 2022
, Accepted 26th October 2022
First published on 29th October 2022
Abstract
Treatment of anxiety and depression predominantly centres around pharmacological interventions, which have faced criticism for their associated side effects, lack of efficacy and low tolerability. Saffron, which is reportedly well tolerated in humans, has been recognised for its antidepressant and anti-anxiety properties. Indeed, we previously reported upon the efficacy of saffron extract supplementation in healthy adults with subclinical anxiety. However, the molecular aetiology remains unclear. In a rodent model of low-grade chronic inflammation, we explored the impact of a saffron extract (Safr'Inside™) supplemented at a physiological dose, which equated to 22 ± 1.2 mg per day human equivalent dose for a person of 60 kg. Behavioural tests (Open Field task, Y maze, Novel object recognition), caecal 16S rRNA microbial sequencing, caecal 1H NMR metabolomic analysis and 2DE brain proteomic analyses were completed to probe gut–brain axis interactions. Time occupying the centre of the Open Field maze (OF) was increased by 62% in saffron supplemented animals. This improvement in anxiety-related behaviour coincided with gut microbial shifts, notably Akkermansia, Muribaculaceae, Christensenellacae and Alloprevotella which significantly increased in response to saffron supplementation. Akkermansia and Muribaculaceae abundance negatively correlated with the neurotoxic metabolite dimethylamine which was reduced in saffron supplemented animals. Brain proteomic analysis highlighted several significantly altered proteins including ketimine reductase mu-crystallin which also correlated with dimethylamine concentration. Both dimethylamine and ketimine reductase mu-crystallin were associated with OF performance. This may be indicative of a novel interaction across the gut–brain axis which contributes to anxiety-related disorders.
1. Introduction
One in four people will experience some form of mental health disorder in their lifetime.1 Of these conditions, depression and anxiety which frequently co-occur together are acknowledged as the most common2 affecting 264 million and 284 million people worldwide respectively.3 As such, mental disorders constitute a major cause of disability, conferring substantial economic and social implications, costing the global economy US$ 1 trillion each year.4 The COVID-19 pandemic accentuated these issues5,6 and exposed the challenges associated with treatments, emphasising the need to improve both effectiveness and accessibility.
Treatment of anxiety and depression has predominantly centred around pharmacological interventions. Despite this, clinical evidence to date shows that traditional antidepressants and anxiolytics approaches are ineffective for a subset of individuals, with efficacy and/or tolerability issues reported.7,8 This likely stems from an inadequate understanding of the disease process which remains only partially understood. In an attempt to improve the outlook for such individuals, other non-pharmaceutical approaches (e.g., electroconvulsive therapy, psychotherapy, and other somatic treatments) have been developed, many of which purportedly confer greater efficacy and patient outcome.9 However, as with pharmacological treatments, considerable interindividual variability in treatment outcome is evident,10,11 emphasising that a “one size fits all” approach to anxiety and depression treatment may not be achievable. Developing novel and well-tolerated interventions to bolster the current repertoire may be of great benefit to those who do not respond adequately to these established approaches.
Diet has long been associated with mental health and mood.12,13 Indeed, observational studies provide compelling evidence that adherence to a Mediterranean diet reduces anxiety and depression risk.14,15 Although the mechanisms responsible for such effects are conceivably complex and multifactorial,16 diet-mediated modulation of the gut microbiota, a collection of approximately 100 trillion microbes17 is emerging as a key contributing factor, providing a route (microbial–gut–brain axis) by which diet can modulate neuronal function.18,19 The composition of this microbiome is central in health and disease, providing a large repertoire of genes, antigens and metabolites that can regulate immune and metabolic functions.20 A close/delicate relationship exists between diet and gut microbiota composition, dysfunction of which is increasingly associated with mental illnesses.
Saffron, obtained from the dried stigmas of Crocus sativus21 has been reported to have anti-anxiety and anti-depressant properties as determined by us and others in both preclinical22–24 and clinical trials.25–27 Regarded as safe/well-tolerated, the effectiveness of saffron in the treatment of both anxiety and depression appears comparable to that of pharmaceutical intervention. Despite these positive reports emphasising saffron's anti-anxiety/depressive properties, the mechanistic picture remains incomplete. Of the limited evidence available, saffron's anti-inflammatory properties are most frequently cited,28 along with modulation of the monoaminergic neurotransmission22,23 and oxidative stress,27 indicating that these mechanisms may be an integral part of the process. Despite evidence of saffron-mediated gut microbial shift,29,30 this has not been explored in the context of mental health/anxiety.
To address this knowledge gap, we investigated the impact of Safr'Inside™, (a standardized saffron extract) supplementation in an LPS model of low-grade inflammation. Gut microbiota speciation and metabolism were conducted by 16S rRNA sequencing and 1H-NMR respectively, together with behavioural assessments of cognition and brain proteomic analysis in an attempt to probe gut–brain axis interactions. Using a model of low-grade inflammation, we investigated the supplementation of Safr'Inside™ on gut microbial and metabolite composition, and further link this to changes in brain proteomic profiles and cognitive performance.
2. Materials and methods
2.1. Study approval
All experimental procedures and protocols performed were reviewed and approved by the Animal Welfare and Ethical Review Body (AWERB) and were conducted in accordance with the specification of the United Kingdom Animal Scientific Procedures Act, 1986 (Amendment Regulations 2012). Reporting of the study outcomes comply with the ARRIVE (Animal Research: Reporting of In Vivo Experiments) guidelines31
2.2. Overview of experimental procedure
Thirty male C57BL/6J mice sourced from Charles River (Margate, UK) were maintained in individually ventilated cages (n = 5 per cage), within a controlled environment (21 ± 2 °C; 12 h light/dark cycle; light from 7:00 AM) and fed ad libitum on a standard chow diet (RM3-P; Special Diet Services (SDS, Horley UK) up to the age of 10 weeks, ensuring normal development and stabilisation of the microbiota.32 After which mice were transferred onto one of two diets, namely control (AIN93-M) and saffron supplemented diet (Safr'Inside™; SAFF) for the remaining of the experimentation. The SAFF diet consisted of the background control diet (AIN93-M) supplemented with 44 mg kg−1 diet of Safr'Inside™ (Activ'Inside, Beychac-et-Caillau, France), a full-spectrum standardised saffron extract obtained according to the patent FR 3054443 – WO2017EP69200. This extract contains ∼150 compounds as measured by UHPLC method33 of which: crocins (mainly trans-4-GG, trans-3-Gg; cis-4-GG, trans-2-G) >3%, safranal >0.2%, picrocrocin derivatives (mainly picrocrocin, HTCC) >1%, and kaempferol derivatives (mainly kaempferol-3-sophoroside-7-glucoside, kaempferol-3-sophoroside) >0.1%, are considered the most bioactive constituents (see ESI Table S1† for full dietary composition). Diets were prepared by Research Diet Inc. (New Brunswick, USA) to comply with animal nutrition requirements. Chronic low-grade inflammation was induced through weekly intraperitoneal injections (IP) of 0.5 mg kg−1 lipopolysaccharide (LPS) for 8 weeks as described previously34 or a SMAM injection consisting of saline. At the end of the experiments and following the completion of behavioural testing (see below for detail), 5-month-old animals were sedated with a mixture of isoflurane (1.5%) in nitrous oxide (70%) and oxygen (30%) and transcardially perfused with ice-cold saline containing 10 UI heparin (Sigma-Aldrich, UK). Sera were isolated via centrifugation at 2,000g for 10 min. Brains were rapidly removed, halved, snap frozen and stored at −80 °C until biochemical analysis. Additionally, caeca were removed, weighed and contents were gently extracted. Samples were then snap-frozen in liquid nitrogen and stored at −80 °C until further analysis.
2.3. Behavioural assessment
All behavioural tests were performed at the experimental endpoint after the 8-week intervention. Prior to commencing, a visual placing test was performed on each animal to ensure animals were not visually impaired.35 All behavioural tests were analysed using the Ethovision software (Tracksys Ltd, Nottingham, UK).
The Open Field (OF) task used as a measure of anxiety,36 was performed as described previously.37 Animals were individually placed within the (50 cm × 50 cm × 50 cm) square arena illuminated with dim lighting (100 lux) and were allowed to move freely for a 10-minute period. Mice were tracked using Ethovision software which determined travel distance, velocity and time spent in the centre/periphery of the maze respectively.
The novel object recognition (NOR) task, a measure of recognition memory was performed as described previously,38,39 with slight modifications. All experimentation was performed in dim lighting (100 lux) lighting. Briefly, on day 1 (habituation), the animal was placed into an empty maze for 10 minutes. On day 2, animals were conditioned to a single object for a 10-minute period. On day 3, mice were exposed to 2 identical objects for 15 minutes. Following an inter-trial interval of one hour, mice were placed back within the testing arena now containing one familiar object and one novel object. Videos were analysed for a 5-minute period, after which if an accumulative object exportation of 8 seconds failed to be reached, the analysis continued for the full 10 min or until 8 seconds was achieved. Those not achieving 8 seconds of exploration were excluded from the analysis.40 Discrimination index was calculated as follows DI = (TN − TF)/(TN + TF), where TN is the time spent exploring the novel object and TF is the time spent exploring the familiar object.
Y maze spontaneous alternation test, a measure of spatial working memory, was performed as previously described.41 Ethovision software analysed each animal for 7 minutes recording zone transitioning and locomotor activity. Spontaneous alternation was calculated using the following formula: (number of alternations/max number of alternations × 100).
A shortened version of the Barnes maze as previously described,42 was performed with slight modifications to assess spatial retrieval memory. Briefly, the maze consisted of a brightly illuminated (800 lux lighting) circular platform (92 cm diameter), with 20 evenly distributed holes located around the circumference and visual cues (4 simple shapes) placed at the periphery. The experiment was conducted over a 4-day period. On day 1 (habituation) animals were placed into the centre of the maze for 2 minutes and were able to explore freely, if animal did not find the escape box within this time frame the animal was guided to it after which they remined in the box for a further 2 minutes. Following habituation each mouse was tested/trained on their ability to locate the escape box on days 1–3 (once on day one, three times on day two and twice on day three). On day 4, a probe test was conducted, the maze was rotated 90°, the escape box was removed, and mice were placed in the centre of the maze in which they were free to navigate for 1 minute. Percentage time in the correct quadrant was determined using Ethovision software.
2.4. Microbial 16S rRNA extraction and sequencing
Microbial DNA was isolated from approximately 50 mg of caecal content using the QIAamp PowerFecal Pro DNA Kit (Qiagen, Manchester, UK) as per the manufacturer's instructions. DNA quantity was assessed using a Nanodrop 2000 Spectrophotometer (Fisher Scientific, UK). Quality assessment was performed by agarose gel electrophoresis to detect DNA integrity, purity, fragment size and concentration. The 16S rRNA amplicon sequencing of the V3–V4 hypervariable region was performed with an Illumina NovaSeq 6000 PE250. Sequence analysis was performed by Uparse software (Uparse v7.0.1001),43 using all the effective tags. Sequences with ≥97% similarity were assigned to the same OTUs. A representative sequence for each OTU was screened for further annotation. For each representative sequence, Mothur software was performed against the SSUrRNA database of SILVA Database 138.44 OTUs abundance information was normalised using a standard of sequence number corresponding to the sample with the least sequences. Alpha-diversity was assessed using both Chao1 and Shannon H diversity index whilst beta diversity was assessed using Bray–Curtis. Statistical significance was determined by Kruskal–Wallis or Permutational Multivariate Analysis of Variance (PERMANOVA). Comparisons at the phylum, family and genus level were made using classical univariate analysis using Kruskal–Wallis combined with a false discovery rate (FDR) approach used to correct for multiple testing.
2.5.
1H NMR metabolomics
Caecal metabolites were analysed and quantified by 1H NMR analysis. The preparation method was similar to that previously described.45,46 Briefly, frozen caecal contents were thoroughly mixed at 5,000 rpm in a Precellys®24 (Bertin Technologies, France) and diluted to a faeces-to-buffer ratio of 13 (e.g., 50 mg faeces in 750 μL buffer) by adding deuterated phosphate buffer (1.9 mM Na2HPO4, 8.1 mM NaH2PO4, and 1 mM sodium 3-(trimethysilyl)-propionate-d4 in deuterated water (Goss Scientifics, Crewe, United Kingdom)). After mixing and centrifugation, 500 μl was transferred into a 5 mm NMR tube for spectral acquisition. High resolution 1H NMR spectra were recorded on a 600 MHz Bruker Avance spectrometer fitted with a 5 mm TCI proton-optimized triple resonance NMR inverse cryoprobe and a 24-slot autosampler (Bruker, Rheinstetten, Germany). Sample temperature was controlled at 300 K. Each spectrum consisted of 128 scans of 65
536 complex data points with a spectral width of 20 ppm (acquisition time 2.6 s). The noesypr1d presaturation sequence was used to suppress the residual water signal with low power selective irradiation at the water frequency during the recycle delay (D1 = 2 s) and mixing time (D8 = 0.01 s). A 90° pulse length of 11.4 μs was set for all samples. Spectra were transformed with a 0.1 Hz line broadening and zero filling, manually phased, baseline corrected, and referenced by setting the trimethylsilylpropanoic acid methyl signal to 0 ppm. Metabolites were identified using information found in the literature or on the web (Human Metabolome Database, https://www.hmdb.ca/) and quantified using the software Chenomx® NMR Suite 8.6™.
2.6. Proteomic analysis
For proteomic analysis, left brain samples (about 0.2 g of tissue) were transferred into a pre-cooled potter, resuspended in 6 volumes (w/v) of rehydration solution (7 M urea, 2 M thiourea, 4% CHAPS, 60 mM dithiothreitol and 0.002% bromophenol blue) added with 50 mM NaF, 2 mM Na3VO4, 10 mM β-glycerophosphate, 1 μL/0.02 g tissue protease inhibitors, 1 μM trichostatin A, 10 mM nicotinamide, and homogenized (15 strokes). After sonication (1 min, 2 times) homogenates were allowed to rehydrate for 1 h at room temperature (RT) with occasional stirring. Thereafter, the solution was centrifuged at 16
000g for 20 min at RT. Protein concentration of the resulting supernatant was determined using the Pierce 660 protein assay (ThermoFisher Scientific). BSA was used as a standard.
The 2DE was carried out as previously described.47 Briefly, 200 μg of proteins were filled up to 350 μL in rehydration solution. Immobiline IPG BlueStrip (SERVA Electrophoresis, GmbH, Heidelberg, Germany), 18 cm, linear-gradient (pH 3–10) were rehydrated overnight in the sample and then transferred to the Ettan IPGphor 2 (GE Health Care Europe; Uppsala, Sweden) for isoelectrofocusing (IEF). The second dimension (Sodium Dodecyl Sulphate-Polyacrylamide Gel Electrophoresis; SDS-PAGE) was carried out by transferring the proteins to 12% polyacrylamide, running at 45 mA per gel and 14 °C for about 7 h, using the Protean® Plus Dodeca Cell (Bio-Rad). The gels were stained with Ruthenium II tris (bathophenanthroline disulfonate) and tetrasodium salt (Cyanagen Srl, Bologna, Italy) (RuBP). ImageQuant LAS4010 (GE Health Care) was used for the acquisition of images. The analysis of images was performed using Same Spot (v4.1, TotalLab; Newcastle Upon Tyne, UK) software. The spot volume ratios among the different conditions (Control, LPS, and SAFF's supplementation) were calculated using the average spot normalised volume of three biological replicates. The software included statistical analysis calculations.
For proteins identification, the gel pieces were trypsin digested as previously described.48 After the digestion protocol, peptides were desalted and concentrated by C18 ZipTip (Millipore) according to the manufacturer suggestions and eluted directly on a Ground Steel plate (Bruker-Daltonics, Bremen, Germany) with 2 μL of a solution of 5 mg mL−1 α-ciano-4-hydroxycinnamic acid (HCCA) (dissolved in 50% acetonitrile, 0.1% trifluoroacetic acid (TFA)). MS spectra were recorded manually on the AutoFlex Speed MALDI-TOF/TOF spectrometer (Bruker Daltonics) operated in positive reflectron mode. Cubic enhanced external calibration of the TOF tube was performed using a standard solution of albumin tryptic peptides, mixed 1
:
1 with the same 5 mg mL−1 α-ciano-4-hydroxycinnamic acid solution. The laser frequency was set to 1 kHz and the number of acquired shots was determined for each sample based on signal intensity. FlexAnalysis v. 3.3 was used to process the raw data and generate the peak list to be submitted to the database search using BioTools 3.2 exploiting the free version of MASCOT search engine (version 2.8.0 at https://www.matrixscience.com) against Uniprot/Swiss-Pro non-redundant database version 2021–04 restricted to the Mus musculus taxonomy.
2.7. Statistical analysis
Data analysis was performed in GraphPad Prism version 8 (GraphPad Software, CA, USA). All data are presented as min to max box-and-whiskers plots unless otherwise stated. After identifying outliers using the ROUT method (q = 1%), data were checked for normality/equal variances using the Shapiro–Wilk test. For analysis of dietary intervention, an ANOVA or Kruskal Wallis test was used followed by Tukey or Dunns's multiple comparison depending on the normality of data. P values of less than 0.05 were considered statistically significant.
Statistical analysis of metabolomics data was carried out using Metaboanalyst 5.0.49 Data was normalised by sum, scaled by autoscaling and square root-transformed. Partial Least-Squares Discriminant Analysis (PLS-DA) was employed to illustrate the clustering of different metabolites across groups. Univariate Analysis was carried out by one-way ANOVA, followed by Tukey HSD. Dendrogram and heatmaps were created with Spearman and Ward. Heatmap shows the significant metabolites based upon ANOVA results.
Correlation analysis between metabolomics data and microbiome data was conducted using M2IA.50 Missing values were filtered if present in more than 80% of samples or the relative standard deviation was smaller than 30%.51 The remaining missing data values were handled using random forest. Data was normalised using total sum scaling. All other correlation analyses were conducted using Spearman's rank-order correlation analysis.52
3. Results
3.1. Chronic low dose LPS exposure and Safr'Inside™ supplementation have no impact upon body weight gain
The experiments were conducted as depicted in Fig. 1A. Whilst body weight increased over the 8-week experiment, it did not significantly fluctuate across the experimental groups (Fig. 1B). Neither LPS treatment nor SAFF intervention influenced overall body weight gain (Fig. 1C). There was no significant difference in mean food intake over the course of the study with mice consuming on average 3.14 ± 0.16 g per day per mouse, providing the animals with 4.5 ± 0.3 mg per kg bw per day of SAFF. Using allometric scaling based on body surface area,53 this dose equates to 22 ± 1.2 mg per day human equivalent dose for a person of 60 kg.
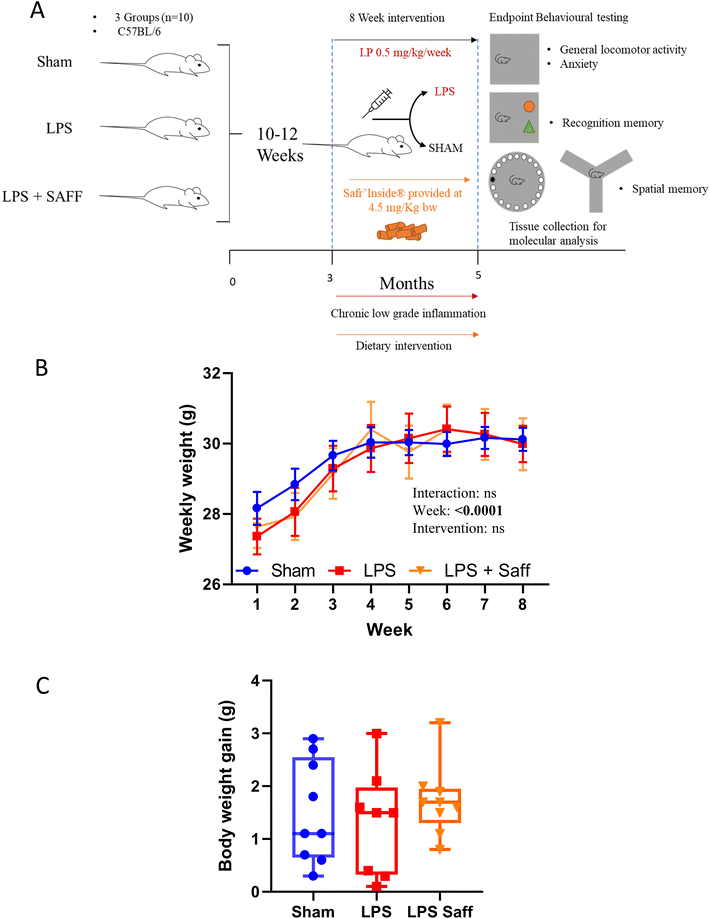 |
| Fig. 1 Administration of LPS (0.5 mg per kg bw per week) and supplementation with SAFF for 8 weeks had minimal impact upon body weight. (A) Schematic of experimental study design. (B) Although body weight increased over the course of the experiment, it was not influenced by the intervention. (n = 10) (C) similarly, overall body weight gain remained constant across all groups and was not altered by LPS treatment nor SAFF supplementation (n = 10). | |
3.2. 8-Week Safr'Inside™ supplementation mitigates anxiety-related behaviour associated with chronic inflammation
Upon completion of the 8-week intervention, a battery of behavioural tests was conducted to assess the impact of LPS and SAFF upon cognitive performance and anxiety-related behaviour. Supplementation with SAFF significantly increased time spent in the centre of the OF maze by 62% when compared to un-supplemented LPS treated animals (p < 0.05; Fig. 2A). Distance travelled within the OF was not altered, suggesting this was not a product of locomotor impairment (Fig. 2B). Representative heatmaps are provided (Fig. 2C). Chronic exposure to LPS for an 8-week period led to significant deficits in recognition memory, which were not restored through SAFF supplementation (Fig. 2D). Neither LPS treatment nor SAFF supplementation significantly impacted spatial memory performance as assessed by the Y maze (Fig. 2E) and Barnes maze (Fig. 2F), although a nominal 10% decrease in Y maze performance was observed in LPS-treated animals (ANOVA p = 0.07).
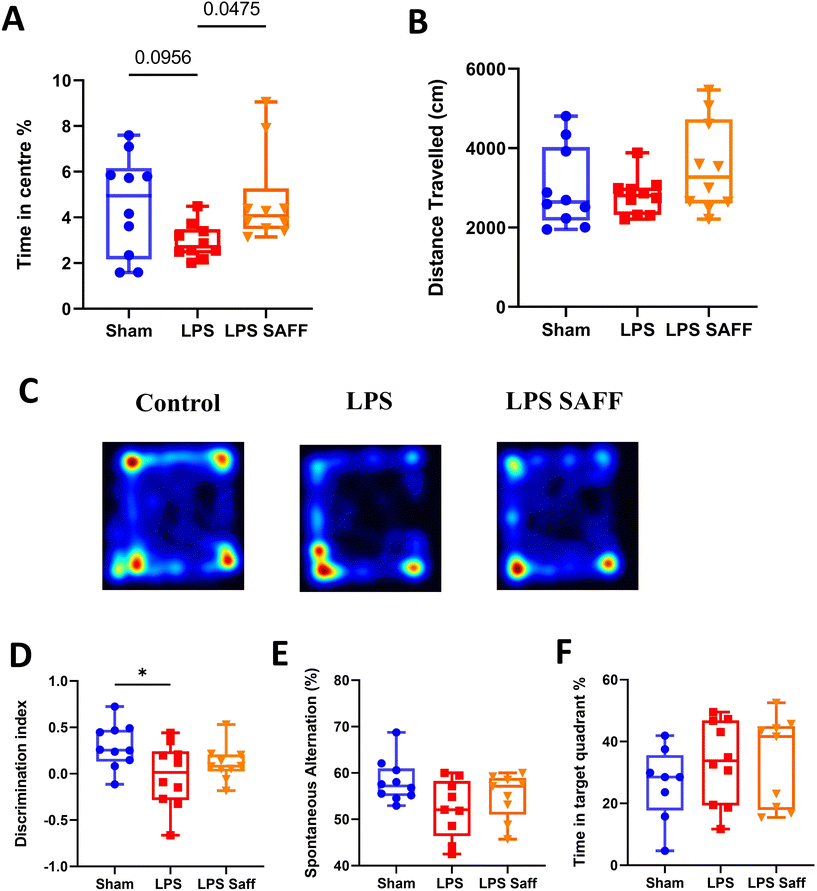 |
| Fig. 2 SAFF improves LPS mediated anxiety-like phenotype. (A) LPS-treated animals spent significantly less time in the centre of the OF compared to their SAFF supplemented counterparts. (n = 10) (B) distance travelled remained unaffected by the interventions. (n = 10) (C) representative heatmaps for the OF task are provided. (D) LPS treatment resulted in diminished recognition memory as assessed by the NOR task however this was not recovered by SAFF treatment. (n = 10) (E and F) spatial memory performance as determined by the Y maze (n ≥ 9) and Barnes maze (n ≥ 8) was not significantly affected by either LPS or SAFF. *P < 0.05. | |
3.3. 8-Week Safr'Inside™ supplementation resulted in a microbiome shift which restored LPS-mediated disturbances
Alpha diversity was measured using Chao1 and Shannon index. Chao1 index, a measure of species richness was significantly increased in response to SAFF supplementation (p < 0.01 Fig. 3A), whilst Shannon index which accounts for both richness and evenness was not significantly altered (Fig. 3B). Furthermore, beta diversity was found to be significantly altered according to Bray–Curtis (p < 0.05 Fig. 3C). Encouraged by the results of the alpha and beta diversity, we explored changes at the phylum, family, and genus levels. At the phylum level SAFF mitigated LPS-induced Bacteriodota and Firmicutes dysregulation (Fig. 3D and E). At the family level Muribaculacaea displayed a similar LPS-mediated reduction in abundance, which was in turn restored through SAFF treatment (Fig. 3F). In addition to the recovery of LPS-mediated gut microbiota dysregulation, SAFF supplementation promoted the abundance of Akkermansiaceae and Christensenellacae (Fig. 3G and H). At the genus level, an increase in Akkermansia (Fig. 3I) and Alloprevotella (Fig. 3J) was observed in the SAFF treatment group. All significant microbial differences present across phylum, family and genus are given in ESI data (Table S2†).
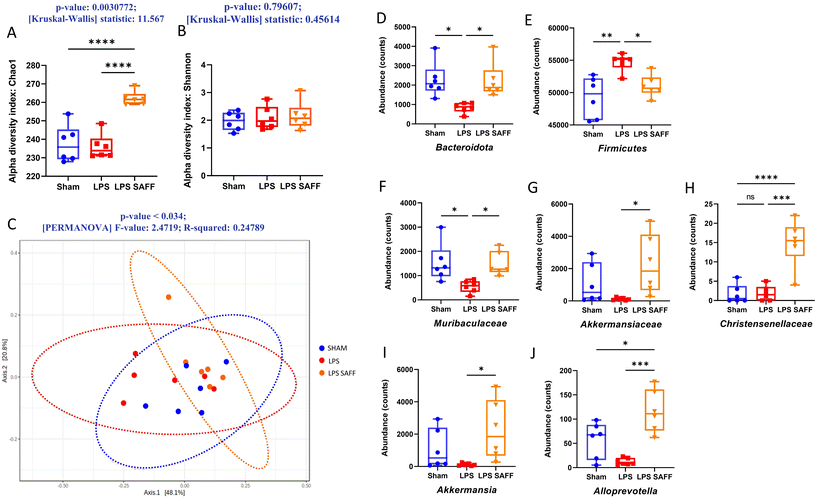 |
| Fig. 3 SAFF supplementation leads to a significant shift in gut microbial profile and ameliorates LPS-associated dysbiosis. (A) Alpha diversity as analysed using chao1 was significantly increased through SAFF treatment (n = 6), (B) but no significant difference was established using Shannon index (n = 6). (C) Beta diversity measured through Bray–Curtis analysis was significantly different across experimental groups (n = 6). (D and E) At the phylum level Bacteroidota and Firmicutes were significantly altered in response to LPS insult but were both restored to control levels via SAFF treatment. (n = 6) (F–H) at the family level recovery of LPS diminished Muribaculacaea was observed in response to SAFF treatment along with SAFF-specific promotion of Akkermansiaceae and Christensenellacae (n = 6). (I and J) Finally at the genus level SAFF specific increase in Akkermansia and Alloprevotella was also apparent (n = 6). *P < 0.05, **P < 0.01, ***P < 0.001, ****P < 0.0001. | |
3.4. Metabolomic profile associated with Safr'Inside™ supplementation reflected gut microbial change
Considering the gut microbial shift mediated through both LPS and SAFF, we extended our line of investigation to encompass the metabolomic profile. PLS-DA plots showed a clear separation of experimental groups indicating a metabolomic shift in response to both LPS and SAFF treatments (Fig. 4A) the extent of which can be seen through the heatmap provided which displays all 12 significantly altered metabolites (Fig. 4B; p < 0.05; FDR < 0.1) See ESI data full for statistical analysis including post-hoc analysis (Table S3†). Having established a shift in both the microbiome and metabolome we performed a Spearman correlation to elucidate potential interactions between the two data sets. This analysis revealed a number of bacterial–metabolite interactions, of particular interest were those interactions relating to the aforementioned Muribaculacaea, Akkermansiaceae, Akkermansia and Alloprevotella which were significantly modulated by SAFF treatment (Fig. 5A). Interestingly, Muribaculacaea (p < 0.01), Muribaculum (p < 0.01) and Akkermansia (p < 0.05) negatively correlated with dimethylamine (DMA) (Fig. 5A), which was in turn negatively correlated with time spent in the centre of the OF test (Fig. 5Bp < 0.01). The other 11 metabolites identified did not significantly correlate with OF performance.
 |
| Fig. 4 LPS treatment and SAFF supplementation lead to altered metabolomic profile. (A) PLS-DA plot showed separation of groups indicative of a metabolomic shift in response to treatment. (n ≥ 5) (B) heatmap depicting the 12 significantly altered metabolites as determined by ANOVA (p < 0.05) (n ≥ 8). *P < 0.05, **P < 0.01, ***P < 0.001, ****P < 0.0001. | |
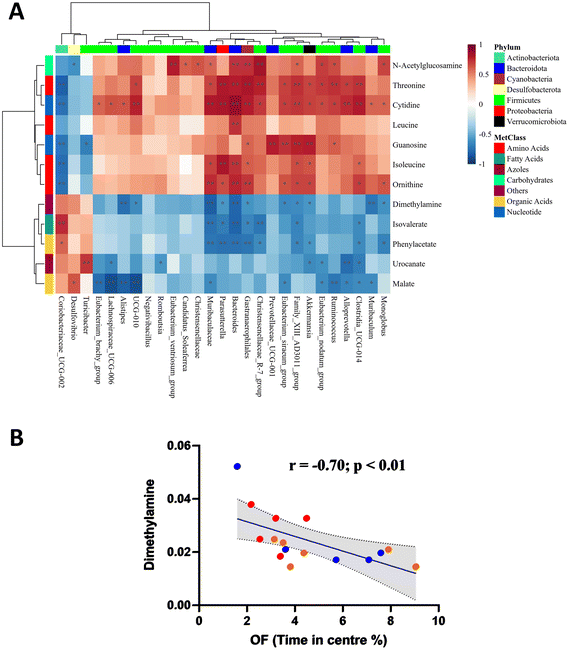 |
| Fig. 5 Gut microbial profile is associated with gut metabolomic profile which may in turn influence anxiety-like behaviour. (A) Interactions between the metabolome and microbiome of LPS SAFF groups expressed in relation to LPS group (n ≥ 5). Interactions were made using Spearman correlation analysis which highlighted a number of key significant microbiome–metabolite interactions (with asterisk) including that of Muribaculacaea and Akkermansia with DMA. (B) Interestingly the metabolite DMA also significantly correlated with OF performance (n ≥ 5). Each spot represents an individual sample (blue: sham; red: LPS; orange: LPS + SAFF). The solid line is a linear trendline which is an indication of the linear (Pearson) correlation between the two variables. (Note: shading shows the 99% confidence interval). *P < 0.05, **P < 0.01. | |
3.5. Brain proteomic analysis revealed protein dysregulation that was associated with DMA
One-way ANOVA of 2DE brain proteomic analysis revealed 17 significantly modulated proteins (Table 1). Of the proteins identified, ketimine reductase mu-crystallin (Fig. 6A) significantly correlated with DMA concentration, along with Heat shock 70 kDa protein 1B, 60S ribosomal protein L29, T-complex protein 1 subunit beta and Septin11 (Fig. S1†). However, only ketimine reductase mu-crystallin subsequently correlated with OF performance (Fig. 6B).
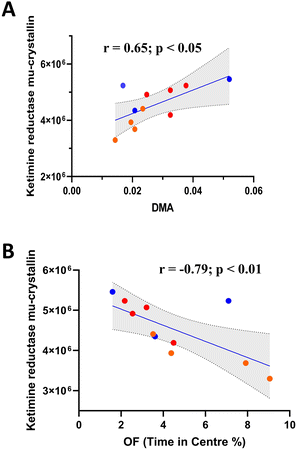 |
| Fig. 6 DMA concentration is associated with a key protein in the brain which was in turn linked to OF performance. (A) DMA is significantly correlated with ketimine reductase mu-crystallin (n ≥ 3), (B) ketimine reductase mu-crystallin is significantly correlated with OF performance (n ≥ 3). Each spot represents an individual sample (blue: sham; red: LPS; orange: LPS + SAFF). The solid line is a linear trendline which is an indication of the linear (Pearson) correlation between the two variables. (Note: shading shows the 99% confidence interval). | |
Table 1 Fold change and p-value of significantly altered proteins in the brain. Proteins that significantly correlate with DMA are highlighted in bold
Protein ID |
Protein name |
Function (key word/s) |
LPS |
LPS SAFF |
Fold change |
ANOVA (p value) |
P05202 |
Aspartate aminotransferase |
Aminotransferase, transferase, lipid transport, transport |
0.74 ± 0.12 |
0.26 ± 0.05 |
3.78 |
0.00423 |
Q60932 |
Voltage-dependent anion-selective channel protein 1 |
Porin, apoptosis, ion transport, transport |
1.08 ± 0.04 |
0.46 ± 0.14 |
2.35 |
0.024124 |
P80314 |
T-complex protein 1 subunit beta
|
Chaperone |
0.63 ± 0.05 |
0.90 ± 0.02 |
1.59 |
0.007097 |
P62196 |
26S proteasome regulatory subunit 8 |
Protein degradation |
1.49 ± 0.09 |
1.14 ± 0.02 |
1.49 |
0.000889 |
P68368 |
Tubulin alpha-4A chain |
Microtubule constituent |
0.73 ± 0.03 |
0.97 ± 0.08 |
1.38 |
0.02232 |
O54983 |
Ketimine reductase mu-crystallin
|
Oxidoreductase, thyroid hormone binding |
0.94 ± 0.04 |
0.74 ± 0.04 |
1.35 |
0.010207 |
Q8C1B7 |
Septin-11
|
Cell cycle, cell division, GABAergic synaptic connectivity |
1.32 ± 0.07 |
1.08 ± 0.04 |
1.32 |
0.016673 |
P63005 |
Platelet-activating factor acetylhydrolase IB |
Cell cycle, cell division, differentiation, lipid degradation, lipid metabolism, mitosis, neurogenesis, transport |
1.29 ± 0.05 |
1.25 ± 0.04 |
1.29 |
0.011588 |
O08553 |
Dihydropyrimidinase-related protein 2 |
Differentiation, neurogenesis |
1.19 ± 0.01 |
1.25 ± 0.06 |
1.25 |
0.010754 |
P17879 |
Heat shock 70 kDa protein 1B
|
Chaperone, stress response |
1.23 ± 0.04 |
1.04 ± 0.03 |
1.23 |
0.026335 |
P97807 |
Fumarate hydratase |
Lyase, DNA damage, DNA repair, tricarboxylic acid cycle |
1.21 ± 0.02 |
1.15 ± 0.04 |
1.21 |
0.003112 |
P47915 |
60S ribosomal protein L29
|
Ribosomal protein |
1.21 ± 0.04 |
1.02 ± 0.04 |
1.21 |
0.019722 |
Q61171 |
Peroxiredoxin-2 |
Antioxidant, oxidoreductase, peroxidase |
0.83 ± 0.05 |
0.87 ± 0.03 |
1.21 |
0.042685 |
Q60930 |
Voltage-dependent anion-selective channel protein 2 |
Porin, ion transport, Transport |
1.07 ± 0.02 |
1.18 ± 0.06 |
1.18 |
0.028904 |
P09411 |
Phosphoglycerate kinase 1 |
Kinase, transferase, glycolysis |
1.08 ± 0.03 |
1.18 ± 0.05 |
1.18 |
0.041683 |
Q04447 |
Creatine kinase B-type |
Kinase, transferase, glycolysis |
0.87 ± 0.03 |
0.85 ± 0.01 |
1.17 |
0.015721 |
Q99JG3 |
Annexin A13 |
Lipid binding |
1.16 ± 0.02 |
1.15 ± 0.04 |
1.16 |
0.006164 |
4. Discussion
Developing a clearer understanding of the underlying mechanisms that cause anxiety-related conditions is an important step in the enhancement and development of novel disease-altering interventions. Gut health has been increasingly implicated in the development of mental health conditions such as anxiety, with modulation of the gut microbiota (via pre/probiotics or transplantation) an increasingly promising prospect.18,54 Here we investigated SAFF supplementation in an LPS model of chronic inflammation. Utilising a cognitive behavioural test battery, 16S rRNA sequencing, 1H NMR metabolomic and 2DE proteomics analyses we were able to explore potential interactions across the gut–brain axis. Our findings highlight a novel association between Muribaculaceae and Akkermanisa abundance and DMA concentration, which were altered by both LPS and SAFF supplementation and subsequently correlated with rodent behaviour on the OF task.
SAFF supplementation mitigated the LPS-mediated reduction in centre exploration time during the OF task. Animals which display increased time in the periphery of the OF maze and decreased time in the centre are considered to be displaying anxiety-like traits. Increased anxiety has been reported in response to LPS challenge,55,56 indeed others have described this same impact upon OF performance,57,58 validating this phenotype in this model of chronic low-grade inflammation. Similarly, saffron's anti-anxiety properties are widely described throughout the literature, with similar OF results reported.59,60 Saffron's ability to improve cognition and/or mitigate neurodegeneration is currently less established, however promising results have been reported.61 In our model of low-grade chronic inflammation in which we observed significantly diminished cognitive performance (NOR and Y maze), we were unable to establish any SAFF-mediated effects. As alluded to, this is in contrast to others. However, these discrepancies may relate to variations in the disease models used as well as the treatment dose, treatment duration and even publication bias.
To ascertain if the gut microbiota may contribute to SAFF's anti-anxiety properties, we evaluated the gut microbial composition in response to both LPS and SAFF. Our results indicated that SAFF supplementation increased species richness but not evenness, suggesting that SAFF may mediate a relatively specific rather than widespread microbial shift. This shift consisted of an increase in the abundance of Muribaculacaea (which was reduced by LPS treatment), Akkermansiaceae, Christensenellacae, Akkermansia and Alloprevotella, all of which are considered important constituents of a healthy microbiota, have been shown to be dysregulated in anxiety-like disorders.62–65 Of these modulated bacteria, Akkermansia is particularly well established in the context of disease, with evidence across both humans and mice that its reduced abundance is a fundamental part of disease processes such as low-grade inflammation.66,67Christensenellacae, on the other hand, is emerging as an important microbial component of human health68 and was specifically increased by SAFF supplementation. As with Akkermansia, Christensenellacae has been linked with metabolic health.68
To explore the role of this microbial shift further we analysed the caecal metabolomic profile. Of the significantly modulated metabolites identified across experimental groups, the SAFF-mediated reduction in DMA concentration was of particular interest given its significant, negative correlation with both Muribaculacaea and Akkermansia as well as OF time in the centre. Interestingly DMA a short-chain aliphatic amine can be formed from dietary choline, as well as TMA and TMAO in a reaction catalysed by enzymes provided by gut bacteria,69 emphasising its inherent relationship with gut microbial composition70 To our knowledge this is the first time a link between Muribaculaceae and DMA has been reported, however a link between Akkermansia and DMA has been previously made in the context of Cardiovascular Risk (in children with chronic kidney disease),71 and sarcopenia.71,72Muribaculaceae have nevertheless been recently reported to play a key role in curcumins (Indian saffron) ability to alleviate anxiety-like behaviours.73 Intriguingly, we are not aware of any study citing DMA as a factor in anxiety, although it has been implicated in depression74,75 neurodegenerative processes,76 neurotoxicity,77 and neuroinflammation.77
Proteomic analysis revealed a number of significantly altered proteins within the brain of treated animals. Of these proteins, there were several that significantly correlated with DMA concentration. Interestingly many of these proteins relate to protein production/conformation/stability (e.g. chaperones) which may be indicative of DMA's impact upon the brain. Interestingly, ketimine reductase mu-crystallin (CRYM/KR) subsequently correlated with OF performance, perhaps revealing a novel gut–brain interactive pathway with implications upon anxiety-like behaviour. Evidence connecting ketimine reductase mu-crystallin (CRYM/KR) to anxiety is scarce, however, it has been shown to be altered in socially isolated rodents.78 Furthermore, CRYM/KR expression has been found to be highly expressed in mice treated with amitriptyline, a tricyclic antidepressant.79 In addition to depression, CRYM/KR has been implicated in schizophrenia, Alzheimer's disease and amyotrophic lateral sclerosis,80 possibly through the generation of reactive oxygen species.81 Interestingly, we recently reported that the circulating human metabolites produced following saffron intake were able to protect human neurons against oxidative-stress-induced neurotoxicity by preserving cell viability in an ex vivo clinical model.27 Although the exact function of CRYM/KR remains to be fully elucidated, it may play important roles in neuroprotection, neurotransmission, cell survival and lysine metabolism through interactions with thyroid hormone T3 or ketimines.82
In addition to the microbiota-gut–brain interaction detailed above, there is a possibility that components of SAFF e.g. safranal cross the BBB and directly impact the brain. Indeed, preclinical and in vitro studies highlight the modulatory actions of safranal upon inflammatory/stress response signalling pathways within the brain.83 However, to our knowledge there is no adequately conducted experiment to date addressing safranal brain bioavailability, highlighting a critical research gap that should be the focus of future experimentation. Amongst the other bioactive constituents of saffron, it has been shown that crocetin crosses the blood–brain barrier when saffron extract is administered intraperitoneally.84 Evidence similarly suggests that crocins also cross the blood–brain barrier and reach the central nervous system according to a study in mice.85 Moreover, it seems that saffron can modulate the blood brain barrier integrity. Indeed, in vitro results showed that Crocus sativus extract increases the tightness of a cell-based blood–brain barrier model. These observations were confirmed in vivo, in an Alzheimer disease model where mice received a saffron extract enriched diet.86
To conclude, we forward a potentially novel gut–brain axis mediated interaction in the development/prevention of anxiety-related behaviours. The results presented here indicate that LPS and SAFF lead to both a reduction and increase in Muribaculaceae respectively, whilst SAFF boosted Akkermanisa abundance. This increase in Muribaculaceae and Akkermansia correlated with DMA concentration which in turn potentially altered brain proteomic profile and OF performance. Thus, highlighting the potential of SAFF, and the importance of the microbiome in the mitigation of anxiety-related disorders.
Author contributions
D.V. conceptualised and designed the experiments and analytical approaches; D.V. provided the Home Office Animal License; M.G.P. performed the animal experiments, the cognitive testing, the microbiome analysis and analysed the data; E.C. and G.L.G performed the metabolomic analyses. L.G, L.Z. and M.R. performed the brain proteomic analyses; L.P and D.G. provided the saffron extract. M.G.P. and D.V. wrote the manuscript with contributions from all the co-authors; M.M. and C.A. critically revised the manuscript. All authors have read and agreed to the published version of the manuscript.
Availability of data and materials
The 16S rRNA gene sequence data have been deposited in the NCBI BioProject database (https://www.ncbi.nlm.nih.gov/bioproject/) under accession number PRJNA858994. Other original data will be made available upon request.
Conflicts of interest
DV received funding from Activ'Inside. L.P, D.G. work for Activ'Inside and provided the saffron extract. Activ'Inside was not involved in the design, implementation, analysis, and interpretation of the data. All the other authors have no conflict of interest to declare.
Acknowledgements
This project was funded by Activ'Inside (grant R209836) who received support for the Silver Brain Food project, a program co-financed by the “Future Investment Program” (Programme d'Investissements d'Avenir PIA3) and managed by the Investment General Secretariat and operated by Bpifrance.
References
-
S. McManus, H. Meltzer, T. Brugha, P. E. Bebbington and R. Jenkins, Adult psychiatric morbidity in England: results of a household survey, Health and Social Care Information Centre, 2009 Search PubMed.
- J. W. Tiller, Depression and anxiety, Med. J. Aust., 2013, 199, S28–S31 CrossRef PubMed.
- Depression, Other common mental disorders: global health estimates World Health Organization, Geneva, 2017, 24 Search PubMed.
- The Lancet Global, Mental health matters, Lancet Glob Health, 2020, 8, e1352 CrossRef.
- J. Bueno-Notivol, P. Gracia-García, B. Olaya, I. Lasheras, R. López-Antón and J. Santabárbara, Prevalence of depression during the COVID-19 outbreak: A meta-analysis of community-based studies, Int. J. Clin. Health Psychol., 2021, 21, 100196 CrossRef PubMed.
- S. Pappa, V. Ntella, T. Giannakas, V. G. Giannakoulis, E. Papoutsi and P. Katsaounou, Prevalence of depression, anxiety, and insomnia among healthcare workers during the COVID-19 pandemic: A systematic review and meta-analysis, Brain, Behav., Immun., 2020, 88, 901–907 CrossRef CAS PubMed.
- J. W. Murrough, S. Yaqubi, S. Sayed and D. S. Charney, Emerging drugs for the treatment of anxiety, Expert Opin. Emerging Drugs, 2015, 20, 393–406 CrossRef CAS PubMed.
- C. Chen and W. Shan, Pharmacological and non-pharmacological treatments for major depressive disorder in adults: A systematic review and network meta-analysis, Psychiatry Res., 2019, 281, 112595 CrossRef CAS PubMed.
- E. Carl, S. M. Witcraft, B. Y. Kauffman, E. M. Gillespie, E. S. Becker, P. Cuijpers, M. Van Ameringen, J. A. J. Smits and M. B. Powers, Psychological and pharmacological treatments for generalized anxiety disorder (GAD): a meta-analysis of randomized controlled trials, Cogn. Behav. Ther., 2019, 49, 1–21 Search PubMed.
- J. K. Carpenter, L. A. Andrews, S. M. Witcraft, M. B. Powers, J. A. J. Smits and S. G. Hofmann, Cognitive behavioral therapy for anxiety and related disorders: A meta-analysis of randomized placebo-controlled trials, Depression Anxiety, 2018, 35, 502–514 CrossRef CAS PubMed.
- P. Cuijpers, E. Karyotaki, E. Weitz, G. Andersson, S. D. Hollon and A. van Straten, The effects of psychotherapies for major depression in adults on remission, recovery and improvement: a meta-analysis, J. Affective Disord., 2014, 159, 118–126 CrossRef PubMed.
- R. Businaro, D. Vauzour, J. Sarris, G. Münch, E. Gyengesi, L. Brogelli and P. Zuzarte, Therapeutic Opportunities for Food Supplements in Neurodegenerative Disease and Depression, Front. Nutr., 2021, 8, 669846 CrossRef PubMed.
- J. Firth, J. E. Gangwisch, A. Borsini, R. E. Wootton and E. A. Mayer, Food and mood: how do diet and nutrition affect mental wellbeing?, Br. Med. J., 2020, 29(369), m2382 CrossRef PubMed.
- C. Lassale, G. D. Batty, A. Baghdadli, F. Jacka, A. Sánchez-Villegas, M. Kivimäki and T. Akbaraly, Healthy dietary indices and risk of depressive outcomes: a systematic review and meta-analysis of observational studies, Mol. Psychiatry, 2019, 24, 965–986 CrossRef PubMed.
- O. Sadeghi, A. H. Keshteli, H. Afshar, A. Esmaillzadeh and P. Adibi, Adherence to Mediterranean dietary pattern is inversely associated with depression, anxiety and psychological distress, Nutr. Neurosci., 2021, 24, 248–259 CrossRef PubMed.
- J. Godos, W. Currenti, D. Angelino, P. Mena, S. Castellano, F. Caraci, F. Galvano, D. Del Rio, R. Ferri and G. Grosso, Diet and Mental Health: Review of the Recent Updates on Molecular Mechanisms, Antioxidants, 2020, 9(4), 346 CrossRef CAS PubMed.
- L. Lin and J. Zhang, Role of intestinal microbiota and metabolites on gut homeostasis and human diseases, BMC Immunol., 2017, 18(1), 2 CrossRef PubMed.
- A. Chakrabarti, L. Geurts, L. Hoyles, P. Iozzo, A. D. Kraneveld, G. La Fata, M. Miani, E. Patterson, B. Pot, C. Shortt and D. Vauzour, The microbiota–gut–brain axis: pathways to better brain health. Perspectives on what we know, what we need to investigate and how to put knowledge into practice, Cell. Mol. Life Sci., 2022, 79(2), 80 CrossRef CAS PubMed.
- K. V. Sandhu, E. Sherwin, H. Schellekens, C. Stanton, T. G. Dinan and J. F. Cryan, Feeding the microbiota-gut-brain axis: diet, microbiome, and neuropsychiatry, Transl. Res., 2017, 179, 223–244 CrossRef CAS PubMed.
- M. G. Pontifex, M. M. A. H. Malik, E. Connell, M. Müller and D. Vauzour, Citrus Polyphenols in Brain Health and Disease: Current Perspectives, Front. Neurosci., 2021, 15, 640648 CrossRef PubMed.
- T. Abu-Izneid, A. Rauf, A. A. Khalil, A. Olatunde, A. Khalid, F. A. Alhumaydhi, A. S. M. Aljohani, Md. Sahab Uddin, M. Heydari, M. Khayrullin, M. A. Shariati, A. O. Aremu, A. Alafnan and K. R. R. Rengasamy, Nutritional and health beneficial properties of saffron (Crocus sativus L): a comprehensive review, Crit. Rev. Food Sci. Nutr., 2020, 62, 2683–2706 CrossRef PubMed.
- C. Monchaux De Oliveira, V. De Smedt-Peyrusse, J. Morael, S. Vancassel, L. Capuron, D. Gaudout, L. Pourtau and N. Castanon, Prevention of Stress-Induced Depressive-like Behavior by Saffron Extract Is Associated with Modulation of Kynurenine Pathway and Monoamine Neurotransmission, Pharmaceutics, 2021, 13(12), 2155 CrossRef CAS PubMed.
- C. Monchaux De Oliveira, L. Pourtau, S. Vancassel, C. Pouchieu, L. Capuron, D. Gaudout and N. Castanon, Saffron Extract-Induced Improvement of Depressive-Like Behavior in Mice Is Associated with Modulation of Monoaminergic Neurotransmission, Nutrients, 2021, 13(3), 904 CrossRef PubMed.
- R. Roustazade, M. Radahmadi and Y. Yazdani, Therapeutic effects of saffron extract on different memory types, anxiety, and hippocampal BDNF and TNF-α gene expressions in sub-chronically stressed rats, Nutr. Neurosci., 2021, 25, 192–206 CrossRef PubMed.
- M. Shafiee, S. Arekhi, A. Omranzadeh and A. Sahebkar, Saffron in the treatment of depression, anxiety and other mental disorders: Current evidence and potential mechanisms of action, J. Affective Disord., 2018, 227, 330–337 CrossRef CAS PubMed.
- P. A. Jackson, J. Forster, J. Khan, C. Pouchieu, S. Dubreuil, D. Gaudout, B. Moras, L. Pourtau, F. Joffre, C. Vaysse, K. Bertrand, H. Abrous, D. Vauzour, J. Brossaud, J. B. Corcuff, L. Capuron and D. O. Kennedy, Effects of Saffron Extract Supplementation on Mood, Well-Being, and Response to a Psychosocial Stressor in Healthy Adults: A Randomized, Double-Blind, Parallel Group, Clinical Trial, Front. Nutr., 2020, 7, 606124 CrossRef PubMed.
- F. Wauquier, L. Boutin-Wittrant, L. Pourtau, D. Gaudout, B. Moras, A. Vignault, C. Monchaux De Oliveira, J. Gabaston, C. Vaysse, K. Bertrand, H. Abrous, L. Capuron, N. Castanon, D. Vauzour, V. Roux, N. Macian, G. Pickering and Y. Wittrant, Circulating Human Serum Metabolites Derived from the Intake of a Saffron Extract (Safr’Inside(TM)) Protect Neurons from Oxidative Stress: Consideration for Depressive Disorders, Nutrients, 2022, 14(7), 1511 CrossRef CAS PubMed.
- O. Asbaghi, M. Sadeghian, O. Sadeghi, S. Rigi, S. C. Tan, A. Shokri and S. M. Mousavi, Effects of saffron (Crocus sativus L.) supplementation on inflammatory biomarkers: A systematic review and meta–analysis, Phytother. Res., 2020, 35, 20–32 CrossRef PubMed.
- J. W. Anderson, P. Baird, R. H. Davis Jr., S. Ferreri, M. Knudtson, A. Koraym, V. Waters and C. L. Williams, Health benefits of dietary fiber, Nutr. Rev., 2009, 67, 188–205 CrossRef PubMed.
- S. Banskota, H. Brim, Y. H. Kwon, G. Singh, S. R. Sinha, H. Wang, W. I. Khan and H. Ashktorab, Saffron Pre-Treatment Promotes Reduction in Tissue Inflammatory Profiles and Alters Microbiome Composition in Experimental Colitis Mice, Molecules, 2021, 26(11), 3351 CrossRef CAS PubMed.
- I. Boutron, N. Percie du Sert, V. Hurst, A. Ahluwalia, S. Alam, M. T. Avey, M. Baker, W. J. Browne, A. Clark, I. C. Cuthill, U. Dirnagl, M. Emerson, P. Garner, S. T. Holgate, D. W. Howells, N. A. Karp, S. E. Lazic, K. Lidster, C. J. MacCallum, M. Macleod, E. J. Pearl, O. H. Petersen, F. Rawle, P. Reynolds, K. Rooney, E. S. Sena, S. D. Silberberg, T. Steckler and H. Würbel, The ARRIVE guidelines 2.0: Updated guidelines for reporting animal research, PLoS Biol., 2020, 18(7), e3000410 CrossRef PubMed.
- D. Laukens, B. M. Brinkman, J. Raes, M. De Vos and P. Vandenabeele, Heterogeneity of the gut microbiome in mice: guidelines for optimizing experimental design, FEMS Microbiol. Rev., 2016, 40, 117–132 CrossRef CAS PubMed.
- B. Moras, L. Loffredo and S. Rey, Quality assessment of saffron (Crocus sativus L.) extracts via UHPLC-DAD-MS analysis and detection of adulteration using gardenia fruit extract (Gardenia jasminoides Ellis), Food Chem., 2018, 257, 325–332 CrossRef CAS PubMed.
- L. Hoyles, M. G. Pontifex, I. Rodriguez-Ramiro, M. A. Anis-Alavi, K. S. Jelane, T. Snelling, E. Solito, S. Fonseca, A. L. Carvalho, S. R. Carding, M. Müller, R. C. Glen, D. Vauzour and S. McArthur, Regulation of blood–brain barrier integrity by microbiome-associated methylamines and cognition by trimethylamine N-oxide, Microbiome, 2021, 9(1), 235 CrossRef CAS PubMed.
- L. H. Pinto and C. Enroth-Cugell, Tests of the mouse visual system, Mamm. Genome, 2000, 11, 531–536 CrossRef CAS PubMed.
- L. B. Tucker and J. T. McCabe, Measuring Anxiety-Like Behaviors in Rodent Models of Traumatic Brain Injury, Front. Behav. Neurosci., 2021, 15, 682935 CrossRef CAS PubMed.
- F. Yu, Z. Wang, F. Tchantchou, C. T. Chiu, Y. Zhang and D. M. Chuang, Lithium ameliorates neurodegeneration, suppresses neuroinflammation, and improves behavioral performance in a mouse model of traumatic brain injury, J. Neurotrauma, 2012, 29, 362–374 CrossRef PubMed.
- K. E. Davis, M. J. Eacott, A. Easton and J. Gigg, Episodic-like memory is sensitive to both Alzheimer's-like pathological accumulation and normal ageing processes in mice, Behav. Brain Res., 2013, 254, 73–82 CrossRef PubMed.
- M. Leger, A. Quiedeville, V. Bouet, B. Haelewyn, M. Boulouard, P. Schumann-Bard and T. Freret, Object recognition test in mice, Nat. Protoc., 2013, 8, 2531–2537 CrossRef CAS PubMed.
- J. K. Denninger, B. M. Smith and E. D. Kirby, Novel Object Recognition and Object Location Behavioral Testing in Mice on a Budget, J. Visualized Exp., 2018, 141 Search PubMed.
- M. G. Pontifex, A. Martinsen, R. N. M. Saleh, G. Harden, N. Tejera, M. Müller, C. Fox, D. Vauzour and A.-M. Minihane, APOE4 genotype exacerbates the impact of menopause on cognition and synaptic plasticity in APOE-TR mice, FASEB J., 2021, 35, e21583 CrossRef CAS PubMed.
- A. Attar, T. Liu, W.-T. C. Chan, J. Hayes, M. Nejad, K. Lei and G. Bitan, A Shortened Barnes Maze Protocol Reveals Memory Deficits at 4-Months of Age in the Triple-Transgenic Mouse Model of Alzheimer's Disease, PLoS One, 2013, 8, e80355 CrossRef PubMed.
- Q. Wang, G. M. Garrity, J. M. Tiedje and J. R. Cole, Naive Bayesian classifier for rapid assignment of rRNA sequences into the new bacterial taxonomy, Appl. Environ. Microbiol., 2007, 73, 5261–5267 CrossRef CAS PubMed.
- C. Quast, E. Pruesse, P. Yilmaz, J. Gerken, T. Schweer, P. Yarza, J. Peplies and F. O. Glöckner, The SILVA ribosomal RNA gene database project: improved data processing and web-based tools, Nucleic Acids Res., 2012, 41, D590–D596 CrossRef PubMed.
- T. T. T. Tran, S. Corsini, L. Kellingray, C. Hegarty, G. Le Gall, A. Narbad, M. Müller, N. Tejera, P. W. O'Toole, A. M. Minihane and D. Vauzour, APOE genotype influences the gut microbiome structure and function in humans and mice: relevance for Alzheimer's disease pathophysiology, FASEB J., 2019, 33, 8221–8231 CrossRef CAS PubMed.
- J. Wu, Y. An, J. Yao, Y. Wang and H. Tang, An optimised sample preparation method for NMR-based faecal metabonomic analysis, Analyst, 2010, 135(5), 1023 RSC.
- M. C. Barbalace, L. Zallocco, D. Beghelli, M. Ronci, S. Scortichini, M. Digiacomo, M. Macchia, M. R. Mazzoni, D. Fiorini, A. Lucacchini, S. Hrelia, L. Giusti and C. Angeloni, Antioxidant and Neuroprotective Activity of Extra Virgin Olive Oil Extracts Obtained from Quercetano Cultivar Trees Grown in Different Areas of the Tuscany Region (Italy), Antioxidants, 2021, 10(3), 421 CrossRef CAS PubMed.
- L. Giusti, C. Angeloni, M. Barbalace, S. Lacerenza, F. Ciregia, M. Ronci, A. Urbani, C. Manera, M. Digiacomo, M. Macchia, M. Mazzoni, A. Lucacchini and S. Hrelia, A Proteomic Approach to Uncover Neuroprotective Mechanisms of Oleocanthal against Oxidative Stress, Int. J. Mol. Sci., 2018, 19(8), 2329 CrossRef PubMed.
- Z. Pang, J. Chong, G. Zhou, D. A. de Lima Morais, L. Chang, M. Barrette, C. Gauthier, P.-É. Jacques, S. Li and J. Xia, MetaboAnalyst 5.0: narrowing the gap between raw spectra and functional insights, Nucleic Acids Res., 2021, 49, W388–W396 CrossRef CAS PubMed.
- Y. Ni, G. Yu, H. Chen, Y. Deng, P. M. Wells, C. J. Steves, F. Ju and J. Fu, M2IA: a web server for microbiome and metabolome integrative analysis, Bioinformatics, 2020, 36, 3493–3498 CrossRef CAS PubMed.
- A. Dhariwal, J. Chong, S. Habib, I. L. King, L. B. Agellon and J. Xia, MicrobiomeAnalyst: a web-based tool for comprehensive statistical, visual and meta-analysis of microbiome data, Nucleic Acids Res., 2017, 45, W180–w188 CrossRef CAS PubMed.
- Y. You, D. Liang, R. Wei, M. Li, Y. Li, J. Wang, X. Wang, X. Zheng, W. Jia and T. Chen, Evaluation of metabolite-microbe correlation detection methods, Anal. Biochem., 2019, 567, 106–111 CrossRef CAS PubMed.
- A. Nair and S. Jacob, A simple practice guide for dose conversion between animals and human, J. Basic Clin. Pharm., 2016, 7(2), 27 CrossRef PubMed.
- E. Gambaro, C. Gramaglia, G. Baldon, E. Chirico, M. Martelli, A. Renolfi and P. Zeppegno, “Gut–brain axis”: Review of the role of the probiotics in anxiety and depressive disorders, Brain Behav., 2020, 10(10), e01803 Search PubMed.
- K. Unno, D. Furushima, Y. Tanaka, T. Tominaga, H. Nakamura, H. Yamada, K. Taguchi, T. Goda and Y. Nakamura, Improvement of Depressed Mood with Green Tea Intake, Nutrients, 2022, 14(14), 2949 CrossRef CAS PubMed.
- M. G. da Silva, G. C. Daros, F. P. Santos, M. Yonamine and R. M. de Bitencourt, Antidepressant and anxiolytic-like effects of ayahuasca in rats subjected to LPS-induced neuroinflammation, Behav. Brain Res., 2022, 114007, DOI:10.1016/j.bbr.2022.114007.
- Y. Yu, Y. Li, K. Qi, W. Xu and Y. Wei, Rosmarinic acid relieves LPS-induced sickness and depressive-like behaviors in mice by activating the BDNF/Nrf2 signaling and autophagy pathway, Behav. Brain Res., 2022, 433, 114006 CrossRef CAS PubMed.
- S. Moradi Vastegani, S. Hajipour, A. Sarkaki, Z. Basir, S. Parisa Navabi, Y. Farbood and S. E. Khoshnam, Curcumin mitigates lipopolysaccharide-induced anxiety/depression-like behaviors, blood-brain barrier dysfunction and brain edema by decreasing cerebral oxidative stress in male rats, Neurosci. Lett., 2022, 782, 136697 CrossRef CAS PubMed.
- R. Roustazade, M. Radahmadi and Y. Yazdani, Therapeutic effects of saffron extract on different memory types, anxiety, and hippocampal BDNF and TNF-α gene expressions in sub-chronically stressed rats, Nutr. Neurosci., 2022, 25, 192–206 CrossRef CAS PubMed.
- Y. Wang, S. Zhou, X. Song, S. Ding, B. Wang, J. Wen and C. Chen, Study on Antidepressant Effect and Mechanism of Crocin Mediated by the mTOR Signaling Pathway, Neurochem. Res., 2022, 1–11, DOI:10.1007/s11064-022-03668-z.
- J. E. Lewis, J. Poles, D. P. Shaw, E. Karhu, S. A. Khan, A. E. Lyons, S. B. Sacco and H. R. McDaniel, The effects of twenty-one nutrients and phytonutrients on cognitive function: A narrative review, J. Clin. Transl. Res., 2021, 7, 575–620 CAS.
- Z. Zhang, C. Yao, M. Li, L. C. Wang, W. Huang and Q. J. Chen, Prophylactic effects of hyperforin on anhedonia-like phenotype in chronic restrain stress model: A role of gut microbiota, Lett. Appl. Microbiol., 2022, 75(5), 1103 CrossRef CAS PubMed.
- J.-K. Kim, S.-K. Han, M.-K. Joo and D.-H. Kim, Buspirone alleviates anxiety, depression, and colitis; and modulates gut microbiota in mice, Sci. Rep., 2021, 11, 6094 CrossRef PubMed.
- Z. Dong, X. Shen, Y. Hao, J. Li, H. Li, H. Xu, L. Yin and W. Kuang, Gut Microbiome: A Potential Indicator for Differential Diagnosis of Major Depressive Disorder and General Anxiety Disorder, Front. Psychiatry, 2021, 12, 651536 CrossRef PubMed.
- Y. Song, B. Shan, S. Zeng, J. Zhang, C. Jin, Z. Liao, T. Wang, Q. Zeng, H. He, F. Wei, Z. Ai and D. Su, Raw and wine processed Schisandra chinensis attenuate anxiety like behavior via modulating gut microbiota and lipid metabolism pathway, J. Ethnopharmacol., 2021, 266, 113426 CrossRef CAS PubMed.
- P. D. Cani, C. Depommier, M. Derrien, A. Everard and W. M. de Vos, Akkermansia muciniphila: paradigm for next-generation beneficial microorganisms, Nat. Rev. Gastroenterol. Hepatol., 2022, 19(10), 625 CrossRef PubMed.
- P. D. Cani and W. M. de Vos, Next-Generation Beneficial Microbes: The Case of Akkermansia muciniphila, Front. Microbiol., 2017, 8, 1765 CrossRef PubMed.
- J. L. Waters and R. E. Ley, The human gut bacteria Christensenellaceae are widespread, heritable, and associated with health, BMC Biol., 2019, 17, 83 CrossRef PubMed.
- Y. Gao, J. Zhang, H. Chen, Z. Wang, J. Hou and L. Wang, Dimethylamine enhances platelet hyperactivity in chronic kidney disease model, J. Bioenerg. Biomembr., 2021, 53, 585–595 CrossRef CAS PubMed.
- S. H. Zeisel, J. S. Wishnok and J. K. Blusztajn, Formation of methylamines from ingested choline and lecithin, J. Pharmacol. Exp. Ther., 1983, 225, 320–324 CAS.
- C. N. Hsu, G. P. Chang-Chien, S. Lin, C. Y. Hou, P. C. Lu and Y. L. Tain, Association of Trimethylamine, Trimethylamine N-oxide, and Dimethylamine with Cardiovascular Risk in Children with Chronic Kidney Disease, J. Clin. Med., 2020, 9(2), 336 CrossRef CAS PubMed.
- F. R. Ponziani, A. Picca, E. Marzetti, R. Calvani, G. Conta, F. Del Chierico, G. Capuani, M. Faccia, F. Fianchi, B. Funaro, H. Josè Coelho-Junior, V. Petito, E. Rinninella, F. Paroni Sterbini, S. Reddel, P. Vernocchi, M. Cristina Mele, A. Miccheli, L. Putignani, M. Sanguinetti, M. Pompili and A. Gasbarrini, Characterization of the gut-liver-muscle axis in cirrhotic patients with sarcopenia, Liver Int., 2021, 41, 1320–1334 CrossRef CAS PubMed.
- F. Zhang, Y. Zhou, H. Chen, H. Jiang, F. Zhou, B. Lv and M. Xu, Curcumin Alleviates DSS-Induced Anxiety-Like Behaviors via the Microbial-Brain-Gut Axis, Oxid. Med. Cell. Longevity, 2022, 2022, 1–19 Search PubMed.
- J. S. Tian, G. J. Peng, X. X. Gao, Y. Z. Zhou, J. Xing, X. M. Qin and G. H. Du, Dynamic analysis of the endogenous metabolites in depressed patients treated with TCM formula Xiaoyaosan using urinary (1)H NMR-based metabolomics, J. Ethnopharmacol., 2014, 158(Pt A), 1–10 CrossRef PubMed.
- J. S. Tian, B. Y. Shi, H. Xiang, S. Gao, X. M. Qin and G. H. Du, 1H-NMR-based metabonomic studies on the anti-depressant effect of genipin in the chronic unpredictable mild stress rat model, PLoS One, 2013, 8, e75721 CrossRef CAS PubMed.
- M. G. Fleszar, J. Wiśniewski, M. Zboch, D. Diakowska, A. Gamian and M. Krzystek-Korpacka, Targeted metabolomic analysis of nitric oxide/L-arginine pathway metabolites in dementia: association with pathology, severity, and structural brain changes, Sci. Rep., 2019, 9, 13764 CrossRef PubMed.
- L. A. Buckley, K. T. Morgan, J. A. Swenberg, R. A. James, T. E. Hamm, Jr. and C. S. Barrow, The toxicity of dimethylamine in F-344 rats and B6C3F1 mice following a 1-year inhalation exposure, Fundam. Appl. Toxicol., 1985, 5, 341–352 CrossRef CAS PubMed.
- D. Filipović, I. Perić, V. Costina, A. Stanisavljević, P. Gass and P. Findeisen, Social isolation stress-resilient rats reveal energy shift from glycolysis to oxidative phosphorylation in hippocampal nonsynaptic mitochondria, Life Sci., 2020, 254, 117790 CrossRef PubMed.
- C. Boehm, D. Newrzella, S. Herberger, N. Schramm, G. Eisenhardt, V. Schenk, V. Sonntag-Buck and O. Sorgenfrei, Effects of antidepressant treatment on gene expression profile in mouse brain: cell type-specific transcription profiling using laser microdissection and microarray analysis, J. Neurochem., 2006, 97, 44–49 CrossRef CAS PubMed.
- A. Hallen, J. F. Jamie and A. J. L. Cooper, Lysine metabolism in mammalian brain: an update on the importance of recent discoveries, Amino Acids, 2013, 45, 1249–1272 CrossRef CAS PubMed.
- J.-E. Choi, J.-J. Lee, W. Kang, H. J. Kim, J.-H. Cho, P.-L. Han and K.-J. Lee, Proteomic Analysis of Hippocampus in a Mouse Model of Depression Reveals Neuroprotective Function of Ubiquitin C-terminal Hydrolase L1 (UCH-L1) via Stress-induced Cysteine Oxidative Modifications, Mol. Cell. Proteomics, 2018, 17, 1803–1823 CrossRef CAS PubMed.
- R. Hommyo, S. O. Suzuki, N. Abolhassani, H. Hamasaki, M. Shijo, N. Maeda, H. Honda, Y. Nakabeppu and T. Iwaki, Expression of CRYM in different rat organs during development and its decreased expression in degenerating pyramidal tracts in amyotrophic lateral sclerosis, Neuropathology, 2018, 38, 247–259 CrossRef CAS PubMed.
- S. Nanda and K. Madan, The role of Safranal and saffron stigma extracts in oxidative stress, diseases and photoaging: A systematic review, Heliyon, 2021, 7(2), e06117 CrossRef CAS PubMed.
- Z. I. Linardaki, M. G. Orkoula, A. G. Kokkosis, F. N. Lamari and M. Margarity, Investigation of the neuroprotective action of saffron (Crocus sativus L.) in aluminum-exposed adult mice through behavioral and neurobiochemical assessment, Food Chem. Toxicol., 2013, 52, 163–170 CrossRef CAS PubMed.
- E. Karkoula, N. Lemonakis, N. Kokras, C. Dalla, E. Gikas, A. L. Skaltsounis and A. Tsarbopoulos, Trans-crocin 4 is not hydrolyzed to crocetin following i.p. administration in mice, while it shows penetration through the blood brain barrier, Fitoterapia, 2018, 129, 62–72 CrossRef CAS PubMed.
- Y. S. Batarseh, S. S. Bharate, V. Kumar, A. Kumar, R. A. Vishwakarma, S. B. Bharate and A. Kaddoumi, Crocus sativus Extract Tightens the Blood-Brain Barrier, Reduces Amyloid beta Load and Related Toxicity in 5XFAD Mice, ACS Chem. Neurosci., 2017, 8, 1756–1766 CrossRef CAS PubMed.
|
This journal is © The Royal Society of Chemistry 2022 |
Click here to see how this site uses Cookies. View our privacy policy here.