DOI:
10.1039/D1NA00529D
(Paper)
Nanoscale Adv., 2022,
4, 163-172
Self-assembly of ultra-small-sized carbon nanoparticles in lipid membrane disrupts its integrity
Received
1st July 2021
, Accepted 12th October 2021
First published on 13th October 2021
Abstract
Although nanomaterials are widely studied in biomedical applications, the major concern of nanotoxicity still exists. Therefore, numerous studies have been conducted on the interactions of various biomolecules with various types of nanomaterials, including carbon nanotubes, graphene, fullerene etc. However, the size effect of nanomaterials is poorly documented, especially ultra-small particles. Here, the interactions of the smallest carbon nanoparticle (NP), C28, with the cell membrane were studied using molecular dynamics (MD) simulations. The results show that similar to fullerene C60, the C28 NPs can self-assemble into stable clusters in water. Inside the membrane, the C28 NPs are more prone to aggregate to form clusters than C60 NPs. The reason for C28 aggregation is characterized by the potential of mean force (PMF) and can be explained by the polarized nature of C28 NPs while the acyl chains of lipids are nonpolar. At the C28 cluster regions, the thickness of the membrane is significantly reduced by the C28 aggregation. Accordingly, the membrane loses its structural integrity, and translocation of water molecules through it was observed. Thus, these results predict a stronger cytotoxicity to cells than C60 NPs. The present findings might shed light on the understanding of the cytotoxicity of NPs with different sizes and would be helpful for the potential biomedical applications of carbon NPs, especially as antibacterial agents.
1. Introduction
With the rapid development of nanotechnology, nanomaterials have attracted enormous attention in various biomedical applications, such as gene detection and gene delivery,1,2 tumor imaging and therapy,3–5 biomarkers and probes,6–8 due to their unique mechanical, optical, and electrical properties.9–11 Prior to biomedical applications, the major concern of nano-toxicity has attracted extensive attention. Large numbers of theoretical and experimental studies reported the interactions between nanomaterials and biomolecules to understand the mechanistic origins of the bio-toxicity of the nanomaterials. For example, recent studies on the adsorption of proteins onto nanomaterials have shown that both structures and functions of proteins can be severely affected by nanomaterials such as carbon nanotubes and graphene.12–23 It is reported that carbon nanotubes can either bind to the receptor protein, thus inhibiting the binding of the native ligand, or directly disrupt the active site of the protein.12–16 In addition, carbon nanotubes can also affect the dynamic properties of proteins, such as binding to the messenger protein CaM, leading to failure in signaling.17 Recently, the Zhou group reported that defective graphene can denature protein with a higher speed than ideal graphene.18,19 There are also many studies on the biosafety of fullerenes and their derivatives. For example, C60, the prototype fullerene, is effective in blocking the ion channels of proteins in the membrane.20 In addition, the Zhao group demonstrated that the metallofullerenol Gd@C82(OH)22 can inhibit the functions of WW/SH3 domains by blocking the ligands binding to the active sites.21–24 These structural and functional changes of biomolecules can lead to cell death.
As the boundary of the cell to the outside environment, the plasma membrane plays an essential role in controlling the cellular substance exchange. Thus, the interactions of the cell membrane with nanomaterials are possibly the primary origins of nano-toxicity, and thus have been studied extensively. For instance, Zhou et al. have demonstrated by experimental and theoretical approaches that graphene nanosheets can penetrate into cell membranes and extract phospholipids from them, causing cell death through disrupting the membrane.25 Very recently, phosphorene has been shown to have similar molecular mechanisms to destruct cell membranes.26 Due to the nanoscale size of nanomaterials, their interactions with biomolecules are very sensitive to the shape, size and even elemental components of nanomaterials. Besides the interactions of 2D nanomaterials with cell membranes, NPs with different shapes interacting with cell membranes have also been studied. Fullerene C60 is shaped like a football with a small diameter of 0.7 nm which makes it have unique interactions with the cell membrane interior which is also hydrophobic. Because of the hydrophobic nature, C60 can easily penetrate into the membrane.27,28 At high concentrations, C60 can form clusters in the membrane and cause deformation of the membrane structure.28,29 Besides C60, numerous carbon particles have been reported with different diameters. However, the effects of the size of carbon NPs, especially ultra-sized NPs, which match the size of biomolecules (amino acids, nucleotides, phospholipid headgroups), on membranes have not been documented.
In this work, using C28, the experimentally reported smallest NP, as the representative NP, we investigated the binding and distributions of C28 NPs in lipid bilayer membranes using molecular dynamics (MD) simulations. Our results reveal that, both in water and membranes, C28 NPs can spontaneously assemble into stable clusters. At high concentrations, the C28 NPs can aggregate to form clusters inside the membrane. This can cause severe distortion to the membrane structure and cause water translocation through the membrane. This reveals that this ultra-small NP can cause significant strong toxicity which might be even stronger than that of C60. This work helps to better understand the size-effect of NPs on their cytotoxicity and could guide the biomedical application studies of nanostructures.
2. Methods
2.1 Models and simulation setup
The C28 NP has a diameter of 0.47 nm, which is the smallest experimentally synthesized endohedral metallofullerene.30 The C28 NP consists of a C28 cage and a uranium atom, which is used to stabilize the strongly reactive C28 cage.31–33 With the uranium atom, the C28 cage has net atomic charges. This is different from the C60 NP which bears no charge and thus is purely hydrophobic.34 However, while the structures and properties of the C28 NP have been well-studied by theoretical and experimental approaches, the interaction process of C28 with biomolecules, like lipid membranes, is yet to be reported. The smallest size of the C28 particle will bring some diverse results when it interacts with the membrane. Quantum chemical calculations for U@C28 were performed using the Gaussian 09 program.35 The geometry of U@C28 was optimized at the PBE/SDD/6-31G* level.36 The standard Gaussian-type basis sets 6-31G* (ref. 37) were used for C atoms. The Stuttgart/Dresden relativistic effective core potentials (SDD)38 and their corresponding valence basis sets39 were applied for the U atom. Single-point energy was calculated for the optimized geometry at the B3LYP/SDD/6-31G* level40,41 to obtain the ESP of the ground state electron density. Subsequently, the atomic charges fit to the ESP at points selected according to the CHelpG scheme42 were calculated by using Multiwfn program.43 These atomic charges were then used for the following classical MD simulations.
The membrane model was constructed using the CHARMM-GUI membrane builder,44 which is composed of 128 POPC lipids with 64 lipids on each leaflet. The membrane was firstly equilibrated for 10 ns at 300 K and 1 bar pressure, and was used as the initial structure for C28 simulation.
Four different systems were used to explore the interactions of different numbers of C28 NPs with the membrane: 128 POPC with one C28 particle (POPC-1C28), five C28 (POPC-5C28), ten C28 (POPC-10C28) and twenty C28 (POPC-20C28). Initially, the C28 NPs were placed with the minimum distance to the lipid membrane surface being larger than 1.0 nm. The complex was then solvated in a water box (6.5 × 6.5 × 10 nm3) under periodic boundary conditions. The TIP3P water model was used.45 The total numbers of atoms are 31
756, 31
749, 31
732 and 31
734 for the four different systems (POPC-1C28, POPC-5C28, POPC-10C28, and POPC-20C28) respectively. In addition to C28-membrane models, control simulations of pure POPC lipids and POPC lipids with twenty C60 NPs were also performed for comparison (POPC-20C60). In addition, a simulation of twenty C28 NPs in a water box was also performed to investigate the self-assembly behavior of C28 NPs.
All the MD simulations were performed with the GROMACS package46 and the lipids were modeled using the GROMOS53A6 force field.47 In the simulations, the covalent bonds involving the H atoms were constrained by the LINCS algorithm.48 The structural configurations were saved every 1 ps for subsequent analysis. The van der Waals (vdW) interactions were calculated with a cutoff distance of 1.0 nm, while the long-range electrostatic interactions were treated with the particle-mesh Ewald (PME) method.49,50 After energy minimization, the complex systems were equilibrated for 2 ns under the NPT ensemble to achieve 300 K and 1 bar. Then, 2 μs simulation was performed for each system under the NPT ensemble for data collection. The semi-isotropic Berendsen barostat51 and the v-rescale thermostat52 were used for pressure coupling and temperature coupling, respectively. Visualizations and analysis were performed using the VMD software package.53
2.2 Umbrella sampling MD simulation
The association free energies between two C28 NPs in water and POPC membrane are described as the potential of mean force (PMF) using the umbrella sampling technique. The distance between the center of mass (COM) of two C28 particles was restrained at certain distance d0 with a harmonic force
where k is the force constant, 3000 kJ mol−1 nm−2. The distance d0 (including x, y and z) was sampled from 0.60 to 1.50 nm with a step of 0.1 nm. At each distance, 10 ns productive simulation has been generated. The final PMF curve was obtained using the weighted histogram analysis method (WHAM).54
The PMF of a single C28 transferring across the lipid monolayer was also calculated with the umbrella sampling technique. The distance between the COM of C28 particle and the COM of the POPC lipids along the normal direction of the membrane was used as a variable. A total of 40 windows were used with a step of 0.1 nm and a force constant of 2000 kJ mol−1 nm−2. For each window, 10 ns simulation has been generated.
3. Results and discussion
3.1 The aggregation of C28 NPs in water
We firstly simulated the aggregations of twenty C28 particles in water as the preliminary case. As shown in Fig. 1(a)–(c), the C28 NPs were initially well separated and then quickly aggregated to form clusters in three independent simulations. To quantify the self-assembly behavior of C28 NPs, we calculated the number of clusters and monitored the largest cluster size of C28 NPs in POPC-20C28 as a function of the simulation time. Here, the C28 NPs were treated to form a cluster if the minimum distance between the COM of two C28 NPs is within 0.98 nm. Here, the value of 0.98 nm corresponds to the first maximum of the PMF for a C28 dimer to dissociate in water which is presented later. In addition, an isolated C28 particle is treated as the smallest cluster. As shown in Fig. 1(d) and (e), at t = 0 ns, the number of clusters and the largest cluster size were respectively 20 and 1 because the NPs were well separated. From 0 ns to 33 ns, the number of clusters gradually reduces and the largest cluster size shows a stepwise increase. After 33 ns, there is no significant change in both the number of clusters and the largest cluster size. At the end of the simulation, the biggest cluster consisting of 12, 9 and 13 C28 NPs was formed in three trajectories respectively. This phenomenon is similar to the self-assembly behavior of C60 NPs in water because of the generally hydrophobic nature of the two types of NPs. To describe the aggregation behavior of C28 NPs in water, we calculated the PMF of two C28 NPs as a function of their COM–COM distance. As shown in Fig. 2(c), the minimum at a distance of 0.78 nm corresponds to the direct contact pair (DCP) of two C28 NPs. The dissociation energy barrier is about 11 kJ mol−1, thus C28 NPs tend to aggregate in water because the DCP state is the global minimum. In addition, we also calculated the PMF of two C28 NPs as a function of their COM–COM distance in the membrane. As shown in Fig. 2(c), at a small distance of less than 0.9 nm, the PMF profiles of two C28 NPs in water and POPC are almost the same. The reason is that two C28 NPs form direct contacts because there is no water or lipid molecules located between them as shown in the 2D density contour maps in Fig. 2(c). Thus the interactions are less affected by the environments. At a larger distance, there are water/lipids between the two C28 which can regulate the interacting energy profile, thus the PMF profiles are different at a large distance.
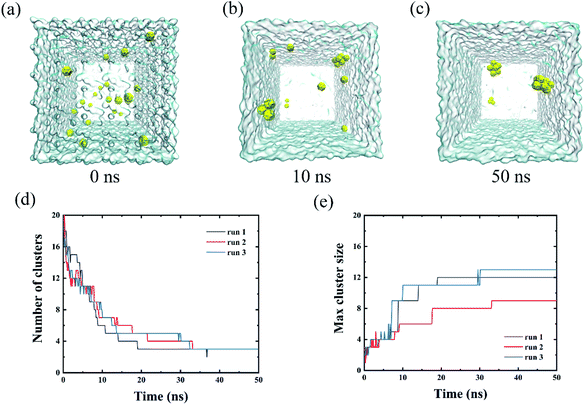 |
| Fig. 1 (a)–(c) Three representative structures at different simulated time points of 20 NPs in water. (d) The number of aggregated clusters and (e) the largest cluster size as a function of simulation time. | |
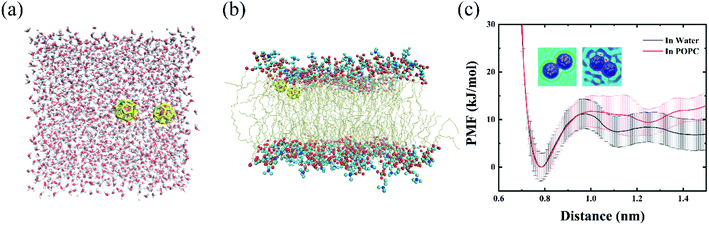 |
| Fig. 2 Snapshots of two C28 NPs in water (a) and membrane (b) used in umbrella sampling simulations. For clarity, the water molecules are not shown in (b). (c) The free energy profiles of two C28 NPs with respect to their COM–COM distance in water and POPC membrane. The direct contacting state (DCS) at 0.78 nm is considered to have a free energy of zero. In (c), the two dimensional density contour maps of the water (left) and lipids (right) of C28 in a cross-section at the COM–COM distance of 0.90 nm are shown. | |
3.2 The intrusion process of C28 NPs into the membrane
To better understand the intrusion process of the C28 particle into the membrane, we firstly simulated one C28 particle interacting with the membrane. The location of C28 (measured from COM) with respect to the membrane is calculated to reflect the C28 position and shown in Fig. 3(a). At the beginning of the simulation, the C28 particle and membrane were well separated, and the C28 particle stayed in the bulk water with a distance 3.0 nm away from the center of the membrane. Then the C28 particle quickly loaded to the surface of membrane at about t = 50 ns, and the COM distance quickly decreases to ∼2.0 nm. Then, the curve declines sharply because the C28 particle intruded into the membrane. We noticed that the C28 prefers to stay at the position which is ∼1.0 nm away from the center of the membrane although random translocations between the two leaflets of the membrane were detected. In detail, three translocation cases happened in 2000 ns simulation. This tendency has also been observed in previous atomistic simulations where C60 particles stayed 1.0 nm away from the membrane center. The dotted line in the middle in Fig. 3(a) is the center of the membrane. In Fig. 3(b), the location of the density peaks of the PCN (the phosphate and part of the choline) can be regarded as the upper and lower boundaries of the membrane which are represented by the two dotted lines in Fig. 3(a).
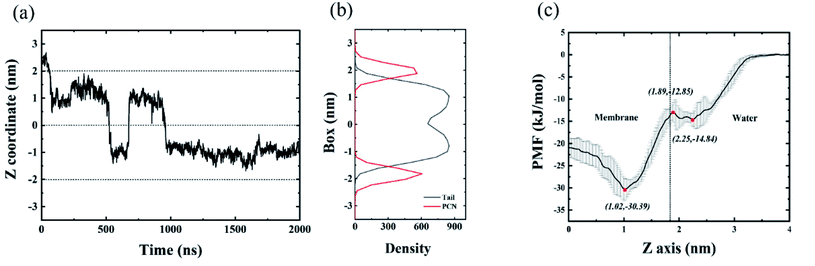 |
| Fig. 3 (a) The z coordinate of the C28 particle in the membrane as a function of the simulation time, the three dashed lines indicate the borderlines of the membrane to water and the center of the membrane which is shown in (b). Density profiles for PCN (the phosphate and part of the choline (CH2CH2N)) and the fatty acid tails of the lipid as a function of the distance from the membrane center (z = 0). (c) PMF of a C28 particle from water into the center of the bilayer (z = 0). Error bar is also shown. | |
To better characterize the binding affinity between the C28 and the membrane, we calculated the PMF of the C28 particle during insertion into the membrane. As shown in Fig. 3(c), the PMF is a two-step decrease from bulk water to the membrane interior. The first step is from the water to the membrane boundary (from 4 nm to 2.25 nm) where the energy well is about −14.84 kJ mol−1. Then the C28 particle overcomes the energy barrier of approximately 2 kJ mol−1 to pass through the membrane surface. This phenomenon has been reported for various types of nanoparticles interacting with the membrane. For instance, the Gao Huajian group reported that the energy profiles of NPs with various types of shapes uniformly show a barrier at the water–bilayer interface.55,56 This is due to the fact that the head groups of lipids are highly charged resulting in high density and high viscosity. The second step is from the inner side of the hydrophobic region to the position 1 nm away from the center of the membrane with an energy decrease of −17.54 kJ mol−1. When the C28 particle further moves to the membrane center (from 1.02 nm to 0 nm), it will be hindered by a ∼9 kJ mol−1 energy barrier. Therefore, the C28 particle prefers to locate at the position which is 1.0 nm away from the center of the membrane. To understand the origin of this PMF barrier, Marrink et al. simulated the insertion of C60 into the membrane and found that the stable position of C60 is about 0.7 to 1.3 nm away from the center of the membrane.57 This is similar to our present study of C28 although the specific position differs. They proposed that the friction coefficient increases upon increasing the penetrant size, making it difficult for the penetrant to pass through this region. This is well consistent with unbiased MD results in Fig. 3(a).
In order to investigate whether a similar phenomenon occurs when a large number of C28 intrudes into the membrane, up to 2000 ns simulations were generated for the three systems (POPC-5C28, POPC-10C28, POPC-20C28). Fig. 4 summarizes three representative structures during 2000 ns simulations for the POPC-5C28 (Fig. 4(a)–(c)), POPC-10C28 (Fig. 4(d)–(f)) and POPC-20C28 (Fig. 4(g)–(i)). At t = 0 ns, the C28 NPs uniformly stayed in the bulk water and away from the membrane. Then the C28 NPs quickly loaded the surface of the lipid membrane within a few nanoseconds. Thereafter, the C28 NPs began to intrude into the lipid membrane. Once they penetrated into the membrane interior, the C28 NPs stayed in the membrane and outward translocation back to the bulk water was never observed.
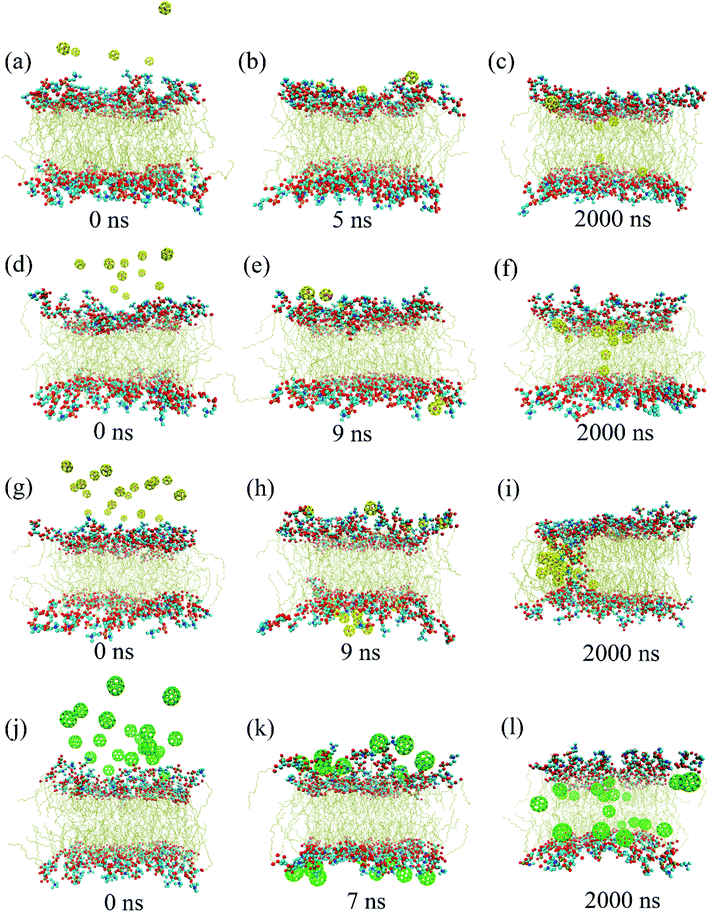 |
| Fig. 4 Three representative structures at different simulation times in the POPC-5C28 (a)–(c), POPC-10C28 (d)–(f), POPC-20C28 (g)–(i) and POPC-10C60 (j)–(l) systems. Oxygen (red), nitrogen (blue), carbon (cyan), and phosphorus (orange) atoms in head groups are shown as spheres, while the phospholipids are represented as a tan line. The C28 and C60 NPs are shown in yellow and green color respectively. For clarity, the water molecules are not shown. | |
The three systems reveal different distributions of C28 in the membrane. For a small C28 ratio of POPC-5C28 and POPC-10C28, C28 NPs are generally dispersed in the membrane. In contrast, at a high C28 ratio, the distribution of C28 in the membrane is different. As shown in Fig. 4(g)–(i), the C28 NPs aggregated and formed a large cluster after penetrating into the membrane interior. This phenomenon is different from the two simulations with low C28 concentration in Fig. 4(a)–(f), which is also in sharp contrast to the C60 NPs in the membrane, where the aggregation degree of C60 NPs was significantly lower (Fig. 4(l)). The aggregation behavior of C28 can be explained by the polarized nature of C28 NPs while the acyl chains of lipids are nonpolar. Thus, inside the membrane, C60 NPs (totally nonpolar) are better dispersed than C28 (partially polar). More importantly, the C28 cluster caused severe structure disruption to the membrane as shown in Fig. 4(i) where the polar “heads” of lipids were found in the interior of the membrane.
In order to describe the distributions of different number of C28 NPs inside the membrane, we calculated the density profiles of various components of the model including water, C28 and lipid tail across the bilayer normal direction. Here, the density profiles were calculated from the last 200 ns of each simulation. As shown in Fig. 5(a)–(c), at low C28 ratio, POPC-1C28, POPC-5C28 and POPC-10C28, the density peaks of C28 are mainly distributed at the position which is about 1.0 nm away from the membrane center while at the center of the membrane the existence of C28 is rare. In contrast, for the POPC-20C28 system, a clear peak of C28 appears at the center of the membrane, indicating strong aggregation of 20C28 inside the membrane.
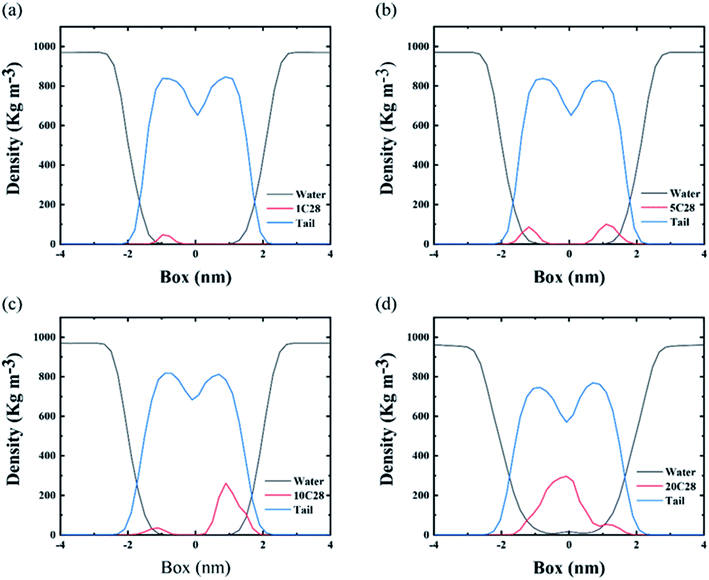 |
| Fig. 5 Density profiles for various parts as a function of the distance from the membrane center (z = 0) in the POPC-1C28 (a), POPC-5C28 (b), POPC-10C28 (c) and POPC-20C28 (d) systems. All the data are averaged over the last 200 ns simulation. | |
To quantitatively assess the tendency of C28 aggregation in the membrane, we compared the PMF of two C28 NPs as a function of their COM–COM distance in water and the membrane. As shown in Fig. 2(c), the dissociation energy barrier in the membrane is about 12 kJ mol−1. At a distance of 1.5 nm (fully separated state), the free-energy in POPC is approximately 13 kJ mol−1, which is larger than 6.8 kJ mol−1 in water. The results indicate that C28 NPs have a biased tendency to aggregate into a large cluster in the membrane and the cluster is very stable. After overcoming the dissociation energy barrier, the PMF curve is relatively smooth, indicating that the aggregations of C28 occur only at high concentrations.
3.3 The effect of C28 and C60 NPs on POPC membrane structure integrity
Many nanomaterials, such as C60, graphene, phosphorene, and phosphorene carbide, can damage the structural integrity of membranes.25–27,58 The C28 NPs also can lead to the deformation of the membrane, because of the aggregation of C28 in the lipid bilayer. In order to investigate the effect of C28 on the lipid membrane, we firstly calculated the deuterium order parameters SCD of lipid bilayers in the four simulated systems. It is generally known that SCD can well describe the average orientation of the deuterium bond vector with respect to the bilayer normal direction. Here, the SCD parameters for two carbon chains, sn-1 and sn-2, were calculated according to the following equation59
where θ is the angle between the bilayer normal and the vector from Ci to its deuterium atom, and angular brackets represent an ensemble average.
The deformation induced by C28 NPs on the membrane depends on its concentration. At low concentrations of C28 (POPC-1C28, POPC-5C28, POPC-10C28), C28 NPs are dispersed in the membrane, so we calculated the deuterium order parameter for the whole membrane over the last 200 ns of each simulation. As shown in Fig. 6(a) and (b), compared to the pure membrane, only slight changes of SCD curves were observed upon the C28 insertion in the POPC-1C28, POPC-5C28 and POPC-10C28 systems. Thus, at low concentrations, C28 NPs caused negligible changes to the membrane. This is because of both the ultra-size of C28 and the disaggregation inside the membrane. In contrast, for the POPC-20C28 system, the C28 NPs aggregated into a large cluster, so we calculated the SCD of lipids with respect to distances of lipids from the C28 cluster. As shown in Fig. 6(c) and (d), when the lipids are close to the C28 clusters, there was a significant change in SCD, indicating significant distortion of the membrane. However, the effect of the C28 cluster on lipids gradually decreases with the increase of distance. At a distance of 1.5 nm, the SCD curve is very close to the normal value. This indicates that the effect of C28 NPs on the membrane structure is local.
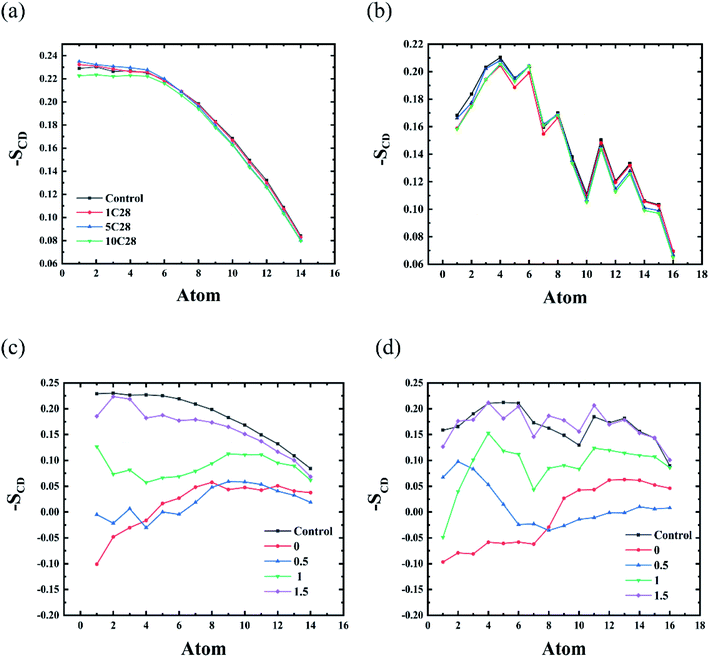 |
| Fig. 6 The deuterium order parameters SCD of the whole membrane calculated for the sn-1 (a) and sn-2 (b) fatty acyl chains for the pure membrane, POPC-1C28, POPC-5C28 and POPC-10C28 systems. For POPC-20C28, the deuterium order parameters SCD of lipids at different distances from the cluster were calculated for the sn-1 (c) and sn-2 (d) fatty acyl chains. | |
The change of membrane structure of POPC-20C28 can be directly assessed by the membrane thickness which was calculated by the software GridMAT-MD.60 As shown in Fig. 7(a), the thickness of the membrane is significantly decreased near the C28 cluster, which is due to the large number of C28 NPs invading the membrane and attracting the head groups of lipids toward the center of the membrane. As shown in Fig. 7(b), this usually results in translocations of water through the membrane which can cause cell death during the experiment.25 However, distant from the C28 cluster, the thickness of the membrane gradually recovered to the normal value (3.85 nm). This is in line with SCD results in Fig. 6(c) and (d) that the C28 cluster has a significant but localized effect on the lipid structure. In contrast, for twenty C60 in the membrane, the change of membrane thickness is much less than that of C28 NPs with the same concentration as can be seen in Fig. 7(c). Moreover, there are almost no water molecules in the membrane which is in sharp contrast to the case of C28. Thus, at high concentrations, the C28 NPs have a larger effect on the structural integrity of membrane lipids than C60 NPs. This might point to a stronger cytotoxicity for the cell which surely deserves further experimental validation.
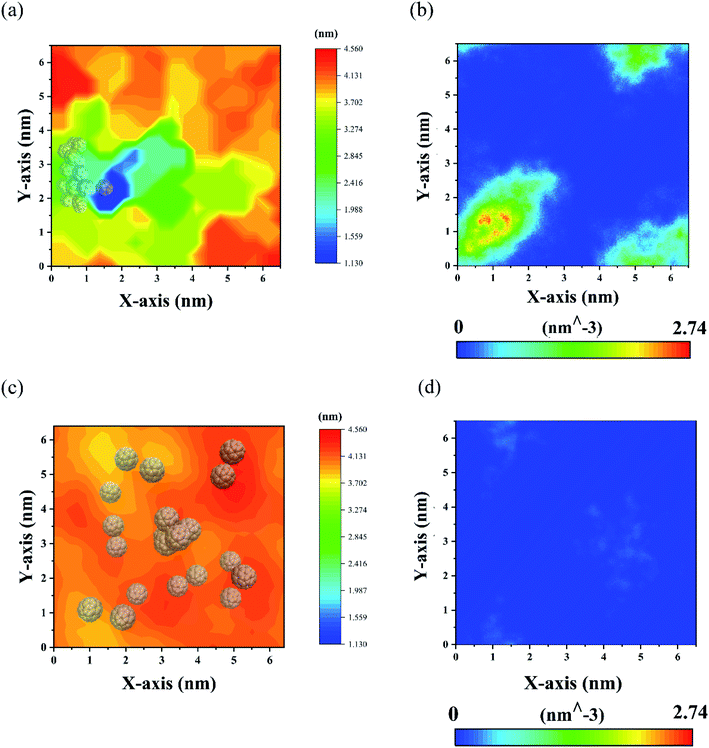 |
| Fig. 7 2D thickness contour of the membrane of (a) POPC-20C28 and (c) POPC-20C60, (b) and (d) depict the relative water density in the membrane. The locations of the C28 and C60 NPs are also shown in (a) and (c). | |
4. Conclusion
In summary, we investigated the interactions of the sub-nanometer C28 particles in a lipid membrane using molecular dynamics (MD) simulations. The results show that similar to C60, the C28 NPs can self-assemble into stable clusters in water. In the membrane, the C28 NPs are more prone to aggregate into big clusters than C60 NPs. Thus, C28 clusters have a larger effect on the structural integrity of membrane lipids than C60 NPs and the thickness of the membrane is significantly reduced by the C28 clusters, causing water translocation. The reason for this phenomenon is characterized by the potential of mean force (PMF) and can be explained by the polarization nature of C28 NPs while the acyl chains of lipids are nonpolar. By comparing the structural changes of the membrane with the existence of C28 or C60, a possibly stronger cytotoxicity of C28 is proposed which deserves future experimental validation. This finding is of crucial importance in terms of the biosafety of ultra-small carbon NPs.
Conflicts of interest
There are no conficts to declare.
Acknowledgements
This work is supported by the Natural Science Foundation of Shandong Province (ZR2020MB074, ZR2020JQ04), and National Natural Science Foundation of China (11874238).
References
- H. Bao, Y. Pan, Y. Ping, N. G. Sahoo, T. Wu, L. Li, J. Li and L. H. Gan, Small, 2011, 7, 1569–1578 CrossRef CAS PubMed.
- Y. Wang, X. Bai, W. Wen, X. Zhang and S. Wang, ACS Appl. Mater. Interfaces, 2015, 7, 18872–18879 CrossRef CAS.
- H. Sharma and S. Mondal, Int. J. Mol. Sci., 2020, 21, 6280 CrossRef CAS.
- Z. Liu, S. Li, X. Xia, Z. Zhu, L. Chen and Z. Chen, Small Methods, 2020, 4, 1900440 CrossRef CAS.
- Y. Cheng, R. A. Morshed, B. Auffinger, A. L. Tobias and M. S. Lesniak, Adv. Drug Delivery Rev., 2014, 66, 42–57 CrossRef CAS PubMed.
- H. Asadi and R. P. Ramasamy, J. Electrochem. Soc., 2020, 167, 167523 CrossRef CAS.
- A. B. Chinen, C. M. Guan, J. R. Ferrer, S. N. Barnaby, T. J. Merkel and C. A. Mirkin, Chem. Rev., 2015, 115, 10530–10574 CrossRef CAS PubMed.
- F. Gong, L. Cheng and Z. Liu, Laser and Optoelectronics Progress, 2020, 57, 35–54 Search PubMed.
- L. Z. Feng and Z. A. Liu, Nanomedicine, 2011, 6, 317–324 CrossRef CAS.
- V. C. Sanchez, A. Jachak, R. H. Hurt and A. B. Kane, Chem. Res. Toxicol., 2012, 25, 15–34 Search PubMed.
- A. K. Geim, Science, 2009, 324, 1530–1534 CrossRef CAS.
- V. E. Kagan, N. V. Konduru, W. Feng, B. L. Allen, J. Conroy, Y. Volkov, I. I. Vlasova, N. A. Belikova, N. Yanamala, A. Kapralov, Y. Y. Tyurina, J. Shi, E. R. Kisin, A. R. Murray, J. Franks, D. Stolz, P. Gou, J. Klein-Seetharaman, B. Fadeel, A. Star and A. A. Shvedova, Nat. Nanotechnol., 2010, 5, 354–359 CrossRef CAS PubMed.
- X. Zhao and R. Liu, Environ. Int., 2012, 40, 244–255 CrossRef CAS.
- G. Zuo, Q. Huang, G. Wei, R. Zhou and H. Fang, ACS Nano, 2010, 4, 7508–7514 CrossRef CAS PubMed.
- G. Zuo, X. Zhou, Q. Huang, H. Fang and R. Zhou, J. Phys. Chem. C, 2011, 115, 23323–23328 CrossRef CAS.
- G. Zuo, S. G. Kang, P. Xiu, Y. Zhao and R. Zhou, Small, 2013, 9, 1546–1556 CrossRef CAS.
- J. Gao, L. Wang, S. G. Kang, L. Zhao, M. Ji, C. Chen, Y. Zhao, R. Zhou and J. Li, Nanoscale, 2014, 6, 12828–12837 RSC.
- B. Li, D. R. Bell, Z. Gu, W. Li and R. Zhou, Carbon, 2019, 146, 257–264 CrossRef CAS.
- Z. Gu, W. Song, S. H. Chen, B. Li, W. Li and R. Zhou, Nanoscale, 2019, 11, 19362–19369 RSC.
- M. Calvaresi, S. Furini, C. Domene, A. Bottoni and F. Zerbetto, ACS Nano, 2015, 9, 4827–4834 CrossRef CAS.
- S. Kraszewski, M. Tarek, W. Treptow and C. Ramseyer, ACS Nano, 2010, 4, 4158–4164 CrossRef CAS.
- M. Calvaresi, S. Furini, C. Domene, A. Bottoni and F. Zerbetto, ACS Nano, 2015, 9, 4827–4834 CrossRef CAS.
- S. G. Kang, T. Huynh and R. Zhou, Nanoscale, 2013, 5, 2703–2712 RSC.
- S. G. Kang, T. Huynh and R. Zhou, Sci. Rep., 2012, 2, 957 CrossRef PubMed.
- Y. Tu, M. Lv, P. Xiu, T. Huynh, M. Zhang, M. Castelli, Z. Liu, Q. Huang, C. Fan, H. Fang and R. Zhou, Nat. Nanotechnol., 2013, 8, 594–601 CrossRef CAS.
- W. Zhang, Y. Chen, T. Huynh, Y. Yang, X. Yang and R. Zhou, Nanoscale, 2020, 12, 2810–2819 RSC.
- S. Zhang, Y. Mu, J. Zhang and W. Xu, PLoS One, 2013, 8, e77436 CrossRef CAS.
- K. A. Russ, P. Elvati, T. L. Parsonage, A. Dews, J. A. Jarvis, M. Ray, B. Schneider, P. J. Smith, P. T. Williamson, A. Violi and M. A. Philbert, Nanoscale, 2016, 8, 4134–4144 RSC.
- M. E. Bozdaganyan, P. S. Orekhov, A. K. Shaytan and K. V. Shaitan, PLoS One, 2014, 9, e102487 CrossRef PubMed.
- P. W. Dunk, N. K. Kaiser, M. Mulet-Gas, A. Rodriguez-Fortea, J. M. Poblet, H. Shinohara, C. L. Hendrickson, A. G. Marshall and H. W. Kroto, J. Am. Chem. Soc., 2012, 134, 9380–9389 CrossRef CAS.
- D. Xu, W. Xie, Y. Gao, W. Jiang and Z. Wang, Chem. Phys. Lett., 2018, 712, 20–24 CrossRef CAS.
- A. Miralrio and L. E. Sansores, Comput. Theor. Chem., 2016, 1083, 53–63 CrossRef CAS.
- A. Munoz-Castro and R. Bruce King, J. Comput. Chem., 2017, 38, 44–50 CrossRef CAS.
- Y. N. Makurin, A. A. Sofronov, A. I. Gusev and A. L. Ivanovsky, Chem. Phys., 2001, 270, 293–308 CrossRef CAS.
-
M. Frisch, G. Trucks, H. Schlegel, G. Scuseria, M. Robb, J. Cheeseman, G. Scalmani, V. Barone, B. Mennucci and G. Petersson, Gaussian 09, 2009 Search PubMed.
- J. P. Perdew, K. Burke and M. Ernzerhof, Phys. Rev. Lett., 1996, 77, 3865 CrossRef CAS.
- W. Hehre, R. Ditchfield and J. Pople, J. Chem. Phys., 1972, 56, 2257 CrossRef CAS.
- W. Küchle, M. Dolg, H. Stoll and H. Preuss, J. Chem. Phys., 1994, 100, 7535–7542 CrossRef.
- X. Cao and M. Dolg, J. Mol. Struct.: THEOCHEM, 2004, 673, 203–209 CrossRef CAS.
- A. D. Becke, J. Chem. Phys., 1993, 98, 5648–5652 CrossRef CAS.
- C. Lee, W. Yang and R. G. Parr, Phys. Rev. B: Condens. Matter Mater. Phys., 1988, 37, 785 CrossRef CAS.
- C. M. Breneman and K. B. Wiberg, J. Comput. Chem., 1990, 11, 361–373 CrossRef CAS.
- T. Lu and F. Chen, J. Comput. Chem., 2012, 33, 580–592 CrossRef CAS.
- B. R. Brooks, R. E. Bruccoleri, B. D. Olafson, D. J. States, S. Swaminathan and M. Karplus, J. Comput. Chem., 1982, 4, 187–217 CrossRef.
- W. L. Jorgensen, J. Chandrasekhar, J. D. Madura, R. W. Impey and M. L. Klein, J. Chem. Phys., 1983, 79, 926–935 CrossRef CAS.
- M. J. Abraham, T. Murtola, R. Schulz, S. Páll, J. C. Smith, B. Hess and E. Lindahl, SoftwareX, 2015, 1–2, 19–25 CrossRef.
- C. Oostenbrink, A. Villa, A. E. Mark and W. F. van Gunsteren, J. Comput. Chem., 2004, 25, 1656–1676 CrossRef CAS PubMed.
- B. Hess, H. Bekker, H. J. C. Berendsen and J. G. E. M. Fraaije, J. Comput. Chem., 1997, 18, 1463–1472 CrossRef CAS.
- T. Darden, D. York and L. Pedersen, J. Chem. Phys., 1993, 98, 10089–10092 CrossRef CAS.
- U. Essmann, L. Perera, M. L. Berkowitz, T. Darden, H. Lee and L. G. Pedersen, J. Chem. Phys., 1995, 103, 8577–8593 CrossRef CAS.
- H. J. C. Berendsen, J. P. M. Postma, W. F. van Gunsteren, A. DiNola and J. R. Haak, J. Chem. Phys., 1984, 81, 3684–3690 CrossRef CAS.
- G. Bussi, D. Donadio and M. Parrinello, J. Chem. Phys., 2007, 126, 014101 CrossRef PubMed.
- W. Humphrey, A. Dalke and K. Schulten, J. Mol. Graphics, 1996, 14, 33–38 CrossRef CAS PubMed.
- S. Kumar, J. M. Rosenberg, D. Bouzida, R. H. Swendsen and P. A. Kollman, J. Comput. Chem., 1992, 13, 1011–1021 CrossRef CAS.
- Y. Li, H. Yuan, A. von dem Bussche, M. Creighton, R. H. Hurt, A. B. Kane and H. Gao, Proc. Natl. Acad. Sci. U. S. A., 2013, 110, 12295–12300 CrossRef CAS.
- C. Shen, G. Zou, W. Guo and H. Gao, Carbon, 2020, 164, 391–397 CrossRef CAS.
- S. J. Marrink and H. J. C. Berendsen, J. Phys. Chem., 1996, 100, 16729–16738 CrossRef CAS.
- Y. Liu, Y. Yang, Y. Qu, Y. Q. Li, M. Zhao and W. Li, Phys. Chem. Chem. Phys., 2020, 22, 23268–23275 RSC.
- D. P. Tieleman, S. J. Marrink and H. J. C. Berendsen, Biochim. Biophys. Acta, Rev. Biomembr., 1997, 1331, 235–270 CrossRef CAS.
- W. J. Allen, J. A. Lemkul and D. R. Bevan, J. Comput. Chem., 2009, 30, 1952–1958 CrossRef CAS.
|
This journal is © The Royal Society of Chemistry 2022 |
Click here to see how this site uses Cookies. View our privacy policy here.