DOI:
10.1039/D1RA05799E
(Paper)
RSC Adv., 2022,
12, 2181-2195
Exploring the potential therapeutic effect of Eucommia ulmoides–Dipsaci Radix herbal pair on osteoporosis based on network pharmacology and molecular docking technology
Received
30th July 2021
, Accepted 14th December 2021
First published on 14th January 2022
Abstract
Eucommia ulmoides–Dipsaci Radix (EU–DR) is a commonly used herbal pair for the treatment of osteoporosis (OP) in China. The purpose of this study was to investigate the potential mechanism of EU–DR on OP through network pharmacology and molecular docking approaches. Combining data from multiple open-source databases and literature mining, the active compounds and potential targets of EU–DR were screened out. The OP related targets were identified from the interactive web tool GEO2R. The shared targets were obtained by intersecting the targets of EU–DR and OP. The protein–protein interaction (PPI) network was constructed via the STRING database and Cytoscape 3.7.2 software. GO and KEGG enrichment analysis were conducted using R 3.6.3 software with adjusted p-value < 0.05. Sybyl-x 2.1.1 and Autodock Vina 1.1.2 software were used to cross validate the affinity between active compounds and target proteins. Our results showed that a total of 50 active compounds were screened, corresponding to 895 EU–DR targets, 2202 OP targets and 144 shared targets. The flavonoids in EU–DR played an important role in anti-OP. The enrichment analysis of GO and KEGG suggested EU–DR exerted a therapeutic effect on OP mainly by regulating the osteoclast differentiation related signaling pathway. Meanwhile, molecular docking results showed that most active compounds in EU–DR had strong binding efficiency to the target proteins. In conclusion, this study elaborated the multi-component, multi-target, and multi-pathway interaction mechanism of the EU–DR herbal pair in the treatment of OP for the first time, which also provided a pharmacological basis for treating OP.
1. Introduction
Osteoporosis (OP) is a chronic metabolic bone disease characterized by bone loss, especially in elderly people and menopausal women.1 It has become one of the most common bone diseases in the world and has caused a heavy economic burden on society. The causes of OP are very complex, including the interaction of endocrine, nutritional status, genetic, physical and immune factors.2 The disorder of dynamic balance between bone formation of osteoblasts and bone resorption of osteoclasts is the main cause of its pathological changes. An increase in the number of osteoclasts may lead to excessive bone resorption, while impaired differentiation of osteoblasts may reduce the formation of new bone, disrupt the balance of bone reconstruction, and ultimately lead to bone loss.3 Currently, the treatment strategies for OP mainly use drug therapy, including calcitonin, bisphosphonates and fluorides.4,5 These drugs can alleviate bone loss and improve the clinical symptoms to a certain extent, but their long-term clinical application is limited by low tolerance, severe side effects and high cost.6 Meanwhile, these drugs cannot fundamentally improve bone metabolism and restore the dynamic balance of osteogenesis and osteoclastic activity.7 Thus, it is very important to develop more effective and safer therapeutic strategies for OP.
Traditional Chinese Medicine (TCM) has been used to prevent and treat various diseases in a multi-target and multi-component manner for thousands of years.8 In the theoretical system of TCM, OP is defined as bone flaccidity or arthralgia syndrome caused by kidney deficiency.9 In the treatment of OP, TCM formulas can not only reduce bone loss, but also comprehensively regulate body functions and relieve back pain. Herbal pairs are common clinical TCM formulas, which generally consist of two kinds of herbs. Compared with TCM formulas containing more herbs, it is simpler and more beneficial to elucidate the complex pharmacological mechanism of a herbal pair.10 Eucommia ulmoides (EU, Chinese name: Duzhong) is the bark of Eucommia ulmoides Oliv., a typical Chinese herb for tonifying the kidney, while Dipsaci Radix (DR, Chinese name: Xuduan) is the dry root of Dipsacus asper Wall., another natural herb for strengthening the bone.11,12 Previous studies have shown that EU and DR are currently the most widely used TCM for the treatment of OP.13 EU contains various chemical components, including lignans, steroids, terpenoids and flavonoids. EU extracts can inhibit bone loss and maintain metabolic balance.14 A variety of chemical components have also been identified in DR, especially saponins, iridoid glycosides and sterols. The crude extracts of DR can increase bone density and change bone histomorphology.15 Moreover, both of EU and DR are generally recognized as a classic herbal pair and play a synergistic effect in anti-OP.13 However, the potential pharmacological mechanism of the EU–DR herbal pair and its interaction with OP related targets and pathways are still unclear, and further exploration is needed.
Modern pharmacological studies have demonstrated that TCM has the characteristics of complex mechanism and multi-pathway interaction in the treatment of diseases.16 Based on the interaction network of multi-compound, multi-target and multi-pathway, network pharmacology can elucidate the mechanism of TCM through constructing a “drug–target–disease” network.17 This method conforms to the fundamental principles of TCM and has the characteristics of holistic and systematic. Molecular docking is a virtual screening technology that explores the interaction between receptors and drugs by simulating the behavior of small molecule ligands at the binding sites of receptor proteins.18 In recent years, the application of molecular docking to explain the relevant mechanism has become a trend in new drug research and development. Therefore, in this study, network pharmacology and molecular docking were carried out to clarify the potential mechanism of the EU–DR herbal pair for the treatment of OP. The flow diagram of the research is shown in Fig. 1.
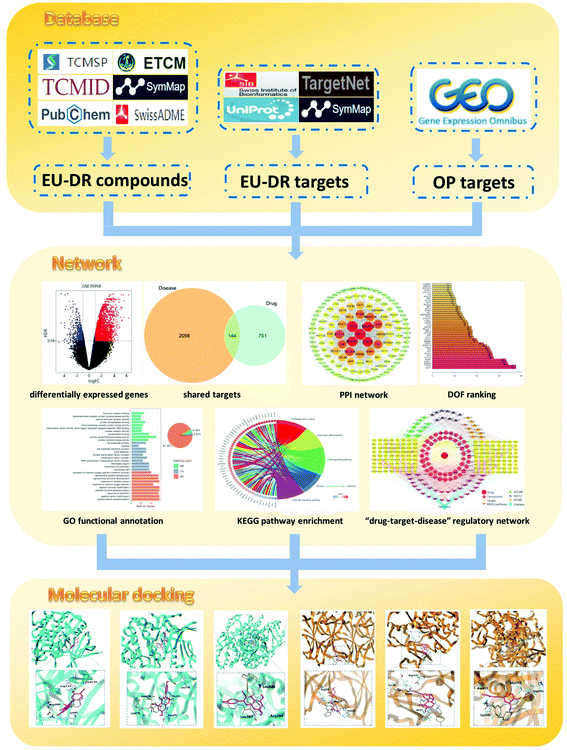 |
| Fig. 1 Schematic diagram for identifying the mechanism of Eucommia ulmoides–Dipsaci Radix herbal pair on OP using network pharmacology. | |
2. Materials and methods
2.1 Identification of active compounds in EU–DR
In order to obtain comprehensive information, the compounds of EU and DR were derived from four databases, including Traditional Chinese Medicine Systems Pharmacology (TCMSP) database (http://tcmspw.com/tcmsp.php), Traditional Chinese Medicine Information Database (TCM-ID) (http://www.megabionet.org/tcmid/), Encyclopedia of Traditional Chinese Medicine (ETCM) database (http://www.tcmip.cn/ETCM/index.php/Home/Index/index.html) and SymMap database (https://www.symmap.org/). The active compounds in EU–DR were screened based on the ADME related model of candidate compounds retrieved from TCMSP and Swiss ADME databases (http://www.swissadme.ch/).19 The screening criteria in the TCMSP database were compounds fulfilling both oral bioavailability (OB) value ≥ 30% and drug-likeness (DL) value ≥ 0.18, and the screening criteria in the Swiss ADME database were as follows: gatrointestinal absorption: high, Lipinski: Yes, Veber: Yes, Ghose: Yes, Muegge: Yes and Egan: Yes.9 In addition, it was also found that some compounds with significant biological activity were removed because their values were lower than the screening criteria. Considering their potential druggability, we used large-scale text mining to supplement compounds with notable pharmacological activity. Finally, we obtained the PubChem ID, 2D structure and canonical SMILES files of the active compounds from PubChem database (https://pubchem.ncbi.nlm.nih.gov/).
2.2 The potential targets of active compounds in EU–DR
To identify the potential targets related to the active compounds, we collected information from three databases, including SymMap database, Swiss Target Prediction database (http://www.swisstargetprediction.ch/) and TargetNet database (http://targetnet.scbdd.com/home/index/). All chemical structures were prepared and converted into canonical SMILES using PubChem. The SMILES files of the compound were put into Swiss Target Prediction database and TargetNet database, then the result with probability greater than 0.9 was output.20 The targets related to the active compounds were collected through the molecular names in SymMap. Data were further analysed using UniProt database (http://www.uniprot.org/) to confirm that these target proteins had been verified and set as “Homo sapiens”.
2.3 OP related targets and shared targets of EU–DR against OP
The interactive web tool GEO2R was used to retrieve the OP related targets. GEO2R is an analytical tool provided by Gene Expression Omnibus (GEO) Dataset (https://www.ncbi.nlm.nih.gov/gds), which aims to compare different sample groups in the GEO Series to identify differentially expressed genes (DEGs) between normal samples and model samples. GSE35958 is a classical gene chip of OP model and is frequently cited.21,22 In order to identify the genes that were significantly differentially expressed in each sample, the Benjamini–Hochberg false discovery rate (FDR) method was employed to adjust P-values in GEO2R analysis. The cutoff criteria for screening DEGs was FDR < 0.05 & |log
FC| > 1.23 Moreover, according to the results of DEGs analysis in GEO2R, a volcano diagram was drawn using the ggplot2 program package in R 3.6.3 software. Subsequently, a venn analysis was performed through the VennDiagram package in R 3.6.3 software to overlap the OP related targets and the potential targets of EU–DR.
2.4 Protein–protein interaction (PPI) and hub target analysis
PPI analysis is helpful to identify the hub target related to EU–DR on OP. The shared targets of EU–DR relevant to OP were retrieved to construct the PPI network via STRING database (https://string-db.org/) with “Homo sapiens” setting. Cytoscape 3.7.2 software was used to visualize the interconnection network, and “Analysis Network” function was performed to analyze the network properties. In the PPI network, the degree of freedom (DOF) is an important index, since the points above the average DOF usually play an important role in the network.24
2.5 GO and KEGG pathway enrichment analysis
In order to explore the core functions and biological pathways related to EU–DR in treatment of OP, we used the ClusterProfiler package in R 3.6.3 software to perform Gene ontology (GO) functional annotation and Kyoto Encyclopedia of Genes and Genomes (KEGG) pathway analysis. GO functions include the biological process (BP), cellular component (CC), and molecular function (MF) terms, which are used to analyze the potential mechanism. KEGG was applied to identify the biological pathways. The enrichment results with FDR less than 0.05 were selected for further analysis.
2.6 Network construction
To better characterize the “multi-compound, multi-target and multi-pathway” therapeutic features of EU–DR, Cytoscape 3.7.2 software was used to construct a primary regulatory network to intuitively display the “drug–target–disease” relationship between EU–DR and OP. In this bilateral network, the nodes represent active compounds, shared targets and pathway, while the edges represent the interactions between compounds, targets and pathway. The DOF of a node represents the number of targets associated with the node in the network.
2.7 Molecular docking simulation
The Surflex-Dock module in Sybyl-X (version 2.1.1, Tripos Inc.) software was used to evaluate the binding ability of active compounds with target proteins. The crystal structures of candidate target proteins were downloaded directly from RCSB Protein Data Bank (PDB, http://www.rcsb.org/) and modified by Sybyl-X software, including water removal, hydrogen addition, side chain repair, amino acid optimization and active pocket construction. The 3D chemical structures of the active compounds were obtained from the PubChem database. Subsequently, the Surflex-Dock docking mode was used for molecular docking and the total score of binding affinity was obtained. In general, total score >4.0 indicates certain binding activity, total score >5.0 indicates good binding activity, while total score >7.0 indicates strong binding activity (the higher the score, the stronger binding activity).25 Besides, we also use Autodock Vina 1.1.2 software, another independent protein–ligand docking program, to cross verify the docking results by calculating the area under the ROC curve (AUC) of Autodock Vina based on the docking results obtained by Sybyl-X, so as to judge the reliability of the docking results.
3. Results
3.1 Collection of active compounds in EU–DR
According to the screening criteria of ADME, a total of 34 EU–DR active compounds were retrieved from the above databases. During the ADME screening process, we noticed that some compounds, such as Aucubin, Geniposide, Geniposidic acid and Asperosaponin VI had high pharmacological activities, but they did not meet all the criteria.26–28 Therefore, we have also retained them for subsequent analysis. After deleting repetitions, a total of 50 active compounds were screened, including 34 active compounds in EU, 20 active compounds in DR, and 4 shared compounds in EU and DR. The details are shown in the Table 1.
Table 1 The information of the active compounds in EU–DR
PubChem CID |
Name |
Molecular formula |
2D structure |
Drug |
443354 |
Geniposidic acid |
C16H22O10 |
 |
EU |
73399 |
Pinoresinol |
C20H22O6 |
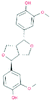 |
EU |
443023 |
(+)-Syringaresinol |
C22H26O8 |
 |
EU |
5280863 |
Kaempferol |
C15H10O6 |
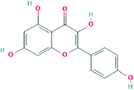 |
EU |
5317205 |
Erythraline |
C18H19NO3 |
 |
EU |
107848 |
Geniposide |
C17H24O10 |
 |
EU |
5280961 |
Genistein |
C15H10O5 |
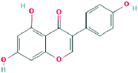 |
EU |
94253 |
Vulgarin |
C15H20O4 |
 |
EU |
5282102 |
Astragalin |
C21H20O11 |
 |
EU |
5320287 |
Ombuin |
C17H14O7 |
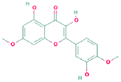 |
EU |
182232 |
(+)-Epicatechin |
C15H14O6 |
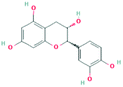 |
EU |
443028 |
Yangambin |
C24H30O8 |
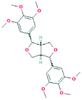 |
EU |
12309360 |
Tabernaemontanine |
C21H26N2O3 |
 |
EU |
91458 |
Aucubin |
C15H22O9 |
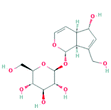 |
EU |
442972 |
Cyclopamine |
C27H41NO2 |
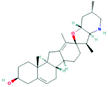 |
EU |
165225 |
Dehydrodieugenol |
C20H22O4 |
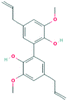 |
EU |
94175 |
Epiquinidine |
C20H24N2O2 |
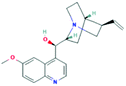 |
EU |
23205 |
Helenalin |
C15H18O4 |
 |
EU |
6710676 |
Kobusone |
C14H22O2 |
 |
EU |
73117 |
(+)-Eudesmin |
C22H26O6 |
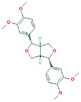 |
EU |
5280457 |
Pinosylvin |
C14H12O2 |
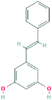 |
EU |
5372367 |
Dehydrodiconiferyl alcohol |
C20H22O6 |
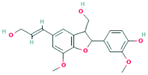 |
EU |
5280343 |
Quercetin |
C15H10O7 |
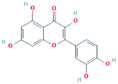 |
EU |
5274623 |
(2R,3S)-Dihydrodehydroconiferyl alcohol |
C20H24O6 |
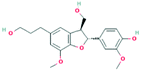 |
EU |
5281953 |
Syringetin |
C17H14O8 |
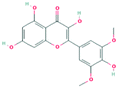 |
EU |
2353 |
Berberine |
C20H18NO4 |
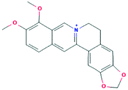 |
EU |
125309 |
Cyrtominetin |
C17H16O6 |
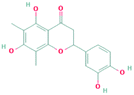 |
EU |
717531 |
3,4-Dimethoxycinnamic acid |
C11H12O4 |
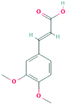 |
EU |
637584 |
Epipinoresinol |
C20H22O6 |
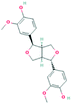 |
EU |
12309361 |
(−)-Dregamine |
C21H26N2O3 |
 |
EU |
14284432 |
Asperosaponin VI |
C47H76O18 |
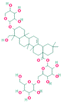 |
DR |
12303645 |
3-Epi-beta-Sitosterol |
C29H50O |
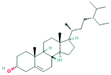 |
DR |
6474310 |
Isochlorogenic acid A |
C25H24O12 |
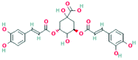 |
DR |
442515 |
Cantleyine |
C11H13NO3 |
 |
DR |
441928 |
Cauloside A |
C35H56O8 |
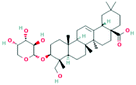 |
DR |
442915 |
Japonine |
C18H17NO3 |
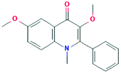 |
DR |
101967018 |
Sylvestroside III |
C27H36O14 |
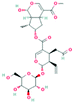 |
DR |
5281636 |
Gentisin |
C14H10O5 |
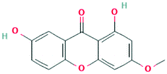 |
DR |
5315179 |
Venoterpine |
C9H11NO |
 |
DR |
5742590 |
Sitogluside |
C35H60O6 |
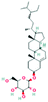 |
DR |
89640 |
Loganic acid |
C16H24O10 |
 |
DR |
87691 |
Loganin |
C17H26O10 |
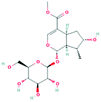 |
DR |
161036 |
Sweroside |
C16H22O9 |
 |
DR |
5281780 |
3,4-Dicaffeoylquinic acid |
C25H24O12 |
 |
DR |
6474309 |
4,5-Di-O-caffeoylquinic acid |
C25H24O12 |
 |
DR |
122228278 |
Triplostoside A |
C35H52O20 |
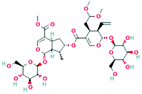 |
DR |
1794427 |
Chlorogenic acid |
C16H18O9 |
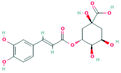 |
EU,DR |
689043 |
Caffeic acid |
C9H8O4 |
 |
EU,DR |
222284 |
Beta-Sitosterol |
C29H50O |
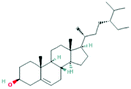 |
EU,DR |
64945 |
Ursolic acid |
C30H48O3 |
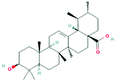 |
EU,DR |
3.2 Screening of potential targets in EU–DR
In this study, 833 targets corresponding to EU and 467 targets corresponding to DR were identified. However, most of the targets were repetitive, revealing that the active compounds of EU and DR may have the same biological effects and achieve synergistic effects when combined. Finally, through further integration of all targets, 895 targets corresponding to EU–DR were obtained.
3.3 Related targets of OP and shared targets of EU–DR against OP
The gene chip GSE35958 contains 4 normal bone marrow samples and 5 OP bone marrow samples to analyze the effect of OP on the transcriptome of human mesenchymal stem cells (hMSCs) from human bone marrow. Following the above screening criteria of FDR < 0.05 & |log
FC| > 1, a total of 2202 DEGs between normal samples and model samples were identified as shown in the volcano diagram (Fig. 2A). As shown in the Venn diagram (Fig. 2B), there were 144 shared targets between 2202 DEGs and 895 potential targets to further clarify the mechanism of EU–DR in OP treatment.
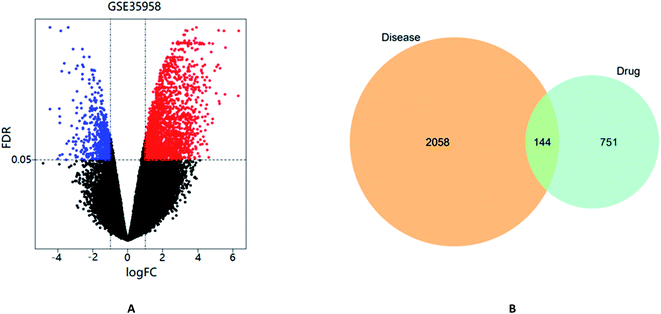 |
| Fig. 2 (A) Differentially expressed genes in OP. According to the standard of FDR < 0.05 & |log FC| > 1, red represent up-regulated genes and blue represented down-regulated genes. (B) The intersection of OP differentially expressed genes and potential targets of EU–DR. | |
3.4 PPI network construction
A total of 144 shared target genes were imported into STRING database for the construction of PPI network. In this study, the 0.4 confidence (medium) was set as the default threshold. In order to obtain a better understanding, the results were further analyzed by Cytoscape 3.7.2 software, and a PPI network with 144 nodes and 1103 edges was obtained (Fig. 3A). The hub target possessed a higher DOF and was more likely to play a critical role in the network of EU–DR on OP, such as RAC-alpha serine/threonine–protein kinase (AKT1), cellular tumor antigen p53 (TP53), mitogen-activated protein kinase 3 (MAPK3), mitogen-activated protein kinase 1 (MAPK1), epidermal growth factor receptor (EGFR) and signal transducer and activator of transcription 3 (STAT3).The average DOF of PPI network was 17, and the top 42 hub targets above the average DOF were shown in Fig. 3B.
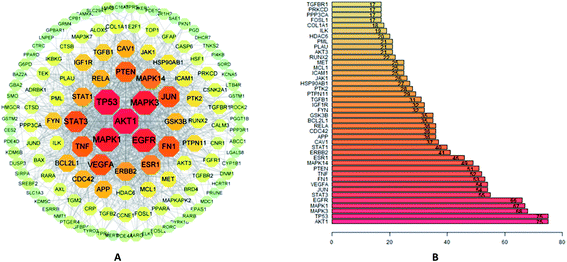 |
| Fig. 3 PPI network of EU–DR on OP. (A) The network contains 144 nodes and 1103 edges. Nodes represent proteins and edges represent protein–protein associations. The darker color nodes represent greater DOF. (B) The top 42 hub targets above the average DOF were shown, with an average DOF of 17. | |
3.5 GO functional annotation and KEGG pathway enrichment analysis
In GO functional annotation analysis, the 144 shared targets were highly enriched in 2115 BP terms, 49 CC terms, and 125 MF terms, with FDR < 0.05. The top 10 enrichment results of terms and the proportions of each part were shown in Fig. 4. Among them, BP terms account for the largest proportion, accounting for 91.39%, followed by MF terms and CC terms, accounting for 6.18% and 2.42% respectively. Peptidyl-serine phosphorylation, peptidyl-serine modification, response to antibiotic, peptidyl-tyrosine phosphorylation and peptidyl-tyrosine modification were closely related to BP. In regard to CC, higher enrichment was found in membrane raft, membrane microdomain, membrane region, RNA polymerase II transcription factor complex and transcription factor complex. The main terms related to OP in MF included protein tyrosine kinase activity, protein serine/threonine kinase activity, phosphatase binding, nuclear receptor activity and transcription factor activity, direct ligand regulated sequence-specific DNA binding. The analysis results showed that these targets were closely related to the processes of regulating steroid hormone activity and promoting steroid hormone receptor activity and binding.
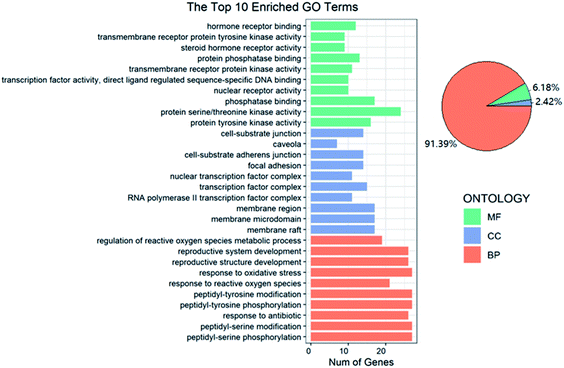 |
| Fig. 4 GO functional annotation of EU–DR on OP. Bar plot shows the top 10 GO enrichment terms of BP, CC and MF, respectively. The pie plot shows the proportion of each part of BP, CC and MF in the whole. | |
The focus of KEGG enrichment analysis of 144 shared targets was the anti-OP pathway of EU–DR, with FDR < 0.05. The results showed that 145 pathways were enriched, and the top 5 pathways with the largest number of enriched targets were shown in Fig. 5. Among them, MAPK signaling pathway (hsa04010), proteoglycans in cancer (hsa05205), PI3K-Akt signaling pathway (hsa04151), human T-cell leukemia virus 1 infection (hsa05166) and osteoclast differentiation (hsa04380) were highly associated with the occurrence and development of OP. In addition, other KEGG pathways were also reported to be relevant to OP, such as estrogen signaling pathway (hsa04915), VEGF signaling pathway (hsa04370) and Wnt signaling pathway (hsa04310). Moreover, gene targets that act on multiple pathways at the same time were found in KEGG enrichment analysis, indicating the importance of specific targets in the entire OP bioinformatics network. For instance, AKT1, MAPK1 and MAPK3 appeared in the 5 pathways with the largest number of enriched targets. These also reflected the “multi-target and multi-pathway” regulation mechanism of EU–DR.
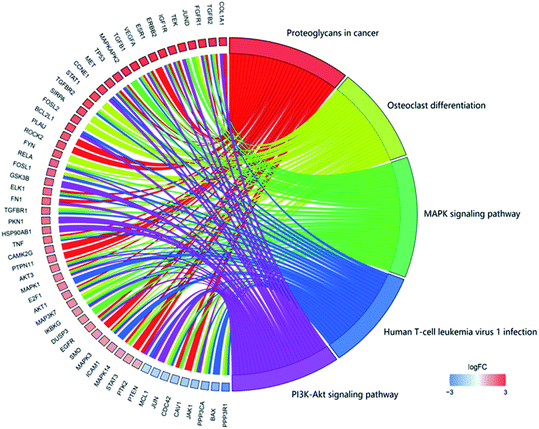 |
| Fig. 5 KEGG enrichment analysis of EU–DR on OP. The chord plot shows the top 5 KEGG pathway terms and corresponding targets. Different colors on the right side of the graph represent different signal pathways, and the left side is the targets with relevance. The more lines in the pathway, the more targets are enriched. | |
3.6 Network construction analysis
As shown in Fig. 6, a regulatory network of “drug–target–disease” was constructed, showing the interactions among 50 compounds, 144 targets, 30 KEGG pathways and 30 GO terms. This network was consisted of 256 nodes and 1848 interaction edges, which indicated that the active compounds of EU–DR act on the whole biological network system through multi-target and multi-pathway. According to the DOF, we found that flavonoids played a greater role in the regulatory network. Among these compounds, Quercetin (degree = 48), Genistein (degree = 42) and Kaempferol (degree = 29) were the most potential compounds. At the same time, the hub targets predicted by PPI also possessed higher DOF in this network, such as MAPK3 (degree = 49), MAPK1 (degree = 49), EGFR (degree = 48), AKT1 (degree = 47), RELA (degree = 39), indicating that they were involved in more signaling pathways.
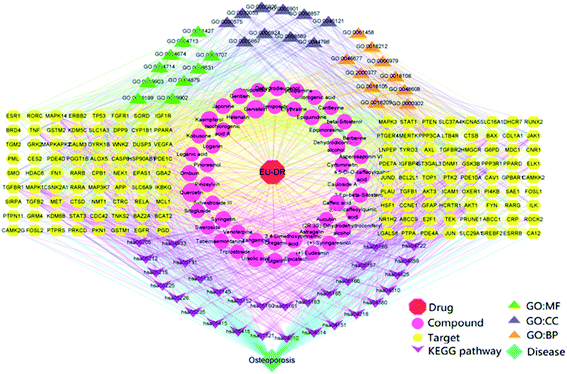 |
| Fig. 6 “Drug–target–disease” regulatory network of EU–DR on OP. The network is constructed by 50 compounds, 144 targets, 30 KEGG pathways and 30 GO terms. | |
3.7 Molecular docking verification
In molecular docking, the ligand binds to one or more amino acid residues with hydrogen bonds in the active pocket, and participates in the process of conformational changes and energy complementation.29 The binding sites and binding score values can intuitively reflect the interaction and stability of the docking model. Here, we select the first 15 targets with higher DOF (except for receptors lacking the original ligand in the PDB) to construct protein receptors based on hub targets of PPI. According to degree of the “drug–target–disease” regulatory network, the first 6 compounds with higher degree values were selected from EU–DR herbal pair for molecular docking verification, including quercetin, genistein, kaempferol, ursolic acid, dehydrodiconiferyl alcohol and caffeic acid. The docking results showed that most of docking scores were greater than 4, indicating that the binding between ligand and receptor was relatively stable (Fig. 7A). Besides, the cross validation between Sybyl-X and Autodock Vina shown AUC = 90.2709, indicating the docking results is reliable (Fig. 7B).
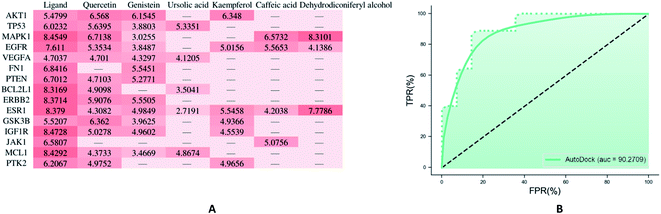 |
| Fig. 7 (A) The docking scores between small molecule ligands and receptor protein. Total scores are expressed in −log 10(Kd) units to represent binding affinities. “—” indicate that the target is not a potential receptor protein related to this small molecule ligand. (B) Cross validation between Sybyl-X and Autodock Vina. AUC represents the area under the ROC curve. | |
The binding modes of 6 targets with their corresponding original ligands and the above 6 active compounds of EU–DR were shown in Fig. 8. It was observed that there was a high resemblance between active compounds and original ligands of protein, and they nearly occupied the same active pocket. The binding mode of quercetin at the active site of GSK3B had been represented in its three-dimensional mode in Fig. 8A. Four amino acid residues formed hydrogen bond interactions with quercetin as follows: the phenolic hydroxyl group on the benzene ring of quercetin linked with Val135, Asp133, Asp200 and Lys85 residue respectively. The binding mode of genintein at the active site of AKT1 was shown in Fig. 8B. The molecule was located in the binding pocket, surrounded by Asn279, Leu156, Thr291 and Asp292 residue. The binding mode between kaempferol and ESR1 was shown in Fig. 8C. The residues of Leu346, Leu387 and Arg394 form hydrogen bond interactions with the hydroxyl groups of kaempferol. Fig. 8D showed the binding mode between ursolic acid and TP53, including a carboxyl group linked with Arg202 residue and the hydroxyl group linked with Pro223 and Val225 residue. Dehydrodiconiferyl alcohol had a strong binding ability with the receptor protein MAPK1 by forming four hydrogen bond interactions. One was the phenolic hydroxyl group connected to Ile31 residue from the benzene ring, and the other three were the hydroxyl group connected to Asp167, Tyr36 and Ala35 residues (Fig. 8E). Caffeic acid and the receptor protein EGFR showed good binding ability. Meanwhile, amino acid residues with caffeic acid form hydrogen bond interactions to maintain conformational stability, including the carboxyl group connected to Lys745, Asp855 residue and phenolic hydroxyl group linked with Gln791 and Met793 residue (Fig. 8F).
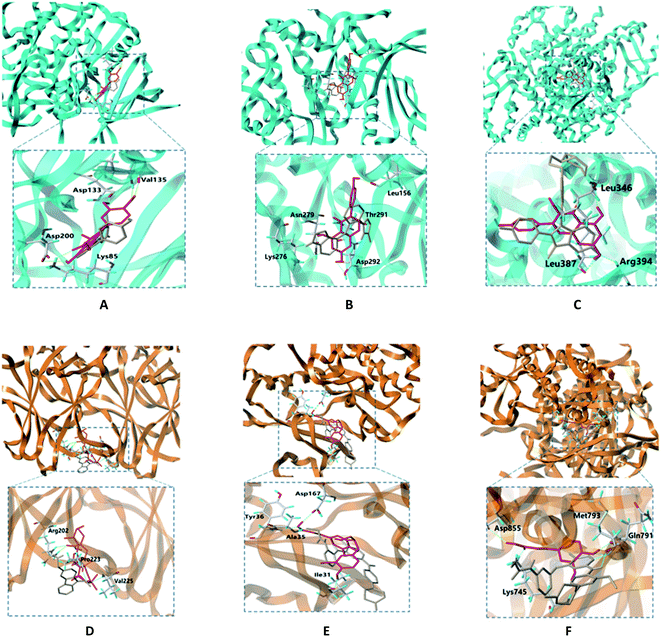 |
| Fig. 8 Molecular docking of active compounds of EU–DR and receptor protein. Gray represents original ligand of crystal structure. Violet represents compounds in EU–DR. (A) GSK3B protein with quercetin; (B) AKT1 protein with genistein; (C) ESR1 protein with kaempferol; (D) TP53 protein with ursolic acid; (E) MAPK1 protein with dehydrodiconiferyl alcohol; (F) EGFR protein with caffeic acid. | |
Taken together, these docking results provided evidences that the active compounds of EU–DR could stably bind to the binding sites of receptor proteins, indicating that EU–DR had a strong effect on the treatment of OP.
4. Discussion
Previous studies have shown that EU and DR are currently the most widely used TCM in treating OP.13 However, due to the complex chemical compositions of EU–DR herbal pair, it is still difficult to elucidate its exact pharmacological mechanism. In this study, we systematically revealed mechanism of EU–DR in the treatment of OP for the first time by using network pharmacology method.
Based on this method, we found that most of the compounds of EU–DR affected multiple targets, and many repetitive targets were identified from different compounds, revealing that EU–DR exerted a therapeutic effect on OP through the synergistic effects of various active components. The results indicated that flavonoids might play a greater role in anti-OP as they affected more targets relevant to OP. Quercetin was the most important active compound, which was involved in the regulation of 48 target genes. Following quercetin, genistein and kaempferol regulated 42 and 29 target genes, respectively. Flavonoids have a variety of beneficial biological activities due to their antioxidant, anti-inflammatory and estrogenic effects, such as quercetin and kaempferol are substances that can both reduce the activity of osteoclastic and increase the activity of osteoblastic.30 Genistein is a natural isoflavonoid that can stimulate the growth of osteoblasts.31 Moreover, we found that AKT1, MAPK1 and MAPK3 were the core target proteins of EU–DR for the treatment of OP. They not only exhibited abundant interactions with other target proteins, but also participated in more signaling pathways. Maintenance of bone homeostasis is a dynamic process regulated by osteoblast-mediated bone formation and osteoclast-mediated bone resorption. AKT1 is considered to be a unique influence factor that mediates osteoblasts and regulates the differentiation of osteoblasts and osteoclasts.9,32 During osteoclast maturation, MAPK1 and MAPK3 regulate osteoclast maturation by up-regulating the expression of osteoclast-related genes.33 The main compounds in EU–DR were identified to bind to these core target proteins, and further confirmed by molecular docking.
Additionally, according to the results of the KEGG pathway analysis, we found that EU–DR mainly involved in osteoclast differentiation related signaling pathways in treating OP (Fig. 9). Osteoclast differentiation is stimulated by macrophage colony-stimulating factor (M-CSF) and the receptor activator of nuclear factor-κB ligand (RANKL).34 M-CSF bind to its receptor c-Fms to induce the proliferation and survival of osteoclast precursor cells through activation of the ERK and PI3K/Akt pathways. The binding of RANKL to RANK recruits TNF receptor-associated factor 6 (TRAF6) to activate MAPK, PI3K/Akt, and NF-κB pathway, and promote the differentiation of osteoclast precursors into osteoclasts. TRAF6 is recruited to RANK to form a signaling complex containing TGF-β-activated kinase (TAK) 1 and TAK-1-binding protein (TAB) 2 to activate all three MAPK pathways, including extracellular signal-regulated kinase (ERK), c-Jun N-terminal kinase (JNK), and p38, and induce the activation of their downstream targets such as c-Fos, AP-1 transcription factors.35 Kaempferol inhibits RANKL-mediated osteoclast differentiation through inhibiting RANKL-mediated phosphorylation of ERK, JNK and p38, and expressions of c-Fos and nuclear factor of activated T cells 1 (NFATc1).36,37 Several studies illustrated that quercetin suppressed the phosphorylation of Akt and affected nuclear factor kappa B (NF-κB), AP-1 and NFATc1 to inhibit osteoclast differentiation.38,39 Activation of NF-κB signaling pathway by RANKL, TNF-α or IL-1 can induce osteoclast differentiation to extend osteoclast survival and increase bone resorption.40 Genistein directly suppressed osteoclastic differentiation induced by TNF-α and suppressed the expression of two transcription factors c-Fos and NFATc1 caused by NF-κB up regulation to inhibit bone resorption.41 In addition, robust NFATc1 induction can be provided through activation of calcium signaling.42 Genistein induce apoptosis of osteoclasts through calcium signaling.43 According to literature and network pharmacological analysis, we believed that flavonoids, the active ingredient of EU–DR herbal pair, could affect the differentiation and survival of osteoclasts, reduce bone resorption, and achieve the purpose of treating OP.
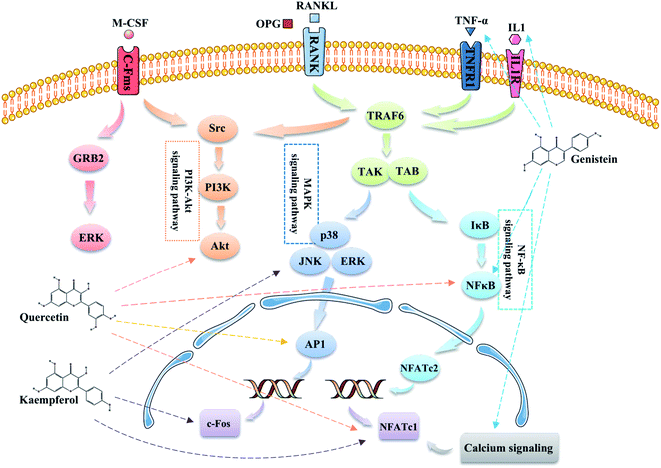 |
| Fig. 9 Illustration of the osteoclast differentiation related signaling pathways regulated by the main active components of EU–DR. | |
Drug therapy is the usual method in treating OP. Regardless of the benefits, most patients treated with these drugs have severe side effects.44 Therefore, a mild treatment strategy is very important for the treatment of OP. In addition to treatment, the prevention of OP is also very important. Regarding preventive care, drugs with less side effects are needed. In this respect, TCM such as EU and DR have great advantages. With more research on EU–DR herbal pair, its medicinal substances and pharmacological mechanisms will become more and more clear.
5. Conclusions
In conclusion, this study elaborated the multi-component, multi-target, and multi-pathway interaction mechanism of EU–DR herbal pair in the treatment of OP through network pharmacology and molecular docking method for the first time. The results indicated that EU–DR herbal pair can regulate the osteoclast differentiation related signaling pathways to affect the proliferation and survival of osteoclasts. The flavonoids in EU–DR played a greater role in anti-OP. The research provided a theoretical basis for the effective ingredients and mechanism of EU–DR herbal pair for treating OP.
Author contributions
Conceptualization, S. F. and T. W.; investigation, L. F.; methodology, M. W. and X. Z.; supervision, X. A. and X. D.; visualization, S. F. and Y. L.; writing—original draft, S. F.; writing—review and editing, Y. C., J. H. and Y. L. All authors have read and agreed to the published version of the manuscript.
Conflicts of interest
There are no conflicts of interest.
Acknowledgements
These works were supported by the National Natural Science Foundation of China (No. 31200253), Natural Science Basic Research Plan in Shaanxi Province of China (No. 2020JZ-45), Natural Science Foundation of Shaanxi Province (No. 2021JQ-935), and Scientific Research Program of Shaanxi Provincial Education Department (No. 18JS109).
References
- T. Sozen, L. Ozisik and N. C. Basaran, Eur. J. Rheumatol., 2017, 4, 46–56 CrossRef PubMed.
- H. Zhao, N. Zhao, P. Zheng, X. Xu, M. Liu, D. Luo, H. Xu and D. Ju, J. Immunol. Res., 2018, 2018, 6345857 Search PubMed.
- G. Borciani, G. Montalbano, N. Baldini, G. Cerqueni, C. Vitale-Brovarone and G. Ciapetti, Acta Biomater., 2020, 108, 22–45 CrossRef CAS PubMed.
- W. P. Olszynski, D. K. Shawn, J. D. Adachi, J. P. Brown, S. R. Cummings, D. A. Hanley, S. P. Harris, A. B. Hodsman, D. Kendler, M. R. McClung, P. D. Miller and C. K. Yuen, Clin. Ther., 2004, 26, 15–28 CrossRef PubMed.
- S. H. Tella and J. C. Gallagher, J. Steroid Biochem. Mol. Biol., 2014, 142, 155–170 CrossRef CAS PubMed.
- S. Aihara, S. Yamada, H. Oka, T. Kamimura, T. Nakano, K. Tsuruya and A. Harada, Ren. Fail., 2019, 41, 88–97 CrossRef CAS PubMed.
- P. Geusens, M. Oates, A. Miyauchi, J. D. Adachi, M. Lazaretti-Castro, P. R. Ebeling, N. C. Perez, C. E. Milmont, A. Grauer and C. Libanati, JBMR Plus, 2019, 3, e10211 CrossRef PubMed.
- J. Chao, Y. Dai, R. Verpoorte, W. Lam, Y. C. Cheng, L. H. Pao, W. Zhang and S. Chen, Biochem. Pharmacol., 2017, 139, 94–104 CrossRef CAS PubMed.
- Z. W. Liu, Z. H. Luo, Q. Q. Meng, P. C. Zhong, Y. J. Hu and X. L. Shen, Comput. Biol. Med., 2020, 127, 104074 CrossRef CAS.
- Y. Liu, Q. Xue, A. Li, K. Li and X. Qin, J. Ethnopharmacol., 2020, 253, 112688 CrossRef CAS PubMed.
- X. He, J. Wang, M. Li, D. Hao, Y. Yang, C. Zhang, R. He and R. Tao, J. Ethnopharmacol., 2014, 151, 78–92 CrossRef PubMed.
- W. Zhang, K. Xue, Y. Gao, Y. Huai, W. Wang, Z. Miao, K. Dang, S. Jiang and A. Qian, Life Sci., 2019, 235, 116820 CrossRef CAS PubMed.
- N. D. Zhang, T. Han, B. K. Huang, K. Rahman, Y. P. Jiang, H. T. Xu, L. P. Qin, H. L. Xin, Q. Y. Zhang and Y. M. Li, J. Ethnopharmacol., 2016, 189, 61–80 CrossRef.
- R. Zhang, Y. L. Pan, S. J. Hu, X. H. Kong, W. Juan and Q. B. Mei, J. Ethnopharmacol., 2014, 155, 104–112 CrossRef CAS.
- R. W. Wong, A. B. Rabie and E. U. Hagg, Phytother. Res., 2007, 21, 596–598 CrossRef PubMed.
- G. H. Jian, B. Z. Su, W. J. Zhou and H. Xiong, BioData Min., 2020, 13, 12 CrossRef CAS PubMed.
- D. C. Hao and P. G. Xiao, Drug Dev. Res., 2014, 75, 299–312 CrossRef CAS PubMed.
- N. S. Pagadala, K. Syed and J. Tuszynski, Biophys. Rev., 2017, 9, 91–102 CrossRef CAS PubMed.
- A. Daina, O. Michielin and V. Zoete, Sci. Rep., 2017, 7, 42717 CrossRef PubMed.
- T. He, J. Liu, X. Wang, C. Duan, X. Li and J. Zhang, Food Chem. Toxicol., 2020, 146, 111845 CrossRef CAS PubMed.
- S. Moradifard, M. Hoseinbeyki, M. M. Emam, F. Parchiniparchin and M. Ebrahimi-Rad, Mutat. Res., 2020, 786, 108339 CAS.
- B. Zhou, K. Peng, G. Wang, W. Chen and Y. Kang, Biochem. Biophys. Res. Commun., 2021, 549, 221–228 CrossRef CAS.
- Y. Y. Li, R. Li, Z. W. Zeng, S. Y. Li, S. Y. Liao, W. H. Ma, C. H. Zhou and D. H. Xu, Phytomedicine Plus., 2021, 1, 100049 CrossRef.
- D. W. Huang, B. T. Sherman, Q. Tan, J. Kir, D. Liu, D. Bryant, Y. Guo, R. Stephens, M. W. Baseler, H. C. Lane and R. A. Lempicki, Nucleic Acids Res., 2007, 35, W169–W175 CrossRef PubMed.
- K. Y. Hsin, S. Ghosh and H. Kitano, PLoS One, 2013, 8, e83922 CrossRef PubMed.
- F. Hu, J. An, W. Li, Z. Zhang, W. Chen, C. Wang and Z. Wang, J. Ethnopharmacol., 2015, 169, 145–155 CrossRef CAS.
- X. Sun, Y. Zhang, Y. Yang, J. Liu, W. Zheng, B. Ma and B. Guo, J. Pharm. Biomed. Anal., 2018, 154, 40–47 CrossRef CAS PubMed.
- K. Liu, Y. Liu, Y. Xu, K. S. Nandakumar, H. Tan, C. He, W. Dang, J. Lin and C. Zhou, Phytomedicine, 2019, 63, 153006 CrossRef CAS PubMed.
- W. Yan, S. X. Li, M. Wei and H. Gao, Oncol. Rep., 2018, 40, 2515–2524 CAS.
- C. Prouillet, J. C. Maziere, C. Maziere, A. Wattel, M. Brazier and S. Kamel, Biochem. Pharmacol., 2004, 67, 1307–1313 CrossRef CAS PubMed.
- Z. L. Wang, J. Y. Sun, D. N. Wang, Y. H. Xie, S. W. Wang and W. M. Zhao, Phytomedicine, 2006, 13, 718–723 CrossRef CAS.
- Q. Tu, J. Zhang, L. Q. Dong, E. Saunders, E. Luo, J. Tang and J. Chen, J. Biol. Chem., 2011, 286, 12542–12553 CrossRef CAS.
- C. F. Cheng, L. J. Chien-Fu, F. J. Tsai, C. J. Chen, J. S. Chiou, C. H. Chou, T. M. Li, T. H. Lin, C. C. Liao, S. M. Huang, J. P. Li, J. C. Lin, C. C. Lin, B. Ban, W. M. Liang and Y. J. Lin, J. Ethnopharmacol., 2019, 244, 112074 CrossRef CAS PubMed.
- J. H. Kim and N. Kim, Chonnam Med. J., 2016, 52, 12–17 CrossRef CAS PubMed.
- J. Mizukami, G. Takaesu, H. Akatsuka, H. Sakurai, J. Ninomiya-Tsuji, K. Matsumoto and N. Sakurai, Mol. Cell. Biol., 2002, 22, 992–1000 CrossRef CAS PubMed.
- W. S. Lee, E. G. Lee, M. S. Sung and W. H. Yoo, Inflammation, 2014, 37, 1221–1230 CrossRef CAS PubMed.
- J. L. Pang, D. A. Ricupero, S. Huang, N. Fatma, D. P. Singh, J. R. Romero and N. Chattopadhyay, Biochem. Pharmacol., 2006, 71, 818–826 CrossRef CAS PubMed.
- A. Wattel, S. Kamel, C. Prouillet, J. P. Petit, F. Lorget, E. Offord and M. Brazier, J. Cell. Biochem., 2004, 92, 285–295 CrossRef CAS.
- M. Masuhara, T. Tsukahara, K. Tomita, M. Furukawa, S. Miyawaki and T. Sato, Biochem. Biophys. Rep., 2016, 8, 389–394 Search PubMed.
- L. G. Ming, K. M. Chen and C. J. Xian, J. Cell. Physiol., 2013, 228, 513–521 CrossRef CAS PubMed.
- S. Karieb and S. W. Fox, J. Cell. Biochem., 2011, 112, 476–487 CrossRef CAS PubMed.
- H. Takayanagi, S. Kim, T. Koga, H. Nishina, M. Isshiki, H. Yoshida, A. Saiura, M. Isobe, T. Yokochi, J. Inoue, E. F. Wagner, T. W. Mak, T. Kodama and T. Taniguchi, Dev. Cell, 2002, 3, 889–901 CrossRef CAS.
- Y. H. Gao and M. Yamaguchi, Biol. Pharm. Bull., 1999, 22, 805–809 CrossRef CAS PubMed.
- S. L. Ruggiero and S. J. Drew, J. Dent. Res., 2007, 86, 1013–1021 CrossRef CAS PubMed.
|
This journal is © The Royal Society of Chemistry 2022 |
Click here to see how this site uses Cookies. View our privacy policy here.